mirror of
https://github.com/ROCm/jax.git
synced 2025-04-16 03:46:06 +00:00
Removed go/jax link and updated wording
This commit is contained in:
parent
948a8db0ad
commit
838d9e12fe
29
README.md
29
README.md
@ -2,27 +2,26 @@
|
||||
|
||||
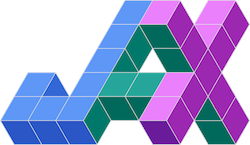
|
||||
|
||||
[JAX](http://go/jax) is [Autograd](https://github.com/hips/autograd) and
|
||||
JAX is [Autograd](https://github.com/hips/autograd) and
|
||||
[XLA](https://github.com/tensorflow/tensorflow/blob/master/tensorflow/compiler/xla/g3doc/overview.md),
|
||||
brought together for high-performance machine learning research.
|
||||
|
||||
With its updated version of [Autograd](https://github.com/hips/autograd), JAX
|
||||
can automatically differentiate native Python and NumPy code. It can
|
||||
differentiate through a large subset of Python’s features, including loops,
|
||||
ifs, recursion, and closures, and it can even take derivatives of derivatives
|
||||
of derivatives. It supports reverse-mode differentiation (a.k.a.
|
||||
backpropagation) as well as forward-mode differentiation, and the two can be
|
||||
composed arbitrarily to any order.
|
||||
With its updated version of Autograd, JAX can automatically differentiate native
|
||||
Python and NumPy functions. It can differentiate through loops, branches,
|
||||
recursion, and closures, and it can take derivatives of derivatives of
|
||||
derivatives. It supports reverse-mode differentiation (a.k.a. backpropagation)
|
||||
as well as forward-mode differentiation, and the two can be composed arbitrarily
|
||||
to any order.
|
||||
|
||||
What’s new is that JAX uses
|
||||
[XLA](https://github.com/tensorflow/tensorflow/blob/master/tensorflow/compiler/xla/g3doc/overview.md)
|
||||
to compile and run your NumPy code on accelerators, like GPUs and TPUs.
|
||||
Compilation happens under the hood by default, with library calls getting
|
||||
just-in-time compiled and executed. But JAX even lets you just-in-time compile
|
||||
your own Python functions into XLA-optimized kernels using a one-function API.
|
||||
Compilation and automatic differentiation can be composed arbitrarily, so you
|
||||
can express sophisticated algorithms and get maximal performance without having
|
||||
to leave Python.
|
||||
to compile and run your NumPy functions on GPUs and TPUs. Compilation happens
|
||||
under the hood by default, with library calls getting just-in-time compiled and
|
||||
executed. But JAX also lets you just-in-time compile your own Python functions
|
||||
into XLA-optimized kernels using a one-function API,
|
||||
[`jit`](#compilation-with-jit). Compilation and automatic differentiation can be
|
||||
composed arbitrarily, so you can express sophisticated algorithms and get
|
||||
maximal performance without leaving Python.
|
||||
|
||||
This is a research project, not an official Google product. Expect bugs and
|
||||
sharp edges. Please help by trying it out, [reporting
|
||||
|
Loading…
x
Reference in New Issue
Block a user