mirror of
https://github.com/llvm/llvm-project.git
synced 2025-04-29 02:06:06 +00:00
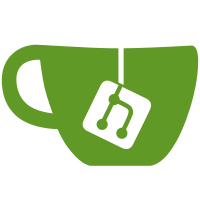
This CL implements a very simple loop vectorization **test** and the basic infrastructure to support it. The test simply consists in: 1. matching the loops in the MLFunction and all the Load/Store operations nested under the loop; 2. testing whether all the Load/Store are contiguous along the innermost memory dimension along that particular loop. If any reference is non-contiguous (i.e. the ForStmt SSAValue appears in the expression), then the loop is not-vectorizable. The simple test above can gradually be extended with more interesting behaviors to account for the fact that a layout permutation may exist that enables contiguity etc. All these will come in due time but it is worthwhile noting that the test already supports detection of outer-vetorizable loops. In implementing this test, I also added a recursive MLFunctionMatcher and some sugar that can capture patterns such as `auto gemmLike = Doall(Doall(Red(LoadStore())))` and allows iterating on the matched IR structures. For now it just uses in order traversal but post-order DFS will be useful in the future once IR rewrites start occuring. One may note that the memory management design decision follows a different pattern from MLIR. After evaluating different designs and how they quickly increase cognitive overhead, I decided to opt for the simplest solution in my view: a class-wide (threadsafe) RAII context. This way, a pass that needs MLFunctionMatcher can just have its own locally scoped BumpPtrAllocator and everything is cleaned up when the pass is destroyed. If passes are expected to have a longer lifetime, then the contexts can easily be scoped inside the runOnMLFunction call and storage lifetime reduced. Lastly, whatever the scope of threading (module, function, pass), this is expected to also be future-proof wrt concurrency (but this is a detail atm). PiperOrigin-RevId: 217622889
200 lines
7.4 KiB
C++
200 lines
7.4 KiB
C++
//===- LoopAnalysis.cpp - Misc loop analysis routines //-------------------===//
|
|
//
|
|
// Copyright 2019 The MLIR Authors.
|
|
//
|
|
// Licensed under the Apache License, Version 2.0 (the "License");
|
|
// you may not use this file except in compliance with the License.
|
|
// You may obtain a copy of the License at
|
|
//
|
|
// http://www.apache.org/licenses/LICENSE-2.0
|
|
//
|
|
// Unless required by applicable law or agreed to in writing, software
|
|
// distributed under the License is distributed on an "AS IS" BASIS,
|
|
// WITHOUT WARRANTIES OR CONDITIONS OF ANY KIND, either express or implied.
|
|
// See the License for the specific language governing permissions and
|
|
// limitations under the License.
|
|
// =============================================================================
|
|
//
|
|
// This file implements miscellaneous loop analysis routines.
|
|
//
|
|
//===----------------------------------------------------------------------===//
|
|
|
|
#include "mlir/Analysis/LoopAnalysis.h"
|
|
|
|
#include "mlir/Analysis/AffineAnalysis.h"
|
|
#include "mlir/Analysis/AffineStructures.h"
|
|
#include "mlir/Analysis/MLFunctionMatcher.h"
|
|
#include "mlir/IR/AffineExpr.h"
|
|
#include "mlir/IR/AffineMap.h"
|
|
#include "mlir/IR/Builders.h"
|
|
#include "mlir/IR/BuiltinOps.h"
|
|
#include "mlir/IR/Statements.h"
|
|
#include "mlir/StandardOps/StandardOps.h"
|
|
#include "mlir/Support/MathExtras.h"
|
|
|
|
using namespace mlir;
|
|
|
|
/// Returns the trip count of the loop as an affine expression if the latter is
|
|
/// expressible as an affine expression, and nullptr otherwise. The trip count
|
|
/// expression is simplified before returning.
|
|
AffineExpr mlir::getTripCountExpr(const ForStmt &forStmt) {
|
|
// upper_bound - lower_bound + 1
|
|
int64_t loopSpan;
|
|
|
|
int64_t step = forStmt.getStep();
|
|
auto *context = forStmt.getContext();
|
|
|
|
if (forStmt.hasConstantBounds()) {
|
|
int64_t lb = forStmt.getConstantLowerBound();
|
|
int64_t ub = forStmt.getConstantUpperBound();
|
|
loopSpan = ub - lb + 1;
|
|
} else {
|
|
auto lbMap = forStmt.getLowerBoundMap();
|
|
auto ubMap = forStmt.getUpperBoundMap();
|
|
// TODO(bondhugula): handle max/min of multiple expressions.
|
|
if (lbMap.getNumResults() != 1 || ubMap.getNumResults() != 1)
|
|
return nullptr;
|
|
|
|
// TODO(bondhugula): handle bounds with different operands.
|
|
// Bounds have different operands, unhandled for now.
|
|
if (!forStmt.matchingBoundOperandList())
|
|
return nullptr;
|
|
|
|
// ub_expr - lb_expr + 1
|
|
AffineExpr lbExpr(lbMap.getResult(0));
|
|
AffineExpr ubExpr(ubMap.getResult(0));
|
|
auto loopSpanExpr = simplifyAffineExpr(
|
|
ubExpr - lbExpr + 1, std::max(lbMap.getNumDims(), ubMap.getNumDims()),
|
|
std::max(lbMap.getNumSymbols(), ubMap.getNumSymbols()));
|
|
auto cExpr = loopSpanExpr.dyn_cast<AffineConstantExpr>();
|
|
if (!cExpr)
|
|
return loopSpanExpr.ceilDiv(step);
|
|
loopSpan = cExpr.getValue();
|
|
}
|
|
|
|
// 0 iteration loops.
|
|
if (loopSpan < 0)
|
|
return 0;
|
|
|
|
return getAffineConstantExpr(static_cast<uint64_t>(ceilDiv(loopSpan, step)),
|
|
context);
|
|
}
|
|
|
|
/// Returns the trip count of the loop if it's a constant, None otherwise. This
|
|
/// method uses affine expression analysis (in turn using getTripCount) and is
|
|
/// able to determine constant trip count in non-trivial cases.
|
|
llvm::Optional<uint64_t> mlir::getConstantTripCount(const ForStmt &forStmt) {
|
|
auto tripCountExpr = getTripCountExpr(forStmt);
|
|
|
|
if (!tripCountExpr)
|
|
return None;
|
|
|
|
if (auto constExpr = tripCountExpr.dyn_cast<AffineConstantExpr>())
|
|
return constExpr.getValue();
|
|
|
|
return None;
|
|
}
|
|
|
|
/// Returns the greatest known integral divisor of the trip count. Affine
|
|
/// expression analysis is used (indirectly through getTripCount), and
|
|
/// this method is thus able to determine non-trivial divisors.
|
|
uint64_t mlir::getLargestDivisorOfTripCount(const ForStmt &forStmt) {
|
|
auto tripCountExpr = getTripCountExpr(forStmt);
|
|
|
|
if (!tripCountExpr)
|
|
return 1;
|
|
|
|
if (auto constExpr = tripCountExpr.dyn_cast<AffineConstantExpr>()) {
|
|
uint64_t tripCount = constExpr.getValue();
|
|
|
|
// 0 iteration loops (greatest divisor is 2^64 - 1).
|
|
if (tripCount == 0)
|
|
return ULONG_MAX;
|
|
|
|
// The greatest divisor is the trip count.
|
|
return tripCount;
|
|
}
|
|
|
|
// Trip count is not a known constant; return its largest known divisor.
|
|
return tripCountExpr.getLargestKnownDivisor();
|
|
}
|
|
|
|
/// Given a MemRef accessed by `indices` and a dimension `dim`, determines
|
|
/// whether indices[dim] is independent of the value `input`.
|
|
// For now we assume no layout map or identity layout map in the MemRef.
|
|
// TODO(ntv): support more than identity layout map.
|
|
static bool isAccessInvariant(MLValue *input, MemRefType *memRefType,
|
|
ArrayRef<MLValue *> indices, unsigned dim) {
|
|
assert(indices.size() == memRefType->getRank());
|
|
assert(dim < indices.size());
|
|
auto layoutMap = memRefType->getAffineMaps();
|
|
assert(layoutMap.size() <= 1);
|
|
// TODO(ntv): remove dependency on Builder once we support non-identity
|
|
// layout map.
|
|
Builder b(memRefType->getContext());
|
|
assert(layoutMap.empty() ||
|
|
layoutMap[0] == b.getMultiDimIdentityMap(indices.size()));
|
|
|
|
SmallVector<OperationStmt *, 4> affineApplyOps;
|
|
getReachableAffineApplyOps({indices[dim]}, affineApplyOps);
|
|
|
|
if (affineApplyOps.empty()) {
|
|
// Pointer equality test because of MLValue pointer semantics.
|
|
return indices[dim] != input;
|
|
}
|
|
|
|
assert(affineApplyOps.size() == 1 &&
|
|
"CompositionAffineMapsPass must have "
|
|
"been run: there should be at most one AffineApplyOp");
|
|
auto composeOp = affineApplyOps[0]->getAs<AffineApplyOp>();
|
|
return !AffineValueMap(*composeOp).isFunctionOf(dim, input);
|
|
}
|
|
|
|
/// Determines whether a load or a store has a contiguous access along the
|
|
/// value `input`. Contiguous is defined as either invariant or varying only
|
|
/// along the fastest varying memory dimension.
|
|
// TODO(ntv): allow more advanced notions of contiguity (non-fastest varying,
|
|
// check strides, ...).
|
|
template <typename LoadOrStoreOpPointer>
|
|
static bool isContiguousAccess(MLValue *input, LoadOrStoreOpPointer memoryOp) {
|
|
auto indicesAsOperandIterators = memoryOp->getIndices();
|
|
auto *memRefType = cast<MemRefType>(memoryOp->getMemRef()->getType());
|
|
SmallVector<MLValue *, 4> indices;
|
|
for (auto *it : indicesAsOperandIterators) {
|
|
indices.push_back(cast<MLValue>(it));
|
|
}
|
|
unsigned numIndices = indices.size();
|
|
for (unsigned d = 0; d < numIndices - 1; ++d) {
|
|
if (!isAccessInvariant(input, memRefType, indices, d)) {
|
|
return false;
|
|
}
|
|
}
|
|
return true;
|
|
}
|
|
|
|
/// Checks whether all the LoadOp and StoreOp matched have access indexing
|
|
/// functions that are are either:
|
|
/// 1. invariant along the `loop` induction variable;
|
|
/// 2. varying along the fastest varying memory dimension only.
|
|
// TODO(ntv): Also need to check the contiguous dimension to discriminate
|
|
// between broadcast (i.e. stride 0), stride 1 and stride > 1 and return the
|
|
// information so we can build a cost model.
|
|
bool mlir::isVectorizableLoop(const ForStmt &loop) {
|
|
// TODO(ntv): check parallel or reduction loop semantics
|
|
using matcher::LoadStores;
|
|
auto *forStmt = &const_cast<ForStmt &>(loop);
|
|
auto loadAndStores = LoadStores();
|
|
auto &matches = loadAndStores.match(forStmt);
|
|
for (auto ls : matches) {
|
|
auto *op = cast<OperationStmt>(ls.first);
|
|
auto load = op->getAs<LoadOp>();
|
|
auto store = op->getAs<StoreOp>();
|
|
bool contiguous = load ? isContiguousAccess(forStmt, load)
|
|
: isContiguousAccess(forStmt, store);
|
|
if (!contiguous) {
|
|
return false;
|
|
}
|
|
}
|
|
return true;
|
|
}
|