mirror of
https://github.com/llvm/llvm-project.git
synced 2025-05-05 00:06:06 +00:00
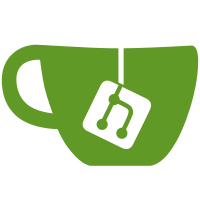
Move `AttributeMask` out of `llvm/IR/Attributes.h` to a new file `llvm/IR/AttributeMask.h`. After doing this we can remove the `#include <bitset>` and `#include <set>` directives from `Attributes.h`. Since there are many headers including `Attributes.h`, but not needing the definition of `AttributeMask`, this causes unnecessary bloating of the translation units and slows down compilation. This commit adds in the include directive for `llvm/IR/AttributeMask.h` to the handful of source files that need to see the definition. This reduces the total number of preprocessing tokens across the LLVM source files in lib from (roughly) 1,917,509,187 to 1,902,982,273 - a reduction of ~0.76%. This should result in a small improvement in compilation time. Differential Revision: https://reviews.llvm.org/D153728
1165 lines
48 KiB
C++
1165 lines
48 KiB
C++
//===- MLRegAllocEvictAdvisor.cpp - ML eviction advisor -------------------===//
|
|
//
|
|
// Part of the LLVM Project, under the Apache License v2.0 with LLVM Exceptions.
|
|
// See https://llvm.org/LICENSE.txt for license information.
|
|
// SPDX-License-Identifier: Apache-2.0 WITH LLVM-exception
|
|
//
|
|
//===----------------------------------------------------------------------===//
|
|
//
|
|
// Implementation of the ML eviction advisor and reward injection pass
|
|
//
|
|
//===----------------------------------------------------------------------===//
|
|
|
|
#include "AllocationOrder.h"
|
|
#include "RegAllocEvictionAdvisor.h"
|
|
#include "RegAllocGreedy.h"
|
|
#include "llvm/Analysis/InteractiveModelRunner.h"
|
|
#include "llvm/Analysis/MLModelRunner.h"
|
|
#include "llvm/Analysis/TensorSpec.h"
|
|
#if defined(LLVM_HAVE_TF_AOT_REGALLOCEVICTMODEL) || defined(LLVM_HAVE_TFLITE)
|
|
#include "llvm/Analysis/ModelUnderTrainingRunner.h"
|
|
#include "llvm/Analysis/NoInferenceModelRunner.h"
|
|
#include "llvm/Analysis/Utils/TrainingLogger.h"
|
|
#endif
|
|
#include "MLRegallocEvictAdvisor.h"
|
|
#include "llvm/Analysis/ReleaseModeModelRunner.h"
|
|
#include "llvm/CodeGen/CalcSpillWeights.h"
|
|
#include "llvm/CodeGen/LiveRegMatrix.h"
|
|
#include "llvm/CodeGen/MachineBlockFrequencyInfo.h"
|
|
#include "llvm/CodeGen/MachineFunction.h"
|
|
#include "llvm/CodeGen/MachineLoopInfo.h"
|
|
#include "llvm/CodeGen/MachineRegisterInfo.h"
|
|
#include "llvm/CodeGen/Passes.h"
|
|
#include "llvm/CodeGen/RegisterClassInfo.h"
|
|
#include "llvm/CodeGen/VirtRegMap.h"
|
|
#include "llvm/InitializePasses.h"
|
|
#include "llvm/Pass.h"
|
|
#include "llvm/PassRegistry.h"
|
|
#include "llvm/Support/CommandLine.h"
|
|
#include "llvm/Support/ErrorHandling.h"
|
|
|
|
#include <array>
|
|
#include <bitset>
|
|
#include <memory>
|
|
|
|
using namespace llvm;
|
|
|
|
#define DEBUG_TYPE "ml-regalloc"
|
|
|
|
// Generated header in release (AOT) mode
|
|
#if defined(LLVM_HAVE_TF_AOT_REGALLOCEVICTMODEL)
|
|
#include "RegallocEvictModel.h"
|
|
using CompiledModelType = RegallocEvictModel;
|
|
#else
|
|
using CompiledModelType = NoopSavedModelImpl;
|
|
#endif
|
|
|
|
static cl::opt<std::string> InteractiveChannelBaseName(
|
|
"regalloc-evict-interactive-channel-base", cl::Hidden,
|
|
cl::desc(
|
|
"Base file path for the interactive mode. The incoming filename should "
|
|
"have the name <regalloc-evict-interactive-channel-base>.in, while the "
|
|
"outgoing name should be "
|
|
"<regalloc-evict-interactive-channel-base>.out"));
|
|
|
|
// Options that only make sense in development mode
|
|
#ifdef LLVM_HAVE_TFLITE
|
|
#include "RegAllocScore.h"
|
|
#include "llvm/Analysis/Utils/TFUtils.h"
|
|
|
|
static cl::opt<std::string> TrainingLog(
|
|
"regalloc-training-log", cl::Hidden,
|
|
cl::desc("Training log for the register allocator eviction model"));
|
|
|
|
static cl::opt<std::string> ModelUnderTraining(
|
|
"regalloc-model", cl::Hidden,
|
|
cl::desc("The model being trained for register allocation eviction"));
|
|
|
|
static cl::opt<bool> EnableDevelopmentFeatures(
|
|
"regalloc-enable-development-features", cl::Hidden,
|
|
cl::desc("Whether or not to enable features under development for the ML "
|
|
"regalloc advisor"));
|
|
|
|
#else
|
|
static const bool EnableDevelopmentFeatures = false;
|
|
#endif // #ifdef LLVM_HAVE_TFLITE
|
|
|
|
/// The score injection pass.
|
|
/// This pass calculates the score for a function and inserts it in the log, but
|
|
/// this happens only in development mode. It's a no-op otherwise.
|
|
namespace llvm {
|
|
extern cl::opt<unsigned> EvictInterferenceCutoff;
|
|
|
|
class RegAllocScoring : public MachineFunctionPass {
|
|
public:
|
|
static char ID;
|
|
|
|
RegAllocScoring() : MachineFunctionPass(ID) {
|
|
initializeRegAllocScoringPass(*PassRegistry::getPassRegistry());
|
|
}
|
|
|
|
~RegAllocScoring() override = default;
|
|
|
|
StringRef getPassName() const override {
|
|
return "Register Allocation Pass Scoring";
|
|
}
|
|
|
|
/// RegAllocReward analysis usage.
|
|
void getAnalysisUsage(AnalysisUsage &AU) const override {
|
|
AU.setPreservesAll();
|
|
AU.addRequired<RegAllocEvictionAdvisorAnalysis>();
|
|
AU.addRequired<RegAllocPriorityAdvisorAnalysis>();
|
|
AU.addRequired<MachineBlockFrequencyInfo>();
|
|
MachineFunctionPass::getAnalysisUsage(AU);
|
|
}
|
|
|
|
/// Performs this pass
|
|
bool runOnMachineFunction(MachineFunction &) override;
|
|
};
|
|
|
|
char RegAllocScoring::ID = 0;
|
|
FunctionPass *createRegAllocScoringPass() { return new RegAllocScoring(); }
|
|
|
|
} // namespace llvm
|
|
|
|
INITIALIZE_PASS(RegAllocScoring, "regallocscoringpass",
|
|
"Register Allocation Scoring Pass", false, false)
|
|
|
|
// ===================================
|
|
// Common ML Advisor declarations
|
|
// ===================================
|
|
namespace {
|
|
// The model can only accept a specified number of opcodes and will error it if
|
|
// fed an opcode it hasn't seen before. This constant sets the current cutoff.
|
|
static const int OpcodeValueCutoff = 17716;
|
|
|
|
// Most features are as described above, so we'll reuse this vector in defining
|
|
// them.
|
|
static const std::vector<int64_t> PerLiveRangeShape{1, NumberOfInterferences};
|
|
|
|
// --------------
|
|
// Features table
|
|
// --------------
|
|
// For each interfering live range (incl. the candidate) we collect a number of
|
|
// features. However, because the features are of different types (and because
|
|
// of ML best practices), we organize the tensors per feature, not per
|
|
// candidate. Each such tensor has a scalar value corresponding to the
|
|
// interferring live range at that position, in the order in AllocationOrder.
|
|
// The last position corresponds to the virt reg seeking allocation.
|
|
// Exception to all that is the progression feature, which is just a scalar (see
|
|
// its documentation for details).
|
|
// Note on naming: the "_by_max" are normalized using the largest value of that
|
|
// tensor, as observed in the current decision making stage (i.e. for the
|
|
// current call to the advisor's tryFindEvictionCandidate)
|
|
//
|
|
// The feature list format: type, name, shape, documentation.
|
|
// Note: we can really just use int64 and float, hence the modeling of some
|
|
// bools as int64 values.
|
|
#define RA_EVICT_FEATURES_LIST(M) \
|
|
M(int64_t, mask, PerLiveRangeShape, \
|
|
"boolean values, 0 for unavailable candidates (i.e. if a position is 0, " \
|
|
"it " \
|
|
"can't be evicted)") \
|
|
M(int64_t, is_free, PerLiveRangeShape, \
|
|
"boolean values, 1 if this phys reg is actually free (no interferences)") \
|
|
M(float, nr_urgent, PerLiveRangeShape, \
|
|
"number of 'urgent' intervals, normalized. Urgent are those that are OK " \
|
|
"to break cascades") \
|
|
M(float, nr_broken_hints, PerLiveRangeShape, \
|
|
"if this position were evicted, how many broken hints would there be") \
|
|
M(int64_t, is_hint, PerLiveRangeShape, \
|
|
"is this a preferred phys reg for the candidate") \
|
|
M(int64_t, is_local, PerLiveRangeShape, \
|
|
"is this live range local to a basic block") \
|
|
M(float, nr_rematerializable, PerLiveRangeShape, \
|
|
"nr rematerializable ranges") \
|
|
M(float, nr_defs_and_uses, PerLiveRangeShape, \
|
|
"bb freq - weighed nr defs and uses") \
|
|
M(float, weighed_reads_by_max, PerLiveRangeShape, \
|
|
"bb freq - weighed nr of reads, normalized") \
|
|
M(float, weighed_writes_by_max, PerLiveRangeShape, \
|
|
"bb feq - weighed nr of writes, normalized") \
|
|
M(float, weighed_read_writes_by_max, PerLiveRangeShape, \
|
|
"bb freq - weighed nr of uses that are both read and writes, normalized") \
|
|
M(float, weighed_indvars_by_max, PerLiveRangeShape, \
|
|
"bb freq - weighed nr of uses that are indvars, normalized") \
|
|
M(float, hint_weights_by_max, PerLiveRangeShape, \
|
|
"bb freq - weighed nr of uses that are hints, normalized") \
|
|
M(float, start_bb_freq_by_max, PerLiveRangeShape, \
|
|
"the freq in the start block, normalized") \
|
|
M(float, end_bb_freq_by_max, PerLiveRangeShape, \
|
|
"freq of end block, normalized") \
|
|
M(float, hottest_bb_freq_by_max, PerLiveRangeShape, \
|
|
"hottest BB freq, normalized") \
|
|
M(float, liverange_size, PerLiveRangeShape, \
|
|
"size (instr index diff) of the LR") \
|
|
M(float, use_def_density, PerLiveRangeShape, \
|
|
"the max weight, as computed by the manual heuristic") \
|
|
M(int64_t, max_stage, PerLiveRangeShape, \
|
|
"largest stage of an interval in this LR") \
|
|
M(int64_t, min_stage, PerLiveRangeShape, \
|
|
"lowest stage of an interval in this LR") \
|
|
M(float, progress, {1}, "ratio of current queue size to initial size")
|
|
|
|
#ifdef LLVM_HAVE_TFLITE
|
|
#define RA_EVICT_FIRST_DEVELOPMENT_FEATURE(M) \
|
|
M(int64_t, instructions, InstructionsShape, \
|
|
"Opcodes of the instructions covered by the eviction problem")
|
|
|
|
#define RA_EVICT_REST_DEVELOPMENT_FEATURES(M) \
|
|
M(int64_t, instructions_mapping, InstructionsMappingShape, \
|
|
"A binary matrix mapping LRs to instruction opcodes") \
|
|
M(float, mbb_frequencies, MBBFrequencyShape, \
|
|
"A vector of machine basic block frequencies") \
|
|
M(int64_t, mbb_mapping, InstructionsShape, \
|
|
"A vector of indicies mapping instructions to MBBs")
|
|
#else
|
|
#define RA_EVICT_FIRST_DEVELOPMENT_FEATURE(M)
|
|
#define RA_EVICT_REST_DEVELOPMENT_FEATURES(M)
|
|
#endif
|
|
|
|
// The model learns to pick one of the mask == 1 interferences. This is the
|
|
// name of the output tensor. The contract with the model is that the output
|
|
// will be guaranteed to be to a mask == 1 position. Using a macro here to
|
|
// avoid 'not used' warnings (and keep cond compilation to a minimum)
|
|
#define DecisionName "index_to_evict"
|
|
static const TensorSpec DecisionSpec =
|
|
TensorSpec::createSpec<int64_t>(DecisionName, {1});
|
|
|
|
// Named features index.
|
|
enum FeatureIDs {
|
|
#define _FEATURE_IDX_SIMPLE(_, name, __, ___) name
|
|
#define _FEATURE_IDX(A, B, C, D) _FEATURE_IDX_SIMPLE(A, B, C, D),
|
|
RA_EVICT_FEATURES_LIST(_FEATURE_IDX) FeatureCount,
|
|
#ifdef LLVM_HAVE_TFLITE
|
|
RA_EVICT_FIRST_DEVELOPMENT_FEATURE(_FEATURE_IDX_SIMPLE) = FeatureCount,
|
|
#else
|
|
RA_EVICT_FIRST_DEVELOPMENT_FEATURE(_FEATURE_IDX)
|
|
#endif // #ifdef LLVM_HAVE_TFLITE
|
|
RA_EVICT_REST_DEVELOPMENT_FEATURES(_FEATURE_IDX) FeaturesWithDevelopmentCount
|
|
#undef _FEATURE_IDX
|
|
#undef _FEATURE_IDX_SIMPLE
|
|
};
|
|
|
|
// The ML advisor will typically have a sparse input to the evaluator, because
|
|
// various phys regs won't be available. It's easier (maintenance-wise) to
|
|
// bulk-reset the state of the evaluator each time we are about to use it
|
|
// again.
|
|
template <typename T> size_t getTotalSize(const std::vector<int64_t> &Shape) {
|
|
size_t Ret = sizeof(T);
|
|
for (const auto V : Shape)
|
|
Ret *= V;
|
|
return Ret;
|
|
}
|
|
|
|
void resetInputs(MLModelRunner &Runner) {
|
|
#define _RESET(TYPE, NAME, SHAPE, __) \
|
|
std::memset(Runner.getTensorUntyped(FeatureIDs::NAME), 0, \
|
|
getTotalSize<TYPE>(SHAPE));
|
|
RA_EVICT_FEATURES_LIST(_RESET)
|
|
if (EnableDevelopmentFeatures) {
|
|
RA_EVICT_FIRST_DEVELOPMENT_FEATURE(_RESET)
|
|
RA_EVICT_REST_DEVELOPMENT_FEATURES(_RESET)
|
|
#undef _RESET
|
|
}
|
|
}
|
|
|
|
// Per-live interval components that get aggregated into the feature values
|
|
// that will be passed to the evaluator.
|
|
struct LIFeatureComponents {
|
|
double R = 0;
|
|
double W = 0;
|
|
double RW = 0;
|
|
double IndVarUpdates = 0;
|
|
double HintWeights = 0.0;
|
|
int64_t NrDefsAndUses = 0;
|
|
float HottestBlockFreq = 0.0;
|
|
bool IsRemat = false;
|
|
};
|
|
|
|
using CandidateRegList =
|
|
std::array<std::pair<MCRegister, bool>, NumberOfInterferences>;
|
|
using FeaturesListNormalizer =
|
|
llvm::SmallVector<float, FeatureIDs::FeatureCount>;
|
|
|
|
/// The ML evictor (commonalities between release and development mode)
|
|
class MLEvictAdvisor : public RegAllocEvictionAdvisor {
|
|
public:
|
|
MLEvictAdvisor(const MachineFunction &MF, const RAGreedy &RA,
|
|
MLModelRunner *Runner, const MachineBlockFrequencyInfo &MBFI,
|
|
const MachineLoopInfo &Loops);
|
|
|
|
protected:
|
|
const RegAllocEvictionAdvisor &getDefaultAdvisor() const {
|
|
return static_cast<const RegAllocEvictionAdvisor &>(DefaultAdvisor);
|
|
}
|
|
|
|
// The assumption is that if the Runner could not be constructed, we emit-ed
|
|
// error, and we shouldn't be asking for it here.
|
|
const MLModelRunner &getRunner() const { return *Runner; }
|
|
|
|
/// This just calls Evaluate on the Runner, but in the development mode
|
|
/// case, if we're just capturing the log of the default advisor, it needs
|
|
/// to call the latter instead, so we need to pass all the necessary
|
|
/// parameters for it. In the development case, it will also log.
|
|
virtual int64_t
|
|
tryFindEvictionCandidatePosition(const LiveInterval &VirtReg,
|
|
const AllocationOrder &Order,
|
|
unsigned OrderLimit, uint8_t CostPerUseLimit,
|
|
const SmallVirtRegSet &FixedRegisters) const;
|
|
|
|
/// Load the features of the given VirtReg (allocated or not) at column Pos,
|
|
/// but if that can't be evicted, return false instead.
|
|
bool
|
|
loadInterferenceFeatures(const LiveInterval &VirtReg, MCRegister PhysReg,
|
|
bool IsHint, const SmallVirtRegSet &FixedRegisters,
|
|
llvm::SmallVectorImpl<float> &Largest, size_t Pos,
|
|
SmallVectorImpl<LRStartEndInfo> &LRPosInfo) const;
|
|
|
|
private:
|
|
static float getInitialQueueSize(const MachineFunction &MF);
|
|
|
|
MCRegister tryFindEvictionCandidate(
|
|
const LiveInterval &VirtReg, const AllocationOrder &Order,
|
|
uint8_t CostPerUseLimit,
|
|
const SmallVirtRegSet &FixedRegisters) const override;
|
|
|
|
void extractFeatures(const SmallVectorImpl<const LiveInterval *> &Intervals,
|
|
llvm::SmallVectorImpl<float> &Largest, size_t Pos,
|
|
int64_t IsHint, int64_t LocalIntfsCount, float NrUrgent,
|
|
SmallVectorImpl<LRStartEndInfo> &LRPosInfo) const;
|
|
|
|
// Point-in-time: we didn't learn this, so we always delegate to the
|
|
// default.
|
|
bool canEvictHintInterference(
|
|
const LiveInterval &VirtReg, MCRegister PhysReg,
|
|
const SmallVirtRegSet &FixedRegisters) const override {
|
|
return getDefaultAdvisor().canEvictHintInterference(VirtReg, PhysReg,
|
|
FixedRegisters);
|
|
}
|
|
|
|
const LIFeatureComponents &
|
|
getLIFeatureComponents(const LiveInterval &LI) const;
|
|
|
|
// Hold on to a default advisor for:
|
|
// 1) the implementation of canEvictHintInterference, because we didn't
|
|
// learn that nuance yet; 2) for bootstrapping (logging) in the development
|
|
// mode case.
|
|
const DefaultEvictionAdvisor DefaultAdvisor;
|
|
MLModelRunner *const Runner;
|
|
const MachineBlockFrequencyInfo &MBFI;
|
|
const MachineLoopInfo &Loops;
|
|
|
|
// Indices of those features we don't want to normalize.
|
|
// This could be static and shared, but its initialization is non-trivial.
|
|
std::bitset<FeatureIDs::FeatureCount> DoNotNormalize;
|
|
const float InitialQSize;
|
|
|
|
using RegID = unsigned;
|
|
mutable DenseMap<RegID, LIFeatureComponents> CachedFeatures;
|
|
};
|
|
|
|
#define _DECL_FEATURES(type, name, shape, _) \
|
|
TensorSpec::createSpec<type>(#name, shape),
|
|
|
|
// ===================================
|
|
// Release (AOT) - specifics
|
|
// ===================================
|
|
class ReleaseModeEvictionAdvisorAnalysis final
|
|
: public RegAllocEvictionAdvisorAnalysis {
|
|
public:
|
|
ReleaseModeEvictionAdvisorAnalysis()
|
|
: RegAllocEvictionAdvisorAnalysis(AdvisorMode::Release) {
|
|
if (EnableDevelopmentFeatures) {
|
|
InputFeatures = {RA_EVICT_FEATURES_LIST(
|
|
_DECL_FEATURES) RA_EVICT_FIRST_DEVELOPMENT_FEATURE(_DECL_FEATURES)
|
|
RA_EVICT_REST_DEVELOPMENT_FEATURES(_DECL_FEATURES)};
|
|
} else {
|
|
InputFeatures = {RA_EVICT_FEATURES_LIST(_DECL_FEATURES)};
|
|
}
|
|
}
|
|
// support for isa<> and dyn_cast.
|
|
static bool classof(const RegAllocEvictionAdvisorAnalysis *R) {
|
|
return R->getAdvisorMode() == AdvisorMode::Release;
|
|
}
|
|
|
|
private:
|
|
std::vector<TensorSpec> InputFeatures;
|
|
|
|
void getAnalysisUsage(AnalysisUsage &AU) const override {
|
|
AU.addRequired<MachineBlockFrequencyInfo>();
|
|
AU.addRequired<MachineLoopInfo>();
|
|
RegAllocEvictionAdvisorAnalysis::getAnalysisUsage(AU);
|
|
}
|
|
|
|
std::unique_ptr<RegAllocEvictionAdvisor>
|
|
getAdvisor(const MachineFunction &MF, const RAGreedy &RA) override {
|
|
if (!Runner) {
|
|
if (InteractiveChannelBaseName.empty())
|
|
Runner = std::make_unique<ReleaseModeModelRunner<CompiledModelType>>(
|
|
MF.getFunction().getContext(), InputFeatures, DecisionName);
|
|
else
|
|
Runner = std::make_unique<InteractiveModelRunner>(
|
|
MF.getFunction().getContext(), InputFeatures, DecisionSpec,
|
|
InteractiveChannelBaseName + ".out",
|
|
InteractiveChannelBaseName + ".in");
|
|
}
|
|
return std::make_unique<MLEvictAdvisor>(
|
|
MF, RA, Runner.get(), getAnalysis<MachineBlockFrequencyInfo>(),
|
|
getAnalysis<MachineLoopInfo>());
|
|
}
|
|
std::unique_ptr<MLModelRunner> Runner;
|
|
};
|
|
|
|
// ===================================
|
|
// Development mode-specifics
|
|
// ===================================
|
|
//
|
|
// Features we log
|
|
#ifdef LLVM_HAVE_TFLITE
|
|
static const TensorSpec Reward = TensorSpec::createSpec<float>("reward", {1});
|
|
|
|
// Features we bind on the model. The tensor names have a prefix, and we also
|
|
// need to include some tensors that are expected to be present by the
|
|
// training algo.
|
|
// TODO: can we just get rid of these?
|
|
#define _DECL_TRAIN_FEATURES(type, name, shape, _) \
|
|
TensorSpec::createSpec<type>(std::string("action_") + #name, shape),
|
|
|
|
class DevelopmentModeEvictAdvisor : public MLEvictAdvisor {
|
|
public:
|
|
DevelopmentModeEvictAdvisor(const MachineFunction &MF, const RAGreedy &RA,
|
|
MLModelRunner *Runner,
|
|
const MachineBlockFrequencyInfo &MBFI,
|
|
const MachineLoopInfo &Loops, Logger *Log)
|
|
: MLEvictAdvisor(MF, RA, Runner, MBFI, Loops), Log(Log) {}
|
|
|
|
private:
|
|
int64_t tryFindEvictionCandidatePosition(
|
|
const LiveInterval &VirtReg, const AllocationOrder &Order,
|
|
unsigned OrderLimit, uint8_t CostPerUseLimit,
|
|
const SmallVirtRegSet &FixedRegisters) const override;
|
|
|
|
Logger *const Log;
|
|
};
|
|
|
|
class DevelopmentModeEvictionAdvisorAnalysis final
|
|
: public RegAllocEvictionAdvisorAnalysis {
|
|
public:
|
|
DevelopmentModeEvictionAdvisorAnalysis()
|
|
: RegAllocEvictionAdvisorAnalysis(AdvisorMode::Development) {
|
|
if (EnableDevelopmentFeatures) {
|
|
InputFeatures = {RA_EVICT_FEATURES_LIST(
|
|
_DECL_FEATURES) RA_EVICT_FIRST_DEVELOPMENT_FEATURE(_DECL_FEATURES)
|
|
RA_EVICT_REST_DEVELOPMENT_FEATURES(_DECL_FEATURES)};
|
|
TrainingInputFeatures = {
|
|
RA_EVICT_FEATURES_LIST(_DECL_TRAIN_FEATURES)
|
|
RA_EVICT_FIRST_DEVELOPMENT_FEATURE(_DECL_TRAIN_FEATURES)
|
|
RA_EVICT_REST_DEVELOPMENT_FEATURES(_DECL_TRAIN_FEATURES)
|
|
TensorSpec::createSpec<float>("action_discount", {1}),
|
|
TensorSpec::createSpec<int32_t>("action_step_type", {1}),
|
|
TensorSpec::createSpec<float>("action_reward", {1})};
|
|
} else {
|
|
InputFeatures = {RA_EVICT_FEATURES_LIST(_DECL_FEATURES)};
|
|
TrainingInputFeatures = {
|
|
RA_EVICT_FEATURES_LIST(_DECL_TRAIN_FEATURES)
|
|
TensorSpec::createSpec<float>("action_discount", {1}),
|
|
TensorSpec::createSpec<int32_t>("action_step_type", {1}),
|
|
TensorSpec::createSpec<float>("action_reward", {1})};
|
|
}
|
|
}
|
|
// support for isa<> and dyn_cast.
|
|
static bool classof(const RegAllocEvictionAdvisorAnalysis *R) {
|
|
return R->getAdvisorMode() == AdvisorMode::Development;
|
|
}
|
|
|
|
void logRewardIfNeeded(const MachineFunction &MF,
|
|
llvm::function_ref<float()> GetReward) override {
|
|
if (!Log || !Log->hasAnyObservationForContext(MF.getName()))
|
|
return;
|
|
// The function pass manager would run all the function passes for a
|
|
// function, so we assume the last context belongs to this function. If
|
|
// this invariant ever changes, we can implement at that time switching
|
|
// contexts. At this point, it'd be an error
|
|
if (Log->currentContext() != MF.getName()) {
|
|
MF.getFunction().getContext().emitError(
|
|
"The training log context shouldn't have had changed.");
|
|
}
|
|
if (Log->hasObservationInProgress())
|
|
Log->logReward<float>(GetReward());
|
|
}
|
|
|
|
private:
|
|
std::vector<TensorSpec> InputFeatures;
|
|
std::vector<TensorSpec> TrainingInputFeatures;
|
|
|
|
void getAnalysisUsage(AnalysisUsage &AU) const override {
|
|
AU.addRequired<MachineBlockFrequencyInfo>();
|
|
AU.addRequired<MachineLoopInfo>();
|
|
RegAllocEvictionAdvisorAnalysis::getAnalysisUsage(AU);
|
|
}
|
|
|
|
bool doInitialization(Module &M) override {
|
|
LLVMContext &Ctx = M.getContext();
|
|
if (ModelUnderTraining.empty() && TrainingLog.empty()) {
|
|
Ctx.emitError("Regalloc development mode should be requested with at "
|
|
"least logging enabled and/or a training model");
|
|
return false;
|
|
}
|
|
if (ModelUnderTraining.empty())
|
|
Runner = std::make_unique<NoInferenceModelRunner>(Ctx, InputFeatures);
|
|
else
|
|
Runner = ModelUnderTrainingRunner::createAndEnsureValid(
|
|
Ctx, ModelUnderTraining, DecisionName, TrainingInputFeatures);
|
|
if (!Runner) {
|
|
Ctx.emitError("Regalloc: could not set up the model runner");
|
|
return false;
|
|
}
|
|
if (TrainingLog.empty())
|
|
return false;
|
|
std::error_code EC;
|
|
auto OS = std::make_unique<raw_fd_ostream>(TrainingLog, EC);
|
|
if (EC) {
|
|
M.getContext().emitError(EC.message() + ":" + TrainingLog);
|
|
return false;
|
|
}
|
|
std::vector<TensorSpec> LFS = InputFeatures;
|
|
if (auto *MUTR = dyn_cast<ModelUnderTrainingRunner>(Runner.get()))
|
|
append_range(LFS, MUTR->extraOutputsForLoggingSpecs());
|
|
// We always log the output; in particular, if we're not evaluating, we
|
|
// don't have an output spec json file. That's why we handle the
|
|
// 'normal' output separately.
|
|
LFS.push_back(DecisionSpec);
|
|
|
|
Log = std::make_unique<Logger>(std::move(OS), LFS, Reward,
|
|
/*IncludeReward*/ true);
|
|
return false;
|
|
}
|
|
|
|
std::unique_ptr<RegAllocEvictionAdvisor>
|
|
getAdvisor(const MachineFunction &MF, const RAGreedy &RA) override {
|
|
if (!Runner)
|
|
return nullptr;
|
|
if (Log)
|
|
Log->switchContext(MF.getName());
|
|
return std::make_unique<DevelopmentModeEvictAdvisor>(
|
|
MF, RA, Runner.get(), getAnalysis<MachineBlockFrequencyInfo>(),
|
|
getAnalysis<MachineLoopInfo>(), Log.get());
|
|
}
|
|
|
|
std::unique_ptr<MLModelRunner> Runner;
|
|
std::unique_ptr<Logger> Log;
|
|
};
|
|
|
|
#endif //#ifdef LLVM_HAVE_TFLITE
|
|
} // namespace
|
|
|
|
float MLEvictAdvisor::getInitialQueueSize(const MachineFunction &MF) {
|
|
auto &MRI = MF.getRegInfo();
|
|
float Ret = 0.0;
|
|
for (unsigned I = 0, E = MRI.getNumVirtRegs(); I != E; ++I) {
|
|
Register Reg = Register::index2VirtReg(I);
|
|
if (MRI.reg_nodbg_empty(Reg))
|
|
continue;
|
|
++Ret;
|
|
}
|
|
return Ret;
|
|
}
|
|
|
|
MLEvictAdvisor::MLEvictAdvisor(const MachineFunction &MF, const RAGreedy &RA,
|
|
MLModelRunner *Runner,
|
|
const MachineBlockFrequencyInfo &MBFI,
|
|
const MachineLoopInfo &Loops)
|
|
: RegAllocEvictionAdvisor(MF, RA), DefaultAdvisor(MF, RA),
|
|
Runner(std::move(Runner)), MBFI(MBFI), Loops(Loops),
|
|
InitialQSize(MLEvictAdvisor::getInitialQueueSize(MF)) {
|
|
assert(this->Runner);
|
|
Runner->switchContext(MF.getName());
|
|
DoNotNormalize.set(FeatureIDs::mask);
|
|
DoNotNormalize.set(FeatureIDs::is_free);
|
|
DoNotNormalize.set(FeatureIDs::is_hint);
|
|
DoNotNormalize.set(FeatureIDs::is_local);
|
|
DoNotNormalize.set(FeatureIDs::min_stage);
|
|
DoNotNormalize.set(FeatureIDs::max_stage);
|
|
DoNotNormalize.set(FeatureIDs::progress);
|
|
}
|
|
|
|
int64_t MLEvictAdvisor::tryFindEvictionCandidatePosition(
|
|
const LiveInterval &, const AllocationOrder &, unsigned, uint8_t,
|
|
const SmallVirtRegSet &) const {
|
|
int64_t Ret = Runner->evaluate<int64_t>();
|
|
assert(Ret >= 0);
|
|
assert(Ret <= CandidateVirtRegPos);
|
|
return Ret;
|
|
}
|
|
|
|
bool MLEvictAdvisor::loadInterferenceFeatures(
|
|
const LiveInterval &VirtReg, MCRegister PhysReg, bool IsHint,
|
|
const SmallVirtRegSet &FixedRegisters,
|
|
llvm::SmallVectorImpl<float> &Largest, size_t Pos,
|
|
llvm::SmallVectorImpl<LRStartEndInfo> &LRPosInfo) const {
|
|
// It is only possible to evict virtual register interference.
|
|
if (Matrix->checkInterference(VirtReg, PhysReg) > LiveRegMatrix::IK_VirtReg) {
|
|
// leave unavailable
|
|
return false;
|
|
}
|
|
|
|
const bool IsLocal = LIS->intervalIsInOneMBB(VirtReg);
|
|
int64_t LocalIntfs = 0;
|
|
float NrUrgent = 0.0f;
|
|
|
|
// The cascade tracking is the same as in the default advisor
|
|
unsigned Cascade = RA.getExtraInfo().getCascadeOrCurrentNext(VirtReg.reg());
|
|
|
|
SmallVector<const LiveInterval *, MaxInterferences> InterferingIntervals;
|
|
for (MCRegUnit Unit : TRI->regunits(PhysReg)) {
|
|
LiveIntervalUnion::Query &Q = Matrix->query(VirtReg, Unit);
|
|
// Different from the default heuristic, we don't make any assumptions
|
|
// about what having more than 10 results in the query may mean.
|
|
const auto &IFIntervals = Q.interferingVRegs(EvictInterferenceCutoff);
|
|
if (IFIntervals.empty() && InterferingIntervals.empty())
|
|
continue;
|
|
if (IFIntervals.size() >= EvictInterferenceCutoff)
|
|
return false;
|
|
InterferingIntervals.append(IFIntervals.begin(), IFIntervals.end());
|
|
for (const LiveInterval *Intf : reverse(IFIntervals)) {
|
|
assert(Intf->reg().isVirtual() &&
|
|
"Only expecting virtual register interference from query");
|
|
// This is the same set of legality checks as in the default case: don't
|
|
// try to evict fixed regs or 'done' ones. Also don't break cascades,
|
|
// except in the urgent case, with the same nuances used in the default
|
|
// heuristic.
|
|
// We could try sharing this between the advisors, but it may end up
|
|
// more complex than it is right now.
|
|
if (FixedRegisters.count(Intf->reg()))
|
|
return false;
|
|
if (RA.getExtraInfo().getStage(*Intf) == RS_Done)
|
|
return false;
|
|
bool Urgent =
|
|
!VirtReg.isSpillable() &&
|
|
(Intf->isSpillable() ||
|
|
RegClassInfo.getNumAllocatableRegs(MRI->getRegClass(VirtReg.reg())) <
|
|
RegClassInfo.getNumAllocatableRegs(
|
|
MRI->getRegClass(Intf->reg())));
|
|
// Only evict older cascades or live ranges without a cascade.
|
|
unsigned IntfCascade = RA.getExtraInfo().getCascade(Intf->reg());
|
|
if (Cascade <= IntfCascade) {
|
|
if (!Urgent)
|
|
return false;
|
|
++NrUrgent;
|
|
}
|
|
|
|
LocalIntfs += (IsLocal && LIS->intervalIsInOneMBB(*Intf) &&
|
|
(!EnableLocalReassign || !canReassign(*Intf, PhysReg)));
|
|
}
|
|
}
|
|
// OK, so if we made it this far, this LR is an eviction candidate, load its
|
|
// features.
|
|
extractFeatures(InterferingIntervals, Largest, Pos, IsHint, LocalIntfs,
|
|
NrUrgent, LRPosInfo);
|
|
return true;
|
|
}
|
|
|
|
MCRegister MLEvictAdvisor::tryFindEvictionCandidate(
|
|
const LiveInterval &VirtReg, const AllocationOrder &Order,
|
|
uint8_t CostPerUseLimit, const SmallVirtRegSet &FixedRegisters) const {
|
|
auto MaybeOrderLimit = getOrderLimit(VirtReg, Order, CostPerUseLimit);
|
|
if (!MaybeOrderLimit)
|
|
return MCRegister::NoRegister;
|
|
unsigned OrderLimit = *MaybeOrderLimit;
|
|
|
|
// The heuristic sets initial costs such as, if CostPerUseLimit is
|
|
// max<uint8_t>, then any of the costs of the legally-evictable intervals
|
|
// would be lower. When that happens, one of those will be selected.
|
|
// Therefore, we allow the candidate be selected, unless the candidate is
|
|
// unspillable, in which case it would be incorrect to not find a register
|
|
// for it.
|
|
const bool MustFindEviction =
|
|
(!VirtReg.isSpillable() && CostPerUseLimit == static_cast<uint8_t>(~0u));
|
|
// Number of available candidates - if 0, no need to continue.
|
|
size_t Available = 0;
|
|
// Make sure we don't have leftover partial state from an attempt where we
|
|
// had no available candidates and bailed out early.
|
|
resetInputs(*Runner);
|
|
|
|
// Track the index->register mapping because AllocationOrder doesn't do that
|
|
// and we'd have to scan it.
|
|
// Also track their mask, to write asserts/debug.
|
|
CandidateRegList Regs;
|
|
Regs.fill({0, false});
|
|
|
|
// Track the largest value of features seen during this eviction session. We
|
|
// only normalize (some of) the float features, but it's just simpler to
|
|
// dimension 'Largest' to all the features, especially since we have the
|
|
// 'DoNotNormalize' list.
|
|
FeaturesListNormalizer Largest(FeatureIDs::FeatureCount, 0.0);
|
|
|
|
// Same overal idea as in the default eviction policy - we visit the values
|
|
// of AllocationOrder one at a time. If it's not legally available, we mask
|
|
// off the corresponding feature column (==do nothing because we already
|
|
// reset all the features to 0) Use Pos to capture the column we load
|
|
// features at - in AllocationOrder order.
|
|
size_t Pos = 0;
|
|
SmallVector<LRStartEndInfo, NumberOfInterferences> LRPosInfo;
|
|
for (auto I = Order.begin(), E = Order.getOrderLimitEnd(OrderLimit); I != E;
|
|
++I, ++Pos) {
|
|
MCRegister PhysReg = *I;
|
|
assert(!Regs[Pos].second);
|
|
assert(PhysReg);
|
|
if (!canAllocatePhysReg(CostPerUseLimit, PhysReg)) {
|
|
continue;
|
|
}
|
|
if (loadInterferenceFeatures(VirtReg, PhysReg, I.isHint(), FixedRegisters,
|
|
Largest, Pos, LRPosInfo)) {
|
|
++Available;
|
|
Regs[Pos] = std::make_pair(PhysReg, true);
|
|
}
|
|
}
|
|
if (Available == 0) {
|
|
// Nothing to decide, nothing to learn.
|
|
assert(!MustFindEviction);
|
|
return MCRegister::NoRegister;
|
|
}
|
|
const size_t ValidPosLimit = Pos;
|
|
// If we must find eviction, the candidate should be masked out of the
|
|
// decision making process.
|
|
Regs[CandidateVirtRegPos].second = !MustFindEviction;
|
|
if (!MustFindEviction)
|
|
extractFeatures(SmallVector<const LiveInterval *, 1>(1, &VirtReg), Largest,
|
|
CandidateVirtRegPos, /*IsHint*/ 0,
|
|
/*LocalIntfsCount*/ 0,
|
|
/*NrUrgent*/ 0.0, LRPosInfo);
|
|
assert(InitialQSize > 0.0 && "We couldn't have gotten here if we had "
|
|
"nothing to allocate initially.");
|
|
#ifdef LLVM_HAVE_TFLITE
|
|
if (EnableDevelopmentFeatures) {
|
|
extractInstructionFeatures(
|
|
LRPosInfo, Runner,
|
|
[this](SlotIndex InputIndex) -> int {
|
|
auto *CurrentMachineInstruction =
|
|
LIS->getInstructionFromIndex(InputIndex);
|
|
if (!CurrentMachineInstruction) {
|
|
return -1;
|
|
}
|
|
return CurrentMachineInstruction->getOpcode();
|
|
},
|
|
[this](SlotIndex InputIndex) -> float {
|
|
auto *CurrentMachineInstruction =
|
|
LIS->getInstructionFromIndex(InputIndex);
|
|
return MBFI.getBlockFreqRelativeToEntryBlock(
|
|
CurrentMachineInstruction->getParent());
|
|
},
|
|
[this](SlotIndex InputIndex) -> MachineBasicBlock * {
|
|
auto *CurrentMachineInstruction =
|
|
LIS->getInstructionFromIndex(InputIndex);
|
|
return CurrentMachineInstruction->getParent();
|
|
},
|
|
FeatureIDs::instructions, FeatureIDs::instructions_mapping,
|
|
FeatureIDs::mbb_frequencies, FeatureIDs::mbb_mapping,
|
|
LIS->getSlotIndexes()->getLastIndex());
|
|
}
|
|
#endif // #ifdef LLVM_HAVE_TFLITE
|
|
// Normalize the features.
|
|
for (auto &V : Largest)
|
|
V = V ? V : 1.0;
|
|
for (size_t FeatureIndex = 0; FeatureIndex < FeatureIDs::FeatureCount;
|
|
++FeatureIndex) {
|
|
if (DoNotNormalize.test(FeatureIndex))
|
|
continue;
|
|
for (size_t Pos = 0; Pos < NumberOfInterferences; ++Pos) {
|
|
Runner->getTensor<float>(FeatureIndex)[Pos] /= Largest[FeatureIndex];
|
|
}
|
|
}
|
|
*Runner->getTensor<float>(FeatureIDs::progress) =
|
|
static_cast<float>(RA.getQueueSize()) / InitialQSize;
|
|
|
|
// Get a decision.
|
|
size_t CandidatePos = tryFindEvictionCandidatePosition(
|
|
VirtReg, Order, OrderLimit, CostPerUseLimit, FixedRegisters);
|
|
// The contract with the ML side is that CandidatePos is mask == 1 (i.e.
|
|
// Regs[CandidatePos].second)
|
|
assert(Regs[CandidatePos].second);
|
|
if (CandidatePos == CandidateVirtRegPos) {
|
|
assert(!MustFindEviction);
|
|
return MCRegister::NoRegister;
|
|
}
|
|
assert(CandidatePos < ValidPosLimit);
|
|
(void)ValidPosLimit;
|
|
return Regs[CandidatePos].first;
|
|
}
|
|
|
|
const LIFeatureComponents &
|
|
MLEvictAdvisor::getLIFeatureComponents(const LiveInterval &LI) const {
|
|
RegID ID = LI.reg().id();
|
|
LIFeatureComponents Empty;
|
|
auto I = CachedFeatures.insert(std::make_pair(ID, Empty));
|
|
LIFeatureComponents &Ret = I.first->getSecond();
|
|
if (!I.second)
|
|
return Ret;
|
|
|
|
SmallPtrSet<MachineInstr *, 8> Visited;
|
|
const TargetRegisterInfo &TRI = *MF.getSubtarget().getRegisterInfo();
|
|
|
|
for (MachineRegisterInfo::reg_instr_nodbg_iterator
|
|
I = MRI->reg_instr_nodbg_begin(LI.reg()),
|
|
E = MRI->reg_instr_nodbg_end();
|
|
I != E;) {
|
|
MachineInstr *MI = &*(I++);
|
|
|
|
++Ret.NrDefsAndUses;
|
|
if (!Visited.insert(MI).second)
|
|
continue;
|
|
|
|
if (MI->isIdentityCopy() || MI->isImplicitDef())
|
|
continue;
|
|
|
|
bool Reads, Writes;
|
|
std::tie(Reads, Writes) = MI->readsWritesVirtualRegister(LI.reg());
|
|
|
|
float Freq = MBFI.getBlockFreqRelativeToEntryBlock(MI->getParent());
|
|
Ret.HottestBlockFreq = std::max(Freq, Ret.HottestBlockFreq);
|
|
|
|
Ret.R += (Reads && !Writes) * Freq;
|
|
Ret.W += (!Reads && Writes) * Freq;
|
|
Ret.RW += (Reads && Writes) * Freq;
|
|
|
|
auto *MBB = MI->getParent();
|
|
auto *Loop = Loops.getLoopFor(MBB);
|
|
bool IsExiting = Loop ? Loop->isLoopExiting(MBB) : false;
|
|
|
|
if (Writes && IsExiting && LIS->isLiveOutOfMBB(LI, MBB))
|
|
Ret.IndVarUpdates += Freq;
|
|
|
|
if (MI->isCopy() && VirtRegAuxInfo::copyHint(MI, LI.reg(), TRI, *MRI))
|
|
Ret.HintWeights += Freq;
|
|
}
|
|
Ret.IsRemat = VirtRegAuxInfo::isRematerializable(
|
|
LI, *LIS, *VRM, *MF.getSubtarget().getInstrInfo());
|
|
return Ret;
|
|
}
|
|
|
|
// Overall, this currently mimics what we do for weight calculation, but instead
|
|
// of accummulating the various features, we keep them separate.
|
|
void MLEvictAdvisor::extractFeatures(
|
|
const SmallVectorImpl<const LiveInterval *> &Intervals,
|
|
llvm::SmallVectorImpl<float> &Largest, size_t Pos, int64_t IsHint,
|
|
int64_t LocalIntfsCount, float NrUrgent,
|
|
SmallVectorImpl<LRStartEndInfo> &LRPosInfo) const {
|
|
int64_t NrDefsAndUses = 0;
|
|
int64_t NrBrokenHints = 0;
|
|
double R = 0.0;
|
|
double W = 0.0;
|
|
double RW = 0.0;
|
|
double IndVarUpdates = 0.0;
|
|
double HintWeights = 0.0;
|
|
float StartBBFreq = 0.0;
|
|
float EndBBFreq = 0.0;
|
|
float HottestBlockFreq = 0.0;
|
|
int32_t NrRematerializable = 0;
|
|
float TotalWeight = 0.0;
|
|
|
|
SlotIndex EndSI = LIS->getSlotIndexes()->getZeroIndex();
|
|
SlotIndex StartSI = LIS->getSlotIndexes()->getLastIndex();
|
|
int64_t MaxStage = 0;
|
|
int64_t MinStage =
|
|
Intervals.empty() ? 0 : std::numeric_limits<int64_t>::max();
|
|
|
|
for (const auto *L : Intervals) {
|
|
const LiveInterval &LI = *L;
|
|
MaxStage = std::max<int64_t>(
|
|
MaxStage, static_cast<int64_t>(RA.getExtraInfo().getStage(LI)));
|
|
MinStage = std::min<int64_t>(
|
|
MinStage, static_cast<int64_t>(RA.getExtraInfo().getStage(LI)));
|
|
|
|
TotalWeight = std::max(TotalWeight, LI.weight());
|
|
|
|
if (LI.beginIndex() < StartSI)
|
|
StartSI = LI.beginIndex();
|
|
|
|
if (LI.endIndex() > EndSI)
|
|
EndSI = LI.endIndex();
|
|
const LIFeatureComponents &LIFC = getLIFeatureComponents(LI);
|
|
NrBrokenHints += VRM->hasPreferredPhys(LI.reg());
|
|
|
|
NrDefsAndUses += LIFC.NrDefsAndUses;
|
|
HottestBlockFreq = std::max(HottestBlockFreq, LIFC.HottestBlockFreq);
|
|
R += LIFC.R;
|
|
W += LIFC.W;
|
|
RW += LIFC.RW;
|
|
|
|
IndVarUpdates += LIFC.IndVarUpdates;
|
|
|
|
HintWeights += LIFC.HintWeights;
|
|
NrRematerializable += LIFC.IsRemat;
|
|
|
|
if (EnableDevelopmentFeatures) {
|
|
for (auto CurrentSegment : LI) {
|
|
LRPosInfo.push_back(
|
|
LRStartEndInfo{CurrentSegment.start, CurrentSegment.end, Pos});
|
|
}
|
|
}
|
|
}
|
|
size_t Size = 0;
|
|
if (!Intervals.empty()) {
|
|
StartBBFreq =
|
|
MBFI.getBlockFreqRelativeToEntryBlock(LIS->getMBBFromIndex(StartSI));
|
|
if (EndSI >= LIS->getSlotIndexes()->getLastIndex())
|
|
EndSI = LIS->getSlotIndexes()->getLastIndex().getPrevIndex();
|
|
EndBBFreq =
|
|
MBFI.getBlockFreqRelativeToEntryBlock(LIS->getMBBFromIndex(EndSI));
|
|
Size = StartSI.distance(EndSI);
|
|
}
|
|
// Set the features at the column 'Pos'.
|
|
#define SET(ID, TYPE, VAL) \
|
|
do { \
|
|
Runner->getTensor<TYPE>(FeatureIDs::ID)[Pos] = static_cast<TYPE>(VAL); \
|
|
if (!DoNotNormalize.test(FeatureIDs::ID)) \
|
|
Largest[FeatureIDs::ID] = \
|
|
std::max(Largest[FeatureIDs::ID], static_cast<float>(VAL)); \
|
|
} while (false)
|
|
SET(mask, int64_t, 1);
|
|
SET(is_free, int64_t, Intervals.empty());
|
|
SET(nr_urgent, float, NrUrgent);
|
|
SET(nr_broken_hints, float, NrBrokenHints);
|
|
SET(is_hint, int64_t, IsHint);
|
|
SET(is_local, int64_t, LocalIntfsCount);
|
|
SET(nr_rematerializable, float, NrRematerializable);
|
|
SET(nr_defs_and_uses, float, NrDefsAndUses);
|
|
SET(weighed_reads_by_max, float, R);
|
|
SET(weighed_writes_by_max, float, W);
|
|
SET(weighed_read_writes_by_max, float, RW);
|
|
SET(weighed_indvars_by_max, float, IndVarUpdates);
|
|
SET(hint_weights_by_max, float, HintWeights);
|
|
SET(start_bb_freq_by_max, float, StartBBFreq);
|
|
SET(end_bb_freq_by_max, float, EndBBFreq);
|
|
SET(hottest_bb_freq_by_max, float, HottestBlockFreq);
|
|
SET(liverange_size, float, Size);
|
|
SET(use_def_density, float, TotalWeight);
|
|
SET(max_stage, int64_t, MaxStage);
|
|
SET(min_stage, int64_t, MinStage);
|
|
#undef SET
|
|
}
|
|
|
|
void extractInstructionFeatures(
|
|
SmallVectorImpl<LRStartEndInfo> &LRPosInfo, MLModelRunner *RegallocRunner,
|
|
function_ref<int(SlotIndex)> GetOpcode,
|
|
function_ref<float(SlotIndex)> GetMBBFreq,
|
|
function_ref<MachineBasicBlock *(SlotIndex)> GetMBBReference,
|
|
const int InstructionsIndex, const int InstructionsMappingIndex,
|
|
const int MBBFreqIndex, const int MBBMappingIndex,
|
|
const SlotIndex LastIndex) {
|
|
// This function extracts instruction based features relevant to the eviction
|
|
// problem currently being solved. This function ends up extracting two
|
|
// tensors.
|
|
// 1 - A vector of size max instruction count. It contains the opcodes of the
|
|
// instructions spanned by all the intervals in the current instance of the
|
|
// eviction problem.
|
|
// 2 - A binary mapping matrix of size (LR count * max
|
|
// instruction count) which maps where the LRs are live to the actual opcodes
|
|
// for which they are live.
|
|
// 3 - A vector of size max supported MBB count storing MBB frequencies,
|
|
// encompassing all of the MBBs covered by the eviction problem.
|
|
// 4 - A vector of size max instruction count of indices to members of the MBB
|
|
// frequency vector, mapping each instruction to its associated MBB.
|
|
|
|
// Start off by sorting the segments based on the beginning slot index.
|
|
std::sort(
|
|
LRPosInfo.begin(), LRPosInfo.end(),
|
|
[](LRStartEndInfo A, LRStartEndInfo B) { return A.Begin < B.Begin; });
|
|
size_t InstructionIndex = 0;
|
|
size_t CurrentSegmentIndex = 0;
|
|
SlotIndex CurrentIndex = LRPosInfo[0].Begin;
|
|
std::map<MachineBasicBlock *, size_t> VisitedMBBs;
|
|
size_t CurrentMBBIndex = 0;
|
|
// This loop processes all the segments sequentially by starting at the
|
|
// beginning slot index of the first segment, iterating through all the slot
|
|
// indices before the end slot index of that segment (while checking for
|
|
// overlaps with segments that start at greater slot indices). After hitting
|
|
// that end index, the current segment being processed gets bumped until they
|
|
// are all processed or the max instruction count is hit, where everything is
|
|
// just truncated.
|
|
while (true) {
|
|
// If the index that we are currently at is within the current segment and
|
|
// we haven't hit the max instruction count, continue processing the current
|
|
// segment.
|
|
while (CurrentIndex <= LRPosInfo[CurrentSegmentIndex].End &&
|
|
InstructionIndex < ModelMaxSupportedInstructionCount) {
|
|
int CurrentOpcode = GetOpcode(CurrentIndex);
|
|
// If the current machine instruction is null, skip it
|
|
if (CurrentOpcode == -1) {
|
|
// If we're currently at the last index in the SlotIndex analysis,
|
|
// we can't go any further, so return from the function
|
|
if (CurrentIndex >= LastIndex) {
|
|
return;
|
|
}
|
|
CurrentIndex = CurrentIndex.getNextIndex();
|
|
continue;
|
|
}
|
|
MachineBasicBlock *CurrentMBBReference = GetMBBReference(CurrentIndex);
|
|
if (VisitedMBBs.count(CurrentMBBReference) == 0) {
|
|
VisitedMBBs[CurrentMBBReference] = CurrentMBBIndex;
|
|
++CurrentMBBIndex;
|
|
}
|
|
extractMBBFrequency(CurrentIndex, InstructionIndex, VisitedMBBs,
|
|
GetMBBFreq, CurrentMBBReference, RegallocRunner,
|
|
MBBFreqIndex, MBBMappingIndex);
|
|
// Current code assumes we're not going to get any disjointed segments
|
|
assert(LRPosInfo[CurrentSegmentIndex].Begin <= CurrentIndex);
|
|
RegallocRunner->getTensor<int64_t>(InstructionsIndex)[InstructionIndex] =
|
|
CurrentOpcode < OpcodeValueCutoff ? CurrentOpcode : 0;
|
|
// set value in the binary mapping matrix for the current instruction
|
|
auto CurrentSegmentPosition = LRPosInfo[CurrentSegmentIndex].Pos;
|
|
RegallocRunner->getTensor<int64_t>(
|
|
InstructionsMappingIndex)[CurrentSegmentPosition *
|
|
ModelMaxSupportedInstructionCount +
|
|
InstructionIndex] = 1;
|
|
// All of the segments are sorted based on the beginning slot index, but
|
|
// this doesn't mean that the beginning slot index of the next segment is
|
|
// after the end segment of the one being currently processed. This while
|
|
// loop checks for overlapping segments and modifies the portion of the
|
|
// column in the mapping matrix for the currently processed instruction
|
|
// for the LR it is checking. Also make sure that the beginning of the
|
|
// current segment we're checking for overlap in is less than the current
|
|
// index, otherwise we're done checking overlaps.
|
|
size_t OverlapCheckCurrentSegment = CurrentSegmentIndex + 1;
|
|
while (OverlapCheckCurrentSegment < LRPosInfo.size() &&
|
|
LRPosInfo[OverlapCheckCurrentSegment].Begin <= CurrentIndex) {
|
|
auto OverlapCurrentSegmentPosition =
|
|
LRPosInfo[OverlapCheckCurrentSegment].Pos;
|
|
if (LRPosInfo[OverlapCheckCurrentSegment].End >= CurrentIndex) {
|
|
RegallocRunner->getTensor<int64_t>(
|
|
InstructionsMappingIndex)[OverlapCurrentSegmentPosition *
|
|
ModelMaxSupportedInstructionCount +
|
|
InstructionIndex] = 1;
|
|
}
|
|
++OverlapCheckCurrentSegment;
|
|
}
|
|
++InstructionIndex;
|
|
if (CurrentIndex >= LastIndex) {
|
|
return;
|
|
}
|
|
CurrentIndex = CurrentIndex.getNextIndex();
|
|
}
|
|
// if we've just finished processing through the last segment or if we've
|
|
// hit the maximum number of instructions, break out of the loop.
|
|
if (CurrentSegmentIndex == LRPosInfo.size() - 1 ||
|
|
InstructionIndex >= ModelMaxSupportedInstructionCount) {
|
|
break;
|
|
}
|
|
// If the segments are not overlapping, we need to move to the beginning
|
|
// index of the next segment to avoid having instructions not attached to
|
|
// any register.
|
|
if (LRPosInfo[CurrentSegmentIndex + 1].Begin >
|
|
LRPosInfo[CurrentSegmentIndex].End) {
|
|
CurrentIndex = LRPosInfo[CurrentSegmentIndex + 1].Begin;
|
|
}
|
|
++CurrentSegmentIndex;
|
|
}
|
|
}
|
|
|
|
void extractMBBFrequency(const SlotIndex CurrentIndex,
|
|
const size_t CurrentInstructionIndex,
|
|
std::map<MachineBasicBlock *, size_t> &VisitedMBBs,
|
|
function_ref<float(SlotIndex)> GetMBBFreq,
|
|
MachineBasicBlock *CurrentMBBReference,
|
|
MLModelRunner *RegallocRunner, const int MBBFreqIndex,
|
|
const int MBBMappingIndex) {
|
|
size_t CurrentMBBIndex = VisitedMBBs[CurrentMBBReference];
|
|
float CurrentMBBFreq = GetMBBFreq(CurrentIndex);
|
|
if (CurrentMBBIndex < ModelMaxSupportedMBBCount) {
|
|
RegallocRunner->getTensor<float>(MBBFreqIndex)[CurrentMBBIndex] =
|
|
CurrentMBBFreq;
|
|
RegallocRunner->getTensor<int64_t>(
|
|
MBBMappingIndex)[CurrentInstructionIndex] = CurrentMBBIndex;
|
|
}
|
|
}
|
|
|
|
// Development mode-specific implementations
|
|
#ifdef LLVM_HAVE_TFLITE
|
|
|
|
RegAllocEvictionAdvisorAnalysis *llvm::createDevelopmentModeAdvisor() {
|
|
return new DevelopmentModeEvictionAdvisorAnalysis();
|
|
}
|
|
|
|
int64_t DevelopmentModeEvictAdvisor::tryFindEvictionCandidatePosition(
|
|
const LiveInterval &VirtReg, const AllocationOrder &Order,
|
|
unsigned OrderLimit, uint8_t CostPerUseLimit,
|
|
const SmallVirtRegSet &FixedRegisters) const {
|
|
int64_t Ret = 0;
|
|
if (isa<ModelUnderTrainingRunner>(getRunner())) {
|
|
Ret = MLEvictAdvisor::tryFindEvictionCandidatePosition(
|
|
VirtReg, Order, OrderLimit, CostPerUseLimit, FixedRegisters);
|
|
} else {
|
|
MCRegister PhysReg = getDefaultAdvisor().tryFindEvictionCandidate(
|
|
VirtReg, Order, CostPerUseLimit, FixedRegisters);
|
|
// Find the index of the selected PhysReg. We need it for logging,
|
|
// otherwise this is wasted cycles (but so would starting development mode
|
|
// without a model nor logging)
|
|
if (!PhysReg)
|
|
Ret = CandidateVirtRegPos;
|
|
else
|
|
for (auto I = Order.begin(), E = Order.getOrderLimitEnd(OrderLimit);
|
|
I != E; ++I, ++Ret)
|
|
if (*I == PhysReg)
|
|
break;
|
|
}
|
|
if (TrainingLog.empty())
|
|
return Ret;
|
|
// TODO(mtrofin): when we support optional rewards, this can go away. In the
|
|
// meantime, we log the "pretend" reward (0) for the previous observation
|
|
// before starting a new one.
|
|
if (Log->hasObservationInProgress())
|
|
Log->logReward<float>(0.0);
|
|
|
|
Log->startObservation();
|
|
size_t CurrentFeature = 0;
|
|
size_t FeatureCount = EnableDevelopmentFeatures
|
|
? FeatureIDs::FeaturesWithDevelopmentCount
|
|
: FeatureIDs::FeatureCount;
|
|
for (; CurrentFeature < FeatureCount; ++CurrentFeature) {
|
|
Log->logTensorValue(CurrentFeature,
|
|
reinterpret_cast<const char *>(
|
|
getRunner().getTensorUntyped(CurrentFeature)));
|
|
}
|
|
if (auto *MUTR = dyn_cast<ModelUnderTrainingRunner>(&getRunner()))
|
|
for (size_t I = 0; I < MUTR->extraOutputsForLoggingSpecs().size();
|
|
++I, ++CurrentFeature)
|
|
Log->logTensorValue(
|
|
CurrentFeature,
|
|
reinterpret_cast<const char *>(MUTR->getUntypedExtraOutputValue(I)));
|
|
// The output is right after the features and the extra outputs
|
|
Log->logTensorValue(CurrentFeature, reinterpret_cast<const char *>(&Ret));
|
|
Log->endObservation();
|
|
return Ret;
|
|
}
|
|
|
|
bool RegAllocScoring::runOnMachineFunction(MachineFunction &MF) {
|
|
std::optional<float> CachedReward;
|
|
auto GetReward = [&]() {
|
|
if (!CachedReward)
|
|
CachedReward = static_cast<float>(
|
|
calculateRegAllocScore(MF, getAnalysis<MachineBlockFrequencyInfo>())
|
|
.getScore());
|
|
return *CachedReward;
|
|
};
|
|
|
|
getAnalysis<RegAllocEvictionAdvisorAnalysis>().logRewardIfNeeded(MF,
|
|
GetReward);
|
|
getAnalysis<RegAllocPriorityAdvisorAnalysis>().logRewardIfNeeded(MF,
|
|
GetReward);
|
|
return false;
|
|
}
|
|
#endif // #ifdef LLVM_HAVE_TFLITE
|
|
|
|
RegAllocEvictionAdvisorAnalysis *llvm::createReleaseModeAdvisor() {
|
|
return llvm::isEmbeddedModelEvaluatorValid<CompiledModelType>() ||
|
|
!InteractiveChannelBaseName.empty()
|
|
? new ReleaseModeEvictionAdvisorAnalysis()
|
|
: nullptr;
|
|
}
|
|
|
|
// In all cases except development mode, we don't need scoring.
|
|
#if !defined(LLVM_HAVE_TFLITE)
|
|
bool RegAllocScoring::runOnMachineFunction(MachineFunction &) { return false; }
|
|
#endif
|