mirror of
https://github.com/llvm/llvm-project.git
synced 2025-05-01 11:06:06 +00:00
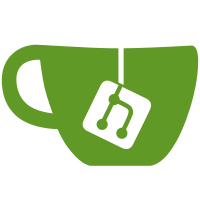
From the beginning, vector_transfer_read and vector_transfer_write opreations were intended as a mid-level vectorization abstraction. In particular, they are lowered to the StandardOps dialect before further processing. As such, it does not make sense to keep them at the same level as StandardOps. Introduce the new SuperVectorOps dialect and move vector_transfer_* operations there. This will be used as a testbed for the generic lowering/legalization pass. PiperOrigin-RevId: 225554492
247 lines
9.6 KiB
C++
247 lines
9.6 KiB
C++
//===- VectorAnalysis.cpp - Analysis for Vectorization --------------------===//
|
|
//
|
|
// Copyright 2019 The MLIR Authors.
|
|
//
|
|
// Licensed under the Apache License, Version 2.0 (the "License");
|
|
// you may not use this file except in compliance with the License.
|
|
// You may obtain a copy of the License at
|
|
//
|
|
// http://www.apache.org/licenses/LICENSE-2.0
|
|
//
|
|
// Unless required by applicable law or agreed to in writing, software
|
|
// distributed under the License is distributed on an "AS IS" BASIS,
|
|
// WITHOUT WARRANTIES OR CONDITIONS OF ANY KIND, either express or implied.
|
|
// See the License for the specific language governing permissions and
|
|
// limitations under the License.
|
|
// =============================================================================
|
|
|
|
#include "mlir/Analysis/VectorAnalysis.h"
|
|
#include "mlir/Analysis/LoopAnalysis.h"
|
|
#include "mlir/IR/Builders.h"
|
|
#include "mlir/IR/BuiltinOps.h"
|
|
#include "mlir/IR/Statements.h"
|
|
#include "mlir/StandardOps/StandardOps.h"
|
|
#include "mlir/SuperVectorOps/SuperVectorOps.h"
|
|
#include "mlir/Support/Functional.h"
|
|
#include "mlir/Support/STLExtras.h"
|
|
|
|
#include "llvm/ADT/DenseSet.h"
|
|
#include "llvm/ADT/SetVector.h"
|
|
|
|
///
|
|
/// Implements Analysis functions specific to vectors which support
|
|
/// the vectorization and vectorization materialization passes.
|
|
///
|
|
|
|
using namespace mlir;
|
|
|
|
using llvm::SetVector;
|
|
|
|
Optional<SmallVector<unsigned, 4>> mlir::shapeRatio(ArrayRef<int> superShape,
|
|
ArrayRef<int> subShape) {
|
|
if (superShape.size() < subShape.size()) {
|
|
return Optional<SmallVector<unsigned, 4>>();
|
|
}
|
|
|
|
// Starting from the end, compute the integer divisors.
|
|
// Set the boolean `divides` if integral division is not possible.
|
|
std::vector<unsigned> result;
|
|
result.reserve(superShape.size());
|
|
bool divides = true;
|
|
auto divide = [÷s, &result](int superSize, int subSize) {
|
|
assert(superSize > 0 && "superSize must be > 0");
|
|
assert(subSize > 0 && "subSize must be > 0");
|
|
divides &= (superSize % subSize == 0);
|
|
result.push_back(superSize / subSize);
|
|
};
|
|
functional::zipApply(
|
|
divide, SmallVector<int, 8>{superShape.rbegin(), superShape.rend()},
|
|
SmallVector<int, 8>{subShape.rbegin(), subShape.rend()});
|
|
|
|
// If integral division does not occur, return and let the caller decide.
|
|
if (!divides) {
|
|
return None;
|
|
}
|
|
|
|
// At this point we computed the ratio (in reverse) for the common
|
|
// size. Fill with the remaining entries from the super-vector shape (still in
|
|
// reverse).
|
|
int commonSize = subShape.size();
|
|
std::copy(superShape.rbegin() + commonSize, superShape.rend(),
|
|
std::back_inserter(result));
|
|
|
|
assert(result.size() == superShape.size() &&
|
|
"super to sub shape ratio is not of the same size as the super rank");
|
|
|
|
// Reverse again to get it back in the proper order and return.
|
|
return SmallVector<unsigned, 4>{result.rbegin(), result.rend()};
|
|
}
|
|
|
|
Optional<SmallVector<unsigned, 4>> mlir::shapeRatio(VectorType superVectorType,
|
|
VectorType subVectorType) {
|
|
assert(superVectorType.getElementType() == subVectorType.getElementType() &&
|
|
"vector types must be of the same elemental type");
|
|
return shapeRatio(superVectorType.getShape(), subVectorType.getShape());
|
|
}
|
|
|
|
/// Constructs a permutation map from memref indices to vector dimension.
|
|
///
|
|
/// The implementation uses the knowledge of the mapping of enclosing loop to
|
|
/// vector dimension. `enclosingLoopToVectorDim` carries this information as a
|
|
/// map with:
|
|
/// - keys representing "vectorized enclosing loops";
|
|
/// - values representing the corresponding vector dimension.
|
|
/// The algorithm traverses "vectorized enclosing loops" and extracts the
|
|
/// at-most-one MemRef index that is invariant along said loop. This index is
|
|
/// guaranteed to be at most one by construction: otherwise the MemRef is not
|
|
/// vectorizable.
|
|
/// If this invariant index is found, it is added to the permutation_map at the
|
|
/// proper vector dimension.
|
|
/// If no index is found to be invariant, 0 is added to the permutation_map and
|
|
/// corresponds to a vector broadcast along that dimension.
|
|
///
|
|
/// Examples can be found in the documentation of `makePermutationMap`, in the
|
|
/// header file.
|
|
static AffineMap makePermutationMap(
|
|
MLIRContext *context,
|
|
llvm::iterator_range<Operation::operand_iterator> indices,
|
|
const DenseMap<ForStmt *, unsigned> &enclosingLoopToVectorDim) {
|
|
using functional::makePtrDynCaster;
|
|
using functional::map;
|
|
auto unwrappedIndices = map(makePtrDynCaster<SSAValue, MLValue>(), indices);
|
|
SmallVector<AffineExpr, 4> perm(enclosingLoopToVectorDim.size(),
|
|
getAffineConstantExpr(0, context));
|
|
for (auto kvp : enclosingLoopToVectorDim) {
|
|
assert(kvp.second < perm.size());
|
|
auto invariants = getInvariantAccesses(*kvp.first, unwrappedIndices);
|
|
unsigned numIndices = unwrappedIndices.size();
|
|
unsigned countInvariantIndices = 0;
|
|
for (unsigned dim = 0; dim < numIndices; ++dim) {
|
|
if (!invariants.count(unwrappedIndices[dim])) {
|
|
assert(perm[kvp.second] == getAffineConstantExpr(0, context) &&
|
|
"permutationMap already has an entry along dim");
|
|
perm[kvp.second] = getAffineDimExpr(dim, context);
|
|
} else {
|
|
++countInvariantIndices;
|
|
}
|
|
}
|
|
assert((countInvariantIndices == numIndices ||
|
|
countInvariantIndices == numIndices - 1) &&
|
|
"Vectorization prerequisite violated: at most 1 index may be "
|
|
"invariant wrt a vectorized loop");
|
|
}
|
|
return AffineMap::get(unwrappedIndices.size(), 0, perm, {});
|
|
}
|
|
|
|
/// Implementation detail that walks up the parents and records the ones with
|
|
/// the specified type.
|
|
/// TODO(ntv): could also be implemented as a collect parents followed by a
|
|
/// filter and made available outside this file.
|
|
template <typename T> static SetVector<T *> getParentsOfType(Statement *stmt) {
|
|
SetVector<T *> res;
|
|
auto *current = stmt;
|
|
while (auto *parent = current->getParentStmt()) {
|
|
auto *typedParent = dyn_cast<T>(parent);
|
|
if (typedParent) {
|
|
assert(res.count(typedParent) == 0 && "Already inserted");
|
|
res.insert(typedParent);
|
|
}
|
|
current = parent;
|
|
}
|
|
return res;
|
|
}
|
|
|
|
/// Returns the enclosing ForStmt, from closest to farthest.
|
|
static SetVector<ForStmt *> getEnclosingForStmts(Statement *stmt) {
|
|
return getParentsOfType<ForStmt>(stmt);
|
|
}
|
|
|
|
AffineMap
|
|
mlir::makePermutationMap(OperationStmt *opStmt,
|
|
const DenseMap<ForStmt *, unsigned> &loopToVectorDim) {
|
|
DenseMap<ForStmt *, unsigned> enclosingLoopToVectorDim;
|
|
auto enclosingLoops = getEnclosingForStmts(opStmt);
|
|
for (auto *forStmt : enclosingLoops) {
|
|
auto it = loopToVectorDim.find(forStmt);
|
|
if (it != loopToVectorDim.end()) {
|
|
enclosingLoopToVectorDim.insert(*it);
|
|
}
|
|
}
|
|
|
|
if (auto load = opStmt->dyn_cast<LoadOp>()) {
|
|
return ::makePermutationMap(opStmt->getContext(), load->getIndices(),
|
|
enclosingLoopToVectorDim);
|
|
}
|
|
|
|
auto store = opStmt->cast<StoreOp>();
|
|
return ::makePermutationMap(opStmt->getContext(), store->getIndices(),
|
|
enclosingLoopToVectorDim);
|
|
}
|
|
|
|
bool mlir::matcher::operatesOnStrictSuperVectors(const OperationStmt &opStmt,
|
|
VectorType subVectorType) {
|
|
// First, extract the vector type and ditinguish between:
|
|
// a. ops that *must* lower a super-vector (i.e. vector_transfer_read,
|
|
// vector_transfer_write); and
|
|
// b. ops that *may* lower a super-vector (all other ops).
|
|
// The ops that *may* lower a super-vector only do so if the super-vector to
|
|
// sub-vector ratio is striclty greater than 1. The ops that *must* lower a
|
|
// super-vector are explicitly checked for this property.
|
|
/// TODO(ntv): there should be a single function for all ops to do this so we
|
|
/// do not have to special case. Maybe a trait, or just a method, unclear atm.
|
|
bool mustDivide = false;
|
|
VectorType superVectorType;
|
|
if (auto read = opStmt.dyn_cast<VectorTransferReadOp>()) {
|
|
superVectorType = read->getResultType();
|
|
mustDivide = true;
|
|
} else if (auto write = opStmt.dyn_cast<VectorTransferWriteOp>()) {
|
|
superVectorType = write->getVectorType();
|
|
mustDivide = true;
|
|
} else if (opStmt.getNumResults() == 0) {
|
|
if (!opStmt.isa<ReturnOp>()) {
|
|
opStmt.emitError("NYI: assuming only return statements can have 0 "
|
|
" results at this point");
|
|
}
|
|
return false;
|
|
} else if (opStmt.getNumResults() == 1) {
|
|
if (auto v = opStmt.getResult(0)->getType().dyn_cast<VectorType>()) {
|
|
superVectorType = v;
|
|
} else {
|
|
// Not a vector type.
|
|
return false;
|
|
}
|
|
} else {
|
|
// Not a vector_transfer and has more than 1 result, fail hard for now to
|
|
// wake us up when something changes.
|
|
opStmt.emitError("NYI: statement has more than 1 result");
|
|
return false;
|
|
}
|
|
|
|
// Get the ratio.
|
|
auto ratio = shapeRatio(superVectorType, subVectorType);
|
|
|
|
// Sanity check.
|
|
assert((ratio.hasValue() || !mustDivide) &&
|
|
"vector_transfer instruction in which super-vector size is not an"
|
|
" integer multiple of sub-vector size");
|
|
|
|
// This catches cases that are not strictly necessary to have multiplicity but
|
|
// still aren't divisible by the sub-vector shape.
|
|
// This could be useful information if we wanted to reshape at the level of
|
|
// the vector type (but we would have to look at the compute and distinguish
|
|
// between parallel, reduction and possibly other cases.
|
|
if (!ratio.hasValue()) {
|
|
return false;
|
|
}
|
|
|
|
// A strict super-vector is at least 2 sub-vectors.
|
|
for (auto m : *ratio) {
|
|
if (m > 1) {
|
|
return true;
|
|
}
|
|
}
|
|
|
|
// Not a strict super-vector.
|
|
return false;
|
|
}
|