mirror of
https://github.com/ROCm/jax.git
synced 2025-04-16 03:46:06 +00:00
DOC: use jupytext to maintain synced markdown versions of notebooks.
This commit is contained in:
parent
e46700d1e3
commit
6eedadc27f
10
.github/workflows/ci-build.yaml
vendored
10
.github/workflows/ci-build.yaml
vendored
@ -11,6 +11,16 @@ on:
|
||||
- master
|
||||
|
||||
jobs:
|
||||
pre-commit:
|
||||
runs-on: ubuntu-latest
|
||||
steps:
|
||||
- uses: actions/checkout@v2
|
||||
- name: Set up Python 3.8
|
||||
uses: actions/setup-python@v1
|
||||
with:
|
||||
python-version: 3.8
|
||||
- uses: pre-commit/action@v2.0.0
|
||||
|
||||
lint_and_typecheck:
|
||||
runs-on: ubuntu-latest
|
||||
strategy:
|
||||
|
@ -1 +1,15 @@
|
||||
# Placeholder for pre-commit configurations.
|
||||
# Install the pre-commit hooks below with
|
||||
# 'pre-commit install'
|
||||
|
||||
# Auto-update the version of the hooks with
|
||||
# 'pre-commit autoupdate'
|
||||
|
||||
# Run the hooks on all files with
|
||||
# 'pre-commit run --all'
|
||||
|
||||
repos:
|
||||
- repo: https://github.com/mwouts/jupytext
|
||||
rev: v1.10.0
|
||||
hooks:
|
||||
- id: jupytext
|
||||
args: [--sync]
|
||||
|
@ -109,8 +109,10 @@ exclude_patterns = [
|
||||
'notebooks/XLA_in_Python.ipynb',
|
||||
# Sometimes sphinx reads its own outputs as inputs!
|
||||
'build/html',
|
||||
'notebooks/README.md',
|
||||
'README.md',
|
||||
# Ignore markdown source for notebooks; nbsphinx builds from the ipynb
|
||||
# These are kept in sync using the jupytext pre-commit hook.
|
||||
'notebooks/*.md'
|
||||
]
|
||||
|
||||
# The name of the Pygments (syntax highlighting) style to use.
|
||||
|
@ -201,11 +201,41 @@ You can then see the generated documentation in
|
||||
|
||||
Update notebooks
|
||||
----------------
|
||||
We use [jupytext](https://jupytext.readthedocs.io/) to maintain two synced copies of the notebooks
|
||||
in `docs/notebooks`: one in `ipynb` format, and one in `md` format. The advantage of the former
|
||||
is that it can be opened and executed directly in Colab; the advantage of the latter is that
|
||||
it makes it much easier to track diffs within version control.
|
||||
|
||||
Open the notebook with http://colab.research.google.com (then `Upload` from your
|
||||
local repo), update it as needed, ``Run all cells`` then
|
||||
``Download ipynb``. You may want to test that it executes properly, using ``sphinx-build`` as
|
||||
explained above.
|
||||
Editing ipynb
|
||||
.............
|
||||
For making large changes that substantially modify code and outputs, it is easiest to
|
||||
edit the notebooks in Jupyter or in Colab. To edit notebooks in the Colab interface,
|
||||
open http://colab.research.google.com and `Upload` from your local repo.
|
||||
Update it as needed, ``Run all cells`` then ``Download ipynb``.
|
||||
You may want to test that it executes properly, using ``sphinx-build`` as explained above.
|
||||
|
||||
Editing md
|
||||
..........
|
||||
For making smaller changes to the text content of the notebooks, it is easiest to edit the
|
||||
``.md`` versions using a text editor.
|
||||
|
||||
Syncing notebooks
|
||||
.................
|
||||
After editing either the ipynb or md versions of the notebooks, you can sync the two versions
|
||||
using [jupytext](https://jupytext.readthedocs.io/) by running::
|
||||
|
||||
$ jupytext --sync docs/notebooks/*
|
||||
|
||||
Alternatively, you can run this command via the [pre-commit](https://pre-commit.com/)
|
||||
framework by executing the folloing in the main JAX directory:
|
||||
|
||||
$ pre-commit run --all
|
||||
|
||||
See the pre-commit framework documentation for information on how to set your local git
|
||||
environment to execute this automatically.
|
||||
|
||||
Notebooks within the sphinx build
|
||||
.................................
|
||||
|
||||
Some of the notebooks are built automatically as part of the Travis pre-submit checks and
|
||||
as part of the `Read the docs <https://jax.readthedocs.io/en/latest>`_ build.
|
||||
|
@ -49,14 +49,6 @@
|
||||
"rcParams['axes.grid'] = False"
|
||||
]
|
||||
},
|
||||
{
|
||||
"cell_type": "markdown",
|
||||
"metadata": {
|
||||
"colab_type": "text",
|
||||
"id": "cxwbr3XK2_mK"
|
||||
},
|
||||
"source": []
|
||||
},
|
||||
{
|
||||
"cell_type": "markdown",
|
||||
"metadata": {
|
||||
@ -76,7 +68,7 @@
|
||||
"source": [
|
||||
"JAX transformation and compilation are designed to work only on Python functions that are functionally pure: all the input data is passed through the function parameters, all the results are output through the function results. A pure function will always return the same result if invoked with the same inputs. \n",
|
||||
"\n",
|
||||
"Here are some examples of functions that are not functially pure for which JAX behaves differently than the Python interpreter. Note that these behaviors are not guaranteed by the JAX system; the proper way to use JAX is to use it only on functionally pure Python functions.\n"
|
||||
"Here are some examples of functions that are not functially pure for which JAX behaves differently than the Python interpreter. Note that these behaviors are not guaranteed by the JAX system; the proper way to use JAX is to use it only on functionally pure Python functions."
|
||||
]
|
||||
},
|
||||
{
|
||||
@ -218,7 +210,6 @@
|
||||
},
|
||||
"outputs": [],
|
||||
"source": [
|
||||
"\n",
|
||||
"def pure_uses_internal_state(x):\n",
|
||||
" state = dict(even=0, odd=0)\n",
|
||||
" for i in range(10):\n",
|
||||
@ -603,7 +594,7 @@
|
||||
"id": "eoXrGARWypdR"
|
||||
},
|
||||
"source": [
|
||||
"However, raising an error on other accelerators can be more difficult. Therefore, JAX does not raise an error, instead the index is clamped to the bounds of the array, meaning that for this example the last value of the array will be returned. "
|
||||
"However, raising an error on other accelerators can be more difficult. Therefore, JAX does not raise an error, instead the index is clamped to the bounds of the array, meaning that for this example the last value of the array will be returned."
|
||||
]
|
||||
},
|
||||
{
|
||||
@ -784,7 +775,7 @@
|
||||
"source": [
|
||||
"The problem with magic PRNG state is that it's hard to reason about how it's being used and updated across different threads, processes, and devices, and it's _very easy_ to screw up when the details of entropy production and consumption are hidden from the end user.\n",
|
||||
"\n",
|
||||
"The Mersenne Twister PRNG is also known to have a [number](https://cs.stackexchange.com/a/53475) of problems, it has a large 2.5Kb state size, which leads to problematic [initialization issues](https://dl.acm.org/citation.cfm?id=1276928). It [fails](http://www.pcg-random.org/pdf/toms-oneill-pcg-family-v1.02.pdf) modern BigCrush tests, and is generally slow. "
|
||||
"The Mersenne Twister PRNG is also known to have a [number](https://cs.stackexchange.com/a/53475) of problems, it has a large 2.5Kb state size, which leads to problematic [initialization issues](https://dl.acm.org/citation.cfm?id=1276928). It [fails](http://www.pcg-random.org/pdf/toms-oneill-pcg-family-v1.02.pdf) modern BigCrush tests, and is generally slow."
|
||||
]
|
||||
},
|
||||
{
|
||||
@ -804,7 +795,6 @@
|
||||
"id": "COjzGBpO4tzL"
|
||||
},
|
||||
"source": [
|
||||
"\n",
|
||||
"JAX instead implements an _explicit_ PRNG where entropy production and consumption are handled by explicitly passing and iterating PRNG state. JAX uses a modern [Threefry counter-based PRNG](https://github.com/google/jax/blob/master/design_notes/prng.md) that's __splittable__. That is, its design allows us to __fork__ the PRNG state into new PRNGs for use with parallel stochastic generation.\n",
|
||||
"\n",
|
||||
"The random state is described by two unsigned-int32s that we call a __key__:"
|
||||
@ -1374,7 +1364,7 @@
|
||||
"source": [
|
||||
"`static_argnums` can be handy if `length` in our example rarely changes, but it would be disastrous if it changed a lot! \n",
|
||||
"\n",
|
||||
"Lastly, if your function has global side-effects, JAX's tracer can cause weird things to happen. A common gotcha is trying to print arrays inside __jit__'d functions: "
|
||||
"Lastly, if your function has global side-effects, JAX's tracer can cause weird things to happen. A common gotcha is trying to print arrays inside __jit__'d functions:"
|
||||
]
|
||||
},
|
||||
{
|
||||
@ -1435,8 +1425,7 @@
|
||||
" - `lax.cond` _differentiable_\n",
|
||||
" - `lax.while_loop` __fwd-mode-differentiable__\n",
|
||||
" - `lax.fori_loop` __fwd-mode-differentiable__\n",
|
||||
" - `lax.scan` _differentiable_\n",
|
||||
"\n"
|
||||
" - `lax.scan` _differentiable_"
|
||||
]
|
||||
},
|
||||
{
|
||||
@ -2784,6 +2773,9 @@
|
||||
"provenance": [],
|
||||
"toc_visible": true
|
||||
},
|
||||
"jupytext": {
|
||||
"formats": "ipynb,md:myst"
|
||||
},
|
||||
"kernelspec": {
|
||||
"display_name": "Python 3",
|
||||
"language": "python",
|
||||
|
1509
docs/notebooks/Common_Gotchas_in_JAX.md
Normal file
1509
docs/notebooks/Common_Gotchas_in_JAX.md
Normal file
File diff suppressed because it is too large
Load Diff
File diff suppressed because one or more lines are too long
1414
docs/notebooks/Custom_derivative_rules_for_Python_code.md
Normal file
1414
docs/notebooks/Custom_derivative_rules_for_Python_code.md
Normal file
File diff suppressed because it is too large
Load Diff
File diff suppressed because it is too large
Load Diff
881
docs/notebooks/How_JAX_primitives_work.md
Normal file
881
docs/notebooks/How_JAX_primitives_work.md
Normal file
@ -0,0 +1,881 @@
|
||||
---
|
||||
jupytext:
|
||||
formats: ipynb,md:myst
|
||||
text_representation:
|
||||
extension: .md
|
||||
format_name: myst
|
||||
format_version: 0.13
|
||||
jupytext_version: 1.10.0
|
||||
kernelspec:
|
||||
display_name: Python 3
|
||||
name: python3
|
||||
---
|
||||
|
||||
+++ {"id": "vfxqky4PCUnh", "colab_type": "text"}
|
||||
|
||||
# How JAX primitives work
|
||||
|
||||
*necula@google.com*, October 2019.
|
||||
|
||||
JAX implements certain transformations of Python functions, e.g., `jit`, `grad`,
|
||||
`vmap`, or `pmap`. The Python functions to be transformed must be JAX-traceable,
|
||||
which means that as the Python function executes
|
||||
the only operations it applies to the data are either inspections of data
|
||||
attributes such as shape or type, or special operations called JAX primitives.
|
||||
In particular, a JAX-traceable function is sometimes invoked by JAX with
|
||||
abstract arguments. An example of a JAX abstract value is `ShapedArray(float32[2,2])`,
|
||||
which captures the type and the shape of values, but not the concrete data values.
|
||||
JAX primitives know how to operate on both concrete data
|
||||
values and on the JAX abstract values.
|
||||
|
||||
|
||||
The JAX-transformed functions must themselves be JAX-traceable functions,
|
||||
to ensure that these transformations
|
||||
can be composed, e.g., `jit(jacfwd(grad(f)))`.
|
||||
|
||||
There are pre-defined JAX primitives corresponding to most XLA operations,
|
||||
e.g., add, matmul, sin, cos, indexing.
|
||||
JAX comes with an implementation of numpy functions in terms of JAX primitives, which means that Python programs
|
||||
using JAX’s implementation of numpy are JAX-traceable and therefore transformable.
|
||||
Other libraries can be made JAX-traceable by implementing them in terms of JAX primitives.
|
||||
|
||||
The set of JAX primitives is extensible. Instead of reimplementing a function in terms of pre-defined JAX primitives,
|
||||
one can define a new primitive that encapsulates the behavior of the function.
|
||||
|
||||
**The goal of this document is to explain the interface that a JAX primitive must support in order to allow JAX to perform all its transformations.**
|
||||
|
||||
Consider that we want to add to JAX support for a multiply-add function with three arguments, defined mathematically
|
||||
as "multiply_add(x, y, z) = x * y + z".
|
||||
This function operates on 3 identically-shaped tensors of floating point
|
||||
values and performs the opertions pointwise.
|
||||
|
||||
|
||||
|
||||
+++ {"id": "HIJYIHNTD1yI", "colab_type": "text"}
|
||||
|
||||
## Using existing primitives
|
||||
|
||||
The easiest way to define new functions is to write them in terms of JAX primitives, or in terms of other
|
||||
functions that are themselves written using JAX primitives, e.g., those
|
||||
defined in the `jax.lax` module:
|
||||
|
||||
```{code-cell}
|
||||
---
|
||||
colab:
|
||||
base_uri: https://localhost:8080/
|
||||
height: 105
|
||||
colab_type: code
|
||||
id: tbOF0LB0EMne
|
||||
outputId: 3fb1c8a7-7a4c-4a3a-f7ff-37b7dc740528
|
||||
---
|
||||
from jax import lax
|
||||
from jax import api
|
||||
|
||||
def multiply_add_lax(x, y, z):
|
||||
"""Implementation of multiply-add using the jax.lax primitives."""
|
||||
return lax.add(lax.mul(x, y), z)
|
||||
|
||||
|
||||
def square_add_lax(a, b):
|
||||
"""A square-add function using the newly defined multiply-add."""
|
||||
return multiply_add_lax(a, a, b)
|
||||
|
||||
print("square_add_lax = ", square_add_lax(2., 10.))
|
||||
# Differentiate w.r.t. the first argument
|
||||
print("grad(square_add_lax) = ", api.grad(square_add_lax, argnums=0)(2.0, 10.))
|
||||
```
|
||||
|
||||
+++ {"id": "Cgv60Wm3E_D5", "colab_type": "text"}
|
||||
|
||||
In order to understand how JAX is internally using the primitives,
|
||||
we add some helpers for tracing function calls.
|
||||
|
||||
```{code-cell}
|
||||
:cellView: form
|
||||
:colab: {}
|
||||
:colab_type: code
|
||||
:id: mQRQGEGiE53K
|
||||
|
||||
#@title Helper functions (execute this cell)
|
||||
import functools
|
||||
import traceback
|
||||
|
||||
_indentation = 0
|
||||
def _trace(msg=None):
|
||||
"""Print a message at current indentation."""
|
||||
if msg is not None:
|
||||
print(" " * _indentation + msg)
|
||||
|
||||
def _trace_indent(msg=None):
|
||||
"""Print a message and then indent the rest."""
|
||||
global _indentation
|
||||
_trace(msg)
|
||||
_indentation = 1 + _indentation
|
||||
|
||||
def _trace_unindent(msg=None):
|
||||
"""Unindent then print a message."""
|
||||
global _indentation
|
||||
_indentation = _indentation - 1
|
||||
_trace(msg)
|
||||
|
||||
def trace(name):
|
||||
"""A decorator for functions to trace arguments and results."""
|
||||
|
||||
def trace_func(func): # pylint: disable=missing-docstring
|
||||
def pp(v):
|
||||
"""Print certain values more succinctly"""
|
||||
vtype = str(type(v))
|
||||
if "jax.lib.xla_bridge._JaxComputationBuilder" in vtype:
|
||||
return "<JaxComputationBuilder>"
|
||||
elif "jaxlib.xla_extension.XlaOp" in vtype:
|
||||
return "<XlaOp at 0x{:x}>".format(id(v))
|
||||
elif ("partial_eval.JaxprTracer" in vtype or
|
||||
"batching.BatchTracer" in vtype or
|
||||
"ad.JVPTracer" in vtype):
|
||||
return "Traced<{}>".format(v.aval)
|
||||
elif isinstance(v, tuple):
|
||||
return "({})".format(pp_values(v))
|
||||
else:
|
||||
return str(v)
|
||||
def pp_values(args):
|
||||
return ", ".join([pp(arg) for arg in args])
|
||||
|
||||
@functools.wraps(func)
|
||||
def func_wrapper(*args):
|
||||
_trace_indent("call {}({})".format(name, pp_values(args)))
|
||||
res = func(*args)
|
||||
_trace_unindent("|<- {} = {}".format(name, pp(res)))
|
||||
return res
|
||||
|
||||
return func_wrapper
|
||||
|
||||
return trace_func
|
||||
|
||||
class expectNotImplementedError(object):
|
||||
"""Context manager to check for NotImplementedError."""
|
||||
def __enter__(self): pass
|
||||
def __exit__(self, type, value, tb):
|
||||
global _indentation
|
||||
_indentation = 0
|
||||
if type is NotImplementedError:
|
||||
print("\nFound expected exception:")
|
||||
traceback.print_exc(limit=3)
|
||||
return True
|
||||
elif type is None: # No exception
|
||||
assert False, "Expected NotImplementedError"
|
||||
else:
|
||||
return False
|
||||
```
|
||||
|
||||
+++ {"id": "Qf4eLrLCFYDl", "colab_type": "text"}
|
||||
|
||||
Instead of using `jax.lax` primitives directly, we can use other functions
|
||||
that are already written in terms of those primitives, such as those in `jax.numpy`:
|
||||
|
||||
```{code-cell}
|
||||
---
|
||||
colab:
|
||||
base_uri: https://localhost:8080/
|
||||
height: 255
|
||||
colab_type: code
|
||||
id: QhKorz6cFRJb
|
||||
outputId: aba3cef3-6bcc-4eb3-c7b3-34e405f2f82a
|
||||
---
|
||||
import jax.numpy as jnp
|
||||
import numpy as np
|
||||
|
||||
@trace("multiply_add_numpy")
|
||||
def multiply_add_numpy(x, y, z):
|
||||
return jnp.add(jnp.multiply(x, y), z)
|
||||
|
||||
@trace("square_add_numpy")
|
||||
def square_add_numpy(a, b):
|
||||
return multiply_add_numpy(a, a, b)
|
||||
|
||||
print("\nNormal evaluation:")
|
||||
print("square_add_numpy = ", square_add_numpy(2., 10.))
|
||||
print("\nGradient evaluation:")
|
||||
print("grad(square_add_numpy) = ", api.grad(square_add_numpy)(2.0, 10.))
|
||||
```
|
||||
|
||||
+++ {"id": "Sg-D8EdeFn4a", "colab_type": "text"}
|
||||
|
||||
Notice that in the process of computing `grad`, JAX invokes `square_add_numpy` and
|
||||
`multiply_add_numpy` with special arguments `ConcreteArray(...)` (described further
|
||||
below in this colab).
|
||||
It is important to remember that a JAX-traceable function must be able to
|
||||
operate not only on concrete arguments but also on special abstract arguments
|
||||
that JAX may use to abstract the function execution.
|
||||
|
||||
The JAX traceability property is satisfied as long as the function is written
|
||||
in terms of JAX primitives.
|
||||
|
||||
+++ {"id": "WxrQO7-XGLcg", "colab_type": "text"}
|
||||
|
||||
## Defining new JAX primitives
|
||||
|
||||
The right way to add support for multiply-add is in terms of existing
|
||||
JAX primitives, as shown above. However, in order to demonstrate how JAX
|
||||
primitives work let us pretend that we want to add a new primitive to
|
||||
JAX for the multiply-add functionality.
|
||||
|
||||
```{code-cell}
|
||||
:colab: {}
|
||||
:colab_type: code
|
||||
:id: cPqAH1XOGTN4
|
||||
|
||||
from jax import core
|
||||
multiply_add_p = core.Primitive("multiply_add") # Create the primitive
|
||||
|
||||
@trace("multiply_add_prim")
|
||||
def multiply_add_prim(x, y, z):
|
||||
"""The JAX-traceable way to use the JAX primitive.
|
||||
|
||||
Note that the traced arguments must be passed as positional arguments
|
||||
to `bind`.
|
||||
"""
|
||||
return multiply_add_p.bind(x, y, z)
|
||||
|
||||
@trace("square_add_prim")
|
||||
def square_add_prim(a, b):
|
||||
"""A square-add function implemented using the new JAX-primitive."""
|
||||
return multiply_add_prim(a, a, b)
|
||||
```
|
||||
|
||||
+++ {"id": "LMzs5PAKGr-4", "colab_type": "text"}
|
||||
|
||||
If we try to call the newly defined functions we get an error, because
|
||||
we have not yet told JAX anything about the semantics of the new primitive.
|
||||
|
||||
```{code-cell}
|
||||
---
|
||||
colab:
|
||||
base_uri: https://localhost:8080/
|
||||
height: 221
|
||||
colab_type: code
|
||||
id: _X3PAYxhGpWd
|
||||
outputId: 90ea2c6a-9ef3-40ea-e9a3-3ab1cfc59fc8
|
||||
---
|
||||
with expectNotImplementedError():
|
||||
square_add_prim(2., 10.)
|
||||
```
|
||||
|
||||
+++ {"id": "elha0FdgHSEF", "colab_type": "text"}
|
||||
|
||||
### Primal evaluation rules
|
||||
|
||||
|
||||
|
||||
```{code-cell}
|
||||
---
|
||||
colab:
|
||||
base_uri: https://localhost:8080/
|
||||
height: 34
|
||||
colab_type: code
|
||||
id: FT34FFAGHARU
|
||||
outputId: 4c54f1c2-8a50-4788-90e1-06aee412c43b
|
||||
---
|
||||
@trace("multiply_add_impl")
|
||||
def multiply_add_impl(x, y, z):
|
||||
"""Concrete implementation of the primitive.
|
||||
|
||||
This function does not need to be JAX traceable.
|
||||
Args:
|
||||
x, y, z: the concrete arguments of the primitive. Will only be called with
|
||||
concrete values.
|
||||
Returns:
|
||||
the concrete result of the primitive.
|
||||
"""
|
||||
# Note that we can use the original numpy, which is not JAX traceable
|
||||
return np.add(np.multiply(x, y), z)
|
||||
|
||||
# Now we register the primal implementation with JAX
|
||||
multiply_add_p.def_impl(multiply_add_impl)
|
||||
```
|
||||
|
||||
```{code-cell}
|
||||
---
|
||||
colab:
|
||||
base_uri: https://localhost:8080/
|
||||
height: 119
|
||||
colab_type: code
|
||||
id: G5bstKaeNAVV
|
||||
outputId: deb94d5b-dfea-4e6f-9ec2-70b416c996c5
|
||||
---
|
||||
assert square_add_prim(2., 10.) == 14.
|
||||
```
|
||||
|
||||
+++ {"id": "upBf-uAuHhPJ", "colab_type": "text"}
|
||||
|
||||
### JIT
|
||||
|
||||
If we now try to use `jit` we get a `NotImplementedError`:
|
||||
|
||||
```{code-cell}
|
||||
---
|
||||
colab:
|
||||
base_uri: https://localhost:8080/
|
||||
height: 241
|
||||
colab_type: code
|
||||
id: QG-LULjiHk4b
|
||||
outputId: d4ef4406-8dae-4c96-97ca-b662340474ee
|
||||
---
|
||||
with expectNotImplementedError():
|
||||
api.jit(square_add_prim)(2., 10.)
|
||||
```
|
||||
|
||||
+++ {"id": "rHS1bAGHH44E", "colab_type": "text"}
|
||||
|
||||
#### Abstract evaluation rules
|
||||
In order to JIT the function, and for other transformations as well,
|
||||
JAX first evaluates it abstractly using only the
|
||||
shape and type of the arguments. This abstract evaluation serves multiple
|
||||
purposes:
|
||||
|
||||
* Gets the sequence of JAX primitives that are used in the computation. This
|
||||
sequence will be compiled.
|
||||
* Computes the shape and type of all vectors and operations used in the computation.
|
||||
|
||||
|
||||
For example, the abstraction of a vector with 3 elements may be `ShapedArray(float32[3])`, or `ConcreteArray([1., 2., 3.])`.
|
||||
In the latter case, JAX uses the actual concrete value wrapped as an abstract value.
|
||||
|
||||
```{code-cell}
|
||||
---
|
||||
colab:
|
||||
base_uri: https://localhost:8080/
|
||||
height: 34
|
||||
colab_type: code
|
||||
id: ctQmEeckIbdo
|
||||
outputId: e751d0cc-460e-4ffd-df2e-fdabf9cffdc2
|
||||
---
|
||||
from jax import abstract_arrays
|
||||
@trace("multiply_add_abstract_eval")
|
||||
def multiply_add_abstract_eval(xs, ys, zs):
|
||||
"""Abstract evaluation of the primitive.
|
||||
|
||||
This function does not need to be JAX traceable. It will be invoked with
|
||||
abstractions of the actual arguments.
|
||||
Args:
|
||||
xs, ys, zs: abstractions of the arguments.
|
||||
Result:
|
||||
a ShapedArray for the result of the primitive.
|
||||
"""
|
||||
assert xs.shape == ys.shape
|
||||
assert xs.shape == zs.shape
|
||||
return abstract_arrays.ShapedArray(xs.shape, xs.dtype)
|
||||
|
||||
# Now we register the abstract evaluation with JAX
|
||||
multiply_add_p.def_abstract_eval(multiply_add_abstract_eval)
|
||||
```
|
||||
|
||||
+++ {"id": "RPN88X6YI43A", "colab_type": "text"}
|
||||
|
||||
If we re-attempt to JIT, we see how the abstract evaluation proceeds, but
|
||||
we get another error, about missing the actual XLA compilation rule:
|
||||
|
||||
```{code-cell}
|
||||
---
|
||||
colab:
|
||||
base_uri: https://localhost:8080/
|
||||
height: 309
|
||||
colab_type: code
|
||||
id: eOcNR92SI2h-
|
||||
outputId: 356ef229-3703-4696-cc3d-7c05de405fb0
|
||||
---
|
||||
with expectNotImplementedError():
|
||||
api.jit(square_add_prim)(2., 10.)
|
||||
```
|
||||
|
||||
+++ {"id": "9IOV1R-fJMHp", "colab_type": "text"}
|
||||
|
||||
#### XLA Compilation rules
|
||||
|
||||
JAX compilation works by compiling each primitive into a graph of XLA operations.
|
||||
|
||||
This is biggest hurdle to adding new functionality to JAX, because the
|
||||
set of XLA operations is limited, and JAX already has pre-defined primitives
|
||||
for most of them. However, XLA includes a `CustomCall` operation that can be used to encapsulate arbitrary functionality defined using C++.
|
||||
|
||||
```{code-cell}
|
||||
:colab: {}
|
||||
:colab_type: code
|
||||
:id: FYQWSSjKJaWP
|
||||
|
||||
from jax.lib import xla_client
|
||||
@trace("multiply_add_xla_translation")
|
||||
def multiply_add_xla_translation(c, xc, yc, zc):
|
||||
"""The compilation to XLA of the primitive.
|
||||
|
||||
Given an XlaBuilder and XlaOps for each argument, return the XlaOp for the
|
||||
result of the function.
|
||||
|
||||
Does not need to be a JAX-traceable function.
|
||||
"""
|
||||
return xla_client.ops.Add(xla_client.ops.Mul(xc, yc), zc)
|
||||
|
||||
# Now we register the XLA compilation rule with JAX
|
||||
# TODO: for GPU? and TPU?
|
||||
from jax.interpreters import xla
|
||||
xla.backend_specific_translations['cpu'][multiply_add_p] = multiply_add_xla_translation
|
||||
```
|
||||
|
||||
+++ {"id": "K98LX-VaJkFu", "colab_type": "text"}
|
||||
|
||||
Now we succeed to JIT. Notice below that JAX first evaluates the function
|
||||
abstractly, which triggers the `multiply_add_abstract_eval` function, and
|
||||
then compiles the set of primitives it has encountered, including `multiply_add`.
|
||||
At this point JAX invokes `multiply_add_xla_translation`.
|
||||
|
||||
```{code-cell}
|
||||
---
|
||||
colab:
|
||||
base_uri: https://localhost:8080/
|
||||
height: 173
|
||||
colab_type: code
|
||||
id: rj3TLsolJgEc
|
||||
outputId: e384bee4-1e9c-4344-f49c-d3b5ec08eb32
|
||||
---
|
||||
assert api.jit(lambda x, y: square_add_prim(x, y))(2., 10.) == 14.
|
||||
```
|
||||
|
||||
+++ {"id": "Omrez-2_KFfo", "colab_type": "text"}
|
||||
|
||||
Below is another use of `jit` where we compile only
|
||||
with respect to the first argument. Notice how the second argument to `square_add_prim` is concrete, which leads
|
||||
in the third argument to `multiply_add_abstract_eval` being
|
||||
`ConcreteArray`. We see that `multiply_add_abstract_eval` may be used with
|
||||
both `ShapedArray` and `ConcreteArray`.
|
||||
|
||||
```{code-cell}
|
||||
---
|
||||
colab:
|
||||
base_uri: https://localhost:8080/
|
||||
height: 173
|
||||
colab_type: code
|
||||
id: mPfTwIBoKOEK
|
||||
outputId: b293b9b6-a2f9-48f5-f7eb-d4f99c3d905b
|
||||
---
|
||||
assert api.jit(lambda x, y: square_add_prim(x, y),
|
||||
static_argnums=1)(2., 10.) == 14.
|
||||
```
|
||||
|
||||
+++ {"id": "_Ya3B5l4J1VA", "colab_type": "text"}
|
||||
|
||||
### Forward differentiation
|
||||
|
||||
JAX implements forward differentiation in the form of
|
||||
a Jacobian-vector product (see the [JAX autodiff cookbook](https://jax.readthedocs.io/en/latest/notebooks/autodiff_cookbook.html#Jacobian-Matrix-and-Matrix-Jacobian-products)).
|
||||
|
||||
If we attempt now to compute the `jvp` function we get an
|
||||
error because we have not yet told JAX how to differentiate
|
||||
the `multiply_add` primitive.
|
||||
|
||||
```{code-cell}
|
||||
---
|
||||
colab:
|
||||
base_uri: https://localhost:8080/
|
||||
height: 340
|
||||
colab_type: code
|
||||
id: OxDx6NQnKwMI
|
||||
outputId: ce659ef3-c03c-4856-f252-49ec4b6eb964
|
||||
---
|
||||
# The second argument `(2., 10.)` are the argument values
|
||||
# where we evaluate the Jacobian, and the third `(1., 1.)`
|
||||
# are the values of the tangents for the arguments.
|
||||
with expectNotImplementedError():
|
||||
api.jvp(square_add_prim, (2., 10.), (1., 1.))
|
||||
```
|
||||
|
||||
```{code-cell}
|
||||
:colab: {}
|
||||
:colab_type: code
|
||||
:id: zxG24C1JMIMM
|
||||
|
||||
from jax.interpreters import ad
|
||||
|
||||
|
||||
@trace("multiply_add_value_and_jvp")
|
||||
def multiply_add_value_and_jvp(arg_values, arg_tangents):
|
||||
"""Evaluates the primal output and the tangents (Jacobian-vector product).
|
||||
|
||||
Given values of the arguments and perturbation of the arguments (tangents),
|
||||
compute the output of the primitive and the perturbation of the output.
|
||||
|
||||
This method must be JAX-traceable. JAX may invoke it with abstract values
|
||||
for the arguments and tangents.
|
||||
|
||||
Args:
|
||||
arg_values: a tuple of arguments
|
||||
arg_tangents: a tuple with the tangents of the arguments. The tuple has
|
||||
the same length as the arg_values. Some of the tangents may also be the
|
||||
special value ad.Zero to specify a zero tangent.
|
||||
Returns:
|
||||
a pair of the primal output and the tangent.
|
||||
"""
|
||||
x, y, z = arg_values
|
||||
xt, yt, zt = arg_tangents
|
||||
_trace("Primal evaluation:")
|
||||
# Now we have a JAX-traceable computation of the output.
|
||||
# Normally, we can use the ma primtive itself to compute the primal output.
|
||||
primal_out = multiply_add_prim(x, y, z)
|
||||
|
||||
_trace("Tangent evaluation:")
|
||||
# We must use a JAX-traceable way to compute the tangent. It turns out that
|
||||
# the output tangent can be computed as (xt * y + x * yt + zt),
|
||||
# which we can implement in a JAX-traceable way using the same "multiply_add_prim" primitive.
|
||||
|
||||
# We do need to deal specially with Zero. Here we just turn it into a
|
||||
# proper tensor of 0s (of the same shape as 'x').
|
||||
# An alternative would be to check for Zero and perform algebraic
|
||||
# simplification of the output tangent computation.
|
||||
def make_zero(tan):
|
||||
return lax.zeros_like_array(x) if type(tan) is ad.Zero else tan
|
||||
|
||||
output_tangent = multiply_add_prim(make_zero(xt), y, multiply_add_prim(x, make_zero(yt), make_zero(zt)))
|
||||
return (primal_out, output_tangent)
|
||||
|
||||
# Register the forward differentiation rule with JAX
|
||||
ad.primitive_jvps[multiply_add_p] = multiply_add_value_and_jvp
|
||||
```
|
||||
|
||||
```{code-cell}
|
||||
---
|
||||
colab:
|
||||
base_uri: https://localhost:8080/
|
||||
height: 357
|
||||
colab_type: code
|
||||
id: ma3KBkiAMfW1
|
||||
outputId: f34cbbc6-20d9-48ca-9a9a-b5d91a972cdd
|
||||
---
|
||||
# Tangent is: xt*y + x*yt + zt = 1.*2. + 2.*1. + 1. = 5.
|
||||
assert api.jvp(square_add_prim, (2., 10.), (1., 1.)) == (14., 5.)
|
||||
```
|
||||
|
||||
+++ {"id": "69QsEcu-lP4u", "colab_type": "text"}
|
||||
|
||||
TO EXPLAIN:
|
||||
|
||||
* Why is JAX using ConcreteArray in square_add_prim? There is no abstract evaluation going on here.
|
||||
* Not sure how to explain that multiply_add_prim is invoked with ConcreteValue, yet
|
||||
we do not call the multiply_add_abstract_eval.
|
||||
* I think it would be useful to show the jaxpr here
|
||||
|
||||
|
||||
+++ {"id": "Sb6e3ZAHOPHv", "colab_type": "text"}
|
||||
|
||||
#### JIT of forward differentiation
|
||||
|
||||
We can apply JIT to the forward differentiation function:
|
||||
|
||||
```{code-cell}
|
||||
---
|
||||
colab:
|
||||
base_uri: https://localhost:8080/
|
||||
height: 479
|
||||
colab_type: code
|
||||
id: hg-hzVu-N-hv
|
||||
outputId: 38d32067-e152-4046-ad80-7f95a31ba628
|
||||
---
|
||||
assert api.jit(lambda arg_values, arg_tangents:
|
||||
api.jvp(square_add_prim, arg_values, arg_tangents))(
|
||||
(2., 10.), (1., 1.)) == (14., 5.)
|
||||
```
|
||||
|
||||
+++ {"id": "jlZt1_v2mU88", "colab_type": "text"}
|
||||
|
||||
Notice that first we evaluate `multiply_add_value_and_jvp` abstractly, which in turn
|
||||
evaluates abstractly both the primal and the tangent evaluation (a total of
|
||||
3 invocations of the `ma` primitive). Then we compile the 3 occurrences
|
||||
of the primitive.
|
||||
|
||||
+++ {"id": "555yt6ZIOePB", "colab_type": "text"}
|
||||
|
||||
### Reverse differentiation
|
||||
|
||||
If we attempt now to use reverse differentiation we
|
||||
see that JAX starts by using the `multiply_add_value_and_jvp` to
|
||||
compute the forward differentiation for abstract values, but then runs
|
||||
into a `NotImplementedError`.
|
||||
|
||||
When computing the reverse differentiation JAX first does abstract evaluation
|
||||
of the forward differentiation code `multiply_add_value_and_jvp` to obtain a
|
||||
trace of primitives that compute the output tangent.
|
||||
Observe that JAX performs this abstract evaluation with concrete values
|
||||
for the differentiation point, and abstract values for the tangents.
|
||||
Observe also that JAX uses the special abstract tangent value `Zero` for
|
||||
the tangent corresponding to the 3rd argument of `ma`. This reflects the
|
||||
fact that we do not differentiate w.r.t. the 2nd argument to `square_add_prim`,
|
||||
which flow to 3rd argument to `multiply_add_prim`.
|
||||
|
||||
Observe also that during the abstract evaluation of the tangent we pass the
|
||||
value 0.0 as the tangent for the 3rd argument. This is due to the use
|
||||
of the `make_zero` function in the definition of `multiply_add_value_and_jvp`.
|
||||
|
||||
```{code-cell}
|
||||
---
|
||||
colab:
|
||||
base_uri: https://localhost:8080/
|
||||
height: 666
|
||||
colab_type: code
|
||||
id: 8eAVnexaOjBn
|
||||
outputId: e4ee89cf-ab4a-4505-9817-fa978a2865ab
|
||||
---
|
||||
# This is reverse differentiation w.r.t. the first argument of square_add_prim
|
||||
with expectNotImplementedError():
|
||||
api.grad(square_add_prim)(2., 10.)
|
||||
```
|
||||
|
||||
+++ {"id": "fSHLUMDN26AY", "colab_type": "text"}
|
||||
|
||||
The above error is because there is a missing piece for JAX to be able
|
||||
to use the forward differentiation code to compute reverse differentiation.
|
||||
|
||||
+++ {"id": "3ibDbGF-PjK9", "colab_type": "text"}
|
||||
|
||||
#### Transposition
|
||||
|
||||
|
||||
As explained above, when computing reverse differentiation JAX obtains
|
||||
a trace of primitives that compute the tangent using forward differentiation.
|
||||
Then, **JAX interprets this trace abstractly backwards** and for each
|
||||
primitive it applies a **transposition** rule.
|
||||
|
||||
To understand what is going on, consider for now a simpler example of the function "f(x, y) = x * y + y". Assume we need to differentiate at the point `(2., 4.)`. JAX will produce the following JVP tangent calculation of `ft` from the tangents of the input `xt` and `yt`:
|
||||
```
|
||||
a = xt * 4.
|
||||
b = 2. * yt
|
||||
c = a + b
|
||||
ft = c + yt
|
||||
```
|
||||
|
||||
By construction, the tangent calculation is always linear in the input tangents.
|
||||
The only non-linear operator that may arise in the tangent calculation is multiplication,
|
||||
but then one of the operands is constant.
|
||||
|
||||
JAX will produce the reverse differentiation computation by processing the
|
||||
JVP computation backwards. For each operation in the tangent computation,
|
||||
it accumulates the cotangents
|
||||
of the variables used by the operation, using the cotangent of the result
|
||||
of the operation:
|
||||
```
|
||||
# Initialize cotangents of inputs and intermediate vars
|
||||
xct = yct = act = bct = cct = 0.
|
||||
# Initialize cotangent of the output
|
||||
fct = 1.
|
||||
# Process "ft = c + yt"
|
||||
cct += fct
|
||||
yct += fct
|
||||
# Process "c = a + b"
|
||||
act += cct
|
||||
bct += cct
|
||||
# Process "b = 2. * yt"
|
||||
yct += 2. * bct
|
||||
# Process "a = xt * 4."
|
||||
xct += act * 4.
|
||||
```
|
||||
|
||||
One can verify that this computation produces `xct = 4.` and `yct = 3.`, which
|
||||
are the partial derivatives of the function `f`.
|
||||
|
||||
JAX knows for each primitive that may appear in a JVP calculation how to transpose it. Conceptually, if the primitive `p(x, y, z)` is linear in the arguments `y` and `z` for a constant value of `x`, e.g., `p(x, y, z) = y*cy + z*cz`, then the transposition of the primitive is:
|
||||
```
|
||||
p_transpose(out_ct, x, _, _) = (None, out_ct*cy, out_ct*cz)
|
||||
```
|
||||
|
||||
Notice that `p_transpose` takes the cotangent of the output of the primitive and a value corresponding to each argument of the primitive. For the linear arguments, the transposition gets an undefined `_` value, and for the other
|
||||
arguments it gets the actual constants. The transposition returns a cotangent value for each argument of the primitive, with the value `None` returned
|
||||
for the constant arguments.
|
||||
|
||||
In particular,
|
||||
```
|
||||
add_transpose(out_ct, _, _) = (out_ct, out_ct)
|
||||
mult_transpose(out_ct, x, _) = (None, x * out_ct)
|
||||
mult_transpose(out_ct, _, y) = (out_ct * y, None)
|
||||
```
|
||||
|
||||
|
||||
|
||||
|
||||
```{code-cell}
|
||||
:colab: {}
|
||||
:colab_type: code
|
||||
:id: JaHxFdkRO42r
|
||||
|
||||
@trace("multiply_add_transpose")
|
||||
def multiply_add_transpose(ct, x, y, z):
|
||||
"""Evaluates the transpose of a linear primitive.
|
||||
|
||||
This method is only used when computing the backward gradient following
|
||||
value_and_jvp, and is only needed for primitives that are used in the JVP
|
||||
calculation for some other primitive. We need transposition for multiply_add_prim,
|
||||
because we have used multiply_add_prim in the computation of the output_tangent in
|
||||
multiply_add_value_and_jvp.
|
||||
|
||||
In our case, multiply_add is not a linear primitive. However, it is used linearly
|
||||
w.r.t. tangents in multiply_add_value_and_jvp:
|
||||
output_tangent(xt, yt, zt) = multiply_add_prim(xt, y, multiply_add_prim(x, yt, zt))
|
||||
|
||||
Always one of the first two multiplicative arguments are constants.
|
||||
|
||||
Args:
|
||||
ct: the cotangent of the output of the primitive.
|
||||
x, y, z: values of the arguments. The arguments that are used linearly
|
||||
get an ad.UndefinedPrimal value. The other arguments get a constant
|
||||
value.
|
||||
Returns:
|
||||
a tuple with the cotangent of the inputs, with the value None
|
||||
corresponding to the constant arguments.
|
||||
"""
|
||||
if not ad.is_undefined_primal(x):
|
||||
# This use of multiply_add is with a constant "x"
|
||||
assert ad.is_undefined_primal(y)
|
||||
ct_y = ad.Zero(y.aval) if type(ct) is ad.Zero else multiply_add_prim(x, ct, lax.zeros_like_array(x))
|
||||
res = None, ct_y, ct
|
||||
else:
|
||||
# This use of multiply_add is with a constant "y"
|
||||
assert ad.is_undefined_primal(x)
|
||||
ct_x = ad.Zero(x.aval) if type(ct) is ad.Zero else multiply_add_prim(ct, y, lax.zeros_like_array(y))
|
||||
res = ct_x, None, ct
|
||||
return res
|
||||
|
||||
|
||||
ad.primitive_transposes[multiply_add_p] = multiply_add_transpose
|
||||
```
|
||||
|
||||
+++ {"id": "PpChox-Jp7wb", "colab_type": "text"}
|
||||
|
||||
Now we can complete the run of the `grad`:
|
||||
|
||||
```{code-cell}
|
||||
---
|
||||
colab:
|
||||
base_uri: https://localhost:8080/
|
||||
height: 581
|
||||
colab_type: code
|
||||
id: PogPKS4MPevd
|
||||
outputId: d33328d4-3e87-45b5-9b31-21ad624b67af
|
||||
---
|
||||
assert api.grad(square_add_prim)(2., 10.) == 4.
|
||||
```
|
||||
|
||||
+++ {"id": "8M1xLCXW4fK7", "colab_type": "text"}
|
||||
|
||||
Notice the two calls to `multiply_add_transpose`. They correspond to the two
|
||||
uses of `multiply_add_prim` in the computation of the `output_tangent` in `multiply_add_value_and_jvp`. The first call to transpose corresponds to the
|
||||
last use of `multiply_add_prim`: `multiply_add_prim(xt, y, ...)` where `y` is the constant 2.0.
|
||||
|
||||
+++ {"id": "EIJs6FYmPg6c", "colab_type": "text"}
|
||||
|
||||
#### JIT of reverse differentiation
|
||||
|
||||
Notice that the abstract evaluation of the `multiply_add_value_and_jvp` is using only
|
||||
abstract values, while in the absensce of JIT we used `ConcreteArray`.
|
||||
|
||||
```{code-cell}
|
||||
---
|
||||
colab:
|
||||
base_uri: https://localhost:8080/
|
||||
height: 649
|
||||
colab_type: code
|
||||
id: FZ-JGbWZPq2-
|
||||
outputId: e42b5222-9c3e-4853-e13a-874f6605d178
|
||||
---
|
||||
assert api.jit(api.grad(square_add_prim))(2., 10.) == 4.
|
||||
```
|
||||
|
||||
+++ {"id": "-3lqPkdQPvl5", "colab_type": "text"}
|
||||
|
||||
### Batching
|
||||
|
||||
The batching transformation takes a point-wise computation and turns it
|
||||
into a computation on vectors. If we try it right now, we get a `NotImplementedError`:
|
||||
|
||||
```{code-cell}
|
||||
---
|
||||
colab:
|
||||
base_uri: https://localhost:8080/
|
||||
height: 360
|
||||
colab_type: code
|
||||
id: hFvBR3I9Pzh3
|
||||
outputId: 434608bc-281f-4d3b-83bd-eaaf3b51b1cd
|
||||
---
|
||||
# The arguments are two vectors instead of two scalars
|
||||
with expectNotImplementedError():
|
||||
api.vmap(square_add_prim, in_axes=0, out_axes=0)(np.array([2., 3.]),
|
||||
np.array([10., 20.]))
|
||||
```
|
||||
|
||||
+++ {"id": "gILasMiP6elR", "colab_type": "text"}
|
||||
|
||||
We need to tell JAX how to evaluate the batched version of the primitive. In this particular case, the `multiply_add_prim` already operates pointwise for any dimension of input vectors. So the batched version can use the same `multiply_add_prim` implementation.
|
||||
|
||||
```{code-cell}
|
||||
:colab: {}
|
||||
:colab_type: code
|
||||
:id: KQfeqRIrP7zg
|
||||
|
||||
from jax.interpreters import batching
|
||||
|
||||
|
||||
@trace("multiply_add_batch")
|
||||
def multiply_add_batch(vector_arg_values, batch_axes):
|
||||
"""Computes the batched version of the primitive.
|
||||
|
||||
This must be a JAX-traceable function.
|
||||
|
||||
Since the multiply_add primitive already operates pointwise on arbitrary
|
||||
dimension tensors, to batch it we can use the primitive itself. This works as
|
||||
long as both the inputs have the same dimensions and are batched along the
|
||||
same axes. The result is batched along the axis that the inputs are batched.
|
||||
|
||||
Args:
|
||||
vector_arg_values: a tuple of two arguments, each being a tensor of matching
|
||||
shape.
|
||||
batch_axes: the axes that are being batched. See vmap documentation.
|
||||
Returns:
|
||||
a tuple of the result, and the result axis that was batched.
|
||||
"""
|
||||
assert batch_axes[0] == batch_axes[1]
|
||||
assert batch_axes[0] == batch_axes[2]
|
||||
_trace("Using multiply_add to compute the batch:")
|
||||
res = multiply_add_prim(*vector_arg_values)
|
||||
return res, batch_axes[0]
|
||||
|
||||
|
||||
batching.primitive_batchers[multiply_add_p] = multiply_add_batch
|
||||
```
|
||||
|
||||
```{code-cell}
|
||||
---
|
||||
colab:
|
||||
base_uri: https://localhost:8080/
|
||||
height: 224
|
||||
colab_type: code
|
||||
id: VwxNk869P_YG
|
||||
outputId: 9d22c921-5803-4d33-9e88-b6e439ba9738
|
||||
---
|
||||
assert np.allclose(api.vmap(square_add_prim, in_axes=0, out_axes=0)(
|
||||
np.array([2., 3.]),
|
||||
np.array([10., 20.])),
|
||||
[14., 29.])
|
||||
```
|
||||
|
||||
+++ {"id": "NmqLlV1TQDCC", "colab_type": "text"}
|
||||
|
||||
#### JIT of batching
|
||||
|
||||
```{code-cell}
|
||||
---
|
||||
colab:
|
||||
base_uri: https://localhost:8080/
|
||||
height: 258
|
||||
colab_type: code
|
||||
id: xqEdXVUgQCTt
|
||||
outputId: 9c22fd9c-919c-491d-bbeb-32c241b808fa
|
||||
---
|
||||
assert np.allclose(api.jit(api.vmap(square_add_prim, in_axes=0, out_axes=0))
|
||||
(np.array([2., 3.]),
|
||||
np.array([10., 20.])),
|
||||
[14., 29.])
|
||||
```
|
@ -626,6 +626,9 @@
|
||||
"provenance": [],
|
||||
"toc_visible": true
|
||||
},
|
||||
"jupytext": {
|
||||
"formats": "ipynb,md:myst"
|
||||
},
|
||||
"kernelspec": {
|
||||
"display_name": "Python 3",
|
||||
"language": "python",
|
||||
|
317
docs/notebooks/Neural_Network_and_Data_Loading.md
Normal file
317
docs/notebooks/Neural_Network_and_Data_Loading.md
Normal file
@ -0,0 +1,317 @@
|
||||
---
|
||||
jupytext:
|
||||
formats: ipynb,md:myst
|
||||
text_representation:
|
||||
extension: .md
|
||||
format_name: myst
|
||||
format_version: 0.13
|
||||
jupytext_version: 1.10.0
|
||||
kernelspec:
|
||||
display_name: Python 3
|
||||
language: python
|
||||
name: python3
|
||||
---
|
||||
|
||||
+++ {"colab_type": "text", "id": "18AF5Ab4p6VL"}
|
||||
|
||||
# Training a Simple Neural Network, with PyTorch Data Loading
|
||||
|
||||
**Copyright 2018 Google LLC.**
|
||||
|
||||
Licensed under the Apache License, Version 2.0 (the "License");you may not use this file except in compliance with the License.
|
||||
You may obtain a copy of the License at
|
||||
|
||||
https://www.apache.org/licenses/LICENSE-2.0
|
||||
|
||||
Unless required by applicable law or agreed to in writing, software
|
||||
distributed under the License is distributed on an "AS IS" BASIS,
|
||||
WITHOUT WARRANTIES OR CONDITIONS OF ANY KIND, either express or implied.
|
||||
See the License for the specific language governing permissions and
|
||||
limitations under the License.
|
||||
|
||||
+++ {"colab_type": "text", "id": "B_XlLLpcWjkA"}
|
||||
|
||||
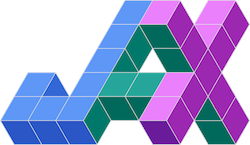
|
||||
|
||||
Let's combine everything we showed in the [quickstart notebook](https://colab.research.google.com/github/google/jax/blob/master/docs/notebooks/quickstart.ipynb) to train a simple neural network. We will first specify and train a simple MLP on MNIST using JAX for the computation. We will use PyTorch's data loading API to load images and labels (because it's pretty great, and the world doesn't need yet another data loading library).
|
||||
|
||||
Of course, you can use JAX with any API that is compatible with NumPy to make specifying the model a bit more plug-and-play. Here, just for explanatory purposes, we won't use any neural network libraries or special APIs for builidng our model.
|
||||
|
||||
```{code-cell} ipython3
|
||||
:colab: {}
|
||||
:colab_type: code
|
||||
:id: OksHydJDtbbI
|
||||
|
||||
import jax.numpy as jnp
|
||||
from jax import grad, jit, vmap
|
||||
from jax import random
|
||||
```
|
||||
|
||||
+++ {"colab_type": "text", "id": "MTVcKi-ZYB3R"}
|
||||
|
||||
## Hyperparameters
|
||||
Let's get a few bookkeeping items out of the way.
|
||||
|
||||
```{code-cell} ipython3
|
||||
:colab: {}
|
||||
:colab_type: code
|
||||
:id: -fmWA06xYE7d
|
||||
|
||||
# A helper function to randomly initialize weights and biases
|
||||
# for a dense neural network layer
|
||||
def random_layer_params(m, n, key, scale=1e-2):
|
||||
w_key, b_key = random.split(key)
|
||||
return scale * random.normal(w_key, (n, m)), scale * random.normal(b_key, (n,))
|
||||
|
||||
# Initialize all layers for a fully-connected neural network with sizes "sizes"
|
||||
def init_network_params(sizes, key):
|
||||
keys = random.split(key, len(sizes))
|
||||
return [random_layer_params(m, n, k) for m, n, k in zip(sizes[:-1], sizes[1:], keys)]
|
||||
|
||||
layer_sizes = [784, 512, 512, 10]
|
||||
param_scale = 0.1
|
||||
step_size = 0.01
|
||||
num_epochs = 8
|
||||
batch_size = 128
|
||||
n_targets = 10
|
||||
params = init_network_params(layer_sizes, random.PRNGKey(0))
|
||||
```
|
||||
|
||||
+++ {"colab_type": "text", "id": "BtoNk_yxWtIw"}
|
||||
|
||||
## Auto-batching predictions
|
||||
|
||||
Let us first define our prediction function. Note that we're defining this for a _single_ image example. We're going to use JAX's `vmap` function to automatically handle mini-batches, with no performance penalty.
|
||||
|
||||
```{code-cell} ipython3
|
||||
:colab: {}
|
||||
:colab_type: code
|
||||
:id: 7APc6tD7TiuZ
|
||||
|
||||
from jax.scipy.special import logsumexp
|
||||
|
||||
def relu(x):
|
||||
return jnp.maximum(0, x)
|
||||
|
||||
def predict(params, image):
|
||||
# per-example predictions
|
||||
activations = image
|
||||
for w, b in params[:-1]:
|
||||
outputs = jnp.dot(w, activations) + b
|
||||
activations = relu(outputs)
|
||||
|
||||
final_w, final_b = params[-1]
|
||||
logits = jnp.dot(final_w, activations) + final_b
|
||||
return logits - logsumexp(logits)
|
||||
```
|
||||
|
||||
+++ {"colab_type": "text", "id": "dRW_TvCTWgaP"}
|
||||
|
||||
Let's check that our prediction function only works on single images.
|
||||
|
||||
```{code-cell} ipython3
|
||||
---
|
||||
colab:
|
||||
base_uri: https://localhost:8080/
|
||||
height: 34
|
||||
colab_type: code
|
||||
id: 4sW2A5mnXHc5
|
||||
outputId: 9d3b29e8-fab3-4ecb-9f63-bc8c092f9006
|
||||
---
|
||||
# This works on single examples
|
||||
random_flattened_image = random.normal(random.PRNGKey(1), (28 * 28,))
|
||||
preds = predict(params, random_flattened_image)
|
||||
print(preds.shape)
|
||||
```
|
||||
|
||||
```{code-cell} ipython3
|
||||
---
|
||||
colab:
|
||||
base_uri: https://localhost:8080/
|
||||
height: 34
|
||||
colab_type: code
|
||||
id: PpyQxuedXfhp
|
||||
outputId: d5d20211-b6da-44e9-f71e-946f2a9d0fc4
|
||||
---
|
||||
# Doesn't work with a batch
|
||||
random_flattened_images = random.normal(random.PRNGKey(1), (10, 28 * 28))
|
||||
try:
|
||||
preds = predict(params, random_flattened_images)
|
||||
except TypeError:
|
||||
print('Invalid shapes!')
|
||||
```
|
||||
|
||||
```{code-cell} ipython3
|
||||
---
|
||||
colab:
|
||||
base_uri: https://localhost:8080/
|
||||
height: 34
|
||||
colab_type: code
|
||||
id: oJOOncKMXbwK
|
||||
outputId: 31285fab-7667-4871-fcba-28e86adc3fc6
|
||||
---
|
||||
# Let's upgrade it to handle batches using `vmap`
|
||||
|
||||
# Make a batched version of the `predict` function
|
||||
batched_predict = vmap(predict, in_axes=(None, 0))
|
||||
|
||||
# `batched_predict` has the same call signature as `predict`
|
||||
batched_preds = batched_predict(params, random_flattened_images)
|
||||
print(batched_preds.shape)
|
||||
```
|
||||
|
||||
+++ {"colab_type": "text", "id": "elsG6nX03BvW"}
|
||||
|
||||
At this point, we have all the ingredients we need to define our neural network and train it. We've built an auto-batched version of `predict`, which we should be able to use in a loss function. We should be able to use `grad` to take the derivative of the loss with respect to the neural network parameters. Last, we should be able to use `jit` to speed up everything.
|
||||
|
||||
+++ {"colab_type": "text", "id": "NwDuFqc9X7ER"}
|
||||
|
||||
## Utility and loss functions
|
||||
|
||||
```{code-cell} ipython3
|
||||
:colab: {}
|
||||
:colab_type: code
|
||||
:id: 6lTI6I4lWdh5
|
||||
|
||||
def one_hot(x, k, dtype=jnp.float32):
|
||||
"""Create a one-hot encoding of x of size k."""
|
||||
return jnp.array(x[:, None] == jnp.arange(k), dtype)
|
||||
|
||||
def accuracy(params, images, targets):
|
||||
target_class = jnp.argmax(targets, axis=1)
|
||||
predicted_class = jnp.argmax(batched_predict(params, images), axis=1)
|
||||
return jnp.mean(predicted_class == target_class)
|
||||
|
||||
def loss(params, images, targets):
|
||||
preds = batched_predict(params, images)
|
||||
return -jnp.mean(preds * targets)
|
||||
|
||||
@jit
|
||||
def update(params, x, y):
|
||||
grads = grad(loss)(params, x, y)
|
||||
return [(w - step_size * dw, b - step_size * db)
|
||||
for (w, b), (dw, db) in zip(params, grads)]
|
||||
```
|
||||
|
||||
+++ {"colab_type": "text", "id": "umJJGZCC2oKl"}
|
||||
|
||||
## Data Loading with PyTorch
|
||||
|
||||
JAX is laser-focused on program transformations and accelerator-backed NumPy, so we don't include data loading or munging in the JAX library. There are already a lot of great data loaders out there, so let's just use them instead of reinventing anything. We'll grab PyTorch's data loader, and make a tiny shim to make it work with NumPy arrays.
|
||||
|
||||
```{code-cell} ipython3
|
||||
---
|
||||
colab:
|
||||
base_uri: https://localhost:8080/
|
||||
height: 139
|
||||
colab_type: code
|
||||
id: gEvWt8_u2pqG
|
||||
outputId: 2c83a679-9ce5-4c67-bccb-9ea835a8eaf6
|
||||
---
|
||||
!pip install torch torchvision
|
||||
```
|
||||
|
||||
```{code-cell} ipython3
|
||||
:cellView: both
|
||||
:colab: {}
|
||||
:colab_type: code
|
||||
:id: 94PjXZ8y3dVF
|
||||
|
||||
import numpy as np
|
||||
from torch.utils import data
|
||||
from torchvision.datasets import MNIST
|
||||
|
||||
def numpy_collate(batch):
|
||||
if isinstance(batch[0], np.ndarray):
|
||||
return np.stack(batch)
|
||||
elif isinstance(batch[0], (tuple,list)):
|
||||
transposed = zip(*batch)
|
||||
return [numpy_collate(samples) for samples in transposed]
|
||||
else:
|
||||
return np.array(batch)
|
||||
|
||||
class NumpyLoader(data.DataLoader):
|
||||
def __init__(self, dataset, batch_size=1,
|
||||
shuffle=False, sampler=None,
|
||||
batch_sampler=None, num_workers=0,
|
||||
pin_memory=False, drop_last=False,
|
||||
timeout=0, worker_init_fn=None):
|
||||
super(self.__class__, self).__init__(dataset,
|
||||
batch_size=batch_size,
|
||||
shuffle=shuffle,
|
||||
sampler=sampler,
|
||||
batch_sampler=batch_sampler,
|
||||
num_workers=num_workers,
|
||||
collate_fn=numpy_collate,
|
||||
pin_memory=pin_memory,
|
||||
drop_last=drop_last,
|
||||
timeout=timeout,
|
||||
worker_init_fn=worker_init_fn)
|
||||
|
||||
class FlattenAndCast(object):
|
||||
def __call__(self, pic):
|
||||
return np.ravel(np.array(pic, dtype=jnp.float32))
|
||||
```
|
||||
|
||||
```{code-cell} ipython3
|
||||
:colab: {}
|
||||
:colab_type: code
|
||||
:id: l314jsfP4TN4
|
||||
|
||||
# Define our dataset, using torch datasets
|
||||
mnist_dataset = MNIST('/tmp/mnist/', download=True, transform=FlattenAndCast())
|
||||
training_generator = NumpyLoader(mnist_dataset, batch_size=batch_size, num_workers=0)
|
||||
```
|
||||
|
||||
```{code-cell} ipython3
|
||||
---
|
||||
colab:
|
||||
base_uri: https://localhost:8080/
|
||||
height: 173
|
||||
colab_type: code
|
||||
id: FTNo4beUvb6t
|
||||
outputId: 65a9087c-c326-49e5-cbfc-e0839212fa31
|
||||
---
|
||||
# Get the full train dataset (for checking accuracy while training)
|
||||
train_images = np.array(mnist_dataset.train_data).reshape(len(mnist_dataset.train_data), -1)
|
||||
train_labels = one_hot(np.array(mnist_dataset.train_labels), n_targets)
|
||||
|
||||
# Get full test dataset
|
||||
mnist_dataset_test = MNIST('/tmp/mnist/', download=True, train=False)
|
||||
test_images = jnp.array(mnist_dataset_test.test_data.numpy().reshape(len(mnist_dataset_test.test_data), -1), dtype=jnp.float32)
|
||||
test_labels = one_hot(np.array(mnist_dataset_test.test_labels), n_targets)
|
||||
```
|
||||
|
||||
+++ {"colab_type": "text", "id": "xxPd6Qw3Z98v"}
|
||||
|
||||
## Training Loop
|
||||
|
||||
```{code-cell} ipython3
|
||||
---
|
||||
colab:
|
||||
base_uri: https://localhost:8080/
|
||||
height: 425
|
||||
colab_type: code
|
||||
id: X2DnZo3iYj18
|
||||
outputId: 0eba3ca2-24a1-4cba-aaf4-3ac61d0c650e
|
||||
---
|
||||
import time
|
||||
|
||||
for epoch in range(num_epochs):
|
||||
start_time = time.time()
|
||||
for x, y in training_generator:
|
||||
y = one_hot(y, n_targets)
|
||||
params = update(params, x, y)
|
||||
epoch_time = time.time() - start_time
|
||||
|
||||
train_acc = accuracy(params, train_images, train_labels)
|
||||
test_acc = accuracy(params, test_images, test_labels)
|
||||
print("Epoch {} in {:0.2f} sec".format(epoch, epoch_time))
|
||||
print("Training set accuracy {}".format(train_acc))
|
||||
print("Test set accuracy {}".format(test_acc))
|
||||
```
|
||||
|
||||
+++ {"colab_type": "text", "id": "xC1CMcVNYwxm"}
|
||||
|
||||
We've now used the whole of the JAX API: `grad` for derivatives, `jit` for speedups and `vmap` for auto-vectorization.
|
||||
We used NumPy to specify all of our computation, and borrowed the great data loaders from PyTorch, and ran the whole thing on the GPU.
|
File diff suppressed because it is too large
Load Diff
377
docs/notebooks/Writing_custom_interpreters_in_Jax.md
Normal file
377
docs/notebooks/Writing_custom_interpreters_in_Jax.md
Normal file
@ -0,0 +1,377 @@
|
||||
---
|
||||
jupytext:
|
||||
formats: ipynb,md:myst
|
||||
text_representation:
|
||||
extension: .md
|
||||
format_name: myst
|
||||
format_version: 0.13
|
||||
jupytext_version: 1.10.0
|
||||
kernelspec:
|
||||
display_name: Python 3
|
||||
language: python
|
||||
name: python3
|
||||
---
|
||||
|
||||
+++ {"colab_type": "text", "id": "M-hPMKlwXjMr"}
|
||||
|
||||
# Writing custom Jaxpr interpreters in JAX
|
||||
|
||||
+++ {"colab_type": "text", "id": "r-3vMiKRYXPJ"}
|
||||
|
||||
JAX offers several composable function transformations (`jit`, `grad`, `vmap`,
|
||||
etc.) that enable writing concise, accelerated code.
|
||||
|
||||
Here we show how to add your own function transformations to the system, by writing a custom Jaxpr interpreter. And we'll get composability with all the other transformations for free.
|
||||
|
||||
**This example uses internal JAX APIs, which may break at any time. Anything not in [the API Documentation](https://jax.readthedocs.io/en/latest/jax.html) should be assumed internal.**
|
||||
|
||||
```{code-cell} ipython3
|
||||
:colab: {}
|
||||
:colab_type: code
|
||||
:id: s27RDKvKXFL8
|
||||
|
||||
import numpy as np
|
||||
import jax
|
||||
import jax.numpy as jnp
|
||||
from jax import jit, grad, vmap
|
||||
from jax import random
|
||||
```
|
||||
|
||||
+++ {"colab_type": "text", "id": "jb_8mEsJboVM"}
|
||||
|
||||
## What is JAX doing?
|
||||
|
||||
+++ {"colab_type": "text", "id": "KxR2WK0Ubs0R"}
|
||||
|
||||
JAX provides a NumPy-like API for numerical computing which can be used as is, but JAX's true power comes from composable function transformations. Take the `jit` function transformation, which takes in a function and returns a semantically identical function but is lazily compiled by XLA for accelerators.
|
||||
|
||||
```{code-cell} ipython3
|
||||
:colab: {}
|
||||
:colab_type: code
|
||||
:id: HmlMcICOcSXR
|
||||
|
||||
x = random.normal(random.PRNGKey(0), (5000, 5000))
|
||||
def f(w, b, x):
|
||||
return jnp.tanh(jnp.dot(x, w) + b)
|
||||
fast_f = jit(f)
|
||||
```
|
||||
|
||||
+++ {"colab_type": "text", "id": "gA8V51wZdsjh"}
|
||||
|
||||
When we call `fast_f`, what happens? JAX traces the function and constructs an XLA computation graph. The graph is then JIT-compiled and executed. Other transformations work similarly in that they first trace the function and handle the output trace in some way. To learn more about Jax's tracing machinery, you can refer to the ["How it works"](https://github.com/google/jax#how-it-works) section in the README.
|
||||
|
||||
+++ {"colab_type": "text", "id": "2Th1vYLVaFBz"}
|
||||
|
||||
## Jaxpr tracer
|
||||
|
||||
A tracer of special importance in Jax is the Jaxpr tracer, which records ops into a Jaxpr (Jax expression). A Jaxpr is a data structure that can be evaluated like a mini functional programming language and
|
||||
thus Jaxprs are a useful intermediate representation
|
||||
for function transformation.
|
||||
|
||||
|
||||
+++ {"colab_type": "text", "id": "pH7s63lpaHJO"}
|
||||
|
||||
To get a first look at Jaxprs, consider the `make_jaxpr` transformation. `make_jaxpr` is essentially a "pretty-printing" transformation:
|
||||
it transforms a function into one that, given example arguments, produces a Jaxpr representation of its computation.
|
||||
Although we can't generally use the Jaxprs that it returns, it is useful for debugging and introspection.
|
||||
Let's use it to look at how some example Jaxprs
|
||||
are structured.
|
||||
|
||||
```{code-cell} ipython3
|
||||
:colab: {}
|
||||
:colab_type: code
|
||||
:id: RSxEiWi-EeYW
|
||||
|
||||
def examine_jaxpr(typed_jaxpr):
|
||||
jaxpr = typed_jaxpr.jaxpr
|
||||
print("invars:", jaxpr.invars)
|
||||
print("outvars:", jaxpr.outvars)
|
||||
print("constvars:", jaxpr.constvars)
|
||||
for eqn in jaxpr.eqns:
|
||||
print("equation:", eqn.invars, eqn.primitive, eqn.outvars, eqn.params)
|
||||
print()
|
||||
print("jaxpr:", jaxpr)
|
||||
|
||||
def foo(x):
|
||||
return x + 1
|
||||
print("foo")
|
||||
print("=====")
|
||||
examine_jaxpr(jax.make_jaxpr(foo)(5))
|
||||
|
||||
print()
|
||||
|
||||
def bar(w, b, x):
|
||||
return jnp.dot(w, x) + b + jnp.ones(5), x
|
||||
print("bar")
|
||||
print("=====")
|
||||
examine_jaxpr(jax.make_jaxpr(bar)(jnp.ones((5, 10)), jnp.ones(5), jnp.ones(10)))
|
||||
```
|
||||
|
||||
+++ {"colab_type": "text", "id": "k-HxK9iagnH6"}
|
||||
|
||||
* `jaxpr.invars` - the `invars` of a Jaxpr are a list of the input variables to Jaxpr, analogous to arguments in Python functions
|
||||
* `jaxpr.outvars` - the `outvars` of a Jaxpr are the variables that are returned by the Jaxpr. Every Jaxpr has multiple outputs.
|
||||
* `jaxpr.constvars` - the `constvars` are a list of variables that are also inputs to the Jaxpr, but correspond to constants from the trace (we'll go over these in more detail later)
|
||||
* `jaxpr.eqns` - a list of equations, which are essentially let-bindings. Each equation is list of input variables, a list of output variables, and a *primitive*, which is used to evaluate inputs to produce outputs. Each equation also has a `params`, a dictionary of parameters.
|
||||
|
||||
All together, a Jaxpr encapsulates a simple program that can be evaluated with inputs to produce an output. We'll go over how exactly to do this later. The important thing to note now is that a Jaxpr is a data structure that can be manipulated and evaluated in whatever way we want.
|
||||
|
||||
+++ {"colab_type": "text", "id": "NwY7TurYn6sr"}
|
||||
|
||||
### Why are Jaxprs useful?
|
||||
|
||||
+++ {"colab_type": "text", "id": "UEy6RorCgdYt"}
|
||||
|
||||
Jaxprs are simple program representations that are easy to transform. And because Jax lets us stage out Jaxprs from Python functions, it gives us a way to transform numerical programs written in Python.
|
||||
|
||||
+++ {"colab_type": "text", "id": "qizTKpbno_ua"}
|
||||
|
||||
## Your first interpreter: `invert`
|
||||
|
||||
+++ {"colab_type": "text", "id": "OIto-KX4pD7j"}
|
||||
|
||||
Let's try to implement a simple function "inverter", which takes in the output of the original function and returns the inputs that produced those outputs. For now, let's focus on simple, unary functions which are composed of other invertible unary functions.
|
||||
|
||||
Goal:
|
||||
```python
|
||||
def f(x):
|
||||
return jnp.exp(jnp.tanh(x))
|
||||
f_inv = inverse(f)
|
||||
assert jnp.allclose(f_inv(f(1.0)), 1.0)
|
||||
```
|
||||
|
||||
The way we'll implement this is by (1) tracing `f` into a Jaxpr, then (2) interpreting the Jaxpr *backwards*. While interpreting the Jaxpr backwards, for each equation we'll look up the primitive's inverse in a table and apply it.
|
||||
|
||||
### 1. Tracing a function
|
||||
|
||||
We can't use `make_jaxpr` for this, because we need to pull out constants created during the trace to pass into the Jaxpr. However, we can write a function that does something very similar to `make_jaxpr`.
|
||||
|
||||
```{code-cell} ipython3
|
||||
:colab: {}
|
||||
:colab_type: code
|
||||
:id: BHkg_3P1pXJj
|
||||
|
||||
# Importing Jax functions useful for tracing/interpreting.
|
||||
import numpy as np
|
||||
from functools import wraps
|
||||
|
||||
from jax import core
|
||||
from jax import lax
|
||||
from jax._src.util import safe_map
|
||||
```
|
||||
|
||||
+++ {"colab_type": "text", "id": "CpTml2PTrzZ4"}
|
||||
|
||||
This function first flattens its arguments into a list, which are the abstracted and wrapped as partial values. The `pe.trace_to_jaxpr` function is used to then trace a function into a Jaxpr
|
||||
from a list of partial value inputs.
|
||||
|
||||
```{code-cell} ipython3
|
||||
:colab: {}
|
||||
:colab_type: code
|
||||
:id: Tc1REN5aq_fH
|
||||
|
||||
def f(x):
|
||||
return jnp.exp(jnp.tanh(x))
|
||||
|
||||
closed_jaxpr = jax.make_jaxpr(f)(jnp.ones(5))
|
||||
print(closed_jaxpr)
|
||||
print(closed_jaxpr.literals)
|
||||
```
|
||||
|
||||
+++ {"colab_type": "text", "id": "WmZ3BcmZsbfR"}
|
||||
|
||||
### 2. Evaluating a Jaxpr
|
||||
|
||||
|
||||
Before we write a custom Jaxpr interpreter, let's first implement the "default" interpreter, `eval_jaxpr`, which evaluates the Jaxpr as-is, computing the same values that the original, un-transformed Python function would.
|
||||
|
||||
To do this, we first create an environment to store the values for each of the variables, and update the environment with each equation we evaluate in the Jaxpr.
|
||||
|
||||
```{code-cell} ipython3
|
||||
:colab: {}
|
||||
:colab_type: code
|
||||
:id: ACMxjIHStHwD
|
||||
|
||||
def eval_jaxpr(jaxpr, consts, *args):
|
||||
# Mapping from variable -> value
|
||||
env = {}
|
||||
|
||||
def read(var):
|
||||
# Literals are values baked into the Jaxpr
|
||||
if type(var) is core.Literal:
|
||||
return var.val
|
||||
return env[var]
|
||||
|
||||
def write(var, val):
|
||||
env[var] = val
|
||||
|
||||
# Bind args and consts to environment
|
||||
write(core.unitvar, core.unit)
|
||||
safe_map(write, jaxpr.invars, args)
|
||||
safe_map(write, jaxpr.constvars, consts)
|
||||
|
||||
# Loop through equations and evaluate primitives using `bind`
|
||||
for eqn in jaxpr.eqns:
|
||||
# Read inputs to equation from environment
|
||||
invals = safe_map(read, eqn.invars)
|
||||
# `bind` is how a primitive is called
|
||||
outvals = eqn.primitive.bind(*invals, **eqn.params)
|
||||
# Primitives may return multiple outputs or not
|
||||
if not eqn.primitive.multiple_results:
|
||||
outvals = [outvals]
|
||||
# Write the results of the primitive into the environment
|
||||
safe_map(write, eqn.outvars, outvals)
|
||||
# Read the final result of the Jaxpr from the environment
|
||||
return safe_map(read, jaxpr.outvars)
|
||||
```
|
||||
|
||||
```{code-cell} ipython3
|
||||
:colab: {}
|
||||
:colab_type: code
|
||||
:id: mGHPc3NruCFV
|
||||
|
||||
closed_jaxpr = jax.make_jaxpr(f)(jnp.ones(5))
|
||||
eval_jaxpr(closed_jaxpr.jaxpr, closed_jaxpr.literals, jnp.ones(5))
|
||||
```
|
||||
|
||||
+++ {"colab_type": "text", "id": "XhZhzbVBvAiT"}
|
||||
|
||||
Notice that `eval_jaxpr` will always return a flat list even if the original function does not.
|
||||
|
||||
Furthermore, this interpreter does not handle `subjaxprs`, which we will not cover in this guide. You can refer to `core.eval_jaxpr` ([link](https://github.com/google/jax/blob/master/jax/core.py#L185-L212)) to see the edge cases that this interpreter does not cover.
|
||||
|
||||
+++ {"colab_type": "text", "id": "0vb2ZoGrCMM4"}
|
||||
|
||||
|
||||
### Custom `inverse` Jaxpr interpreter
|
||||
|
||||
An `inverse` interpreter doesn't look too different from `eval_jaxpr`. We'll first set up the registry which will map primitives to their inverses. We'll then write a custom interpreter that looks up primitives in the registry.
|
||||
|
||||
It turns out that this interpreter will also look similar to the "transpose" interpreter used in reverse-mode autodifferentiation [found here](https://github.com/google/jax/blob/master/jax/interpreters/ad.py#L141-L187).
|
||||
|
||||
```{code-cell} ipython3
|
||||
:colab: {}
|
||||
:colab_type: code
|
||||
:id: gSMIT2z1vUpO
|
||||
|
||||
inverse_registry = {}
|
||||
```
|
||||
|
||||
+++ {"colab_type": "text", "id": "JgrpMgDyCrC7"}
|
||||
|
||||
We'll now register inverses for some of the primitives. By convention, primitives in Jax end in `_p` and a lot of the popular ones live in `lax`.
|
||||
|
||||
```{code-cell} ipython3
|
||||
:colab: {}
|
||||
:colab_type: code
|
||||
:id: fUerorGkCqhw
|
||||
|
||||
inverse_registry[lax.exp_p] = jnp.log
|
||||
inverse_registry[lax.tanh_p] = jnp.arctanh
|
||||
```
|
||||
|
||||
+++ {"colab_type": "text", "id": "mDtH_lYDC5WK"}
|
||||
|
||||
`inverse` will first trace the function, then custom-interpret the Jaxpr. Let's set up a simple skeleton.
|
||||
|
||||
```{code-cell} ipython3
|
||||
:colab: {}
|
||||
:colab_type: code
|
||||
:id: jGNfV6JJC1B3
|
||||
|
||||
def inverse(fun):
|
||||
@wraps(fun)
|
||||
def wrapped(*args, **kwargs):
|
||||
# Since we assume unary functions, we won't
|
||||
# worry about flattening and
|
||||
# unflattening arguments
|
||||
closed_jaxpr = jax.make_jaxpr(fun)(*args, **kwargs)
|
||||
out = inverse_jaxpr(closed_jaxpr.jaxpr, closed_jaxpr.literals, *args)
|
||||
return out[0]
|
||||
return wrapped
|
||||
```
|
||||
|
||||
+++ {"colab_type": "text", "id": "g6v6wV7SDM7g"}
|
||||
|
||||
Now we just need to define `inverse_jaxpr`, which will walk through the Jaxpr backward and invert primitives when it can.
|
||||
|
||||
```{code-cell} ipython3
|
||||
:colab: {}
|
||||
:colab_type: code
|
||||
:id: uUAd-L-BDKT5
|
||||
|
||||
def inverse_jaxpr(jaxpr, consts, *args):
|
||||
env = {}
|
||||
|
||||
def read(var):
|
||||
if type(var) is core.Literal:
|
||||
return var.val
|
||||
return env[var]
|
||||
|
||||
def write(var, val):
|
||||
env[var] = val
|
||||
# Args now correspond to Jaxpr outvars
|
||||
write(core.unitvar, core.unit)
|
||||
safe_map(write, jaxpr.outvars, args)
|
||||
safe_map(write, jaxpr.constvars, consts)
|
||||
|
||||
# Looping backward
|
||||
for eqn in jaxpr.eqns[::-1]:
|
||||
# outvars are now invars
|
||||
invals = safe_map(read, eqn.outvars)
|
||||
if eqn.primitive not in inverse_registry:
|
||||
raise NotImplementedError("{} does not have registered inverse.".format(
|
||||
eqn.primitive
|
||||
))
|
||||
# Assuming a unary function
|
||||
outval = inverse_registry[eqn.primitive](*invals)
|
||||
safe_map(write, eqn.invars, [outval])
|
||||
return safe_map(read, jaxpr.invars)
|
||||
```
|
||||
|
||||
+++ {"colab_type": "text", "id": "M8i3wGbVERhA"}
|
||||
|
||||
That's it!
|
||||
|
||||
```{code-cell} ipython3
|
||||
:colab: {}
|
||||
:colab_type: code
|
||||
:id: cjEKWso-D5Bu
|
||||
|
||||
def f(x):
|
||||
return jnp.exp(jnp.tanh(x))
|
||||
|
||||
f_inv = inverse(f)
|
||||
assert jnp.allclose(f_inv(f(1.0)), 1.0)
|
||||
```
|
||||
|
||||
+++ {"colab_type": "text", "id": "Ny7Oo4WLHdXt"}
|
||||
|
||||
Importantly, you can trace through a Jaxpr interpreter.
|
||||
|
||||
```{code-cell} ipython3
|
||||
:colab: {}
|
||||
:colab_type: code
|
||||
:id: j6ov_rveHmTb
|
||||
|
||||
jax.make_jaxpr(inverse(f))(f(1.))
|
||||
```
|
||||
|
||||
+++ {"colab_type": "text", "id": "yfWVBsKwH0j6"}
|
||||
|
||||
That's all it takes to add a new transformation to a system, and you get composition with all the others for free! For example, we can use `jit`, `vmap`, and `grad` with `inverse`!
|
||||
|
||||
```{code-cell} ipython3
|
||||
:colab: {}
|
||||
:colab_type: code
|
||||
:id: 3tjNk21CH4yZ
|
||||
|
||||
jit(vmap(grad(inverse(f))))((jnp.arange(5) + 1.) / 5.)
|
||||
```
|
||||
|
||||
+++ {"colab_type": "text", "id": "APtG-u_6E4tK"}
|
||||
|
||||
## Exercises for the reader
|
||||
|
||||
* Handle primitives with multiple arguments where inputs are partially known, for example `lax.add_p`, `lax.mul_p`.
|
||||
* Handle `xla_call` and `xla_pmap` primitives, which will not work with both `eval_jaxpr` and `inverse_jaxpr` as written.
|
File diff suppressed because it is too large
Load Diff
585
docs/notebooks/XLA_in_Python.md
Normal file
585
docs/notebooks/XLA_in_Python.md
Normal file
@ -0,0 +1,585 @@
|
||||
---
|
||||
jupytext:
|
||||
formats: ipynb,md:myst
|
||||
text_representation:
|
||||
extension: .md
|
||||
format_name: myst
|
||||
format_version: 0.13
|
||||
jupytext_version: 1.10.0
|
||||
kernelspec:
|
||||
display_name: Python 3
|
||||
language: python
|
||||
name: python3
|
||||
---
|
||||
|
||||
+++ {"id": "sAgUgR5Mzzz2"}
|
||||
|
||||
# XLA in Python
|
||||
|
||||
<img style="height:100px;" src="https://raw.githubusercontent.com/tensorflow/tensorflow/master/tensorflow/compiler/xla/g3doc/images/xlalogo.png"> <img style="height:100px;" src="https://upload.wikimedia.org/wikipedia/commons/c/c3/Python-logo-notext.svg">
|
||||
|
||||
_Anselm Levskaya_, _Qiao Zhang_
|
||||
|
||||
XLA is the compiler that JAX uses, and the compiler that TF uses for TPUs and will soon use for all devices, so it's worth some study. However, it's not exactly easy to play with XLA computations directly using the raw C++ interface. JAX exposes the underlying XLA computation builder API through a python wrapper, and makes interacting with the XLA compute model accessible for messing around and prototyping.
|
||||
|
||||
XLA computations are built as computation graphs in HLO IR, which is then lowered to LLO that is device specific (CPU, GPU, TPU, etc.).
|
||||
|
||||
As end users we interact with the computational primitives offered to us by the HLO spec.
|
||||
|
||||
# Caution: This is a pedagogical notebook covering some low level XLA details, the APIs herein are neither public nor stable!
|
||||
|
||||
+++ {"id": "EZK5RseuvZkr"}
|
||||
|
||||
## References
|
||||
|
||||
__xla__: the doc that defines what's in HLO - but note that the doc is incomplete and omits some ops.
|
||||
|
||||
https://www.tensorflow.org/xla/operation_semantics
|
||||
|
||||
more details on ops in the source code.
|
||||
|
||||
https://github.com/tensorflow/tensorflow/blob/master/tensorflow/compiler/xla/client/xla_builder.h
|
||||
|
||||
__python xla client__: this is the XLA python client for JAX, and what we're using here.
|
||||
|
||||
https://github.com/tensorflow/tensorflow/blob/master/tensorflow/compiler/xla/python/xla_client.py
|
||||
|
||||
https://github.com/tensorflow/tensorflow/blob/master/tensorflow/compiler/xla/python/xla_client_test.py
|
||||
|
||||
__jax__: you can see how jax interacts with the XLA compute layer for execution and JITing in these files.
|
||||
|
||||
https://github.com/google/jax/blob/master/jax/lax.py
|
||||
|
||||
https://github.com/google/jax/blob/master/jax/lib/xla_bridge.py
|
||||
|
||||
https://github.com/google/jax/blob/master/jax/interpreters/xla.py
|
||||
|
||||
+++ {"id": "3XR2NGmrzBGe"}
|
||||
|
||||
## Colab Setup and Imports
|
||||
|
||||
```{code-cell} ipython3
|
||||
:id: Ogo2SBd3u18P
|
||||
|
||||
import numpy as np
|
||||
|
||||
# We only need to import JAX's xla_client, not all of JAX.
|
||||
from jax.lib import xla_client as xc
|
||||
xops = xc.ops
|
||||
|
||||
# Plotting
|
||||
import matplotlib as mpl
|
||||
from matplotlib import pyplot as plt
|
||||
from matplotlib import gridspec
|
||||
from matplotlib import rcParams
|
||||
rcParams['image.interpolation'] = 'nearest'
|
||||
rcParams['image.cmap'] = 'viridis'
|
||||
rcParams['axes.grid'] = False
|
||||
```
|
||||
|
||||
+++ {"id": "odmjXyhMuNJ5"}
|
||||
|
||||
## Simple Computations
|
||||
|
||||
```{code-cell} ipython3
|
||||
---
|
||||
colab:
|
||||
base_uri: https://localhost:8080/
|
||||
id: UYUtxVzMYIiv
|
||||
outputId: 5c603ab4-0295-472c-b462-9928b2a9520d
|
||||
---
|
||||
# make a computation builder
|
||||
c = xc.XlaBuilder("simple_scalar")
|
||||
|
||||
# define a parameter shape and parameter
|
||||
param_shape = xc.Shape.array_shape(np.dtype(np.float32), ())
|
||||
x = xops.Parameter(c, 0, param_shape)
|
||||
|
||||
# define computation graph
|
||||
y = xops.Sin(x)
|
||||
|
||||
# build computation graph
|
||||
# Keep in mind that incorrectly constructed graphs can cause
|
||||
# your notebook kernel to crash!
|
||||
computation = c.Build()
|
||||
|
||||
# get a cpu backend
|
||||
cpu_backend = xc.get_local_backend("cpu")
|
||||
|
||||
# compile graph based on shape
|
||||
compiled_computation = cpu_backend.compile(computation)
|
||||
|
||||
# define a host variable with above parameter shape
|
||||
host_input = np.array(3.0, dtype=np.float32)
|
||||
|
||||
# place host variable on device and execute
|
||||
device_input = cpu_backend.buffer_from_pyval(host_input)
|
||||
device_out = compiled_computation.execute([device_input ,])
|
||||
|
||||
# retrive the result
|
||||
device_out[0].to_py()
|
||||
```
|
||||
|
||||
```{code-cell} ipython3
|
||||
---
|
||||
colab:
|
||||
base_uri: https://localhost:8080/
|
||||
id: rIA-IVMVvQs2
|
||||
outputId: a4d8ef32-43f3-4a48-f732-e85e158b602e
|
||||
---
|
||||
# same as above with vector type:
|
||||
|
||||
c = xc.XlaBuilder("simple_vector")
|
||||
param_shape = xc.Shape.array_shape(np.dtype(np.float32), (3,))
|
||||
x = xops.Parameter(c, 0, param_shape)
|
||||
|
||||
# chain steps by reference:
|
||||
y = xops.Sin(x)
|
||||
z = xops.Abs(y)
|
||||
computation = c.Build()
|
||||
|
||||
# get a cpu backend
|
||||
cpu_backend = xc.get_local_backend("cpu")
|
||||
|
||||
# compile graph based on shape
|
||||
compiled_computation = cpu_backend.compile(computation)
|
||||
|
||||
host_input = np.array([3.0, 4.0, 5.0], dtype=np.float32)
|
||||
|
||||
device_input = cpu_backend.buffer_from_pyval(host_input)
|
||||
device_out = compiled_computation.execute([device_input ,])
|
||||
|
||||
# retrive the result
|
||||
device_out[0].to_py()
|
||||
```
|
||||
|
||||
+++ {"id": "F8kWlLaVuQ1b"}
|
||||
|
||||
## Simple While Loop
|
||||
|
||||
```{code-cell} ipython3
|
||||
---
|
||||
colab:
|
||||
base_uri: https://localhost:8080/
|
||||
id: MDQP1qW515Ao
|
||||
outputId: 53245817-b5fb-4285-ee62-7eb33a822be4
|
||||
---
|
||||
# trivial while loop, decrement until 0
|
||||
# x = 5
|
||||
# while x > 0:
|
||||
# x = x - 1
|
||||
#
|
||||
in_shape = xc.Shape.array_shape(np.dtype(np.int32), ())
|
||||
|
||||
# body computation:
|
||||
bcb = xc.XlaBuilder("bodycomp")
|
||||
x = xops.Parameter(bcb, 0, in_shape)
|
||||
const1 = xops.Constant(bcb, np.int32(1))
|
||||
y = xops.Sub(x, const1)
|
||||
body_computation = bcb.Build()
|
||||
|
||||
# test computation:
|
||||
tcb = xc.XlaBuilder("testcomp")
|
||||
x = xops.Parameter(tcb, 0, in_shape)
|
||||
const0 = xops.Constant(tcb, np.int32(0))
|
||||
y = xops.Gt(x, const0)
|
||||
test_computation = tcb.Build()
|
||||
|
||||
# while computation:
|
||||
wcb = xc.XlaBuilder("whilecomp")
|
||||
x = xops.Parameter(wcb, 0, in_shape)
|
||||
xops.While(test_computation, body_computation, x)
|
||||
while_computation = wcb.Build()
|
||||
|
||||
# Now compile and execute:
|
||||
# get a cpu backend
|
||||
cpu_backend = xc.get_local_backend("cpu")
|
||||
|
||||
# compile graph based on shape
|
||||
compiled_computation = cpu_backend.compile(while_computation)
|
||||
|
||||
host_input = np.array(5, dtype=np.int32)
|
||||
|
||||
device_input = cpu_backend.buffer_from_pyval(host_input)
|
||||
device_out = compiled_computation.execute([device_input ,])
|
||||
|
||||
# retrive the result
|
||||
device_out[0].to_py()
|
||||
```
|
||||
|
||||
+++ {"id": "7UOnXlY8slI6"}
|
||||
|
||||
## While loops w/ Tuples - Newton's Method for sqrt
|
||||
|
||||
```{code-cell} ipython3
|
||||
---
|
||||
colab:
|
||||
base_uri: https://localhost:8080/
|
||||
id: HEWz-vzd6QPR
|
||||
outputId: ad4c4247-8e81-4739-866f-2950fec5e759
|
||||
---
|
||||
Xsqr = 2
|
||||
guess = 1.0
|
||||
converged_delta = 0.001
|
||||
maxit = 1000
|
||||
|
||||
in_shape_0 = xc.Shape.array_shape(np.dtype(np.float32), ())
|
||||
in_shape_1 = xc.Shape.array_shape(np.dtype(np.float32), ())
|
||||
in_shape_2 = xc.Shape.array_shape(np.dtype(np.int32), ())
|
||||
in_tuple_shape = xc.Shape.tuple_shape([in_shape_0, in_shape_1, in_shape_2])
|
||||
|
||||
# body computation:
|
||||
# x_{i+1} = x_i - (x_i**2 - y) / (2 * x_i)
|
||||
bcb = xc.XlaBuilder("bodycomp")
|
||||
intuple = xops.Parameter(bcb, 0, in_tuple_shape)
|
||||
y = xops.GetTupleElement(intuple, 0)
|
||||
x = xops.GetTupleElement(intuple, 1)
|
||||
guard_cntr = xops.GetTupleElement(intuple, 2)
|
||||
new_x = xops.Sub(x, xops.Div(xops.Sub(xops.Mul(x, x), y), xops.Add(x, x)))
|
||||
result = xops.Tuple(bcb, [y, new_x, xops.Sub(guard_cntr, xops.Constant(bcb, np.int32(1)))])
|
||||
body_computation = bcb.Build()
|
||||
|
||||
# test computation -- convergence and max iteration test
|
||||
tcb = xc.XlaBuilder("testcomp")
|
||||
intuple = xops.Parameter(tcb, 0, in_tuple_shape)
|
||||
y = xops.GetTupleElement(intuple, 0)
|
||||
x = xops.GetTupleElement(intuple, 1)
|
||||
guard_cntr = xops.GetTupleElement(intuple, 2)
|
||||
criterion = xops.Abs(xops.Sub(xops.Mul(x, x), y))
|
||||
# stop at convergence criteria or too many iterations
|
||||
test = xops.And(xops.Gt(criterion, xops.Constant(tcb, np.float32(converged_delta))),
|
||||
xops.Gt(guard_cntr, xops.Constant(tcb, np.int32(0))))
|
||||
test_computation = tcb.Build()
|
||||
|
||||
# while computation:
|
||||
# since jax does not allow users to create a tuple input directly, we need to
|
||||
# take multiple parameters and make a intermediate tuple before feeding it as
|
||||
# an initial carry to while loop
|
||||
wcb = xc.XlaBuilder("whilecomp")
|
||||
y = xops.Parameter(wcb, 0, in_shape_0)
|
||||
x = xops.Parameter(wcb, 1, in_shape_1)
|
||||
guard_cntr = xops.Parameter(wcb, 2, in_shape_2)
|
||||
tuple_init_carry = xops.Tuple(wcb, [y, x, guard_cntr])
|
||||
xops.While(test_computation, body_computation, tuple_init_carry)
|
||||
while_computation = wcb.Build()
|
||||
|
||||
# Now compile and execute:
|
||||
cpu_backend = xc.get_local_backend("cpu")
|
||||
|
||||
# compile graph based on shape
|
||||
compiled_computation = cpu_backend.compile(while_computation)
|
||||
|
||||
y = np.array(Xsqr, dtype=np.float32)
|
||||
x = np.array(guess, dtype=np.float32)
|
||||
maxit = np.array(maxit, dtype=np.int32)
|
||||
|
||||
device_input_y = cpu_backend.buffer_from_pyval(y)
|
||||
device_input_x = cpu_backend.buffer_from_pyval(x)
|
||||
device_input_maxit = cpu_backend.buffer_from_pyval(maxit)
|
||||
device_out = compiled_computation.execute([device_input_y, device_input_x, device_input_maxit])
|
||||
|
||||
# retrive the result
|
||||
print("square root of {y} is {x}".format(y=y, x=device_out[1].to_py()))
|
||||
```
|
||||
|
||||
+++ {"id": "yETVIzTInFYr"}
|
||||
|
||||
## Calculate Symm Eigenvalues
|
||||
|
||||
+++ {"id": "AiyR1e2NubKa"}
|
||||
|
||||
Let's exploit the XLA QR implementation to solve some eigenvalues for symmetric matrices.
|
||||
|
||||
This is the naive QR algorithm, without acceleration for closely-spaced eigenvalue convergence, nor any permutation to sort eigenvalues by magnitude.
|
||||
|
||||
```{code-cell} ipython3
|
||||
---
|
||||
colab:
|
||||
base_uri: https://localhost:8080/
|
||||
height: 451
|
||||
id: wjxDPbqCcuXT
|
||||
outputId: 2380db52-799d-494e-ded2-856e91f01b0f
|
||||
---
|
||||
Niter = 200
|
||||
matrix_shape = (10, 10)
|
||||
|
||||
in_shape_0 = xc.Shape.array_shape(np.dtype(np.float32), matrix_shape)
|
||||
in_shape_1 = xc.Shape.array_shape(np.dtype(np.int32), ())
|
||||
in_tuple_shape = xc.Shape.tuple_shape([in_shape_0, in_shape_1])
|
||||
|
||||
# body computation -- QR loop: X_i = Q R , X_{i+1} = R Q
|
||||
|
||||
bcb = xc.XlaBuilder("bodycomp")
|
||||
intuple = xops.Parameter(bcb, 0, in_tuple_shape)
|
||||
x = xops.GetTupleElement(intuple, 0)
|
||||
cntr = xops.GetTupleElement(intuple, 1)
|
||||
Q, R = xops.QR(x, True)
|
||||
RQ = xops.Dot(R, Q)
|
||||
xops.Tuple(bcb, [RQ, xops.Sub(cntr, xops.Constant(bcb, np.int32(1)))])
|
||||
body_computation = bcb.Build()
|
||||
|
||||
# test computation -- just a for loop condition
|
||||
tcb = xc.XlaBuilder("testcomp")
|
||||
intuple = xops.Parameter(tcb, 0, in_tuple_shape)
|
||||
cntr = xops.GetTupleElement(intuple, 1)
|
||||
test = xops.Gt(cntr, xops.Constant(tcb, np.int32(0)))
|
||||
test_computation = tcb.Build()
|
||||
|
||||
# while computation:
|
||||
wcb = xc.XlaBuilder("whilecomp")
|
||||
x = xops.Parameter(wcb, 0, in_shape_0)
|
||||
cntr = xops.Parameter(wcb, 1, in_shape_1)
|
||||
tuple_init_carry = xops.Tuple(wcb, [x, cntr])
|
||||
xops.While(test_computation, body_computation, tuple_init_carry)
|
||||
while_computation = wcb.Build()
|
||||
|
||||
# Now compile and execute:
|
||||
cpu_backend = xc.get_local_backend("cpu")
|
||||
|
||||
# compile graph based on shape
|
||||
compiled_computation = cpu_backend.compile(while_computation)
|
||||
|
||||
X = np.random.random(matrix_shape).astype(np.float32)
|
||||
X = (X + X.T) / 2.0
|
||||
it = np.array(Niter, dtype=np.int32)
|
||||
|
||||
device_input_x = cpu_backend.buffer_from_pyval(X)
|
||||
device_input_it = cpu_backend.buffer_from_pyval(it)
|
||||
device_out = compiled_computation.execute([device_input_x, device_input_it])
|
||||
|
||||
host_out = device_out[0].to_py()
|
||||
eigh_vals = host_out.diagonal()
|
||||
|
||||
plt.title('D')
|
||||
plt.imshow(host_out)
|
||||
print('sorted eigenvalues')
|
||||
print(np.sort(eigh_vals))
|
||||
print('sorted eigenvalues from numpy')
|
||||
print(np.sort(np.linalg.eigh(X)[0]))
|
||||
print('sorted error')
|
||||
print(np.sort(eigh_vals) - np.sort(np.linalg.eigh(X)[0]))
|
||||
```
|
||||
|
||||
+++ {"id": "FpggTihknAOw"}
|
||||
|
||||
## Calculate Full Symm Eigensystem
|
||||
|
||||
+++ {"id": "Qos4ankYuj1T"}
|
||||
|
||||
We can also calculate the eigenbasis by accumulating the Qs.
|
||||
|
||||
```{code-cell} ipython3
|
||||
---
|
||||
colab:
|
||||
base_uri: https://localhost:8080/
|
||||
height: 979
|
||||
id: Kp3A-aAiZk0g
|
||||
outputId: bbaff039-20f4-45cd-b8fe-5a664d413f5b
|
||||
---
|
||||
Niter = 100
|
||||
matrix_shape = (10, 10)
|
||||
|
||||
in_shape_0 = xc.Shape.array_shape(np.dtype(np.float32), matrix_shape)
|
||||
in_shape_1 = xc.Shape.array_shape(np.dtype(np.float32), matrix_shape)
|
||||
in_shape_2 = xc.Shape.array_shape(np.dtype(np.int32), ())
|
||||
in_tuple_shape = xc.Shape.tuple_shape([in_shape_0, in_shape_1, in_shape_2])
|
||||
|
||||
# body computation -- QR loop: X_i = Q R , X_{i+1} = R Q
|
||||
bcb = xc.XlaBuilder("bodycomp")
|
||||
intuple = xops.Parameter(bcb, 0, in_tuple_shape)
|
||||
X = xops.GetTupleElement(intuple, 0)
|
||||
O = xops.GetTupleElement(intuple, 1)
|
||||
cntr = xops.GetTupleElement(intuple, 2)
|
||||
Q, R = xops.QR(X, True)
|
||||
RQ = xops.Dot(R, Q)
|
||||
Onew = xops.Dot(O, Q)
|
||||
xops.Tuple(bcb, [RQ, Onew, xops.Sub(cntr, xops.Constant(bcb, np.int32(1)))])
|
||||
body_computation = bcb.Build()
|
||||
|
||||
# test computation -- just a for loop condition
|
||||
tcb = xc.XlaBuilder("testcomp")
|
||||
intuple = xops.Parameter(tcb, 0, in_tuple_shape)
|
||||
cntr = xops.GetTupleElement(intuple, 2)
|
||||
test = xops.Gt(cntr, xops.Constant(tcb, np.int32(0)))
|
||||
test_computation = tcb.Build()
|
||||
|
||||
# while computation:
|
||||
wcb = xc.XlaBuilder("whilecomp")
|
||||
X = xops.Parameter(wcb, 0, in_shape_0)
|
||||
O = xops.Parameter(wcb, 1, in_shape_1)
|
||||
cntr = xops.Parameter(wcb, 2, in_shape_2)
|
||||
tuple_init_carry = xops.Tuple(wcb, [X, O, cntr])
|
||||
xops.While(test_computation, body_computation, tuple_init_carry)
|
||||
while_computation = wcb.Build()
|
||||
|
||||
# Now compile and execute:
|
||||
cpu_backend = xc.get_local_backend("cpu")
|
||||
|
||||
# compile graph based on shape
|
||||
compiled_computation = cpu_backend.compile(while_computation)
|
||||
|
||||
X = np.random.random(matrix_shape).astype(np.float32)
|
||||
X = (X + X.T) / 2.0
|
||||
Omat = np.eye(matrix_shape[0], dtype=np.float32)
|
||||
it = np.array(Niter, dtype=np.int32)
|
||||
|
||||
device_input_X = cpu_backend.buffer_from_pyval(X)
|
||||
device_input_Omat = cpu_backend.buffer_from_pyval(Omat)
|
||||
device_input_it = cpu_backend.buffer_from_pyval(it)
|
||||
device_out = compiled_computation.execute([device_input_X, device_input_Omat, device_input_it])
|
||||
|
||||
host_out = device_out[0].to_py()
|
||||
eigh_vals = host_out.diagonal()
|
||||
eigh_mat = device_out[1].to_py()
|
||||
|
||||
plt.title('D')
|
||||
plt.imshow(host_out)
|
||||
plt.figure()
|
||||
plt.title('U')
|
||||
plt.imshow(eigh_mat)
|
||||
plt.figure()
|
||||
plt.title('U^T A U')
|
||||
plt.imshow(np.dot(np.dot(eigh_mat.T, X), eigh_mat))
|
||||
print('sorted eigenvalues')
|
||||
print(np.sort(eigh_vals))
|
||||
print('sorted eigenvalues from numpy')
|
||||
print(np.sort(np.linalg.eigh(X)[0]))
|
||||
print('sorted error')
|
||||
print(np.sort(eigh_vals) - np.sort(np.linalg.eigh(X)[0]))
|
||||
```
|
||||
|
||||
+++ {"id": "Ee3LMzOvlCuK"}
|
||||
|
||||
## Convolutions
|
||||
|
||||
I keep hearing from the AGI folks that we can use convolutions to build artificial life. Let's try it out.
|
||||
|
||||
```{code-cell} ipython3
|
||||
:id: 9xh6yeXKS9Vg
|
||||
|
||||
# Here we borrow convenience functions from LAX to handle conv dimension numbers.
|
||||
from typing import NamedTuple, Sequence
|
||||
|
||||
class ConvDimensionNumbers(NamedTuple):
|
||||
"""Describes batch, spatial, and feature dimensions of a convolution.
|
||||
|
||||
Args:
|
||||
lhs_spec: a tuple of nonnegative integer dimension numbers containing
|
||||
`(batch dimension, feature dimension, spatial dimensions...)`.
|
||||
rhs_spec: a tuple of nonnegative integer dimension numbers containing
|
||||
`(out feature dimension, in feature dimension, spatial dimensions...)`.
|
||||
out_spec: a tuple of nonnegative integer dimension numbers containing
|
||||
`(batch dimension, feature dimension, spatial dimensions...)`.
|
||||
"""
|
||||
lhs_spec: Sequence[int]
|
||||
rhs_spec: Sequence[int]
|
||||
out_spec: Sequence[int]
|
||||
|
||||
def _conv_general_proto(dimension_numbers):
|
||||
assert type(dimension_numbers) is ConvDimensionNumbers
|
||||
lhs_spec, rhs_spec, out_spec = dimension_numbers
|
||||
proto = xc.ConvolutionDimensionNumbers()
|
||||
proto.input_batch_dimension = lhs_spec[0]
|
||||
proto.input_feature_dimension = lhs_spec[1]
|
||||
proto.output_batch_dimension = out_spec[0]
|
||||
proto.output_feature_dimension = out_spec[1]
|
||||
proto.kernel_output_feature_dimension = rhs_spec[0]
|
||||
proto.kernel_input_feature_dimension = rhs_spec[1]
|
||||
proto.input_spatial_dimensions.extend(lhs_spec[2:])
|
||||
proto.kernel_spatial_dimensions.extend(rhs_spec[2:])
|
||||
proto.output_spatial_dimensions.extend(out_spec[2:])
|
||||
return proto
|
||||
```
|
||||
|
||||
```{code-cell} ipython3
|
||||
---
|
||||
colab:
|
||||
base_uri: https://localhost:8080/
|
||||
height: 110
|
||||
id: J8QkirDalBse
|
||||
outputId: 543a03fd-f038-46f2-9a76-a6532b86874e
|
||||
---
|
||||
Niter=13
|
||||
matrix_shape = (1, 1, 20, 20)
|
||||
in_shape_0 = xc.Shape.array_shape(np.dtype(np.int32), matrix_shape)
|
||||
in_shape_1 = xc.Shape.array_shape(np.dtype(np.int32), ())
|
||||
in_tuple_shape = xc.Shape.tuple_shape([in_shape_0, in_shape_1])
|
||||
|
||||
# Body computation -- Conway Update
|
||||
bcb = xc.XlaBuilder("bodycomp")
|
||||
intuple = xops.Parameter(bcb, 0, in_tuple_shape)
|
||||
x = xops.GetTupleElement(intuple, 0)
|
||||
cntr = xops.GetTupleElement(intuple, 1)
|
||||
# convs require floating-point type
|
||||
xf = xops.ConvertElementType(x, xc.DTYPE_TO_XLA_ELEMENT_TYPE['float32'])
|
||||
stamp = xops.Constant(bcb, np.ones((1,1,3,3), dtype=np.float32))
|
||||
conv_dim_num_proto = _conv_general_proto(ConvDimensionNumbers(lhs_spec=(0,1,2,3), rhs_spec=(0,1,2,3), out_spec=(0,1,2,3)))
|
||||
convd = xops.ConvGeneralDilated(xf, stamp, [1, 1], [(1, 1), (1, 1)], (), (), conv_dim_num_proto)
|
||||
# # logic ops require integer types
|
||||
convd = xops.ConvertElementType(convd, xc.DTYPE_TO_XLA_ELEMENT_TYPE['int32'])
|
||||
bool_x = xops.Eq(x, xops.Constant(bcb, np.int32(1)))
|
||||
# core update rule
|
||||
res = xops.Or(
|
||||
# birth rule
|
||||
xops.And(xops.Not(bool_x), xops.Eq(convd, xops.Constant(bcb, np.int32(3)))),
|
||||
# survival rule
|
||||
xops.And(bool_x, xops.Or(
|
||||
# these are +1 the normal numbers since conv-sum counts self
|
||||
xops.Eq(convd, xops.Constant(bcb, np.int32(4))),
|
||||
xops.Eq(convd, xops.Constant(bcb, np.int32(3))))
|
||||
)
|
||||
)
|
||||
# Convert output back to int type for type constancy
|
||||
int_res = xops.ConvertElementType(res, xc.DTYPE_TO_XLA_ELEMENT_TYPE['int32'])
|
||||
xops.Tuple(bcb, [int_res, xops.Sub(cntr, xops.Constant(bcb, np.int32(1)))])
|
||||
body_computation = bcb.Build()
|
||||
|
||||
# Test computation -- just a for loop condition
|
||||
tcb = xc.XlaBuilder("testcomp")
|
||||
intuple = xops.Parameter(tcb, 0, in_tuple_shape)
|
||||
cntr = xops.GetTupleElement(intuple, 1)
|
||||
test = xops.Gt(cntr, xops.Constant(tcb, np.int32(0)))
|
||||
test_computation = tcb.Build()
|
||||
|
||||
# While computation:
|
||||
wcb = xc.XlaBuilder("whilecomp")
|
||||
x = xops.Parameter(wcb, 0, in_shape_0)
|
||||
cntr = xops.Parameter(wcb, 1, in_shape_1)
|
||||
tuple_init_carry = xops.Tuple(wcb, [x, cntr])
|
||||
xops.While(test_computation, body_computation, tuple_init_carry)
|
||||
while_computation = wcb.Build()
|
||||
|
||||
# Now compile and execute:
|
||||
cpu_backend = xc.get_local_backend("cpu")
|
||||
|
||||
# compile graph based on shape
|
||||
compiled_computation = cpu_backend.compile(while_computation)
|
||||
|
||||
# Set up initial state
|
||||
X = np.zeros(matrix_shape, dtype=np.int32)
|
||||
X[0,0, 5:8, 5:8] = np.array([[0,1,0],[0,0,1],[1,1,1]])
|
||||
|
||||
# Evolve
|
||||
movie = np.zeros((Niter,)+matrix_shape[-2:], dtype=np.int32)
|
||||
for it in range(Niter):
|
||||
itr = np.array(it, dtype=np.int32)
|
||||
device_input_x = cpu_backend.buffer_from_pyval(X)
|
||||
device_input_it = cpu_backend.buffer_from_pyval(itr)
|
||||
device_out = compiled_computation.execute([device_input_x, device_input_it])
|
||||
movie[it] = device_out[0].to_py()[0,0]
|
||||
|
||||
# Plot
|
||||
fig = plt.figure(figsize=(15,2))
|
||||
gs = gridspec.GridSpec(1,Niter)
|
||||
for i in range(Niter):
|
||||
ax1 = plt.subplot(gs[:, i])
|
||||
ax1.axis('off')
|
||||
ax1.imshow(movie[i])
|
||||
plt.subplots_adjust(left=0.0, right=1.0, top=1.0, bottom=0.0, hspace=0.0, wspace=0.05)
|
||||
```
|
||||
|
||||
+++ {"id": "9-0PJlqv237S"}
|
||||
|
||||
## Fin
|
||||
|
||||
There's much more to XLA, but this hopefully highlights how easy it is to play with via the python client!
|
File diff suppressed because it is too large
Load Diff
1024
docs/notebooks/autodiff_cookbook.md
Normal file
1024
docs/notebooks/autodiff_cookbook.md
Normal file
File diff suppressed because it is too large
Load Diff
File diff suppressed because one or more lines are too long
482
docs/notebooks/maml.md
Normal file
482
docs/notebooks/maml.md
Normal file
@ -0,0 +1,482 @@
|
||||
---
|
||||
jupytext:
|
||||
formats: ipynb,md:myst
|
||||
text_representation:
|
||||
extension: .md
|
||||
format_name: myst
|
||||
format_version: 0.13
|
||||
jupytext_version: 1.10.0
|
||||
kernelspec:
|
||||
display_name: Python 3
|
||||
name: python3
|
||||
---
|
||||
|
||||
+++ {"id": "oDP4nK_Zgyg-", "colab_type": "text"}
|
||||
|
||||
# MAML Tutorial with JAX
|
||||
|
||||
Eric Jang
|
||||
|
||||
Blog post: https://blog.evjang.com/2019/02/maml-jax.html
|
||||
|
||||
|
||||
21 Feb 2019
|
||||
|
||||
Pedagogical tutorial for implementing Model-Agnostic Meta-Learning with JAX's awesome `grad` and `vmap` and `jit` operators.
|
||||
|
||||
## Overview
|
||||
|
||||
In this notebook we'll go through:
|
||||
|
||||
- how to take gradients, gradients of gradients.
|
||||
- how to fit a sinusoid function with a neural network (and do auto-batching with vmap)
|
||||
- how to implement MAML and check its numerics
|
||||
- how to implement MAML for sinusoid task (single-task objective, batching task instances).
|
||||
- extending MAML to handle batching at the task-level
|
||||
|
||||
```{code-cell} ipython3
|
||||
:colab: {}
|
||||
:colab_type: code
|
||||
:id: zKVdo3FtgyhE
|
||||
|
||||
### import jax.numpy (almost-drop-in for numpy) and gradient operators.
|
||||
import jax.numpy as jnp
|
||||
from jax import grad
|
||||
```
|
||||
|
||||
+++ {"id": "gMgclHhxgyhI", "colab_type": "text"}
|
||||
|
||||
## Gradients of Gradients
|
||||
|
||||
JAX makes it easy to compute gradients of python functions. Here, we thrice-differentiate $e^x$ and $x^2$
|
||||
|
||||
```{code-cell} ipython3
|
||||
---
|
||||
colab:
|
||||
base_uri: https://localhost:8080/
|
||||
height: 123
|
||||
colab_type: code
|
||||
id: Mt-uRwBGgyhJ
|
||||
outputId: db7f718c-c2fb-4f7e-f31c-39a0d36c7051
|
||||
---
|
||||
f = lambda x : jnp.exp(x)
|
||||
g = lambda x : jnp.square(x)
|
||||
print(grad(f)(1.)) # = e^{1}
|
||||
print(grad(grad(f))(1.))
|
||||
print(grad(grad(grad(f)))(1.))
|
||||
|
||||
print(grad(g)(2.)) # 2x = 4
|
||||
print(grad(grad(g))(2.)) # x = 2
|
||||
print(grad(grad(grad(g)))(2.)) # x = 0
|
||||
```
|
||||
|
||||
+++ {"id": "7mAd3We_gyhP", "colab_type": "text"}
|
||||
|
||||
## Sinusoid Regression and vmap
|
||||
|
||||
To get you familiar with JAX syntax first, we'll optimize neural network params with fixed inputs on a mean-squared error loss to $f_\theta(x) = sin(x)$.
|
||||
|
||||
```{code-cell} ipython3
|
||||
:colab: {}
|
||||
:colab_type: code
|
||||
:id: JN9KA1PvgyhQ
|
||||
|
||||
from jax import vmap # for auto-vectorizing functions
|
||||
from functools import partial # for use with vmap
|
||||
from jax import jit # for compiling functions for speedup
|
||||
from jax import random # stax initialization uses jax.random
|
||||
from jax.experimental import stax # neural network library
|
||||
from jax.experimental.stax import Conv, Dense, MaxPool, Relu, Flatten, LogSoftmax # neural network layers
|
||||
import matplotlib.pyplot as plt # visualization
|
||||
```
|
||||
|
||||
```{code-cell} ipython3
|
||||
:colab: {}
|
||||
:colab_type: code
|
||||
:id: DeEALFIHgyhU
|
||||
|
||||
# Use stax to set up network initialization and evaluation functions
|
||||
net_init, net_apply = stax.serial(
|
||||
Dense(40), Relu,
|
||||
Dense(40), Relu,
|
||||
Dense(1)
|
||||
)
|
||||
|
||||
rng = random.PRNGKey(0)
|
||||
in_shape = (-1, 1,)
|
||||
out_shape, net_params = net_init(rng, in_shape)
|
||||
```
|
||||
|
||||
```{code-cell} ipython3
|
||||
:colab: {}
|
||||
:colab_type: code
|
||||
:id: izIi-P1agyhY
|
||||
|
||||
def loss(params, inputs, targets):
|
||||
# Computes average loss for the batch
|
||||
predictions = net_apply(params, inputs)
|
||||
return jnp.mean((targets - predictions)**2)
|
||||
```
|
||||
|
||||
```{code-cell} ipython3
|
||||
---
|
||||
colab:
|
||||
base_uri: https://localhost:8080/
|
||||
height: 287
|
||||
colab_type: code
|
||||
id: sROmpDEmgyhb
|
||||
outputId: d1bf00d7-99e7-445e-b439-ea2fabd7a646
|
||||
---
|
||||
# batch the inference across K=100
|
||||
xrange_inputs = jnp.linspace(-5,5,100).reshape((100, 1)) # (k, 1)
|
||||
targets = jnp.sin(xrange_inputs)
|
||||
predictions = vmap(partial(net_apply, net_params))(xrange_inputs)
|
||||
losses = vmap(partial(loss, net_params))(xrange_inputs, targets) # per-input loss
|
||||
plt.plot(xrange_inputs, predictions, label='prediction')
|
||||
plt.plot(xrange_inputs, losses, label='loss')
|
||||
plt.plot(xrange_inputs, targets, label='target')
|
||||
plt.legend()
|
||||
```
|
||||
|
||||
```{code-cell} ipython3
|
||||
:colab: {}
|
||||
:colab_type: code
|
||||
:id: PxAEhrPGgyhh
|
||||
|
||||
import numpy as np
|
||||
from jax.experimental import optimizers
|
||||
from jax.tree_util import tree_multimap # Element-wise manipulation of collections of numpy arrays
|
||||
```
|
||||
|
||||
```{code-cell} ipython3
|
||||
:colab: {}
|
||||
:colab_type: code
|
||||
:id: iZtAZfEZgyhk
|
||||
|
||||
opt_init, opt_update, get_params = optimizers.adam(step_size=1e-2)
|
||||
opt_state = opt_init(net_params)
|
||||
|
||||
# Define a compiled update step
|
||||
@jit
|
||||
def step(i, opt_state, x1, y1):
|
||||
p = get_params(opt_state)
|
||||
g = grad(loss)(p, x1, y1)
|
||||
return opt_update(i, g, opt_state)
|
||||
|
||||
for i in range(100):
|
||||
opt_state = step(i, opt_state, xrange_inputs, targets)
|
||||
net_params = get_params(opt_state)
|
||||
```
|
||||
|
||||
```{code-cell} ipython3
|
||||
---
|
||||
colab:
|
||||
base_uri: https://localhost:8080/
|
||||
height: 287
|
||||
colab_type: code
|
||||
id: Rm9WIz2egyho
|
||||
outputId: 183de82d-fdf0-4b81-9b14-01a85e6b8839
|
||||
---
|
||||
# batch the inference across K=100
|
||||
targets = jnp.sin(xrange_inputs)
|
||||
predictions = vmap(partial(net_apply, net_params))(xrange_inputs)
|
||||
losses = vmap(partial(loss, net_params))(xrange_inputs, targets) # per-input loss
|
||||
plt.plot(xrange_inputs, predictions, label='prediction')
|
||||
plt.plot(xrange_inputs, losses, label='loss')
|
||||
plt.plot(xrange_inputs, targets, label='target')
|
||||
plt.legend()
|
||||
```
|
||||
|
||||
+++ {"id": "7E8gAJBzgyhs", "colab_type": "text"}
|
||||
|
||||
## MAML: Optimizing for Generalization
|
||||
|
||||
Suppose task loss function $\mathcal{L}$ is defined with respect to model parameters $\theta$, input features $X$, input labels $Y$. MAML optimizes the following:
|
||||
|
||||
$\mathcal{L}(\theta - \nabla \mathcal{L}(\theta, x_1, y_1), x_2, y_2)$
|
||||
|
||||
$x_1, y_2$ and $x_2, y_2$ are identically distributed from $X, Y$. Therefore, MAML objective can be thought of as a differentiable cross-validation error (w.r.t. $x_2, y_2$) for a model that learns (via a single gradient descent step) from $x_1, y_1$. Minimizing cross-validation error provides an inductive bias on generalization.
|
||||
|
||||
The following toy example checks MAML numerics via parameter $x$ and input $y$.
|
||||
|
||||
```{code-cell} ipython3
|
||||
---
|
||||
colab:
|
||||
base_uri: https://localhost:8080/
|
||||
height: 88
|
||||
colab_type: code
|
||||
id: 2YBFsM2dgyht
|
||||
outputId: 46160194-04b7-46c9-897d-ecb11e9738be
|
||||
---
|
||||
# gradients of gradients test for MAML
|
||||
# check numerics
|
||||
g = lambda x, y : jnp.square(x) + y
|
||||
x0 = 2.
|
||||
y0 = 1.
|
||||
print('grad(g)(x0) = {}'.format(grad(g)(x0, y0))) # 2x = 4
|
||||
print('x0 - grad(g)(x0) = {}'.format(x0 - grad(g)(x0, y0))) # x - 2x = -2
|
||||
def maml_objective(x, y):
|
||||
return g(x - grad(g)(x, y), y)
|
||||
print('maml_objective(x,y)={}'.format(maml_objective(x0, y0))) # x**2 + 1 = 5
|
||||
print('x0 - maml_objective(x,y) = {}'.format(x0 - grad(maml_objective)(x0, y0))) # x - (2x)
|
||||
```
|
||||
|
||||
+++ {"id": "V9G-PMxygyhx", "colab_type": "text"}
|
||||
|
||||
## Sinusoid Task + MAML
|
||||
|
||||
|
||||
Now let's re-implement the Sinusoidal regression task from Chelsea Finn's [MAML paper](https://arxiv.org/abs/1703.03400).
|
||||
|
||||
```{code-cell} ipython3
|
||||
:colab: {}
|
||||
:colab_type: code
|
||||
:id: s1v5VABkgyhy
|
||||
|
||||
alpha = .1
|
||||
def inner_update(p, x1, y1):
|
||||
grads = grad(loss)(p, x1, y1)
|
||||
inner_sgd_fn = lambda g, state: (state - alpha*g)
|
||||
return tree_multimap(inner_sgd_fn, grads, p)
|
||||
|
||||
def maml_loss(p, x1, y1, x2, y2):
|
||||
p2 = inner_update(p, x1, y1)
|
||||
return loss(p2, x2, y2)
|
||||
```
|
||||
|
||||
```{code-cell} ipython3
|
||||
---
|
||||
colab:
|
||||
base_uri: https://localhost:8080/
|
||||
height: 35
|
||||
colab_type: code
|
||||
id: bQvg749Xgyh2
|
||||
outputId: 5043f859-c537-41b8-c390-23670795d57b
|
||||
---
|
||||
x1 = xrange_inputs
|
||||
y1 = targets
|
||||
x2 = jnp.array([0.])
|
||||
y2 = jnp.array([0.])
|
||||
maml_loss(net_params, x1, y1, x2, y2)
|
||||
```
|
||||
|
||||
+++ {"id": "zMB6BwPogyh6", "colab_type": "text"}
|
||||
|
||||
Let's try minimizing the MAML loss (without batching across multiple tasks, which we will do in the next section)
|
||||
|
||||
```{code-cell} ipython3
|
||||
---
|
||||
colab:
|
||||
base_uri: https://localhost:8080/
|
||||
height: 371
|
||||
colab_type: code
|
||||
id: pB5ldBO-gyh7
|
||||
outputId: b2365aa4-d7b8-40a0-d759-8257d3e4d768
|
||||
---
|
||||
opt_init, opt_update, get_params = optimizers.adam(step_size=1e-3) # this LR seems to be better than 1e-2 and 1e-4
|
||||
out_shape, net_params = net_init(rng, in_shape)
|
||||
opt_state = opt_init(net_params)
|
||||
|
||||
@jit
|
||||
def step(i, opt_state, x1, y1, x2, y2):
|
||||
p = get_params(opt_state)
|
||||
g = grad(maml_loss)(p, x1, y1, x2, y2)
|
||||
l = maml_loss(p, x1, y1, x2, y2)
|
||||
return opt_update(i, g, opt_state), l
|
||||
K=20
|
||||
|
||||
np_maml_loss = []
|
||||
|
||||
# Adam optimization
|
||||
for i in range(20000):
|
||||
# define the task
|
||||
A = np.random.uniform(low=0.1, high=.5)
|
||||
phase = np.random.uniform(low=0., high=jnp.pi)
|
||||
# meta-training inner split (K examples)
|
||||
x1 = np.random.uniform(low=-5., high=5., size=(K,1))
|
||||
y1 = A * np.sin(x1 + phase)
|
||||
# meta-training outer split (1 example). Like cross-validating with respect to one example.
|
||||
x2 = np.random.uniform(low=-5., high=5.)
|
||||
y2 = A * np.sin(x2 + phase)
|
||||
opt_state, l = step(i, opt_state, x1, y1, x2, y2)
|
||||
np_maml_loss.append(l)
|
||||
if i % 1000 == 0:
|
||||
print(i)
|
||||
net_params = get_params(opt_state)
|
||||
```
|
||||
|
||||
```{code-cell} ipython3
|
||||
---
|
||||
colab:
|
||||
base_uri: https://localhost:8080/
|
||||
height: 287
|
||||
colab_type: code
|
||||
id: ogcpFdJ9gyh_
|
||||
outputId: 856924a3-ede5-44ba-ba3c-381673713fad
|
||||
---
|
||||
# batch the inference across K=100
|
||||
targets = jnp.sin(xrange_inputs)
|
||||
predictions = vmap(partial(net_apply, net_params))(xrange_inputs)
|
||||
plt.plot(xrange_inputs, predictions, label='pre-update predictions')
|
||||
plt.plot(xrange_inputs, targets, label='target')
|
||||
|
||||
x1 = np.random.uniform(low=-5., high=5., size=(K,1))
|
||||
y1 = 1. * np.sin(x1 + 0.)
|
||||
|
||||
for i in range(1,5):
|
||||
net_params = inner_update(net_params, x1, y1)
|
||||
predictions = vmap(partial(net_apply, net_params))(xrange_inputs)
|
||||
plt.plot(xrange_inputs, predictions, label='{}-shot predictions'.format(i))
|
||||
plt.legend()
|
||||
```
|
||||
|
||||
+++ {"id": "7TMYcZKVgyiD", "colab_type": "text"}
|
||||
|
||||
## Batching Meta-Gradient Across Tasks
|
||||
|
||||
Kind of does the job but not that great. Let's reduce the variance of gradients in outer loop by averaging across a batch of tasks (not just one task at a time).
|
||||
|
||||
vmap is awesome it enables nice handling of batching at two levels: inner-level "intra-task" batching, and outer level batching across tasks.
|
||||
|
||||
From a software engineering perspective, it is nice because the "task-batched" MAML implementation simply re-uses code from the non-task batched MAML algorithm, without losing any vectorization benefits.
|
||||
|
||||
```{code-cell} ipython3
|
||||
:colab: {}
|
||||
:colab_type: code
|
||||
:id: 9Pj04Z7MgyiF
|
||||
|
||||
def sample_tasks(outer_batch_size, inner_batch_size):
|
||||
# Select amplitude and phase for the task
|
||||
As = []
|
||||
phases = []
|
||||
for _ in range(outer_batch_size):
|
||||
As.append(np.random.uniform(low=0.1, high=.5))
|
||||
phases.append(np.random.uniform(low=0., high=jnp.pi))
|
||||
def get_batch():
|
||||
xs, ys = [], []
|
||||
for A, phase in zip(As, phases):
|
||||
x = np.random.uniform(low=-5., high=5., size=(inner_batch_size, 1))
|
||||
y = A * np.sin(x + phase)
|
||||
xs.append(x)
|
||||
ys.append(y)
|
||||
return jnp.stack(xs), jnp.stack(ys)
|
||||
x1, y1 = get_batch()
|
||||
x2, y2 = get_batch()
|
||||
return x1, y1, x2, y2
|
||||
```
|
||||
|
||||
```{code-cell} ipython3
|
||||
---
|
||||
colab:
|
||||
base_uri: https://localhost:8080/
|
||||
height: 287
|
||||
colab_type: code
|
||||
id: 7dCIGObKgyiJ
|
||||
outputId: c169b529-0f16-4f20-d20e-d802765e4068
|
||||
---
|
||||
outer_batch_size = 2
|
||||
x1, y1, x2, y2 = sample_tasks(outer_batch_size, 50)
|
||||
for i in range(outer_batch_size):
|
||||
plt.scatter(x1[i], y1[i], label='task{}-train'.format(i))
|
||||
for i in range(outer_batch_size):
|
||||
plt.scatter(x2[i], y2[i], label='task{}-val'.format(i))
|
||||
plt.legend()
|
||||
```
|
||||
|
||||
```{code-cell} ipython3
|
||||
---
|
||||
colab:
|
||||
base_uri: https://localhost:8080/
|
||||
height: 35
|
||||
colab_type: code
|
||||
id: BrSX--wpgyiP
|
||||
outputId: 6d81e7ff-7cd9-4aef-c665-952d442369d5
|
||||
---
|
||||
x2.shape
|
||||
```
|
||||
|
||||
```{code-cell} ipython3
|
||||
---
|
||||
colab:
|
||||
base_uri: https://localhost:8080/
|
||||
height: 371
|
||||
colab_type: code
|
||||
id: P3WQ8_k2gyiU
|
||||
outputId: fed1b78b-7910-4e44-a80b-18f447379022
|
||||
---
|
||||
opt_init, opt_update, get_params = optimizers.adam(step_size=1e-3)
|
||||
out_shape, net_params = net_init(rng, in_shape)
|
||||
opt_state = opt_init(net_params)
|
||||
|
||||
# vmapped version of maml loss.
|
||||
# returns scalar for all tasks.
|
||||
def batch_maml_loss(p, x1_b, y1_b, x2_b, y2_b):
|
||||
task_losses = vmap(partial(maml_loss, p))(x1_b, y1_b, x2_b, y2_b)
|
||||
return jnp.mean(task_losses)
|
||||
|
||||
@jit
|
||||
def step(i, opt_state, x1, y1, x2, y2):
|
||||
p = get_params(opt_state)
|
||||
g = grad(batch_maml_loss)(p, x1, y1, x2, y2)
|
||||
l = batch_maml_loss(p, x1, y1, x2, y2)
|
||||
return opt_update(i, g, opt_state), l
|
||||
|
||||
np_batched_maml_loss = []
|
||||
K=20
|
||||
for i in range(20000):
|
||||
x1_b, y1_b, x2_b, y2_b = sample_tasks(4, K)
|
||||
opt_state, l = step(i, opt_state, x1_b, y1_b, x2_b, y2_b)
|
||||
np_batched_maml_loss.append(l)
|
||||
if i % 1000 == 0:
|
||||
print(i)
|
||||
net_params = get_params(opt_state)
|
||||
```
|
||||
|
||||
```{code-cell} ipython3
|
||||
---
|
||||
colab:
|
||||
base_uri: https://localhost:8080/
|
||||
height: 287
|
||||
colab_type: code
|
||||
id: PmxHLrhYgyiX
|
||||
outputId: 33ac699e-c66d-46e2-affa-98ae948d52e8
|
||||
---
|
||||
# batch the inference across K=100
|
||||
targets = jnp.sin(xrange_inputs)
|
||||
predictions = vmap(partial(net_apply, net_params))(xrange_inputs)
|
||||
plt.plot(xrange_inputs, predictions, label='pre-update predictions')
|
||||
plt.plot(xrange_inputs, targets, label='target')
|
||||
|
||||
x1 = np.random.uniform(low=-5., high=5., size=(10,1))
|
||||
y1 = 1. * np.sin(x1 + 0.)
|
||||
|
||||
for i in range(1,3):
|
||||
net_params = inner_update(net_params, x1, y1)
|
||||
predictions = vmap(partial(net_apply, net_params))(xrange_inputs)
|
||||
plt.plot(xrange_inputs, predictions, label='{}-shot predictions'.format(i))
|
||||
plt.legend()
|
||||
```
|
||||
|
||||
```{code-cell} ipython3
|
||||
---
|
||||
colab:
|
||||
base_uri: https://localhost:8080/
|
||||
height: 287
|
||||
colab_type: code
|
||||
id: cQf2BeDjgyib
|
||||
outputId: fc52caf6-1379-4d60-fe44-99f4e4518698
|
||||
---
|
||||
# Comparison of maml_loss for task batch size = 1 vs. task batch size = 8
|
||||
plt.plot(np.convolve(np_maml_loss, [.05]*20), label='task_batch=1')
|
||||
plt.plot(np.convolve(np_batched_maml_loss, [.05]*20), label='task_batch=4')
|
||||
plt.ylim(0., 1e-1)
|
||||
plt.legend()
|
||||
```
|
||||
|
||||
```{code-cell} ipython3
|
||||
:colab: {}
|
||||
:colab_type: code
|
||||
:id: vCHCvXh-mm1v
|
||||
|
||||
|
||||
```
|
@ -462,6 +462,9 @@
|
||||
"toc_visible": true,
|
||||
"version": "0.3.2"
|
||||
},
|
||||
"jupytext": {
|
||||
"formats": "ipynb,md:myst"
|
||||
},
|
||||
"kernelspec": {
|
||||
"display_name": "Python 3",
|
||||
"language": "python",
|
||||
|
279
docs/notebooks/neural_network_with_tfds_data.md
Normal file
279
docs/notebooks/neural_network_with_tfds_data.md
Normal file
@ -0,0 +1,279 @@
|
||||
---
|
||||
jupytext:
|
||||
formats: ipynb,md:myst
|
||||
text_representation:
|
||||
extension: .md
|
||||
format_name: myst
|
||||
format_version: 0.13
|
||||
jupytext_version: 1.10.0
|
||||
kernelspec:
|
||||
display_name: Python 3
|
||||
language: python
|
||||
name: python3
|
||||
---
|
||||
|
||||
+++ {"colab_type": "text", "id": "18AF5Ab4p6VL"}
|
||||
|
||||
##### Copyright 2018 Google LLC.
|
||||
|
||||
Licensed under the Apache License, Version 2.0 (the "License");
|
||||
|
||||
+++ {"colab_type": "text", "id": "crfqaJOyp8bq"}
|
||||
|
||||
Licensed under the Apache License, Version 2.0 (the "License");
|
||||
you may not use this file except in compliance with the License.
|
||||
You may obtain a copy of the License at
|
||||
|
||||
https://www.apache.org/licenses/LICENSE-2.0
|
||||
|
||||
Unless required by applicable law or agreed to in writing, software
|
||||
distributed under the License is distributed on an "AS IS" BASIS,
|
||||
WITHOUT WARRANTIES OR CONDITIONS OF ANY KIND, either express or implied.
|
||||
See the License for the specific language governing permissions and
|
||||
limitations under the License.
|
||||
|
||||
+++ {"colab_type": "text", "id": "B_XlLLpcWjkA"}
|
||||
|
||||
# Training a Simple Neural Network, with tensorflow/datasets Data Loading
|
||||
|
||||
_Forked from_ `neural_network_and_data_loading.ipynb`
|
||||
|
||||
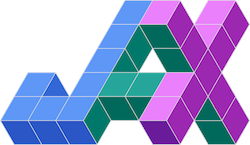
|
||||
|
||||
Let's combine everything we showed in the [quickstart notebook](https://colab.research.google.com/github/google/jax/blob/master/notebooks/quickstart.ipynb) to train a simple neural network. We will first specify and train a simple MLP on MNIST using JAX for the computation. We will use `tensorflow/datasets` data loading API to load images and labels (because it's pretty great, and the world doesn't need yet another data loading library :P).
|
||||
|
||||
Of course, you can use JAX with any API that is compatible with NumPy to make specifying the model a bit more plug-and-play. Here, just for explanatory purposes, we won't use any neural network libraries or special APIs for builidng our model.
|
||||
|
||||
```{code-cell} ipython3
|
||||
:colab: {}
|
||||
:colab_type: code
|
||||
:id: OksHydJDtbbI
|
||||
|
||||
import jax.numpy as jnp
|
||||
from jax import grad, jit, vmap
|
||||
from jax import random
|
||||
```
|
||||
|
||||
+++ {"colab_type": "text", "id": "MTVcKi-ZYB3R"}
|
||||
|
||||
### Hyperparameters
|
||||
Let's get a few bookkeeping items out of the way.
|
||||
|
||||
```{code-cell} ipython3
|
||||
:colab: {}
|
||||
:colab_type: code
|
||||
:id: -fmWA06xYE7d
|
||||
:outputId: 520e5fd5-97c4-43eb-ef0e-b714d5287689
|
||||
|
||||
# A helper function to randomly initialize weights and biases
|
||||
# for a dense neural network layer
|
||||
def random_layer_params(m, n, key, scale=1e-2):
|
||||
w_key, b_key = random.split(key)
|
||||
return scale * random.normal(w_key, (n, m)), scale * random.normal(b_key, (n,))
|
||||
|
||||
# Initialize all layers for a fully-connected neural network with sizes "sizes"
|
||||
def init_network_params(sizes, key):
|
||||
keys = random.split(key, len(sizes))
|
||||
return [random_layer_params(m, n, k) for m, n, k in zip(sizes[:-1], sizes[1:], keys)]
|
||||
|
||||
layer_sizes = [784, 512, 512, 10]
|
||||
param_scale = 0.1
|
||||
step_size = 0.01
|
||||
num_epochs = 10
|
||||
batch_size = 128
|
||||
n_targets = 10
|
||||
params = init_network_params(layer_sizes, random.PRNGKey(0))
|
||||
```
|
||||
|
||||
+++ {"colab_type": "text", "id": "BtoNk_yxWtIw"}
|
||||
|
||||
### Auto-batching predictions
|
||||
|
||||
Let us first define our prediction function. Note that we're defining this for a _single_ image example. We're going to use JAX's `vmap` function to automatically handle mini-batches, with no performance penalty.
|
||||
|
||||
```{code-cell} ipython3
|
||||
:colab: {}
|
||||
:colab_type: code
|
||||
:id: 7APc6tD7TiuZ
|
||||
|
||||
from jax.scipy.special import logsumexp
|
||||
|
||||
def relu(x):
|
||||
return jnp.maximum(0, x)
|
||||
|
||||
def predict(params, image):
|
||||
# per-example predictions
|
||||
activations = image
|
||||
for w, b in params[:-1]:
|
||||
outputs = jnp.dot(w, activations) + b
|
||||
activations = relu(outputs)
|
||||
|
||||
final_w, final_b = params[-1]
|
||||
logits = jnp.dot(final_w, activations) + final_b
|
||||
return logits - logsumexp(logits)
|
||||
```
|
||||
|
||||
+++ {"colab_type": "text", "id": "dRW_TvCTWgaP"}
|
||||
|
||||
Let's check that our prediction function only works on single images.
|
||||
|
||||
```{code-cell} ipython3
|
||||
:colab: {}
|
||||
:colab_type: code
|
||||
:id: 4sW2A5mnXHc5
|
||||
:outputId: ce9d86ed-a830-4832-e04d-10d1abb1fb8a
|
||||
|
||||
# This works on single examples
|
||||
random_flattened_image = random.normal(random.PRNGKey(1), (28 * 28,))
|
||||
preds = predict(params, random_flattened_image)
|
||||
print(preds.shape)
|
||||
```
|
||||
|
||||
```{code-cell} ipython3
|
||||
:colab: {}
|
||||
:colab_type: code
|
||||
:id: PpyQxuedXfhp
|
||||
:outputId: f43bbc9d-bc8f-4168-ee7b-79ee9d33f245
|
||||
|
||||
# Doesn't work with a batch
|
||||
random_flattened_images = random.normal(random.PRNGKey(1), (10, 28 * 28))
|
||||
try:
|
||||
preds = predict(params, random_flattened_images)
|
||||
except TypeError:
|
||||
print('Invalid shapes!')
|
||||
```
|
||||
|
||||
```{code-cell} ipython3
|
||||
:colab: {}
|
||||
:colab_type: code
|
||||
:id: oJOOncKMXbwK
|
||||
:outputId: fa380024-aaf8-4789-d3a2-f060134930e6
|
||||
|
||||
# Let's upgrade it to handle batches using `vmap`
|
||||
|
||||
# Make a batched version of the `predict` function
|
||||
batched_predict = vmap(predict, in_axes=(None, 0))
|
||||
|
||||
# `batched_predict` has the same call signature as `predict`
|
||||
batched_preds = batched_predict(params, random_flattened_images)
|
||||
print(batched_preds.shape)
|
||||
```
|
||||
|
||||
+++ {"colab_type": "text", "id": "elsG6nX03BvW"}
|
||||
|
||||
At this point, we have all the ingredients we need to define our neural network and train it. We've built an auto-batched version of `predict`, which we should be able to use in a loss function. We should be able to use `grad` to take the derivative of the loss with respect to the neural network parameters. Last, we should be able to use `jit` to speed up everything.
|
||||
|
||||
+++ {"colab_type": "text", "id": "NwDuFqc9X7ER"}
|
||||
|
||||
### Utility and loss functions
|
||||
|
||||
```{code-cell} ipython3
|
||||
:colab: {}
|
||||
:colab_type: code
|
||||
:id: 6lTI6I4lWdh5
|
||||
|
||||
def one_hot(x, k, dtype=jnp.float32):
|
||||
"""Create a one-hot encoding of x of size k."""
|
||||
return jnp.array(x[:, None] == jnp.arange(k), dtype)
|
||||
|
||||
def accuracy(params, images, targets):
|
||||
target_class = jnp.argmax(targets, axis=1)
|
||||
predicted_class = jnp.argmax(batched_predict(params, images), axis=1)
|
||||
return jnp.mean(predicted_class == target_class)
|
||||
|
||||
def loss(params, images, targets):
|
||||
preds = batched_predict(params, images)
|
||||
return -jnp.mean(preds * targets)
|
||||
|
||||
@jit
|
||||
def update(params, x, y):
|
||||
grads = grad(loss)(params, x, y)
|
||||
return [(w - step_size * dw, b - step_size * db)
|
||||
for (w, b), (dw, db) in zip(params, grads)]
|
||||
```
|
||||
|
||||
+++ {"colab_type": "text", "id": "umJJGZCC2oKl"}
|
||||
|
||||
### Data Loading with `tensorflow/datasets`
|
||||
|
||||
JAX is laser-focused on program transformations and accelerator-backed NumPy, so we don't include data loading or munging in the JAX library. There are already a lot of great data loaders out there, so let's just use them instead of reinventing anything. We'll use the `tensorflow/datasets` data loader.
|
||||
|
||||
```{code-cell} ipython3
|
||||
:colab: {}
|
||||
:colab_type: code
|
||||
:id: uWvo1EgZCvnK
|
||||
|
||||
import tensorflow_datasets as tfds
|
||||
|
||||
data_dir = '/tmp/tfds'
|
||||
|
||||
# Fetch full datasets for evaluation
|
||||
# tfds.load returns tf.Tensors (or tf.data.Datasets if batch_size != -1)
|
||||
# You can convert them to NumPy arrays (or iterables of NumPy arrays) with tfds.dataset_as_numpy
|
||||
mnist_data, info = tfds.load(name="mnist", batch_size=-1, data_dir=data_dir, with_info=True)
|
||||
mnist_data = tfds.as_numpy(mnist_data)
|
||||
train_data, test_data = mnist_data['train'], mnist_data['test']
|
||||
num_labels = info.features['label'].num_classes
|
||||
h, w, c = info.features['image'].shape
|
||||
num_pixels = h * w * c
|
||||
|
||||
# Full train set
|
||||
train_images, train_labels = train_data['image'], train_data['label']
|
||||
train_images = jnp.reshape(train_images, (len(train_images), num_pixels))
|
||||
train_labels = one_hot(train_labels, num_labels)
|
||||
|
||||
# Full test set
|
||||
test_images, test_labels = test_data['image'], test_data['label']
|
||||
test_images = jnp.reshape(test_images, (len(test_images), num_pixels))
|
||||
test_labels = one_hot(test_labels, num_labels)
|
||||
```
|
||||
|
||||
```{code-cell} ipython3
|
||||
:colab: {}
|
||||
:colab_type: code
|
||||
:id: 7VMSC03gCvnO
|
||||
:outputId: e565586e-d598-4fa1-dd6f-10ba39617f6a
|
||||
|
||||
print('Train:', train_images.shape, train_labels.shape)
|
||||
print('Test:', test_images.shape, test_labels.shape)
|
||||
```
|
||||
|
||||
+++ {"colab_type": "text", "id": "xxPd6Qw3Z98v"}
|
||||
|
||||
### Training Loop
|
||||
|
||||
```{code-cell} ipython3
|
||||
:colab: {}
|
||||
:colab_type: code
|
||||
:id: X2DnZo3iYj18
|
||||
:outputId: bad334e0-127a-40fe-ec21-b0db77c73088
|
||||
|
||||
import time
|
||||
|
||||
def get_train_batches():
|
||||
# as_supervised=True gives us the (image, label) as a tuple instead of a dict
|
||||
ds = tfds.load(name='mnist', split='train', as_supervised=True, data_dir=data_dir)
|
||||
# You can build up an arbitrary tf.data input pipeline
|
||||
ds = ds.batch(batch_size).prefetch(1)
|
||||
# tfds.dataset_as_numpy converts the tf.data.Dataset into an iterable of NumPy arrays
|
||||
return tfds.as_numpy(ds)
|
||||
|
||||
for epoch in range(num_epochs):
|
||||
start_time = time.time()
|
||||
for x, y in get_train_batches():
|
||||
x = jnp.reshape(x, (len(x), num_pixels))
|
||||
y = one_hot(y, num_labels)
|
||||
params = update(params, x, y)
|
||||
epoch_time = time.time() - start_time
|
||||
|
||||
train_acc = accuracy(params, train_images, train_labels)
|
||||
test_acc = accuracy(params, test_images, test_labels)
|
||||
print("Epoch {} in {:0.2f} sec".format(epoch, epoch_time))
|
||||
print("Training set accuracy {}".format(train_acc))
|
||||
print("Test set accuracy {}".format(test_acc))
|
||||
```
|
||||
|
||||
+++ {"colab_type": "text", "id": "xC1CMcVNYwxm"}
|
||||
|
||||
We've now used the whole of the JAX API: `grad` for derivatives, `jit` for speedups and `vmap` for auto-vectorization.
|
||||
We used NumPy to specify all of our computation, and borrowed the great data loaders from `tensorflow/datasets`, and ran the whole thing on the GPU.
|
File diff suppressed because it is too large
Load Diff
317
docs/notebooks/quickstart.md
Normal file
317
docs/notebooks/quickstart.md
Normal file
@ -0,0 +1,317 @@
|
||||
---
|
||||
jupytext:
|
||||
formats: ipynb,md:myst
|
||||
text_representation:
|
||||
extension: .md
|
||||
format_name: myst
|
||||
format_version: 0.13
|
||||
jupytext_version: 1.10.0
|
||||
kernelspec:
|
||||
display_name: Python 3
|
||||
language: python
|
||||
name: python3
|
||||
---
|
||||
|
||||
+++ {"colab_type": "text", "id": "xtWX4x9DCF5_"}
|
||||
|
||||
# JAX Quickstart
|
||||
|
||||
**JAX is NumPy on the CPU, GPU, and TPU, with great automatic differentiation for high-performance machine learning research.**
|
||||
|
||||
With its updated version of [Autograd](https://github.com/hips/autograd), JAX
|
||||
can automatically differentiate native Python and NumPy code. It can
|
||||
differentiate through a large subset of Python’s features, including loops, ifs,
|
||||
recursion, and closures, and it can even take derivatives of derivatives of
|
||||
derivatives. It supports reverse-mode as well as forward-mode differentiation, and the two can be composed arbitrarily
|
||||
to any order.
|
||||
|
||||
What’s new is that JAX uses
|
||||
[XLA](https://www.tensorflow.org/xla)
|
||||
to compile and run your NumPy code on accelerators, like GPUs and TPUs.
|
||||
Compilation happens under the hood by default, with library calls getting
|
||||
just-in-time compiled and executed. But JAX even lets you just-in-time compile
|
||||
your own Python functions into XLA-optimized kernels using a one-function API.
|
||||
Compilation and automatic differentiation can be composed arbitrarily, so you
|
||||
can express sophisticated algorithms and get maximal performance without having
|
||||
to leave Python.
|
||||
|
||||
```{code-cell}
|
||||
:colab: {}
|
||||
:colab_type: code
|
||||
:id: SY8mDvEvCGqk
|
||||
|
||||
import jax.numpy as jnp
|
||||
from jax import grad, jit, vmap
|
||||
from jax import random
|
||||
```
|
||||
|
||||
+++ {"colab_type": "text", "id": "FQ89jHCYfhpg"}
|
||||
|
||||
## Multiplying Matrices
|
||||
|
||||
+++ {"colab_type": "text", "id": "Xpy1dSgNqCP4"}
|
||||
|
||||
We'll be generating random data in the following examples. One big difference between NumPy and JAX is how you generate random numbers. For more details, see [Common Gotchas in JAX].
|
||||
|
||||
[Common Gotchas in JAX]: https://jax.readthedocs.io/en/latest/notebooks/Common_Gotchas_in_JAX.html#%F0%9F%94%AA-Random-Numbers
|
||||
|
||||
```{code-cell}
|
||||
:colab: {}
|
||||
:colab_type: code
|
||||
:id: u0nseKZNqOoH
|
||||
|
||||
key = random.PRNGKey(0)
|
||||
x = random.normal(key, (10,))
|
||||
print(x)
|
||||
```
|
||||
|
||||
+++ {"colab_type": "text", "id": "hDJF0UPKnuqB"}
|
||||
|
||||
Let's dive right in and multiply two big matrices.
|
||||
|
||||
```{code-cell}
|
||||
:colab: {}
|
||||
:colab_type: code
|
||||
:id: eXn8GUl6CG5N
|
||||
|
||||
size = 3000
|
||||
x = random.normal(key, (size, size), dtype=jnp.float32)
|
||||
%timeit jnp.dot(x, x.T).block_until_ready() # runs on the GPU
|
||||
```
|
||||
|
||||
+++ {"colab_type": "text", "id": "0AlN7EbonyaR"}
|
||||
|
||||
We added that `block_until_ready` because [JAX uses asynchronous execution by default](https://jax.readthedocs.io/en/latest/async_dispatch.html).
|
||||
|
||||
JAX NumPy functions work on regular NumPy arrays.
|
||||
|
||||
```{code-cell}
|
||||
:colab: {}
|
||||
:colab_type: code
|
||||
:id: ZPl0MuwYrM7t
|
||||
|
||||
import numpy as np
|
||||
x = np.random.normal(size=(size, size)).astype(np.float32)
|
||||
%timeit jnp.dot(x, x.T).block_until_ready()
|
||||
```
|
||||
|
||||
+++ {"colab_type": "text", "id": "_SrcB2IurUuE"}
|
||||
|
||||
That's slower because it has to transfer data to the GPU every time. You can ensure that an NDArray is backed by device memory using `device_put`.
|
||||
|
||||
```{code-cell}
|
||||
:colab: {}
|
||||
:colab_type: code
|
||||
:id: Jj7M7zyRskF0
|
||||
|
||||
from jax import device_put
|
||||
|
||||
x = np.random.normal(size=(size, size)).astype(np.float32)
|
||||
x = device_put(x)
|
||||
%timeit jnp.dot(x, x.T).block_until_ready()
|
||||
```
|
||||
|
||||
+++ {"colab_type": "text", "id": "clO9djnen8qi"}
|
||||
|
||||
The output of `device_put` still acts like an NDArray, but it only copies values back to the CPU when they're needed for printing, plotting, saving to disk, branching, etc. The behavior of `device_put` is equivalent to the function `jit(lambda x: x)`, but it's faster.
|
||||
|
||||
+++ {"colab_type": "text", "id": "ghkfKNQttDpg"}
|
||||
|
||||
If you have a GPU (or TPU!) these calls run on the accelerator and have the potential to be much faster than on CPU.
|
||||
|
||||
```{code-cell}
|
||||
:colab: {}
|
||||
:colab_type: code
|
||||
:id: RzXK8GnIs7VV
|
||||
|
||||
x = np.random.normal(size=(size, size)).astype(np.float32)
|
||||
%timeit np.dot(x, x.T)
|
||||
```
|
||||
|
||||
+++ {"colab_type": "text", "id": "iOzp0P_GoJhb"}
|
||||
|
||||
JAX is much more than just a GPU-backed NumPy. It also comes with a few program transformations that are useful when writing numerical code. For now, there's three main ones:
|
||||
|
||||
- `jit`, for speeding up your code
|
||||
- `grad`, for taking derivatives
|
||||
- `vmap`, for automatic vectorization or batching.
|
||||
|
||||
Let's go over these, one-by-one. We'll also end up composing these in interesting ways.
|
||||
|
||||
+++ {"colab_type": "text", "id": "bTTrTbWvgLUK"}
|
||||
|
||||
## Using `jit` to speed up functions
|
||||
|
||||
+++ {"colab_type": "text", "id": "YrqE32mvE3b7"}
|
||||
|
||||
JAX runs transparently on the GPU (or CPU, if you don't have one, and TPU coming soon!). However, in the above example, JAX is dispatching kernels to the GPU one operation at a time. If we have a sequence of operations, we can use the `@jit` decorator to compile multiple operations together using [XLA](https://www.tensorflow.org/xla). Let's try that.
|
||||
|
||||
```{code-cell}
|
||||
:colab: {}
|
||||
:colab_type: code
|
||||
:id: qLGdCtFKFLOR
|
||||
|
||||
def selu(x, alpha=1.67, lmbda=1.05):
|
||||
return lmbda * jnp.where(x > 0, x, alpha * jnp.exp(x) - alpha)
|
||||
|
||||
x = random.normal(key, (1000000,))
|
||||
%timeit selu(x).block_until_ready()
|
||||
```
|
||||
|
||||
+++ {"colab_type": "text", "id": "a_V8SruVHrD_"}
|
||||
|
||||
We can speed it up with `@jit`, which will jit-compile the first time `selu` is called and will be cached thereafter.
|
||||
|
||||
```{code-cell}
|
||||
:colab: {}
|
||||
:colab_type: code
|
||||
:id: fh4w_3NpFYTp
|
||||
|
||||
selu_jit = jit(selu)
|
||||
%timeit selu_jit(x).block_until_ready()
|
||||
```
|
||||
|
||||
+++ {"colab_type": "text", "id": "HxpBc4WmfsEU"}
|
||||
|
||||
## Taking derivatives with `grad`
|
||||
|
||||
In addition to evaluating numerical functions, we also want to transform them. One transformation is [automatic differentiation](https://en.wikipedia.org/wiki/Automatic_differentiation). In JAX, just like in [Autograd](https://github.com/HIPS/autograd), you can compute gradients with the `grad` function.
|
||||
|
||||
```{code-cell}
|
||||
:colab: {}
|
||||
:colab_type: code
|
||||
:id: IMAgNJaMJwPD
|
||||
|
||||
def sum_logistic(x):
|
||||
return jnp.sum(1.0 / (1.0 + jnp.exp(-x)))
|
||||
|
||||
x_small = jnp.arange(3.)
|
||||
derivative_fn = grad(sum_logistic)
|
||||
print(derivative_fn(x_small))
|
||||
```
|
||||
|
||||
+++ {"colab_type": "text", "id": "PtNs881Ohioc"}
|
||||
|
||||
Let's verify with finite differences that our result is correct.
|
||||
|
||||
```{code-cell}
|
||||
:colab: {}
|
||||
:colab_type: code
|
||||
:id: JXI7_OZuKZVO
|
||||
|
||||
def first_finite_differences(f, x):
|
||||
eps = 1e-3
|
||||
return jnp.array([(f(x + eps * v) - f(x - eps * v)) / (2 * eps)
|
||||
for v in jnp.eye(len(x))])
|
||||
|
||||
|
||||
print(first_finite_differences(sum_logistic, x_small))
|
||||
```
|
||||
|
||||
+++ {"colab_type": "text", "id": "Q2CUZjOWNZ-3"}
|
||||
|
||||
Taking derivatives is as easy as calling `grad`. `grad` and `jit` compose and can be mixed arbitrarily. In the above example we jitted `sum_logistic` and then took its derivative. We can go further:
|
||||
|
||||
```{code-cell}
|
||||
:colab: {}
|
||||
:colab_type: code
|
||||
:id: TO4g8ny-OEi4
|
||||
|
||||
print(grad(jit(grad(jit(grad(sum_logistic)))))(1.0))
|
||||
```
|
||||
|
||||
+++ {"colab_type": "text", "id": "yCJ5feKvhnBJ"}
|
||||
|
||||
For more advanced autodiff, you can use `jax.vjp` for reverse-mode vector-Jacobian products and `jax.jvp` for forward-mode Jacobian-vector products. The two can be composed arbitrarily with one another, and with other JAX transformations. Here's one way to compose them to make a function that efficiently computes full Hessian matrices:
|
||||
|
||||
```{code-cell}
|
||||
:colab: {}
|
||||
:colab_type: code
|
||||
:id: Z-JxbiNyhxEW
|
||||
|
||||
from jax import jacfwd, jacrev
|
||||
def hessian(fun):
|
||||
return jit(jacfwd(jacrev(fun)))
|
||||
```
|
||||
|
||||
+++ {"colab_type": "text", "id": "TI4nPsGafxbL"}
|
||||
|
||||
## Auto-vectorization with `vmap`
|
||||
|
||||
+++ {"colab_type": "text", "id": "PcxkONy5aius"}
|
||||
|
||||
JAX has one more transformation in its API that you might find useful: `vmap`, the vectorizing map. It has the familiar semantics of mapping a function along array axes, but instead of keeping the loop on the outside, it pushes the loop down into a function’s primitive operations for better performance. When composed with `jit`, it can be just as fast as adding the batch dimensions by hand.
|
||||
|
||||
+++ {"colab_type": "text", "id": "TPiX4y-bWLFS"}
|
||||
|
||||
We're going to work with a simple example, and promote matrix-vector products into matrix-matrix products using `vmap`. Although this is easy to do by hand in this specific case, the same technique can apply to more complicated functions.
|
||||
|
||||
```{code-cell}
|
||||
:colab: {}
|
||||
:colab_type: code
|
||||
:id: 8w0Gpsn8WYYj
|
||||
|
||||
mat = random.normal(key, (150, 100))
|
||||
batched_x = random.normal(key, (10, 100))
|
||||
|
||||
def apply_matrix(v):
|
||||
return jnp.dot(mat, v)
|
||||
```
|
||||
|
||||
+++ {"id": "0zWsc0RisQWx", "colab_type": "text"}
|
||||
|
||||
Given a function such as `apply_matrix`, we can loop over a batch dimension in Python, but usually the performance of doing so is poor.
|
||||
|
||||
```{code-cell}
|
||||
:colab: {}
|
||||
:colab_type: code
|
||||
:id: KWVc9BsZv0Ki
|
||||
|
||||
def naively_batched_apply_matrix(v_batched):
|
||||
return jnp.stack([apply_matrix(v) for v in v_batched])
|
||||
|
||||
print('Naively batched')
|
||||
%timeit naively_batched_apply_matrix(batched_x).block_until_ready()
|
||||
```
|
||||
|
||||
+++ {"id": "qHfKaLE9stbA", "colab_type": "text"}
|
||||
|
||||
We know how to batch this operation manually. In this case, `jnp.dot` handles extra batch dimensions transparently.
|
||||
|
||||
```{code-cell}
|
||||
:colab: {}
|
||||
:colab_type: code
|
||||
:id: ipei6l8nvrzH
|
||||
|
||||
@jit
|
||||
def batched_apply_matrix(v_batched):
|
||||
return jnp.dot(v_batched, mat.T)
|
||||
|
||||
print('Manually batched')
|
||||
%timeit batched_apply_matrix(batched_x).block_until_ready()
|
||||
```
|
||||
|
||||
+++ {"id": "1eF8Nhb-szAb", "colab_type": "text"}
|
||||
|
||||
However, suppose we had a more complicated function without batching support. We can use `vmap` to add batching support automatically.
|
||||
|
||||
```{code-cell}
|
||||
:colab: {}
|
||||
:colab_type: code
|
||||
:id: 67Oeknf5vuCl
|
||||
|
||||
@jit
|
||||
def vmap_batched_apply_matrix(v_batched):
|
||||
return vmap(apply_matrix)(v_batched)
|
||||
|
||||
print('Auto-vectorized with vmap')
|
||||
%timeit vmap_batched_apply_matrix(batched_x).block_until_ready()
|
||||
```
|
||||
|
||||
+++ {"colab_type": "text", "id": "pYVl3Z2nbZhO"}
|
||||
|
||||
Of course, `vmap` can be arbitrarily composed with `jit`, `grad`, and any other JAX transformation.
|
||||
|
||||
+++ {"id": "WwNnjaI4th_8", "colab_type": "text"}
|
||||
|
||||
This is just a taste of what JAX can do. We're really excited to see what you do with it!
|
File diff suppressed because one or more lines are too long
502
docs/notebooks/score_matching.md
Normal file
502
docs/notebooks/score_matching.md
Normal file
@ -0,0 +1,502 @@
|
||||
---
|
||||
jupytext:
|
||||
formats: ipynb,md:myst
|
||||
text_representation:
|
||||
extension: .md
|
||||
format_name: myst
|
||||
format_version: 0.13
|
||||
jupytext_version: 1.10.0
|
||||
kernelspec:
|
||||
display_name: Python 3
|
||||
language: python
|
||||
name: python3
|
||||
---
|
||||
|
||||
+++ {"colab_type": "text", "id": "U6IRW9a8G6TB"}
|
||||
|
||||
# Generative Modeling by Estimating Gradients of Data Distribution in JAX
|
||||
|
||||
[](https://colab.sandbox.google.com/github/google/jax/blob/master/docs/notebooks/score_matching.ipynb)
|
||||
|
||||
In this notebook we'll implement __Generative Modeling by Estimating Gradients of the Data Distribution__ [[arxiv]](https://arxiv.org/abs/1907.05600).
|
||||
|
||||
The paper builds on a technique called __Score Matching__ to iteratively refine images into samples from the real data. This technique takes advantage of some recent theorems to learn the gradients of data probability distribution. Implementing this in your typical DL framework would become an issue. However, [__`JAX`__](https://github.com/google/jax) ain't your typical DL framework. JAX makes implementing complex math no harder than writing it on a piece of paper.
|
||||
|
||||
Let's begin with a simple task: learn to sample from a peculiar probability distribution. Like a swiss roll, for example:
|
||||
|
||||

|
||||
|
||||
well... minus the chocolate. Sorry for that.
|
||||
|
||||
```{code-cell} ipython3
|
||||
---
|
||||
colab:
|
||||
base_uri: https://localhost:8080/
|
||||
height: 286
|
||||
colab_type: code
|
||||
id: 0P1xCZPNG6TE
|
||||
outputId: 69be38a1-1f02-462e-f4f1-16a41c35fddf
|
||||
---
|
||||
import matplotlib.pyplot as plt
|
||||
%matplotlib inline
|
||||
import numpy as np
|
||||
|
||||
from sklearn.datasets import make_swiss_roll
|
||||
|
||||
def sample_batch(size, noise=1.0):
|
||||
x, _= make_swiss_roll(size, noise=noise)
|
||||
x = x[:, [0, 2]] / 10.0
|
||||
return np.array(x)
|
||||
|
||||
plt.scatter(*sample_batch(10**4).T, alpha=0.1)
|
||||
```
|
||||
|
||||
+++ {"colab_type": "text", "id": "5X-LN4rwG6TH"}
|
||||
|
||||
### Compute score matching objective
|
||||
|
||||
The method we apply here was originally proposed by [Hyvarinen et al. (2005)](http://jmlr.org/papers/volume6/hyvarinen05a/old.pdf). The idea behind score matching is to __learn scores:__ the gradients of $\log p(x)$ w.r.t. $x$. When trained this model can "improve" a sample $x$ by changing it in the direction of highest log-probability. However, training such model can get tricky. When predicting a continuous variable, ML folks usually minimize squared error:
|
||||
|
||||
$$ L_{mse} = E_{x \sim p(x)} \left\lVert model(x) - \nabla_x \log p(x) \right\lVert_2^2 $$
|
||||
|
||||
One can't minimize this explicitly because the real $\nabla_x log p(x)$ is usually unknown. However under broad assumptions on p(x) and a sufficiently powerful model, one can say that ` ... math happens ... ` and therefore the arg-minimum of $L_{mse}$ can be found by minimizing a more tractable objective:
|
||||
|
||||
$$ L_{matching} = E_{x \sim p(x)} \space tr( \space \mathbf{J}_x [\space model(x) \space]) + \frac12 \left\Vert model(x) \right\lVert_2^2 $$
|
||||
|
||||
Here $tr( \space \mathbf{J}_x [\space model(x) \space])$ is a trace of Jacobian of $model(x)$ w.r.t. $x$. Now all it takes is to minimize the second objective with backpropagation... that is, if you can compute jacobians. Thankfully, we have __jax__!
|
||||
|
||||
```{code-cell} ipython3
|
||||
:colab: {}
|
||||
:colab_type: code
|
||||
:id: 98wjxKcNG6TI
|
||||
|
||||
import jax
|
||||
import jax.numpy as jnp
|
||||
from jax.experimental import optimizers
|
||||
from jax.experimental import stax
|
||||
from functools import partial
|
||||
|
||||
# Set up network to predict scores
|
||||
net_init, net_apply = stax.serial(
|
||||
stax.Dense(128), stax.Softplus,
|
||||
stax.Dense(128), stax.Softplus,
|
||||
stax.Dense(2),
|
||||
)
|
||||
|
||||
# Create optimizer. Note that both network and optimizer returns pure (stateless) functions
|
||||
opt_init, opt_update, get_params = optimizers.adam(1e-3)
|
||||
```
|
||||
|
||||
```{code-cell} ipython3
|
||||
:colab: {}
|
||||
:colab_type: code
|
||||
:id: FgH-YVaZG6TJ
|
||||
|
||||
# v-- jax.jit compiles a function for efficient CPU and GPU execution
|
||||
|
||||
@jax.jit
|
||||
def compute_loss(net_params, inputs):
|
||||
# v-- a function that computes jacobian by forward mode differentiation
|
||||
jacobian = jax.jacfwd(net_apply, argnums=-1)
|
||||
|
||||
# we use jax.vmap to vectorize jacobian function along batch dimension
|
||||
batch_jacobian = jax.vmap(partial(jacobian, net_params))(inputs) # [batch, dim, dim]
|
||||
|
||||
trace_jacobian = jnp.trace(batch_jacobian, axis1=1, axis2=2)
|
||||
output_norm_sq = jnp.square(net_apply(net_params, inputs)).sum(axis=1)
|
||||
|
||||
return jnp.mean(trace_jacobian + 1/2 * output_norm_sq)
|
||||
|
||||
|
||||
@jax.jit
|
||||
def train_step(step_i, opt_state, batch):
|
||||
net_params = get_params(opt_state)
|
||||
loss = compute_loss(net_params, batch)
|
||||
grads = jax.grad(compute_loss, argnums=0)(net_params, batch)
|
||||
return loss, opt_update(step_i, grads, opt_state)
|
||||
|
||||
```
|
||||
|
||||
+++ {"id": "LkTYRi6qCwn8", "colab_type": "text"}
|
||||
|
||||
__Note__: we use `jax.jacfwd` since the input dimension is only 2
|
||||
|
||||
+++ {"colab_type": "text", "id": "Qxza8fDvG6TL"}
|
||||
|
||||
### Training loop
|
||||
|
||||
```{code-cell} ipython3
|
||||
:colab: {}
|
||||
:colab_type: code
|
||||
:id: NNlbbWNIG6TM
|
||||
|
||||
from IPython.display import clear_output
|
||||
|
||||
out_shape, net_params = net_init(jax.random.PRNGKey(seed=42), input_shape=(-1, 2))
|
||||
opt_state = opt_init(net_params)
|
||||
|
||||
loss_history = []
|
||||
```
|
||||
|
||||
```{code-cell} ipython3
|
||||
---
|
||||
colab:
|
||||
base_uri: https://localhost:8080/
|
||||
height: 499
|
||||
colab_type: code
|
||||
id: evDOnCHiG6TN
|
||||
outputId: 989db5fe-24a2-41ba-fb01-6d981df7cd06
|
||||
---
|
||||
for i in range(2000):
|
||||
x = sample_batch(size=128)
|
||||
loss, opt_state = train_step(i, opt_state, x)
|
||||
loss_history.append(loss.item())
|
||||
|
||||
if i % 200 == 0:
|
||||
clear_output(True)
|
||||
plt.figure(figsize=[16, 8])
|
||||
plt.subplot(1, 2, 1)
|
||||
plt.title("mean loss = %.3f" % jnp.mean(jnp.array(loss_history[-32:])))
|
||||
plt.scatter(jnp.arange(len(loss_history)), loss_history)
|
||||
plt.grid()
|
||||
|
||||
plt.subplot(1, 2, 2)
|
||||
net_params = get_params(opt_state)
|
||||
xx = jnp.stack(jnp.meshgrid(jnp.linspace(-1.5, 2.0, 50), jnp.linspace(-1.5, 2.0, 50)), axis=-1).reshape(-1, 2)
|
||||
scores = net_apply(net_params, xx)
|
||||
scores_norm = jnp.linalg.norm(scores, axis=-1, ord=2, keepdims=True)
|
||||
scores_log1p = scores / (scores_norm + 1e-9) * jnp.log1p(scores_norm)
|
||||
|
||||
plt.quiver(*xx.T, *scores_log1p.T, width=0.002, color='green')
|
||||
plt.xlim(-1.5, 2.0)
|
||||
plt.ylim(-1.5, 2.0)
|
||||
plt.show()
|
||||
```
|
||||
|
||||
+++ {"colab_type": "text", "id": "Ug91tS-RG6TP"}
|
||||
|
||||
### Plot gradient directions
|
||||
Once the model is trained we can use it to predict scores at each point. Since those are gradient vectors, we'll use [`Quiver Plot`](https://matplotlib.org/3.1.1/api/_as_gen/matplotlib.pyplot.quiver.html) to draw them.
|
||||
|
||||
```{code-cell} ipython3
|
||||
---
|
||||
colab:
|
||||
base_uri: https://localhost:8080/
|
||||
height: 938
|
||||
colab_type: code
|
||||
id: x6SkLg0VG6TQ
|
||||
outputId: 710ab2f4-c3c7-4a3b-f929-e84957fbb233
|
||||
---
|
||||
plt.figure(figsize=[16, 16])
|
||||
|
||||
net_params = get_params(opt_state)
|
||||
xx = jnp.stack(jnp.meshgrid(jnp.linspace(-1.5, 1.5, 50), jnp.linspace(-1.5, 1.5, 50)), axis=-1).reshape(-1, 2)
|
||||
scores = net_apply(net_params, xx)
|
||||
scores_norm = jnp.linalg.norm(scores, axis=-1, ord=2, keepdims=True)
|
||||
scores_log1p = scores / (scores_norm + 1e-9) * jnp.log1p(scores_norm)
|
||||
|
||||
plt.quiver(*xx.T, *scores_log1p.T, width=0.002, color='green')
|
||||
plt.scatter(*sample_batch(10_000).T, alpha=0.25)
|
||||
```
|
||||
|
||||
+++ {"colab_type": "text", "id": "yewoL6wqG6TS"}
|
||||
|
||||
A hot new paper by [Song et al. (2019)](https://arxiv.org/abs/1907.05600) uses this method to generate images by iterative refinement... Apparently it took DL researchers 14 years to understand the proof :)
|
||||
|
||||
Seriously though, this paper takes advantage of two new ideas: sampling with __Langevin Dynamics__ and scaling to high dimensions with __Sliced Score Matching__. We'll cover them one at a time.
|
||||
|
||||
+++ {"colab_type": "text", "id": "gsXvXhgfG6TS"}
|
||||
|
||||
```
|
||||
|
||||
```
|
||||
|
||||
```
|
||||
|
||||
```
|
||||
|
||||
```
|
||||
|
||||
```
|
||||
|
||||
```
|
||||
|
||||
```
|
||||
|
||||
```
|
||||
|
||||
```
|
||||
|
||||
```
|
||||
|
||||
```
|
||||
|
||||
|
||||
|
||||
### Sampling with Langevin Dynamics
|
||||
|
||||
Once we have $\nabla_x log p(x)$, we can use it to generate data. One simple thing you can do is a gradient ascent w.r.t image to find a local maximum of p(x):
|
||||
$$\hat x_{t + 1} := x_t + \epsilon \nabla_{x_t} log p(x_t)$$
|
||||
|
||||
In order to sample $x \sim p(x)$, one can run a slightly more sophisticated procedure:
|
||||
|
||||
$$\hat x_{t+1} := \hat x_t + \frac \epsilon 2 \nabla_{\hat x_t} log p(\hat x_t) + \sqrt \epsilon z_t, \quad z_t \sim N(0, I)$$
|
||||
|
||||
|
||||
Performing this update multiple times in an MCMC fashion is a special case of Langevin Dynamics. Under $\epsilon \rightarrow 0, t \rightarrow \inf$: $\hat x_t$ converges to a sample from $p(x)$. You can find a more detailed explanation and a formal proof in [Welling et al. (2011)](https://www.ics.uci.edu/~welling/publications/papers/stoclangevin_v6.pdf) and further exploration of SGLD in [The et al. (2014)](https://arxiv.org/abs/1409.0578) and [Vollmer et al. (2015)](https://arxiv.org/abs/1501.00438).
|
||||
|
||||
In practice, we can initialize $x_0$ from some initial guess (e.g. uniform distribution over data space) and $\epsilon$ to some positive value. As the sampling progresses, we can anneal $\epsilon$ it until we are satisfied with the samples. Okay, now let's go implement that :)
|
||||
|
||||
```{code-cell} ipython3
|
||||
:colab: {}
|
||||
:colab_type: code
|
||||
:id: Byq9q1XdG6TT
|
||||
|
||||
def sample_langevin(x_initial, *, net_params, key, eps=1e-2, eps_decay=0.9, num_steps=15, temperature=1.0):
|
||||
""" sample x ~ p(x) by applying approximate Langvenin Dynamics, return a sequence of x_t """
|
||||
x_t, x_sequence = x_initial, [x_initial]
|
||||
|
||||
for t in range(num_steps):
|
||||
key, subkey = jax.random.split(key)
|
||||
z_t = jax.random.normal(subkey, shape=x_t.shape)
|
||||
x_t = x_t + eps / 2 * net_apply(net_params, x_t) + jnp.sqrt(eps) * temperature * z_t
|
||||
x_sequence.append(x_t)
|
||||
eps *= eps_decay
|
||||
|
||||
return jnp.stack(x_sequence)
|
||||
```
|
||||
|
||||
```{code-cell} ipython3
|
||||
---
|
||||
colab:
|
||||
base_uri: https://localhost:8080/
|
||||
height: 938
|
||||
colab_type: code
|
||||
id: n6ZWX9Z1G6TV
|
||||
outputId: 4e061bf6-93c5-4d96-cc9b-7e2d8b8899af
|
||||
---
|
||||
plt.figure(figsize=[16, 16])
|
||||
|
||||
key = jax.random.PRNGKey(42)
|
||||
net_params = get_params(opt_state)
|
||||
|
||||
for x_initial in jnp.array([[-1.5, -1.5], [0, 0], [1.5, 0]]):
|
||||
key, subkey = jax.random.split(key)
|
||||
# sample x sequence
|
||||
xx = sample_langevin(x_initial, key=subkey, net_params=net_params)
|
||||
plt.scatter(xx.T[0], xx.T[1], color="blue")
|
||||
|
||||
# draw arrows for each mcmc step
|
||||
deltas = (xx[1:] - xx[:-1])
|
||||
deltas = deltas - deltas / jnp.linalg.norm(deltas, keepdims=True, axis=-1) * 0.04
|
||||
for i, arrow in enumerate(deltas):
|
||||
plt.arrow(xx[i][0], xx[i][1], arrow[0], arrow[1], width=1e-4, head_width=2e-2, color="orange")
|
||||
|
||||
# plot data points and gradients
|
||||
plt.plot()
|
||||
xx = jnp.stack(jnp.meshgrid(jnp.linspace(-1.5, 1.5, 50), jnp.linspace(-1.5, 1.5, 50)), axis=-1).reshape(-1, 2)
|
||||
scores = net_apply(net_params, xx)
|
||||
scores_norm = jnp.linalg.norm(scores, axis=-1, ord=2, keepdims=True)
|
||||
scores_log1p = scores / (scores_norm + 1e-9) * jnp.log1p(scores_norm)
|
||||
plt.quiver(*xx.T, *scores_log1p.T, width=0.002, color='green')
|
||||
plt.scatter(*sample_batch(10_000).T, alpha=0.025)
|
||||
```
|
||||
|
||||
+++ {"colab_type": "text", "id": "vZrW00brG6TX"}
|
||||
|
||||
### Sliced Score Matching
|
||||
|
||||
Now the problem with our previous loss function is that the computation of $tr(\mathbf{J}_x [\space model(x)])$ takes a $O(N^2 + N)$ time to compute, thus not being suitable for high-dimensional problems. The solution is using jacobian vector products which can be easily computed using forward mode auto-differentiation. This method is called Sliced Score Matching and was proposed by [Yang Song et al. (2019)](https://arxiv.org/abs/1905.07088).
|
||||
|
||||
Our new objective looks like this:
|
||||
|
||||
$$E_{\mathbf{v} \sim \mathcal{N}(0, 1)} E_{x \sim p(x)} [ \mathbf{v}^T \mathbf{J}_x[model(x)] \mathbf{v} + \frac{1}{2} (\mathbf{v}^T model(x))^2 ]$$
|
||||
|
||||
Jacobian Vector products, by the way, can be easily computed using `jax.jvp`.
|
||||
|
||||
```{code-cell} ipython3
|
||||
:colab: {}
|
||||
:colab_type: code
|
||||
:id: MkAXz0SmG6TY
|
||||
|
||||
@jax.jit
|
||||
def compute_ssm_loss(net_params, inputs, key):
|
||||
apply = jax.jit(partial(net_apply, net_params))
|
||||
batch_dot = partial(jnp.einsum, 'bu,bu->b')
|
||||
|
||||
# generate random vectors from N(0, I)
|
||||
v = jax.random.normal(key, shape=inputs.shape)
|
||||
|
||||
# predict score and comput jacobian of score times v
|
||||
score, jac_v = jax.jvp(apply, [inputs], [v])
|
||||
|
||||
return jnp.mean(batch_dot(v, jac_v) + 1/2 * batch_dot(v, score) ** 2)
|
||||
|
||||
@jax.jit
|
||||
def train_step(step_i, opt_state, batch, key):
|
||||
# the new compute_loss is random key dependent, thus we need a new train_step function
|
||||
net_params = get_params(opt_state)
|
||||
loss = compute_ssm_loss(net_params, batch, key)
|
||||
grads = jax.grad(compute_ssm_loss, argnums=0)(net_params, batch, key)
|
||||
return loss, opt_update(step_i, grads, opt_state)
|
||||
```
|
||||
|
||||
+++ {"id": "GWaKgphWCwoi", "colab_type": "text"}
|
||||
|
||||
__Note:__ we compute Jacobian with `jax.jacfwd` (forward-mode differentiation) because the input dimension of the network is just 2. You can read more about autograd modes in jax [documentation](https://jax.readthedocs.io/en/latest/jax.html?highlight=jacfwd#jax.jacfwd) and on wikipedia [wiki](https://en.wikipedia.org/wiki/Automatic_differentiation)
|
||||
|
||||
```{code-cell} ipython3
|
||||
:colab: {}
|
||||
:colab_type: code
|
||||
:id: 8dxK2pCxG6Tb
|
||||
|
||||
key = jax.random.PRNGKey(42)
|
||||
key, subkey = jax.random.split(key)
|
||||
out_shape, net_params = net_init(subkey, input_shape=(-1, 2))
|
||||
opt_state = opt_init(net_params)
|
||||
|
||||
loss_history = []
|
||||
```
|
||||
|
||||
```{code-cell} ipython3
|
||||
---
|
||||
colab:
|
||||
base_uri: https://localhost:8080/
|
||||
height: 499
|
||||
colab_type: code
|
||||
id: hQyo8kvTG6Tc
|
||||
outputId: 184f28fc-4c6d-418a-9c28-e248b8633fbe
|
||||
---
|
||||
for i in range(2_000):
|
||||
x = sample_batch(size=128)
|
||||
|
||||
key, subkey = jax.random.split(key)
|
||||
loss, opt_state = train_step(i, opt_state, x, subkey)
|
||||
loss_history.append(loss.item())
|
||||
|
||||
if i % 200 == 0:
|
||||
clear_output(True)
|
||||
plt.figure(figsize=[16, 8])
|
||||
plt.subplot(1, 2, 1)
|
||||
plt.title("mean loss = %.3f" % jnp.mean(jnp.array(loss_history[-32:])))
|
||||
plt.scatter(jnp.arange(len(loss_history)), loss_history)
|
||||
plt.grid()
|
||||
|
||||
plt.subplot(1, 2, 2)
|
||||
net_params = get_params(opt_state)
|
||||
xx = jnp.stack(jnp.meshgrid(jnp.linspace(-1.5, 2.0, 50), jnp.linspace(-1.5, 2.0, 50)), axis=-1).reshape(-1, 2)
|
||||
scores = net_apply(net_params, xx)
|
||||
scores_norm = jnp.linalg.norm(scores, axis=-1, ord=2, keepdims=True)
|
||||
scores_log1p = scores / (scores_norm + 1e-9) * jnp.log1p(scores_norm)
|
||||
|
||||
plt.quiver(*xx.T, *scores_log1p.T, width=0.002, color='green')
|
||||
plt.xlim(-1.5, 2.0)
|
||||
plt.ylim(-1.5, 2.0)
|
||||
plt.show()
|
||||
```
|
||||
|
||||
+++ {"colab_type": "text", "id": "A8Ni7_cGG6Tf"}
|
||||
|
||||
## Easy? Let's go deeper!
|
||||
MNIST 8x8, computing full jacobian would require 64 passes through the network
|
||||
|
||||
```{code-cell} ipython3
|
||||
---
|
||||
colab:
|
||||
base_uri: https://localhost:8080/
|
||||
height: 109
|
||||
colab_type: code
|
||||
id: Y2ZgeMq-G6Tf
|
||||
outputId: 435e69a1-3544-4364-b30c-c066feda7064
|
||||
---
|
||||
from sklearn.datasets import load_digits
|
||||
import numpy as np
|
||||
|
||||
X, _ = load_digits(return_X_y=True)
|
||||
|
||||
for i in range(5):
|
||||
plt.subplot(1, 5, i + 1)
|
||||
plt.imshow(X[i].reshape(8, 8), cmap='gray')
|
||||
|
||||
|
||||
def sample_batch(size, noise=0.1):
|
||||
ix = np.random.randint(0, len(X), size=size)
|
||||
return jnp.array(X[ix] / 16 + noise * np.random.randn(size, 64))
|
||||
```
|
||||
|
||||
```{code-cell} ipython3
|
||||
:colab: {}
|
||||
:colab_type: code
|
||||
:id: rKSjSWQXG6Th
|
||||
|
||||
# Set up network to predict scores
|
||||
net_init, net_apply = stax.serial(
|
||||
stax.Dense(128), stax.Softplus,
|
||||
stax.Dense(128), stax.Softplus,
|
||||
stax.Dense(64),
|
||||
)
|
||||
|
||||
# Create optimizer. Note that both network and optimizer returns pure (stateless) functions
|
||||
opt_init, opt_update, get_params = optimizers.adam(1e-3)
|
||||
|
||||
key = jax.random.PRNGKey(seed=42)
|
||||
key, subkey = jax.random.split(key)
|
||||
out_shape, net_params = net_init(subkey, input_shape=(-1, 64))
|
||||
opt_state = opt_init(net_params)
|
||||
|
||||
loss_history = []
|
||||
```
|
||||
|
||||
```{code-cell} ipython3
|
||||
---
|
||||
colab:
|
||||
base_uri: https://localhost:8080/
|
||||
height: 281
|
||||
colab_type: code
|
||||
id: YxWvSQJAG6Ti
|
||||
outputId: ae47197d-0aa3-496c-83f6-d10328461a00
|
||||
---
|
||||
for i in range(5_000):
|
||||
x = sample_batch(size=128)
|
||||
key, subkey = jax.random.split(key)
|
||||
loss, opt_state = train_step(i, opt_state, x, subkey)
|
||||
loss_history.append(loss.item())
|
||||
|
||||
if i % 500 == 0:
|
||||
clear_output(True)
|
||||
plt.title("mean loss = %.3f" % jnp.mean(jnp.array(loss_history[-32:])))
|
||||
plt.scatter(jnp.arange(len(loss_history)), loss_history)
|
||||
plt.show()
|
||||
```
|
||||
|
||||
```{code-cell} ipython3
|
||||
---
|
||||
colab:
|
||||
base_uri: https://localhost:8080/
|
||||
height: 281
|
||||
colab_type: code
|
||||
id: gof2XcxwG6Tk
|
||||
outputId: 02472a07-4931-4444-d406-344907619a01
|
||||
---
|
||||
key, subkey = jax.random.split(key)
|
||||
x = 0.1 * jax.random.uniform(subkey, shape=(64,))
|
||||
|
||||
xx = sample_langevin(x, net_params=get_params(opt_state), key=key,
|
||||
eps=0.05, eps_decay=0.98, num_steps=50,
|
||||
temperature=0.02) # set low temperature to compensate for noise in training data
|
||||
|
||||
for t, x_t in enumerate(xx):
|
||||
clear_output(True)
|
||||
plt.imshow(x_t.reshape(8, 8), cmap='gray')
|
||||
plt.title('step %i' % t); plt.colorbar(); plt.show()
|
||||
```
|
||||
|
||||
+++ {"colab_type": "text", "id": "jMfcQxhWG6Tm"}
|
||||
|
||||
### This is just the beginning
|
||||
|
||||
In their paper, [Song et al. (2019)](https://arxiv.org/abs/1907.05600) propose a more sophisticated sampling procedure that can efficiently sample larger images. They also utilize a technique called _Denoising Score Matching_ which can be safely ported even to earthling frameworks like tensorflow and pytorch. Go take a look!
|
||||
|
||||
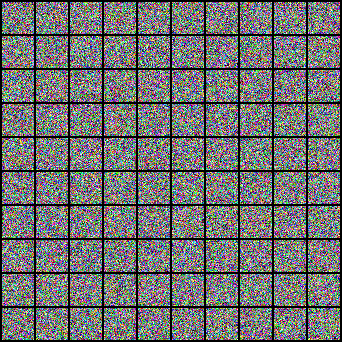
|
||||
|
||||
Notebook author: [Denis Mazur](https://github.com/deniskamazur), edited by [Just Heuristic](https://github.com/justheuristic)
|
File diff suppressed because one or more lines are too long
579
docs/notebooks/thinking_in_jax.md
Normal file
579
docs/notebooks/thinking_in_jax.md
Normal file
@ -0,0 +1,579 @@
|
||||
---
|
||||
jupytext:
|
||||
formats: ipynb,md:myst
|
||||
text_representation:
|
||||
extension: .md
|
||||
format_name: myst
|
||||
format_version: 0.13
|
||||
jupytext_version: 1.10.0
|
||||
kernelspec:
|
||||
display_name: Python 3
|
||||
name: python3
|
||||
---
|
||||
|
||||
```{code-cell}
|
||||
:id: aPUwOm-eCSFD
|
||||
:nbsphinx: hidden
|
||||
|
||||
# Configure ipython to hide long tracebacks.
|
||||
import sys
|
||||
ipython = get_ipython()
|
||||
|
||||
def minimal_traceback(*args, **kwargs):
|
||||
etype, value, tb = sys.exc_info()
|
||||
value.__cause__ = None # suppress chained exceptions
|
||||
stb = ipython.InteractiveTB.structured_traceback(etype, value, tb)
|
||||
del stb[3:-1]
|
||||
return ipython._showtraceback(etype, value, stb)
|
||||
|
||||
ipython.showtraceback = minimal_traceback
|
||||
```
|
||||
|
||||
+++ {"id": "LQHmwePqryRU"}
|
||||
|
||||
# How to Think in JAX
|
||||
|
||||
JAX provides a simple and powerful API for writing accelerated numerical code, but working effectively in JAX sometimes requires extra consideration. This document is meant to help build a ground-up understanding of how JAX operates, so that you can use it more effectively.
|
||||
|
||||
+++ {"id": "nayIExVUtsVD"}
|
||||
|
||||
## JAX vs. NumPy
|
||||
|
||||
**Key Concepts:**
|
||||
|
||||
- JAX provides a NumPy-inspired interface for convenience.
|
||||
- Through duck-typing, JAX arrays can often be used as drop-in replacements of NumPy arrays.
|
||||
- Unlike NumPy arrays, JAX arrays are always immutable.
|
||||
|
||||
NumPy provides a well-known, powerful API for working with numerical data. For convenience, JAX provides `jax.numpy` which closely mirrors the numpy API and provides easy entry into JAX. Almost anything that can be done with `numpy` can be done with `jax.numpy`:
|
||||
|
||||
```{code-cell}
|
||||
---
|
||||
colab:
|
||||
base_uri: https://localhost:8080/
|
||||
height: 265
|
||||
id: kZaOXL7-uvUP
|
||||
outputId: 17a9ee0a-8719-44bb-a9fe-4c9f24649fef
|
||||
---
|
||||
import matplotlib.pyplot as plt
|
||||
import numpy as np
|
||||
|
||||
x_np = np.linspace(0, 10, 1000)
|
||||
y_np = 2 * np.sin(x_np) * np.cos(x_np)
|
||||
plt.plot(x_np, y_np);
|
||||
```
|
||||
|
||||
```{code-cell}
|
||||
---
|
||||
colab:
|
||||
base_uri: https://localhost:8080/
|
||||
height: 282
|
||||
id: 18XbGpRLuZlr
|
||||
outputId: 9e98d928-1925-45b1-d886-37956ca95e7c
|
||||
---
|
||||
import jax.numpy as jnp
|
||||
|
||||
x_jnp = jnp.linspace(0, 10, 1000)
|
||||
y_jnp = 2 * jnp.sin(x_jnp) * jnp.cos(x_jnp)
|
||||
plt.plot(x_jnp, y_jnp);
|
||||
```
|
||||
|
||||
+++ {"id": "kTZcsCJiuPG8"}
|
||||
|
||||
The code blocks are identical aside from replacing `np` with `jnp`, and the results are the same. As we can see, JAX arrays can often be used directly in place of NumPy arrays for things like plotting.
|
||||
|
||||
The arrays themselves are implemented as different Python types:
|
||||
|
||||
```{code-cell}
|
||||
---
|
||||
colab:
|
||||
base_uri: https://localhost:8080/
|
||||
id: PjFFunI7xNe8
|
||||
outputId: e1706c61-2821-437a-efcd-d8082f913c1f
|
||||
---
|
||||
type(x_np)
|
||||
```
|
||||
|
||||
```{code-cell}
|
||||
---
|
||||
colab:
|
||||
base_uri: https://localhost:8080/
|
||||
id: kpv5K7QYxQnX
|
||||
outputId: 8a3f1cb6-c6d6-494c-8efe-24a8217a9d55
|
||||
---
|
||||
type(x_jnp)
|
||||
```
|
||||
|
||||
+++ {"id": "Mx94Ri7euEZm"}
|
||||
|
||||
Python's [duck-typing](https://en.wikipedia.org/wiki/Duck_typing) allows JAX arrays and NumPy arrays to be used interchangeably in many places.
|
||||
|
||||
However, there is one important difference between JAX and NumPy arrays: JAX arrays are immutable, meaning that once created their contents cannot be changed.
|
||||
|
||||
Here is an example of mutating an array in NumPy:
|
||||
|
||||
```{code-cell}
|
||||
---
|
||||
colab:
|
||||
base_uri: https://localhost:8080/
|
||||
id: fzp-y1ZVyGD4
|
||||
outputId: 300a44cc-1ccd-4fb2-f0ee-2179763f7690
|
||||
---
|
||||
# NumPy: mutable arrays
|
||||
x = np.arange(10)
|
||||
x[0] = 10
|
||||
print(x)
|
||||
```
|
||||
|
||||
+++ {"id": "nQ-De0xcJ1lT"}
|
||||
|
||||
The equivalent in JAX results in an error, as JAX arrays are immutable:
|
||||
|
||||
```{code-cell}
|
||||
---
|
||||
colab:
|
||||
base_uri: https://localhost:8080/
|
||||
height: 215
|
||||
id: pCPX0JR-yM4i
|
||||
outputId: 02a442bc-8f23-4dce-9500-81cd28c0b21f
|
||||
tags: [raises-exception]
|
||||
---
|
||||
# JAX: immutable arrays
|
||||
x = jnp.arange(10)
|
||||
x[0] = 10
|
||||
```
|
||||
|
||||
+++ {"id": "yRYF0YgO3F4H"}
|
||||
|
||||
For updating individual elements, JAX provides an [indexed update syntax](https://jax.readthedocs.io/en/latest/jax.ops.html#syntactic-sugar-for-indexed-update-operators) that returns an updated copy:
|
||||
|
||||
```{code-cell}
|
||||
---
|
||||
colab:
|
||||
base_uri: https://localhost:8080/
|
||||
id: 8zqPEAeP3UK5
|
||||
outputId: 7e6c996d-d0b0-4d52-e722-410ba78eb3b1
|
||||
---
|
||||
y = x.at[0].set(10)
|
||||
print(x)
|
||||
print(y)
|
||||
```
|
||||
|
||||
+++ {"id": "886BGDPeyXCu"}
|
||||
|
||||
## NumPy, lax & XLA: JAX API layering
|
||||
|
||||
**Key Concepts:**
|
||||
|
||||
- `jax.numpy` is a high-level wrapper that provides a familiar interface.
|
||||
- `jax.lax` is a lower-level API that is stricter and often more powerful.
|
||||
- All JAX operations are implemented in terms of operations in [XLA](https://www.tensorflow.org/xla/) – the Accelerated Linear Algebra compiler.
|
||||
|
||||
+++ {"id": "BjE4m2sZy4hh"}
|
||||
|
||||
If you look at the source of `jax.numpy`, you'll see that all the operations are eventually expressed in terms of functions defined in `jax.lax`. You can think of `jax.lax` as a stricter, but often more powerful, API for working with multi-dimensional arrays.
|
||||
|
||||
For example, while `jax.numpy` will implicitly promote arguments to allow operations between mixed data types, `jax.lax` will not:
|
||||
|
||||
```{code-cell}
|
||||
---
|
||||
colab:
|
||||
base_uri: https://localhost:8080/
|
||||
id: c6EFPcj12mw0
|
||||
outputId: 730e2ca4-30a5-45bc-923c-c3a5143496e2
|
||||
---
|
||||
import jax.numpy as jnp
|
||||
jnp.add(1, 1.0) # jax.numpy API implicitly promotes mixed types.
|
||||
```
|
||||
|
||||
```{code-cell}
|
||||
---
|
||||
colab:
|
||||
base_uri: https://localhost:8080/
|
||||
height: 181
|
||||
id: 0VkqlcXL2qSp
|
||||
outputId: 601b0562-3e6a-402d-f83b-3afdd1e7e7c4
|
||||
tags: [raises-exception]
|
||||
---
|
||||
from jax import lax
|
||||
lax.add(1, 1.0) # jax.lax API requires explicit type promotion.
|
||||
```
|
||||
|
||||
+++ {"id": "aC9TkXaTEu7A"}
|
||||
|
||||
If using `jax.lax` directly, you'll have to do type promotion explicitly in such cases:
|
||||
|
||||
```{code-cell}
|
||||
---
|
||||
colab:
|
||||
base_uri: https://localhost:8080/
|
||||
id: 3PNQlieT81mi
|
||||
outputId: cb3ed074-f410-456f-c086-23107eae2634
|
||||
---
|
||||
lax.add(jnp.float32(1), 1.0)
|
||||
```
|
||||
|
||||
+++ {"id": "M3HDuM4x2eTL"}
|
||||
|
||||
Along with this strictness, `jax.lax` also provides efficient APIs for some more general operations than are supported by NumPy.
|
||||
|
||||
For example, consider a 1D convolution, which can be expressed in NumPy this way:
|
||||
|
||||
```{code-cell}
|
||||
---
|
||||
colab:
|
||||
base_uri: https://localhost:8080/
|
||||
id: Bv-7XexyzVCN
|
||||
outputId: f5d38cd8-e7fc-49e2-bff3-a0eee306cb54
|
||||
---
|
||||
x = jnp.array([1, 2, 1])
|
||||
y = jnp.ones(10)
|
||||
jnp.convolve(x, y)
|
||||
```
|
||||
|
||||
+++ {"id": "0GPqgT7S0q8r"}
|
||||
|
||||
Under the hood, this NumPy operation is translated to a much more general convolution implemented by [`lax.conv_general_dilated`](https://jax.readthedocs.io/en/latest/_autosummary/jax.lax.conv_general_dilated.html):
|
||||
|
||||
```{code-cell}
|
||||
---
|
||||
colab:
|
||||
base_uri: https://localhost:8080/
|
||||
id: pi4f6ikjzc3l
|
||||
outputId: b9b37edc-b911-4010-aaf8-ee8f500111d7
|
||||
---
|
||||
from jax import lax
|
||||
result = lax.conv_general_dilated(
|
||||
x.reshape(1, 1, 3).astype(float), # note: explicit promotion
|
||||
y.reshape(1, 1, 10),
|
||||
window_strides=(1,),
|
||||
padding=[(len(y) - 1, len(y) - 1)]) # equivalent of padding='full' in NumPy
|
||||
result[0, 0]
|
||||
```
|
||||
|
||||
+++ {"id": "7mdo6ycczlbd"}
|
||||
|
||||
This is a batched convolution operation designed to be efficient for the types of convolutions often used in deep neural nets. It requires much more boilerplate, but is far more flexible and scalable than the convolution provided by NumPy (See [JAX Sharp Bits: Convolutions](https://jax.readthedocs.io/en/latest/notebooks/Common_Gotchas_in_JAX.html#%F0%9F%94%AA-Convolutions) for more detail on JAX convolutions).
|
||||
|
||||
At their heart, all `jax.lax` operations are Python wrappers for operations in XLA; here, for example, the convolution implementation is provided by [XLA:ConvWithGeneralPadding](https://www.tensorflow.org/xla/operation_semantics#convwithgeneralpadding_convolution).
|
||||
Every JAX operation is eventually expressed in terms of these fundamental XLA operations, which is what enables just-in-time (JIT) compilation.
|
||||
|
||||
+++ {"id": "NJfWa2PktD5_"}
|
||||
|
||||
## To JIT or not to JIT
|
||||
|
||||
**Key Concepts:**
|
||||
|
||||
- By default JAX executes operations one at a time, in sequence.
|
||||
- Using a just-in-time (JIT) compilation decorator, sequences of operations can be optimized together and run at once.
|
||||
- Not all JAX code can be JIT compiled, as it requires array shapes to be static & known at compile time.
|
||||
|
||||
The fact that all JAX operations are expressed in terms of XLA allows JAX to use the XLA compiler to execute blocks of code very efficiently.
|
||||
|
||||
For example, consider this function that normalizes the rows of a 2D matrix, expressed in terms of `jax.numpy` operations:
|
||||
|
||||
```{code-cell}
|
||||
:id: SQj_UKGc-7kQ
|
||||
|
||||
import jax.numpy as jnp
|
||||
|
||||
def norm(X):
|
||||
X = X - X.mean(0)
|
||||
return X / X.std(0)
|
||||
```
|
||||
|
||||
+++ {"id": "0yVo_OKSAolW"}
|
||||
|
||||
A just-in-time compiled version of the function can be created using the `jax.jit` transform:
|
||||
|
||||
```{code-cell}
|
||||
:id: oHLwGmhZAnCY
|
||||
|
||||
from jax import jit
|
||||
norm_compiled = jit(norm)
|
||||
```
|
||||
|
||||
+++ {"id": "Q3H9ig5GA2Ms"}
|
||||
|
||||
This function returns the same results as the original, up to standard floating-point accuracy:
|
||||
|
||||
```{code-cell}
|
||||
---
|
||||
colab:
|
||||
base_uri: https://localhost:8080/
|
||||
id: oz7zzyS3AwMc
|
||||
outputId: 914f9242-82c4-4365-abb2-77843a704e03
|
||||
---
|
||||
np.random.seed(1701)
|
||||
X = jnp.array(np.random.rand(10000, 10))
|
||||
np.allclose(norm(X), norm_compiled(X), atol=1E-6)
|
||||
```
|
||||
|
||||
+++ {"id": "3GvisB-CA9M8"}
|
||||
|
||||
But due to the compilation (which includes fusing of operations, avoidance of allocating temporary arrays, and a host of other tricks), execution times can be orders of magnitude faster in the JIT-compiled case (note the use of `block_until_ready()` to account for JAX's [asynchronous dispatch](https://jax.readthedocs.io/en/latest/async_dispatch.html)):
|
||||
|
||||
```{code-cell}
|
||||
---
|
||||
colab:
|
||||
base_uri: https://localhost:8080/
|
||||
id: 6mUB6VdDAEIY
|
||||
outputId: 5d7e1bbd-4064-4fe3-f3d9-5435b5283199
|
||||
---
|
||||
%timeit norm(X).block_until_ready()
|
||||
%timeit norm_compiled(X).block_until_ready()
|
||||
```
|
||||
|
||||
+++ {"id": "B1eGBGn0tMba"}
|
||||
|
||||
That said, `jax.jit` does have limitations: in particular, it requires all arrays to have static shapes. That means that some JAX operations are incompatible with JIT compilation.
|
||||
|
||||
For example, this operation can be executed in op-by-op mode:
|
||||
|
||||
```{code-cell}
|
||||
---
|
||||
colab:
|
||||
base_uri: https://localhost:8080/
|
||||
id: YfZd9mW7CSKM
|
||||
outputId: 899fedcc-0857-4381-8f57-bb653e0aa2f1
|
||||
---
|
||||
def get_negatives(x):
|
||||
return x[x < 0]
|
||||
|
||||
x = jnp.array(np.random.randn(10))
|
||||
get_negatives(x)
|
||||
```
|
||||
|
||||
+++ {"id": "g6niKxoQC2mZ"}
|
||||
|
||||
But it returns an error if you attempt to execute it in jit mode:
|
||||
|
||||
```{code-cell}
|
||||
---
|
||||
colab:
|
||||
base_uri: https://localhost:8080/
|
||||
height: 164
|
||||
id: yYWvE4rxCjPK
|
||||
outputId: 765b46d3-49cd-41b7-9815-e8bb7cd80175
|
||||
tags: [raises-exception]
|
||||
---
|
||||
jit(get_negatives)(x)
|
||||
```
|
||||
|
||||
+++ {"id": "vFL6DNpECfVz"}
|
||||
|
||||
This is because the function generates an array whose shape is not known at compile time: the size of the output depends on the values of the input array, and so it is not compatible with JIT.
|
||||
|
||||
+++ {"id": "BzBnKbXwXjLV"}
|
||||
|
||||
## JIT mechanics: tracing and static variables
|
||||
|
||||
**Key Concepts:**
|
||||
|
||||
- JIT and other JAX transforms work by *tracing* a function to determine its effect on inputs of a specific shape and type.
|
||||
|
||||
- Variables that you don't want to be traced can be marked as *static*
|
||||
|
||||
To use `jax.jit` effectively, it is useful to understand how it works. Let's put a few `print()` statements within a JIT-compiled function and then call the function:
|
||||
|
||||
```{code-cell}
|
||||
---
|
||||
colab:
|
||||
base_uri: https://localhost:8080/
|
||||
id: TfjVIVuD4gnc
|
||||
outputId: df6ad898-b047-4ad1-eb18-2fbcb3fd2ab3
|
||||
---
|
||||
@jit
|
||||
def f(x, y):
|
||||
print("Running f():")
|
||||
print(f" x = {x}")
|
||||
print(f" y = {y}")
|
||||
result = jnp.dot(x + 1, y + 1)
|
||||
print(f" result = {result}")
|
||||
return result
|
||||
|
||||
x = np.random.randn(3, 4)
|
||||
y = np.random.randn(4)
|
||||
f(x, y)
|
||||
```
|
||||
|
||||
+++ {"id": "Ts1fP45A40QV"}
|
||||
|
||||
Notice that the print statements execute, but rather than printing the data we passed to the function, though, it prints *tracer* objects that stand-in for them.
|
||||
|
||||
These tracer objects are what `jax.jit` uses to extract the sequence of operations specified by the function. Basic tracers are stand-ins that encode the **shape** and **dtype** of the arrays, but are agnostic to the values. This recorded sequence of computations can then be efficiently applied within XLA to new inputs with the same shape and dtype, without having to re-execute the Python code.
|
||||
|
||||
When we call the compiled fuction again on matching inputs, no re-compilation is required and nothing is printed because the result is computed in compiled XLA rather than in Python:
|
||||
|
||||
```{code-cell}
|
||||
---
|
||||
colab:
|
||||
base_uri: https://localhost:8080/
|
||||
id: xGntvzNH7skE
|
||||
outputId: 66694b8b-181f-4635-a8e2-1fc7f244d94b
|
||||
---
|
||||
x2 = np.random.randn(3, 4)
|
||||
y2 = np.random.randn(4)
|
||||
f(x2, y2)
|
||||
```
|
||||
|
||||
+++ {"id": "9EB9WkRX7fm0"}
|
||||
|
||||
The extracted sequence of operations is encoded in a JAX expression, or *jaxpr* for short. You can view the jaxpr using the `jax.make_jaxpr` transformation:
|
||||
|
||||
```{code-cell}
|
||||
---
|
||||
colab:
|
||||
base_uri: https://localhost:8080/
|
||||
id: 89TMp_Op5-JZ
|
||||
outputId: 151210e2-af6f-4950-ac1e-9fdb81d4aae1
|
||||
---
|
||||
from jax import make_jaxpr
|
||||
|
||||
def f(x, y):
|
||||
return jnp.dot(x + 1, y + 1)
|
||||
|
||||
make_jaxpr(f)(x, y)
|
||||
```
|
||||
|
||||
+++ {"id": "0Oq9S4MZ90TL"}
|
||||
|
||||
Note one consequence of this: because JIT compilation is done *without* information on the content of the array, control flow statements in the function cannot depend on traced values. For example, this fails:
|
||||
|
||||
```{code-cell}
|
||||
---
|
||||
colab:
|
||||
base_uri: https://localhost:8080/
|
||||
height: 419
|
||||
id: A0rFdM95-Ix_
|
||||
outputId: d7ffa367-b241-488e-df96-ad0576536605
|
||||
tags: [raises-exception]
|
||||
---
|
||||
@jit
|
||||
def f(x, neg):
|
||||
return -x if neg else x
|
||||
|
||||
f(1, True)
|
||||
```
|
||||
|
||||
+++ {"id": "DkTO9m8j-TYI"}
|
||||
|
||||
If there are variables that you would not like to be traced, they can be marked as static for the purposes of JIT compilation:
|
||||
|
||||
```{code-cell}
|
||||
---
|
||||
colab:
|
||||
base_uri: https://localhost:8080/
|
||||
id: K1C7ZnVv-lbv
|
||||
outputId: cdbdf152-30fd-4ecb-c9ec-1d1124f337f7
|
||||
---
|
||||
from functools import partial
|
||||
|
||||
@partial(jit, static_argnums=(1,))
|
||||
def f(x, neg):
|
||||
return -x if neg else x
|
||||
|
||||
f(1, True)
|
||||
```
|
||||
|
||||
+++ {"id": "dD7p4LRsGzhx"}
|
||||
|
||||
Note that calling a JIT-compiled function with a different static argument results in re-compilation, so the function still works as expected:
|
||||
|
||||
```{code-cell}
|
||||
---
|
||||
colab:
|
||||
base_uri: https://localhost:8080/
|
||||
id: sXqczBOrG7-w
|
||||
outputId: 3a3f50e6-d1fc-42bb-d6df-eb3d206e4b67
|
||||
---
|
||||
f(1, False)
|
||||
```
|
||||
|
||||
+++ {"id": "ZESlrDngGVb1"}
|
||||
|
||||
Understanding which values and operations will be static and which will be traced is a key part of using `jax.jit` effectively.
|
||||
|
||||
+++ {"id": "r-RCl_wD5lI7"}
|
||||
|
||||
## Static vs Traced Operations
|
||||
|
||||
**Key Concepts:**
|
||||
|
||||
- Just as values can be either static or traced, operations can be static or traced.
|
||||
|
||||
- Static operations are evaluated at compile-time in Python; traced operations are compiled & evaluated at run-time in XLA.
|
||||
|
||||
- Use `numpy` for operations that you want to be static; use `jax.numpy` for operations that you want to be traced.
|
||||
|
||||
This distinction between static and traced values makes it important to think about how to keep a static value static. Consider this function:
|
||||
|
||||
```{code-cell}
|
||||
---
|
||||
colab:
|
||||
base_uri: https://localhost:8080/
|
||||
height: 385
|
||||
id: XJCQ7slcD4iU
|
||||
outputId: a89a5614-7359-4dc7-c165-03e7d0fc6610
|
||||
tags: [raises-exception]
|
||||
---
|
||||
import jax.numpy as jnp
|
||||
from jax import jit
|
||||
|
||||
@jit
|
||||
def f(x):
|
||||
return x.reshape(jnp.array(x.shape).prod())
|
||||
|
||||
x = jnp.ones((2, 3))
|
||||
f(x)
|
||||
```
|
||||
|
||||
+++ {"id": "ZO3GMGrHBZDS"}
|
||||
|
||||
This fails with an error specifying that a tracer was found in `jax.numpy.reshape`. Let's add some print statements to the function to understand why this is happening:
|
||||
|
||||
```{code-cell}
|
||||
---
|
||||
colab:
|
||||
base_uri: https://localhost:8080/
|
||||
id: Cb4mbeVZEi_q
|
||||
outputId: f72c1ce3-950c-400f-bfea-10c0d0118911
|
||||
---
|
||||
@jit
|
||||
def f(x):
|
||||
print(f"x = {x}")
|
||||
print(f"x.shape = {x.shape}")
|
||||
print(f"jnp.array(x.shape).prod() = {jnp.array(x.shape).prod()}")
|
||||
# comment this out to avoid the error:
|
||||
# return x.reshape(jnp.array(x.shape).prot())
|
||||
|
||||
f(x)
|
||||
```
|
||||
|
||||
+++ {"id": "viSQPc3jEwJr"}
|
||||
|
||||
Notice that although `x` is traced, `x.shape` is a static value. However, when we use `jnp.array` and `jnp.prod` on this static value, it becomes a traced value, at which point it cannot be used in a function like `reshape()` that requires a static input (recall: array shapes must be static).
|
||||
|
||||
A useful pattern is to use `numpy` for operations that should be static (i.e. done at compile-time), and use `jax.numpy` for operations that should be traced (i.e. compiled and executed at run-time). For this function, it might look like this:
|
||||
|
||||
```{code-cell}
|
||||
---
|
||||
colab:
|
||||
base_uri: https://localhost:8080/
|
||||
id: GiovOOPcGJhg
|
||||
outputId: 399ee059-1807-4866-9beb-1c5131e38e15
|
||||
---
|
||||
from jax import jit
|
||||
import jax.numpy as jnp
|
||||
import numpy as np
|
||||
|
||||
@jit
|
||||
def f(x):
|
||||
return x.reshape((np.prod(x.shape),))
|
||||
|
||||
f(x)
|
||||
```
|
||||
|
||||
+++ {"id": "C-QZ5d1DG-dv"}
|
||||
|
||||
For this reason, a standard convention in JAX programs is to `import numpy as np` and `import jax.numpy as jnp` so that both interfaces are available for finer control over whether operations are performed in a static matter (with `numpy`, once at compile-time) or a traced manner (with `jax.numpy`, optimized at run-time).
|
File diff suppressed because one or more lines are too long
303
docs/notebooks/vmapped_log_probs.md
Normal file
303
docs/notebooks/vmapped_log_probs.md
Normal file
@ -0,0 +1,303 @@
|
||||
---
|
||||
jupytext:
|
||||
formats: ipynb,md:myst
|
||||
text_representation:
|
||||
extension: .md
|
||||
format_name: myst
|
||||
format_version: 0.13
|
||||
jupytext_version: 1.10.0
|
||||
kernelspec:
|
||||
display_name: Python 3
|
||||
language: python
|
||||
name: python3
|
||||
---
|
||||
|
||||
+++ {"colab_type": "text", "id": "6umP1IKf4Dg6"}
|
||||
|
||||
# Autobatching log-densities example
|
||||
|
||||
This notebook demonstrates a simple Bayesian inference example where autobatching makes user code easier to write, easier to read, and less likely to include bugs.
|
||||
|
||||
Inspired by a notebook by @davmre.
|
||||
|
||||
```{code-cell} ipython3
|
||||
:colab: {}
|
||||
:colab_type: code
|
||||
:id: 8RZDkfbV3zdR
|
||||
|
||||
import functools
|
||||
import itertools
|
||||
import re
|
||||
import sys
|
||||
import time
|
||||
|
||||
from matplotlib.pyplot import *
|
||||
|
||||
import jax
|
||||
|
||||
from jax import lax
|
||||
import jax.numpy as jnp
|
||||
import jax.scipy as jsp
|
||||
from jax import random
|
||||
|
||||
import numpy as np
|
||||
import scipy as sp
|
||||
```
|
||||
|
||||
+++ {"colab_type": "text", "id": "p2VcZS1d34C6"}
|
||||
|
||||
## Generate a fake binary classification dataset
|
||||
|
||||
```{code-cell} ipython3
|
||||
:colab: {}
|
||||
:colab_type: code
|
||||
:id: pq41hMvn4c_i
|
||||
|
||||
np.random.seed(10009)
|
||||
|
||||
num_features = 10
|
||||
num_points = 100
|
||||
|
||||
true_beta = np.random.randn(num_features).astype(jnp.float32)
|
||||
all_x = np.random.randn(num_points, num_features).astype(jnp.float32)
|
||||
y = (np.random.rand(num_points) < sp.special.expit(all_x.dot(true_beta))).astype(jnp.int32)
|
||||
```
|
||||
|
||||
```{code-cell} ipython3
|
||||
---
|
||||
colab:
|
||||
base_uri: https://localhost:8080/
|
||||
height: 102
|
||||
colab_type: code
|
||||
id: O0nVumAw7IlT
|
||||
outputId: 751a3290-a81b-4538-9183-16cd685fbaf9
|
||||
---
|
||||
y
|
||||
```
|
||||
|
||||
+++ {"colab_type": "text", "id": "DZRVvhpn5aB1"}
|
||||
|
||||
## Write the log-joint function for the model
|
||||
|
||||
We'll write a non-batched version, a manually batched version, and an autobatched version.
|
||||
|
||||
+++ {"colab_type": "text", "id": "C_mDXInL7nsP"}
|
||||
|
||||
### Non-batched
|
||||
|
||||
```{code-cell} ipython3
|
||||
:colab: {}
|
||||
:colab_type: code
|
||||
:id: ZHyL2sJh5ajG
|
||||
|
||||
def log_joint(beta):
|
||||
result = 0.
|
||||
# Note that no `axis` parameter is provided to `jnp.sum`.
|
||||
result = result + jnp.sum(jsp.stats.norm.logpdf(beta, loc=0., scale=1.))
|
||||
result = result + jnp.sum(-jnp.log(1 + jnp.exp(-(2*y-1) * jnp.dot(all_x, beta))))
|
||||
return result
|
||||
```
|
||||
|
||||
```{code-cell} ipython3
|
||||
---
|
||||
colab:
|
||||
base_uri: https://localhost:8080/
|
||||
height: 34
|
||||
colab_type: code
|
||||
id: e51qW0ro6J7C
|
||||
outputId: 2ec6bbbd-12ee-45bc-af76-5111c53e4d5a
|
||||
---
|
||||
log_joint(np.random.randn(num_features))
|
||||
```
|
||||
|
||||
```{code-cell} ipython3
|
||||
---
|
||||
colab:
|
||||
base_uri: https://localhost:8080/
|
||||
height: 34
|
||||
colab_type: code
|
||||
id: fglQXK1Y6wnm
|
||||
outputId: 2b934336-08ad-4776-9a58-aa575bf601eb
|
||||
---
|
||||
# This doesn't work, because we didn't write `log_prob()` to handle batching.
|
||||
try:
|
||||
batch_size = 10
|
||||
batched_test_beta = np.random.randn(batch_size, num_features)
|
||||
|
||||
log_joint(np.random.randn(batch_size, num_features))
|
||||
except ValueError as e:
|
||||
print("Caught expected exception " + str(e))
|
||||
```
|
||||
|
||||
+++ {"colab_type": "text", "id": "_lQ8MnKq7sLU"}
|
||||
|
||||
### Manually batched
|
||||
|
||||
```{code-cell} ipython3
|
||||
:colab: {}
|
||||
:colab_type: code
|
||||
:id: 2g5-4bQE7gRA
|
||||
|
||||
def batched_log_joint(beta):
|
||||
result = 0.
|
||||
# Here (and below) `sum` needs an `axis` parameter. At best, forgetting to set axis
|
||||
# or setting it incorrectly yields an error; at worst, it silently changes the
|
||||
# semantics of the model.
|
||||
result = result + jnp.sum(jsp.stats.norm.logpdf(beta, loc=0., scale=1.),
|
||||
axis=-1)
|
||||
# Note the multiple transposes. Getting this right is not rocket science,
|
||||
# but it's also not totally mindless. (I didn't get it right on the first
|
||||
# try.)
|
||||
result = result + jnp.sum(-jnp.log(1 + jnp.exp(-(2*y-1) * jnp.dot(all_x, beta.T).T)),
|
||||
axis=-1)
|
||||
return result
|
||||
```
|
||||
|
||||
```{code-cell} ipython3
|
||||
---
|
||||
colab:
|
||||
base_uri: https://localhost:8080/
|
||||
height: 68
|
||||
colab_type: code
|
||||
id: KdDMr-Gy85CO
|
||||
outputId: db746654-68e9-43b8-ce3b-6e5682e22eb5
|
||||
---
|
||||
batch_size = 10
|
||||
batched_test_beta = np.random.randn(batch_size, num_features)
|
||||
|
||||
batched_log_joint(batched_test_beta)
|
||||
```
|
||||
|
||||
+++ {"colab_type": "text", "id": "-uuGlHQ_85kd"}
|
||||
|
||||
### Autobatched with vmap
|
||||
|
||||
It just works.
|
||||
|
||||
```{code-cell} ipython3
|
||||
---
|
||||
colab:
|
||||
base_uri: https://localhost:8080/
|
||||
height: 68
|
||||
colab_type: code
|
||||
id: SU20bouH8-Za
|
||||
outputId: ee450298-982f-4b9a-bed9-a6f9b8f63d92
|
||||
---
|
||||
vmap_batched_log_joint = jax.vmap(log_joint)
|
||||
vmap_batched_log_joint(batched_test_beta)
|
||||
```
|
||||
|
||||
+++ {"colab_type": "text", "id": "L1KNBo9y_yZJ"}
|
||||
|
||||
## Self-contained variational inference example
|
||||
|
||||
A little code is copied from above.
|
||||
|
||||
+++ {"colab_type": "text", "id": "lQTPaaQMJh8Y"}
|
||||
|
||||
### Set up the (batched) log-joint function
|
||||
|
||||
```{code-cell} ipython3
|
||||
:colab: {}
|
||||
:colab_type: code
|
||||
:id: AITXbaofA3Pm
|
||||
|
||||
@jax.jit
|
||||
def log_joint(beta):
|
||||
result = 0.
|
||||
# Note that no `axis` parameter is provided to `jnp.sum`.
|
||||
result = result + jnp.sum(jsp.stats.norm.logpdf(beta, loc=0., scale=10.))
|
||||
result = result + jnp.sum(-jnp.log(1 + jnp.exp(-(2*y-1) * jnp.dot(all_x, beta))))
|
||||
return result
|
||||
|
||||
batched_log_joint = jax.jit(jax.vmap(log_joint))
|
||||
```
|
||||
|
||||
+++ {"colab_type": "text", "id": "UmmFMQ8LJk6a"}
|
||||
|
||||
### Define the ELBO and its gradient
|
||||
|
||||
```{code-cell} ipython3
|
||||
:colab: {}
|
||||
:colab_type: code
|
||||
:id: MJtnskL6BKwV
|
||||
|
||||
def elbo(beta_loc, beta_log_scale, epsilon):
|
||||
beta_sample = beta_loc + jnp.exp(beta_log_scale) * epsilon
|
||||
return jnp.mean(batched_log_joint(beta_sample), 0) + jnp.sum(beta_log_scale - 0.5 * np.log(2*np.pi))
|
||||
|
||||
elbo = jax.jit(elbo)
|
||||
elbo_val_and_grad = jax.jit(jax.value_and_grad(elbo, argnums=(0, 1)))
|
||||
```
|
||||
|
||||
+++ {"colab_type": "text", "id": "oQC7xKYnJrp5"}
|
||||
|
||||
### Optimize the ELBO using SGD
|
||||
|
||||
```{code-cell} ipython3
|
||||
---
|
||||
colab:
|
||||
base_uri: https://localhost:8080/
|
||||
height: 1000
|
||||
colab_type: code
|
||||
id: 9JrD5nNgH715
|
||||
outputId: 80bf62d8-821a-45c4-885c-528b2e449e97
|
||||
---
|
||||
def normal_sample(key, shape):
|
||||
"""Convenience function for quasi-stateful RNG."""
|
||||
new_key, sub_key = random.split(key)
|
||||
return new_key, random.normal(sub_key, shape)
|
||||
|
||||
normal_sample = jax.jit(normal_sample, static_argnums=(1,))
|
||||
|
||||
key = random.PRNGKey(10003)
|
||||
|
||||
beta_loc = jnp.zeros(num_features, jnp.float32)
|
||||
beta_log_scale = jnp.zeros(num_features, jnp.float32)
|
||||
|
||||
step_size = 0.01
|
||||
batch_size = 128
|
||||
epsilon_shape = (batch_size, num_features)
|
||||
for i in range(1000):
|
||||
key, epsilon = normal_sample(key, epsilon_shape)
|
||||
elbo_val, (beta_loc_grad, beta_log_scale_grad) = elbo_val_and_grad(
|
||||
beta_loc, beta_log_scale, epsilon)
|
||||
beta_loc += step_size * beta_loc_grad
|
||||
beta_log_scale += step_size * beta_log_scale_grad
|
||||
if i % 10 == 0:
|
||||
print('{}\t{}'.format(i, elbo_val))
|
||||
```
|
||||
|
||||
+++ {"colab_type": "text", "id": "b3ZAe5fJJ2KM"}
|
||||
|
||||
### Display the results
|
||||
|
||||
Coverage isn't quite as good as we might like, but it's not bad, and nobody said variational inference was exact.
|
||||
|
||||
```{code-cell} ipython3
|
||||
---
|
||||
colab:
|
||||
base_uri: https://localhost:8080/
|
||||
height: 463
|
||||
colab_type: code
|
||||
id: zt1NBLoVHtOG
|
||||
outputId: fb159795-e6e7-497c-e501-9933ec761af4
|
||||
---
|
||||
figure(figsize=(7, 7))
|
||||
plot(true_beta, beta_loc, '.', label='Approximated Posterior Means')
|
||||
plot(true_beta, beta_loc + 2*jnp.exp(beta_log_scale), 'r.', label='Approximated Posterior $2\sigma$ Error Bars')
|
||||
plot(true_beta, beta_loc - 2*jnp.exp(beta_log_scale), 'r.')
|
||||
plot_scale = 3
|
||||
plot([-plot_scale, plot_scale], [-plot_scale, plot_scale], 'k')
|
||||
xlabel('True beta')
|
||||
ylabel('Estimated beta')
|
||||
legend(loc='best')
|
||||
```
|
||||
|
||||
```{code-cell} ipython3
|
||||
:colab: {}
|
||||
:colab_type: code
|
||||
:id: _bXdOlvUEJl0
|
||||
|
||||
|
||||
```
|
Loading…
x
Reference in New Issue
Block a user