mirror of
https://github.com/llvm/llvm-project.git
synced 2025-05-01 11:06:06 +00:00
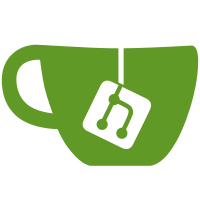
This patch implements tile and fuse transformation for ops that implement the tiling interface. To do so, - `TilingInterface` needs a new method that generates a tiled implementation of the operation based on the tile of the result needed. - A pattern is added that replaces a `tensor.extract_slice` whose source is defined by an operation that implements the `TilingInterface` with a tiled implementation that produces the extracted slice in-place (using the method added to `TilingInterface`). - A pattern is added that takes a sequence of operations that implement the `TilingInterface` (for now `LinalgOp`s), tiles the consumer, and greedily fuses its producers iteratively. Differential Revision: https://reviews.llvm.org/D127809
195 lines
12 KiB
MLIR
195 lines
12 KiB
MLIR
// RUN: mlir-opt -test-tiling-interface=tile-using-scf-for -split-input-file %s | FileCheck %s
|
|
|
|
func.func @simple_matmul(%arg0 : tensor<?x?xf32>, %arg1 : tensor<?x?xf32>,
|
|
%arg2 : tensor<?x?xf32>) -> tensor<?x?xf32> {
|
|
%0 = linalg.matmul {__internal_linalg_transform__ = "simple_gemm"}
|
|
ins(%arg0, %arg1 : tensor<?x?xf32>, tensor<?x?xf32>)
|
|
outs(%arg2 : tensor<?x?xf32>) -> tensor<?x?xf32>
|
|
return %0 : tensor<?x?xf32>
|
|
}
|
|
// CHECK-DAG: #[[MAP0:.+]] = affine_map<(d0)[s0, s1] -> (10, -d0 + s1)>
|
|
// CHECK-DAG: #[[MAP1:.+]] = affine_map<(d0)[s0, s1] -> (20, -d0 + s1)>
|
|
// CHECK: func.func @simple_matmul(
|
|
// CHECK-SAME: %[[ARG0:[a-zA-Z0-9]+]]: tensor<?x?xf32>
|
|
// CHECK-SAME: %[[ARG1:[a-zA-Z0-9]+]]: tensor<?x?xf32>
|
|
// CHECK-SAME: %[[ARG2:[a-zA-Z0-9]+]]: tensor<?x?xf32>
|
|
// CHECK-DAG: %[[C0:.+]] = arith.constant 0 : index
|
|
// CHECK-DAG: %[[C1:.+]] = arith.constant 1 : index
|
|
// CHECK-DAG: %[[C10:.+]] = arith.constant 10 : index
|
|
// CHECK-DAG: %[[C20:.+]] = arith.constant 20 : index
|
|
// CHECK-DAG: %[[M:.+]] = tensor.dim %[[ARG0]], %[[C0]]
|
|
// CHECK-DAG: %[[K:.+]] = tensor.dim %[[ARG0]], %[[C1]]
|
|
// CHECK-DAG: %[[N:.+]] = tensor.dim %[[ARG1]], %[[C1]]
|
|
// CHECK: %[[OUTER:[a-zA-Z0-9]+]] = scf.for %[[IV0:[a-zA-Z0-9]+]] = %[[C0]] to %[[M]] step %[[C10]]
|
|
// CHECK-SAME: iter_args(%[[INIT0:.+]] = %[[ARG2]])
|
|
// CHECK: %[[TS_Y:.+]] = affine.min #[[MAP0]](%[[IV0]])[%[[C10]], %[[M]]]
|
|
// CHECK: %[[INNER:[a-zA-Z0-9]+]] = scf.for %[[IV1:[a-zA-Z0-9]+]] = %[[C0]] to %[[N]] step %[[C20]]
|
|
// CHECK-SAME: iter_args(%[[INIT1:.+]] = %[[INIT0]])
|
|
// CHECK: %[[TS_X:.+]] = affine.min #[[MAP1]](%[[IV1]])[%[[C20]], %[[N]]]
|
|
// CHECK-DAG: %[[LHS_TILE:.+]] = tensor.extract_slice %[[ARG0]]
|
|
// CHECK-SAME: [%[[IV0]], 0] [%[[TS_Y]], %[[K]]] [1, 1]
|
|
// CHECK-DAG: %[[RHS_TILE:.+]] = tensor.extract_slice %[[ARG1]]
|
|
// CHECK-SAME: [0, %[[IV1]]] [%[[K]], %[[TS_X]]] [1, 1]
|
|
// CHECK-DAG: %[[INIT_TILE:.+]] = tensor.extract_slice %[[INIT1]]
|
|
// CHECK-SAME: [%[[IV0]], %[[IV1]]] [%[[TS_Y]], %[[TS_X]]] [1, 1]
|
|
// CHECK: %[[GEMM_TILE:.+]] = linalg.matmul
|
|
// CHECK-SAME: ins(%[[LHS_TILE]], %[[RHS_TILE]] :
|
|
// CHECK-SAME: outs(%[[INIT_TILE]] :
|
|
// CHECK: %[[UPDATE:.+]] = tensor.insert_slice %[[GEMM_TILE]] into %[[INIT1]]
|
|
// CHECK-SAME: [%[[IV0]], %[[IV1]]] [%[[TS_Y]], %[[TS_X]]] [1, 1]
|
|
// CHECK: scf.yield %[[UPDATE]]
|
|
// CHECK: scf.yield %[[INNER]]
|
|
// CHECK: return %[[OUTER]]
|
|
|
|
// -----
|
|
|
|
func.func @simple_matmul_memref(%arg0 : memref<?x?xf32>, %arg1 : memref<?x?xf32>,
|
|
%arg2 : memref<?x?xf32>) {
|
|
linalg.matmul {__internal_linalg_transform__ = "simple_gemm_memref"}
|
|
ins(%arg0, %arg1 : memref<?x?xf32>, memref<?x?xf32>)
|
|
outs(%arg2 : memref<?x?xf32>)
|
|
return
|
|
}
|
|
// CHECK-DAG: #[[MAP0:.+]] = affine_map<(d0)[s0, s1] -> (10, -d0 + s1)>
|
|
// CHECK-DAG: #[[MAP1:.+]] = affine_map<(d0)[s0, s1] -> (20, -d0 + s1)>
|
|
// CHECK-DAG: #[[MAP2:.+]] = affine_map<(d0)[s0, s1] -> (30, -d0 + s1)>
|
|
// CHECK: func.func @simple_matmul_memref(
|
|
// CHECK-SAME: %[[ARG0:[a-zA-Z0-9]+]]: memref<?x?xf32>
|
|
// CHECK-SAME: %[[ARG1:[a-zA-Z0-9]+]]: memref<?x?xf32>
|
|
// CHECK-SAME: %[[ARG2:[a-zA-Z0-9]+]]: memref<?x?xf32>
|
|
// CHECK-DAG: %[[C0:.+]] = arith.constant 0 : index
|
|
// CHECK-DAG: %[[C1:.+]] = arith.constant 1 : index
|
|
// CHECK-DAG: %[[C10:.+]] = arith.constant 10 : index
|
|
// CHECK-DAG: %[[C20:.+]] = arith.constant 20 : index
|
|
// CHECK-DAG: %[[C30:.+]] = arith.constant 30 : index
|
|
// CHECK-DAG: %[[M:.+]] = memref.dim %[[ARG0]], %[[C0]]
|
|
// CHECK-DAG: %[[K:.+]] = memref.dim %[[ARG0]], %[[C1]]
|
|
// CHECK-DAG: %[[N:.+]] = memref.dim %[[ARG1]], %[[C1]]
|
|
// CHECK: scf.for %[[IV0:[a-zA-Z0-9]+]] = %[[C0]] to %[[M]] step %[[C10]]
|
|
// CHECK: %[[TS_M:.+]] = affine.min #[[MAP0]](%[[IV0]])[%[[C10]], %[[M]]]
|
|
// CHECK: scf.for %[[IV1:[a-zA-Z0-9]+]] = %[[C0]] to %[[N]] step %[[C20]]
|
|
// CHECK: %[[TS_N:.+]] = affine.min #[[MAP1]](%[[IV1]])[%[[C20]], %[[N]]]
|
|
// CHECK: scf.for %[[IV2:[a-zA-Z0-9]+]] = %[[C0]] to %[[K]] step %[[C30]]
|
|
// CHECK: %[[TS_K:.+]] = affine.min #[[MAP2]](%[[IV2]])[%[[C30]], %[[K]]]
|
|
// CHECK-DAG: %[[LHS_TILE:.+]] = memref.subview %[[ARG0]]
|
|
// CHECK-SAME: [%[[IV0]], %[[IV2]]] [%[[TS_M]], %[[TS_K]]] [1, 1]
|
|
// CHECK-DAG: %[[RHS_TILE:.+]] = memref.subview %[[ARG1]]
|
|
// CHECK-SAME: [%[[IV2]], %[[IV1]]] [%[[TS_K]], %[[TS_N]]] [1, 1]
|
|
// CHECK-DAG: %[[OUT_TILE:.+]] = memref.subview %[[ARG2]]
|
|
// CHECK-SAME: [%[[IV0]], %[[IV1]]] [%[[TS_M]], %[[TS_N]]] [1, 1]
|
|
// CHECK: linalg.matmul
|
|
// CHECK-SAME: ins(%[[LHS_TILE]], %[[RHS_TILE]] :
|
|
// CHECK-SAME: outs(%[[OUT_TILE]] :
|
|
|
|
// -----
|
|
|
|
#map0 = affine_map<(d0, d1, d2) -> (d0, d1, d2)>
|
|
#map1 = affine_map<(d0, d1, d2) -> (d0, d2, d1)>
|
|
#map2 = affine_map<(d0, d1, d2) -> (d2, d0, d1)>
|
|
func.func @multi_result(%arg0 : tensor<128x200x300xf32>) -> (tensor<128x300x200xf32>, tensor<300x128x200xf32>) {
|
|
%init0 = linalg.init_tensor [128, 300, 200] : tensor<128x300x200xf32>
|
|
%init1 = linalg.init_tensor [300, 128, 200] : tensor<300x128x200xf32>
|
|
%0:2 = linalg.generic {
|
|
indexing_maps = [#map0, #map1, #map2],
|
|
iterator_types = ["parallel", "parallel", "parallel"]}
|
|
{__internal_linalg_transform__ = "parallel_generic_transpose"}
|
|
ins(%arg0 : tensor<128x200x300xf32>)
|
|
outs(%init0, %init1 : tensor<128x300x200xf32>, tensor<300x128x200xf32>) {
|
|
^bb0(%b0 : f32, %b1 : f32, %b2 : f32):
|
|
linalg.yield %b0, %b0 : f32, f32
|
|
} -> (tensor<128x300x200xf32>, tensor<300x128x200xf32>)
|
|
return %0#0, %0#1 : tensor<128x300x200xf32>, tensor<300x128x200xf32>
|
|
}
|
|
// CHECK-DAG: #[[MAP0:.+]] = affine_map<(d0)[s0, s1] -> (10, -d0 + s1)>
|
|
// CHECK-DAG: #[[MAP1:.+]] = affine_map<(d0)[s0, s1] -> (20, -d0 + s1)>
|
|
// CHECK: func.func @multi_result(
|
|
// CHECK-SAME: %[[ARG0:[a-zA-Z0-9]+]]: tensor<128x200x300xf32>)
|
|
// CHECK-DAG: %[[C0:.+]] = arith.constant 0 : index
|
|
// CHECK-DAG: %[[C10:.+]] = arith.constant 10 : index
|
|
// CHECK-DAG: %[[C20:.+]] = arith.constant 20 : index
|
|
// CHECK-DAG: %[[C128:.+]] = arith.constant 128 : index
|
|
// CHECK-DAG: %[[C300:.+]] = arith.constant 300 : index
|
|
// CHECK-DAG: %[[INIT0:.+]] = linalg.init_tensor [128, 300, 200]
|
|
// CHECK-DAG: %[[INIT1:.+]] = linalg.init_tensor [300, 128, 200]
|
|
// CHECK: %[[OUTER:[a-zA-Z0-9]+]]:2 = scf.for %[[IV0:[a-zA-Z0-9]+]] = %[[C0]] to %[[C128]] step %[[C10]]
|
|
// CHECK-SAME: iter_args(%[[ARG1:[a-zA-Z0-9]+]] = %[[INIT0]], %[[ARG2:[a-zA-Z0-9]+]] = %[[INIT1]])
|
|
// CHECK: %[[TS_Y:.+]] = affine.min #[[MAP0]](%[[IV0]])[%[[C10]], %[[C128]]]
|
|
// CHECK: %[[INNER:[a-zA-Z0-9]+]]:2 = scf.for %[[IV1:[a-zA-Z0-9]+]] = %[[C0]] to %[[C300]] step %[[C20]]
|
|
// CHECK-SAME: iter_args(%[[ARG3:[a-zA-Z0-9]+]] = %[[ARG1]], %[[ARG4:[a-zA-Z0-9]+]] = %[[ARG2]])
|
|
// CHECK: %[[TS_X:.+]] = affine.min #[[MAP1]](%[[IV1]])[%[[C20]], %[[C300]]]
|
|
// CHECK-DAG: %[[ARG_TILE:.+]] = tensor.extract_slice %[[ARG0]]
|
|
// CHECK-SAME: [%[[IV0]], 0, %[[IV1]]] [%[[TS_Y]], 200, %[[TS_X]]] [1, 1, 1]
|
|
// CHECK-DAG: %[[INIT0_TILE:.+]] = tensor.extract_slice %[[ARG3]]
|
|
// CHECK-SAME: [%[[IV0]], %[[IV1]], 0] [%[[TS_Y]], %[[TS_X]], 200] [1, 1, 1]
|
|
// CHECK-DAG: %[[INIT1_TILE:.+]] = tensor.extract_slice %[[ARG4]]
|
|
// CHECK-SAME: [%[[IV1]], %[[IV0]], 0] [%[[TS_X]], %[[TS_Y]], 200] [1, 1, 1]
|
|
// CHECK: %[[RESULT_TILE:.+]]:2 = linalg.generic
|
|
// CHECK-SAME: ins(%[[ARG_TILE]] :
|
|
// CHECK-SAME: outs(%[[INIT0_TILE]], %[[INIT1_TILE]] :
|
|
// CHECK: %[[UPDATE0:.+]] = tensor.insert_slice %[[RESULT_TILE]]#0 into %[[ARG3]]
|
|
// CHECK-SAME: [%[[IV0]], %[[IV1]], 0] [%[[TS_Y]], %[[TS_X]], 200] [1, 1, 1]
|
|
// CHECK: %[[UPDATE1:.+]] = tensor.insert_slice %[[RESULT_TILE]]#1 into %[[ARG4]]
|
|
// CHECK-SAME: [%[[IV1]], %[[IV0]], 0] [%[[TS_X]], %[[TS_Y]], 200] [1, 1, 1]
|
|
// CHECK: scf.yield %[[UPDATE0]], %[[UPDATE1]]
|
|
// CHECK: scf.yield %[[INNER]]#0, %[[INNER]]#1
|
|
// CHECK: return %[[OUTER]]#0, %[[OUTER]]#1
|
|
|
|
// -----
|
|
|
|
func.func @conv2D(%arg0 : tensor<?x?x?x?xf32>, %arg1 : tensor<?x?x?x?xf32>,
|
|
%arg2 : tensor<?x?x?x?xf32>) -> tensor<?x?x?x?xf32> {
|
|
%0 = linalg.conv_2d_nhwc_hwcf {
|
|
strides = dense<[2, 3]> : tensor<2xi64>,
|
|
dilation = dense<[4, 5]> : tensor<2xi64>,
|
|
__internal_linalg_transform__ = "simple_conv"}
|
|
ins(%arg0, %arg1 : tensor<?x?x?x?xf32>, tensor<?x?x?x?xf32>)
|
|
outs(%arg2 : tensor<?x?x?x?xf32>) -> tensor<?x?x?x?xf32>
|
|
return %0 : tensor<?x?x?x?xf32>
|
|
}
|
|
// CHECK-DAG: #[[MAP0:.+]] = affine_map<(d0)[s0, s1] -> (10, -d0 + s1)>
|
|
// CHECK-DAG: #[[MAP1:.+]] = affine_map<(d0)[s0, s1] -> (20, -d0 + s1)>
|
|
// CHECK-DAG: #[[MAP2:.+]] = affine_map<(d0)[s0, s1] -> (30, -d0 + s1)>
|
|
// CHECK-DAG: #[[MAP3:.+]] = affine_map<(d0)[s0] -> (d0 + s0 * 2 - 2)>
|
|
// CHECK-DAG: #[[MAP4:.+]] = affine_map<(d0)[s0] -> (d0 + s0 * 3 - 3)>
|
|
// CHECK: func.func @conv2D(
|
|
// CHECK-SAME: %[[INPUT:[a-zA-Z0-9]+]]: tensor<?x?x?x?xf32>
|
|
// CHECK-SAME: %[[FILTER:[a-zA-Z0-9]+]]: tensor<?x?x?x?xf32>
|
|
// CHECK-SAME: %[[INIT:[a-zA-Z0-9]+]]: tensor<?x?x?x?xf32>
|
|
// CHECK-DAG: %[[C0:.+]] = arith.constant 0 : index
|
|
// CHECK-DAG: %[[C1:.+]] = arith.constant 1 : index
|
|
// CHECK-DAG: %[[C2:.+]] = arith.constant 2 : index
|
|
// CHECK-DAG: %[[C3:.+]] = arith.constant 3 : index
|
|
// CHECK-DAG: %[[C10:.+]] = arith.constant 10 : index
|
|
// CHECK-DAG: %[[C20:.+]] = arith.constant 20 : index
|
|
// CHECK-DAG: %[[C30:.+]] = arith.constant 30 : index
|
|
// CHECK-DAG: %[[N:.+]] = tensor.dim %[[INPUT]], %[[C0]]
|
|
// CHECK-DAG: %[[C:.+]] = tensor.dim %[[INPUT]], %[[C3]]
|
|
// CHECK-DAG: %[[P:.+]] = tensor.dim %[[FILTER]], %[[C0]]
|
|
// CHECK-DAG: %[[Q:.+]] = tensor.dim %[[FILTER]], %[[C1]]
|
|
// CHECK-DAG: %[[F:.+]] = tensor.dim %[[FILTER]], %[[C3]]
|
|
// CHECK-DAG: %[[R:.+]] = tensor.dim %[[INIT]], %[[C1]]
|
|
// CHECK-DAG: %[[S:.+]] = tensor.dim %[[INIT]], %[[C2]]
|
|
// CHECK: scf.for %[[IV0:[a-zA-Z0-9]+]] = %[[C0]] to %[[P]] step %[[C10]]
|
|
// CHECK-SAME: iter_args(%[[INIT0:.+]] = %[[INIT]])
|
|
// CHECK: %[[TS_P:.+]] = affine.min #[[MAP0]](%[[IV0]])[%[[C10]], %[[P]]]
|
|
// CHECK: scf.for %[[IV1:[a-zA-Z0-9]+]] = %[[C0]] to %[[Q]] step %[[C20]]
|
|
// CHECK-SAME: iter_args(%[[INIT1:.+]] = %[[INIT0]])
|
|
// CHECK: %[[TS_Q:.+]] = affine.min #[[MAP1]](%[[IV1]])[%[[C20]], %[[Q]]]
|
|
// CHECK: scf.for %[[IV2:[a-zA-Z0-9]+]] = %[[C0]] to %[[C]] step %[[C30]]
|
|
// CHECK-SAME: iter_args(%[[INIT2:.+]] = %[[INIT1]])
|
|
// CHECK-DAG: %[[TS_C:.+]] = affine.min #[[MAP2]](%[[IV2]])[%[[C30]], %[[C]]]
|
|
// CHECK-DAG: %[[TS_H:.+]] = affine.apply #[[MAP3]](%[[TS_P]])[%[[R]]]
|
|
// CHECK-DAG: %[[TS_W:.+]] = affine.apply #[[MAP4]](%[[TS_Q]])[%[[S]]]
|
|
// CHECK-DAG: %[[INPUT_TILE:.+]] = tensor.extract_slice %[[INPUT]]
|
|
// CHECK-SAME: [0, %[[IV0]], %[[IV1]], %[[IV2]]] [%[[N]], %[[TS_H]], %[[TS_W]], %[[TS_C]]]
|
|
// CHECK-DAG: %[[FILTER_TILE:.+]] = tensor.extract_slice %[[FILTER]]
|
|
// CHECK-SAME: [%[[IV0]], %[[IV1]], %[[IV2]], 0] [%[[TS_P]], %[[TS_Q]], %[[TS_C]], %[[F]]]
|
|
// CHECK-DAG: %[[INIT_TILE:.+]] = tensor.extract_slice %[[INIT2]]
|
|
// CHECK-SAME: [0, 0, 0, 0] [%[[N]], %[[R]], %[[S]], %[[F]]]
|
|
// CHECK: %[[CONV_TILE:.+]] = linalg.conv_2d_nhwc_hwcf
|
|
// CHECK-SAME: dilation = dense<[4, 5]> : tensor<2xi64>, strides = dense<[2, 3]> : tensor<2xi64>
|
|
// CHECK-SAME: ins(%[[INPUT_TILE]], %[[FILTER_TILE]] :
|
|
// CHECK-SAME: outs(%[[INIT_TILE]] :
|
|
// CHECK: tensor.insert_slice %[[CONV_TILE]] into %[[INIT2]]
|
|
// CHECK-SAME: [0, 0, 0, 0] [%[[N]], %[[R]], %[[S]], %[[F]]]
|