mirror of
https://github.com/ROCm/jax.git
synced 2025-04-16 03:46:06 +00:00
Replace references to jax.readthedocs.io with docs.jax.dev.
PiperOrigin-RevId: 745156931
This commit is contained in:
parent
2c17538838
commit
c4340d966e
68
CHANGELOG.md
68
CHANGELOG.md
@ -1,6 +1,6 @@
|
||||
# Change log
|
||||
|
||||
Best viewed [here](https://jax.readthedocs.io/en/latest/changelog.html).
|
||||
Best viewed [here](https://docs.jax.dev/en/latest/changelog.html).
|
||||
For the changes specific to the experimental Pallas APIs,
|
||||
see {ref}`pallas-changelog`.
|
||||
|
||||
@ -126,7 +126,7 @@ Patch release of 0.5.1
|
||||
## jax 0.5.0 (Jan 17, 2025)
|
||||
|
||||
As of this release, JAX now uses
|
||||
[effort-based versioning](https://jax.readthedocs.io/en/latest/jep/25516-effver.html).
|
||||
[effort-based versioning](https://docs.jax.dev/en/latest/jep/25516-effver.html).
|
||||
Since this release makes a breaking change to PRNG key semantics that
|
||||
may require users to update their code, we are bumping the "meso" version of JAX
|
||||
to signify this.
|
||||
@ -217,7 +217,7 @@ to signify this.
|
||||
* New Features
|
||||
* {func}`jax.export.export` can be used for device-polymorphic export with
|
||||
shardings constructed with {func}`jax.sharding.AbstractMesh`.
|
||||
See the [jax.export documentation](https://jax.readthedocs.io/en/latest/export/export.html#device-polymorphic-export).
|
||||
See the [jax.export documentation](https://docs.jax.dev/en/latest/export/export.html#device-polymorphic-export).
|
||||
* Added {func}`jax.lax.split`. This is a primitive version of
|
||||
{func}`jax.numpy.split`, added because it yields a more compact
|
||||
transpose during automatic differentiation.
|
||||
@ -259,7 +259,7 @@ This is a patch release of jax 0.4.36. Only "jax" was released at this version.
|
||||
after being deprecated in JAX v0.4.31. Instead use `xb = jax.lib.xla_bridge`,
|
||||
`xc = jax.lib.xla_client`, and `xe = jax.lib.xla_extension`.
|
||||
* The deprecated module `jax.experimental.export` has been removed. It was replaced
|
||||
by {mod}`jax.export` in JAX v0.4.30. See the [migration guide](https://jax.readthedocs.io/en/latest/export/export.html#migration-guide-from-jax-experimental-export)
|
||||
by {mod}`jax.export` in JAX v0.4.30. See the [migration guide](https://docs.jax.dev/en/latest/export/export.html#migration-guide-from-jax-experimental-export)
|
||||
for information on migrating to the new API.
|
||||
* The `initial` argument to {func}`jax.nn.softmax` and {func}`jax.nn.log_softmax`
|
||||
has been removed, after being deprecated in v0.4.27.
|
||||
@ -297,7 +297,7 @@ This is a patch release of jax 0.4.36. Only "jax" was released at this version.
|
||||
call that we guarantee export stability. This is because this custom call
|
||||
relies on Triton IR, which is not guaranteed to be stable. If you need
|
||||
to export code that uses this custom call, you can use the `disabled_checks`
|
||||
parameter. See more details in the [documentation](https://jax.readthedocs.io/en/latest/export/export.html#compatibility-guarantees-for-custom-calls).
|
||||
parameter. See more details in the [documentation](https://docs.jax.dev/en/latest/export/export.html#compatibility-guarantees-for-custom-calls).
|
||||
|
||||
* New Features
|
||||
* {func}`jax.jit` got a new `compiler_options: dict[str, Any]` argument, for
|
||||
@ -577,7 +577,7 @@ See the 0.4.33 release notes for more details.
|
||||
* Added an API for exporting and serializing JAX functions. This used
|
||||
to exist in `jax.experimental.export` (which is being deprecated),
|
||||
and will now live in `jax.export`.
|
||||
See the [documentation](https://jax.readthedocs.io/en/latest/export/index.html).
|
||||
See the [documentation](https://docs.jax.dev/en/latest/export/index.html).
|
||||
|
||||
* Deprecations
|
||||
* Internal pretty-printing tools `jax.core.pp_*` are deprecated, and will be removed
|
||||
@ -586,7 +586,7 @@ See the 0.4.33 release notes for more details.
|
||||
release. This previously was the case, but there was an inadvertent regression in
|
||||
the last several JAX releases.
|
||||
* `jax.experimental.export` is deprecated. Use {mod}`jax.export` instead.
|
||||
See the [migration guide](https://jax.readthedocs.io/en/latest/export/export.html#migration-guide-from-jax-experimental-export).
|
||||
See the [migration guide](https://docs.jax.dev/en/latest/export/export.html#migration-guide-from-jax-experimental-export).
|
||||
* Passing an array in place of a dtype is now deprecated in most cases; e.g. for arrays
|
||||
`x` and `y`, `x.astype(y)` will raise a warning. To silence it use `x.astype(y.dtype)`.
|
||||
* `jax.xla_computation` is deprecated and will be removed in a future release.
|
||||
@ -798,7 +798,7 @@ See the 0.4.33 release notes for more details.
|
||||
deprecated. Use `jax.experimental.shard_map` or `jax.vmap` with the
|
||||
`spmd_axis_name` argument for expressing SPMD device-parallel computations.
|
||||
* The `jax.experimental.host_callback` module is deprecated.
|
||||
Use instead the [new JAX external callbacks](https://jax.readthedocs.io/en/latest/notebooks/external_callbacks.html).
|
||||
Use instead the [new JAX external callbacks](https://docs.jax.dev/en/latest/notebooks/external_callbacks.html).
|
||||
Added `JAX_HOST_CALLBACK_LEGACY` flag to assist in the transition to the
|
||||
new callbacks. See {jax-issue}`#20385` for a discussion.
|
||||
* Passing arguments to {func}`jax.numpy.array_equal` and {func}`jax.numpy.array_equiv`
|
||||
@ -1270,9 +1270,9 @@ See the 0.4.33 release notes for more details.
|
||||
|
||||
* Deprecations
|
||||
* Python 3.8 support has been dropped as per
|
||||
https://jax.readthedocs.io/en/latest/deprecation.html
|
||||
https://docs.jax.dev/en/latest/deprecation.html
|
||||
* JAX now requires NumPy 1.22 or newer as per
|
||||
https://jax.readthedocs.io/en/latest/deprecation.html
|
||||
https://docs.jax.dev/en/latest/deprecation.html
|
||||
* Passing optional arguments to {func}`jax.numpy.ndarray.at` by position is
|
||||
no longer supported, after being deprecated in JAX version 0.4.7.
|
||||
For example, instead of `x.at[i].get(True)`, use `x.at[i].get(indices_are_sorted=True)`
|
||||
@ -1317,7 +1317,7 @@ See the 0.4.33 release notes for more details.
|
||||
|
||||
* Deprecations
|
||||
* Python 3.8 support has been dropped as per
|
||||
https://jax.readthedocs.io/en/latest/deprecation.html
|
||||
https://docs.jax.dev/en/latest/deprecation.html
|
||||
|
||||
## jax 0.4.13 (June 22, 2023)
|
||||
|
||||
@ -1496,7 +1496,7 @@ See the 0.4.33 release notes for more details.
|
||||
## jax 0.4.7 (March 27, 2023)
|
||||
|
||||
* Changes
|
||||
* As per https://jax.readthedocs.io/en/latest/jax_array_migration.html#jax-array-migration
|
||||
* As per https://docs.jax.dev/en/latest/jax_array_migration.html#jax-array-migration
|
||||
`jax.config.jax_array` cannot be disabled anymore.
|
||||
* `jax.config.jax_jit_pjit_api_merge` cannot be disabled anymore.
|
||||
* {func}`jax.experimental.jax2tf.convert` now supports the `native_serialization`
|
||||
@ -1580,7 +1580,7 @@ Changes:
|
||||
on top of each other. With the `jit`-`pjit` implementation merge, `jit`
|
||||
becomes an initial style primitive which means that we trace to jaxpr
|
||||
as early as possible. For more information see
|
||||
[this section in autodidax](https://jax.readthedocs.io/en/latest/autodidax.html#on-the-fly-final-style-and-staged-initial-style-processing).
|
||||
[this section in autodidax](https://docs.jax.dev/en/latest/autodidax.html#on-the-fly-final-style-and-staged-initial-style-processing).
|
||||
Moving to initial style should simplify JAX's internals and make
|
||||
development of features like dynamic shapes, etc easier.
|
||||
You can disable it only via the environment variable i.e.
|
||||
@ -1665,9 +1665,9 @@ Changes:
|
||||
simplifies and unifies JAX internals, and allows us to unify `jit` and
|
||||
`pjit`. `jax.Array` has been enabled by default in JAX 0.4 and makes some
|
||||
breaking change to the `pjit` API. The [jax.Array migration
|
||||
guide](https://jax.readthedocs.io/en/latest/jax_array_migration.html) can
|
||||
guide](https://docs.jax.dev/en/latest/jax_array_migration.html) can
|
||||
help you migrate your codebase to `jax.Array`. You can also look at the
|
||||
[Distributed arrays and automatic parallelization](https://jax.readthedocs.io/en/latest/notebooks/Distributed_arrays_and_automatic_parallelization.html)
|
||||
[Distributed arrays and automatic parallelization](https://docs.jax.dev/en/latest/notebooks/Distributed_arrays_and_automatic_parallelization.html)
|
||||
tutorial to understand the new concepts.
|
||||
* `PartitionSpec` and `Mesh` are now out of experimental. The new API endpoints
|
||||
are `jax.sharding.PartitionSpec` and `jax.sharding.Mesh`.
|
||||
@ -1696,7 +1696,7 @@ Changes:
|
||||
* The behavior of `XLA_PYTHON_CLIENT_MEM_FRACTION=.XX` has been changed to allocate XX% of
|
||||
the total GPU memory instead of the previous behavior of using currently available GPU memory
|
||||
to calculate preallocation. Please refer to
|
||||
[GPU memory allocation](https://jax.readthedocs.io/en/latest/gpu_memory_allocation.html) for
|
||||
[GPU memory allocation](https://docs.jax.dev/en/latest/gpu_memory_allocation.html) for
|
||||
more details.
|
||||
* The deprecated method `.block_host_until_ready()` has been removed. Use
|
||||
`.block_until_ready()` instead.
|
||||
@ -1810,7 +1810,7 @@ Changes:
|
||||
* Changes
|
||||
* Ahead-of-time lowering and compilation functionality (tracked in
|
||||
{jax-issue}`#7733`) is stable and public. See [the
|
||||
overview](https://jax.readthedocs.io/en/latest/aot.html) and the API docs
|
||||
overview](https://docs.jax.dev/en/latest/aot.html) and the API docs
|
||||
for {mod}`jax.stages`.
|
||||
* Introduced {class}`jax.Array`, intended to be used for both `isinstance` checks
|
||||
and type annotations for array types in JAX. Notice that this included some subtle
|
||||
@ -1831,7 +1831,7 @@ Changes:
|
||||
* Breaking changes
|
||||
* {func}`jax.checkpoint`, also known as {func}`jax.remat`, no longer supports
|
||||
the `concrete` option, following the previous version's deprecation; see
|
||||
[JEP 11830](https://jax.readthedocs.io/en/latest/jep/11830-new-remat-checkpoint.html).
|
||||
[JEP 11830](https://docs.jax.dev/en/latest/jep/11830-new-remat-checkpoint.html).
|
||||
* Changes
|
||||
* Added {func}`jax.pure_callback` that enables calling back to pure Python functions from compiled functions (e.g. functions decorated with `jax.jit` or `jax.pmap`).
|
||||
* Deprecations:
|
||||
@ -1843,7 +1843,7 @@ Changes:
|
||||
* [GitHub commits](https://github.com/jax-ml/jax/compare/jax-v0.3.15...main).
|
||||
* Breaking changes
|
||||
* Support for NumPy 1.19 has been dropped, per the
|
||||
[deprecation policy](https://jax.readthedocs.io/en/latest/deprecation.html).
|
||||
[deprecation policy](https://docs.jax.dev/en/latest/deprecation.html).
|
||||
Please upgrade to NumPy 1.20 or newer.
|
||||
* Changes
|
||||
* Added {mod}`jax.debug` that includes utilities for runtime value debugging such at {func}`jax.debug.print` and {func}`jax.debug.breakpoint`.
|
||||
@ -1861,7 +1861,7 @@ Changes:
|
||||
{mod}`jax.example_libraries.optimizers`.
|
||||
* {func}`jax.checkpoint`, also known as {func}`jax.remat`, has a new
|
||||
implementation switched on by default, meaning the old implementation is
|
||||
deprecated; see [JEP 11830](https://jax.readthedocs.io/en/latest/jep/11830-new-remat-checkpoint.html).
|
||||
deprecated; see [JEP 11830](https://docs.jax.dev/en/latest/jep/11830-new-remat-checkpoint.html).
|
||||
|
||||
## jax 0.3.15 (July 22, 2022)
|
||||
* [GitHub commits](https://github.com/jax-ml/jax/compare/jax-v0.3.14...jax-v0.3.15).
|
||||
@ -1993,7 +1993,7 @@ Changes:
|
||||
* {func}`jax.numpy.linalg.matrix_rank` on TPUs now accepts complex input.
|
||||
* {func}`jax.scipy.cluster.vq.vq` has been added.
|
||||
* `jax.experimental.maps.mesh` has been deleted.
|
||||
Please use `jax.experimental.maps.Mesh`. Please see https://jax.readthedocs.io/en/latest/_autosummary/jax.experimental.maps.Mesh.html#jax.experimental.maps.Mesh
|
||||
Please use `jax.experimental.maps.Mesh`. Please see https://docs.jax.dev/en/latest/_autosummary/jax.experimental.maps.Mesh.html#jax.experimental.maps.Mesh
|
||||
for more information.
|
||||
* {func}`jax.scipy.linalg.qr` now returns a length-1 tuple rather than the raw array when
|
||||
`mode='r'`, in order to match the behavior of `scipy.linalg.qr` ({jax-issue}`#10452`)
|
||||
@ -2109,7 +2109,7 @@ Changes:
|
||||
* Changes:
|
||||
* The functions `jax.ops.index_update`, `jax.ops.index_add`, which were
|
||||
deprecated in 0.2.22, have been removed. Please use
|
||||
[the `.at` property on JAX arrays](https://jax.readthedocs.io/en/latest/_autosummary/jax.numpy.ndarray.at.html)
|
||||
[the `.at` property on JAX arrays](https://docs.jax.dev/en/latest/_autosummary/jax.numpy.ndarray.at.html)
|
||||
instead, e.g., `x.at[idx].set(y)`.
|
||||
* Moved `jax.experimental.ann.approx_*_k` into `jax.lax`. These functions are
|
||||
optimized alternatives to `jax.lax.top_k`.
|
||||
@ -2155,13 +2155,13 @@ Changes:
|
||||
commits](https://github.com/jax-ml/jax/compare/jax-v0.2.28...jax-v0.3.0).
|
||||
|
||||
* Changes
|
||||
* jax version has been bumped to 0.3.0. Please see the [design doc](https://jax.readthedocs.io/en/latest/design_notes/jax_versioning.html)
|
||||
* jax version has been bumped to 0.3.0. Please see the [design doc](https://docs.jax.dev/en/latest/design_notes/jax_versioning.html)
|
||||
for the explanation.
|
||||
|
||||
## jaxlib 0.3.0 (Feb 10, 2022)
|
||||
* Changes
|
||||
* Bazel 5.0.0 is now required to build jaxlib.
|
||||
* jaxlib version has been bumped to 0.3.0. Please see the [design doc](https://jax.readthedocs.io/en/latest/design_notes/jax_versioning.html)
|
||||
* jaxlib version has been bumped to 0.3.0. Please see the [design doc](https://docs.jax.dev/en/latest/design_notes/jax_versioning.html)
|
||||
for the explanation.
|
||||
|
||||
## jax 0.2.28 (Feb 1, 2022)
|
||||
@ -2183,7 +2183,7 @@ Changes:
|
||||
by default.
|
||||
* Breaking changes
|
||||
* Support for NumPy 1.18 has been dropped, per the
|
||||
[deprecation policy](https://jax.readthedocs.io/en/latest/deprecation.html).
|
||||
[deprecation policy](https://docs.jax.dev/en/latest/deprecation.html).
|
||||
Please upgrade to a supported NumPy version.
|
||||
* Bug fixes
|
||||
* Fixed a bug where apparently identical pytreedef objects constructed by different routes
|
||||
@ -2195,7 +2195,7 @@ Changes:
|
||||
|
||||
* Breaking changes:
|
||||
* Support for NumPy 1.18 has been dropped, per the
|
||||
[deprecation policy](https://jax.readthedocs.io/en/latest/deprecation.html).
|
||||
[deprecation policy](https://docs.jax.dev/en/latest/deprecation.html).
|
||||
Please upgrade to a supported NumPy version.
|
||||
* The host_callback primitives have been simplified to drop the
|
||||
special autodiff handling for hcb.id_tap and id_print.
|
||||
@ -2322,7 +2322,7 @@ Changes:
|
||||
* Deprecations
|
||||
* The functions `jax.ops.index_update`, `jax.ops.index_add` etc. are
|
||||
deprecated and will be removed in a future JAX release. Please use
|
||||
[the `.at` property on JAX arrays](https://jax.readthedocs.io/en/latest/_autosummary/jax.numpy.ndarray.at.html)
|
||||
[the `.at` property on JAX arrays](https://docs.jax.dev/en/latest/_autosummary/jax.numpy.ndarray.at.html)
|
||||
instead, e.g., `x.at[idx].set(y)`. For now, these functions produce a
|
||||
`DeprecationWarning`.
|
||||
* New features:
|
||||
@ -2386,7 +2386,7 @@ Changes:
|
||||
commits](https://github.com/jax-ml/jax/compare/jax-v0.2.18...jax-v0.2.19).
|
||||
* Breaking changes:
|
||||
* Support for NumPy 1.17 has been dropped, per the
|
||||
[deprecation policy](https://jax.readthedocs.io/en/latest/deprecation.html).
|
||||
[deprecation policy](https://docs.jax.dev/en/latest/deprecation.html).
|
||||
Please upgrade to a supported NumPy version.
|
||||
* The `jit` decorator has been added around the implementation of a number of
|
||||
operators on JAX arrays. This speeds up dispatch times for common
|
||||
@ -2407,10 +2407,10 @@ Changes:
|
||||
## jaxlib 0.1.70 (Aug 9, 2021)
|
||||
* Breaking changes:
|
||||
* Support for Python 3.6 has been dropped, per the
|
||||
[deprecation policy](https://jax.readthedocs.io/en/latest/deprecation.html).
|
||||
[deprecation policy](https://docs.jax.dev/en/latest/deprecation.html).
|
||||
Please upgrade to a supported Python version.
|
||||
* Support for NumPy 1.17 has been dropped, per the
|
||||
[deprecation policy](https://jax.readthedocs.io/en/latest/deprecation.html).
|
||||
[deprecation policy](https://docs.jax.dev/en/latest/deprecation.html).
|
||||
Please upgrade to a supported NumPy version.
|
||||
|
||||
* The host_callback mechanism now uses one thread per local device for
|
||||
@ -2424,7 +2424,7 @@ Changes:
|
||||
|
||||
* Breaking changes:
|
||||
* Support for Python 3.6 has been dropped, per the
|
||||
[deprecation policy](https://jax.readthedocs.io/en/latest/deprecation.html).
|
||||
[deprecation policy](https://docs.jax.dev/en/latest/deprecation.html).
|
||||
Please upgrade to a supported Python version.
|
||||
* The minimum jaxlib version is now 0.1.69.
|
||||
* The `backend` argument to {py:func}`jax.dlpack.from_dlpack` has been
|
||||
@ -2473,7 +2473,7 @@ Changes:
|
||||
|
||||
* Breaking changes:
|
||||
* Support for NumPy 1.16 has been dropped, per the
|
||||
[deprecation policy](https://jax.readthedocs.io/en/latest/deprecation.html).
|
||||
[deprecation policy](https://docs.jax.dev/en/latest/deprecation.html).
|
||||
|
||||
* Bug fixes:
|
||||
* Fixed bug that prevented round-tripping from JAX to TF and back:
|
||||
@ -3013,7 +3013,7 @@ Changes:
|
||||
* Support for reduction over subsets of a pmapped axis using `axis_index_groups`
|
||||
{jax-issue}`#2382`.
|
||||
* Experimental support for printing and calling host-side Python function from
|
||||
compiled code. See [id_print and id_tap](https://jax.readthedocs.io/en/latest/jax.experimental.host_callback.html)
|
||||
compiled code. See [id_print and id_tap](https://docs.jax.dev/en/latest/jax.experimental.host_callback.html)
|
||||
({jax-issue}`#3006`).
|
||||
* Notable changes:
|
||||
* The visibility of names exported from {mod}`jax.numpy` has been
|
||||
@ -3085,7 +3085,7 @@ Changes:
|
||||
## jax 0.1.63 (April 12, 2020)
|
||||
|
||||
* [GitHub commits](https://github.com/jax-ml/jax/compare/jax-v0.1.62...jax-v0.1.63).
|
||||
* Added `jax.custom_jvp` and `jax.custom_vjp` from {jax-issue}`#2026`, see the [tutorial notebook](https://jax.readthedocs.io/en/latest/notebooks/Custom_derivative_rules_for_Python_code.html). Deprecated `jax.custom_transforms` and removed it from the docs (though it still works).
|
||||
* Added `jax.custom_jvp` and `jax.custom_vjp` from {jax-issue}`#2026`, see the [tutorial notebook](https://docs.jax.dev/en/latest/notebooks/Custom_derivative_rules_for_Python_code.html). Deprecated `jax.custom_transforms` and removed it from the docs (though it still works).
|
||||
* Add `scipy.sparse.linalg.cg` {jax-issue}`#2566`.
|
||||
* Changed how Tracers are printed to show more useful information for debugging {jax-issue}`#2591`.
|
||||
* Made `jax.numpy.isclose` handle `nan` and `inf` correctly {jax-issue}`#2501`.
|
||||
|
@ -1,4 +1,4 @@
|
||||
# Contributing to JAX
|
||||
|
||||
For information on how to contribute to JAX, see
|
||||
[Contributing to JAX](https://jax.readthedocs.io/en/latest/contributing.html)
|
||||
[Contributing to JAX](https://docs.jax.dev/en/latest/contributing.html)
|
||||
|
60
README.md
60
README.md
@ -11,8 +11,8 @@
|
||||
| [**Transformations**](#transformations)
|
||||
| [**Install guide**](#installation)
|
||||
| [**Neural net libraries**](#neural-network-libraries)
|
||||
| [**Change logs**](https://jax.readthedocs.io/en/latest/changelog.html)
|
||||
| [**Reference docs**](https://jax.readthedocs.io/en/latest/)
|
||||
| [**Change logs**](https://docs.jax.dev/en/latest/changelog.html)
|
||||
| [**Reference docs**](https://docs.jax.dev/en/latest/)
|
||||
|
||||
|
||||
## What is JAX?
|
||||
@ -48,7 +48,7 @@ are instances of such transformations. Others are
|
||||
parallel programming of multiple accelerators, with more to come.
|
||||
|
||||
This is a research project, not an official Google product. Expect
|
||||
[sharp edges](https://jax.readthedocs.io/en/latest/notebooks/Common_Gotchas_in_JAX.html).
|
||||
[sharp edges](https://docs.jax.dev/en/latest/notebooks/Common_Gotchas_in_JAX.html).
|
||||
Please help by trying it out, [reporting
|
||||
bugs](https://github.com/jax-ml/jax/issues), and letting us know what you
|
||||
think!
|
||||
@ -83,15 +83,15 @@ perex_grads = jit(vmap(grad_loss, in_axes=(None, 0, 0))) # fast per-example gra
|
||||
## Quickstart: Colab in the Cloud
|
||||
Jump right in using a notebook in your browser, connected to a Google Cloud GPU.
|
||||
Here are some starter notebooks:
|
||||
- [The basics: NumPy on accelerators, `grad` for differentiation, `jit` for compilation, and `vmap` for vectorization](https://jax.readthedocs.io/en/latest/quickstart.html)
|
||||
- [The basics: NumPy on accelerators, `grad` for differentiation, `jit` for compilation, and `vmap` for vectorization](https://docs.jax.dev/en/latest/quickstart.html)
|
||||
- [Training a Simple Neural Network, with TensorFlow Dataset Data Loading](https://colab.research.google.com/github/jax-ml/jax/blob/main/docs/notebooks/neural_network_with_tfds_data.ipynb)
|
||||
|
||||
**JAX now runs on Cloud TPUs.** To try out the preview, see the [Cloud TPU
|
||||
Colabs](https://github.com/jax-ml/jax/tree/main/cloud_tpu_colabs).
|
||||
|
||||
For a deeper dive into JAX:
|
||||
- [The Autodiff Cookbook, Part 1: easy and powerful automatic differentiation in JAX](https://jax.readthedocs.io/en/latest/notebooks/autodiff_cookbook.html)
|
||||
- [Common gotchas and sharp edges](https://jax.readthedocs.io/en/latest/notebooks/Common_Gotchas_in_JAX.html)
|
||||
- [The Autodiff Cookbook, Part 1: easy and powerful automatic differentiation in JAX](https://docs.jax.dev/en/latest/notebooks/autodiff_cookbook.html)
|
||||
- [Common gotchas and sharp edges](https://docs.jax.dev/en/latest/notebooks/Common_Gotchas_in_JAX.html)
|
||||
- See the [full list of
|
||||
notebooks](https://github.com/jax-ml/jax/tree/main/docs/notebooks).
|
||||
|
||||
@ -105,7 +105,7 @@ Here are four transformations of primary interest: `grad`, `jit`, `vmap`, and
|
||||
|
||||
JAX has roughly the same API as [Autograd](https://github.com/hips/autograd).
|
||||
The most popular function is
|
||||
[`grad`](https://jax.readthedocs.io/en/latest/jax.html#jax.grad)
|
||||
[`grad`](https://docs.jax.dev/en/latest/jax.html#jax.grad)
|
||||
for reverse-mode gradients:
|
||||
|
||||
```python
|
||||
@ -129,13 +129,13 @@ print(grad(grad(grad(tanh)))(1.0))
|
||||
```
|
||||
|
||||
For more advanced autodiff, you can use
|
||||
[`jax.vjp`](https://jax.readthedocs.io/en/latest/jax.html#jax.vjp) for
|
||||
[`jax.vjp`](https://docs.jax.dev/en/latest/jax.html#jax.vjp) for
|
||||
reverse-mode vector-Jacobian products and
|
||||
[`jax.jvp`](https://jax.readthedocs.io/en/latest/jax.html#jax.jvp) for
|
||||
[`jax.jvp`](https://docs.jax.dev/en/latest/jax.html#jax.jvp) for
|
||||
forward-mode Jacobian-vector products. The two can be composed arbitrarily with
|
||||
one another, and with other JAX transformations. Here's one way to compose those
|
||||
to make a function that efficiently computes [full Hessian
|
||||
matrices](https://jax.readthedocs.io/en/latest/_autosummary/jax.hessian.html#jax.hessian):
|
||||
matrices](https://docs.jax.dev/en/latest/_autosummary/jax.hessian.html#jax.hessian):
|
||||
|
||||
```python
|
||||
from jax import jit, jacfwd, jacrev
|
||||
@ -160,15 +160,15 @@ print(abs_val_grad(-1.0)) # prints -1.0 (abs_val is re-evaluated)
|
||||
```
|
||||
|
||||
See the [reference docs on automatic
|
||||
differentiation](https://jax.readthedocs.io/en/latest/jax.html#automatic-differentiation)
|
||||
differentiation](https://docs.jax.dev/en/latest/jax.html#automatic-differentiation)
|
||||
and the [JAX Autodiff
|
||||
Cookbook](https://jax.readthedocs.io/en/latest/notebooks/autodiff_cookbook.html)
|
||||
Cookbook](https://docs.jax.dev/en/latest/notebooks/autodiff_cookbook.html)
|
||||
for more.
|
||||
|
||||
### Compilation with `jit`
|
||||
|
||||
You can use XLA to compile your functions end-to-end with
|
||||
[`jit`](https://jax.readthedocs.io/en/latest/jax.html#just-in-time-compilation-jit),
|
||||
[`jit`](https://docs.jax.dev/en/latest/jax.html#just-in-time-compilation-jit),
|
||||
used either as an `@jit` decorator or as a higher-order function.
|
||||
|
||||
```python
|
||||
@ -189,12 +189,12 @@ You can mix `jit` and `grad` and any other JAX transformation however you like.
|
||||
|
||||
Using `jit` puts constraints on the kind of Python control flow
|
||||
the function can use; see
|
||||
the tutorial on [Control Flow and Logical Operators with JIT](https://jax.readthedocs.io/en/latest/control-flow.html)
|
||||
the tutorial on [Control Flow and Logical Operators with JIT](https://docs.jax.dev/en/latest/control-flow.html)
|
||||
for more.
|
||||
|
||||
### Auto-vectorization with `vmap`
|
||||
|
||||
[`vmap`](https://jax.readthedocs.io/en/latest/jax.html#vectorization-vmap) is
|
||||
[`vmap`](https://docs.jax.dev/en/latest/jax.html#vectorization-vmap) is
|
||||
the vectorizing map.
|
||||
It has the familiar semantics of mapping a function along array axes, but
|
||||
instead of keeping the loop on the outside, it pushes the loop down into a
|
||||
@ -259,7 +259,7 @@ differentiation for fast Jacobian and Hessian matrix calculations in
|
||||
### SPMD programming with `pmap`
|
||||
|
||||
For parallel programming of multiple accelerators, like multiple GPUs, use
|
||||
[`pmap`](https://jax.readthedocs.io/en/latest/jax.html#parallelization-pmap).
|
||||
[`pmap`](https://docs.jax.dev/en/latest/jax.html#parallelization-pmap).
|
||||
With `pmap` you write single-program multiple-data (SPMD) programs, including
|
||||
fast parallel collective communication operations. Applying `pmap` will mean
|
||||
that the function you write is compiled by XLA (similarly to `jit`), then
|
||||
@ -284,7 +284,7 @@ print(pmap(jnp.mean)(result))
|
||||
```
|
||||
|
||||
In addition to expressing pure maps, you can use fast [collective communication
|
||||
operations](https://jax.readthedocs.io/en/latest/jax.lax.html#parallel-operators)
|
||||
operations](https://docs.jax.dev/en/latest/jax.lax.html#parallel-operators)
|
||||
between devices:
|
||||
|
||||
```python
|
||||
@ -341,20 +341,20 @@ for more.
|
||||
|
||||
For a more thorough survey of current gotchas, with examples and explanations,
|
||||
we highly recommend reading the [Gotchas
|
||||
Notebook](https://jax.readthedocs.io/en/latest/notebooks/Common_Gotchas_in_JAX.html).
|
||||
Notebook](https://docs.jax.dev/en/latest/notebooks/Common_Gotchas_in_JAX.html).
|
||||
Some standouts:
|
||||
|
||||
1. JAX transformations only work on [pure functions](https://en.wikipedia.org/wiki/Pure_function), which don't have side-effects and respect [referential transparency](https://en.wikipedia.org/wiki/Referential_transparency) (i.e. object identity testing with `is` isn't preserved). If you use a JAX transformation on an impure Python function, you might see an error like `Exception: Can't lift Traced...` or `Exception: Different traces at same level`.
|
||||
1. [In-place mutating updates of
|
||||
arrays](https://jax.readthedocs.io/en/latest/notebooks/Common_Gotchas_in_JAX.html#in-place-updates), like `x[i] += y`, aren't supported, but [there are functional alternatives](https://jax.readthedocs.io/en/latest/jax.ops.html). Under a `jit`, those functional alternatives will reuse buffers in-place automatically.
|
||||
arrays](https://docs.jax.dev/en/latest/notebooks/Common_Gotchas_in_JAX.html#in-place-updates), like `x[i] += y`, aren't supported, but [there are functional alternatives](https://docs.jax.dev/en/latest/jax.ops.html). Under a `jit`, those functional alternatives will reuse buffers in-place automatically.
|
||||
1. [Random numbers are
|
||||
different](https://jax.readthedocs.io/en/latest/random-numbers.html), but for [good reasons](https://github.com/jax-ml/jax/blob/main/docs/jep/263-prng.md).
|
||||
different](https://docs.jax.dev/en/latest/random-numbers.html), but for [good reasons](https://github.com/jax-ml/jax/blob/main/docs/jep/263-prng.md).
|
||||
1. If you're looking for [convolution
|
||||
operators](https://jax.readthedocs.io/en/latest/notebooks/convolutions.html),
|
||||
operators](https://docs.jax.dev/en/latest/notebooks/convolutions.html),
|
||||
they're in the `jax.lax` package.
|
||||
1. JAX enforces single-precision (32-bit, e.g. `float32`) values by default, and
|
||||
[to enable
|
||||
double-precision](https://jax.readthedocs.io/en/latest/notebooks/Common_Gotchas_in_JAX.html#double-64bit-precision)
|
||||
double-precision](https://docs.jax.dev/en/latest/notebooks/Common_Gotchas_in_JAX.html#double-64bit-precision)
|
||||
(64-bit, e.g. `float64`) one needs to set the `jax_enable_x64` variable at
|
||||
startup (or set the environment variable `JAX_ENABLE_X64=True`).
|
||||
On TPU, JAX uses 32-bit values by default for everything _except_ internal
|
||||
@ -368,14 +368,14 @@ Some standouts:
|
||||
and NumPy types aren't preserved, namely `np.add(1, np.array([2],
|
||||
np.float32)).dtype` is `float64` rather than `float32`.
|
||||
1. Some transformations, like `jit`, [constrain how you can use Python control
|
||||
flow](https://jax.readthedocs.io/en/latest/control-flow.html).
|
||||
flow](https://docs.jax.dev/en/latest/control-flow.html).
|
||||
You'll always get loud errors if something goes wrong. You might have to use
|
||||
[`jit`'s `static_argnums`
|
||||
parameter](https://jax.readthedocs.io/en/latest/jax.html#just-in-time-compilation-jit),
|
||||
parameter](https://docs.jax.dev/en/latest/jax.html#just-in-time-compilation-jit),
|
||||
[structured control flow
|
||||
primitives](https://jax.readthedocs.io/en/latest/jax.lax.html#control-flow-operators)
|
||||
primitives](https://docs.jax.dev/en/latest/jax.lax.html#control-flow-operators)
|
||||
like
|
||||
[`lax.scan`](https://jax.readthedocs.io/en/latest/_autosummary/jax.lax.scan.html#jax.lax.scan),
|
||||
[`lax.scan`](https://docs.jax.dev/en/latest/_autosummary/jax.lax.scan.html#jax.lax.scan),
|
||||
or just use `jit` on smaller subfunctions.
|
||||
|
||||
## Installation
|
||||
@ -403,7 +403,7 @@ Some standouts:
|
||||
| Mac GPU | Follow [Apple's instructions](https://developer.apple.com/metal/jax/). |
|
||||
| Intel GPU | Follow [Intel's instructions](https://github.com/intel/intel-extension-for-openxla/blob/main/docs/acc_jax.md). |
|
||||
|
||||
See [the documentation](https://jax.readthedocs.io/en/latest/installation.html)
|
||||
See [the documentation](https://docs.jax.dev/en/latest/installation.html)
|
||||
for information on alternative installation strategies. These include compiling
|
||||
from source, installing with Docker, using other versions of CUDA, a
|
||||
community-supported conda build, and answers to some frequently-asked questions.
|
||||
@ -417,7 +417,7 @@ for training neural networks in JAX. If you want a fully featured library for ne
|
||||
training with examples and how-to guides, try
|
||||
[Flax](https://github.com/google/flax) and its [documentation site](https://flax.readthedocs.io/en/latest/nnx/index.html).
|
||||
|
||||
Check out the [JAX Ecosystem section](https://jax.readthedocs.io/en/latest/#ecosystem)
|
||||
Check out the [JAX Ecosystem section](https://docs.jax.dev/en/latest/#ecosystem)
|
||||
on the JAX documentation site for a list of JAX-based network libraries, which includes
|
||||
[Optax](https://github.com/deepmind/optax) for gradient processing and
|
||||
optimization, [chex](https://github.com/deepmind/chex) for reliable code and testing, and
|
||||
@ -452,7 +452,7 @@ paper.
|
||||
## Reference documentation
|
||||
|
||||
For details about the JAX API, see the
|
||||
[reference documentation](https://jax.readthedocs.io/).
|
||||
[reference documentation](https://docs.jax.dev/).
|
||||
|
||||
For getting started as a JAX developer, see the
|
||||
[developer documentation](https://jax.readthedocs.io/en/latest/developer.html).
|
||||
[developer documentation](https://docs.jax.dev/en/latest/developer.html).
|
||||
|
@ -225,7 +225,7 @@
|
||||
"* Jacobian pre-accumulation for elementwise operations (like `gelu`)\n",
|
||||
"\n",
|
||||
"\n",
|
||||
"For much more, see the [JAX Autodiff Cookbook (Part 1)](https://jax.readthedocs.io/en/latest/notebooks/autodiff_cookbook.html)."
|
||||
"For much more, see the [JAX Autodiff Cookbook (Part 1)](https://docs.jax.dev/en/latest/notebooks/autodiff_cookbook.html)."
|
||||
]
|
||||
},
|
||||
{
|
||||
|
@ -315,7 +315,7 @@
|
||||
"* Jacobian pre-accumulation for elementwise operations (like `gelu`)\n",
|
||||
"\n",
|
||||
"\n",
|
||||
"For much more, see the [JAX Autodiff Cookbook (Part 1)](https://jax.readthedocs.io/en/latest/notebooks/autodiff_cookbook.html)."
|
||||
"For much more, see the [JAX Autodiff Cookbook (Part 1)](https://docs.jax.dev/en/latest/notebooks/autodiff_cookbook.html)."
|
||||
]
|
||||
},
|
||||
{
|
||||
|
@ -59,7 +59,7 @@
|
||||
"id": "2e_06-OAJNyi"
|
||||
},
|
||||
"source": [
|
||||
"A basic starting point is expressing parallel maps with [`pmap`](https://jax.readthedocs.io/en/latest/jax.html#jax.pmap):"
|
||||
"A basic starting point is expressing parallel maps with [`pmap`](https://docs.jax.dev/en/latest/jax.html#jax.pmap):"
|
||||
]
|
||||
},
|
||||
{
|
||||
@ -407,7 +407,7 @@
|
||||
"source": [
|
||||
"When writing nested `pmap` functions in the decorator style, axis names are resolved according to lexical scoping.\n",
|
||||
"\n",
|
||||
"Check [the JAX reference documentation](https://jax.readthedocs.io/en/latest/jax.lax.html#parallel-operators) for a complete list of the parallel operators. More are being added!\n",
|
||||
"Check [the JAX reference documentation](https://docs.jax.dev/en/latest/jax.lax.html#parallel-operators) for a complete list of the parallel operators. More are being added!\n",
|
||||
"\n",
|
||||
"Here's how to use `lax.ppermute` to implement a simple halo exchange for a [Rule 30](https://en.wikipedia.org/wiki/Rule_30) simulation:"
|
||||
]
|
||||
|
@ -4,7 +4,7 @@ The same JAX code that runs on CPU and GPU can also be run on TPU. Cloud TPUs
|
||||
have the advantage of quickly giving you access to multiple TPU accelerators,
|
||||
including in [Colab](https://research.google.com/colaboratory/). All of the
|
||||
example notebooks here use
|
||||
[`jax.pmap`](https://jax.readthedocs.io/en/latest/jax.html#jax.pmap) to run JAX
|
||||
[`jax.pmap`](https://docs.jax.dev/en/latest/jax.html#jax.pmap) to run JAX
|
||||
computation across multiple TPU cores from Colab. You can also run the same code
|
||||
directly on a [Cloud TPU
|
||||
VM](https://cloud.google.com/tpu/docs/jax-quickstart-tpu-vm).
|
||||
|
@ -1,2 +1,2 @@
|
||||
To rebuild the documentation,
|
||||
see [Update Documentation](https://jax.readthedocs.io/en/latest/developer.html#update-documentation).
|
||||
see [Update Documentation](https://docs.jax.dev/en/latest/developer.html#update-documentation).
|
||||
|
@ -19,7 +19,7 @@ technology stack](#components). First, we design the `jax` module
|
||||
to be
|
||||
[composable](https://github.com/jax-ml/jax?tab=readme-ov-file#transformations)
|
||||
and
|
||||
[extensible](https://jax.readthedocs.io/en/latest/jax.extend.html), so
|
||||
[extensible](https://docs.jax.dev/en/latest/jax.extend.html), so
|
||||
that a wide variety of domain-specific libraries can thrive outside of
|
||||
it in a decentralized manner. Second, we lean heavily on a modular
|
||||
backend stack (compiler and runtime) to target different
|
||||
@ -42,10 +42,10 @@ scale.
|
||||
JAX's day-to-day development takes place in the open on GitHub, using
|
||||
pull requests, the issue tracker, discussions, and [JAX Enhancement
|
||||
Proposals
|
||||
(JEPs)](https://jax.readthedocs.io/en/latest/jep/index.html). Reading
|
||||
(JEPs)](https://docs.jax.dev/en/latest/jep/index.html). Reading
|
||||
and participating in these is a good way to get involved. We also
|
||||
maintain [developer
|
||||
notes](https://jax.readthedocs.io/en/latest/contributor_guide.html)
|
||||
notes](https://docs.jax.dev/en/latest/contributor_guide.html)
|
||||
that cover JAX's internal design.
|
||||
|
||||
The JAX core team determines whether to accept changes and
|
||||
@ -56,7 +56,7 @@ intricate decision structure over time (e.g. with designated area
|
||||
owners) if/when it becomes useful to do so.
|
||||
|
||||
For more see [contributing to
|
||||
JAX](https://jax.readthedocs.io/en/latest/contributing.html).
|
||||
JAX](https://docs.jax.dev/en/latest/contributing.html).
|
||||
|
||||
(components)=
|
||||
## A modular stack
|
||||
@ -71,7 +71,7 @@ and (b) an advancing hardware landscape, we lean heavily on
|
||||
While the JAX core library focuses on the fundamentals, we want to
|
||||
encourage domain-specific libraries and tools to be built on top of
|
||||
JAX. Indeed, [many
|
||||
libraries](https://jax.readthedocs.io/en/latest/#ecosystem) have
|
||||
libraries](https://docs.jax.dev/en/latest/#ecosystem) have
|
||||
emerged around JAX to offer higher-level features and extensions.
|
||||
|
||||
How do we encourage such decentralized development? We guide it with
|
||||
@ -80,11 +80,11 @@ building blocks (e.g. numerical primitives, NumPy operations, arrays,
|
||||
and transformations), encouraging auxiliary libraries to develop
|
||||
utilities as needed for their domain. In addition, JAX exposes a
|
||||
handful of more advanced APIs for
|
||||
[customization](https://jax.readthedocs.io/en/latest/notebooks/Custom_derivative_rules_for_Python_code.html)
|
||||
[customization](https://docs.jax.dev/en/latest/notebooks/Custom_derivative_rules_for_Python_code.html)
|
||||
and
|
||||
[extensibility](https://jax.readthedocs.io/en/latest/jax.extend.html). Libraries
|
||||
[extensibility](https://docs.jax.dev/en/latest/jax.extend.html). Libraries
|
||||
can [lean on these
|
||||
APIs](https://jax.readthedocs.io/en/latest/building_on_jax.html) in
|
||||
APIs](https://docs.jax.dev/en/latest/building_on_jax.html) in
|
||||
order to use JAX as an internal means of implementation, to integrate
|
||||
more with its transformations like autodiff, and more.
|
||||
|
||||
|
@ -876,7 +876,7 @@ There are two ways to define differentiation rules in JAX:
|
||||
1. Using {func}`jax.custom_jvp` and {func}`jax.custom_vjp` to define custom differentiation rules for Python functions that are already JAX-transformable; and
|
||||
2. Defining new `core.Primitive` instances along with all their transformation rules, for example to call into functions from other systems like solvers, simulators, or general numerical computing systems.
|
||||
|
||||
This notebook is about #1. To read instead about #2, refer to the [notebook on adding primitives](https://jax.readthedocs.io/en/latest/notebooks/How_JAX_primitives_work.html).
|
||||
This notebook is about #1. To read instead about #2, refer to the [notebook on adding primitives](https://docs.jax.dev/en/latest/notebooks/How_JAX_primitives_work.html).
|
||||
|
||||
|
||||
### TL;DR: Custom JVPs with {func}`jax.custom_jvp`
|
||||
@ -1608,7 +1608,7 @@ Array(-0.91113025, dtype=float32)
|
||||
|
||||
#### Working with `list` / `tuple` / `dict` containers (and other pytrees)
|
||||
|
||||
You should expect standard Python containers like lists, tuples, namedtuples, and dicts to just work, along with nested versions of those. In general, any [pytrees](https://jax.readthedocs.io/en/latest/pytrees.html) are permissible, so long as their structures are consistent according to the type constraints.
|
||||
You should expect standard Python containers like lists, tuples, namedtuples, and dicts to just work, along with nested versions of those. In general, any [pytrees](https://docs.jax.dev/en/latest/pytrees.html) are permissible, so long as their structures are consistent according to the type constraints.
|
||||
|
||||
Here's a contrived example with {func}`jax.custom_jvp`:
|
||||
|
||||
|
@ -26,7 +26,7 @@ are arrays, JAX does the following in order:
|
||||
carries out this specialization by a process that we call
|
||||
_tracing_. During tracing, JAX stages the specialization of `F` to
|
||||
a jaxpr, which is a function in the [Jaxpr intermediate
|
||||
language](https://jax.readthedocs.io/en/latest/jaxpr.html).
|
||||
language](https://docs.jax.dev/en/latest/jaxpr.html).
|
||||
|
||||
2. **Lower** this specialized, staged-out computation to the XLA compiler's
|
||||
input language, StableHLO.
|
||||
|
@ -91,7 +91,7 @@ guarantees of the main JAX package. If you have code that uses `jax.extend`,
|
||||
we would strongly recommend CI tests against JAX's nightly releases, so as to
|
||||
catch potential changes before they are released.
|
||||
|
||||
For details on `jax.extend`, see the [`jax.extend` module docuementation](https://jax.readthedocs.io/en/latest/jax.extend.html), or the design document, {ref}`jax-extend-jep`.
|
||||
For details on `jax.extend`, see the [`jax.extend` module docuementation](https://docs.jax.dev/en/latest/jax.extend.html), or the design document, {ref}`jax-extend-jep`.
|
||||
|
||||
## Numerics and randomness
|
||||
|
||||
|
@ -72,7 +72,7 @@
|
||||
"outputs, we want to override primitive application and let different values\n",
|
||||
"flow through our program. For example, we might want to replace the\n",
|
||||
"application of every primitive with an application of [its JVP\n",
|
||||
"rule](https://jax.readthedocs.io/en/latest/notebooks/autodiff_cookbook.html),\n",
|
||||
"rule](https://docs.jax.dev/en/latest/notebooks/autodiff_cookbook.html),\n",
|
||||
"and let primal-tangent pairs flow through our program. Moreover, we want to be\n",
|
||||
"able to compose multiple transformations, leading to stacks of interpreters."
|
||||
]
|
||||
@ -3620,7 +3620,7 @@
|
||||
"source": [
|
||||
"Notice that we're not currently supporting the case where the predicate value\n",
|
||||
"itself is batched. In mainline JAX, we handle this case by transforming the\n",
|
||||
"conditional to a [select primitive](https://jax.readthedocs.io/en/latest/_autosummary/jax.lax.select.html).\n",
|
||||
"conditional to a [select primitive](https://docs.jax.dev/en/latest/_autosummary/jax.lax.select.html).\n",
|
||||
"That transformation is semantically correct so long as `true_fun` and\n",
|
||||
"`false_fun` do not involve any side-effecting primitives.\n",
|
||||
"\n",
|
||||
|
@ -72,7 +72,7 @@ where we apply primitive operations to numerical inputs to produce numerical
|
||||
outputs, we want to override primitive application and let different values
|
||||
flow through our program. For example, we might want to replace the
|
||||
application of every primitive with an application of [its JVP
|
||||
rule](https://jax.readthedocs.io/en/latest/notebooks/autodiff_cookbook.html),
|
||||
rule](https://docs.jax.dev/en/latest/notebooks/autodiff_cookbook.html),
|
||||
and let primal-tangent pairs flow through our program. Moreover, we want to be
|
||||
able to compose multiple transformations, leading to stacks of interpreters.
|
||||
|
||||
@ -2843,7 +2843,7 @@ print(out)
|
||||
|
||||
Notice that we're not currently supporting the case where the predicate value
|
||||
itself is batched. In mainline JAX, we handle this case by transforming the
|
||||
conditional to a [select primitive](https://jax.readthedocs.io/en/latest/_autosummary/jax.lax.select.html).
|
||||
conditional to a [select primitive](https://docs.jax.dev/en/latest/_autosummary/jax.lax.select.html).
|
||||
That transformation is semantically correct so long as `true_fun` and
|
||||
`false_fun` do not involve any side-effecting primitives.
|
||||
|
||||
|
@ -62,7 +62,7 @@
|
||||
# outputs, we want to override primitive application and let different values
|
||||
# flow through our program. For example, we might want to replace the
|
||||
# application of every primitive with an application of [its JVP
|
||||
# rule](https://jax.readthedocs.io/en/latest/notebooks/autodiff_cookbook.html),
|
||||
# rule](https://docs.jax.dev/en/latest/notebooks/autodiff_cookbook.html),
|
||||
# and let primal-tangent pairs flow through our program. Moreover, we want to be
|
||||
# able to compose multiple transformations, leading to stacks of interpreters.
|
||||
|
||||
@ -2837,7 +2837,7 @@ print(out)
|
||||
|
||||
# Notice that we're not currently supporting the case where the predicate value
|
||||
# itself is batched. In mainline JAX, we handle this case by transforming the
|
||||
# conditional to a [select primitive](https://jax.readthedocs.io/en/latest/_autosummary/jax.lax.select.html).
|
||||
# conditional to a [select primitive](https://docs.jax.dev/en/latest/_autosummary/jax.lax.select.html).
|
||||
# That transformation is semantically correct so long as `true_fun` and
|
||||
# `false_fun` do not involve any side-effecting primitives.
|
||||
#
|
||||
|
@ -45,8 +45,8 @@ Here are more specific examples of each pattern.
|
||||
|
||||
### Direct usage
|
||||
Jax can be directly imported and utilized to build models “from scratch” as shown across this website,
|
||||
for example in [JAX Tutorials](https://jax.readthedocs.io/en/latest/tutorials.html)
|
||||
or [Neural Network with JAX](https://jax.readthedocs.io/en/latest/notebooks/neural_network_with_tfds_data.html).
|
||||
for example in [JAX Tutorials](https://docs.jax.dev/en/latest/tutorials.html)
|
||||
or [Neural Network with JAX](https://docs.jax.dev/en/latest/notebooks/neural_network_with_tfds_data.html).
|
||||
This may be the best option if you are unable to find prebuilt code
|
||||
for your particular challenge, or if you're looking to reduce the number
|
||||
of dependencies in your codebase.
|
||||
|
@ -6,7 +6,7 @@ Everyone can contribute to JAX, and we value everyone's contributions. There are
|
||||
ways to contribute, including:
|
||||
|
||||
- Answering questions on JAX's [discussions page](https://github.com/jax-ml/jax/discussions)
|
||||
- Improving or expanding JAX's [documentation](http://jax.readthedocs.io/)
|
||||
- Improving or expanding JAX's [documentation](http://docs.jax.dev/)
|
||||
- Contributing to JAX's [code-base](http://github.com/jax-ml/jax/)
|
||||
- Contributing in any of the above ways to the broader ecosystem of [libraries built on JAX](https://github.com/jax-ml/jax#neural-network-libraries)
|
||||
|
||||
|
@ -244,19 +244,19 @@ lax.cond(False, lambda x: x+1, lambda x: x-1, operand)
|
||||
|
||||
`jax.lax` provides two other functions that allow branching on dynamic predicates:
|
||||
|
||||
- [`lax.select`](https://jax.readthedocs.io/en/latest/_autosummary/jax.lax.select.html) is
|
||||
- [`lax.select`](https://docs.jax.dev/en/latest/_autosummary/jax.lax.select.html) is
|
||||
like a batched version of `lax.cond`, with the choices expressed as pre-computed arrays
|
||||
rather than as functions.
|
||||
- [`lax.switch`](https://jax.readthedocs.io/en/latest/_autosummary/jax.lax.switch.html) is
|
||||
- [`lax.switch`](https://docs.jax.dev/en/latest/_autosummary/jax.lax.switch.html) is
|
||||
like `lax.cond`, but allows switching between any number of callable choices.
|
||||
|
||||
In addition, `jax.numpy` provides several numpy-style interfaces to these functions:
|
||||
|
||||
- [`jnp.where`](https://jax.readthedocs.io/en/latest/_autosummary/jax.numpy.where.html) with
|
||||
- [`jnp.where`](https://docs.jax.dev/en/latest/_autosummary/jax.numpy.where.html) with
|
||||
three arguments is the numpy-style wrapper of `lax.select`.
|
||||
- [`jnp.piecewise`](https://jax.readthedocs.io/en/latest/_autosummary/jax.numpy.piecewise.html)
|
||||
- [`jnp.piecewise`](https://docs.jax.dev/en/latest/_autosummary/jax.numpy.piecewise.html)
|
||||
is a numpy-style wrapper of `lax.switch`, but switches on a list of boolean conditions rather than a single scalar index.
|
||||
- [`jnp.select`](https://jax.readthedocs.io/en/latest/_autosummary/jax.numpy.select.html) has
|
||||
- [`jnp.select`](https://docs.jax.dev/en/latest/_autosummary/jax.numpy.select.html) has
|
||||
an API similar to `jnp.piecewise`, but the choices are given as pre-computed arrays rather
|
||||
than as functions. It is implemented in terms of multiple calls to `lax.select`.
|
||||
|
||||
|
@ -789,7 +789,7 @@ desired formats, and which the `jupytext --sync` command recognizes when invoked
|
||||
#### Notebooks within the Sphinx build
|
||||
|
||||
Some of the notebooks are built automatically as part of the pre-submit checks and
|
||||
as part of the [Read the docs](https://jax.readthedocs.io/en/latest) build.
|
||||
as part of the [Read the docs](https://docs.jax.dev/en/latest) build.
|
||||
The build will fail if cells raise errors. If the errors are intentional, you can either catch them,
|
||||
or tag the cell with `raises-exceptions` metadata ([example PR](https://github.com/jax-ml/jax/pull/2402/files)).
|
||||
You have to add this metadata by hand in the `.ipynb` file. It will be preserved when somebody else
|
||||
@ -800,7 +800,7 @@ See `exclude_patterns` in [conf.py](https://github.com/jax-ml/jax/blob/main/docs
|
||||
|
||||
### Documentation building on `readthedocs.io`
|
||||
|
||||
JAX's auto-generated documentation is at <https://jax.readthedocs.io/>.
|
||||
JAX's auto-generated documentation is at <https://docs.jax.dev/>.
|
||||
|
||||
The documentation building is controlled for the entire project by the
|
||||
[readthedocs JAX settings](https://readthedocs.org/dashboard/jax). The current settings
|
||||
@ -813,7 +813,7 @@ For each automated documentation build you can see the
|
||||
|
||||
If you want to test the documentation generation on Readthedocs, you can push code to the `test-docs`
|
||||
branch. That branch is also built automatically, and you can
|
||||
see the generated documentation [here](https://jax.readthedocs.io/en/test-docs/). If the documentation build
|
||||
see the generated documentation [here](https://docs.jax.dev/en/test-docs/). If the documentation build
|
||||
fails you may want to [wipe the build environment for test-docs](https://docs.readthedocs.io/en/stable/guides/wipe-environment.html).
|
||||
|
||||
For a local test, I was able to do it in a fresh directory by replaying the commands
|
||||
|
@ -161,7 +161,7 @@ e.g., the inference system.)
|
||||
What **matters is when the exporting and consuming components were built**,
|
||||
not the time when the exporting and the compilation happen.
|
||||
For external JAX users, it is
|
||||
[possible to run JAX and jaxlib at different versions](https://jax.readthedocs.io/en/latest/jep/9419-jax-versioning.html#how-are-jax-and-jaxlib-versioned);
|
||||
[possible to run JAX and jaxlib at different versions](https://docs.jax.dev/en/latest/jep/9419-jax-versioning.html#how-are-jax-and-jaxlib-versioned);
|
||||
what matters is when the jaxlib release was built.
|
||||
|
||||
To reduce chances of incompatibility, internal JAX users should:
|
||||
|
@ -86,7 +86,7 @@ matching the structure of the arguments passed to it.
|
||||
The polymorphic shapes specification can be a
|
||||
pytree prefix in cases where one specification should apply
|
||||
to multiple arguments, as in the above example.
|
||||
See [how optional parameters are matched to arguments](https://jax.readthedocs.io/en/latest/pytrees.html#applying-optional-parameters-to-pytrees).
|
||||
See [how optional parameters are matched to arguments](https://docs.jax.dev/en/latest/pytrees.html#applying-optional-parameters-to-pytrees).
|
||||
|
||||
|
||||
A few examples of shape specifications:
|
||||
@ -609,7 +609,7 @@ Division had remainder 1 when computing the value of 'd'.
|
||||
Using the following polymorphic shapes specifications:
|
||||
args[0].shape = (b, b, 2*d).
|
||||
Obtained dimension variables: 'b' = 3 from specification 'b' for dimension args[0].shape[0] (= 3), .
|
||||
Please see https://jax.readthedocs.io/en/latest/export/shape_poly.html#shape-assertion-errors for more details.
|
||||
Please see https://docs.jax.dev/en/latest/export/shape_poly.html#shape-assertion-errors for more details.
|
||||
|
||||
```
|
||||
|
||||
|
18
docs/faq.rst
18
docs/faq.rst
@ -4,7 +4,7 @@ Frequently asked questions (FAQ)
|
||||
.. comment RST primer for Sphinx: https://thomas-cokelaer.info/tutorials/sphinx/rest_syntax.html
|
||||
.. comment Some links referenced here. Use `JAX - The Sharp Bits`_ (underscore at the end) to reference
|
||||
|
||||
.. _JAX - The Sharp Bits: https://jax.readthedocs.io/en/latest/notebooks/Common_Gotchas_in_JAX.html
|
||||
.. _JAX - The Sharp Bits: https://docs.jax.dev/en/latest/notebooks/Common_Gotchas_in_JAX.html
|
||||
|
||||
We are collecting answers to frequently asked questions here.
|
||||
Contributions welcome!
|
||||
@ -116,7 +116,7 @@ code in JAX's internal representation, typically because it makes heavy use of
|
||||
Python control flow such as ``for`` loops. For a handful of loop iterations,
|
||||
Python is OK, but if you need *many* loop iterations, you should rewrite your
|
||||
code to make use of JAX's
|
||||
`structured control flow primitives <https://jax.readthedocs.io/en/latest/control-flow.html#Structured-control-flow-primitives>`_
|
||||
`structured control flow primitives <https://docs.jax.dev/en/latest/control-flow.html#Structured-control-flow-primitives>`_
|
||||
(such as :func:`lax.scan`) or avoid wrapping the loop with ``jit`` (you can
|
||||
still use ``jit`` decorated functions *inside* the loop).
|
||||
|
||||
@ -454,8 +454,8 @@ performing matrix-matrix multiplication) to amortize the increased overhead of
|
||||
JAX/accelerators vs NumPy/CPU. For example, if we switch this example to use
|
||||
10x10 input instead, JAX/GPU runs 10x slower than NumPy/CPU (100 µs vs 10 µs).
|
||||
|
||||
.. _To JIT or not to JIT: https://jax.readthedocs.io/en/latest/notebooks/thinking_in_jax.html#to-jit-or-not-to-jit
|
||||
.. _Double (64 bit) precision: https://jax.readthedocs.io/en/latest/notebooks/Common_Gotchas_in_JAX.html#double-64bit-precision
|
||||
.. _To JIT or not to JIT: https://docs.jax.dev/en/latest/notebooks/thinking_in_jax.html#to-jit-or-not-to-jit
|
||||
.. _Double (64 bit) precision: https://docs.jax.dev/en/latest/notebooks/Common_Gotchas_in_JAX.html#double-64bit-precision
|
||||
.. _`%time and %timeit magics`: https://ipython.readthedocs.io/en/stable/interactive/magics.html#magic-time
|
||||
.. _Colab: https://colab.research.google.com/
|
||||
|
||||
@ -841,12 +841,12 @@ reducing :code:`XLA_PYTHON_CLIENT_MEM_FRACTION` from the default of :code:`.75`,
|
||||
or setting :code:`XLA_PYTHON_CLIENT_PREALLOCATE=false`. For more details, please
|
||||
see the page on `JAX GPU memory allocation`_.
|
||||
|
||||
.. _JIT mechanics: https://jax.readthedocs.io/en/latest/notebooks/thinking_in_jax.html#jit-mechanics-tracing-and-static-variables
|
||||
.. _External callbacks in JAX: https://jax.readthedocs.io/en/latest/notebooks/external_callbacks.html
|
||||
.. _Pure callback example: https://jax.readthedocs.io/en/latest/notebooks/external_callbacks.html#example-pure-callback-with-custom-jvp
|
||||
.. _IO callback example: https://jax.readthedocs.io/en/latest/notebooks/external_callbacks.html#exploring-jax-experimental-io-callback
|
||||
.. _JIT mechanics: https://docs.jax.dev/en/latest/notebooks/thinking_in_jax.html#jit-mechanics-tracing-and-static-variables
|
||||
.. _External callbacks in JAX: https://docs.jax.dev/en/latest/notebooks/external_callbacks.html
|
||||
.. _Pure callback example: https://docs.jax.dev/en/latest/notebooks/external_callbacks.html#example-pure-callback-with-custom-jvp
|
||||
.. _IO callback example: https://docs.jax.dev/en/latest/notebooks/external_callbacks.html#exploring-jax-experimental-io-callback
|
||||
.. _Heaviside Step Function: https://en.wikipedia.org/wiki/Heaviside_step_function
|
||||
.. _Sigmoid Function: https://en.wikipedia.org/wiki/Sigmoid_function
|
||||
.. _algebraic_simplifier.cc: https://github.com/openxla/xla/blob/33f815e190982dac4f20d1f35adb98497a382377/xla/hlo/transforms/simplifiers/algebraic_simplifier.cc#L4851
|
||||
.. _JAX GPU memory allocation: https://jax.readthedocs.io/en/latest/gpu_memory_allocation.html
|
||||
.. _JAX GPU memory allocation: https://docs.jax.dev/en/latest/gpu_memory_allocation.html
|
||||
.. _dynamic linker search pattern: https://man7.org/linux/man-pages/man8/ld.so.8.html
|
||||
|
@ -439,7 +439,7 @@
|
||||
"As far as JAX is concerned, the foreign function is a black box that can't be inspected to determine the appropriate behavior when differentiated.\n",
|
||||
"Therefore, it is the {func}`~jax.ffi.ffi_call` user's responsibility to define a custom derivative rule.\n",
|
||||
"\n",
|
||||
"More details about custom derivative rules can be found in the [custom derivatives tutorial](https://jax.readthedocs.io/en/latest/notebooks/Custom_derivative_rules_for_Python_code.html), but the most common pattern used for implementing differentiation for foreign functions is to define a {func}`~jax.custom_vjp` which itself calls a foreign function.\n",
|
||||
"More details about custom derivative rules can be found in the [custom derivatives tutorial](https://docs.jax.dev/en/latest/notebooks/Custom_derivative_rules_for_Python_code.html), but the most common pattern used for implementing differentiation for foreign functions is to define a {func}`~jax.custom_vjp` which itself calls a foreign function.\n",
|
||||
"In this case, we actually define two new FFI calls:\n",
|
||||
"\n",
|
||||
"1. `rms_norm_fwd` returns two outputs: (a) the \"primal\" result, and (b) the \"residuals\" which are used in the backwards pass.\n",
|
||||
@ -785,7 +785,7 @@
|
||||
"{func}`~jax.experimental.custom_partitioning.custom_partitioning` works by adding Python callbacks into the XLA compiler's partitioning pass, which allows very flexible logic, but also comes with some rough edges.\n",
|
||||
"We won't go into too much detail on the caveats here, but the main issues that you should be aware of are:\n",
|
||||
"\n",
|
||||
"1. `custom_partitioning` can cause unexpected cache misses when used with the JAX's [Persistent compilation cache](https://jax.readthedocs.io/en/latest/persistent_compilation_cache.html). This can be mitigated using the `jax_remove_custom_partitioning_ptr_from_cache_key` configuration flag, but that isn't always appropriate either.\n",
|
||||
"1. `custom_partitioning` can cause unexpected cache misses when used with the JAX's [Persistent compilation cache](https://docs.jax.dev/en/latest/persistent_compilation_cache.html). This can be mitigated using the `jax_remove_custom_partitioning_ptr_from_cache_key` configuration flag, but that isn't always appropriate either.\n",
|
||||
"2. Debugging `custom_partitioning` logic can be tedious because Python errors don't always get propagated, instead causing your Python process to exit. That being said, any exceptions will show up in the process logs, so you should be able to track them down there.\n",
|
||||
"\n",
|
||||
"All that being said, here's how we can wrap our FFI implementation of `rms_norm` using {func}`~jax.experimental.custom_partitioning.custom_partitioning`:"
|
||||
|
@ -353,7 +353,7 @@ Unlike with batching, {func}`~jax.ffi.ffi_call` doesn't provide any default supp
|
||||
As far as JAX is concerned, the foreign function is a black box that can't be inspected to determine the appropriate behavior when differentiated.
|
||||
Therefore, it is the {func}`~jax.ffi.ffi_call` user's responsibility to define a custom derivative rule.
|
||||
|
||||
More details about custom derivative rules can be found in the [custom derivatives tutorial](https://jax.readthedocs.io/en/latest/notebooks/Custom_derivative_rules_for_Python_code.html), but the most common pattern used for implementing differentiation for foreign functions is to define a {func}`~jax.custom_vjp` which itself calls a foreign function.
|
||||
More details about custom derivative rules can be found in the [custom derivatives tutorial](https://docs.jax.dev/en/latest/notebooks/Custom_derivative_rules_for_Python_code.html), but the most common pattern used for implementing differentiation for foreign functions is to define a {func}`~jax.custom_vjp` which itself calls a foreign function.
|
||||
In this case, we actually define two new FFI calls:
|
||||
|
||||
1. `rms_norm_fwd` returns two outputs: (a) the "primal" result, and (b) the "residuals" which are used in the backwards pass.
|
||||
@ -591,7 +591,7 @@ If you can't use {func}`~jax.experimental.shard_map.shard_map`, an alternative a
|
||||
{func}`~jax.experimental.custom_partitioning.custom_partitioning` works by adding Python callbacks into the XLA compiler's partitioning pass, which allows very flexible logic, but also comes with some rough edges.
|
||||
We won't go into too much detail on the caveats here, but the main issues that you should be aware of are:
|
||||
|
||||
1. `custom_partitioning` can cause unexpected cache misses when used with the JAX's [Persistent compilation cache](https://jax.readthedocs.io/en/latest/persistent_compilation_cache.html). This can be mitigated using the `jax_remove_custom_partitioning_ptr_from_cache_key` configuration flag, but that isn't always appropriate either.
|
||||
1. `custom_partitioning` can cause unexpected cache misses when used with the JAX's [Persistent compilation cache](https://docs.jax.dev/en/latest/persistent_compilation_cache.html). This can be mitigated using the `jax_remove_custom_partitioning_ptr_from_cache_key` configuration flag, but that isn't always appropriate either.
|
||||
2. Debugging `custom_partitioning` logic can be tedious because Python errors don't always get propagated, instead causing your Python process to exit. That being said, any exceptions will show up in the process logs, so you should be able to track them down there.
|
||||
|
||||
All that being said, here's how we can wrap our FFI implementation of `rms_norm` using {func}`~jax.experimental.custom_partitioning.custom_partitioning`:
|
||||
|
@ -69,7 +69,7 @@ Common causes of OOM failures
|
||||
disabling the automatic remat pass produces different trade-offs between compute and
|
||||
memory. Note however, that the algorithm is basic and you can often get better
|
||||
trade-off between compute and memory by disabling the automatic remat pass and doing
|
||||
it manually with `the jax.remat API <https://jax.readthedocs.io/en/latest/jep/11830-new-remat-checkpoint.html>`_
|
||||
it manually with `the jax.remat API <https://docs.jax.dev/en/latest/jep/11830-new-remat-checkpoint.html>`_
|
||||
|
||||
|
||||
Experimental features
|
||||
|
@ -229,7 +229,7 @@ refer to
|
||||
JAX has experimental ROCm support. There are two ways to install JAX:
|
||||
|
||||
* Use [AMD's Docker container](https://hub.docker.com/r/rocm/jax-community/tags); or
|
||||
* Build from source. Refer to the section [Additional notes for building a ROCm jaxlib for AMD GPUs](https://jax.readthedocs.io/en/latest/developer.html#additional-notes-for-building-a-rocm-jaxlib-for-amd-gpus).
|
||||
* Build from source. Refer to the section [Additional notes for building a ROCm jaxlib for AMD GPUs](https://docs.jax.dev/en/latest/developer.html#additional-notes-for-building-a-rocm-jaxlib-for-amd-gpus).
|
||||
|
||||
(install-intel-gpu)=
|
||||
## Intel GPU
|
||||
|
@ -300,7 +300,7 @@ def multiply_add_lowering(ctx, xc, yc, zc):
|
||||
return [hlo.AddOp(hlo.MulOp(xc, yc), zc).result]
|
||||
|
||||
# Now, register the lowering rule with JAX.
|
||||
# For GPU, refer to the https://jax.readthedocs.io/en/latest/Custom_Operation_for_GPUs.html
|
||||
# For GPU, refer to the https://docs.jax.dev/en/latest/Custom_Operation_for_GPUs.html
|
||||
from jax.interpreters import mlir
|
||||
|
||||
mlir.register_lowering(multiply_add_p, multiply_add_lowering, platform='cpu')
|
||||
|
@ -27,7 +27,7 @@ the unified jax.Array
|
||||
After the migration is complete `jax.Array` will be the only type of array in
|
||||
JAX.
|
||||
|
||||
This doc explains how to migrate existing codebases to `jax.Array`. For more information on using `jax.Array` and JAX parallelism APIs, see the [Distributed arrays and automatic parallelization](https://jax.readthedocs.io/en/latest/notebooks/Distributed_arrays_and_automatic_parallelization.html) tutorial.
|
||||
This doc explains how to migrate existing codebases to `jax.Array`. For more information on using `jax.Array` and JAX parallelism APIs, see the [Distributed arrays and automatic parallelization](https://docs.jax.dev/en/latest/notebooks/Distributed_arrays_and_automatic_parallelization.html) tutorial.
|
||||
|
||||
|
||||
### How to enable jax.Array?
|
||||
|
@ -47,7 +47,7 @@ g()
|
||||
In many cases, JAX will execute `f` and `g` *in parallel*, dispatching
|
||||
the computations onto different threads -- `g` might actually be executed
|
||||
before `f`. Parallel execution is a nice performance optimization, especially if copying
|
||||
to and from a device is expensive (see the [asynchronous dispatch note](https://jax.readthedocs.io/en/latest/async_dispatch.html) for more details).
|
||||
to and from a device is expensive (see the [asynchronous dispatch note](https://docs.jax.dev/en/latest/async_dispatch.html) for more details).
|
||||
In practice, however, we often don't need to
|
||||
think about asynchronous dispatch because we're writing pure functions and only
|
||||
care about the inputs and outputs of functions -- we'll naturally block on future
|
||||
|
@ -35,7 +35,7 @@ def slice(operand: Array, start_indices: Sequence[int],
|
||||
|
||||
For the purposes of static type checking, this use of `Array = Any` for array type annotations puts no constraint on the argument values (`Any` is equivalent to no annotation at all), but it does serve as a form of useful in-code documentation for the developer.
|
||||
|
||||
For the sake of generated documentation, the name of the alias gets lost (the [HTML docs](https://jax.readthedocs.io/en/latest/_autosummary/jax.lax.slice.html) for `jax.lax.slice` report operand as type `Any`), so the documentation benefit does not go beyond the source code (though we could enable some `sphinx-autodoc` options to improve this: See [autodoc_type_aliases](https://www.sphinx-doc.org/en/master/usage/extensions/autodoc.html#confval-autodoc_type_aliases)).
|
||||
For the sake of generated documentation, the name of the alias gets lost (the [HTML docs](https://docs.jax.dev/en/latest/_autosummary/jax.lax.slice.html) for `jax.lax.slice` report operand as type `Any`), so the documentation benefit does not go beyond the source code (though we could enable some `sphinx-autodoc` options to improve this: See [autodoc_type_aliases](https://www.sphinx-doc.org/en/master/usage/extensions/autodoc.html#confval-autodoc_type_aliases)).
|
||||
|
||||
A benefit of this level of type annotation is that it is never wrong to annotate a value with `Any`, so it will provide a concrete benefit to developers and users in the form of documentation, without added complexity of satisfying the stricter needs of any particular static type checker.
|
||||
|
||||
|
@ -4,7 +4,7 @@
|
||||
*January 2023*
|
||||
|
||||
**This was the design doc proposing `shard_map`. You may instead want
|
||||
[the up-to-date user docs](https://jax.readthedocs.io/en/latest/notebooks/shard_map.html).**
|
||||
[the up-to-date user docs](https://docs.jax.dev/en/latest/notebooks/shard_map.html).**
|
||||
|
||||
## Motivation
|
||||
|
||||
@ -18,7 +18,7 @@ We need great APIs for both, and rather than being mutually exclusive
|
||||
alternatives, they need to compose with each other.
|
||||
|
||||
With `pjit` (now just `jit`) we have [a next-gen
|
||||
API](https://jax.readthedocs.io/en/latest/notebooks/Distributed_arrays_and_automatic_parallelization.html)
|
||||
API](https://docs.jax.dev/en/latest/notebooks/Distributed_arrays_and_automatic_parallelization.html)
|
||||
for the first school. But we haven't quite leveled-up the second school. `pmap`
|
||||
follows the second school, but over time we found it has [fatal
|
||||
flaws](#why-dont-pmap-or-xmap-already-solve-this). `xmap` solved those flaws,
|
||||
|
@ -14,13 +14,13 @@ import jax.extend as jex
|
||||
|
||||
Several projects depend on JAX's codebase internals, often to use its
|
||||
core machinery (e.g. to write a
|
||||
[transformation over its IR](https://jax.readthedocs.io/en/latest/notebooks/Writing_custom_interpreters_in_Jax.html))
|
||||
[transformation over its IR](https://docs.jax.dev/en/latest/notebooks/Writing_custom_interpreters_in_Jax.html))
|
||||
or to extend it (e.g. to
|
||||
[define new primitives](https://github.com/dfm/extending-jax)).
|
||||
Two challenges for these dependencies are (a) that our internals
|
||||
aren't all solidly designed for external use, and (b) that
|
||||
circumventing JAX's public API is
|
||||
[unsupported](https://jax.readthedocs.io/en/latest/api_compatibility.html).
|
||||
[unsupported](https://docs.jax.dev/en/latest/api_compatibility.html).
|
||||
In other words, our internals are often used like a library, but are
|
||||
neither structured nor updated like one.
|
||||
|
||||
@ -50,12 +50,12 @@ removed altogether.
|
||||
|
||||
To keep development overhead low, `jax.extend` would not follow the
|
||||
public
|
||||
[API compatibility](https://jax.readthedocs.io/en/latest/api_compatibility.html)
|
||||
[API compatibility](https://docs.jax.dev/en/latest/api_compatibility.html)
|
||||
policy. It would promise no deprecation windows nor backwards
|
||||
compatibility between releases. Every release may break existing
|
||||
callers without simple recourse (e.g. without a flag reintroducing
|
||||
prior behavior). We would rely on the
|
||||
[changelog](https://jax.readthedocs.io/en/latest/changelog.html)
|
||||
[changelog](https://docs.jax.dev/en/latest/changelog.html)
|
||||
to call out such changes.
|
||||
|
||||
Callers of `jax.extend` that need to upgrade their code regularly
|
||||
@ -108,7 +108,7 @@ to process the Jaxpr IR (the output of
|
||||
At initialization, this module will contain many more symbols than
|
||||
what's needed to define primitives and rules, including various names
|
||||
used in setting up
|
||||
["final-style transformations"](https://jax.readthedocs.io/en/latest/autodidax.html#on-the-fly-final-style-and-staged-initial-style-processing),
|
||||
["final-style transformations"](https://docs.jax.dev/en/latest/autodidax.html#on-the-fly-final-style-and-staged-initial-style-processing),
|
||||
such as the current `jax._src.core.Trace` and `Tracer` classes. We can
|
||||
revisit whether `jex.core` should also support final-style extensions
|
||||
alongside initial style approaches, and whether it can do so by a more
|
||||
@ -137,7 +137,7 @@ tracer types from `jex`.
|
||||
|
||||
This module plus `jex.core` ought to suffice for replicating today's
|
||||
custom primitive tutorials (e.g.
|
||||
[ours](https://jax.readthedocs.io/en/latest/notebooks/How_JAX_primitives_work.html)
|
||||
[ours](https://docs.jax.dev/en/latest/notebooks/How_JAX_primitives_work.html)
|
||||
and
|
||||
[dfm's](https://github.com/dfm/extending-jax)).
|
||||
For instance, defining a primitive and its behavior under `jax.jit`
|
||||
@ -184,6 +184,6 @@ arrays.
|
||||
|
||||
We have only one item in mind for now. The XLA compiler's
|
||||
array sharding format is more expressive than [those provided by
|
||||
JAX](https://jax.readthedocs.io/en/latest/jax.sharding.html). We could
|
||||
JAX](https://docs.jax.dev/en/latest/jax.sharding.html). We could
|
||||
provide this as `jex.sharding.XlaOpShardingProto`, corresponding to
|
||||
today's `jax._src.lib.xla_client.OpSharding` internally.
|
||||
|
@ -497,7 +497,7 @@ of every function instance along which the outputs are mapped, whereas for mesh
|
||||
axes over which the output is unmapped only one copy of the value is used.
|
||||
|
||||
See [the `shmap`
|
||||
JEP](https://jax.readthedocs.io/en/latest/jep/14273-shard-map.html) for examples
|
||||
JEP](https://docs.jax.dev/en/latest/jep/14273-shard-map.html) for examples
|
||||
of unmapped inputs and outputs. For comparison, in `vmap` unmapped
|
||||
inputs/outputs are indicated by using `in_axes` / `out_axes` of `None` (rather
|
||||
than an `int`).
|
||||
|
@ -2,7 +2,7 @@
|
||||
|
||||
This is a design document, explaining some of the thinking behind the design and
|
||||
implementation of `jax.custom_jvp` and `jax.custom_vjp`. For user-oriented
|
||||
documentation, see [the tutorial notebook](https://jax.readthedocs.io/en/latest/notebooks/Custom_derivative_rules_for_Python_code.html).
|
||||
documentation, see [the tutorial notebook](https://docs.jax.dev/en/latest/notebooks/Custom_derivative_rules_for_Python_code.html).
|
||||
|
||||
There are two ways to define differentiation rules in JAX:
|
||||
1. using `jax.custom_jvp` and `jax.custom_vjp` to define custom differentiation
|
||||
|
@ -4,7 +4,7 @@ _Oct 14 2020_
|
||||
|
||||
This doc assumes familiarity with `jax.custom_vjp`, as described in the [Custom
|
||||
derivative rules for JAX-transformable Python
|
||||
functions](https://jax.readthedocs.io/en/latest/notebooks/Custom_derivative_rules_for_Python_code.html)
|
||||
functions](https://docs.jax.dev/en/latest/notebooks/Custom_derivative_rules_for_Python_code.html)
|
||||
notebook.
|
||||
|
||||
## What to update
|
||||
|
@ -266,7 +266,7 @@ While tracing the function ex1 at ex1.py:4, this value became a tracer due to JA
|
||||
|
||||
You can use transformation parameters such as `static_argnums` for `jit` to avoid tracing particular arguments of transformed functions.
|
||||
|
||||
See https://jax.readthedocs.io/en/latest/faq.html#abstract-tracer-value-encountered-where-concrete-value-is-expected-error for more information.
|
||||
See https://docs.jax.dev/en/latest/faq.html#abstract-tracer-value-encountered-where-concrete-value-is-expected-error for more information.
|
||||
|
||||
Encountered tracer value: Traced<ShapedArray(int32[])>with<DynamicJaxprTrace(level=0/1)>
|
||||
```
|
||||
|
@ -12,7 +12,7 @@
|
||||
"\n",
|
||||
"*Jake VanderPlas, December 2021*\n",
|
||||
"\n",
|
||||
"One of the challenges faced in the design of any numerical computing library is the choice of how to handle operations between values of different types. This document outlines the thought process behind the promotion semantics used by JAX, summarized in [JAX Type Promotion Semantics](https://jax.readthedocs.io/en/latest/type_promotion.html)."
|
||||
"One of the challenges faced in the design of any numerical computing library is the choice of how to handle operations between values of different types. This document outlines the thought process behind the promotion semantics used by JAX, summarized in [JAX Type Promotion Semantics](https://docs.jax.dev/en/latest/type_promotion.html)."
|
||||
]
|
||||
},
|
||||
{
|
||||
@ -1335,7 +1335,7 @@
|
||||
"However, these advantages comes with a few tradeoffs:\n",
|
||||
"\n",
|
||||
"- mixed float/integer promotion is very prone to precision loss: for example, `int64` (with a maximum value of $9.2 \\times 10^{18}$) can be promoted to `float16` (with a maximum value of $6.5 \\times 10^4$), meaning most representable values will become `inf`.\n",
|
||||
"- as mentioned above, `f*` can no longer be thought of as a \"scalar type\", but as a different flavor of float64. In JAX's parlance, this is referred to as a [*weak type*](https://jax.readthedocs.io/en/latest/type_promotion.html#weakly-typed-values-in-jax), in that it is represented as 64-bit, but only weakly holds to this bit width in promotion with other values.\n",
|
||||
"- as mentioned above, `f*` can no longer be thought of as a \"scalar type\", but as a different flavor of float64. In JAX's parlance, this is referred to as a [*weak type*](https://docs.jax.dev/en/latest/type_promotion.html#weakly-typed-values-in-jax), in that it is represented as 64-bit, but only weakly holds to this bit width in promotion with other values.\n",
|
||||
"\n",
|
||||
"Note that also, this approach still leaves the `uint64` promotion question unanswered, although it is perhaps reasonable to close the lattice by connecting `u64` to `f*`."
|
||||
]
|
||||
@ -1413,7 +1413,7 @@
|
||||
"id": "o0-E2KWjYEXO"
|
||||
},
|
||||
"source": [
|
||||
"The behavior resulting from this choice is summarized in [JAX Type Promotion Semantics](https://jax.readthedocs.io/en/latest/type_promotion.html). Notably, aside from the inclusion of larger unsigned types (`u16`, `u32`, `u64`) and some details about the behavior of scalar/weak types (`i*`, `f*`, `c*`), this type promotion scheme turns out to be very close to that chosen by PyTorch.\n",
|
||||
"The behavior resulting from this choice is summarized in [JAX Type Promotion Semantics](https://docs.jax.dev/en/latest/type_promotion.html). Notably, aside from the inclusion of larger unsigned types (`u16`, `u32`, `u64`) and some details about the behavior of scalar/weak types (`i*`, `f*`, `c*`), this type promotion scheme turns out to be very close to that chosen by PyTorch.\n",
|
||||
"\n",
|
||||
"For those interested, the appendix below prints the full promotion tables used by NumPy, Tensorflow, PyTorch, and JAX."
|
||||
]
|
||||
@ -2883,7 +2883,7 @@
|
||||
"source": [
|
||||
"### JAX Type Promotion: `jax.numpy`\n",
|
||||
"\n",
|
||||
"`jax.numpy` follows type promotion rules laid out at https://jax.readthedocs.io/en/latest/type_promotion.html. Here we use `i*`, `f*`, `c*` to indicate both Python scalars and weakly-typed arrays."
|
||||
"`jax.numpy` follows type promotion rules laid out at https://docs.jax.dev/en/latest/type_promotion.html. Here we use `i*`, `f*`, `c*` to indicate both Python scalars and weakly-typed arrays."
|
||||
]
|
||||
},
|
||||
{
|
||||
|
@ -20,7 +20,7 @@ kernelspec:
|
||||
|
||||
*Jake VanderPlas, December 2021*
|
||||
|
||||
One of the challenges faced in the design of any numerical computing library is the choice of how to handle operations between values of different types. This document outlines the thought process behind the promotion semantics used by JAX, summarized in [JAX Type Promotion Semantics](https://jax.readthedocs.io/en/latest/type_promotion.html).
|
||||
One of the challenges faced in the design of any numerical computing library is the choice of how to handle operations between values of different types. This document outlines the thought process behind the promotion semantics used by JAX, summarized in [JAX Type Promotion Semantics](https://docs.jax.dev/en/latest/type_promotion.html).
|
||||
|
||||
+++ {"id": "Rod6OOyUVbQ8"}
|
||||
|
||||
@ -680,7 +680,7 @@ This is important because `f16` and `bf16` are not comparable because they utili
|
||||
However, these advantages comes with a few tradeoffs:
|
||||
|
||||
- mixed float/integer promotion is very prone to precision loss: for example, `int64` (with a maximum value of $9.2 \times 10^{18}$) can be promoted to `float16` (with a maximum value of $6.5 \times 10^4$), meaning most representable values will become `inf`.
|
||||
- as mentioned above, `f*` can no longer be thought of as a "scalar type", but as a different flavor of float64. In JAX's parlance, this is referred to as a [*weak type*](https://jax.readthedocs.io/en/latest/type_promotion.html#weakly-typed-values-in-jax), in that it is represented as 64-bit, but only weakly holds to this bit width in promotion with other values.
|
||||
- as mentioned above, `f*` can no longer be thought of as a "scalar type", but as a different flavor of float64. In JAX's parlance, this is referred to as a [*weak type*](https://docs.jax.dev/en/latest/type_promotion.html#weakly-typed-values-in-jax), in that it is represented as 64-bit, but only weakly holds to this bit width in promotion with other values.
|
||||
|
||||
Note that also, this approach still leaves the `uint64` promotion question unanswered, although it is perhaps reasonable to close the lattice by connecting `u64` to `f*`.
|
||||
|
||||
@ -730,7 +730,7 @@ nx.draw(graph, with_labels=True, node_size=1500, node_color='lightgray', pos=pos
|
||||
|
||||
+++ {"id": "o0-E2KWjYEXO"}
|
||||
|
||||
The behavior resulting from this choice is summarized in [JAX Type Promotion Semantics](https://jax.readthedocs.io/en/latest/type_promotion.html). Notably, aside from the inclusion of larger unsigned types (`u16`, `u32`, `u64`) and some details about the behavior of scalar/weak types (`i*`, `f*`, `c*`), this type promotion scheme turns out to be very close to that chosen by PyTorch.
|
||||
The behavior resulting from this choice is summarized in [JAX Type Promotion Semantics](https://docs.jax.dev/en/latest/type_promotion.html). Notably, aside from the inclusion of larger unsigned types (`u16`, `u32`, `u64`) and some details about the behavior of scalar/weak types (`i*`, `f*`, `c*`), this type promotion scheme turns out to be very close to that chosen by PyTorch.
|
||||
|
||||
For those interested, the appendix below prints the full promotion tables used by NumPy, Tensorflow, PyTorch, and JAX.
|
||||
|
||||
@ -900,7 +900,7 @@ display.HTML(table.to_html())
|
||||
|
||||
### JAX Type Promotion: `jax.numpy`
|
||||
|
||||
`jax.numpy` follows type promotion rules laid out at https://jax.readthedocs.io/en/latest/type_promotion.html. Here we use `i*`, `f*`, `c*` to indicate both Python scalars and weakly-typed arrays.
|
||||
`jax.numpy` follows type promotion rules laid out at https://docs.jax.dev/en/latest/type_promotion.html. Here we use `i*`, `f*`, `c*` to indicate both Python scalars and weakly-typed arrays.
|
||||
|
||||
```{code-cell}
|
||||
:cellView: form
|
||||
|
@ -55,7 +55,7 @@ The {ref}`jax-internals-jaxpr` section of the documentation provides more inform
|
||||
|
||||
Importantly, notice that the jaxpr does not capture the side-effect present in the function: there is nothing in it corresponding to `global_list.append(x)`.
|
||||
This is a feature, not a bug: JAX transformations are designed to understand side-effect-free (a.k.a. functionally pure) code.
|
||||
If *pure function* and *side-effect* are unfamiliar terms, this is explained in a little more detail in [🔪 JAX - The Sharp Bits 🔪: Pure Functions](https://jax.readthedocs.io/en/latest/notebooks/Common_Gotchas_in_JAX.html#pure-functions).
|
||||
If *pure function* and *side-effect* are unfamiliar terms, this is explained in a little more detail in [🔪 JAX - The Sharp Bits 🔪: Pure Functions](https:docs.jax.devio/en/latest/notebooks/Common_Gotchas_in_JAX.html#pure-functions).
|
||||
|
||||
Impure functions are dangerous because under JAX transformations they are likely not to behave as intended; they might fail silently, or produce surprising downstream errors like leaked Tracers.
|
||||
Moreover, JAX often can't detect when side effects are present.
|
||||
|
@ -346,7 +346,7 @@
|
||||
"evalue": "ignored",
|
||||
"output_type": "error",
|
||||
"traceback": [
|
||||
"\u001b[0;31mTypeError\u001b[0m\u001b[0;31m:\u001b[0m '<class 'jaxlib.xla_extension.ArrayImpl'>' object does not support item assignment. JAX arrays are immutable. Instead of ``x[idx] = y``, use ``x = x.at[idx].set(y)`` or another .at[] method: https://jax.readthedocs.io/en/latest/_autosummary/jax.numpy.ndarray.at.html\n"
|
||||
"\u001b[0;31mTypeError\u001b[0m\u001b[0;31m:\u001b[0m '<class 'jaxlib.xla_extension.ArrayImpl'>' object does not support item assignment. JAX arrays are immutable. Instead of ``x[idx] = y``, use ``x = x.at[idx].set(y)`` or another .at[] method: https://docs.jax.dev/en/latest/_autosummary/jax.numpy.ndarray.at.html\n"
|
||||
]
|
||||
}
|
||||
],
|
||||
@ -365,7 +365,7 @@
|
||||
"source": [
|
||||
"Allowing mutation of variables in-place makes program analysis and transformation difficult. JAX requires that programs are pure functions.\n",
|
||||
"\n",
|
||||
"Instead, JAX offers a _functional_ array update using the [`.at` property on JAX arrays](https://jax.readthedocs.io/en/latest/_autosummary/jax.numpy.ndarray.at.html#jax.numpy.ndarray.at)."
|
||||
"Instead, JAX offers a _functional_ array update using the [`.at` property on JAX arrays](https://docs.jax.dev/en/latest/_autosummary/jax.numpy.ndarray.at.html#jax.numpy.ndarray.at)."
|
||||
]
|
||||
},
|
||||
{
|
||||
@ -521,7 +521,7 @@
|
||||
"id": "sTjJ3WuaDyqU"
|
||||
},
|
||||
"source": [
|
||||
"For more details on indexed array updates, see the [documentation for the `.at` property](https://jax.readthedocs.io/en/latest/_autosummary/jax.numpy.ndarray.at.html#jax.numpy.ndarray.at)."
|
||||
"For more details on indexed array updates, see the [documentation for the `.at` property](https://docs.jax.dev/en/latest/_autosummary/jax.numpy.ndarray.at.html#jax.numpy.ndarray.at)."
|
||||
]
|
||||
},
|
||||
{
|
||||
@ -604,7 +604,7 @@
|
||||
"id": "NAcXJNAcDi_v"
|
||||
},
|
||||
"source": [
|
||||
"If you would like finer-grained control over the behavior for out-of-bound indices, you can use the optional parameters of [`ndarray.at`](https://jax.readthedocs.io/en/latest/_autosummary/jax.numpy.ndarray.at.html); for example:"
|
||||
"If you would like finer-grained control over the behavior for out-of-bound indices, you can use the optional parameters of [`ndarray.at`](https://docs.jax.dev/en/latest/_autosummary/jax.numpy.ndarray.at.html); for example:"
|
||||
]
|
||||
},
|
||||
{
|
||||
@ -971,7 +971,7 @@
|
||||
"evalue": "ignored",
|
||||
"output_type": "error",
|
||||
"traceback": [
|
||||
"\u001b[0;31mNonConcreteBooleanIndexError\u001b[0m\u001b[0;31m:\u001b[0m Array boolean indices must be concrete; got ShapedArray(bool[5])\n\nSee https://jax.readthedocs.io/en/latest/errors.html#jax.errors.NonConcreteBooleanIndexError\n"
|
||||
"\u001b[0;31mNonConcreteBooleanIndexError\u001b[0m\u001b[0;31m:\u001b[0m Array boolean indices must be concrete; got ShapedArray(bool[5])\n\nSee https://docs.jax.dev/en/latest/errors.html#jax.errors.NonConcreteBooleanIndexError\n"
|
||||
]
|
||||
}
|
||||
],
|
||||
@ -1296,7 +1296,7 @@
|
||||
"While `jax.numpy` makes every attempt to replicate the behavior of numpy's API, there do exist corner cases where the behaviors differ.\n",
|
||||
"Many such cases are discussed in detail in the sections above; here we list several other known places where the APIs diverge.\n",
|
||||
"\n",
|
||||
"- For binary operations, JAX's type promotion rules differ somewhat from those used by NumPy. See [Type Promotion Semantics](https://jax.readthedocs.io/en/latest/type_promotion.html) for more details.\n",
|
||||
"- For binary operations, JAX's type promotion rules differ somewhat from those used by NumPy. See [Type Promotion Semantics](https://docs.jax.dev/en/latest/type_promotion.html) for more details.\n",
|
||||
"- When performing unsafe type casts (i.e. casts in which the target dtype cannot represent the input value), JAX's behavior may be backend dependent, and in general may diverge from NumPy's behavior. Numpy allows control over the result in these scenarios via the `casting` argument (see [`np.ndarray.astype`](https://numpy.org/devdocs/reference/generated/numpy.ndarray.astype.html)); JAX does not provide any such configuration, instead directly inheriting the behavior of [XLA:ConvertElementType](https://www.tensorflow.org/xla/operation_semantics#convertelementtype).\n",
|
||||
"\n",
|
||||
" Here is an example of an unsafe cast with differing results between NumPy and JAX:\n",
|
||||
|
@ -201,7 +201,7 @@ jax_array[1, :] = 1.0
|
||||
|
||||
Allowing mutation of variables in-place makes program analysis and transformation difficult. JAX requires that programs are pure functions.
|
||||
|
||||
Instead, JAX offers a _functional_ array update using the [`.at` property on JAX arrays](https://jax.readthedocs.io/en/latest/_autosummary/jax.numpy.ndarray.at.html#jax.numpy.ndarray.at).
|
||||
Instead, JAX offers a _functional_ array update using the [`.at` property on JAX arrays](https://docs.jax.dev/en/latest/_autosummary/jax.numpy.ndarray.at.html#jax.numpy.ndarray.at).
|
||||
|
||||
+++ {"id": "hfloZ1QXCS_J"}
|
||||
|
||||
@ -261,7 +261,7 @@ print(new_jax_array)
|
||||
|
||||
+++ {"id": "sTjJ3WuaDyqU"}
|
||||
|
||||
For more details on indexed array updates, see the [documentation for the `.at` property](https://jax.readthedocs.io/en/latest/_autosummary/jax.numpy.ndarray.at.html#jax.numpy.ndarray.at).
|
||||
For more details on indexed array updates, see the [documentation for the `.at` property](https://docs.jax.dev/en/latest/_autosummary/jax.numpy.ndarray.at.html#jax.numpy.ndarray.at).
|
||||
|
||||
+++ {"id": "oZ_jE2WAypdL"}
|
||||
|
||||
@ -292,7 +292,7 @@ jnp.arange(10)[11]
|
||||
|
||||
+++ {"id": "NAcXJNAcDi_v"}
|
||||
|
||||
If you would like finer-grained control over the behavior for out-of-bound indices, you can use the optional parameters of [`ndarray.at`](https://jax.readthedocs.io/en/latest/_autosummary/jax.numpy.ndarray.at.html); for example:
|
||||
If you would like finer-grained control over the behavior for out-of-bound indices, you can use the optional parameters of [`ndarray.at`](https://docs.jax.dev/en/latest/_autosummary/jax.numpy.ndarray.at.html); for example:
|
||||
|
||||
```{code-cell} ipython3
|
||||
:id: -0-MaFddO-xy
|
||||
@ -664,7 +664,7 @@ x.dtype # --> dtype('float64')
|
||||
While `jax.numpy` makes every attempt to replicate the behavior of numpy's API, there do exist corner cases where the behaviors differ.
|
||||
Many such cases are discussed in detail in the sections above; here we list several other known places where the APIs diverge.
|
||||
|
||||
- For binary operations, JAX's type promotion rules differ somewhat from those used by NumPy. See [Type Promotion Semantics](https://jax.readthedocs.io/en/latest/type_promotion.html) for more details.
|
||||
- For binary operations, JAX's type promotion rules differ somewhat from those used by NumPy. See [Type Promotion Semantics](https://docs.jax.dev/en/latest/type_promotion.html) for more details.
|
||||
- When performing unsafe type casts (i.e. casts in which the target dtype cannot represent the input value), JAX's behavior may be backend dependent, and in general may diverge from NumPy's behavior. Numpy allows control over the result in these scenarios via the `casting` argument (see [`np.ndarray.astype`](https://numpy.org/devdocs/reference/generated/numpy.ndarray.astype.html)); JAX does not provide any such configuration, instead directly inheriting the behavior of [XLA:ConvertElementType](https://www.tensorflow.org/xla/operation_semantics#convertelementtype).
|
||||
|
||||
Here is an example of an unsafe cast with differing results between NumPy and JAX:
|
||||
|
@ -17,9 +17,9 @@
|
||||
"1. using `jax.custom_jvp` and `jax.custom_vjp` to define custom differentiation rules for Python functions that are already JAX-transformable; and\n",
|
||||
"2. defining new `core.Primitive` instances along with all their transformation rules, for example to call into functions from other systems like solvers, simulators, or general numerical computing systems.\n",
|
||||
"\n",
|
||||
"This notebook is about #1. To read instead about #2, see the [notebook on adding primitives](https://jax.readthedocs.io/en/latest/notebooks/How_JAX_primitives_work.html).\n",
|
||||
"This notebook is about #1. To read instead about #2, see the [notebook on adding primitives](https://docs.jax.dev/en/latest/notebooks/How_JAX_primitives_work.html).\n",
|
||||
"\n",
|
||||
"For an introduction to JAX's automatic differentiation API, see [The Autodiff Cookbook](https://jax.readthedocs.io/en/latest/notebooks/autodiff_cookbook.html). This notebook assumes some familiarity with [jax.jvp](https://jax.readthedocs.io/en/latest/jax.html#jax.jvp) and [jax.grad](https://jax.readthedocs.io/en/latest/jax.html#jax.grad), and the mathematical meaning of JVPs and VJPs."
|
||||
"For an introduction to JAX's automatic differentiation API, see [The Autodiff Cookbook](https://docs.jax.dev/en/latest/notebooks/autodiff_cookbook.html). This notebook assumes some familiarity with [jax.jvp](https://docs.jax.dev/en/latest/jax.html#jax.jvp) and [jax.grad](https://docs.jax.dev/en/latest/jax.html#jax.grad), and the mathematical meaning of JVPs and VJPs."
|
||||
]
|
||||
},
|
||||
{
|
||||
@ -2035,7 +2035,7 @@
|
||||
"source": [
|
||||
"### Working with `list` / `tuple` / `dict` containers (and other pytrees)\n",
|
||||
"\n",
|
||||
"You should expect standard Python containers like lists, tuples, namedtuples, and dicts to just work, along with nested versions of those. In general, any [pytrees](https://jax.readthedocs.io/en/latest/pytrees.html) are permissible, so long as their structures are consistent according to the type constraints. \n",
|
||||
"You should expect standard Python containers like lists, tuples, namedtuples, and dicts to just work, along with nested versions of those. In general, any [pytrees](https://docs.jax.dev/en/latest/pytrees.html) are permissible, so long as their structures are consistent according to the type constraints. \n",
|
||||
"\n",
|
||||
"Here's a contrived example with `jax.custom_jvp`:"
|
||||
]
|
||||
|
@ -24,9 +24,9 @@ There are two ways to define differentiation rules in JAX:
|
||||
1. using `jax.custom_jvp` and `jax.custom_vjp` to define custom differentiation rules for Python functions that are already JAX-transformable; and
|
||||
2. defining new `core.Primitive` instances along with all their transformation rules, for example to call into functions from other systems like solvers, simulators, or general numerical computing systems.
|
||||
|
||||
This notebook is about #1. To read instead about #2, see the [notebook on adding primitives](https://jax.readthedocs.io/en/latest/notebooks/How_JAX_primitives_work.html).
|
||||
This notebook is about #1. To read instead about #2, see the [notebook on adding primitives](https://docs.jax.dev/en/latest/notebooks/How_JAX_primitives_work.html).
|
||||
|
||||
For an introduction to JAX's automatic differentiation API, see [The Autodiff Cookbook](https://jax.readthedocs.io/en/latest/notebooks/autodiff_cookbook.html). This notebook assumes some familiarity with [jax.jvp](https://jax.readthedocs.io/en/latest/jax.html#jax.jvp) and [jax.grad](https://jax.readthedocs.io/en/latest/jax.html#jax.grad), and the mathematical meaning of JVPs and VJPs.
|
||||
For an introduction to JAX's automatic differentiation API, see [The Autodiff Cookbook](https://docs.jax.dev/en/latest/notebooks/autodiff_cookbook.html). This notebook assumes some familiarity with [jax.jvp](https://docs.jax.dev/en/latest/jax.html#jax.jvp) and [jax.grad](https://docs.jax.dev/en/latest/jax.html#jax.grad), and the mathematical meaning of JVPs and VJPs.
|
||||
|
||||
+++ {"id": "9Fg3NFNY-2RY"}
|
||||
|
||||
@ -1048,7 +1048,7 @@ Array(-0.91113025, dtype=float32)
|
||||
|
||||
### Working with `list` / `tuple` / `dict` containers (and other pytrees)
|
||||
|
||||
You should expect standard Python containers like lists, tuples, namedtuples, and dicts to just work, along with nested versions of those. In general, any [pytrees](https://jax.readthedocs.io/en/latest/pytrees.html) are permissible, so long as their structures are consistent according to the type constraints.
|
||||
You should expect standard Python containers like lists, tuples, namedtuples, and dicts to just work, along with nested versions of those. In general, any [pytrees](https://docs.jax.dev/en/latest/pytrees.html) are permissible, so long as their structures are consistent according to the type constraints.
|
||||
|
||||
Here's a contrived example with `jax.custom_jvp`:
|
||||
|
||||
|
@ -1276,7 +1276,7 @@
|
||||
"id": "3qfPjJdhgerc"
|
||||
},
|
||||
"source": [
|
||||
"So computation follows data placement: when we explicitly shard data with `jax.device_put`, and apply functions to that data, the compiler attempts to parallelize the computation and decide the output sharding. This policy for sharded data is a generalization of [JAX's policy of following explicit device placement](https://jax.readthedocs.io/en/latest/faq.html#controlling-data-and-computation-placement-on-devices)."
|
||||
"So computation follows data placement: when we explicitly shard data with `jax.device_put`, and apply functions to that data, the compiler attempts to parallelize the computation and decide the output sharding. This policy for sharded data is a generalization of [JAX's policy of following explicit device placement](https://docs.jax.dev/en/latest/faq.html#controlling-data-and-computation-placement-on-devices)."
|
||||
]
|
||||
},
|
||||
{
|
||||
@ -1382,7 +1382,7 @@
|
||||
"id": "6ZYcK8eXrn0p"
|
||||
},
|
||||
"source": [
|
||||
"We say arrays that have been explicitly placed or sharded with `jax.device_put` are _committed_ to their device(s), and so won't be automatically moved. See the [device placement FAQ](https://jax.readthedocs.io/en/latest/faq.html#controlling-data-and-computation-placement-on-devices) for more information.\n",
|
||||
"We say arrays that have been explicitly placed or sharded with `jax.device_put` are _committed_ to their device(s), and so won't be automatically moved. See the [device placement FAQ](https://docs.jax.dev/en/latest/faq.html#controlling-data-and-computation-placement-on-devices) for more information.\n",
|
||||
"\n",
|
||||
"When arrays are _not_ explicitly placed or sharded with `jax.device_put`, they are placed _uncommitted_ on the default device.\n",
|
||||
"Unlike committed arrays, uncommitted arrays can be moved and resharded automatically: that is, uncommitted arrays can be arguments to a computation even if other arguments are explicitly placed on different devices.\n",
|
||||
@ -2339,7 +2339,7 @@
|
||||
"source": [
|
||||
"### Generating random numbers\n",
|
||||
"\n",
|
||||
"JAX comes with a functional, deterministic [random number generator](https://jax.readthedocs.io/en/latest/jep/263-prng.html). It underlies the various sampling functions in the [`jax.random` module](https://jax.readthedocs.io/en/latest/jax.random.html), such as `jax.random.uniform`.\n",
|
||||
"JAX comes with a functional, deterministic [random number generator](https://docs.jax.dev/en/latest/jep/263-prng.html). It underlies the various sampling functions in the [`jax.random` module](https://docs.jax.dev/en/latest/jax.random.html), such as `jax.random.uniform`.\n",
|
||||
"\n",
|
||||
"JAX's random numbers are produced by a counter-based PRNG, so in principle, random number generation should be a pure map over counter values. A pure map is a trivially partitionable operation in principle. It should require no cross-device communication, nor any redundant computation across devices.\n",
|
||||
"\n",
|
||||
|
@ -427,7 +427,7 @@ jax.debug.visualize_array_sharding(w_copy)
|
||||
|
||||
+++ {"id": "3qfPjJdhgerc"}
|
||||
|
||||
So computation follows data placement: when we explicitly shard data with `jax.device_put`, and apply functions to that data, the compiler attempts to parallelize the computation and decide the output sharding. This policy for sharded data is a generalization of [JAX's policy of following explicit device placement](https://jax.readthedocs.io/en/latest/faq.html#controlling-data-and-computation-placement-on-devices).
|
||||
So computation follows data placement: when we explicitly shard data with `jax.device_put`, and apply functions to that data, the compiler attempts to parallelize the computation and decide the output sharding. This policy for sharded data is a generalization of [JAX's policy of following explicit device placement](https://docs.jax.dev/en/latest/faq.html#controlling-data-and-computation-placement-on-devices).
|
||||
|
||||
+++ {"id": "QRB95LaWuT80"}
|
||||
|
||||
@ -484,7 +484,7 @@ except ValueError as e: print_exception(e)
|
||||
|
||||
+++ {"id": "6ZYcK8eXrn0p"}
|
||||
|
||||
We say arrays that have been explicitly placed or sharded with `jax.device_put` are _committed_ to their device(s), and so won't be automatically moved. See the [device placement FAQ](https://jax.readthedocs.io/en/latest/faq.html#controlling-data-and-computation-placement-on-devices) for more information.
|
||||
We say arrays that have been explicitly placed or sharded with `jax.device_put` are _committed_ to their device(s), and so won't be automatically moved. See the [device placement FAQ](https://docs.jax.dev/en/latest/faq.html#controlling-data-and-computation-placement-on-devices) for more information.
|
||||
|
||||
When arrays are _not_ explicitly placed or sharded with `jax.device_put`, they are placed _uncommitted_ on the default device.
|
||||
Unlike committed arrays, uncommitted arrays can be moved and resharded automatically: that is, uncommitted arrays can be arguments to a computation even if other arguments are explicitly placed on different devices.
|
||||
@ -854,7 +854,7 @@ outputId: 479c4d81-cb0b-40a5-89ba-394c10dc3297
|
||||
|
||||
### Generating random numbers
|
||||
|
||||
JAX comes with a functional, deterministic [random number generator](https://jax.readthedocs.io/en/latest/jep/263-prng.html). It underlies the various sampling functions in the [`jax.random` module](https://jax.readthedocs.io/en/latest/jax.random.html), such as `jax.random.uniform`.
|
||||
JAX comes with a functional, deterministic [random number generator](https://docs.jax.dev/en/latest/jep/263-prng.html). It underlies the various sampling functions in the [`jax.random` module](https://docs.jax.dev/en/latest/jax.random.html), such as `jax.random.uniform`.
|
||||
|
||||
JAX's random numbers are produced by a counter-based PRNG, so in principle, random number generation should be a pure map over counter values. A pure map is a trivially partitionable operation in principle. It should require no cross-device communication, nor any redundant computation across devices.
|
||||
|
||||
|
@ -1,2 +1,2 @@
|
||||
For instructions on how to change and test notebooks, see
|
||||
[Update Documentation](https://jax.readthedocs.io/en/latest/developer.html#update-documentation).
|
||||
[Update Documentation](https://docs.jax.dev/en/latest/developer.html#update-documentation).
|
||||
|
@ -24,7 +24,7 @@
|
||||
"\n",
|
||||
"Here we show how to add your own function transformations to the system, by writing a custom Jaxpr interpreter. And we'll get composability with all the other transformations for free.\n",
|
||||
"\n",
|
||||
"**This example uses internal JAX APIs, which may break at any time. Anything not in [the API Documentation](https://jax.readthedocs.io/en/latest/jax.html) should be assumed internal.**"
|
||||
"**This example uses internal JAX APIs, which may break at any time. Anything not in [the API Documentation](https://docs.jax.dev/en/latest/jax.html) should be assumed internal.**"
|
||||
]
|
||||
},
|
||||
{
|
||||
|
@ -27,7 +27,7 @@ etc.) that enable writing concise, accelerated code.
|
||||
|
||||
Here we show how to add your own function transformations to the system, by writing a custom Jaxpr interpreter. And we'll get composability with all the other transformations for free.
|
||||
|
||||
**This example uses internal JAX APIs, which may break at any time. Anything not in [the API Documentation](https://jax.readthedocs.io/en/latest/jax.html) should be assumed internal.**
|
||||
**This example uses internal JAX APIs, which may break at any time. Anything not in [the API Documentation](https://docs.jax.dev/en/latest/jax.html) should be assumed internal.**
|
||||
|
||||
```{code-cell} ipython3
|
||||
:id: s27RDKvKXFL8
|
||||
|
@ -348,7 +348,7 @@
|
||||
"source": [
|
||||
"### Let's think step by step\n",
|
||||
"\n",
|
||||
"You might want to first (re)read [the Autodiff Cookbook Part 1](https://jax.readthedocs.io/en/latest/notebooks/autodiff_cookbook.html)."
|
||||
"You might want to first (re)read [the Autodiff Cookbook Part 1](https://docs.jax.dev/en/latest/notebooks/autodiff_cookbook.html)."
|
||||
]
|
||||
},
|
||||
{
|
||||
|
@ -156,7 +156,7 @@ print_fwd_bwd(f3, W1, W2, W3, x)
|
||||
|
||||
### Let's think step by step
|
||||
|
||||
You might want to first (re)read [the Autodiff Cookbook Part 1](https://jax.readthedocs.io/en/latest/notebooks/autodiff_cookbook.html).
|
||||
You might want to first (re)read [the Autodiff Cookbook Part 1](https://docs.jax.dev/en/latest/notebooks/autodiff_cookbook.html).
|
||||
|
||||
+++ {"id": "VMfwm_yinvoZ"}
|
||||
|
||||
|
@ -46,7 +46,7 @@
|
||||
"\n",
|
||||
"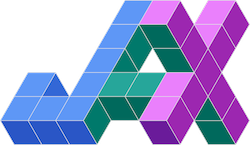\n",
|
||||
"\n",
|
||||
"Let's combine everything we showed in the [quickstart](https://jax.readthedocs.io/en/latest/quickstart.html) to train a simple neural network. We will first specify and train a simple MLP on MNIST using JAX for the computation. We will use `tensorflow/datasets` data loading API to load images and labels (because it's pretty great, and the world doesn't need yet another data loading library :P).\n",
|
||||
"Let's combine everything we showed in the [quickstart](https://docs.jax.dev/en/latest/quickstart.html) to train a simple neural network. We will first specify and train a simple MLP on MNIST using JAX for the computation. We will use `tensorflow/datasets` data loading API to load images and labels (because it's pretty great, and the world doesn't need yet another data loading library :P).\n",
|
||||
"\n",
|
||||
"Of course, you can use JAX with any API that is compatible with NumPy to make specifying the model a bit more plug-and-play. Here, just for explanatory purposes, we won't use any neural network libraries or special APIs for building our model."
|
||||
]
|
||||
|
@ -44,7 +44,7 @@ _Forked from_ `neural_network_and_data_loading.ipynb`
|
||||
|
||||
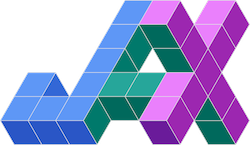
|
||||
|
||||
Let's combine everything we showed in the [quickstart](https://jax.readthedocs.io/en/latest/quickstart.html) to train a simple neural network. We will first specify and train a simple MLP on MNIST using JAX for the computation. We will use `tensorflow/datasets` data loading API to load images and labels (because it's pretty great, and the world doesn't need yet another data loading library :P).
|
||||
Let's combine everything we showed in the [quickstart](https://docs.jax.dev/en/latest/quickstart.html) to train a simple neural network. We will first specify and train a simple MLP on MNIST using JAX for the computation. We will use `tensorflow/datasets` data loading API to load images and labels (because it's pretty great, and the world doesn't need yet another data loading library :P).
|
||||
|
||||
Of course, you can use JAX with any API that is compatible with NumPy to make specifying the model a bit more plug-and-play. Here, just for explanatory purposes, we won't use any neural network libraries or special APIs for building our model.
|
||||
|
||||
|
@ -13,9 +13,9 @@
|
||||
"\n",
|
||||
"`shard_map` is a single-program multiple-data (SPMD) multi-device parallelism API to map a function over shards of data. Mapped function applications, or _instances_, communicate with each other via explicit collective communication operations.\n",
|
||||
"\n",
|
||||
"`shard_map` is complementary to, and composable with, the automatic compiler-based parallelization built into `jit`. With `jit` you write code as if for a single device, and [the compiler can automatically partition computation over multiple devices](https://jax.readthedocs.io/en/latest/notebooks/Distributed_arrays_and_automatic_parallelization.html), generating per-device code and communication collectives behind the scenes. With `shard_map` you take control, writing your own partitioned code and explicit collectives. Or you can do a bit of both: take manual control across groups of devices while leaving within-group device partitioning up to the compiler. The two approaches can be mixed, matched, and composed as needed.\n",
|
||||
"`shard_map` is complementary to, and composable with, the automatic compiler-based parallelization built into `jit`. With `jit` you write code as if for a single device, and [the compiler can automatically partition computation over multiple devices](https://docs.jax.dev/en/latest/notebooks/Distributed_arrays_and_automatic_parallelization.html), generating per-device code and communication collectives behind the scenes. With `shard_map` you take control, writing your own partitioned code and explicit collectives. Or you can do a bit of both: take manual control across groups of devices while leaving within-group device partitioning up to the compiler. The two approaches can be mixed, matched, and composed as needed.\n",
|
||||
"\n",
|
||||
"If you're familiar with `pmap`, think of `shard_map` as an evolution. It's more expressive, performant, and composable with other JAX APIs. It even works eagerly, for easier debugging! (For more, see [a detailed comparison to `pmap`.](https://jax.readthedocs.io/en/latest/jep/14273-shard-map.html#why-don-t-pmap-or-xmap-already-solve-this))\n",
|
||||
"If you're familiar with `pmap`, think of `shard_map` as an evolution. It's more expressive, performant, and composable with other JAX APIs. It even works eagerly, for easier debugging! (For more, see [a detailed comparison to `pmap`.](https://docs.jax.dev/en/latest/jep/14273-shard-map.html#why-don-t-pmap-or-xmap-already-solve-this))\n",
|
||||
"\n",
|
||||
"By reading this tutorial, you'll learn how to use `shard_map` to get full control over your multi-device code. You'll see in detail how it composes with `jax.jit`'s automatic parallelization and `jax.grad`'s automatic differentiation. We'll also give some basic examples of neural network parallelization strategies.\n",
|
||||
"\n",
|
||||
@ -499,7 +499,7 @@
|
||||
"* `mesh` encodes devices arranged in an array and with associated axis names, just like it does for `sharding.NamedSharding`;\n",
|
||||
"* `in_specs` and `out_specs` are `PartitionSpec`s which can affinely mention axis names from `mesh` to express slicing/unconcatenation and concatenation of inputs and outputs, respectively, with unmentioned names corresponding to replication and untiling (assert-replicated-so-give-me-one-copy), respectively;\n",
|
||||
"* `auto` is an optional set of axis names corresponding to the subset of names of `mesh` to treat automatically in the body, as in the caller, rather than manually;\n",
|
||||
"* `check_rep` is an optional boolean indicating whether to check statically for any replication errors in `out_specs`, and also whether to enable a related automatic differentiation optimization (see [JEP](https://jax.readthedocs.io/en/latest/jep/17111-shmap-transpose.html)).\n",
|
||||
"* `check_rep` is an optional boolean indicating whether to check statically for any replication errors in `out_specs`, and also whether to enable a related automatic differentiation optimization (see [JEP](https://docs.jax.dev/en/latest/jep/17111-shmap-transpose.html)).\n",
|
||||
"\n",
|
||||
"The shapes of the arguments passed to `f` have the same ranks as the arguments\n",
|
||||
"passed to `shard_map`-of-`f`, and the shape of an argument to `f` is computed\n",
|
||||
@ -1520,7 +1520,7 @@
|
||||
"source": [
|
||||
"Compare these examples with the purely [automatic partitioning examples in the\n",
|
||||
"\"Distributed arrays and automatic partitioning\"\n",
|
||||
"doc](https://jax.readthedocs.io/en/latest/notebooks/Distributed_arrays_and_automatic_parallelization.html).\n",
|
||||
"doc](https://docs.jax.dev/en/latest/notebooks/Distributed_arrays_and_automatic_parallelization.html).\n",
|
||||
"While in those automatic partitioning examples we don't need to edit the model\n",
|
||||
"functions to use different parallelization strategies, with `shard_map` we\n",
|
||||
"often do.\n",
|
||||
@ -1626,7 +1626,7 @@
|
||||
"parameters from the forward pass for use on the backward pass. Instead, we want\n",
|
||||
"to gather them again on the backward pass. We can express that by using\n",
|
||||
"`jax.remat` with a [custom\n",
|
||||
"policy](https://jax.readthedocs.io/en/latest/notebooks/autodiff_remat.html#custom-policies-for-what-s-saveable)\n",
|
||||
"policy](https://docs.jax.dev/en/latest/notebooks/autodiff_remat.html#custom-policies-for-what-s-saveable)\n",
|
||||
"(or a `custom_vjp`), though XLA typically does that rematerialization\n",
|
||||
"automatically.\n",
|
||||
"\n",
|
||||
|
@ -22,9 +22,9 @@ kernelspec:
|
||||
|
||||
`shard_map` is a single-program multiple-data (SPMD) multi-device parallelism API to map a function over shards of data. Mapped function applications, or _instances_, communicate with each other via explicit collective communication operations.
|
||||
|
||||
`shard_map` is complementary to, and composable with, the automatic compiler-based parallelization built into `jit`. With `jit` you write code as if for a single device, and [the compiler can automatically partition computation over multiple devices](https://jax.readthedocs.io/en/latest/notebooks/Distributed_arrays_and_automatic_parallelization.html), generating per-device code and communication collectives behind the scenes. With `shard_map` you take control, writing your own partitioned code and explicit collectives. Or you can do a bit of both: take manual control across groups of devices while leaving within-group device partitioning up to the compiler. The two approaches can be mixed, matched, and composed as needed.
|
||||
`shard_map` is complementary to, and composable with, the automatic compiler-based parallelization built into `jit`. With `jit` you write code as if for a single device, and [the compiler can automatically partition computation over multiple devices](https://docs.jax.dev/en/latest/notebooks/Distributed_arrays_and_automatic_parallelization.html), generating per-device code and communication collectives behind the scenes. With `shard_map` you take control, writing your own partitioned code and explicit collectives. Or you can do a bit of both: take manual control across groups of devices while leaving within-group device partitioning up to the compiler. The two approaches can be mixed, matched, and composed as needed.
|
||||
|
||||
If you're familiar with `pmap`, think of `shard_map` as an evolution. It's more expressive, performant, and composable with other JAX APIs. It even works eagerly, for easier debugging! (For more, see [a detailed comparison to `pmap`.](https://jax.readthedocs.io/en/latest/jep/14273-shard-map.html#why-don-t-pmap-or-xmap-already-solve-this))
|
||||
If you're familiar with `pmap`, think of `shard_map` as an evolution. It's more expressive, performant, and composable with other JAX APIs. It even works eagerly, for easier debugging! (For more, see [a detailed comparison to `pmap`.](https://docs.jax.dev/en/latest/jep/14273-shard-map.html#why-don-t-pmap-or-xmap-already-solve-this))
|
||||
|
||||
By reading this tutorial, you'll learn how to use `shard_map` to get full control over your multi-device code. You'll see in detail how it composes with `jax.jit`'s automatic parallelization and `jax.grad`'s automatic differentiation. We'll also give some basic examples of neural network parallelization strategies.
|
||||
|
||||
@ -346,7 +346,7 @@ where:
|
||||
* `mesh` encodes devices arranged in an array and with associated axis names, just like it does for `sharding.NamedSharding`;
|
||||
* `in_specs` and `out_specs` are `PartitionSpec`s which can affinely mention axis names from `mesh` to express slicing/unconcatenation and concatenation of inputs and outputs, respectively, with unmentioned names corresponding to replication and untiling (assert-replicated-so-give-me-one-copy), respectively;
|
||||
* `auto` is an optional set of axis names corresponding to the subset of names of `mesh` to treat automatically in the body, as in the caller, rather than manually;
|
||||
* `check_rep` is an optional boolean indicating whether to check statically for any replication errors in `out_specs`, and also whether to enable a related automatic differentiation optimization (see [JEP](https://jax.readthedocs.io/en/latest/jep/17111-shmap-transpose.html)).
|
||||
* `check_rep` is an optional boolean indicating whether to check statically for any replication errors in `out_specs`, and also whether to enable a related automatic differentiation optimization (see [JEP](https://docs.jax.dev/en/latest/jep/17111-shmap-transpose.html)).
|
||||
|
||||
The shapes of the arguments passed to `f` have the same ranks as the arguments
|
||||
passed to `shard_map`-of-`f`, and the shape of an argument to `f` is computed
|
||||
@ -1061,7 +1061,7 @@ params, batch = init(jax.random.key(0), layer_sizes, batch_size)
|
||||
|
||||
Compare these examples with the purely [automatic partitioning examples in the
|
||||
"Distributed arrays and automatic partitioning"
|
||||
doc](https://jax.readthedocs.io/en/latest/notebooks/Distributed_arrays_and_automatic_parallelization.html).
|
||||
doc](https://docs.jax.dev/en/latest/notebooks/Distributed_arrays_and_automatic_parallelization.html).
|
||||
While in those automatic partitioning examples we don't need to edit the model
|
||||
functions to use different parallelization strategies, with `shard_map` we
|
||||
often do.
|
||||
@ -1137,7 +1137,7 @@ There's one other ingredient we need: we don't want to store the fully gathered
|
||||
parameters from the forward pass for use on the backward pass. Instead, we want
|
||||
to gather them again on the backward pass. We can express that by using
|
||||
`jax.remat` with a [custom
|
||||
policy](https://jax.readthedocs.io/en/latest/notebooks/autodiff_remat.html#custom-policies-for-what-s-saveable)
|
||||
policy](https://docs.jax.dev/en/latest/notebooks/autodiff_remat.html#custom-policies-for-what-s-saveable)
|
||||
(or a `custom_vjp`), though XLA typically does that rematerialization
|
||||
automatically.
|
||||
|
||||
|
@ -248,7 +248,7 @@
|
||||
"id": "yRYF0YgO3F4H"
|
||||
},
|
||||
"source": [
|
||||
"For updating individual elements, JAX provides an [indexed update syntax](https://jax.readthedocs.io/en/latest/_autosummary/jax.numpy.ndarray.at.html#jax-numpy-ndarray-at) that returns an updated copy:"
|
||||
"For updating individual elements, JAX provides an [indexed update syntax](https://docs.jax.dev/en/latest/_autosummary/jax.numpy.ndarray.at.html#jax-numpy-ndarray-at) that returns an updated copy:"
|
||||
]
|
||||
},
|
||||
{
|
||||
@ -423,7 +423,7 @@
|
||||
"id": "0GPqgT7S0q8r"
|
||||
},
|
||||
"source": [
|
||||
"Under the hood, this NumPy operation is translated to a much more general convolution implemented by [`lax.conv_general_dilated`](https://jax.readthedocs.io/en/latest/_autosummary/jax.lax.conv_general_dilated.html):"
|
||||
"Under the hood, this NumPy operation is translated to a much more general convolution implemented by [`lax.conv_general_dilated`](https://docs.jax.dev/en/latest/_autosummary/jax.lax.conv_general_dilated.html):"
|
||||
]
|
||||
},
|
||||
{
|
||||
@ -461,7 +461,7 @@
|
||||
"id": "7mdo6ycczlbd"
|
||||
},
|
||||
"source": [
|
||||
"This is a batched convolution operation designed to be efficient for the types of convolutions often used in deep neural nets. It requires much more boilerplate, but is far more flexible and scalable than the convolution provided by NumPy (See [Convolutions in JAX](https://jax.readthedocs.io/en/latest/notebooks/convolutions.html) for more detail on JAX convolutions).\n",
|
||||
"This is a batched convolution operation designed to be efficient for the types of convolutions often used in deep neural nets. It requires much more boilerplate, but is far more flexible and scalable than the convolution provided by NumPy (See [Convolutions in JAX](https://docs.jax.dev/en/latest/notebooks/convolutions.html) for more detail on JAX convolutions).\n",
|
||||
"\n",
|
||||
"At their heart, all `jax.lax` operations are Python wrappers for operations in XLA; here, for example, the convolution implementation is provided by [XLA:ConvWithGeneralPadding](https://www.tensorflow.org/xla/operation_semantics#convwithgeneralpadding_convolution).\n",
|
||||
"Every JAX operation is eventually expressed in terms of these fundamental XLA operations, which is what enables just-in-time (JIT) compilation."
|
||||
@ -562,7 +562,7 @@
|
||||
"id": "3GvisB-CA9M8"
|
||||
},
|
||||
"source": [
|
||||
"But due to the compilation (which includes fusing of operations, avoidance of allocating temporary arrays, and a host of other tricks), execution times can be orders of magnitude faster in the JIT-compiled case (note the use of `block_until_ready()` to account for JAX's [asynchronous dispatch](https://jax.readthedocs.io/en/latest/async_dispatch.html)):"
|
||||
"But due to the compilation (which includes fusing of operations, avoidance of allocating temporary arrays, and a host of other tricks), execution times can be orders of magnitude faster in the JIT-compiled case (note the use of `block_until_ready()` to account for JAX's [asynchronous dispatch](https://docs.jax.dev/en/latest/async_dispatch.html)):"
|
||||
]
|
||||
},
|
||||
{
|
||||
@ -650,7 +650,7 @@
|
||||
"evalue": "ignored",
|
||||
"output_type": "error",
|
||||
"traceback": [
|
||||
"\u001b[0;31mNonConcreteBooleanIndexError\u001b[0m\u001b[0;31m:\u001b[0m Array boolean indices must be concrete; got ShapedArray(bool[10])\n\nSee https://jax.readthedocs.io/en/latest/errors.html#jax.errors.NonConcreteBooleanIndexError\n"
|
||||
"\u001b[0;31mNonConcreteBooleanIndexError\u001b[0m\u001b[0;31m:\u001b[0m Array boolean indices must be concrete; got ShapedArray(bool[10])\n\nSee https://docs.jax.dev/en/latest/errors.html#jax.errors.NonConcreteBooleanIndexError\n"
|
||||
]
|
||||
}
|
||||
],
|
||||
@ -835,7 +835,7 @@
|
||||
"evalue": "ignored",
|
||||
"output_type": "error",
|
||||
"traceback": [
|
||||
"\u001b[0;31mTracerBoolConversionError\u001b[0m\u001b[0;31m:\u001b[0m Attempted boolean conversion of traced array with shape bool[]..\nThe error occurred while tracing the function f at <ipython-input-24-acbedba5ce66>:1 for jit. This concrete value was not available in Python because it depends on the value of the argument neg.\nSee https://jax.readthedocs.io/en/latest/errors.html#jax.errors.TracerBoolConversionError\n"
|
||||
"\u001b[0;31mTracerBoolConversionError\u001b[0m\u001b[0;31m:\u001b[0m Attempted boolean conversion of traced array with shape bool[]..\nThe error occurred while tracing the function f at <ipython-input-24-acbedba5ce66>:1 for jit. This concrete value was not available in Python because it depends on the value of the argument neg.\nSee https://docs.jax.dev/en/latest/errors.html#jax.errors.TracerBoolConversionError\n"
|
||||
]
|
||||
}
|
||||
],
|
||||
|
@ -117,7 +117,7 @@ x[0] = 10
|
||||
|
||||
+++ {"id": "yRYF0YgO3F4H"}
|
||||
|
||||
For updating individual elements, JAX provides an [indexed update syntax](https://jax.readthedocs.io/en/latest/_autosummary/jax.numpy.ndarray.at.html#jax-numpy-ndarray-at) that returns an updated copy:
|
||||
For updating individual elements, JAX provides an [indexed update syntax](https://docs.jax.dev/en/latest/_autosummary/jax.numpy.ndarray.at.html#jax-numpy-ndarray-at) that returns an updated copy:
|
||||
|
||||
```{code-cell} ipython3
|
||||
:id: 8zqPEAeP3UK5
|
||||
@ -189,7 +189,7 @@ jnp.convolve(x, y)
|
||||
|
||||
+++ {"id": "0GPqgT7S0q8r"}
|
||||
|
||||
Under the hood, this NumPy operation is translated to a much more general convolution implemented by [`lax.conv_general_dilated`](https://jax.readthedocs.io/en/latest/_autosummary/jax.lax.conv_general_dilated.html):
|
||||
Under the hood, this NumPy operation is translated to a much more general convolution implemented by [`lax.conv_general_dilated`](https://docs.jax.dev/en/latest/_autosummary/jax.lax.conv_general_dilated.html):
|
||||
|
||||
```{code-cell} ipython3
|
||||
:id: pi4f6ikjzc3l
|
||||
@ -206,7 +206,7 @@ result[0, 0]
|
||||
|
||||
+++ {"id": "7mdo6ycczlbd"}
|
||||
|
||||
This is a batched convolution operation designed to be efficient for the types of convolutions often used in deep neural nets. It requires much more boilerplate, but is far more flexible and scalable than the convolution provided by NumPy (See [Convolutions in JAX](https://jax.readthedocs.io/en/latest/notebooks/convolutions.html) for more detail on JAX convolutions).
|
||||
This is a batched convolution operation designed to be efficient for the types of convolutions often used in deep neural nets. It requires much more boilerplate, but is far more flexible and scalable than the convolution provided by NumPy (See [Convolutions in JAX](https://docs.jax.dev/en/latest/notebooks/convolutions.html) for more detail on JAX convolutions).
|
||||
|
||||
At their heart, all `jax.lax` operations are Python wrappers for operations in XLA; here, for example, the convolution implementation is provided by [XLA:ConvWithGeneralPadding](https://www.tensorflow.org/xla/operation_semantics#convwithgeneralpadding_convolution).
|
||||
Every JAX operation is eventually expressed in terms of these fundamental XLA operations, which is what enables just-in-time (JIT) compilation.
|
||||
@ -261,7 +261,7 @@ np.allclose(norm(X), norm_compiled(X), atol=1E-6)
|
||||
|
||||
+++ {"id": "3GvisB-CA9M8"}
|
||||
|
||||
But due to the compilation (which includes fusing of operations, avoidance of allocating temporary arrays, and a host of other tricks), execution times can be orders of magnitude faster in the JIT-compiled case (note the use of `block_until_ready()` to account for JAX's [asynchronous dispatch](https://jax.readthedocs.io/en/latest/async_dispatch.html)):
|
||||
But due to the compilation (which includes fusing of operations, avoidance of allocating temporary arrays, and a host of other tricks), execution times can be orders of magnitude faster in the JIT-compiled case (note the use of `block_until_ready()` to account for JAX's [asynchronous dispatch](https://docs.jax.dev/en/latest/async_dispatch.html)):
|
||||
|
||||
```{code-cell} ipython3
|
||||
:id: 6mUB6VdDAEIY
|
||||
|
@ -5,7 +5,7 @@
|
||||
<!--* freshness: { reviewed: '2024-07-11' } *-->
|
||||
|
||||
This is the list of changes specific to {class}`jax.experimental.pallas`.
|
||||
For the overall JAX change log see [here](https://jax.readthedocs.io/en/latest/changelog.html).
|
||||
For the overall JAX change log see [here](https://docs.jax.dev/en/latest/changelog.html).
|
||||
|
||||
<!--
|
||||
Remember to align the itemized text with the first line of an item within a list.
|
||||
|
@ -58,7 +58,7 @@ print(selu(x))
|
||||
```
|
||||
|
||||
You'll find a few differences between JAX arrays and NumPy arrays once you begin digging-in;
|
||||
these are explored in [🔪 JAX - The Sharp Bits 🔪](https://jax.readthedocs.io/en/latest/notebooks/Common_Gotchas_in_JAX.html).
|
||||
these are explored in [🔪 JAX - The Sharp Bits 🔪](https:docs.jax.devio/en/latest/notebooks/Common_Gotchas_in_JAX.html).
|
||||
|
||||
## Just-in-time compilation with {func}`jax.jit`
|
||||
JAX runs transparently on the GPU or TPU (falling back to CPU if you don't have one). However, in the above example, JAX is dispatching kernels to the chip one operation at a time. If we have a sequence of operations, we can use the {func}`jax.jit` function to compile this sequence of operations together using XLA.
|
||||
|
@ -20,7 +20,7 @@ kernelspec:
|
||||
JAX transformations like {func}`~jax.jit`, {func}`~jax.vmap`, {func}`~jax.grad`, require the functions
|
||||
they wrap to be pure: that is, functions whose outputs depend *solely* on the inputs, and which have
|
||||
no side effects such as updating of global state.
|
||||
You can find a discussion of this in [JAX sharp bits: Pure functions](https://jax.readthedocs.io/en/latest/notebooks/Common_Gotchas_in_JAX.html#pure-functions).
|
||||
You can find a discussion of this in [JAX sharp bits: Pure functions](https://docs.jax.dev/en/latest/notebooks/Common_Gotchas_in_JAX.html#pure-functions).
|
||||
|
||||
This constraint can pose some challenges in the context of machine learning, where state may exist in
|
||||
many forms. For example:
|
||||
|
@ -4,7 +4,7 @@ Type promotion semantics
|
||||
========================
|
||||
|
||||
This document describes JAX's type promotion rules–i.e., the result of :func:`jax.numpy.promote_types` for each pair of types.
|
||||
For some background on the considerations that went into the design of what is described below, see `Design of Type Promotion Semantics for JAX <https://jax.readthedocs.io/en/latest/jep/9407-type-promotion.html>`_.
|
||||
For some background on the considerations that went into the design of what is described below, see `Design of Type Promotion Semantics for JAX <https://docs.jax.dev/en/latest/jep/9407-type-promotion.html>`_.
|
||||
|
||||
JAX's type promotion behavior is determined via the following type promotion lattice:
|
||||
|
||||
|
@ -85,4 +85,4 @@ XLA_FLAGS='--flag1=value1 --flag2=value2' python3 source.py
|
||||
| `xla_gpu_enable_reduce_scatter_combine_by_dim` | Boolean (true/false) | Combine reduce-scatter ops with the same dimension or irrespective of their dimension. |
|
||||
|
||||
**Additional reading:**
|
||||
* [GPU performance tips](https://jax.readthedocs.io/en/latest/gpu_performance_tips.html#xla-performance-flags)
|
||||
* [GPU performance tips](https://docs.jax.dev/en/latest/gpu_performance_tips.html#xla-performance-flags)
|
||||
|
@ -2,7 +2,7 @@
|
||||
|
||||
This directory includes an example project demonstrating the use of JAX's
|
||||
foreign function interface (FFI). The JAX docs provide more information about
|
||||
this interface in [the FFI tutorial](https://jax.readthedocs.io/en/latest/ffi.html),
|
||||
this interface in [the FFI tutorial](https://docs.jax.dev/en/latest/ffi.html),
|
||||
but the example in this directory complements that document by demonstrating
|
||||
(and testing!) the full packaging workflow, and some more advanced use cases.
|
||||
Within the example project, there are several example calls:
|
||||
|
@ -14,7 +14,7 @@
|
||||
"""An example demontrating the basic end-to-end use of the JAX FFI.
|
||||
|
||||
This example is exactly the same as the one in the `FFI tutorial
|
||||
<https://jax.readthedocs.io/en/latest/ffi.html>`, so more details can be found
|
||||
<https://docs.jax.dev/en/latest/ffi.html>`, so more details can be found
|
||||
on that page. But, the high level summary is that we implement our custom
|
||||
extension in ``rms_norm.cc``, then call it usin ``jax.ffi.ffi_call`` in
|
||||
this module. The behavior under autodiff is implemented using
|
||||
|
@ -93,7 +93,7 @@ package_group(
|
||||
includes = [":internal"],
|
||||
packages = [
|
||||
# Intentionally avoid jax dependencies on jax.extend.
|
||||
# See https://jax.readthedocs.io/en/latest/jep/15856-jex.html
|
||||
# See https://docs.jax.dev/en/latest/jep/15856-jex.html
|
||||
"//tests/...",
|
||||
] + jax_extend_internal_users,
|
||||
)
|
||||
|
@ -430,7 +430,7 @@ def _trace_to_jaxpr(fun: Callable,
|
||||
"Consider using the `static_argnums` parameter for `jax.remat` or "
|
||||
"`jax.checkpoint`. See the `jax.checkpoint` docstring and its example "
|
||||
"involving `static_argnums`:\n"
|
||||
"https://jax.readthedocs.io/en/latest/_autosummary/jax.checkpoint.html"
|
||||
"https://docs.jax.dev/en/latest/_autosummary/jax.checkpoint.html"
|
||||
"\n")
|
||||
e.args = msg,
|
||||
raise
|
||||
@ -875,7 +875,7 @@ def checkpoint_wrapper(
|
||||
" else:\n"
|
||||
" return g(x)\n"
|
||||
"\n"
|
||||
"See https://jax.readthedocs.io/en/latest/jep/11830-new-remat-checkpoint.html\n")
|
||||
"See https://docs.jax.dev/en/latest/jep/11830-new-remat-checkpoint.html\n")
|
||||
raise NotImplementedError(msg)
|
||||
return checkpoint(fun, prevent_cse=prevent_cse, policy=policy,
|
||||
static_argnums=static_argnums)
|
||||
|
@ -232,7 +232,7 @@ def jit(
|
||||
be donated.
|
||||
|
||||
For more details on buffer donation see the
|
||||
`FAQ <https://jax.readthedocs.io/en/latest/faq.html#buffer-donation>`_.
|
||||
`FAQ <https://docs.jax.dev/en/latest/faq.html#buffer-donation>`_.
|
||||
donate_argnames: optional, a string or collection of strings specifying
|
||||
which named arguments are donated to the computation. See the
|
||||
comment on ``donate_argnums`` for details. If not
|
||||
@ -856,7 +856,7 @@ def vmap(fun: F,
|
||||
be a container with a matching pytree structure specifying the mapping of its
|
||||
container elements. In other words, ``in_axes`` must be a container tree prefix
|
||||
of the positional argument tuple passed to ``fun``. See this link for more detail:
|
||||
https://jax.readthedocs.io/en/latest/pytrees.html#applying-optional-parameters-to-pytrees
|
||||
https://docs.jax.dev/en/latest/pytrees.html#applying-optional-parameters-to-pytrees
|
||||
|
||||
Either ``axis_size`` must be provided explicitly, or at least one
|
||||
positional argument must have ``in_axes`` not None. The sizes of the
|
||||
@ -1242,7 +1242,7 @@ def pmap(
|
||||
arguments will not be donated.
|
||||
|
||||
For more details on buffer donation see the
|
||||
`FAQ <https://jax.readthedocs.io/en/latest/faq.html#buffer-donation>`_.
|
||||
`FAQ <https://docs.jax.dev/en/latest/faq.html#buffer-donation>`_.
|
||||
|
||||
Returns:
|
||||
A parallelized version of ``fun`` with arguments that correspond to those of
|
||||
@ -1489,7 +1489,7 @@ def _prepare_pmap(fun: Callable, in_axes, out_axes, static_broadcasted_tuple,
|
||||
"Instead, each argument passed by keyword is mapped over its "
|
||||
"leading axis. See the description of `in_axes` in the `pmap` "
|
||||
"docstring: "
|
||||
"https://jax.readthedocs.io/en/latest/_autosummary/jax.pmap.html#jax.pmap")
|
||||
"https://docs.jax.dev/en/latest/_autosummary/jax.pmap.html#jax.pmap")
|
||||
msg += ("\n\nCheck that the value of the `in_axes` argument to `pmap` "
|
||||
"is a tree prefix of the tuple of arguments passed positionally to "
|
||||
"the pmapped function.")
|
||||
|
@ -142,7 +142,7 @@ class Array:
|
||||
a + b # Raises an error
|
||||
```
|
||||
|
||||
See https://jax.readthedocs.io/en/latest/faq.html#controlling-data-and-computation-placement-on-devices
|
||||
See https://docs.jax.dev/en/latest/faq.html#controlling-data-and-computation-placement-on-devices
|
||||
for more information.
|
||||
"""
|
||||
raise NotImplementedError
|
||||
|
@ -371,7 +371,7 @@ def pure_callback(
|
||||
(4,) (4,)
|
||||
Array([1., 2., 3., 4.], dtype=float32)
|
||||
|
||||
.. _External Callbacks: https://jax.readthedocs.io/en/latest/external-callbacks.html
|
||||
.. _External Callbacks: https://docs.jax.dev/en/latest/external-callbacks.html
|
||||
"""
|
||||
if not isinstance(vectorized, DeprecatedArg) and not vectorized is None:
|
||||
deprecations.warn(
|
||||
@ -580,7 +580,7 @@ def io_callback(
|
||||
- :func:`jax.debug.callback`: callback designed for general-purpose debugging.
|
||||
- :func:`jax.debug.print`: callback designed for printing.
|
||||
|
||||
.. _External Callbacks: https://jax.readthedocs.io/en/latest/notebooks/external_callbacks.html
|
||||
.. _External Callbacks: https://docs.jax.dev/en/latest/notebooks/external_callbacks.html
|
||||
"""
|
||||
flat_args, in_tree = tree_util.tree_flatten((args, kwargs))
|
||||
tree_util.tree_map(_check_shape_dtype, result_shape_dtypes)
|
||||
|
@ -275,7 +275,7 @@ def put_executable_and_time(
|
||||
f"PERSISTENT CACHE WRITE with key {cache_key}, this is unexpected because "
|
||||
"JAX_COMPILATION_CACHE_EXPECT_PGLE is set. The execution that populated the "
|
||||
"cache may lack coverage, "
|
||||
"https://jax.readthedocs.io/en/latest/persistent_compilation_cache.html may "
|
||||
"https://docs.jax.dev/en/latest/persistent_compilation_cache.html may "
|
||||
"help debug why this has happened")
|
||||
|
||||
cache.put(cache_key, executable_and_time)
|
||||
|
@ -359,7 +359,7 @@ UPGRADE_BOOL_HELP = (
|
||||
" This will be enabled by default in future versions of JAX, at which "
|
||||
"point all uses of the flag will be considered deprecated (following "
|
||||
"the `API compatibility policy "
|
||||
"<https://jax.readthedocs.io/en/latest/api_compatibility.html>`_).")
|
||||
"<https://docs.jax.dev/en/latest/api_compatibility.html>`_).")
|
||||
|
||||
UPGRADE_BOOL_EXTRA_DESC = " (transient)"
|
||||
|
||||
@ -911,7 +911,7 @@ jax_export_calling_convention_version = int_state(
|
||||
'The calling convention version number to use for exporting. This must be '
|
||||
'within the range of versions supported by the tf.XlaCallModule '
|
||||
'used in your deployment environment. '
|
||||
'See https://jax.readthedocs.io/en/latest/export/shape_poly.html#calling-convention-versions.'
|
||||
'See https://docs.jax.dev/en/latest/export/shape_poly.html#calling-convention-versions.'
|
||||
)
|
||||
)
|
||||
|
||||
@ -920,7 +920,7 @@ export_ignore_forward_compatibility = bool_state(
|
||||
default=bool_env('JAX_EXPORT_IGNORE_FORWARD_COMPATIBILIY', False),
|
||||
help=(
|
||||
'Whether to ignore the forward compatibility lowering rules. '
|
||||
'See https://jax.readthedocs.io/en/latest/export/export.html#compatibility-guarantees-for-custom-calls.'
|
||||
'See https://docs.jax.dev/en/latest/export/export.html#compatibility-guarantees-for-custom-calls.'
|
||||
)
|
||||
)
|
||||
|
||||
@ -1668,7 +1668,7 @@ def transfer_guard(new_val: str) -> Iterator[None]:
|
||||
"""A contextmanager to control the transfer guard level for all transfers.
|
||||
|
||||
For more information, see
|
||||
https://jax.readthedocs.io/en/latest/transfer_guard.html
|
||||
https://docs.jax.dev/en/latest/transfer_guard.html
|
||||
|
||||
Args:
|
||||
new_val: The new thread-local transfer guard level for all transfers.
|
||||
|
@ -130,7 +130,7 @@ class custom_jvp(Generic[ReturnValue]):
|
||||
|
||||
For a more detailed introduction, see the tutorial_.
|
||||
|
||||
.. _tutorial: https://jax.readthedocs.io/en/latest/notebooks/Custom_derivative_rules_for_Python_code.html
|
||||
.. _tutorial: https://docs.jax.dev/en/latest/notebooks/Custom_derivative_rules_for_Python_code.html
|
||||
"""
|
||||
fun: Callable[..., ReturnValue]
|
||||
nondiff_argnums: Sequence[int]
|
||||
@ -521,7 +521,7 @@ class custom_vjp(Generic[ReturnValue]):
|
||||
|
||||
For a more detailed introduction, see the tutorial_.
|
||||
|
||||
.. _tutorial: https://jax.readthedocs.io/en/latest/notebooks/Custom_derivative_rules_for_Python_code.html
|
||||
.. _tutorial: https://docs.jax.dev/en/latest/notebooks/Custom_derivative_rules_for_Python_code.html
|
||||
"""
|
||||
|
||||
def __init__(self,
|
||||
|
@ -284,7 +284,7 @@ def debug_callback(callback: Callable[..., None], *args: Any,
|
||||
- :func:`jax.pure_callback`: callback designed for pure functions.
|
||||
- :func:`jax.debug.print`: callback designed for printing.
|
||||
|
||||
.. _External Callbacks: https://jax.readthedocs.io/en/latest/notebooks/external_callbacks.html
|
||||
.. _External Callbacks: https://docs.jax.dev/en/latest/notebooks/external_callbacks.html
|
||||
"""
|
||||
if not callable(callback):
|
||||
raise TypeError("first argument to jax.debug.callback must be callable, "
|
||||
|
@ -47,7 +47,7 @@ dispatching a computation and on which we can block until is ready. We store
|
||||
for each thread the `RuntimeToken` returned by the last dispatched computation.
|
||||
|
||||
For more details, see the design note:
|
||||
https://jax.readthedocs.io/en/latest/jep/10657-sequencing-effects.html.
|
||||
https://docs.jax.dev/en/latest/jep/10657-sequencing-effects.html.
|
||||
"""
|
||||
|
||||
from __future__ import annotations
|
||||
|
@ -21,7 +21,7 @@ export = set_module('jax.errors')
|
||||
|
||||
class _JAXErrorMixin:
|
||||
"""Mixin for JAX-specific errors"""
|
||||
_error_page = 'https://jax.readthedocs.io/en/latest/errors.html'
|
||||
_error_page = 'https://docs.jax.dev/en/latest/errors.html'
|
||||
_module_name = "jax.errors"
|
||||
|
||||
def __init__(self, message: str):
|
||||
@ -306,7 +306,7 @@ class TracerArrayConversionError(JAXTypeError):
|
||||
and concrete vs. abstract values, you may want to read
|
||||
:ref:`faq-different-kinds-of-jax-values`.
|
||||
|
||||
.. _External Callbacks: https://jax.readthedocs.io/en/latest/notebooks/external_callbacks.html
|
||||
.. _External Callbacks: https://docs.jax.dev/en/latest/notebooks/external_callbacks.html
|
||||
"""
|
||||
def __init__(self, tracer: core.Tracer):
|
||||
super().__init__(
|
||||
@ -530,7 +530,7 @@ class UnexpectedTracerError(JAXTypeError):
|
||||
function ``f`` that stores, in some scope outside of ``f``, a reference to
|
||||
an intermediate value, that value is considered to have been leaked.
|
||||
Leaking values is a side effect. (Read more about avoiding side effects in
|
||||
`Pure Functions <https://jax.readthedocs.io/en/latest/notebooks/Common_Gotchas_in_JAX.html#pure-functions>`_)
|
||||
`Pure Functions <https://docs.jax.dev/en/latest/notebooks/Common_Gotchas_in_JAX.html#pure-functions>`_)
|
||||
|
||||
JAX detects leaks when you then use the leaked value in another
|
||||
operation later on, at which point it raises an ``UnexpectedTracerError``.
|
||||
@ -678,5 +678,5 @@ class KeyReuseError(JAXTypeError):
|
||||
|
||||
This sort of key reuse is problematic because the JAX PRNG is stateless, and keys
|
||||
must be manually split; For more information on this see `the Pseudorandom Numbers
|
||||
tutorial <https://jax.readthedocs.io/en/latest/random-numbers.html>`_.
|
||||
tutorial <https://docs.jax.dev/en/latest/random-numbers.html>`_.
|
||||
"""
|
||||
|
@ -67,7 +67,7 @@ LoweringSharding = Union[sharding.Sharding, pxla.UnspecifiedValue]
|
||||
HloSharding = xla_client.HloSharding
|
||||
|
||||
# The minimum and maximum supported calling convention version.
|
||||
# See https://jax.readthedocs.io/en/latest/export/export.html#export-calling-convention-version
|
||||
# See https://docs.jax.dev/en/latest/export/export.html#export-calling-convention-version
|
||||
minimum_supported_calling_convention_version = 9
|
||||
maximum_supported_calling_convention_version = 9
|
||||
|
||||
@ -153,16 +153,16 @@ class Exported:
|
||||
platforms: a tuple containing the platforms for which the function should
|
||||
be exported. The set of platforms in JAX is open-ended; users can
|
||||
add platforms. JAX built-in platforms are: 'tpu', 'cpu', 'cuda', 'rocm'.
|
||||
See https://jax.readthedocs.io/en/latest/export/export.html#cross-platform-and-multi-platform-export.
|
||||
See https://docs.jax.dev/en/latest/export/export.html#cross-platform-and-multi-platform-export.
|
||||
ordered_effects: the ordered effects present in the serialized module.
|
||||
This is present from serialization version 9. See https://jax.readthedocs.io/en/latest/export/export.html#module-calling-convention
|
||||
This is present from serialization version 9. See https://docs.jax.dev/en/latest/export/export.html#module-calling-convention
|
||||
for the calling convention in presence of ordered effects.
|
||||
unordered_effects: the unordered effects present in the serialized module.
|
||||
This is present from serialization version 9.
|
||||
mlir_module_serialized: the serialized lowered VHLO module.
|
||||
calling_convention_version: a version number for the calling
|
||||
convention of the exported module.
|
||||
See more versioning details at https://jax.readthedocs.io/en/latest/export/export.html#calling-convention-versions.
|
||||
See more versioning details at https://docs.jax.dev/en/latest/export/export.html#calling-convention-versions.
|
||||
module_kept_var_idx: the sorted indices of the arguments among `in_avals` that
|
||||
must be passed to the module. The other arguments have been dropped
|
||||
because they are not used.
|
||||
@ -181,7 +181,7 @@ class Exported:
|
||||
for each primal output. It returns a tuple with the cotangents
|
||||
corresponding to the flattened primal inputs.
|
||||
|
||||
See a [description of the calling convention for the `mlir_module`](https://jax.readthedocs.io/en/latest/export/export.html#module-calling-convention).
|
||||
See a [description of the calling convention for the `mlir_module`](https://docs.jax.dev/en/latest/export/export.html#module-calling-convention).
|
||||
"""
|
||||
fun_name: str
|
||||
in_tree: tree_util.PyTreeDef
|
||||
@ -306,7 +306,7 @@ class Exported:
|
||||
|
||||
The invocation supports reverse-mode AD, and all the features supported
|
||||
by exporting: shape polymorphism, multi-platform, device polymorphism.
|
||||
See the examples in the [JAX export documentation](https://jax.readthedocs.io/en/latest/export/export.html).
|
||||
See the examples in the [JAX export documentation](https://docs.jax.dev/en/latest/export/export.html).
|
||||
"""
|
||||
return call_exported(self)(*args, **kwargs)
|
||||
|
||||
@ -541,7 +541,7 @@ def export(
|
||||
the exported code takes an argument specifying the platform.
|
||||
If None, then use the default JAX backend.
|
||||
The calling convention for multiple platforms is explained at
|
||||
https://jax.readthedocs.io/en/latest/export/export.html#module-calling-convention.
|
||||
https://docs.jax.dev/en/latest/export/export.html#module-calling-convention.
|
||||
_override_lowering_rules: an optional sequence of custom lowering rules
|
||||
for some JAX primitives. Each element of the sequence is a pair
|
||||
of a JAX primitive and a lowering function. Defining lowering rules
|
||||
@ -593,7 +593,7 @@ def _export_internal(
|
||||
|
||||
Note: this function exists only for internal usage by jax2tf. Use
|
||||
`jax.export` instead.
|
||||
See https://jax.readthedocs.io/en/latest/export/export.html
|
||||
See https://docs.jax.dev/en/latest/export/export.html
|
||||
|
||||
See docstring of `export` for more details.
|
||||
"""
|
||||
@ -837,7 +837,7 @@ def _wrap_main_func(
|
||||
) -> ir.Module:
|
||||
"""Wraps the lowered module with a new "main" handling dimension arguments.
|
||||
|
||||
See calling convention documentation https://jax.readthedocs.io/en/latest/export/export.html#module-calling-convention.
|
||||
See calling convention documentation https://docs.jax.dev/en/latest/export/export.html#module-calling-convention.
|
||||
|
||||
Args:
|
||||
module: the HLO module as obtained from lowering.
|
||||
@ -1187,7 +1187,7 @@ def _check_module(mod: ir.Module, *,
|
||||
disallowed_custom_call_ops_str = "\n".join(disallowed_custom_call_ops)
|
||||
msg = ("Cannot serialize code with custom calls whose targets have no "
|
||||
"compatibility guarantees. "
|
||||
"See https://jax.readthedocs.io/en/latest/export/export.html#compatibility-guarantees-for-custom-calls. "
|
||||
"See https://docs.jax.dev/en/latest/export/export.html#compatibility-guarantees-for-custom-calls. "
|
||||
"Examples are:\n"
|
||||
f"{disallowed_custom_call_ops_str}.\n")
|
||||
raise ValueError(msg)
|
||||
|
@ -13,7 +13,7 @@
|
||||
# limitations under the License.
|
||||
"""Shape polymorphism support.
|
||||
|
||||
See documentation at https://jax.readthedocs.io/en/latest/export/shape_poly.html.
|
||||
See documentation at https://docs.jax.dev/en/latest/export/shape_poly.html.
|
||||
"""
|
||||
|
||||
from __future__ import annotations
|
||||
@ -70,7 +70,7 @@ This error arises for comparison operations with shapes that
|
||||
are non-constant, and the result of the operation cannot be represented as
|
||||
a boolean value for all values of the symbolic dimensions involved.
|
||||
|
||||
Please see https://jax.readthedocs.io/en/latest/export/shape_poly.html#comparison-of-symbolic-dimensions-is-partially-supported
|
||||
Please see https://docs.jax.dev/en/latest/export/shape_poly.html#comparison-of-symbolic-dimensions-is-partially-supported
|
||||
for more details.
|
||||
"""
|
||||
|
||||
@ -227,7 +227,7 @@ class _DimFactor:
|
||||
return normalized_var._evaluate(env) # type: ignore
|
||||
err_msg = (
|
||||
f"Encountered dimension variable '{self.var}' that is not appearing in the shapes of the function arguments.\n"
|
||||
"Please see https://jax.readthedocs.io/en/latest/export/shape_poly.html#dimension-variables-must-be-solvable-from-the-input-shapes for more details.")
|
||||
"Please see https://docs.jax.dev/en/latest/export/shape_poly.html#dimension-variables-must-be-solvable-from-the-input-shapes for more details.")
|
||||
raise UnexpectedDimVar(err_msg)
|
||||
else:
|
||||
operand_values = [opnd._evaluate(env) for opnd in self.operands]
|
||||
@ -654,7 +654,7 @@ class _DimExpr:
|
||||
# Here we really ought to raise InconclusiveDimensionOperation, but __eq__
|
||||
# cannot raise exceptions, because it is used indirectly when hashing.
|
||||
# So, we say that the expressions are disequal, which is really unsound.
|
||||
# See https://jax.readthedocs.io/en/latest/export/shape_poly.html#comparison-of-symbolic-dimensions-is-partially-supported
|
||||
# See https://docs.jax.dev/en/latest/export/shape_poly.html#comparison-of-symbolic-dimensions-is-partially-supported
|
||||
return False
|
||||
|
||||
return diff == 0
|
||||
@ -841,7 +841,7 @@ class _DimExpr:
|
||||
# Here we really ought to raise InconclusiveDimensionOperation, but __eq__
|
||||
# cannot raise exceptions, because it is used indirectly when hashing.
|
||||
# So, we say that the expressions are disequal, which is really unsound.
|
||||
# See https://jax.readthedocs.io/en/latest/export/shape_poly.html#comparison-of-symbolic-dimensions-is-partially-supported
|
||||
# See https://docs.jax.dev/en/latest/export/shape_poly.html#comparison-of-symbolic-dimensions-is-partially-supported
|
||||
return False
|
||||
|
||||
return diff == 0
|
||||
@ -986,7 +986,7 @@ class SymbolicScope:
|
||||
|
||||
Holds the constraints on symbolic expressions.
|
||||
|
||||
See [the README](https://jax.readthedocs.io/en/latest/export/shape_poly.html#user-specified-symbolic-constraints)
|
||||
See [the README](https://docs.jax.dev/en/latest/export/shape_poly.html#user-specified-symbolic-constraints)
|
||||
for more details.
|
||||
|
||||
Args:
|
||||
@ -1112,7 +1112,7 @@ class SymbolicScope:
|
||||
f"Invalid mixing of symbolic scopes {when}.\n"
|
||||
f"Expected {self_descr}scope {self}\n"
|
||||
f"and found for '{other}' ({other_descr}) scope {other.scope}\n"
|
||||
f"See https://jax.readthedocs.io/en/latest/export/shape_poly.html#user-specified-symbolic-constraints.")
|
||||
f"See https://docs.jax.dev/en/latest/export/shape_poly.html#user-specified-symbolic-constraints.")
|
||||
|
||||
def _clear_caches(self):
|
||||
self._bounds_cache.clear()
|
||||
@ -1384,7 +1384,7 @@ def symbolic_shape(shape_spec: str | None,
|
||||
) -> Sequence[DimSize]:
|
||||
"""Constructs a symbolic shape from a string representation.
|
||||
|
||||
See https://jax.readthedocs.io/en/latest/export/shape_poly.html for examples.
|
||||
See https://docs.jax.dev/en/latest/export/shape_poly.html for examples.
|
||||
|
||||
Args:
|
||||
shape_spec: a symbolic shape specification. None stands for "...".
|
||||
@ -1396,13 +1396,13 @@ def symbolic_shape(shape_spec: str | None,
|
||||
mod(e1, e2), max(e1, e2), or min(e1, e2).
|
||||
constraints: a sequence of constraints on symbolic dimension expressions, of
|
||||
the form `e1 >= e2` or `e1 <= e2`, or `e1 == e2`.
|
||||
See [the documentation](https://jax.readthedocs.io/en/latest/export/shape_poly.html#user-specified-symbolic-constraints)
|
||||
See [the documentation](https://docs.jax.dev/en/latest/export/shape_poly.html#user-specified-symbolic-constraints)
|
||||
for usage.
|
||||
scope: optionally, you can specify that the parsed symbolic expressions
|
||||
be created in the given scope. If this is missing, then a new
|
||||
`SymbolicScope` is created with the given `constraints`.
|
||||
You cannot specify both a `scope` and `constraints`.
|
||||
See [the documentation](https://jax.readthedocs.io/en/latest/export/shape_poly.html#user-specified-symbolic-constraints)
|
||||
See [the documentation](https://docs.jax.dev/en/latest/export/shape_poly.html#user-specified-symbolic-constraints)
|
||||
for usage.
|
||||
like: when `shape_spec` contains placeholders ("_", "..."), use this
|
||||
shape to fill in the placeholders.
|
||||
@ -1437,7 +1437,7 @@ def symbolic_args_specs(
|
||||
"""Constructs a pytree of jax.ShapeDtypeSpec arguments specs for `export`.
|
||||
|
||||
See the documentation of :func:`jax.export.symbolic_shape` and
|
||||
the [shape polymorphism documentation](https://jax.readthedocs.io/en/latest/export/shape_poly.html) for details.
|
||||
the [shape polymorphism documentation](https://docs.jax.dev/en/latest/export/shape_poly.html) for details.
|
||||
|
||||
Args:
|
||||
args: a pytree of arguments. These can be jax.Array, or jax.ShapeDTypeSpec.
|
||||
@ -1450,7 +1450,7 @@ def symbolic_args_specs(
|
||||
applies to all arguments), or a pytree matching a prefix
|
||||
of the `args`.
|
||||
See [how optional parameters are matched to
|
||||
arguments](https://jax.readthedocs.io/en/latest/pytrees.html#applying-optional-parameters-to-pytrees).
|
||||
arguments](https://docs.jax.dev/en/latest/pytrees.html#applying-optional-parameters-to-pytrees).
|
||||
constraints: as for :func:`jax.export.symbolic_shape`.
|
||||
scope: as for :func:`jax.export.symbolic_shape`.
|
||||
|
||||
@ -2038,7 +2038,7 @@ def _solve_dim_equations(
|
||||
" Using the following polymorphic shapes specifications: " +
|
||||
",".join(f"{arg_name}.shape = {arg_spec}"
|
||||
for arg_name, arg_spec in polymorphic_shape_specs)) + "."
|
||||
solution_err_msg_trailer_errors = ". Please see https://jax.readthedocs.io/en/latest/export/shape_poly.html#shape-assertion-errors for more details."
|
||||
solution_err_msg_trailer_errors = ". Please see https://docs.jax.dev/en/latest/export/shape_poly.html#shape-assertion-errors for more details."
|
||||
|
||||
shape_constraints = ShapeConstraints() # accumulate shape constraints
|
||||
scope: SymbolicScope | None = None
|
||||
@ -2171,6 +2171,6 @@ def _solve_dim_equations(
|
||||
" Unprocessed specifications: " +
|
||||
", ".join(f"'{eqn.aval_dim_expr}' for dimension size {eqn.dim_name}"
|
||||
for eqn in eqns) +
|
||||
". Please see https://jax.readthedocs.io/en/latest/export/shape_poly.html#dimension-variables-must-be-solvable-from-the-input-shapes for more details."
|
||||
". Please see https://docs.jax.dev/en/latest/export/shape_poly.html#dimension-variables-must-be-solvable-from-the-input-shapes for more details."
|
||||
)
|
||||
raise ValueError(err_msg)
|
||||
|
@ -41,7 +41,7 @@ def ravel_pytree(pytree):
|
||||
component of the output.
|
||||
|
||||
For details on dtype promotion, see
|
||||
https://jax.readthedocs.io/en/latest/type_promotion.html.
|
||||
https://docs.jax.dev/en/latest/type_promotion.html.
|
||||
|
||||
"""
|
||||
leaves, treedef = tree_flatten(pytree)
|
||||
|
@ -677,7 +677,7 @@ class LoweringParameters:
|
||||
# Signals that we are lowering for exporting.
|
||||
|
||||
for_export: bool = False
|
||||
# See usage in https://jax.readthedocs.io/en/latest/export/export.html#ensuring-forward-and-backward-compatibility
|
||||
# See usage in https://docs.jax.dev/en/latest/export/export.html#ensuring-forward-and-backward-compatibility
|
||||
# We have this here to ensure it is reflected in the cache keys
|
||||
export_ignore_forward_compatibility: bool = False
|
||||
|
||||
@ -1179,7 +1179,7 @@ def lower_jaxpr_to_module(
|
||||
donated_args[input_id] = False
|
||||
if any(donated_args):
|
||||
unused_donations = [str(a) for a, d in zip(in_avals, donated_args) if d]
|
||||
msg = "See an explanation at https://jax.readthedocs.io/en/latest/faq.html#buffer-donation."
|
||||
msg = "See an explanation at https://docs.jax.dev/en/latest/faq.html#buffer-donation."
|
||||
if not platforms_with_donation:
|
||||
msg = f"Donation is not implemented for {platforms}.\n{msg}"
|
||||
if unused_donations:
|
||||
|
@ -1953,7 +1953,7 @@ def composite_jvp(*args, **_):
|
||||
raise ValueError(
|
||||
"JVP rule for composite not implemented. You can use `jax.custom_jvp` to "
|
||||
"add support. See "
|
||||
"https://jax.readthedocs.io/en/latest/_autosummary/jax.custom_jvp.html"
|
||||
"https://docs.jax.dev/en/latest/_autosummary/jax.custom_jvp.html"
|
||||
)
|
||||
|
||||
|
||||
@ -1962,7 +1962,7 @@ def composite_transpose(*args, **_):
|
||||
raise ValueError(
|
||||
"Transpose rule for composite not implemented. You can use"
|
||||
"`jax.custom_jvp` or `jax.custom_vjp` to add support. See "
|
||||
"https://jax.readthedocs.io/en/latest/_autosummary/jax.custom_jvp.html"
|
||||
"https://docs.jax.dev/en/latest/_autosummary/jax.custom_jvp.html"
|
||||
)
|
||||
|
||||
|
||||
|
@ -214,7 +214,7 @@ class Mesh(_BaseMesh, contextlib.ContextDecorator):
|
||||
"""Declare the hardware resources available in the scope of this manager.
|
||||
|
||||
See the Distributed arrays and automatic parallelization tutorial
|
||||
(https://jax.readthedocs.io/en/latest/notebooks/Distributed_arrays_and_automatic_parallelization.html)
|
||||
(https://docs.jax.dev/en/latest/notebooks/Distributed_arrays_and_automatic_parallelization.html)
|
||||
and Explicit sharding tutorial (https://docs.jax.dev/en/latest/notebooks/explicit-sharding.html)
|
||||
|
||||
Args:
|
||||
|
@ -92,7 +92,7 @@ class NamedSharding(JSharding.Sharding):
|
||||
across ``y`` axis of the mesh.
|
||||
|
||||
The Distributed arrays and automatic parallelization
|
||||
(https://jax.readthedocs.io/en/latest/notebooks/Distributed_arrays_and_automatic_parallelization.html#namedsharding-gives-a-way-to-express-shardings-with-names)
|
||||
(https://docs.jax.dev/en/latest/notebooks/Distributed_arrays_and_automatic_parallelization.html#namedsharding-gives-a-way-to-express-shardings-with-names)
|
||||
tutorial has more details and diagrams that explain how
|
||||
:class:`Mesh` and :class:`PartitionSpec` are used.
|
||||
|
||||
|
@ -588,7 +588,7 @@ def _defer_to_unrecognized_arg(opchar, binary_op, swap=False):
|
||||
def _unimplemented_setitem(self, i, x):
|
||||
msg = ("JAX arrays are immutable and do not support in-place item assignment."
|
||||
" Instead of x[idx] = y, use x = x.at[idx].set(y) or another .at[] method:"
|
||||
" https://jax.readthedocs.io/en/latest/_autosummary/jax.numpy.ndarray.at.html")
|
||||
" https://docs.jax.dev/en/latest/_autosummary/jax.numpy.ndarray.at.html")
|
||||
raise TypeError(msg.format(type(self)))
|
||||
|
||||
def _operator_round(number: ArrayLike, ndigits: int | None = None) -> Array:
|
||||
|
@ -552,7 +552,7 @@ def result_type(*args: Any) -> DType:
|
||||
|
||||
For details on 64-bit values, refer to `Sharp bits - double precision`_:
|
||||
|
||||
.. _Sharp bits - double precision: https://jax.readthedocs.io/en/latest/notebooks/Common_Gotchas_in_JAX.html#double-64bit-precision
|
||||
.. _Sharp bits - double precision: https://docs.jax.dev/en/latest/notebooks/Common_Gotchas_in_JAX.html#double-64bit-precision
|
||||
"""
|
||||
return dtypes.result_type(*args)
|
||||
|
||||
@ -2814,7 +2814,7 @@ def where(condition, x=None, y=None, /, *, size=None, fill_value=None):
|
||||
(reverse-mode differentiation), a NaN in either ``x`` or ``y`` will propagate into the
|
||||
gradient, regardless of the value of ``condition``. More information on this behavior
|
||||
and workarounds is available in the `JAX FAQ
|
||||
<https://jax.readthedocs.io/en/latest/faq.html#gradients-contain-nan-where-using-where>`_.
|
||||
<https://docs.jax.dev/en/latest/faq.html#gradients-contain-nan-where-using-where>`_.
|
||||
|
||||
Examples:
|
||||
When ``x`` and ``y`` are not provided, ``where`` behaves equivalently to
|
||||
@ -5903,14 +5903,14 @@ def fromfile(*args, **kwargs):
|
||||
``jnp.asarray(np.fromfile(...))`` instead, although care should be taken if ``np.fromfile``
|
||||
is used within jax transformations because of its potential side-effect of consuming the
|
||||
file object; for more information see `Common Gotchas: Pure Functions
|
||||
<https://jax.readthedocs.io/en/latest/notebooks/Common_Gotchas_in_JAX.html#pure-functions>`_.
|
||||
<https://docs.jax.dev/en/latest/notebooks/Common_Gotchas_in_JAX.html#pure-functions>`_.
|
||||
"""
|
||||
raise NotImplementedError(
|
||||
"jnp.fromfile() is not implemented because it may be non-pure and thus unsafe for use "
|
||||
"with JIT and other JAX transformations. Consider using jnp.asarray(np.fromfile(...)) "
|
||||
"instead, although care should be taken if np.fromfile is used within a jax transformations "
|
||||
"because of its potential side-effect of consuming the file object; for more information see "
|
||||
"https://jax.readthedocs.io/en/latest/notebooks/Common_Gotchas_in_JAX.html#pure-functions")
|
||||
"https://docs.jax.dev/en/latest/notebooks/Common_Gotchas_in_JAX.html#pure-functions")
|
||||
|
||||
|
||||
@export
|
||||
@ -5922,14 +5922,14 @@ def fromiter(*args, **kwargs):
|
||||
``jnp.asarray(np.fromiter(...))`` instead, although care should be taken if ``np.fromiter``
|
||||
is used within jax transformations because of its potential side-effect of consuming the
|
||||
iterable object; for more information see `Common Gotchas: Pure Functions
|
||||
<https://jax.readthedocs.io/en/latest/notebooks/Common_Gotchas_in_JAX.html#pure-functions>`_.
|
||||
<https://docs.jax.dev/en/latest/notebooks/Common_Gotchas_in_JAX.html#pure-functions>`_.
|
||||
"""
|
||||
raise NotImplementedError(
|
||||
"jnp.fromiter() is not implemented because it may be non-pure and thus unsafe for use "
|
||||
"with JIT and other JAX transformations. Consider using jnp.asarray(np.fromiter(...)) "
|
||||
"instead, although care should be taken if np.fromiter is used within a jax transformations "
|
||||
"because of its potential side-effect of consuming the iterable object; for more information see "
|
||||
"https://jax.readthedocs.io/en/latest/notebooks/Common_Gotchas_in_JAX.html#pure-functions")
|
||||
"https://docs.jax.dev/en/latest/notebooks/Common_Gotchas_in_JAX.html#pure-functions")
|
||||
|
||||
|
||||
@export
|
||||
|
@ -70,13 +70,13 @@ def _rank_promotion_warning_or_error(fun_name: str, shapes: Sequence[Shape]):
|
||||
msg = ("Following NumPy automatic rank promotion for {} on shapes {}. "
|
||||
"Set the jax_numpy_rank_promotion config option to 'allow' to "
|
||||
"disable this warning; for more information, see "
|
||||
"https://jax.readthedocs.io/en/latest/rank_promotion_warning.html.")
|
||||
"https://docs.jax.dev/en/latest/rank_promotion_warning.html.")
|
||||
warnings.warn(msg.format(fun_name, ' '.join(map(str, shapes))))
|
||||
elif config.numpy_rank_promotion.value == "raise":
|
||||
msg = ("Operands could not be broadcast together for {} on shapes {} "
|
||||
"and with the config option jax_numpy_rank_promotion='raise'. "
|
||||
"For more information, see "
|
||||
"https://jax.readthedocs.io/en/latest/rank_promotion_warning.html.")
|
||||
"https://docs.jax.dev/en/latest/rank_promotion_warning.html.")
|
||||
raise ValueError(msg.format(fun_name, ' '.join(map(str, shapes))))
|
||||
|
||||
|
||||
|
@ -307,7 +307,7 @@ def vectorize(pyfunc, *, excluded=frozenset(), signature=None):
|
||||
f" promotion for jnp.vectorize function with signature {signature}."
|
||||
" Set the jax_numpy_rank_promotion config option to 'allow' to"
|
||||
" disable this message; for more information, see"
|
||||
" https://jax.readthedocs.io/en/latest/rank_promotion_warning.html.")
|
||||
" https://docs.jax.dev/en/latest/rank_promotion_warning.html.")
|
||||
if config.numpy_rank_promotion.value == "warn":
|
||||
warnings.warn(msg)
|
||||
elif config.numpy_rank_promotion.value == "raise":
|
||||
|
@ -576,7 +576,7 @@ def _check_block_mappings(
|
||||
# TODO(necula): add index_map source location info
|
||||
f"and index_map {bm.index_map_jaxpr.jaxpr}, in "
|
||||
f"memory space {bm.block_aval.memory_space}."
|
||||
"\nSee details at https://jax.readthedocs.io/en/latest/pallas/grid_blockspec.html#pallas-blockspec")
|
||||
"\nSee details at https://docs.jax.dev/en/latest/pallas/grid_blockspec.html#pallas-blockspec")
|
||||
if rank < 1:
|
||||
raise ValueError(
|
||||
"The Pallas TPU lowering currently supports only blocks of "
|
||||
|
@ -473,7 +473,7 @@ def _check_block_mappings(
|
||||
f" and index_map {bm.index_map_jaxpr.jaxpr} in"
|
||||
f" memory space {bm.transformed_block_aval.memory_space}."
|
||||
" See details at"
|
||||
" https://jax.readthedocs.io/en/latest/pallas/grid_blockspec.html#pallas-blockspec."
|
||||
" https://docs.jax.dev/en/latest/pallas/grid_blockspec.html#pallas-blockspec."
|
||||
)
|
||||
|
||||
for bm in block_mappings:
|
||||
|
@ -1234,7 +1234,7 @@ def _unsupported_lowering_error(platform: str) -> Exception:
|
||||
f"Cannot lower pallas_call on platform: {platform}. To use Pallas on GPU,"
|
||||
" install jaxlib GPU 0.4.24 or newer. To use Pallas on TPU, install"
|
||||
" jaxlib TPU and libtpu. See"
|
||||
" https://jax.readthedocs.io/en/latest/installation.html."
|
||||
" https://docs.jax.dev/en/latest/installation.html."
|
||||
)
|
||||
|
||||
_Backend = Literal["mosaic_tpu", "triton", "mosaic_gpu"]
|
||||
@ -1489,7 +1489,7 @@ def pallas_call(
|
||||
) -> Callable[..., Any]:
|
||||
"""Invokes a Pallas kernel on some inputs.
|
||||
|
||||
See `Pallas Quickstart <https://jax.readthedocs.io/en/latest/pallas/quickstart.html>`_.
|
||||
See `Pallas Quickstart <https://docs.jax.dev/en/latest/pallas/quickstart.html>`_.
|
||||
|
||||
Args:
|
||||
kernel: the kernel function, that receives a Ref for each input and output.
|
||||
|
@ -953,7 +953,7 @@ def pjit(
|
||||
be donated.
|
||||
|
||||
For more details on buffer donation see the
|
||||
`FAQ <https://jax.readthedocs.io/en/latest/faq.html#buffer-donation>`_.
|
||||
`FAQ <https://docs.jax.dev/en/latest/faq.html#buffer-donation>`_.
|
||||
donate_argnames: An optional string or collection of strings specifying
|
||||
which named arguments are donated to the computation. See the
|
||||
comment on ``donate_argnums`` for details. If not
|
||||
@ -1269,7 +1269,7 @@ def explain_tracing_cache_miss(
|
||||
if add_weak_type_hint:
|
||||
p('where weak_type=True often means a Python builtin numeric value, and ')
|
||||
p('weak_type=False means a jax.Array.')
|
||||
p('See https://jax.readthedocs.io/en/latest/type_promotion.html#weak-types')
|
||||
p('See https://docs.jax.dev/en/latest/type_promotion.html#weak-types')
|
||||
return done()
|
||||
|
||||
# we think this is unreachable...
|
||||
@ -2564,7 +2564,7 @@ def with_sharding_constraint(x, shardings):
|
||||
Returns:
|
||||
x_with_shardings: PyTree of jax.Arrays with specified sharding constraints.
|
||||
|
||||
.. _Distributed arrays and automatic parallelization: https://jax.readthedocs.io/en/latest/notebooks/Distributed_arrays_and_automatic_parallelization.html
|
||||
.. _Distributed arrays and automatic parallelization: https://docs.jax.dev/en/latest/notebooks/Distributed_arrays_and_automatic_parallelization.html
|
||||
"""
|
||||
x_flat, tree = tree_flatten(x)
|
||||
|
||||
|
@ -225,7 +225,7 @@ def PRNGKey(seed: int | ArrayLike, *,
|
||||
|
||||
This function produces old-style legacy PRNG keys, which are arrays
|
||||
of dtype ``uint32``. For more, see the note in the `PRNG keys
|
||||
<https://jax.readthedocs.io/en/latest/jax.random.html#prng-keys>`_
|
||||
<https://docs.jax.dev/en/latest/jax.random.html#prng-keys>`_
|
||||
section. When possible, :func:`jax.random.key` is recommended for
|
||||
use instead.
|
||||
|
||||
|
@ -134,7 +134,7 @@ def tpu_client_timer_callback(timer_secs: float) -> xla_client.Client | None:
|
||||
warnings.warn(
|
||||
f'TPU backend initialization is taking more than {timer_secs} seconds. '
|
||||
'Did you run your code on all TPU hosts? '
|
||||
'See https://jax.readthedocs.io/en/latest/multi_process.html '
|
||||
'See https://docs.jax.dev/en/latest/multi_process.html '
|
||||
'for more information.')
|
||||
|
||||
# Will log a warning after `timer_secs`.
|
||||
@ -290,7 +290,7 @@ def _check_cuda_compute_capability(devices_to_check):
|
||||
f"Device {idx} has CUDA compute capability {compute_cap/10} which is "
|
||||
"lower than the minimum supported compute capability "
|
||||
f"{MIN_COMPUTE_CAPABILITY/10}. See "
|
||||
"https://jax.readthedocs.io/en/latest/installation.html#nvidia-gpu for "
|
||||
"https://docs.jax.dev/en/latest/installation.html#nvidia-gpu for "
|
||||
"more details",
|
||||
RuntimeWarning
|
||||
)
|
||||
@ -899,7 +899,7 @@ def _suggest_missing_backends():
|
||||
warning_msg += (
|
||||
"This may be due to JAX pre-allocating too much device "
|
||||
"memory, leaving too little for CUDA library initialization. See "
|
||||
"https://jax.readthedocs.io/en/latest/gpu_memory_allocation.html "
|
||||
"https://docs.jax.dev/en/latest/gpu_memory_allocation.html "
|
||||
"for more details and potential workarounds."
|
||||
)
|
||||
warning_msg += "(Set TF_CPP_MIN_LOG_LEVEL=0 and rerun for more info.)"
|
||||
|
16
jax/core.py
16
jax/core.py
@ -99,32 +99,32 @@ _deprecations = {
|
||||
# Added 2024-12-10
|
||||
"full_lower": ("jax.core.full_lower is deprecated. It is a no-op as of JAX v0.4.36.", None),
|
||||
"jaxpr_as_fun": ("jax.core.jaxpr_as_fun was removed in JAX v0.6.0. Use jax.extend.core.jaxpr_as_fun instead, "
|
||||
"and see https://jax.readthedocs.io/en/latest/jax.extend.html for details.",
|
||||
"and see https://docs.jax.dev/en/latest/jax.extend.html for details.",
|
||||
None),
|
||||
"lattice_join": ("jax.core.lattice_join is deprecated. It is a no-op as of JAX v0.4.36.", None),
|
||||
# Finalized 2025-03-25 for JAX v0.6.0; remove after 2025-06-25
|
||||
"AxisSize": ("jax.core.AxisSize was removed in JAX v0.6.0.", None),
|
||||
"ClosedJaxpr": ("jax.core.ClosedJaxpr was removed in JAX v0.6.0. Use jax.extend.core.ClosedJaxpr instead, "
|
||||
"and see https://jax.readthedocs.io/en/latest/jax.extend.html for details.", None),
|
||||
"and see https://docs.jax.dev/en/latest/jax.extend.html for details.", None),
|
||||
"EvalTrace": ("jax.core.EvalTrace was removed in JAX v0.6.0.", None),
|
||||
"InDBIdx": ("jax.core.InDBIdx was removed in JAX v0.6.0.", None),
|
||||
"InputType": ("jax.core.InputType was removed in JAX v0.6.0.", None),
|
||||
"Jaxpr": ("jax.core.Jaxpr was removed in JAX v0.6.0. Use jax.extend.core.Jaxpr instead, "
|
||||
"and see https://jax.readthedocs.io/en/latest/jax.extend.html for details.", None),
|
||||
"and see https://docs.jax.dev/en/latest/jax.extend.html for details.", None),
|
||||
"JaxprEqn": ("jax.core.JaxprEqn was removed in JAX v0.6.0. Use jax.extend.core.JaxprEqn instead, "
|
||||
"and see https://jax.readthedocs.io/en/latest/jax.extend.html for details.", None),
|
||||
"and see https://docs.jax.dev/en/latest/jax.extend.html for details.", None),
|
||||
"Literal": ("jax.core.Literal was removed in JAX v0.6.0. Use jax.extend.core.Literal instead, "
|
||||
"and see https://jax.readthedocs.io/en/latest/jax.extend.html for details.", None),
|
||||
"and see https://docs.jax.dev/en/latest/jax.extend.html for details.", None),
|
||||
"MapPrimitive": ("jax.core.MapPrimitive was removed in JAX v0.6.0.", None),
|
||||
"OpaqueTraceState": ("jax.core.OpaqueTraceState was removed in JAX v0.6.0.", None),
|
||||
"OutDBIdx": ("jax.core.OutDBIdx was removed in JAX v0.6.0.", None),
|
||||
"Primitive": ("jax.core.Primitive was removed in JAX v0.6.0. Use jax.extend.core.Primitive instead, "
|
||||
"and see https://jax.readthedocs.io/en/latest/jax.extend.html for details.", None),
|
||||
"and see https://docs.jax.dev/en/latest/jax.extend.html for details.", None),
|
||||
"Token": ("jax.core.Token was removed in JAX v0.6.0. Use jax.extend.core.Token instead, "
|
||||
"and see https://jax.readthedocs.io/en/latest/jax.extend.html for details.", None),
|
||||
"and see https://docs.jax.dev/en/latest/jax.extend.html for details.", None),
|
||||
"TRACER_LEAK_DEBUGGER_WARNING": ("jax.core.TRACER_LEAK_DEBUGGER_WARNING was removed in JAX v0.6.0.", None),
|
||||
"Var": ("jax.core.Var was removed in JAX v0.6.0. Use jax.extend.core.Var instead, "
|
||||
"and see https://jax.readthedocs.io/en/latest/jax.extend.html for details.", None),
|
||||
"and see https://docs.jax.dev/en/latest/jax.extend.html for details.", None),
|
||||
"concrete_aval": ("jax.core.concrete_aval was removed in JAX v0.6.0.", None),
|
||||
"dedup_referents": ("jax.core.dedup_referents was removed in JAX v0.6.0.", None),
|
||||
"escaped_tracer_error": ("jax.core.escaped_tracer_error was removed in JAX v0.6.0.", None),
|
||||
|
@ -16,7 +16,7 @@
|
||||
.. warning::
|
||||
The host_callback APIs are deprecated as of March 20, 2024.
|
||||
The functionality is subsumed by the
|
||||
`new JAX external callbacks <https://jax.readthedocs.io/en/latest/notebooks/external_callbacks.html>`_
|
||||
`new JAX external callbacks <https://docs.jax.dev/en/latest/notebooks/external_callbacks.html>`_
|
||||
See https://github.com/jax-ml/jax/issues/20385.
|
||||
|
||||
"""
|
||||
|
@ -138,7 +138,7 @@ f_tf_graph = tf.function(f_tf, autograph=False)
|
||||
```
|
||||
|
||||
Note that when using the default native serialization, the target JAX function
|
||||
must be jittable (see [JAX - The Sharp Bits](https://jax.readthedocs.io/en/latest/notebooks/Common_Gotchas_in_JAX.html)).
|
||||
must be jittable (see [JAX - The Sharp Bits](https://docs.jax.dev/en/latest/notebooks/Common_Gotchas_in_JAX.html)).
|
||||
In the native serialization mode, under TensorFlow eager
|
||||
the whole JAX function executes as one op.
|
||||
|
||||
@ -461,7 +461,7 @@ presence of shape polymorphism, some dimensions may be dimension variables.
|
||||
The `polymorphic_shapes` parameter must be either `None`,
|
||||
or a pytree of shape specifiers corresponding to the pytree of arguments.
|
||||
(A value `None` for `polymorphic_shapes` is equivalent to a list of `None`.
|
||||
See [how optional parameters are matched to arguments](https://jax.readthedocs.io/en/latest/pytrees.html#applying-optional-parameters-to-pytrees).)
|
||||
See [how optional parameters are matched to arguments](https://docs.jax.dev/en/latest/pytrees.html#applying-optional-parameters-to-pytrees).)
|
||||
A shape specifier is combined with a `TensorSpec` as follows:
|
||||
|
||||
* A shape specifier of `None` means that the shape is given
|
||||
@ -1024,7 +1024,7 @@ always behaves like the JAX function.
|
||||
|
||||
JAX interprets the type of Python scalars differently based on
|
||||
`JAX_ENABLE_X64` flag. (See
|
||||
[JAX - The Sharp Bits: Double (64bit) precision](https://jax.readthedocs.io/en/latest/notebooks/Common_Gotchas_in_JAX.html#double-64bit-precision).)
|
||||
[JAX - The Sharp Bits: Double (64bit) precision](https://docs.jax.dev/en/latest/notebooks/Common_Gotchas_in_JAX.html#double-64bit-precision).)
|
||||
In the default configuration, the
|
||||
flag is unset, and JAX interprets Python constants as 32-bit,
|
||||
e.g., the type of `3.14` is `float32`. This is also what
|
||||
@ -1086,7 +1086,7 @@ Applies to both native and non-native serialization.
|
||||
|
||||
`jax2tf` can lower functions with arguments and results that are nested
|
||||
collections (tuples, lists, dictionaries) of numeric values or JAX arrays
|
||||
([pytrees](https://jax.readthedocs.io/en/latest/pytrees.html)). The
|
||||
([pytrees](https://docs.jax.dev/en/latest/pytrees.html)). The
|
||||
resulting TensorFlow function will take the same kind of arguments except the
|
||||
leaves can be numeric values or TensorFlow tensors (`tf.Tensor`, `tf.TensorSpec`, `tf.Variable`).
|
||||
|
||||
@ -1285,7 +1285,7 @@ per PRNG operation. The "unsafe" part is that it doesn't guarantee
|
||||
determinism across JAX/XLA versions, and the quality of random
|
||||
streams it generates from different keys is less well understood.
|
||||
Nevertheless, this should be fine for most inference/serving cases.
|
||||
See more details in the [JAX PRNG documentation](https://jax.readthedocs.io/en/latest/jax.random.html?highlight=unsafe_rbg#advanced-rng-configuration).
|
||||
See more details in the [JAX PRNG documentation](https://docs.jax.dev/en/latest/jax.random.html?highlight=unsafe_rbg#advanced-rng-configuration).
|
||||
|
||||
### SavedModel supports only first-order gradients
|
||||
|
||||
|
@ -24,7 +24,7 @@ partial support.
|
||||
For a detailed description of these XLA ops, please see the
|
||||
[XLA Operation Semantics documentation](https://www.tensorflow.org/xla/operation_semantics).
|
||||
|
||||
| XLA ops ([documentation](https://www.tensorflow.org/xla/operation_semantics)) | JAX primitive(s) ([documentation](https://jax.readthedocs.io/en/latest/jax.lax.html)) | Supported |
|
||||
| XLA ops ([documentation](https://www.tensorflow.org/xla/operation_semantics)) | JAX primitive(s) ([documentation](https://docs.jax.dev/en/latest/jax.lax.html)) | Supported |
|
||||
| ------- | ---------------- | ------- |
|
||||
| XlaDot | `lax.dot_general` | Full |
|
||||
| XlaDynamicSlice | `lax.dynamic_slice` | Full |
|
||||
@ -47,7 +47,7 @@ support and which not.
|
||||
### XlaConv
|
||||
|
||||
JAX convolutions are done using
|
||||
[`lax.conv_general_dilated`](https://jax.readthedocs.io/en/latest/_autosummary/jax.lax.conv_general_dilated.html).
|
||||
[`lax.conv_general_dilated`](https://docs.jax.dev/en/latest/_autosummary/jax.lax.conv_general_dilated.html).
|
||||
|
||||
```
|
||||
lax.conv_general_dilated(
|
||||
@ -88,7 +88,7 @@ instance, parallelization primitives `vmap` and `pmap` use gather to specify a
|
||||
batch dimension, and it is used for slices or multidimensional indexing as well,
|
||||
e.g. `x[0, 1]`, `x[:, :1]`, or `x[[0], [1]]`.
|
||||
|
||||
The signature of [`lax.gather`](https://jax.readthedocs.io/en/latest/_autosummary/jax.lax.gather.html#jax.lax.gather)
|
||||
The signature of [`lax.gather`](https://docs.jax.dev/en/latest/_autosummary/jax.lax.gather.html#jax.lax.gather)
|
||||
is as follows:
|
||||
|
||||
```
|
||||
@ -128,7 +128,7 @@ All other cases of `lax.gather` are currently not supported.
|
||||
|
||||
### XlaReduceWindow
|
||||
|
||||
The signature of [`lax.reduce_window`](https://jax.readthedocs.io/en/latest/_autosummary/jax.lax.reduce_window.html)
|
||||
The signature of [`lax.reduce_window`](https://docs.jax.dev/en/latest/_autosummary/jax.lax.reduce_window.html)
|
||||
is as follows:
|
||||
|
||||
```
|
||||
|
@ -272,7 +272,7 @@ def convert(fun_jax: Callable,
|
||||
should be `None` (monomorphic argument), or a Python object with the
|
||||
same pytree structure as the argument.
|
||||
See [how optional parameters are matched to
|
||||
arguments](https://jax.readthedocs.io/en/latest/pytrees.html#applying-optional-parameters-to-pytrees).
|
||||
arguments](https://docs.jax.dev/en/latest/pytrees.html#applying-optional-parameters-to-pytrees).
|
||||
|
||||
A shape specification for an array argument should be an object
|
||||
`PolyShape(dim0, dim1, ..., dimn)`
|
||||
|
@ -595,7 +595,7 @@ class ShapePolyTest(tf_test_util.JaxToTfTestCase):
|
||||
"Using the following polymorphic shapes specifications: args[0].shape = (2*b + a, a, c + b + a). "
|
||||
"Obtained dimension variables: 'a' = 2 from specification 'a' for dimension args[0].shape[1] (= 2), "
|
||||
"'b' = 0 from specification '2*b + a' for dimension args[0].shape[0] (= 2), . "
|
||||
"Please see https://jax.readthedocs.io/en/latest/export/shape_poly.html#shape-assertion-errors for more details."
|
||||
"Please see https://docs.jax.dev/en/latest/export/shape_poly.html#shape-assertion-errors for more details."
|
||||
)),
|
||||
dict(shape=(3, 2, 6), # a = 2, b = 0.5, c = 4 - b is not integer
|
||||
poly_spec="(a + 2*b, a, a + b + c)",
|
||||
@ -604,7 +604,7 @@ class ShapePolyTest(tf_test_util.JaxToTfTestCase):
|
||||
"Division had remainder 1 when computing the value of 'b'. "
|
||||
"Using the following polymorphic shapes specifications: args[0].shape = (2*b + a, a, c + b + a). "
|
||||
"Obtained dimension variables: 'a' = 2 from specification 'a' for dimension args[0].shape[1] (= 2), . "
|
||||
"Please see https://jax.readthedocs.io/en/latest/export/shape_poly.html#shape-assertion-errors for more details."
|
||||
"Please see https://docs.jax.dev/en/latest/export/shape_poly.html#shape-assertion-errors for more details."
|
||||
)),
|
||||
dict(shape=(8, 2, 6), # a = 2, b = 3 - inconsistency
|
||||
poly_spec="(a + 2*b, a, a + b)",
|
||||
@ -614,7 +614,7 @@ class ShapePolyTest(tf_test_util.JaxToTfTestCase):
|
||||
"Using the following polymorphic shapes specifications: args[0].shape = (2*b + a, a, b + a). "
|
||||
"Obtained dimension variables: 'a' = 2 from specification 'a' for dimension args[0].shape[1] (= 2), "
|
||||
"'b' = 4 from specification 'b + a' for dimension args[0].shape[2] (= 6), . "
|
||||
"Please see https://jax.readthedocs.io/en/latest/export/shape_poly.html#shape-assertion-errors for more details."
|
||||
"Please see https://docs.jax.dev/en/latest/export/shape_poly.html#shape-assertion-errors for more details."
|
||||
)),
|
||||
dict(shape=(7, 2, 36), # a = 2, b = 3, c = 6 - cannot solve c
|
||||
poly_spec="(2 * a + b, a, c * c)",
|
||||
@ -623,7 +623,7 @@ class ShapePolyTest(tf_test_util.JaxToTfTestCase):
|
||||
"We can only solve linear uni-variate constraints. "
|
||||
"Using the following polymorphic shapes specifications: args[0].shape = (b + 2*a, a, c^2). "
|
||||
"Unprocessed specifications: 'c^2' for dimension size args[0].shape[2]. "
|
||||
"Please see https://jax.readthedocs.io/en/latest/export/shape_poly.html#dimension-variables-must-be-solvable-from-the-input-shapes for more details."
|
||||
"Please see https://docs.jax.dev/en/latest/export/shape_poly.html#dimension-variables-must-be-solvable-from-the-input-shapes for more details."
|
||||
)),
|
||||
])
|
||||
def test_shape_constraints_errors(self, *,
|
||||
|
@ -15,7 +15,7 @@
|
||||
"""Module for Pallas, a JAX extension for custom kernels.
|
||||
|
||||
See the Pallas documentation at
|
||||
https://jax.readthedocs.io/en/latest/pallas.html.
|
||||
https://docs.jax.dev/en/latest/pallas.html.
|
||||
"""
|
||||
|
||||
from jax._src.pallas.core import Blocked as Blocked
|
||||
|
@ -14,7 +14,7 @@
|
||||
|
||||
"""Example matmul TPU kernel.
|
||||
|
||||
See discussion in https://jax.readthedocs.io/en/latest/pallas/tpu/matmul.html.
|
||||
See discussion in https://docs.jax.dev/en/latest/pallas/tpu/matmul.html.
|
||||
"""
|
||||
|
||||
import functools
|
||||
|
@ -136,7 +136,7 @@ def shard_map(f: Callable, mesh: Mesh | AbstractMesh, in_specs: Specs,
|
||||
Examples:
|
||||
For examples, refer to :ref:`sharded-computation` or `SPMD multi-device parallelism with shard_map`_.
|
||||
|
||||
.. _SPMD multi-device parallelism with shard_map: https://jax.readthedocs.io/en/latest/notebooks/shard_map.html
|
||||
.. _SPMD multi-device parallelism with shard_map: https://docs.jax.dev/en/latest/notebooks/shard_map.html
|
||||
"""
|
||||
return _shard_map(f, mesh, in_specs, out_specs, check_rep, auto)
|
||||
|
||||
|
Some files were not shown because too many files have changed in this diff Show More
Loading…
x
Reference in New Issue
Block a user