mirror of
https://github.com/llvm/llvm-project.git
synced 2025-04-24 12:16:07 +00:00
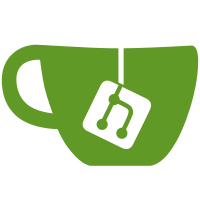
This is a companion to #118583, although it can be landed independently because since #117922 dialects do not have to use the same Python binding framework as the Python core code. This PR ports all of the in-tree dialect and pass extensions to nanobind, with the exception of those that remain for testing pybind11 support. This PR also: * removes CollectDiagnosticsToStringScope from NanobindAdaptors.h. This was overlooked in a previous PR and it is duplicated in Diagnostics.h. --------- Co-authored-by: Jacques Pienaar <jpienaar@google.com>
149 lines
6.4 KiB
C++
149 lines
6.4 KiB
C++
//===- DialectSparseTensor.cpp - 'sparse_tensor' dialect submodule --------===//
|
|
//
|
|
// Part of the LLVM Project, under the Apache License v2.0 with LLVM Exceptions.
|
|
// See https://llvm.org/LICENSE.txt for license information.
|
|
// SPDX-License-Identifier: Apache-2.0 WITH LLVM-exception
|
|
//
|
|
//===----------------------------------------------------------------------===//
|
|
|
|
#include <optional>
|
|
#include <vector>
|
|
|
|
#include "mlir-c/AffineMap.h"
|
|
#include "mlir-c/Dialect/SparseTensor.h"
|
|
#include "mlir-c/IR.h"
|
|
#include "mlir/Bindings/Python/NanobindAdaptors.h"
|
|
#include "mlir/Bindings/Python/Nanobind.h"
|
|
|
|
namespace nb = nanobind;
|
|
using namespace llvm;
|
|
using namespace mlir;
|
|
using namespace mlir::python::nanobind_adaptors;
|
|
|
|
static void populateDialectSparseTensorSubmodule(const nb::module_ &m) {
|
|
nb::enum_<MlirSparseTensorLevelFormat>(m, "LevelFormat", nb::is_arithmetic(),
|
|
nb::is_flag())
|
|
.value("dense", MLIR_SPARSE_TENSOR_LEVEL_DENSE)
|
|
.value("n_out_of_m", MLIR_SPARSE_TENSOR_LEVEL_N_OUT_OF_M)
|
|
.value("compressed", MLIR_SPARSE_TENSOR_LEVEL_COMPRESSED)
|
|
.value("singleton", MLIR_SPARSE_TENSOR_LEVEL_SINGLETON)
|
|
.value("loose_compressed", MLIR_SPARSE_TENSOR_LEVEL_LOOSE_COMPRESSED);
|
|
|
|
nb::enum_<MlirSparseTensorLevelPropertyNondefault>(m, "LevelProperty")
|
|
.value("non_ordered", MLIR_SPARSE_PROPERTY_NON_ORDERED)
|
|
.value("non_unique", MLIR_SPARSE_PROPERTY_NON_UNIQUE)
|
|
.value("soa", MLIR_SPARSE_PROPERTY_SOA);
|
|
|
|
mlir_attribute_subclass(m, "EncodingAttr",
|
|
mlirAttributeIsASparseTensorEncodingAttr)
|
|
.def_classmethod(
|
|
"get",
|
|
[](nb::object cls, std::vector<MlirSparseTensorLevelType> lvlTypes,
|
|
std::optional<MlirAffineMap> dimToLvl,
|
|
std::optional<MlirAffineMap> lvlToDim, int posWidth, int crdWidth,
|
|
std::optional<MlirAttribute> explicitVal,
|
|
std::optional<MlirAttribute> implicitVal, MlirContext context) {
|
|
return cls(mlirSparseTensorEncodingAttrGet(
|
|
context, lvlTypes.size(), lvlTypes.data(),
|
|
dimToLvl ? *dimToLvl : MlirAffineMap{nullptr},
|
|
lvlToDim ? *lvlToDim : MlirAffineMap{nullptr}, posWidth,
|
|
crdWidth, explicitVal ? *explicitVal : MlirAttribute{nullptr},
|
|
implicitVal ? *implicitVal : MlirAttribute{nullptr}));
|
|
},
|
|
nb::arg("cls"), nb::arg("lvl_types"), nb::arg("dim_to_lvl").none(),
|
|
nb::arg("lvl_to_dim").none(), nb::arg("pos_width"),
|
|
nb::arg("crd_width"), nb::arg("explicit_val").none() = nb::none(),
|
|
nb::arg("implicit_val").none() = nb::none(),
|
|
nb::arg("context").none() = nb::none(),
|
|
"Gets a sparse_tensor.encoding from parameters.")
|
|
.def_classmethod(
|
|
"build_level_type",
|
|
[](nb::object cls, MlirSparseTensorLevelFormat lvlFmt,
|
|
const std::vector<MlirSparseTensorLevelPropertyNondefault>
|
|
&properties,
|
|
unsigned n, unsigned m) {
|
|
return mlirSparseTensorEncodingAttrBuildLvlType(
|
|
lvlFmt, properties.data(), properties.size(), n, m);
|
|
},
|
|
nb::arg("cls"), nb::arg("lvl_fmt"),
|
|
nb::arg("properties") =
|
|
std::vector<MlirSparseTensorLevelPropertyNondefault>(),
|
|
nb::arg("n") = 0, nb::arg("m") = 0,
|
|
"Builds a sparse_tensor.encoding.level_type from parameters.")
|
|
.def_property_readonly(
|
|
"lvl_types",
|
|
[](MlirAttribute self) {
|
|
const int lvlRank = mlirSparseTensorEncodingGetLvlRank(self);
|
|
std::vector<MlirSparseTensorLevelType> ret;
|
|
ret.reserve(lvlRank);
|
|
for (int l = 0; l < lvlRank; ++l)
|
|
ret.push_back(mlirSparseTensorEncodingAttrGetLvlType(self, l));
|
|
return ret;
|
|
})
|
|
.def_property_readonly(
|
|
"dim_to_lvl",
|
|
[](MlirAttribute self) -> std::optional<MlirAffineMap> {
|
|
MlirAffineMap ret = mlirSparseTensorEncodingAttrGetDimToLvl(self);
|
|
if (mlirAffineMapIsNull(ret))
|
|
return {};
|
|
return ret;
|
|
})
|
|
.def_property_readonly(
|
|
"lvl_to_dim",
|
|
[](MlirAttribute self) -> std::optional<MlirAffineMap> {
|
|
MlirAffineMap ret = mlirSparseTensorEncodingAttrGetLvlToDim(self);
|
|
if (mlirAffineMapIsNull(ret))
|
|
return {};
|
|
return ret;
|
|
})
|
|
.def_property_readonly("pos_width",
|
|
mlirSparseTensorEncodingAttrGetPosWidth)
|
|
.def_property_readonly("crd_width",
|
|
mlirSparseTensorEncodingAttrGetCrdWidth)
|
|
.def_property_readonly(
|
|
"explicit_val",
|
|
[](MlirAttribute self) -> std::optional<MlirAttribute> {
|
|
MlirAttribute ret =
|
|
mlirSparseTensorEncodingAttrGetExplicitVal(self);
|
|
if (mlirAttributeIsNull(ret))
|
|
return {};
|
|
return ret;
|
|
})
|
|
.def_property_readonly(
|
|
"implicit_val",
|
|
[](MlirAttribute self) -> std::optional<MlirAttribute> {
|
|
MlirAttribute ret =
|
|
mlirSparseTensorEncodingAttrGetImplicitVal(self);
|
|
if (mlirAttributeIsNull(ret))
|
|
return {};
|
|
return ret;
|
|
})
|
|
.def_property_readonly(
|
|
"structured_n",
|
|
[](MlirAttribute self) -> unsigned {
|
|
const int lvlRank = mlirSparseTensorEncodingGetLvlRank(self);
|
|
return mlirSparseTensorEncodingAttrGetStructuredN(
|
|
mlirSparseTensorEncodingAttrGetLvlType(self, lvlRank - 1));
|
|
})
|
|
.def_property_readonly(
|
|
"structured_m",
|
|
[](MlirAttribute self) -> unsigned {
|
|
const int lvlRank = mlirSparseTensorEncodingGetLvlRank(self);
|
|
return mlirSparseTensorEncodingAttrGetStructuredM(
|
|
mlirSparseTensorEncodingAttrGetLvlType(self, lvlRank - 1));
|
|
})
|
|
.def_property_readonly("lvl_formats_enum", [](MlirAttribute self) {
|
|
const int lvlRank = mlirSparseTensorEncodingGetLvlRank(self);
|
|
std::vector<MlirSparseTensorLevelFormat> ret;
|
|
ret.reserve(lvlRank);
|
|
for (int l = 0; l < lvlRank; l++)
|
|
ret.push_back(mlirSparseTensorEncodingAttrGetLvlFmt(self, l));
|
|
return ret;
|
|
});
|
|
}
|
|
|
|
NB_MODULE(_mlirDialectsSparseTensor, m) {
|
|
m.doc() = "MLIR SparseTensor dialect.";
|
|
populateDialectSparseTensorSubmodule(m);
|
|
}
|