mirror of
https://github.com/llvm/llvm-project.git
synced 2025-04-27 12:36:09 +00:00
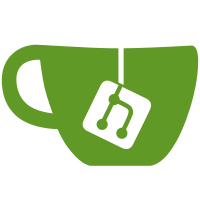
This commit adds functionality needed for implementation of convolutions with linalg.generic op. Since linalg.generic right now expects indexing maps to be just permutations, offset indexing needed in convolutions is not possible. Therefore in this commit we address the issue by adding support for symbols inside indexing maps which enables more advanced indexing. The upcoming commit will solve the problem of computing loop bounds from such maps. Differential Revision: https://reviews.llvm.org/D83158
1033 lines
44 KiB
C++
1033 lines
44 KiB
C++
//===- Fusion.cpp - Implementation of linalg Fusion -----------------------===//
|
|
//
|
|
// Part of the LLVM Project, under the Apache License v2.0 with LLVM Exceptions.
|
|
// See https://llvm.org/LICENSE.txt for license information.
|
|
// SPDX-License-Identifier: Apache-2.0 WITH LLVM-exception
|
|
//
|
|
//===----------------------------------------------------------------------===//
|
|
//
|
|
// This file implements the linalg dialect Fusion pass.
|
|
//
|
|
//===----------------------------------------------------------------------===//
|
|
|
|
#include "PassDetail.h"
|
|
#include "mlir/Dialect/Affine/IR/AffineOps.h"
|
|
#include "mlir/Dialect/Linalg/Analysis/DependenceAnalysis.h"
|
|
#include "mlir/Dialect/Linalg/EDSC/FoldedIntrinsics.h"
|
|
#include "mlir/Dialect/Linalg/IR/LinalgOps.h"
|
|
#include "mlir/Dialect/Linalg/IR/LinalgTypes.h"
|
|
#include "mlir/Dialect/Linalg/Passes.h"
|
|
#include "mlir/Dialect/Linalg/Utils/Utils.h"
|
|
#include "mlir/Dialect/StandardOps/EDSC/Intrinsics.h"
|
|
#include "mlir/IR/AffineExpr.h"
|
|
#include "mlir/IR/AffineMap.h"
|
|
#include "mlir/IR/Dominance.h"
|
|
#include "mlir/IR/PatternMatch.h"
|
|
#include "mlir/Support/LLVM.h"
|
|
#include "mlir/Transforms/FoldUtils.h"
|
|
#include "llvm/ADT/SetVector.h"
|
|
#include "llvm/Support/CommandLine.h"
|
|
#include "llvm/Support/Debug.h"
|
|
|
|
#define DEBUG_TYPE "linalg-fusion"
|
|
|
|
using namespace mlir;
|
|
using namespace mlir::edsc;
|
|
using namespace mlir::edsc::intrinsics;
|
|
using namespace mlir::linalg;
|
|
|
|
using folded_std_constant_index = FoldedValueBuilder<ConstantIndexOp>;
|
|
|
|
using llvm::dbgs;
|
|
|
|
/// Implements a simple high-level fusion pass of linalg library operations.
|
|
///
|
|
/// In each block, linalg ops are processed in reverse textual order.
|
|
/// Given a linalg op `O`, fusion occurs by:
|
|
/// 1. inspecting the linalg ops that write into the views read by `O`. This
|
|
/// uses the SSA value of the views and a simple subview/slice analysis to
|
|
/// determine producer-consumer dependences;
|
|
/// 2. greedily fuse the linalg ops that produce subview
|
|
/// 3. inspect the fused ops and determine whether they have other remaining
|
|
/// LinalgOp uses. If not, then erase the original producing linalg op.
|
|
///
|
|
/// More advanced use cases, analyses as well as profitability heuristics are
|
|
/// left for future work.
|
|
|
|
// Return a cloned version of `op` that operates on `loopRanges`, assumed to be
|
|
// a subset of the original loop ranges of `op`.
|
|
// This is achieved by applying the `loopToOperandRangesMaps` permutation maps
|
|
// to the `loopRanges` in order to obtain view ranges.
|
|
static LinalgOp cloneWithLoopRanges(OpBuilder &b, Location loc, LinalgOp op,
|
|
ArrayRef<SubViewOp::Range> loopRanges) {
|
|
assert(op.hasBufferSemantics() && "expected linalg op with buffer semantics");
|
|
auto maps = op.indexing_maps();
|
|
SmallVector<Value, 8> clonedViews;
|
|
clonedViews.reserve(op.getNumInputsAndOutputs());
|
|
// Iterate over the inputs and outputs in order.
|
|
// Extract the subranges from the linearized ranges.
|
|
SmallVector<Value, 8> ios(op.getInputsAndOutputBuffers());
|
|
for (auto en : llvm::enumerate(ios)) {
|
|
unsigned idx = en.index();
|
|
auto map = maps[idx].cast<AffineMapAttr>().getValue();
|
|
LLVM_DEBUG(dbgs() << "map: " << map << "\n");
|
|
Value view = en.value();
|
|
SmallVector<SubViewOp::Range, 4> viewRanges(map.getNumResults());
|
|
for (auto en2 : llvm::enumerate(map.getResults())) {
|
|
unsigned d = en2.index();
|
|
// loopToOperandRangesMaps are permutations-only.
|
|
unsigned loopPos = en2.value().cast<AffineDimExpr>().getPosition();
|
|
viewRanges[d] = loopRanges[loopPos];
|
|
LLVM_DEBUG(dbgs() << "\ni,j: " << en.index() << ", " << en2.index()
|
|
<< "\t"
|
|
<< "loopPos: " << loopPos << "\t" << viewRanges[d]);
|
|
}
|
|
// Construct a new subview for the tile.
|
|
unsigned rank = viewRanges.size();
|
|
SmallVector<Value, 4> offsets, sizes, strides;
|
|
offsets.reserve(rank);
|
|
sizes.reserve(rank);
|
|
strides.reserve(rank);
|
|
for (auto r : viewRanges) {
|
|
offsets.push_back(r.offset);
|
|
sizes.push_back(r.size);
|
|
strides.push_back(r.stride);
|
|
}
|
|
clonedViews.push_back(
|
|
b.create<SubViewOp>(loc, view, offsets, sizes, strides));
|
|
}
|
|
auto operands = getAssumedNonViewOperands(op);
|
|
clonedViews.append(operands.begin(), operands.end());
|
|
|
|
Operation *clonedOp = op.clone(b, loc, clonedViews);
|
|
// When the producer is an IndexedGenercOp, we have to transform its block
|
|
// IV arguments according to the tiling of the consumer, i.e. offset them by
|
|
// the values computed in `loopRanges`.
|
|
if (auto indexedGenericOp = dyn_cast<IndexedGenericOp>(clonedOp)) {
|
|
auto &block = indexedGenericOp.region().front();
|
|
|
|
OpBuilder::InsertionGuard g(b);
|
|
b.setInsertionPointToStart(&block);
|
|
for (unsigned i = 0, e = indexedGenericOp.getNumLoops(); i < e; ++i) {
|
|
Value oldIndex = block.getArgument(i);
|
|
AddIOp newIndex = b.create<AddIOp>(indexedGenericOp.getLoc(), oldIndex,
|
|
loopRanges[i].offset);
|
|
oldIndex.replaceAllUsesExcept(newIndex,
|
|
SmallPtrSet<Operation *, 1>{newIndex});
|
|
}
|
|
}
|
|
return clonedOp;
|
|
}
|
|
|
|
struct ViewDimension {
|
|
Value view;
|
|
unsigned dimension;
|
|
};
|
|
|
|
// Given an `op`, returns the first (`view`, `dimension`) pair that identifies
|
|
// the loop range at `loopDepth`. The semantics of the loopToOperandRangesMaps
|
|
// guarantees at least one such dimension is found. If multiple candidates exist
|
|
// they must agree by construction (i.e. have the same size) and we just return
|
|
// the first one.
|
|
static ViewDimension getViewDefiningLoopRange(LinalgOp op, unsigned loopDepth) {
|
|
assert(op.hasBufferSemantics() && "expected linalg op with buffer semantics");
|
|
auto maps = op.indexing_maps();
|
|
// Iterate over the inputs and outputs in order.
|
|
// Extract the subranges from the linearized ranges.
|
|
SmallVector<Value, 8> ios(op.getInputsAndOutputBuffers());
|
|
for (auto en : llvm::enumerate(ios)) {
|
|
unsigned idx = en.index();
|
|
auto map = maps[idx].cast<AffineMapAttr>().getValue();
|
|
LLVM_DEBUG(dbgs() << "getViewDefiningLoopRange I/O idx: " << idx << "\n");
|
|
LLVM_DEBUG(dbgs() << "getViewDefiningLoopRange map: " << map << "\n");
|
|
Value view = en.value();
|
|
SmallVector<Value, 8> viewRanges(map.getNumResults(), nullptr);
|
|
for (auto en2 : llvm::enumerate(map.getResults())) {
|
|
if (loopDepth == en2.value().cast<AffineDimExpr>().getPosition()) {
|
|
LLVM_DEBUG(dbgs() << "getViewDefiningLoopRange loopDepth: " << loopDepth
|
|
<< "\n");
|
|
LLVM_DEBUG(dbgs() << "getViewDefiningLoopRange view: " << view << "\n");
|
|
return ViewDimension{view, static_cast<unsigned>(en2.index())};
|
|
}
|
|
}
|
|
}
|
|
llvm_unreachable("Expect to be able to extract a view defining loop range");
|
|
}
|
|
|
|
static LinalgOp fuse(Value producedView, LinalgOp producer, LinalgOp consumer,
|
|
unsigned consumerIdx, unsigned producerIdx,
|
|
OperationFolder *folder) {
|
|
assert(producer.hasBufferSemantics() &&
|
|
"expected linalg op with buffer semantics");
|
|
assert(consumer.hasBufferSemantics() &&
|
|
"expected linalg op with buffer semantics");
|
|
|
|
auto subView = dyn_cast_or_null<SubViewOp>(
|
|
consumer.getBuffer(consumerIdx).getDefiningOp());
|
|
auto slice = dyn_cast_or_null<SliceOp>(
|
|
consumer.getBuffer(consumerIdx).getDefiningOp());
|
|
assert(subView || slice);
|
|
(void)subView;
|
|
(void)slice;
|
|
|
|
// loopToOperandRangesMaps are permutations-only by construction:
|
|
// we can always identify a data dimension with a (at least one) loop
|
|
// dimension.
|
|
AffineMap producerMap =
|
|
producer.indexing_maps()[producer.getNumInputs() + producerIdx]
|
|
.cast<AffineMapAttr>()
|
|
.getValue();
|
|
LLVM_DEBUG(dbgs() << "Producer Idx: " << producerIdx
|
|
<< ", producer map: " << producerMap << "\n");
|
|
|
|
unsigned nPar = producer.getNumParallelLoops();
|
|
unsigned nRed = producer.getNumReductionLoops();
|
|
unsigned nWin = producer.getNumWindowLoops();
|
|
SmallVector<SubViewOp::Range, 8> loopRanges(nPar + nRed + nWin);
|
|
|
|
OpBuilder b(consumer.getOperation());
|
|
auto loc = consumer.getLoc();
|
|
// Iterate over dimensions identified by the producer map for `producerIdx`.
|
|
// This defines a subset of the loop ranges that we need to complete later.
|
|
for (auto en : llvm::enumerate(producerMap.getResults())) {
|
|
unsigned posInProducerLoop = en.value().cast<AffineDimExpr>().getPosition();
|
|
loopRanges[posInProducerLoop] =
|
|
subView.getOrCreateRanges(b, loc)[en.index()];
|
|
}
|
|
|
|
// Iterate over all dimensions. For the dimensions not identified by the
|
|
// producer map for `producerIdx`, we need to explicitly compute the view that
|
|
// defines the loop ranges using the `producer`.
|
|
for (unsigned i = 0, nLoops = loopRanges.size(); i < nLoops; ++i) {
|
|
if (loopRanges[i].offset)
|
|
LLVM_DEBUG(llvm::dbgs()
|
|
<< "existing LoopRange: " << loopRanges[i] << "\n");
|
|
else {
|
|
auto viewDim = getViewDefiningLoopRange(producer, i);
|
|
loopRanges[i] = SubViewOp::Range{folded_std_constant_index(folder, 0),
|
|
std_dim(viewDim.view, viewDim.dimension),
|
|
folded_std_constant_index(folder, 1)};
|
|
LLVM_DEBUG(llvm::dbgs() << "new LoopRange: " << loopRanges[i] << "\n");
|
|
}
|
|
}
|
|
|
|
return cloneWithLoopRanges(b, loc, producer, loopRanges);
|
|
}
|
|
|
|
// Encode structural fusion safety preconditions.
|
|
// Some of these will be lifted in the future with better analysis.
|
|
static bool isStructurallyFusableProducer(LinalgOp producer, Value consumedView,
|
|
LinalgOp consumer) {
|
|
assert(producer.hasBufferSemantics() &&
|
|
"expected linalg op with buffer semantics");
|
|
assert(consumer.hasBufferSemantics() &&
|
|
"expected linalg op with buffer semantics");
|
|
if (producer.getNumOutputs() != 1) {
|
|
LLVM_DEBUG(dbgs() << "\nNot structurally fusable (multi-output)");
|
|
return false;
|
|
}
|
|
// Only fuse when the producer block dominates.
|
|
DominanceInfo dom(producer.getOperation());
|
|
if (!dom.dominates(producer.getOperation()->getBlock(),
|
|
consumer.getOperation()->getBlock())) {
|
|
LLVM_DEBUG(
|
|
dbgs()
|
|
<< "\nNot structurally fusable (producer block does not dominate)");
|
|
return false;
|
|
}
|
|
return true;
|
|
}
|
|
|
|
bool mlir::linalg::isProducerLastWriteOfView(const LinalgDependenceGraph &graph,
|
|
LinalgOp consumer,
|
|
Value consumedView,
|
|
LinalgOp producer) {
|
|
assert(producer.hasBufferSemantics() &&
|
|
"expected linalg op with buffer semantics");
|
|
assert(consumer.hasBufferSemantics() &&
|
|
"expected linalg op with buffer semantics");
|
|
// Make some simple structural checks that alleviate the need for more
|
|
// complex analyses.
|
|
if (!isStructurallyFusableProducer(producer, consumedView, consumer)) {
|
|
LLVM_DEBUG(dbgs() << "\n***Not static last write due to structure:\t"
|
|
<< *producer.getOperation());
|
|
return false;
|
|
}
|
|
// Check for any interleaved write to consumedView.
|
|
if (!graph.findCoveringWrites(producer, consumer, consumedView).empty()) {
|
|
LLVM_DEBUG(dbgs() << "\n***Not fusable due to interleaved write:\t"
|
|
<< *producer.getOperation());
|
|
return false;
|
|
}
|
|
return true;
|
|
}
|
|
|
|
bool mlir::linalg::isFusableInto(const LinalgDependenceGraph &graph,
|
|
LinalgOp consumer, Value consumedView,
|
|
LinalgOp producer) {
|
|
assert(producer.hasBufferSemantics() &&
|
|
"expected linalg op with buffer semantics");
|
|
assert(consumer.hasBufferSemantics() &&
|
|
"expected linalg op with buffer semantics");
|
|
if (!isProducerLastWriteOfView(graph, consumer, consumedView, producer))
|
|
return false;
|
|
// Check for any fusion-preventing dependence to any view read/written that
|
|
// would violate dependences.
|
|
if (!graph.findCoveringDependences(producer, consumer).empty()) {
|
|
LLVM_DEBUG(dbgs() << "\n***Not fusable due to an interleaved dependence:\t"
|
|
<< *producer.getOperation());
|
|
return false;
|
|
}
|
|
if (auto convOp = dyn_cast<linalg::ConvOp>(producer.getOperation())) {
|
|
// TODO: add a level of indirection to linalg.generic.
|
|
if (convOp.padding())
|
|
return false;
|
|
}
|
|
if (auto convOp = dyn_cast<linalg::ConvOp>(consumer.getOperation())) {
|
|
// TODO: add a level of indirection to linalg.generic.
|
|
if (convOp.padding())
|
|
return false;
|
|
}
|
|
return true;
|
|
}
|
|
|
|
static bool isSameSubView(Value a, Value b) {
|
|
if (a == b)
|
|
return true;
|
|
auto sva = a.getDefiningOp<SubViewOp>();
|
|
auto svb = b.getDefiningOp<SubViewOp>();
|
|
if (!sva || !svb)
|
|
return false;
|
|
if (!isSameSubView(sva.getViewSource(), svb.getViewSource()))
|
|
return false;
|
|
if (sva.getType() != svb.getType())
|
|
return false;
|
|
if (sva.getRank() != svb.getRank())
|
|
return false;
|
|
if (sva.getNumOperands() != svb.getNumOperands())
|
|
return false;
|
|
if (sva.static_offsets() != svb.static_offsets())
|
|
return false;
|
|
if (sva.static_sizes() != svb.static_sizes())
|
|
return false;
|
|
if (sva.static_strides() != svb.static_strides())
|
|
return false;
|
|
/// Skip the "viewSource" operand.
|
|
for (unsigned idx = 1, e = sva.getNumOperands(); idx != e; ++idx)
|
|
if (sva.getOperand(idx) != svb.getOperand(idx))
|
|
return false;
|
|
return true;
|
|
}
|
|
|
|
static Optional<FusionInfo>
|
|
fuseProducerOfDep(OpBuilder &b, LinalgOp consumer, unsigned consumerIdx,
|
|
const LinalgDependenceGraph &graph, OperationFolder *folder,
|
|
LinalgDependenceGraph::DependenceType depType) {
|
|
assert(consumer.hasBufferSemantics() &&
|
|
"expected linalg op with buffer semantics");
|
|
LLVM_DEBUG(dbgs() << "\nStart examining consumer: "
|
|
<< *consumer.getOperation());
|
|
for (auto dependence : graph.getDependencesInto(consumer, depType)) {
|
|
LLVM_DEBUG(dbgs() << "\n***Consider producer:\t"
|
|
<< *dependence.dependentOpView.op << "\n");
|
|
auto producer = cast<LinalgOp>(dependence.dependentOpView.op);
|
|
|
|
// Check that the dependence is indeed on the input `consumerIdx` view.
|
|
auto consumedView = dependence.indexingView;
|
|
if (!isSameSubView(consumer.getBuffer(consumerIdx), consumedView))
|
|
continue;
|
|
|
|
// Consumer consumes this view, `isStructurallyFusableProducer` also checks
|
|
// whether it is a strict subview of the producer view.
|
|
auto producedView = dependence.dependentOpView.view;
|
|
auto producerIdx = producer.getIndexOfOutputBuffer(producedView).getValue();
|
|
// `consumerIdx` and `producerIdx` exist by construction.
|
|
LLVM_DEBUG(dbgs() << "\n"
|
|
<< LinalgDependenceGraph::getDependenceTypeStr(depType)
|
|
<< "producer: " << *producer.getOperation() << " view: "
|
|
<< producedView << " output index: " << producerIdx);
|
|
|
|
// Must be a subview or a slice to guarantee there are loops we can fuse
|
|
// into.
|
|
auto subView = consumedView.getDefiningOp<SubViewOp>();
|
|
auto slice = consumedView.getDefiningOp<SliceOp>();
|
|
if (!subView && !slice) {
|
|
LLVM_DEBUG(dbgs() << "\nNot fusable (not a subview or slice)");
|
|
continue;
|
|
}
|
|
|
|
// Simple fusability checks.
|
|
if (!isFusableInto(graph, consumer, consumedView, producer))
|
|
continue;
|
|
|
|
// Fuse `producer` just before `consumer`.
|
|
OpBuilder::InsertionGuard g(b);
|
|
b.setInsertionPoint(consumer.getOperation());
|
|
ScopedContext scope(b, consumer.getLoc());
|
|
LLVM_DEBUG(dbgs() << "Fuse into consumer: " << *consumer << "\n");
|
|
auto fusedProducer = fuse(producedView, producer, consumer, consumerIdx,
|
|
producerIdx, folder);
|
|
|
|
return FusionInfo{producer, fusedProducer};
|
|
}
|
|
return llvm::None;
|
|
}
|
|
|
|
// Only consider RAW and WAW atm.
|
|
Optional<FusionInfo> mlir::linalg::fuseProducerOf(
|
|
OpBuilder &b, LinalgOp consumer, unsigned consumerIdx,
|
|
const LinalgDependenceGraph &graph, OperationFolder *folder) {
|
|
SmallVector<LinalgDependenceGraph::DependenceType, 4> deps = {
|
|
LinalgDependenceGraph::DependenceType::RAW,
|
|
LinalgDependenceGraph::DependenceType::WAW,
|
|
};
|
|
for (auto dep : deps) {
|
|
if (auto res =
|
|
fuseProducerOfDep(b, consumer, consumerIdx, graph, folder, dep))
|
|
return res;
|
|
}
|
|
return llvm::None;
|
|
}
|
|
|
|
static void fuseLinalgOpsGreedily(FuncOp f) {
|
|
LLVM_DEBUG(f.print(dbgs() << "\nBefore linalg-fusion: \n"));
|
|
|
|
OpBuilder b(f);
|
|
OperationFolder folder(f.getContext());
|
|
DenseSet<Operation *> eraseSet;
|
|
|
|
// Save original Linalg ops, we only want to make a pass over those.
|
|
SmallVector<Operation *, 8> linalgOps;
|
|
f.walk([&](LinalgOp op) {
|
|
if (op.hasBufferSemantics())
|
|
linalgOps.push_back(op);
|
|
});
|
|
|
|
// TODO: LinalgDependenceGraph should be able to update itself.
|
|
// The current naive and expensive reconstruction of the graph should be
|
|
// removed.
|
|
for (auto *op : llvm::reverse(linalgOps)) {
|
|
for (unsigned id = 0, e = LinalgOp(op).getNumInputsAndOutputBuffers();
|
|
id < e; ++id) {
|
|
linalg::Aliases aliases;
|
|
linalg::LinalgDependenceGraph graph(aliases, linalgOps);
|
|
if (auto info = fuseProducerOf(b, op, id, graph, &folder)) {
|
|
auto *originalOp = info->originalProducer.getOperation();
|
|
eraseSet.insert(originalOp);
|
|
auto *originalOpInLinalgOpsVector =
|
|
std::find(linalgOps.begin(), linalgOps.end(), originalOp);
|
|
*originalOpInLinalgOpsVector = info->fusedProducer.getOperation();
|
|
}
|
|
}
|
|
}
|
|
// The `fuseProducerOf` function performs structural checks and in particular
|
|
// that no covering read or write exist between the consumer and the producer.
|
|
// As a consequence, the only fusions that may occur preserve subsequent
|
|
// dependences and are guaranteed by construction to produce the whole view.
|
|
// We may thus erase the producer once it is fused.
|
|
for (auto *e : eraseSet)
|
|
e->erase();
|
|
LLVM_DEBUG(f.print(dbgs() << "\nAfter linalg-fusion: \n"));
|
|
}
|
|
|
|
//====---------------------------------------------------------------------===//
|
|
// Fusion on Tensor operation.
|
|
//====---------------------------------------------------------------------===//
|
|
|
|
namespace {
|
|
|
|
/// Implementation of fusion of generic ops and indexed_generic ops.
|
|
struct FuseGenericOpsOnTensors {
|
|
static bool isFusible(LinalgOp producer, LinalgOp consumer,
|
|
unsigned consumerIdx) {
|
|
// Verify that
|
|
// - the producer has all "parallel" iterator type.
|
|
if (producer.getNumParallelLoops() != producer.getNumLoops())
|
|
return false;
|
|
|
|
// Get the consumer index map. The number of results of the consumer index
|
|
// map must match the number of loops of the producer.
|
|
AffineMap consumerIndexMap = consumer.getIndexingMap(consumerIdx);
|
|
if (consumerIndexMap.getNumResults() != producer.getNumLoops())
|
|
return false;
|
|
|
|
// Finally the index_map for the result must be invertible. For now just
|
|
// verify it is a permutation.
|
|
AffineMap producerResultIndexMap = producer.getOutputIndexingMap(0);
|
|
return producerResultIndexMap.isPermutation();
|
|
}
|
|
|
|
static Operation *fuse(LinalgOp producer, LinalgOp consumer,
|
|
unsigned consumerIdx, PatternRewriter &rewriter,
|
|
OperationFolder *folder = nullptr) {
|
|
if (!isFusible(producer, consumer, consumerIdx))
|
|
return nullptr;
|
|
|
|
unsigned numFusedOperands = producer.getOperation()->getNumOperands() +
|
|
consumer.getOperation()->getNumOperands() - 1;
|
|
|
|
// Compute the fused operands list,
|
|
SmallVector<Value, 2> fusedOperands;
|
|
fusedOperands.reserve(numFusedOperands);
|
|
auto consumerOperands = consumer.getOperation()->getOperands();
|
|
auto producerOperands = producer.getOperation()->getOperands();
|
|
fusedOperands.assign(consumerOperands.begin(),
|
|
std::next(consumerOperands.begin(), consumerIdx));
|
|
fusedOperands.append(producerOperands.begin(), producerOperands.end());
|
|
fusedOperands.append(std::next(consumerOperands.begin(), consumerIdx + 1),
|
|
consumerOperands.end());
|
|
|
|
// Compute indexing_maps for the fused operation. The indexing_maps for the
|
|
// operands of the consumers that arent fused are the same. The
|
|
// indexing_maps for the producers need to be computed based on the
|
|
// indexing_map of the operand at consumerIdx in the consumer.
|
|
SmallVector<Attribute, 4> fusedIndexMaps;
|
|
auto consumerIndexMaps = consumer.indexing_maps();
|
|
fusedIndexMaps.reserve(fusedOperands.size() +
|
|
consumer.getOperation()->getNumResults());
|
|
fusedIndexMaps.assign(consumerIndexMaps.begin(),
|
|
std::next(consumerIndexMaps.begin(), consumerIdx));
|
|
// Compute indexing maps for the producer args in the fused operation.
|
|
computeProducerOperandIndex(
|
|
producer, consumer.getInputIndexingMap(consumerIdx), fusedIndexMaps);
|
|
|
|
// Append the indexing maps for the remaining consumer operands.
|
|
fusedIndexMaps.append(std::next(consumerIndexMaps.begin(), consumerIdx + 1),
|
|
consumerIndexMaps.end());
|
|
|
|
// Generate the fused op.
|
|
LinalgOp fusedOp;
|
|
if (isa<GenericOp>(producer.getOperation()) &&
|
|
isa<GenericOp>(consumer.getOperation())) {
|
|
fusedOp =
|
|
rewriter
|
|
.create<GenericOp>(
|
|
rewriter.getUnknownLoc(),
|
|
consumer.getOperation()->getResultTypes(), fusedOperands,
|
|
rewriter.getI64IntegerAttr(fusedOperands.size()),
|
|
rewriter.getI64IntegerAttr(
|
|
consumer.getOperation()->getNumResults()),
|
|
rewriter.getArrayAttr(fusedIndexMaps),
|
|
consumer.iterator_types(),
|
|
/*doc=*/nullptr,
|
|
/*library_call=*/nullptr,
|
|
/*symbol_source=*/nullptr)
|
|
.getOperation();
|
|
} else {
|
|
fusedOp =
|
|
rewriter
|
|
.create<IndexedGenericOp>(
|
|
rewriter.getUnknownLoc(),
|
|
consumer.getOperation()->getResultTypes(), fusedOperands,
|
|
rewriter.getI64IntegerAttr(fusedOperands.size()),
|
|
rewriter.getI64IntegerAttr(
|
|
consumer.getOperation()->getNumResults()),
|
|
rewriter.getArrayAttr(fusedIndexMaps),
|
|
consumer.iterator_types(),
|
|
/*doc=*/nullptr,
|
|
/*library_call=*/nullptr,
|
|
/*symbol_source=*/nullptr)
|
|
.getOperation();
|
|
}
|
|
|
|
// Construct an AffineMap from consumer loops to producer loops.
|
|
// consumer loop -> tensor index
|
|
AffineMap consumerResultIndexMap =
|
|
consumer.getInputIndexingMap(consumerIdx);
|
|
// producer loop -> tensor index
|
|
AffineMap producerResultIndexMap = producer.getOutputIndexingMap(0);
|
|
// tensor index -> producer loop
|
|
AffineMap invProducerResultIndexMap =
|
|
inversePermutation(producerResultIndexMap);
|
|
assert(invProducerResultIndexMap &&
|
|
"expected producer result indexig map to be invertible");
|
|
// consumer loop -> producer loop
|
|
AffineMap consumerToProducerLoopsMap =
|
|
invProducerResultIndexMap.compose(consumerResultIndexMap);
|
|
|
|
generateFusedRegion(rewriter, fusedOp, producer, consumer,
|
|
consumerToProducerLoopsMap, consumerIdx,
|
|
consumer.getNumLoops());
|
|
return fusedOp;
|
|
}
|
|
|
|
private:
|
|
/// Append to `fusedOpIndexingMapAttrs` the indexing maps for the operands of
|
|
/// the `producer` to use in the fused operation given the indexing map of the
|
|
/// result of the producer in the consumer.
|
|
static void computeProducerOperandIndex(
|
|
LinalgOp producer, AffineMap fusedConsumerArgIndexMap,
|
|
SmallVectorImpl<Attribute> &fusedOpIndexingMapAttrs) {
|
|
// The indexing map in the consumer op (fusedConsumerArgIndexMap) is a map
|
|
// from consumer loop -> consumer arg tensor index/producer result tensor
|
|
// index. The fused loop is same as the consumer loop. For each producer arg
|
|
// the indexing map to be computed is a map from consumer loop -> producer
|
|
// arg tensor index.
|
|
|
|
AffineMap producerResultIndexMap = producer.getOutputIndexingMap(0);
|
|
// producerResultIndexMap is a map from producer loop -> tensor index.
|
|
// Compute the inverse to get map from tensor index -> producer loop.
|
|
// The inverse is a map from producer result tensor index -> producer loop.
|
|
AffineMap invProducerResultIndexMap =
|
|
inversePermutation(producerResultIndexMap);
|
|
assert(invProducerResultIndexMap &&
|
|
"expected producer result indexig map to be invertible");
|
|
for (unsigned argNum : llvm::seq<unsigned>(0, producer.getNumInputs())) {
|
|
// argMap is a map from producer loop -> producer arg tensor index.
|
|
AffineMap argMap = producer.getInputIndexingMap(argNum);
|
|
|
|
// Compose argMap with invProducerResultIndexMap to get a map from
|
|
// producer result tensor index -> producer arg tensor index.
|
|
AffineMap t1 = argMap.compose(invProducerResultIndexMap);
|
|
|
|
// Compose t1 with fusedConsumerArgIndexMap gives an indexing map from
|
|
// consumer loop/ fused loop -> producer arg tensor index.
|
|
AffineMap indexingMap = t1.compose(fusedConsumerArgIndexMap);
|
|
fusedOpIndexingMapAttrs.push_back(AffineMapAttr::get(indexingMap));
|
|
}
|
|
}
|
|
|
|
/// Generate the region of the fused operation. The region of the fused op
|
|
/// must be empty.
|
|
static void generateFusedRegion(PatternRewriter &rewriter, Operation *fusedOp,
|
|
LinalgOp producer, LinalgOp consumer,
|
|
AffineMap consumerToProducerLoopsMap,
|
|
unsigned consumerIdx, unsigned nloops) {
|
|
// Build the region of the fused op.
|
|
Block &producerBlock = producer.getOperation()->getRegion(0).front();
|
|
Block &consumerBlock = consumer.getOperation()->getRegion(0).front();
|
|
Block *fusedBlock = new Block();
|
|
fusedOp->getRegion(0).push_back(fusedBlock);
|
|
BlockAndValueMapping mapper;
|
|
OpBuilder::InsertionGuard guard(rewriter);
|
|
rewriter.setInsertionPointToStart(fusedBlock);
|
|
|
|
// The block arguments are
|
|
// [index_0, index_1, ... ,
|
|
// consumer_operand_0, ... , consumer_operand_(`consumerIdx`-1),
|
|
// producer_operand_0, ... , producer_operand_(n-1)],
|
|
// consumer_operand_(`consumerIdx`), .. consumer_operand_(m-1)]
|
|
// , where n is the number of producer's operand and m is the number
|
|
// consumer's operand.
|
|
// If both `numProducerIndices` and `numConsumerIndices` are zero, this is a
|
|
// generic op. In this case, there are no indices in block arguments.
|
|
unsigned numProducerIndices =
|
|
isa<IndexedGenericOp>(producer.getOperation()) ? nloops : 0;
|
|
unsigned numConsumerIndices =
|
|
isa<IndexedGenericOp>(consumer.getOperation()) ? nloops : 0;
|
|
// Firstly, add all the indices to the block arguments.
|
|
for (unsigned i = 0, e = std::max(numProducerIndices, numConsumerIndices);
|
|
i < e; ++i)
|
|
fusedBlock->addArgument(rewriter.getIndexType());
|
|
// Map the arguments for the unmodified args from the consumer.
|
|
for (auto consumerArg : llvm::enumerate(consumerBlock.getArguments())) {
|
|
if (consumerArg.index() == consumerIdx + numConsumerIndices) {
|
|
// Map the arguments for the args from the producer.
|
|
for (auto producerArg : llvm::enumerate(producerBlock.getArguments())) {
|
|
// If producer is an indexed_generic op, map the indices from consumer
|
|
// loop to producer loop (because the fusedOp is built based on
|
|
// consumer's perspective).
|
|
if (producerArg.index() < numProducerIndices) {
|
|
auto newIndex = rewriter.create<mlir::AffineApplyOp>(
|
|
producer.getLoc(),
|
|
consumerToProducerLoopsMap.getSubMap(producerArg.index()),
|
|
fusedBlock->getArguments().take_front(nloops));
|
|
mapper.map(producerArg.value(), newIndex);
|
|
} else {
|
|
mapper.map(producerArg.value(),
|
|
fusedBlock->addArgument(producerArg.value().getType()));
|
|
}
|
|
}
|
|
continue;
|
|
}
|
|
|
|
// If consumer is an indexed_generic op, map the indices to the block
|
|
// arguments directly. Otherwise, add the same type of arugment and map to
|
|
// it.
|
|
if (consumerArg.index() < numConsumerIndices) {
|
|
mapper.map(consumerArg.value(),
|
|
fusedBlock->getArgument(consumerArg.index()));
|
|
} else {
|
|
mapper.map(consumerArg.value(),
|
|
fusedBlock->addArgument(consumerArg.value().getType()));
|
|
}
|
|
}
|
|
|
|
// Add operations from producer (except the yield operation) to the fused
|
|
// op.
|
|
for (auto &op : producerBlock.getOperations()) {
|
|
if (auto yieldOp = dyn_cast<YieldOp>(op)) {
|
|
// Lookup the value the yield operation is mapped to.
|
|
Value yieldVal = yieldOp.getOperand(0);
|
|
if (Value clonedVal = mapper.lookupOrNull(yieldVal))
|
|
mapper.map(
|
|
consumerBlock.getArgument(consumerIdx + numConsumerIndices),
|
|
clonedVal);
|
|
continue;
|
|
}
|
|
rewriter.clone(op, mapper);
|
|
}
|
|
for (auto &op : consumerBlock.getOperations())
|
|
rewriter.clone(op, mapper);
|
|
}
|
|
};
|
|
} // namespace
|
|
|
|
/// Linearize the expressions in `sourceMap` based on the `reassociationMaps`
|
|
/// provided, given the shape of the source tensor that corresponds to the
|
|
/// `sourceMap`. Note that this implicitly assumes that the tensors dimensions
|
|
/// are "row-major" ordered logically.
|
|
///
|
|
/// For example:
|
|
///
|
|
/// %0 = op ... : tensor<?x?x4x5xf32>
|
|
/// with output index_map `affine_map<(d0, d1, d2, d3) -> (d0, d1, d2, d3)>`
|
|
///
|
|
/// and reshape:
|
|
/// %1 = linalg.tensor_reshape %0 [affine_map<(i, j, k, l) -> (i)>,
|
|
/// affine_map<(i, j, k, l) -> (j, k, l)>] :
|
|
/// tensor<?x?x4x5xf32> into tensor<?x?xf32>
|
|
///
|
|
/// would be rewritten into:
|
|
/// %0 = op ... : tensor<?x?x4x5xf32>
|
|
/// with output index_map
|
|
/// `affine_map<(d0, d1, d2, d3) -> (d0, d1 * 20 + d2 * 5 + d3)>`
|
|
static AffineMap linearizeCollapsedDims(AffineMap sourceMap,
|
|
ArrayRef<int64_t> sourceShape,
|
|
ArrayRef<AffineMap> reassociationMaps) {
|
|
SmallVector<AffineExpr, 4> resultExprs;
|
|
resultExprs.reserve(reassociationMaps.size());
|
|
ArrayRef<AffineExpr> sourceExprs = sourceMap.getResults();
|
|
MLIRContext *context = sourceMap.getContext();
|
|
|
|
// Compute the result exprs based on the reassociation maps.
|
|
for (AffineMap map : reassociationMaps) {
|
|
ArrayRef<AffineExpr> collapsedDims = map.getResults();
|
|
// Assume that they are in-order and contiguous (already checked in
|
|
// verifier).
|
|
assert(!collapsedDims.empty());
|
|
unsigned startDim =
|
|
collapsedDims.front().cast<AffineDimExpr>().getPosition();
|
|
AffineExpr linearizedExpr = makeCanonicalStridedLayoutExpr(
|
|
sourceShape.slice(startDim, collapsedDims.size()),
|
|
sourceExprs.slice(startDim, collapsedDims.size()), context);
|
|
resultExprs.push_back(linearizedExpr);
|
|
}
|
|
return AffineMap::get(sourceMap.getNumDims(), sourceMap.getNumSymbols(),
|
|
resultExprs, context);
|
|
}
|
|
|
|
/// Checks if the `reshapeOp` can be fused with it consumer (if `asProducer` is
|
|
/// true) or its producer (if `asProducer` is false) given the indexing map at
|
|
/// its use.
|
|
static bool isTensorReshapeOpFusible(TensorReshapeOp reshapeOp,
|
|
AffineMap useIndexMap, bool asProducer) {
|
|
RankedTensorType returnType = reshapeOp.getResultType();
|
|
RankedTensorType operandType = reshapeOp.getSrcType();
|
|
// Reshape is fusible with its consumer (i.e. reshape as a producer) when its
|
|
// operand is of lesser rank than the result. Fusing when operand has higher
|
|
// rank will require use of mods and divs in the indexing maps of the fused op
|
|
// which would make it non-invertible. Similarly reshape is fused with its
|
|
// producer (i.e. reshape as consumer) only if the return type has lesser
|
|
// rank.
|
|
if ((asProducer && returnType.getRank() < operandType.getRank()) ||
|
|
(!asProducer && operandType.getRank() < returnType.getRank()))
|
|
return false;
|
|
return useIndexMap.isIdentity();
|
|
}
|
|
|
|
namespace {
|
|
/// Implementation of fusion on tensor ops when producer is a TensorReshapeOp.
|
|
template <typename LinalgOpTy> struct FuseTensorReshapeOpAsProducer {
|
|
static bool isFusible(TensorReshapeOp producer, LinalgOpTy consumer,
|
|
unsigned consumerIdx) {
|
|
return isTensorReshapeOpFusible(
|
|
producer, consumer.getInputIndexingMap(consumerIdx), true);
|
|
}
|
|
|
|
static Operation *fuse(TensorReshapeOp producer, LinalgOpTy consumer,
|
|
unsigned consumerIdx, PatternRewriter &rewriter,
|
|
OperationFolder *folder = nullptr) {
|
|
if (!isFusible(producer, consumer, consumerIdx))
|
|
return nullptr;
|
|
|
|
// Compute the fused operands list,
|
|
SmallVector<Value, 2> fusedOperands(consumer.operand_begin(),
|
|
consumer.operand_end());
|
|
fusedOperands[consumerIdx] = producer.src();
|
|
|
|
// Compute indexing_maps for the fused operation. The indexing_maps for the
|
|
// operands of the consumers that arent fused are the same.
|
|
SmallVector<AffineMap, 4> fusedIndexMaps =
|
|
llvm::to_vector<4>(llvm::map_range(
|
|
consumer.indexing_maps(), [](Attribute attr) -> AffineMap {
|
|
return attr.cast<AffineMapAttr>().getValue();
|
|
}));
|
|
|
|
// Compute the indexing map to use for the operand of the producer.
|
|
AffineMap modifiedMap = linearizeCollapsedDims(
|
|
fusedIndexMaps[consumerIdx], producer.getResultType().getShape(),
|
|
producer.getReassociationMaps());
|
|
for (AffineExpr expr : modifiedMap.getResults()) {
|
|
if (!expr.isPureAffine())
|
|
return nullptr;
|
|
}
|
|
fusedIndexMaps[consumerIdx] = modifiedMap;
|
|
|
|
// Further check that the resulting index maps can be fused and
|
|
// inverted. Without this the resultant op is not legal.
|
|
if (!inversePermutation(concatAffineMaps(fusedIndexMaps)))
|
|
return nullptr;
|
|
|
|
SmallVector<Attribute, 4> indexMapAttrs = llvm::to_vector<4>(
|
|
llvm::map_range(fusedIndexMaps, [](AffineMap map) -> Attribute {
|
|
return AffineMapAttr::get(map);
|
|
}));
|
|
auto fusedOp = rewriter.create<LinalgOpTy>(
|
|
rewriter.getUnknownLoc(), consumer.getResultTypes(), fusedOperands,
|
|
rewriter.getI64IntegerAttr(fusedOperands.size()),
|
|
rewriter.getI64IntegerAttr(consumer.getNumResults()),
|
|
rewriter.getArrayAttr(indexMapAttrs), consumer.iterator_types(),
|
|
/*doc=*/nullptr,
|
|
/*library_call=*/nullptr,
|
|
/*symbol_source=*/nullptr);
|
|
auto &fusedRegion = fusedOp.region();
|
|
rewriter.cloneRegionBefore(consumer.region(), fusedRegion,
|
|
fusedRegion.begin());
|
|
return fusedOp;
|
|
}
|
|
};
|
|
|
|
/// Implementation of fusion on tensor ops when consumer is a TensorReshapeOp.
|
|
template <typename LinalgOpTy> struct FuseTensorReshapeOpAsConsumer {
|
|
static bool isFusible(LinalgOpTy producer, TensorReshapeOp consumer,
|
|
unsigned consumerIdx) {
|
|
return isTensorReshapeOpFusible(consumer, producer.getOutputIndexingMap(0),
|
|
false);
|
|
}
|
|
|
|
static Operation *fuse(LinalgOpTy producer, TensorReshapeOp consumer,
|
|
unsigned consumerIdx, PatternRewriter &rewriter,
|
|
OperationFolder *folder = nullptr) {
|
|
if (!isFusible(producer, consumer, consumerIdx))
|
|
return nullptr;
|
|
|
|
// The indexing_maps for the operands of the fused operation are same as
|
|
// those for the operands of the producer.
|
|
SmallVector<AffineMap, 4> fusedIndexMaps =
|
|
llvm::to_vector<4>(llvm::map_range(
|
|
producer.indexing_maps(), [](Attribute attr) -> AffineMap {
|
|
return attr.cast<AffineMapAttr>().getValue();
|
|
}));
|
|
// Compute the indexing map to use for the operand of the producer.
|
|
AffineMap modifiedMap = linearizeCollapsedDims(
|
|
producer.getOutputIndexingMap(0), consumer.getSrcType().getShape(),
|
|
consumer.getReassociationMaps());
|
|
for (AffineExpr expr : modifiedMap.getResults()) {
|
|
if (!expr.isPureAffine())
|
|
return nullptr;
|
|
}
|
|
fusedIndexMaps.back() = modifiedMap;
|
|
|
|
// Further check that the resulting index maps can be fused and
|
|
// inverted. Without this the resultant op is not legal.
|
|
if (!inversePermutation(concatAffineMaps(fusedIndexMaps)))
|
|
return nullptr;
|
|
|
|
SmallVector<Attribute, 4> indexMapAttrs = llvm::to_vector<4>(
|
|
llvm::map_range(fusedIndexMaps, [](AffineMap map) -> Attribute {
|
|
return AffineMapAttr::get(map);
|
|
}));
|
|
|
|
auto fusedOp = rewriter.create<LinalgOpTy>(
|
|
rewriter.getUnknownLoc(), consumer.getResultType(),
|
|
producer.getOperands(),
|
|
rewriter.getI64IntegerAttr(producer.getNumOperands()),
|
|
rewriter.getI64IntegerAttr(1), rewriter.getArrayAttr(indexMapAttrs),
|
|
producer.iterator_types(),
|
|
/*doc=*/nullptr,
|
|
/*library_call=*/nullptr,
|
|
/*symbol_source=*/nullptr);
|
|
auto &fusedRegion = fusedOp.region();
|
|
rewriter.cloneRegionBefore(producer.region(), fusedRegion,
|
|
fusedRegion.begin());
|
|
return fusedOp;
|
|
}
|
|
};
|
|
|
|
/// Implementation of fusion on tensor ops when producer is a splat constant.
|
|
template <typename LinalgOpTy> struct FuseConstantOpAsProducer {
|
|
static bool isFusible(ConstantOp producer, LinalgOpTy consumer,
|
|
unsigned consumerIdx) {
|
|
return producer.getResult().getType().isa<RankedTensorType>() &&
|
|
producer.value().template cast<DenseElementsAttr>().isSplat();
|
|
}
|
|
|
|
static Operation *fuse(ConstantOp producer, LinalgOpTy consumer,
|
|
unsigned consumerIdx, PatternRewriter &rewriter,
|
|
OperationFolder *folder = nullptr) {
|
|
if (!isFusible(producer, consumer, consumerIdx))
|
|
return nullptr;
|
|
|
|
// The indexing_maps for the operands of the fused operation are same as
|
|
// those for the operands of the consumer without the indexing map at
|
|
// consumerIdx
|
|
SmallVector<AffineMap, 4> fusedIndexMaps =
|
|
llvm::to_vector<4>(llvm::map_range(
|
|
consumer.indexing_maps(), [](Attribute attr) -> AffineMap {
|
|
return attr.cast<AffineMapAttr>().getValue();
|
|
}));
|
|
fusedIndexMaps.erase(std::next(fusedIndexMaps.begin(), consumerIdx));
|
|
|
|
// The operands list is same as the consumer with the argument for constant
|
|
// index dropped.
|
|
SmallVector<Value, 4> fusedOperands(consumer.operand_begin(),
|
|
consumer.operand_end());
|
|
fusedOperands.erase(std::next(fusedOperands.begin(), consumerIdx));
|
|
|
|
// Create a constant scalar value from the splat constant.
|
|
Value scalarConstant = rewriter.create<ConstantOp>(
|
|
producer.getLoc(),
|
|
producer.value().template cast<DenseElementsAttr>().getSplatValue());
|
|
|
|
auto fusedOp = rewriter.create<LinalgOpTy>(
|
|
rewriter.getUnknownLoc(), consumer.getResultTypes(), fusedOperands,
|
|
rewriter.getI64IntegerAttr(consumer.getNumOperands() - 1),
|
|
rewriter.getI64IntegerAttr(consumer.getNumResults()),
|
|
rewriter.getAffineMapArrayAttr(fusedIndexMaps),
|
|
consumer.iterator_types(),
|
|
/*doc=*/nullptr,
|
|
/*library_call=*/nullptr,
|
|
/*symbol_source=*/nullptr);
|
|
|
|
// Map the block argument corresponding to the replaced argument with the
|
|
// scalar constant.
|
|
Region &consumerRegion = consumer.region();
|
|
Block &entryBlock = *consumerRegion.begin();
|
|
unsigned argIndex =
|
|
entryBlock.getNumArguments() - consumer.getNumOperands() + consumerIdx;
|
|
BlockAndValueMapping mapping;
|
|
mapping.map(entryBlock.getArgument(argIndex), scalarConstant);
|
|
Region &fusedRegion = fusedOp.region();
|
|
rewriter.cloneRegionBefore(consumerRegion, fusedRegion, fusedRegion.begin(),
|
|
mapping);
|
|
return fusedOp;
|
|
}
|
|
};
|
|
|
|
} // namespace
|
|
|
|
Operation *mlir::linalg::fuseTensorOps(PatternRewriter &rewriter,
|
|
Operation *consumer,
|
|
unsigned consumerIdx,
|
|
OperationFolder *folder) {
|
|
if (consumerIdx >= consumer->getNumOperands())
|
|
return nullptr;
|
|
Operation *producer = consumer->getOperand(consumerIdx).getDefiningOp();
|
|
if (!producer || producer->getNumResults() != 1)
|
|
return nullptr;
|
|
|
|
// Fuse when consumer is GenericOp or IndexedGenericOp.
|
|
if (isa<GenericOp, IndexedGenericOp>(consumer)) {
|
|
auto linalgOpConsumer = cast<LinalgOp>(consumer);
|
|
if (!linalgOpConsumer.hasTensorSemantics())
|
|
return nullptr;
|
|
if (isa<GenericOp, IndexedGenericOp>(producer)) {
|
|
auto linalgOpProducer = cast<LinalgOp>(producer);
|
|
if (linalgOpProducer.hasTensorSemantics())
|
|
return FuseGenericOpsOnTensors::fuse(linalgOpProducer, linalgOpConsumer,
|
|
consumerIdx, rewriter, folder);
|
|
} else if (auto reshapeOpProducer = dyn_cast<TensorReshapeOp>(producer)) {
|
|
if (auto genericOpConsumer = dyn_cast<GenericOp>(consumer)) {
|
|
return FuseTensorReshapeOpAsProducer<GenericOp>::fuse(
|
|
reshapeOpProducer, genericOpConsumer, consumerIdx, rewriter,
|
|
folder);
|
|
} else if (auto indexedGenericOpConsumer =
|
|
dyn_cast<IndexedGenericOp>(consumer)) {
|
|
return FuseTensorReshapeOpAsProducer<IndexedGenericOp>::fuse(
|
|
reshapeOpProducer, indexedGenericOpConsumer, consumerIdx, rewriter,
|
|
folder);
|
|
}
|
|
} else if (auto constantOpProducer = dyn_cast<ConstantOp>(producer)) {
|
|
if (auto genericOpConsumer = dyn_cast<GenericOp>(consumer)) {
|
|
return FuseConstantOpAsProducer<GenericOp>::fuse(
|
|
constantOpProducer, genericOpConsumer, consumerIdx, rewriter,
|
|
folder);
|
|
}
|
|
}
|
|
return nullptr;
|
|
}
|
|
|
|
// Fuse when consumer is a TensorReshapeOp.
|
|
if (TensorReshapeOp reshapeOp = dyn_cast<TensorReshapeOp>(consumer)) {
|
|
if (auto genericOpProducer = dyn_cast<GenericOp>(producer)) {
|
|
if (genericOpProducer.hasTensorSemantics())
|
|
return FuseTensorReshapeOpAsConsumer<GenericOp>::fuse(
|
|
genericOpProducer, reshapeOp, consumerIdx, rewriter, folder);
|
|
} else if (auto indexedGenericOpProducer =
|
|
dyn_cast<IndexedGenericOp>(producer)) {
|
|
if (indexedGenericOpProducer.hasTensorSemantics())
|
|
return FuseTensorReshapeOpAsConsumer<IndexedGenericOp>::fuse(
|
|
indexedGenericOpProducer, reshapeOp, consumerIdx, rewriter, folder);
|
|
}
|
|
return nullptr;
|
|
}
|
|
|
|
return nullptr;
|
|
}
|
|
|
|
namespace {
|
|
/// Patterns to fuse a generic op, with the producer of its operands.
|
|
template <typename LinalgOpTy>
|
|
struct FuseTensorOps : public OpRewritePattern<LinalgOpTy> {
|
|
using OpRewritePattern<LinalgOpTy>::OpRewritePattern;
|
|
|
|
LogicalResult matchAndRewrite(LinalgOpTy op,
|
|
PatternRewriter &rewriter) const override {
|
|
// Find the first operand that is defined by another generic op on tensors.
|
|
for (auto operandNum :
|
|
llvm::seq<unsigned>(0, op.getOperation()->getNumOperands())) {
|
|
Operation *producer =
|
|
op.getOperation()->getOperand(operandNum).getDefiningOp();
|
|
if (Operation *fusedOp = fuseTensorOps(rewriter, op, operandNum)) {
|
|
rewriter.replaceOp(op, fusedOp->getResults());
|
|
if (producer && llvm::all_of(producer->getResults(),
|
|
[](Value val) { return val.use_empty(); }))
|
|
rewriter.eraseOp(producer);
|
|
return success();
|
|
}
|
|
}
|
|
return failure();
|
|
}
|
|
};
|
|
|
|
/// Pass that fuses generic ops on tensors. Used only for testing.
|
|
struct FusionOfTensorOpsPass
|
|
: public LinalgFusionOfTensorOpsBase<FusionOfTensorOpsPass> {
|
|
void runOnOperation() override {
|
|
OwningRewritePatternList patterns;
|
|
Operation *op = getOperation();
|
|
populateLinalgTensorOpsFusionPatterns(op->getContext(), patterns);
|
|
applyPatternsAndFoldGreedily(op->getRegions(), patterns);
|
|
};
|
|
};
|
|
|
|
struct LinalgFusionPass : public LinalgFusionBase<LinalgFusionPass> {
|
|
void runOnFunction() override { fuseLinalgOpsGreedily(getFunction()); }
|
|
};
|
|
} // namespace
|
|
|
|
void mlir::populateLinalgTensorOpsFusionPatterns(
|
|
MLIRContext *context, OwningRewritePatternList &patterns) {
|
|
patterns.insert<FuseTensorOps<GenericOp>, FuseTensorOps<IndexedGenericOp>,
|
|
FuseTensorOps<TensorReshapeOp>>(context);
|
|
}
|
|
|
|
std::unique_ptr<OperationPass<FuncOp>> mlir::createLinalgFusionPass() {
|
|
return std::make_unique<LinalgFusionPass>();
|
|
}
|
|
|
|
std::unique_ptr<Pass> mlir::createLinalgFusionOfTensorOpsPass() {
|
|
return std::make_unique<FusionOfTensorOpsPass>();
|
|
}
|