mirror of
https://github.com/llvm/llvm-project.git
synced 2025-05-10 00:36:06 +00:00
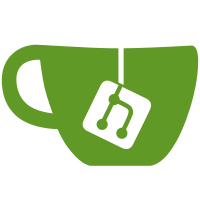
This revision reintroduces tensor.insert_slice verification which seems to have vanished over time: a verifier was initially introduced in cf9503c1b752062d9abfb2c7922a50574d9c5de4 but for some reason the invalid.mlir was not properly updated; as time passed the verifier was not called anymore and later the code was deleted. As a consequence, a non-negligible portion of tests has run astray using invalid tensor.insert_slice semantics and needed to be fixed. Also, extract isRankReducedType from TensorOps for better reuse Originally, this facility was used by both tensor and memref forms but it got copied around as dialects were split. Differential Revision: https://reviews.llvm.org/D114715
1334 lines
53 KiB
C++
1334 lines
53 KiB
C++
//===----------------------------------------------------------------------===//
|
|
//
|
|
// Part of the LLVM Project, under the Apache License v2.0 with LLVM Exceptions.
|
|
// See https://llvm.org/LICENSE.txt for license information.
|
|
// SPDX-License-Identifier: Apache-2.0 WITH LLVM-exception
|
|
//
|
|
//===----------------------------------------------------------------------===//
|
|
|
|
#include "mlir/Dialect/Arithmetic/IR/Arithmetic.h"
|
|
#include "mlir/Dialect/StandardOps/Utils/Utils.h"
|
|
#include "mlir/Dialect/Tensor/IR/Tensor.h"
|
|
#include "mlir/Dialect/Utils/StaticValueUtils.h"
|
|
#include "mlir/IR/BlockAndValueMapping.h"
|
|
#include "mlir/IR/Builders.h"
|
|
#include "mlir/IR/BuiltinAttributeInterfaces.h"
|
|
#include "mlir/IR/Matchers.h"
|
|
#include "mlir/IR/PatternMatch.h"
|
|
#include "mlir/IR/TypeUtilities.h"
|
|
#include "llvm/ADT/STLExtras.h"
|
|
|
|
using namespace mlir;
|
|
using namespace mlir::tensor;
|
|
|
|
/// Materialize a single constant operation from a given attribute value with
|
|
/// the desired resultant type.
|
|
Operation *TensorDialect::materializeConstant(OpBuilder &builder,
|
|
Attribute value, Type type,
|
|
Location loc) {
|
|
if (arith::ConstantOp::isBuildableWith(value, type))
|
|
return builder.create<arith::ConstantOp>(loc, value, type);
|
|
if (ConstantOp::isBuildableWith(value, type))
|
|
return builder.create<ConstantOp>(loc, value, type);
|
|
return nullptr;
|
|
}
|
|
|
|
//===----------------------------------------------------------------------===//
|
|
// CastOp
|
|
//===----------------------------------------------------------------------===//
|
|
|
|
/// Returns true if `target` is a ranked tensor type that preserves static
|
|
/// information available in the `source` ranked tensor type.
|
|
bool mlir::tensor::preservesStaticInformation(Type source, Type target) {
|
|
auto sourceType = source.dyn_cast<RankedTensorType>();
|
|
auto targetType = target.dyn_cast<RankedTensorType>();
|
|
|
|
// Requires RankedTensorType.
|
|
if (!sourceType || !targetType)
|
|
return false;
|
|
|
|
// Requires same elemental type.
|
|
if (sourceType.getElementType() != targetType.getElementType())
|
|
return false;
|
|
|
|
// Requires same rank.
|
|
if (sourceType.getRank() != targetType.getRank())
|
|
return false;
|
|
|
|
// If cast is towards more static sizes along any dimension, don't fold.
|
|
for (auto t : llvm::zip(sourceType.getShape(), targetType.getShape())) {
|
|
if (!ShapedType::isDynamic(std::get<0>(t)) &&
|
|
ShapedType::isDynamic(std::get<1>(t)))
|
|
return false;
|
|
}
|
|
|
|
return true;
|
|
}
|
|
|
|
/// Determines whether tensor::CastOp casts to a more dynamic version of the
|
|
/// source tensor. This is useful to fold a tensor.cast into a consuming op and
|
|
/// implement canonicalization patterns for ops in different dialects that may
|
|
/// consume the results of tensor.cast operations. Such foldable tensor.cast
|
|
/// operations are typically inserted as `slice` ops and are canonicalized,
|
|
/// to preserve the type compatibility of their uses.
|
|
///
|
|
/// Returns true when all conditions are met:
|
|
/// 1. source and result are ranked tensors with same element type and rank.
|
|
/// 2. the tensor type has more static information than the result
|
|
///
|
|
/// Example:
|
|
/// ```mlir
|
|
/// %1 = tensor.cast %0 : tensor<8x16xf32> to tensor<?x?xf32>
|
|
/// %2 = consumer %1 ... : tensor<?x?xf32> ...
|
|
/// ```
|
|
///
|
|
/// folds into:
|
|
///
|
|
/// ```mlir
|
|
/// %2 = consumer %0 ... : tensor<8x16xf32> ...
|
|
/// ```
|
|
bool mlir::tensor::canFoldIntoConsumerOp(CastOp castOp) {
|
|
if (!castOp)
|
|
return false;
|
|
|
|
// Can fold if the source of cast has at least as much static information as
|
|
// its results.
|
|
return preservesStaticInformation(castOp.getType(),
|
|
castOp.source().getType());
|
|
}
|
|
|
|
/// Performs folding of any operand of `op` if it comes from a tensor::CastOp
|
|
/// that can be folded.
|
|
LogicalResult mlir::tensor::foldTensorCast(Operation *op) {
|
|
bool folded = false;
|
|
for (OpOperand &operand : op->getOpOperands()) {
|
|
auto castOp = operand.get().getDefiningOp<tensor::CastOp>();
|
|
if (castOp && tensor::canFoldIntoConsumerOp(castOp)) {
|
|
operand.set(castOp.getOperand());
|
|
folded = true;
|
|
}
|
|
}
|
|
return success(folded);
|
|
}
|
|
|
|
bool CastOp::areCastCompatible(TypeRange inputs, TypeRange outputs) {
|
|
if (inputs.size() != 1 || outputs.size() != 1)
|
|
return false;
|
|
Type a = inputs.front(), b = outputs.front();
|
|
auto aT = a.dyn_cast<TensorType>();
|
|
auto bT = b.dyn_cast<TensorType>();
|
|
if (!aT || !bT)
|
|
return false;
|
|
|
|
if (aT.getElementType() != bT.getElementType())
|
|
return false;
|
|
|
|
return succeeded(verifyCompatibleShape(aT, bT));
|
|
}
|
|
|
|
/// Compute a TensorType that has the joined shape knowledge of the two
|
|
/// given TensorTypes. The element types need to match.
|
|
static TensorType joinShapes(TensorType one, TensorType two) {
|
|
assert(one.getElementType() == two.getElementType());
|
|
|
|
if (!one.hasRank())
|
|
return two;
|
|
if (!two.hasRank())
|
|
return one;
|
|
|
|
int64_t rank = one.getRank();
|
|
if (rank != two.getRank())
|
|
return {};
|
|
|
|
SmallVector<int64_t, 4> join;
|
|
join.reserve(rank);
|
|
for (int64_t i = 0; i < rank; ++i) {
|
|
if (one.isDynamicDim(i)) {
|
|
join.push_back(two.getDimSize(i));
|
|
continue;
|
|
}
|
|
if (two.isDynamicDim(i)) {
|
|
join.push_back(one.getDimSize(i));
|
|
continue;
|
|
}
|
|
if (one.getDimSize(i) != two.getDimSize(i))
|
|
return {};
|
|
join.push_back(one.getDimSize(i));
|
|
}
|
|
return RankedTensorType::get(join, one.getElementType());
|
|
}
|
|
|
|
namespace {
|
|
|
|
/// Replaces chains of two tensor.cast operations by a single tensor.cast
|
|
/// operation if doing so does not remove runtime constraints.
|
|
struct ChainedTensorCast : public OpRewritePattern<CastOp> {
|
|
using OpRewritePattern<CastOp>::OpRewritePattern;
|
|
|
|
LogicalResult matchAndRewrite(CastOp tensorCast,
|
|
PatternRewriter &rewriter) const final {
|
|
auto tensorCastOperand = tensorCast.getOperand().getDefiningOp<CastOp>();
|
|
|
|
if (!tensorCastOperand)
|
|
return failure();
|
|
|
|
auto sourceType =
|
|
tensorCastOperand.getOperand().getType().cast<TensorType>();
|
|
auto intermediateType = tensorCastOperand.getType().cast<TensorType>();
|
|
auto resultType = tensorCast.getType().cast<TensorType>();
|
|
|
|
// We can remove the intermediate cast if joining all three produces the
|
|
// same result as just joining the source and result shapes.
|
|
auto firstJoin =
|
|
joinShapes(joinShapes(sourceType, intermediateType), resultType);
|
|
|
|
// The join might not exist if the cast sequence would fail at runtime.
|
|
if (!firstJoin)
|
|
return failure();
|
|
|
|
// The newJoin always exists if the above join exists, it might just contain
|
|
// less information. If so, we cannot drop the intermediate cast, as doing
|
|
// so would remove runtime checks.
|
|
auto newJoin = joinShapes(sourceType, resultType);
|
|
if (firstJoin != newJoin)
|
|
return failure();
|
|
|
|
rewriter.replaceOpWithNewOp<CastOp>(tensorCast, resultType,
|
|
tensorCastOperand.getOperand());
|
|
return success();
|
|
}
|
|
};
|
|
|
|
} // namespace
|
|
|
|
void CastOp::getCanonicalizationPatterns(RewritePatternSet &results,
|
|
MLIRContext *context) {
|
|
results.add<ChainedTensorCast>(context);
|
|
}
|
|
|
|
//===----------------------------------------------------------------------===//
|
|
// DimOp
|
|
//===----------------------------------------------------------------------===//
|
|
|
|
void DimOp::build(OpBuilder &builder, OperationState &result, Value source,
|
|
int64_t index) {
|
|
auto loc = result.location;
|
|
Value indexValue = builder.create<arith::ConstantIndexOp>(loc, index);
|
|
build(builder, result, source, indexValue);
|
|
}
|
|
|
|
Optional<int64_t> DimOp::getConstantIndex() {
|
|
if (auto constantOp = index().getDefiningOp<arith::ConstantOp>())
|
|
return constantOp.getValue().cast<IntegerAttr>().getInt();
|
|
return {};
|
|
}
|
|
|
|
static LogicalResult verify(DimOp op) {
|
|
// Assume unknown index to be in range.
|
|
Optional<int64_t> index = op.getConstantIndex();
|
|
if (!index.hasValue())
|
|
return success();
|
|
|
|
// Check that constant index is not knowingly out of range.
|
|
auto type = op.source().getType();
|
|
if (auto tensorType = type.dyn_cast<RankedTensorType>()) {
|
|
if (index.getValue() >= tensorType.getRank())
|
|
return op.emitOpError("index is out of range");
|
|
} else if (type.isa<UnrankedTensorType>()) {
|
|
// Assume index to be in range.
|
|
} else {
|
|
llvm_unreachable("expected operand with tensor type");
|
|
}
|
|
return success();
|
|
}
|
|
|
|
OpFoldResult DimOp::fold(ArrayRef<Attribute> operands) {
|
|
// All forms of folding require a known index.
|
|
auto index = operands[1].dyn_cast_or_null<IntegerAttr>();
|
|
if (!index)
|
|
return {};
|
|
|
|
// Folding for unranked types (UnrankedTensorType) is not supported.
|
|
auto tensorType = source().getType().dyn_cast<RankedTensorType>();
|
|
if (!tensorType)
|
|
return {};
|
|
|
|
// Fold if the shape extent along the given index is known.
|
|
if (!tensorType.isDynamicDim(index.getInt())) {
|
|
Builder builder(getContext());
|
|
return builder.getIndexAttr(tensorType.getShape()[index.getInt()]);
|
|
}
|
|
|
|
Operation *definingOp = source().getDefiningOp();
|
|
|
|
// Fold dim to the operand of tensor.generate.
|
|
if (auto fromElements = dyn_cast_or_null<tensor::GenerateOp>(definingOp)) {
|
|
auto resultType =
|
|
fromElements.getResult().getType().cast<RankedTensorType>();
|
|
// The case where the type encodes the size of the dimension is handled
|
|
// above.
|
|
assert(resultType.getShape()[index.getInt()] ==
|
|
RankedTensorType::kDynamicSize);
|
|
|
|
// Find the operand of the fromElements that corresponds to this index.
|
|
auto dynExtents = fromElements.dynamicExtents().begin();
|
|
for (auto dim : resultType.getShape().take_front(index.getInt()))
|
|
if (dim == RankedTensorType::kDynamicSize)
|
|
dynExtents++;
|
|
|
|
return Value{*dynExtents};
|
|
}
|
|
|
|
// The size at the given index is now known to be a dynamic size.
|
|
unsigned unsignedIndex = index.getValue().getZExtValue();
|
|
|
|
if (auto sliceOp = dyn_cast_or_null<tensor::ExtractSliceOp>(definingOp)) {
|
|
// Fold only for non-rank reduced ops. For the rank-reduced version, rely on
|
|
// `resolve-shaped-type-result-dims` pass.
|
|
if (sliceOp.getType().getRank() == sliceOp.getSourceType().getRank() &&
|
|
sliceOp.isDynamicSize(unsignedIndex)) {
|
|
return {sliceOp.getDynamicSize(unsignedIndex)};
|
|
}
|
|
}
|
|
|
|
// dim(cast) -> dim
|
|
if (succeeded(foldTensorCast(*this)))
|
|
return getResult();
|
|
|
|
return {};
|
|
}
|
|
|
|
namespace {
|
|
/// Fold dim of a cast into the dim of the source of the tensor cast.
|
|
struct DimOfCastOp : public OpRewritePattern<DimOp> {
|
|
using OpRewritePattern<DimOp>::OpRewritePattern;
|
|
|
|
LogicalResult matchAndRewrite(DimOp dimOp,
|
|
PatternRewriter &rewriter) const override {
|
|
auto castOp = dimOp.source().getDefiningOp<CastOp>();
|
|
if (!castOp)
|
|
return failure();
|
|
Value newSource = castOp.getOperand();
|
|
rewriter.replaceOpWithNewOp<DimOp>(dimOp, newSource, dimOp.index());
|
|
return success();
|
|
}
|
|
};
|
|
} // end anonymous namespace.
|
|
|
|
void DimOp::getCanonicalizationPatterns(RewritePatternSet &results,
|
|
MLIRContext *context) {
|
|
results.add<DimOfCastOp>(context);
|
|
}
|
|
|
|
//===----------------------------------------------------------------------===//
|
|
// ExtractOp
|
|
//===----------------------------------------------------------------------===//
|
|
|
|
static LogicalResult verify(ExtractOp op) {
|
|
// Verify the # indices match if we have a ranked type.
|
|
if (auto tensorType = op.tensor().getType().dyn_cast<RankedTensorType>())
|
|
if (tensorType.getRank() != static_cast<int64_t>(op.indices().size()))
|
|
return op.emitOpError("incorrect number of indices for extract_element");
|
|
|
|
return success();
|
|
}
|
|
|
|
OpFoldResult ExtractOp::fold(ArrayRef<Attribute> operands) {
|
|
// The tensor operand must be a known constant.
|
|
Attribute tensor = operands.front();
|
|
if (!tensor)
|
|
return {};
|
|
// If this is a splat elements attribute, simply return the value. All of the
|
|
// elements of a splat attribute are the same.
|
|
if (auto splatTensor = tensor.dyn_cast<SplatElementsAttr>())
|
|
return splatTensor.getSplatValue<Attribute>();
|
|
|
|
// Otherwise, collect the constant indices into the tensor.
|
|
SmallVector<uint64_t, 8> indices;
|
|
for (Attribute indice : llvm::drop_begin(operands, 1)) {
|
|
if (!indice || !indice.isa<IntegerAttr>())
|
|
return {};
|
|
indices.push_back(indice.cast<IntegerAttr>().getInt());
|
|
}
|
|
|
|
// If this is an elements attribute, query the value at the given indices.
|
|
auto elementsAttr = tensor.dyn_cast<ElementsAttr>();
|
|
if (elementsAttr && elementsAttr.isValidIndex(indices))
|
|
return elementsAttr.getValues<Attribute>()[indices];
|
|
return {};
|
|
}
|
|
|
|
//===----------------------------------------------------------------------===//
|
|
// FromElementsOp
|
|
//===----------------------------------------------------------------------===//
|
|
|
|
void FromElementsOp::build(OpBuilder &builder, OperationState &result,
|
|
Type elementType, ValueRange elements) {
|
|
Type resultTy = RankedTensorType::get({static_cast<int64_t>(elements.size())},
|
|
elementType);
|
|
result.addOperands(elements);
|
|
result.addTypes(resultTy);
|
|
}
|
|
|
|
void FromElementsOp::build(OpBuilder &builder, OperationState &result,
|
|
ValueRange elements) {
|
|
assert(!elements.empty() && "expected at least one element");
|
|
build(builder, result, elements.front().getType(), elements);
|
|
}
|
|
|
|
OpFoldResult FromElementsOp::fold(ArrayRef<Attribute> operands) {
|
|
if (!llvm::is_contained(operands, nullptr))
|
|
return DenseElementsAttr::get(getType(), operands);
|
|
return {};
|
|
}
|
|
|
|
namespace {
|
|
|
|
// Canonicalizes the pattern of the form
|
|
//
|
|
// %tensor = tensor.from_elements(%element) : (i32) -> tensor<1xi32>
|
|
// %extracted_element = tensor.extract %tensor[%c0] : tensor<1xi32>
|
|
//
|
|
// to just %element.
|
|
struct ExtractElementFromTensorFromElements
|
|
: public OpRewritePattern<tensor::ExtractOp> {
|
|
using OpRewritePattern<tensor::ExtractOp>::OpRewritePattern;
|
|
|
|
LogicalResult matchAndRewrite(tensor::ExtractOp extract,
|
|
PatternRewriter &rewriter) const final {
|
|
if (extract.indices().size() != 1)
|
|
return failure();
|
|
|
|
auto tensorFromElements = extract.tensor().getDefiningOp<FromElementsOp>();
|
|
if (tensorFromElements == nullptr)
|
|
return failure();
|
|
|
|
APInt index;
|
|
if (!matchPattern(*extract.indices().begin(), m_ConstantInt(&index)))
|
|
return failure();
|
|
// Prevent out of bounds accesses. This can happen in invalid code that will
|
|
// never execute.
|
|
if (tensorFromElements->getNumOperands() <= index.getZExtValue() ||
|
|
index.getSExtValue() < 0)
|
|
return failure();
|
|
rewriter.replaceOp(extract,
|
|
tensorFromElements.getOperand(index.getZExtValue()));
|
|
return success();
|
|
}
|
|
};
|
|
|
|
} // namespace
|
|
|
|
void FromElementsOp::getCanonicalizationPatterns(RewritePatternSet &results,
|
|
MLIRContext *context) {
|
|
results.add<ExtractElementFromTensorFromElements>(context);
|
|
}
|
|
|
|
//===----------------------------------------------------------------------===//
|
|
// InsertOp
|
|
//===----------------------------------------------------------------------===//
|
|
|
|
static LogicalResult verify(InsertOp op) {
|
|
// Verify the # indices match if we have a ranked type.
|
|
if (auto destType = op.dest().getType().dyn_cast<RankedTensorType>())
|
|
if (destType.getRank() != static_cast<int64_t>(op.indices().size()))
|
|
return op.emitOpError("incorrect number of indices");
|
|
return success();
|
|
}
|
|
|
|
OpFoldResult InsertOp::fold(ArrayRef<Attribute> operands) {
|
|
Attribute scalar = operands[0];
|
|
Attribute dest = operands[1];
|
|
if (scalar && dest)
|
|
if (auto splatDest = dest.dyn_cast<SplatElementsAttr>())
|
|
if (scalar == splatDest.getSplatValue<Attribute>())
|
|
return dest;
|
|
return {};
|
|
}
|
|
|
|
//===----------------------------------------------------------------------===//
|
|
// GenerateOp
|
|
//===----------------------------------------------------------------------===//
|
|
|
|
static LogicalResult verify(GenerateOp op) {
|
|
// Ensure that the tensor type has as many dynamic dimensions as are specified
|
|
// by the operands.
|
|
RankedTensorType resultTy = op.getType().cast<RankedTensorType>();
|
|
if (op.getNumOperands() != resultTy.getNumDynamicDims())
|
|
return op.emitError("must have as many index operands as dynamic extents "
|
|
"in the result type");
|
|
|
|
// Ensure that region arguments span the index space.
|
|
if (!llvm::all_of(op.body().getArgumentTypes(),
|
|
[](Type ty) { return ty.isIndex(); }))
|
|
return op.emitError("all body arguments must be index");
|
|
if (op.body().getNumArguments() != resultTy.getRank())
|
|
return op.emitError("must have one body argument per input dimension");
|
|
|
|
// Ensure that the region yields an element of the right type.
|
|
auto yieldOp =
|
|
llvm::cast<YieldOp>(op.body().getBlocks().front().getTerminator());
|
|
if (yieldOp.value().getType() != resultTy.getElementType())
|
|
return op.emitOpError(
|
|
"body must be terminated with a `yield` operation of the tensor "
|
|
"element type");
|
|
|
|
return success();
|
|
}
|
|
|
|
void GenerateOp::build(
|
|
OpBuilder &b, OperationState &result, Type resultTy,
|
|
ValueRange dynamicExtents,
|
|
function_ref<void(OpBuilder &, Location, ValueRange)> bodyBuilder) {
|
|
build(b, result, resultTy, dynamicExtents);
|
|
|
|
// Build and populate body.
|
|
OpBuilder::InsertionGuard guard(b);
|
|
Region *bodyRegion = result.regions.front().get();
|
|
auto rank = resultTy.cast<RankedTensorType>().getRank();
|
|
SmallVector<Type, 2> argumentTypes(rank, b.getIndexType());
|
|
Block *bodyBlock =
|
|
b.createBlock(bodyRegion, bodyRegion->end(), argumentTypes);
|
|
bodyBuilder(b, result.location, bodyBlock->getArguments());
|
|
}
|
|
|
|
namespace {
|
|
|
|
/// Canonicalizes tensor.generate operations with a constant
|
|
/// operand into the equivalent operation with the operand expressed in the
|
|
/// result type, instead. We also insert a type cast to make sure that the
|
|
/// resulting IR is still well-typed.
|
|
struct StaticTensorGenerate : public OpRewritePattern<GenerateOp> {
|
|
using OpRewritePattern<GenerateOp>::OpRewritePattern;
|
|
|
|
LogicalResult matchAndRewrite(GenerateOp tensorFromElements,
|
|
PatternRewriter &rewriter) const final {
|
|
auto resultType =
|
|
tensorFromElements.getResult().getType().cast<RankedTensorType>();
|
|
|
|
if (resultType.hasStaticShape())
|
|
return failure();
|
|
|
|
SmallVector<Value, 4> newOperands;
|
|
SmallVector<int64_t, 4> newShape;
|
|
auto operandsIt = tensorFromElements.dynamicExtents().begin();
|
|
|
|
for (int64_t dim : resultType.getShape()) {
|
|
if (dim != RankedTensorType::kDynamicSize) {
|
|
newShape.push_back(dim);
|
|
continue;
|
|
}
|
|
APInt index;
|
|
if (!matchPattern(*operandsIt, m_ConstantInt(&index))) {
|
|
newShape.push_back(RankedTensorType::kDynamicSize);
|
|
newOperands.push_back(*operandsIt++);
|
|
continue;
|
|
}
|
|
newShape.push_back(index.getSExtValue());
|
|
operandsIt++;
|
|
}
|
|
|
|
if (newOperands.size() == tensorFromElements.dynamicExtents().size())
|
|
return failure();
|
|
|
|
auto loc = tensorFromElements.getLoc();
|
|
auto newOp = rewriter.create<GenerateOp>(
|
|
loc, RankedTensorType::get(newShape, resultType.getElementType()),
|
|
newOperands);
|
|
rewriter.inlineRegionBefore(tensorFromElements.body(), newOp.body(),
|
|
newOp.body().begin());
|
|
rewriter.replaceOpWithNewOp<tensor::CastOp>(tensorFromElements, resultType,
|
|
newOp);
|
|
return success();
|
|
}
|
|
};
|
|
|
|
/// Canonicalizes the pattern of the form
|
|
///
|
|
/// %tensor = tensor.generate %x {
|
|
/// ^bb0(%arg0: index): // no predecessors
|
|
/// <computation>
|
|
/// yield %1 : index
|
|
/// } : tensor<?xindex>
|
|
/// %extracted_element = tensor.extract %tensor[%c0] : tensor<?xi32>
|
|
///
|
|
/// to just <computation> with %arg0 replaced by %c0. We only do this if the
|
|
/// tensor.generate operation has no side-effects.
|
|
struct ExtractFromTensorGenerate : public OpRewritePattern<tensor::ExtractOp> {
|
|
using OpRewritePattern<tensor::ExtractOp>::OpRewritePattern;
|
|
|
|
LogicalResult matchAndRewrite(tensor::ExtractOp extract,
|
|
PatternRewriter &rewriter) const final {
|
|
auto tensorFromElements = extract.tensor().getDefiningOp<GenerateOp>();
|
|
if (!tensorFromElements || !wouldOpBeTriviallyDead(tensorFromElements))
|
|
return failure();
|
|
|
|
BlockAndValueMapping mapping;
|
|
Block *body = tensorFromElements.getBody();
|
|
mapping.map(body->getArguments(), extract.indices());
|
|
for (auto &op : body->without_terminator())
|
|
rewriter.clone(op, mapping);
|
|
|
|
auto yield = cast<YieldOp>(body->getTerminator());
|
|
|
|
rewriter.replaceOp(extract, mapping.lookupOrDefault(yield.value()));
|
|
return success();
|
|
}
|
|
};
|
|
|
|
/// Canonicalizes the pattern of the form
|
|
///
|
|
/// %val = tensor.cast %source : : tensor<?xi32> to tensor<2xi32>
|
|
/// %extracted_element = tensor.extract %val[%c0] : tensor<2xi32>
|
|
///
|
|
/// to
|
|
///
|
|
/// %extracted_element = tensor.extract %source[%c0] : tensor<?xi32>
|
|
struct ExtractFromTensorCast : public OpRewritePattern<tensor::ExtractOp> {
|
|
using OpRewritePattern<tensor::ExtractOp>::OpRewritePattern;
|
|
|
|
LogicalResult matchAndRewrite(tensor::ExtractOp extract,
|
|
PatternRewriter &rewriter) const final {
|
|
auto tensorCast = extract.tensor().getDefiningOp<tensor::CastOp>();
|
|
if (!tensorCast)
|
|
return failure();
|
|
|
|
rewriter.replaceOpWithNewOp<tensor::ExtractOp>(extract, tensorCast.source(),
|
|
extract.indices());
|
|
return success();
|
|
}
|
|
};
|
|
|
|
} // namespace
|
|
|
|
void GenerateOp::getCanonicalizationPatterns(RewritePatternSet &results,
|
|
MLIRContext *context) {
|
|
// TODO: Move extract patterns to tensor::ExtractOp.
|
|
results.add<ExtractFromTensorGenerate, ExtractFromTensorCast,
|
|
StaticTensorGenerate>(context);
|
|
}
|
|
|
|
//===----------------------------------------------------------------------===//
|
|
// ReshapeOp
|
|
//===----------------------------------------------------------------------===//
|
|
|
|
static int64_t GetNumElements(ShapedType type) {
|
|
int64_t numElements = 1;
|
|
for (auto dim : type.getShape())
|
|
numElements *= dim;
|
|
return numElements;
|
|
}
|
|
|
|
static LogicalResult verify(ReshapeOp op) {
|
|
TensorType operandType = op.source().getType().cast<TensorType>();
|
|
TensorType resultType = op.result().getType().cast<TensorType>();
|
|
|
|
if (operandType.getElementType() != resultType.getElementType())
|
|
return op.emitOpError("element types of source and destination tensor "
|
|
"types should be the same");
|
|
|
|
int64_t shapeSize =
|
|
op.shape().getType().cast<RankedTensorType>().getDimSize(0);
|
|
auto resultRankedType = resultType.dyn_cast<RankedTensorType>();
|
|
auto operandRankedType = operandType.dyn_cast<RankedTensorType>();
|
|
|
|
if (resultRankedType) {
|
|
if (operandRankedType && resultRankedType.hasStaticShape() &&
|
|
operandRankedType.hasStaticShape()) {
|
|
if (GetNumElements(operandRankedType) != GetNumElements(resultRankedType))
|
|
return op.emitOpError("source and destination tensor should have the "
|
|
"same number of elements");
|
|
}
|
|
if (shapeSize == TensorType::kDynamicSize)
|
|
return op.emitOpError("cannot use shape operand with dynamic length to "
|
|
"reshape to statically-ranked tensor type");
|
|
if (shapeSize != resultRankedType.getRank())
|
|
return op.emitOpError(
|
|
"length of shape operand differs from the result's tensor rank");
|
|
}
|
|
return success();
|
|
}
|
|
|
|
//===----------------------------------------------------------------------===//
|
|
// ExtractSliceOp
|
|
//===----------------------------------------------------------------------===//
|
|
|
|
/// An extract_slice op result type can be fully inferred from the source type
|
|
/// and the static representation of offsets, sizes and strides. Special
|
|
/// sentinels encode the dynamic case.
|
|
RankedTensorType
|
|
ExtractSliceOp::inferResultType(RankedTensorType sourceRankedTensorType,
|
|
ArrayRef<int64_t> leadingStaticOffsets,
|
|
ArrayRef<int64_t> leadingStaticSizes,
|
|
ArrayRef<int64_t> leadingStaticStrides) {
|
|
// An extract_slice op may specify only a leading subset of offset/sizes/
|
|
// strides in which case we complete with offset=0, sizes from memref type and
|
|
// strides=1.
|
|
unsigned rank = sourceRankedTensorType.getRank();
|
|
assert(leadingStaticSizes.size() <= rank &&
|
|
"unexpected leadingStaticSizes overflow");
|
|
auto staticSizes = llvm::to_vector<4>(leadingStaticSizes);
|
|
unsigned numTrailingSizes = rank - staticSizes.size();
|
|
llvm::append_range(staticSizes, sourceRankedTensorType.getShape().take_back(
|
|
numTrailingSizes));
|
|
return RankedTensorType::get(staticSizes,
|
|
sourceRankedTensorType.getElementType());
|
|
}
|
|
|
|
RankedTensorType
|
|
ExtractSliceOp::inferResultType(RankedTensorType sourceRankedTensorType,
|
|
ArrayRef<OpFoldResult> leadingStaticOffsets,
|
|
ArrayRef<OpFoldResult> leadingStaticSizes,
|
|
ArrayRef<OpFoldResult> leadingStaticStrides) {
|
|
SmallVector<int64_t> staticOffsets, staticSizes, staticStrides;
|
|
SmallVector<Value> dynamicOffsets, dynamicSizes, dynamicStrides;
|
|
dispatchIndexOpFoldResults(leadingStaticOffsets, dynamicOffsets,
|
|
staticOffsets, ShapedType::kDynamicStrideOrOffset);
|
|
dispatchIndexOpFoldResults(leadingStaticSizes, dynamicSizes, staticSizes,
|
|
ShapedType::kDynamicSize);
|
|
dispatchIndexOpFoldResults(leadingStaticStrides, dynamicStrides,
|
|
staticStrides, ShapedType::kDynamicStrideOrOffset);
|
|
return ExtractSliceOp::inferResultType(sourceRankedTensorType, staticOffsets,
|
|
staticSizes, staticStrides);
|
|
}
|
|
|
|
/// An extract_slice op result type can be fully inferred from the source type
|
|
/// and the static representation of offsets, sizes and strides. Special
|
|
/// sentinels encode the dynamic case.
|
|
RankedTensorType ExtractSliceOp::inferRankReducedResultType(
|
|
unsigned resultRank, RankedTensorType sourceRankedTensorType,
|
|
ArrayRef<int64_t> leadingStaticOffsets,
|
|
ArrayRef<int64_t> leadingStaticSizes,
|
|
ArrayRef<int64_t> leadingStaticStrides) {
|
|
auto inferredType =
|
|
inferResultType(sourceRankedTensorType, leadingStaticOffsets,
|
|
leadingStaticSizes, leadingStaticStrides)
|
|
.cast<RankedTensorType>();
|
|
int rankDiff = inferredType.getRank() - resultRank;
|
|
if (rankDiff > 0) {
|
|
auto shape = inferredType.getShape();
|
|
llvm::SmallDenseSet<unsigned> dimsToProject;
|
|
mlir::getPositionsOfShapeOne(rankDiff, shape, dimsToProject);
|
|
SmallVector<int64_t> projectedShape;
|
|
for (unsigned pos = 0, e = shape.size(); pos < e; ++pos)
|
|
if (!dimsToProject.contains(pos))
|
|
projectedShape.push_back(shape[pos]);
|
|
inferredType =
|
|
RankedTensorType::get(projectedShape, inferredType.getElementType());
|
|
}
|
|
return inferredType;
|
|
}
|
|
|
|
RankedTensorType ExtractSliceOp::inferRankReducedResultType(
|
|
unsigned resultRank, RankedTensorType sourceRankedTensorType,
|
|
ArrayRef<OpFoldResult> leadingStaticOffsets,
|
|
ArrayRef<OpFoldResult> leadingStaticSizes,
|
|
ArrayRef<OpFoldResult> leadingStaticStrides) {
|
|
SmallVector<int64_t> staticOffsets, staticSizes, staticStrides;
|
|
SmallVector<Value> dynamicOffsets, dynamicSizes, dynamicStrides;
|
|
dispatchIndexOpFoldResults(leadingStaticOffsets, dynamicOffsets,
|
|
staticOffsets, ShapedType::kDynamicStrideOrOffset);
|
|
dispatchIndexOpFoldResults(leadingStaticSizes, dynamicSizes, staticSizes,
|
|
ShapedType::kDynamicSize);
|
|
dispatchIndexOpFoldResults(leadingStaticStrides, dynamicStrides,
|
|
staticStrides, ShapedType::kDynamicStrideOrOffset);
|
|
return ExtractSliceOp::inferRankReducedResultType(
|
|
resultRank, sourceRankedTensorType, staticOffsets, staticSizes,
|
|
staticStrides);
|
|
}
|
|
|
|
/// Build an ExtractSliceOp with mixed static and dynamic entries and custom
|
|
/// result type. If the type passed is nullptr, it is inferred.
|
|
void ExtractSliceOp::build(OpBuilder &b, OperationState &result,
|
|
RankedTensorType resultType, Value source,
|
|
ArrayRef<OpFoldResult> offsets,
|
|
ArrayRef<OpFoldResult> sizes,
|
|
ArrayRef<OpFoldResult> strides,
|
|
ArrayRef<NamedAttribute> attrs) {
|
|
SmallVector<int64_t> staticOffsets, staticSizes, staticStrides;
|
|
SmallVector<Value> dynamicOffsets, dynamicSizes, dynamicStrides;
|
|
dispatchIndexOpFoldResults(offsets, dynamicOffsets, staticOffsets,
|
|
|
|
ShapedType::kDynamicStrideOrOffset);
|
|
dispatchIndexOpFoldResults(sizes, dynamicSizes, staticSizes,
|
|
ShapedType::kDynamicSize);
|
|
dispatchIndexOpFoldResults(strides, dynamicStrides, staticStrides,
|
|
|
|
ShapedType::kDynamicStrideOrOffset);
|
|
auto sourceRankedTensorType = source.getType().cast<RankedTensorType>();
|
|
// Structuring implementation this way avoids duplication between builders.
|
|
if (!resultType) {
|
|
resultType =
|
|
ExtractSliceOp::inferResultType(sourceRankedTensorType, staticOffsets,
|
|
staticSizes, staticStrides)
|
|
.cast<RankedTensorType>();
|
|
}
|
|
build(b, result, resultType, source, dynamicOffsets, dynamicSizes,
|
|
dynamicStrides, b.getI64ArrayAttr(staticOffsets),
|
|
b.getI64ArrayAttr(staticSizes), b.getI64ArrayAttr(staticStrides));
|
|
result.addAttributes(attrs);
|
|
}
|
|
|
|
/// Build an ExtractSliceOp with mixed static and dynamic entries and inferred
|
|
/// result type.
|
|
void ExtractSliceOp::build(OpBuilder &b, OperationState &result, Value source,
|
|
ArrayRef<OpFoldResult> offsets,
|
|
ArrayRef<OpFoldResult> sizes,
|
|
ArrayRef<OpFoldResult> strides,
|
|
ArrayRef<NamedAttribute> attrs) {
|
|
build(b, result, RankedTensorType(), source, offsets, sizes, strides, attrs);
|
|
}
|
|
|
|
/// Build an ExtractSliceOp with dynamic entries and custom result type. If the
|
|
/// type passed is nullptr, it is inferred.
|
|
void ExtractSliceOp::build(OpBuilder &b, OperationState &result,
|
|
RankedTensorType resultType, Value source,
|
|
ValueRange offsets, ValueRange sizes,
|
|
ValueRange strides, ArrayRef<NamedAttribute> attrs) {
|
|
SmallVector<OpFoldResult> offsetValues = llvm::to_vector<4>(
|
|
llvm::map_range(offsets, [](Value v) -> OpFoldResult { return v; }));
|
|
SmallVector<OpFoldResult> sizeValues = llvm::to_vector<4>(
|
|
llvm::map_range(sizes, [](Value v) -> OpFoldResult { return v; }));
|
|
SmallVector<OpFoldResult> strideValues = llvm::to_vector<4>(
|
|
llvm::map_range(strides, [](Value v) -> OpFoldResult { return v; }));
|
|
build(b, result, resultType, source, offsetValues, sizeValues, strideValues);
|
|
}
|
|
|
|
/// Build an ExtractSliceOp with dynamic entries and inferred result type.
|
|
void ExtractSliceOp::build(OpBuilder &b, OperationState &result, Value source,
|
|
ValueRange offsets, ValueRange sizes,
|
|
ValueRange strides, ArrayRef<NamedAttribute> attrs) {
|
|
build(b, result, RankedTensorType(), source, offsets, sizes, strides, attrs);
|
|
}
|
|
|
|
template <typename OpTy>
|
|
static LogicalResult produceSliceErrorMsg(SliceVerificationResult result,
|
|
OpTy op, Type expectedType) {
|
|
auto memrefType = expectedType.cast<ShapedType>();
|
|
switch (result) {
|
|
case SliceVerificationResult::Success:
|
|
return success();
|
|
case SliceVerificationResult::RankTooLarge:
|
|
return op.emitError("expected rank to be smaller or equal to ")
|
|
<< "the other rank. ";
|
|
case SliceVerificationResult::SizeMismatch:
|
|
return op.emitError("expected type to be ")
|
|
<< expectedType << " or a rank-reduced version. (size mismatch) ";
|
|
case SliceVerificationResult::ElemTypeMismatch:
|
|
return op.emitError("expected element type to be ")
|
|
<< memrefType.getElementType();
|
|
default:
|
|
llvm_unreachable("unexpected extract_slice op verification result");
|
|
}
|
|
}
|
|
|
|
/// Verifier for ExtractSliceOp.
|
|
static LogicalResult verify(ExtractSliceOp op) {
|
|
// Verify result type against inferred type.
|
|
auto expectedType =
|
|
ExtractSliceOp::inferResultType(op.getSourceType(), op.getMixedOffsets(),
|
|
op.getMixedSizes(), op.getMixedStrides());
|
|
auto result =
|
|
isRankReducedType(expectedType.cast<ShapedType>(), op.getType());
|
|
return produceSliceErrorMsg(result, op, expectedType);
|
|
}
|
|
|
|
/// Infer the canonical type of the result of an extract_slice op. Returns a
|
|
/// type with rank `resultRank` that is either the rank of the rank-reduced
|
|
/// type, or the non-rank-reduced type.
|
|
static RankedTensorType
|
|
getCanonicalSliceResultType(unsigned resultRank, RankedTensorType sourceType,
|
|
ArrayRef<OpFoldResult> mixedOffsets,
|
|
ArrayRef<OpFoldResult> mixedSizes,
|
|
ArrayRef<OpFoldResult> mixedStrides) {
|
|
auto resultType =
|
|
ExtractSliceOp::inferRankReducedResultType(
|
|
resultRank, sourceType, mixedOffsets, mixedSizes, mixedStrides)
|
|
.cast<RankedTensorType>();
|
|
if (resultType.getRank() != resultRank) {
|
|
resultType = ExtractSliceOp::inferResultType(sourceType, mixedOffsets,
|
|
mixedSizes, mixedStrides)
|
|
.cast<RankedTensorType>();
|
|
}
|
|
return resultType;
|
|
}
|
|
|
|
llvm::SmallDenseSet<unsigned> ExtractSliceOp::getDroppedDims() {
|
|
llvm::SmallDenseSet<unsigned> droppedDims;
|
|
ArrayRef<int64_t> resultShape = getType().getShape();
|
|
SmallVector<OpFoldResult> mixedSizes = getMixedSizes();
|
|
unsigned shapePos = 0;
|
|
for (auto size : enumerate(mixedSizes)) {
|
|
Optional<int64_t> sizeVal = getConstantIntValue(size.value());
|
|
// If the size is not 1, or if the current matched dimension of the result
|
|
// is the same static shape as the size value (which is 1), then the
|
|
// dimension is preserved.
|
|
if (!sizeVal || sizeVal.getValue() != 1 ||
|
|
(shapePos < resultShape.size() && resultShape[shapePos] == 1)) {
|
|
shapePos++;
|
|
continue;
|
|
}
|
|
droppedDims.insert(size.index());
|
|
}
|
|
return droppedDims;
|
|
}
|
|
|
|
LogicalResult ExtractSliceOp::reifyResultShapes(
|
|
OpBuilder &builder, ReifiedRankedShapedTypeDims &reifiedReturnShapes) {
|
|
reifiedReturnShapes.resize(1);
|
|
reifiedReturnShapes[0].reserve(getType().getRank());
|
|
SmallVector<OpFoldResult> mixedSizes = getMixedSizes();
|
|
llvm::SmallDenseSet<unsigned> droppedDims = getDroppedDims();
|
|
Location loc = getLoc();
|
|
for (auto size : enumerate(mixedSizes)) {
|
|
if (droppedDims.count(size.index()))
|
|
continue;
|
|
if (auto attr = size.value().dyn_cast<Attribute>()) {
|
|
reifiedReturnShapes[0].push_back(builder.create<arith::ConstantIndexOp>(
|
|
loc, attr.cast<IntegerAttr>().getInt()));
|
|
continue;
|
|
}
|
|
reifiedReturnShapes[0].push_back(size.value().get<Value>());
|
|
}
|
|
return success();
|
|
}
|
|
|
|
namespace {
|
|
/// Pattern to rewrite an extract_slice op with tensor::Cast arguments.
|
|
/// This essentially pushes memref_cast past its consuming slice when
|
|
/// `canFoldIntoConsumerOp` is true.
|
|
///
|
|
/// Example:
|
|
/// ```
|
|
/// %0 = tensor.cast %V : tensor<16x16xf32> to tensor<?x?xf32>
|
|
/// %1 = tensor.extract_slice %0[0, 0][3, 4][1, 1] : tensor<?x?xf32> to
|
|
/// tensor<3x4xf32>
|
|
/// ```
|
|
/// is rewritten into:
|
|
/// ```
|
|
/// %0 = tensor.extract_slice %V[0, 0][3, 4][1, 1] : tensor<16x16xf32> to
|
|
/// tensor<3x4xf32> %1 = tensor.cast %0: tensor<3x4xf32> to tensor<3x4xf32>
|
|
/// ```
|
|
class ExtractSliceOpCastFolder final : public OpRewritePattern<ExtractSliceOp> {
|
|
public:
|
|
using OpRewritePattern<ExtractSliceOp>::OpRewritePattern;
|
|
|
|
LogicalResult matchAndRewrite(ExtractSliceOp sliceOp,
|
|
PatternRewriter &rewriter) const override {
|
|
// Any constant operand, just return to let SubViewOpConstantFolder kick in.
|
|
if (llvm::any_of(sliceOp.getOperands(), [](Value operand) {
|
|
return matchPattern(operand, matchConstantIndex());
|
|
}))
|
|
return failure();
|
|
|
|
auto castOp = sliceOp.source().getDefiningOp<tensor::CastOp>();
|
|
if (!castOp)
|
|
return failure();
|
|
|
|
if (!canFoldIntoConsumerOp(castOp))
|
|
return failure();
|
|
|
|
/// Deduce the type of the result to use for the canonicalized operation.
|
|
RankedTensorType resultType = getCanonicalSliceResultType(
|
|
sliceOp.getType().getRank(), sliceOp.getSourceType(),
|
|
sliceOp.getMixedOffsets(), sliceOp.getMixedSizes(),
|
|
sliceOp.getMixedStrides());
|
|
Value newSlice = rewriter.create<ExtractSliceOp>(
|
|
sliceOp.getLoc(), resultType, castOp.source(), sliceOp.offsets(),
|
|
sliceOp.sizes(), sliceOp.strides(), sliceOp.static_offsets(),
|
|
sliceOp.static_sizes(), sliceOp.static_strides());
|
|
rewriter.replaceOpWithNewOp<tensor::CastOp>(sliceOp, sliceOp.getType(),
|
|
newSlice);
|
|
return success();
|
|
}
|
|
};
|
|
} // namespace
|
|
|
|
/// Return the canonical type of the result of an extract_slice op.
|
|
struct SliceReturnTypeCanonicalizer {
|
|
RankedTensorType operator()(ExtractSliceOp op,
|
|
ArrayRef<OpFoldResult> mixedOffsets,
|
|
ArrayRef<OpFoldResult> mixedSizes,
|
|
ArrayRef<OpFoldResult> mixedStrides) {
|
|
return getCanonicalSliceResultType(op.getType().getRank(),
|
|
op.getSourceType(), mixedOffsets,
|
|
mixedSizes, mixedStrides);
|
|
}
|
|
};
|
|
|
|
/// A canonicalizer wrapper to replace ExtractSliceOps.
|
|
struct SliceCanonicalizer {
|
|
void operator()(PatternRewriter &rewriter, ExtractSliceOp op,
|
|
ExtractSliceOp newOp) {
|
|
Value replacement = newOp.getResult();
|
|
if (replacement.getType() != op.getType())
|
|
replacement = rewriter.create<tensor::CastOp>(op.getLoc(), op.getType(),
|
|
replacement);
|
|
rewriter.replaceOp(op, replacement);
|
|
}
|
|
};
|
|
|
|
void ExtractSliceOp::getCanonicalizationPatterns(RewritePatternSet &results,
|
|
MLIRContext *context) {
|
|
results.add<
|
|
OpWithOffsetSizesAndStridesConstantArgumentFolder<
|
|
ExtractSliceOp, SliceReturnTypeCanonicalizer, SliceCanonicalizer>,
|
|
ExtractSliceOpCastFolder>(context);
|
|
}
|
|
|
|
//
|
|
static LogicalResult
|
|
foldIdentityOffsetSizeAndStrideOpInterface(OffsetSizeAndStrideOpInterface op,
|
|
ShapedType shapedType) {
|
|
OpBuilder b(op.getContext());
|
|
for (OpFoldResult ofr : op.getMixedOffsets())
|
|
if (getConstantIntValue(ofr) != static_cast<int64_t>(0))
|
|
return failure();
|
|
// Rank-reducing noops only need to inspect the leading dimensions: llvm::zip
|
|
// is appropriate.
|
|
auto shape = shapedType.getShape();
|
|
for (auto it : llvm::zip(op.getMixedSizes(), shape))
|
|
if (getConstantIntValue(std::get<0>(it)) != std::get<1>(it))
|
|
return failure();
|
|
for (OpFoldResult ofr : op.getMixedStrides())
|
|
if (getConstantIntValue(ofr) != static_cast<int64_t>(1))
|
|
return failure();
|
|
return success();
|
|
}
|
|
|
|
/// If we have an ExtractSliceOp consuming an InsertSliceOp with the same slice,
|
|
/// we can return the InsertSliceOp's source directly.
|
|
// TODO: This only checks the immediate producer; extend to go up the
|
|
// insert/extract chain if the slices are disjoint.
|
|
static Value foldExtractAfterInsertSlice(ExtractSliceOp extractOp) {
|
|
auto insertOp = extractOp.source().getDefiningOp<InsertSliceOp>();
|
|
|
|
auto isSame = [](OpFoldResult a, OpFoldResult b) { return a == b; };
|
|
if (insertOp && insertOp.source().getType() == extractOp.getType() &&
|
|
insertOp.isSameAs(extractOp, isSame))
|
|
return insertOp.source();
|
|
|
|
return {};
|
|
}
|
|
|
|
OpFoldResult ExtractSliceOp::fold(ArrayRef<Attribute>) {
|
|
if (getSourceType() == getType() &&
|
|
succeeded(foldIdentityOffsetSizeAndStrideOpInterface(*this, getType())))
|
|
return this->source();
|
|
if (Value slice = foldExtractAfterInsertSlice(*this))
|
|
return slice;
|
|
return OpFoldResult();
|
|
}
|
|
|
|
Value mlir::tensor::createCanonicalRankReducingExtractSliceOp(
|
|
OpBuilder &b, Location loc, Value tensor, RankedTensorType targetType) {
|
|
auto rankedTensorType = tensor.getType().cast<RankedTensorType>();
|
|
unsigned rank = rankedTensorType.getRank();
|
|
auto shape = rankedTensorType.getShape();
|
|
SmallVector<OpFoldResult> offsets(rank, b.getIndexAttr(0));
|
|
SmallVector<OpFoldResult> sizes;
|
|
for (unsigned i = 0, e = rank; i < e; ++i) {
|
|
OpFoldResult dim;
|
|
if (rankedTensorType.isDynamicDim(i))
|
|
dim = b.createOrFold<tensor::DimOp>(
|
|
loc, tensor, b.create<arith::ConstantIndexOp>(loc, i));
|
|
else
|
|
dim = b.getIndexAttr(shape[i]);
|
|
sizes.push_back(dim);
|
|
}
|
|
SmallVector<OpFoldResult> strides(rank, b.getIndexAttr(1));
|
|
return b.createOrFold<tensor::ExtractSliceOp>(loc, targetType, tensor,
|
|
offsets, sizes, strides);
|
|
}
|
|
|
|
//===----------------------------------------------------------------------===//
|
|
// InsertSliceOp
|
|
//===----------------------------------------------------------------------===//
|
|
|
|
// Build a InsertSliceOp with mixed static and dynamic entries.
|
|
void InsertSliceOp::build(OpBuilder &b, OperationState &result, Value source,
|
|
Value dest, ArrayRef<OpFoldResult> offsets,
|
|
ArrayRef<OpFoldResult> sizes,
|
|
ArrayRef<OpFoldResult> strides,
|
|
ArrayRef<NamedAttribute> attrs) {
|
|
SmallVector<int64_t> staticOffsets, staticSizes, staticStrides;
|
|
SmallVector<Value> dynamicOffsets, dynamicSizes, dynamicStrides;
|
|
dispatchIndexOpFoldResults(offsets, dynamicOffsets, staticOffsets,
|
|
|
|
ShapedType::kDynamicStrideOrOffset);
|
|
dispatchIndexOpFoldResults(sizes, dynamicSizes, staticSizes,
|
|
ShapedType::kDynamicSize);
|
|
dispatchIndexOpFoldResults(strides, dynamicStrides, staticStrides,
|
|
|
|
ShapedType::kDynamicStrideOrOffset);
|
|
build(b, result, dest.getType(), source, dest, dynamicOffsets, dynamicSizes,
|
|
dynamicStrides, b.getI64ArrayAttr(staticOffsets),
|
|
b.getI64ArrayAttr(staticSizes), b.getI64ArrayAttr(staticStrides));
|
|
result.addAttributes(attrs);
|
|
}
|
|
|
|
// Build a InsertSliceOp with dynamic entries.
|
|
void InsertSliceOp::build(OpBuilder &b, OperationState &result, Value source,
|
|
Value dest, ValueRange offsets, ValueRange sizes,
|
|
ValueRange strides, ArrayRef<NamedAttribute> attrs) {
|
|
SmallVector<OpFoldResult> offsetValues = llvm::to_vector<4>(
|
|
llvm::map_range(offsets, [](Value v) -> OpFoldResult { return v; }));
|
|
SmallVector<OpFoldResult> sizeValues = llvm::to_vector<4>(
|
|
llvm::map_range(sizes, [](Value v) -> OpFoldResult { return v; }));
|
|
SmallVector<OpFoldResult> strideValues = llvm::to_vector<4>(
|
|
llvm::map_range(strides, [](Value v) -> OpFoldResult { return v; }));
|
|
build(b, result, source, dest, offsetValues, sizeValues, strideValues);
|
|
}
|
|
|
|
/// Verifier for InsertSliceOp.
|
|
static LogicalResult verify(InsertSliceOp op) {
|
|
// insert_slice is the inverse of extract_slice, use the same type inference.
|
|
auto expectedType = ExtractSliceOp::inferRankReducedResultType(
|
|
op.getSourceType().getRank(), op.getType(),
|
|
extractFromI64ArrayAttr(op.static_offsets()),
|
|
extractFromI64ArrayAttr(op.static_sizes()),
|
|
extractFromI64ArrayAttr(op.static_strides()));
|
|
auto result =
|
|
isRankReducedType(expectedType.cast<ShapedType>(), op.getSourceType());
|
|
return produceSliceErrorMsg(result, op, expectedType);
|
|
}
|
|
|
|
/// If we have two consecutive InsertSliceOp writing to the same slice, we
|
|
/// can mutate the second InsertSliceOp's destination to the first one's.
|
|
///
|
|
/// Example:
|
|
///
|
|
/// ```mlir
|
|
/// %0 = tensor.insert_slice %slice0 into %input[0, 0] [64, 64] [1, 1]
|
|
/// %1 = tensor.insert_slice %slice1 into %0[0, 0] [64, 64] [1, 1]
|
|
/// ```
|
|
///
|
|
/// folds into:
|
|
///
|
|
/// ```mlir
|
|
/// %1 = tensor.insert_slice %slice1 into %input[0, 0] [64, 64] [1, 1]
|
|
/// ```
|
|
static LogicalResult foldInsertAfterInsertSlice(InsertSliceOp insertOp) {
|
|
auto prevInsertOp = insertOp.dest().getDefiningOp<InsertSliceOp>();
|
|
|
|
auto isSame = [](OpFoldResult a, OpFoldResult b) { return a == b; };
|
|
if (!prevInsertOp ||
|
|
prevInsertOp.source().getType() != insertOp.source().getType() ||
|
|
!prevInsertOp.isSameAs(insertOp, isSame))
|
|
return failure();
|
|
|
|
insertOp.destMutable().assign(prevInsertOp.dest());
|
|
return success();
|
|
}
|
|
|
|
OpFoldResult InsertSliceOp::fold(ArrayRef<Attribute>) {
|
|
if (getSourceType().hasStaticShape() && getType().hasStaticShape() &&
|
|
getSourceType() == getType() &&
|
|
succeeded(foldIdentityOffsetSizeAndStrideOpInterface(*this, getType())))
|
|
return this->source();
|
|
if (succeeded(foldInsertAfterInsertSlice(*this)))
|
|
return getResult();
|
|
return OpFoldResult();
|
|
}
|
|
|
|
LogicalResult InsertSliceOp::reifyResultShapes(
|
|
OpBuilder &builder, ReifiedRankedShapedTypeDims &reifiedReturnShapes) {
|
|
reifiedReturnShapes.resize(1, SmallVector<Value>(getType().getRank()));
|
|
for (auto dim : llvm::seq<int64_t>(0, getType().getRank())) {
|
|
reifiedReturnShapes[0][dim] =
|
|
builder.createOrFold<tensor::DimOp>(getLoc(), dest(), dim);
|
|
}
|
|
return success();
|
|
}
|
|
|
|
namespace {
|
|
/// Pattern to rewrite a insert_slice op with constant arguments.
|
|
class InsertSliceOpConstantArgumentFolder final
|
|
: public OpRewritePattern<InsertSliceOp> {
|
|
public:
|
|
using OpRewritePattern<InsertSliceOp>::OpRewritePattern;
|
|
|
|
LogicalResult matchAndRewrite(InsertSliceOp insertSliceOp,
|
|
PatternRewriter &rewriter) const override {
|
|
// No constant operand, just return.
|
|
if (llvm::none_of(insertSliceOp.getOperands(), [](Value operand) {
|
|
return matchPattern(operand, matchConstantIndex());
|
|
}))
|
|
return failure();
|
|
|
|
// At least one of offsets/sizes/strides is a new constant.
|
|
// Form the new list of operands and constant attributes from the
|
|
// existing.
|
|
SmallVector<OpFoldResult> mixedOffsets(insertSliceOp.getMixedOffsets());
|
|
SmallVector<OpFoldResult> mixedSizes(insertSliceOp.getMixedSizes());
|
|
SmallVector<OpFoldResult> mixedStrides(insertSliceOp.getMixedStrides());
|
|
canonicalizeSubViewPart(mixedOffsets, ShapedType::isDynamicStrideOrOffset);
|
|
canonicalizeSubViewPart(mixedSizes, ShapedType::isDynamic);
|
|
canonicalizeSubViewPart(mixedStrides, ShapedType::isDynamicStrideOrOffset);
|
|
|
|
// Create the new op in canonical form.
|
|
auto sourceType = ExtractSliceOp::inferRankReducedResultType(
|
|
insertSliceOp.getSourceType().getRank(), insertSliceOp.getType(),
|
|
mixedOffsets, mixedSizes, mixedStrides);
|
|
Value toInsert = insertSliceOp.source();
|
|
if (sourceType != insertSliceOp.getSourceType())
|
|
toInsert = rewriter.create<tensor::CastOp>(insertSliceOp.getLoc(),
|
|
sourceType, toInsert);
|
|
rewriter.replaceOpWithNewOp<InsertSliceOp>(
|
|
insertSliceOp, toInsert, insertSliceOp.dest(), mixedOffsets, mixedSizes,
|
|
mixedStrides);
|
|
return success();
|
|
}
|
|
};
|
|
|
|
/// Fold tensor_casts with insert_slice operations. If the source or destination
|
|
/// tensor is a tensor_cast that removes static type information, the cast is
|
|
/// folded into the insert_slice operation. E.g.:
|
|
///
|
|
/// ```mlir
|
|
/// %1 = tensor.cast %0 : tensor<8x16xf32> to tensor<?x?xf32>
|
|
/// %2 = tensor.insert_slice %1 into ... : tensor<?x?xf32> into ...
|
|
/// ```
|
|
///
|
|
/// folds into:
|
|
///
|
|
/// ```mlir
|
|
/// %2 = tensor.insert_slice %0 into ... : tensor<8x16xf32> into ...
|
|
/// ```
|
|
///
|
|
/// Note: When folding a cast on the destination tensor, the result of the
|
|
/// insert_slice operation is casted to ensure that the type of the result did
|
|
/// not change.
|
|
struct InsertSliceOpCastFolder final : public OpRewritePattern<InsertSliceOp> {
|
|
using OpRewritePattern<InsertSliceOp>::OpRewritePattern;
|
|
|
|
LogicalResult matchAndRewrite(InsertSliceOp insertSliceOp,
|
|
PatternRewriter &rewriter) const override {
|
|
if (llvm::any_of(insertSliceOp.getOperands(), [](Value operand) {
|
|
return matchPattern(operand, matchConstantIndex());
|
|
}))
|
|
return failure();
|
|
|
|
auto getSourceOfCastOp = [](Value v) -> Optional<Value> {
|
|
auto castOp = v.getDefiningOp<tensor::CastOp>();
|
|
if (!castOp || !canFoldIntoConsumerOp(castOp))
|
|
return llvm::None;
|
|
return castOp.source();
|
|
};
|
|
Optional<Value> sourceCastSource =
|
|
getSourceOfCastOp(insertSliceOp.source());
|
|
Optional<Value> destCastSource = getSourceOfCastOp(insertSliceOp.dest());
|
|
if (!sourceCastSource && !destCastSource)
|
|
return failure();
|
|
|
|
Value replacement = rewriter.create<InsertSliceOp>(
|
|
insertSliceOp.getLoc(),
|
|
(sourceCastSource ? *sourceCastSource : insertSliceOp.source()),
|
|
(destCastSource ? *destCastSource : insertSliceOp.dest()),
|
|
insertSliceOp.getMixedOffsets(), insertSliceOp.getMixedSizes(),
|
|
insertSliceOp.getMixedStrides());
|
|
|
|
if (replacement.getType() != insertSliceOp.getType()) {
|
|
replacement = rewriter.create<tensor::CastOp>(
|
|
insertSliceOp.getLoc(), insertSliceOp.getType(), replacement);
|
|
}
|
|
rewriter.replaceOp(insertSliceOp, replacement);
|
|
return success();
|
|
}
|
|
};
|
|
|
|
/// If additional static type information can be deduced from a insert_slice's
|
|
/// size operands, insert an explicit cast of the op's source operand. This
|
|
/// enables other canonicalization patterns that are matching for tensor_cast
|
|
/// ops such as `ForOpTensorCastFolder` in SCF.
|
|
///
|
|
/// Example:
|
|
///
|
|
/// ```mlir
|
|
/// %r = tensor.insert_slice %0 into %1[...] [64, 64] [1, 1]
|
|
/// : tensor<?x?xf32> into ...
|
|
/// ```
|
|
///
|
|
/// folds into:
|
|
///
|
|
/// ```mlir
|
|
/// %tmp = tensor.cast %0 : tensor<?x?xf32> to tensor<64x64xf32>
|
|
/// %r = tensor.insert_slice %tmp into %1[...] [64, 64] [1, 1]
|
|
/// : tensor<64x64xf32> into ...
|
|
/// ```
|
|
struct InsertSliceOpSourceCastInserter final
|
|
: public OpRewritePattern<InsertSliceOp> {
|
|
using OpRewritePattern<InsertSliceOp>::OpRewritePattern;
|
|
|
|
LogicalResult matchAndRewrite(InsertSliceOp insertSliceOp,
|
|
PatternRewriter &rewriter) const override {
|
|
RankedTensorType srcType = insertSliceOp.getSourceType();
|
|
if (srcType.getRank() != insertSliceOp.getType().getRank())
|
|
return failure();
|
|
SmallVector<int64_t> newSrcShape(srcType.getShape().begin(),
|
|
srcType.getShape().end());
|
|
for (int64_t i = 0; i < srcType.getRank(); ++i) {
|
|
if (Optional<int64_t> constInt =
|
|
getConstantIntValue(insertSliceOp.getMixedSizes()[i]))
|
|
newSrcShape[i] = *constInt;
|
|
}
|
|
|
|
RankedTensorType newSrcType =
|
|
RankedTensorType::get(newSrcShape, srcType.getElementType());
|
|
if (srcType == newSrcType ||
|
|
!preservesStaticInformation(srcType, newSrcType) ||
|
|
!tensor::CastOp::areCastCompatible(srcType, newSrcType))
|
|
return failure();
|
|
|
|
// newSrcType is:
|
|
// 1) Different from srcType.
|
|
// 2) "More static" than srcType.
|
|
// 3) Cast-compatible with srcType.
|
|
// Insert the cast.
|
|
Value cast = rewriter.create<tensor::CastOp>(
|
|
insertSliceOp.getLoc(), newSrcType, insertSliceOp.source());
|
|
rewriter.replaceOpWithNewOp<InsertSliceOp>(
|
|
insertSliceOp, cast, insertSliceOp.dest(),
|
|
insertSliceOp.getMixedOffsets(), insertSliceOp.getMixedSizes(),
|
|
insertSliceOp.getMixedStrides());
|
|
return success();
|
|
}
|
|
};
|
|
} // namespace
|
|
|
|
void InsertSliceOp::getCanonicalizationPatterns(RewritePatternSet &results,
|
|
MLIRContext *context) {
|
|
results.add<InsertSliceOpConstantArgumentFolder, InsertSliceOpCastFolder,
|
|
InsertSliceOpSourceCastInserter>(context);
|
|
}
|
|
|
|
Value mlir::tensor::createCanonicalRankReducingInsertSliceOp(OpBuilder &b,
|
|
Location loc,
|
|
Value tensor,
|
|
Value dest) {
|
|
auto rankedTensorType = dest.getType().cast<RankedTensorType>();
|
|
unsigned rank = rankedTensorType.getRank();
|
|
auto shape = rankedTensorType.getShape();
|
|
SmallVector<OpFoldResult> offsets(rank, b.getIndexAttr(0));
|
|
SmallVector<OpFoldResult> sizes;
|
|
for (unsigned i = 0, e = rank; i < e; ++i) {
|
|
OpFoldResult dim;
|
|
if (rankedTensorType.isDynamicDim(i))
|
|
dim = b.createOrFold<tensor::DimOp>(
|
|
loc, dest, b.create<arith::ConstantIndexOp>(loc, i));
|
|
else
|
|
dim = b.getIndexAttr(shape[i]);
|
|
sizes.push_back(dim);
|
|
}
|
|
SmallVector<OpFoldResult> strides(rank, b.getIndexAttr(1));
|
|
return b.createOrFold<tensor::InsertSliceOp>(loc, tensor, dest, offsets,
|
|
sizes, strides);
|
|
}
|
|
|
|
//===----------------------------------------------------------------------===//
|
|
// TableGen'd op method definitions
|
|
//===----------------------------------------------------------------------===//
|
|
|
|
#define GET_OP_CLASSES
|
|
#include "mlir/Dialect/Tensor/IR/TensorOps.cpp.inc"
|