mirror of
https://github.com/ROCm/jax.git
synced 2025-04-14 10:56:06 +00:00
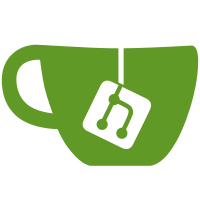
This avoids a bunch of list bookkeeping in cases where we are iterating only for a side effect and do not care about the results. I would have named this iter() to match OCaml's list.iter(), but unfortunately iter() is a Python builtin. PiperOrigin-RevId: 736859418
286 lines
8.0 KiB
Python
286 lines
8.0 KiB
Python
# Copyright 2018 The JAX Authors.
|
|
#
|
|
# Licensed under the Apache License, Version 2.0 (the "License");
|
|
# you may not use this file except in compliance with the License.
|
|
# You may obtain a copy of the License at
|
|
#
|
|
# https://www.apache.org/licenses/LICENSE-2.0
|
|
#
|
|
# Unless required by applicable law or agreed to in writing, software
|
|
# distributed under the License is distributed on an "AS IS" BASIS,
|
|
# WITHOUT WARRANTIES OR CONDITIONS OF ANY KIND, either express or implied.
|
|
# See the License for the specific language governing permissions and
|
|
# limitations under the License.
|
|
|
|
|
|
from collections import namedtuple
|
|
from functools import partial
|
|
import numpy.random as npr
|
|
|
|
from absl.testing import absltest
|
|
from absl.testing import parameterized
|
|
|
|
import itertools as it
|
|
import jax.numpy as jnp
|
|
import jax
|
|
from jax import jit, jvp, vjp
|
|
import jax._src.test_util as jtu
|
|
|
|
jax.config.parse_flags_with_absl()
|
|
|
|
npr.seed(0)
|
|
|
|
from jax._src.util import foreach, unzip2, safe_zip, safe_map
|
|
|
|
map = safe_map
|
|
zip = safe_zip
|
|
|
|
subfun_prob = 0.5
|
|
thin_prob = 0.1
|
|
size_reduction_factor = 3
|
|
|
|
Eqn = namedtuple('Eqn', ['in_vars', 'out_vars', 'fun'])
|
|
Prim = namedtuple('Prim', ['fun'])
|
|
ArrayType = namedtuple('ArrayType', ['shape', 'dtype'])
|
|
Var = namedtuple('Var', ['name', 'vartype'])
|
|
Fun = namedtuple('Fun', ['in_vars', 'out_vars', 'eqns'])
|
|
|
|
def gen_fun_and_types(size):
|
|
in_types = [gen_array_type(size) for _ in range(gen_nonneg_int(size))]
|
|
fun, _ = gen_function(size, in_types)
|
|
return fun
|
|
|
|
def gen_function(size, in_types):
|
|
eqns = []
|
|
in_vars = map(fresh_var, in_types)
|
|
cur_vars = in_vars[:]
|
|
for _ in range(gen_nonneg_int(size)):
|
|
if not cur_vars:
|
|
break
|
|
if npr.rand() < subfun_prob:
|
|
arg_vars = gen_subset(cur_vars)
|
|
arg_types = [v.vartype for v in arg_vars]
|
|
fun, out_types = gen_function(size / size_reduction_factor, arg_types)
|
|
fun = partial(eval_fun, fun)
|
|
fun = maybe_jit(fun, len(arg_types))
|
|
else:
|
|
arity = choice(list(primitive_generators))
|
|
arg_vars = gen_sized_subset(cur_vars, arity)
|
|
arg_types = [v.vartype for v in arg_vars]
|
|
prim_gen = weighted_choice(primitive_generators[arity])
|
|
fun, out_type = prim_gen(size, *arg_types)
|
|
fun = wrap_singleton(fun)
|
|
out_types = [out_type]
|
|
|
|
out_vars = map(fresh_var, out_types)
|
|
eqns.append(Eqn(arg_vars, out_vars, fun))
|
|
cur_vars.extend(out_vars)
|
|
cur_vars = thin(cur_vars, thin_prob)
|
|
|
|
out_vars = gen_subset(cur_vars)
|
|
return Fun(in_vars, out_vars, eqns), [v.vartype for v in out_vars]
|
|
|
|
def eval_fun(fun, *args):
|
|
def read(v):
|
|
return env[v]
|
|
def write(v, x):
|
|
env[v] = x
|
|
|
|
env = {}
|
|
foreach(write, fun.in_vars, args)
|
|
for in_vars, out_vars, f in fun.eqns:
|
|
out_vals = f(*map(read, in_vars))
|
|
foreach(write, out_vars, out_vals)
|
|
|
|
return map(read, fun.out_vars)
|
|
|
|
def maybe_jit(f, num_args):
|
|
static_argnums = thin(range(num_args), 0.5)
|
|
|
|
def fun(*args):
|
|
partial_args = list(args)
|
|
for i in static_argnums:
|
|
partial_args[i] = None
|
|
|
|
@jit
|
|
def jitted_fun(*partial_args):
|
|
full_args = list(partial_args)
|
|
for i in static_argnums:
|
|
full_args[i] = args[i]
|
|
return f(*full_args)
|
|
|
|
return jitted_fun(*partial_args)
|
|
|
|
return fun
|
|
|
|
counter = it.count()
|
|
def fresh_var(ty):
|
|
return Var(next(counter), ty)
|
|
|
|
def gen_array_type(size):
|
|
# TODO(dougalm): randomize this
|
|
return ArrayType((2,2), jnp.float32)
|
|
|
|
def gen_array_val(array_type):
|
|
# TODO(dougalm): different sizes and dtypes
|
|
return npr.randn(*array_type.shape)
|
|
|
|
def gen_neg(size, t):
|
|
return (lambda x: -x), t
|
|
|
|
def gen_trig(size, t):
|
|
op = choice([jnp.sin, jnp.cos])
|
|
return op, t
|
|
|
|
def gen_binop(size, t1, t2):
|
|
unifier, t_out = gen_broadcasting_unifier(t1, t2)
|
|
binop = choice([lambda x, y: x + y,
|
|
lambda x, y: x * y])
|
|
def unify_and_binop(x, y):
|
|
x_, y_ = unifier(x, y)
|
|
return binop(x_, y_)
|
|
|
|
return unify_and_binop, t_out
|
|
|
|
def thin(xs, p):
|
|
return [x for x in xs if npr.rand() > p]
|
|
|
|
def gen_broadcasting_unifier(t1, t2):
|
|
assert t1.shape == t2.shape
|
|
return lambda x, y: (x,y), t1
|
|
# TODO: generate slices and paddings to match shapes
|
|
|
|
def wrap_singleton(f):
|
|
return lambda *xs: (f(*xs),)
|
|
|
|
unary_primitive_generators = [
|
|
(3, gen_trig),
|
|
(1, gen_neg) ]
|
|
|
|
binary_primitive_generators = [
|
|
(1, gen_binop)]
|
|
|
|
primitive_generators = { 1: unary_primitive_generators,
|
|
2: binary_primitive_generators }
|
|
|
|
def gen_nonneg_int(size):
|
|
return npr.randint(size)
|
|
|
|
def choice(xs, weights=None):
|
|
# npr.choice isn't actually RS -> [a] -> a
|
|
# because it inspects the components to see if they're array-like
|
|
assert xs
|
|
n = len(xs)
|
|
if weights is None:
|
|
i = npr.randint(n)
|
|
else:
|
|
normalizer = float(sum(weights))
|
|
weights = [w / normalizer for w in weights]
|
|
i = npr.choice(range(n), p=weights)
|
|
return xs[i]
|
|
|
|
def weighted_choice(weighted_choices):
|
|
weights, choices = unzip2(weighted_choices)
|
|
return choice(choices, weights)
|
|
|
|
def gen_sized_subset(xs, size):
|
|
return [choice(xs) for _ in range(size)]
|
|
|
|
def gen_subset(xs):
|
|
if not xs:
|
|
return []
|
|
|
|
return gen_sized_subset(xs, npr.randint(len(xs) + 1))
|
|
|
|
def gen_vals(vs):
|
|
return [gen_array_val(v.vartype) for v in vs]
|
|
|
|
def inner_prod(xs, ys):
|
|
xys = zip(xs, ys)
|
|
assert all(x.shape == y.shape for x, y in xys)
|
|
return sum(jnp.sum(x * y) for x, y in xys)
|
|
|
|
def jvp_fd(fun, args, tangents):
|
|
EPS = 1e-3
|
|
def eval_eps(eps):
|
|
return fun(*[x if t is None else x + eps * t
|
|
for x, t in zip(args, tangents)])
|
|
|
|
ys_neg = eval_eps(-EPS)
|
|
ys_pos = eval_eps(EPS)
|
|
ys = eval_eps(0.0)
|
|
deriv = [(y_pos - y_neg) / (2 * EPS) for y_neg, y_pos in zip(ys_neg, ys_pos)]
|
|
return ys, deriv
|
|
|
|
def check_all_close(xs, ys, tol=1e-3):
|
|
for x, y in zip(xs, ys):
|
|
check_close(x, y, tol)
|
|
|
|
def check_close(x, y, tol=1e-3):
|
|
assert jnp.shape(x) == jnp.shape(y)
|
|
# TODO(dougalm): re-enable once we've tackled the less pendantic bugs
|
|
# assert x.dtype == y.dtype
|
|
assert jnp.allclose(x, y, rtol=tol, atol=tol), \
|
|
f"Value mismatch:\n{x}\n vs\n{y}\n"
|
|
|
|
def partial_argnums(f, args, dyn_argnums):
|
|
fixed_args = [None if i in dyn_argnums else arg for i, arg in enumerate(args)]
|
|
def f_(*dyn_args):
|
|
args = fixed_args[:]
|
|
for i, arg in zip(dyn_argnums, dyn_args):
|
|
args[i] = arg
|
|
return f(*args)
|
|
|
|
dyn_args = [args[i] for i in dyn_argnums]
|
|
return f_, dyn_args
|
|
|
|
|
|
class GeneratedFunTest(jtu.JaxTestCase):
|
|
"""Tests of transformations on randomly generated functions."""
|
|
|
|
@parameterized.named_parameters(jtu.cases_from_gens(gen_fun_and_types))
|
|
def testJitIsIdentity(self, fun):
|
|
vals = gen_vals(fun.in_vars)
|
|
fun = partial(eval_fun, fun)
|
|
ans = fun(*vals)
|
|
ans_jitted = maybe_jit(fun, len(vals))(*vals)
|
|
try:
|
|
check_all_close(ans, ans_jitted)
|
|
except:
|
|
print(fun)
|
|
raise
|
|
|
|
@parameterized.named_parameters(jtu.cases_from_gens(gen_fun_and_types))
|
|
def testJVPMatchesFD(self, fun):
|
|
vals = gen_vals(fun.in_vars)
|
|
tangents = gen_vals(fun.in_vars)
|
|
fun = partial(eval_fun, fun)
|
|
dyn_argnums = thin(range(len(vals)), 0.5)
|
|
tangents = [tangents[i] for i in dyn_argnums]
|
|
fun, vals = partial_argnums(fun, vals, dyn_argnums)
|
|
ans1, deriv1 = jvp_fd(fun, vals, tangents)
|
|
ans2, deriv2 = jvp(fun, tuple(vals), tuple(tangents))
|
|
check_all_close(ans1, ans2)
|
|
check_all_close(deriv1, deriv2)
|
|
|
|
@parameterized.named_parameters(jtu.cases_from_gens(gen_fun_and_types))
|
|
def vjp_matches_fd(self, fun):
|
|
vals = gen_vals(fun.in_vars)
|
|
in_tangents = gen_vals(fun.in_vars)
|
|
in_cotangents = gen_vals(fun.out_vars)
|
|
fun = partial(eval_fun, fun)
|
|
dyn_argnums = thin(range(len(vals)), 0.5)
|
|
in_tangents = [in_tangents[i] for i in dyn_argnums]
|
|
fun, vals = partial_argnums(fun, vals, dyn_argnums)
|
|
ans1, out_tangents = jvp_fd(fun, vals, in_tangents)
|
|
ans2, vjpfun = vjp(fun, *vals)
|
|
out_cotangents = vjpfun(in_cotangents)
|
|
check_all_close(ans1, ans2)
|
|
inner_prod_fd = inner_prod(out_tangents, in_cotangents)
|
|
inner_prod_ad = inner_prod(in_tangents, out_cotangents)
|
|
check_close(inner_prod_fd, inner_prod_ad)
|
|
|
|
|
|
if __name__ == "__main__":
|
|
absltest.main(testLoader=jtu.JaxTestLoader())
|