mirror of
https://github.com/ROCm/jax.git
synced 2025-04-14 10:56:06 +00:00
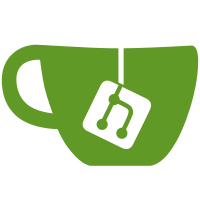
These docstrings do not make the tests any more clear and typically just duplicate the test module name. PiperOrigin-RevId: 737611977
3691 lines
164 KiB
Python
3691 lines
164 KiB
Python
# Copyright 2020 The JAX Authors.
|
|
#
|
|
# Licensed under the Apache License, Version 2.0 (the "License");
|
|
# you may not use this file except in compliance with the License.
|
|
# You may obtain a copy of the License at
|
|
#
|
|
# https://www.apache.org/licenses/LICENSE-2.0
|
|
#
|
|
# Unless required by applicable law or agreed to in writing, software
|
|
# distributed under the License is distributed on an "AS IS" BASIS,
|
|
# WITHOUT WARRANTIES OR CONDITIONS OF ANY KIND, either express or implied.
|
|
# See the License for the specific language governing permissions and
|
|
# limitations under the License.
|
|
|
|
from __future__ import annotations
|
|
|
|
import enum
|
|
from collections.abc import Callable, Sequence
|
|
import cProfile
|
|
import itertools
|
|
import math
|
|
import os
|
|
from pstats import Stats
|
|
from typing import Any
|
|
import unittest
|
|
|
|
from absl import logging
|
|
from absl.testing import absltest
|
|
|
|
import collections
|
|
import functools
|
|
from functools import partial
|
|
import operator as op
|
|
import re
|
|
|
|
import jax
|
|
from jax import export
|
|
from jax.experimental import pjit
|
|
from jax import lax
|
|
import jax.numpy as jnp
|
|
from jax import ops
|
|
from jax import random
|
|
from jax._src import config
|
|
from jax._src import core
|
|
from jax._src import test_util as jtu
|
|
from jax._src.export import shape_poly
|
|
from jax._src.export import shape_poly_decision
|
|
from jax._src.lax import lax as lax_internal
|
|
from jax._src.lax import control_flow as lax_control_flow
|
|
from jax._src.state import discharge
|
|
from jax._src.state import primitives as ref_primitives
|
|
|
|
import numpy as np
|
|
|
|
config.parse_flags_with_absl()
|
|
|
|
# Import after parsing flags
|
|
from jax._src.internal_test_util import test_harnesses
|
|
from jax._src.internal_test_util.test_harnesses import Harness, CustomArg, RandArg, StaticArg
|
|
|
|
_f32 = np.float32
|
|
_i32 = np.int32
|
|
DimSize = shape_poly.DimSize
|
|
|
|
expect_error_associative_scan = (
|
|
NotImplementedError,
|
|
"associative scan over axis of non-constant size",
|
|
)
|
|
|
|
# We want to have a complete test suite, even if the decision procedure is not
|
|
# yet as tight as we want. We will write the expected values as
|
|
# _expect(best=..., current=...) and we use the `current` value in the
|
|
# test, but we aspire to a decision procedure where we could compute `best`.
|
|
def _expect(*, current, best):
|
|
return current
|
|
|
|
def _bounds(e: shape_poly.DimSize) -> tuple[float, float]:
|
|
return shape_poly._bounds_decision(e, shape_poly.BoundsPrecision.BEST)
|
|
|
|
def _assert_equal_bounds(tst: jtu.JaxTestCase,
|
|
e: shape_poly.DimSize,
|
|
bounds: tuple[float, float]):
|
|
if isinstance(e, shape_poly._DimExpr):
|
|
scope = e.scope
|
|
else:
|
|
scope = shape_poly.SymbolicScope()
|
|
decision = shape_poly._make_decision_state(scope)
|
|
found_bounds = decision.bounds(e)
|
|
tst.assertEqual(bounds, found_bounds)
|
|
|
|
def _start_profile(tst: jtu.JaxTestCase):
|
|
tst.prof = None
|
|
if os.getenv("JAX_PROFILE_TEST", False):
|
|
tst.prof = cProfile.Profile()
|
|
tst.prof.enable()
|
|
|
|
def _stop_profile(tst: jtu.JaxTestCase):
|
|
if tst.prof is not None:
|
|
outfile = os.getenv("JAX_PROFILE_OUT")
|
|
if outfile:
|
|
tst.prof.dump_stats(outfile)
|
|
p = Stats(tst.prof)
|
|
p.strip_dirs()
|
|
p.sort_stats("cumtime").print_stats(.2)
|
|
p.print_callers(.2)
|
|
|
|
|
|
class DimExprTest(jtu.JaxTestCase):
|
|
|
|
def setUp(self):
|
|
_start_profile(self)
|
|
super().setUp()
|
|
|
|
def tearDown(self):
|
|
super().tearDown()
|
|
_stop_profile(self)
|
|
|
|
class AssertionType(enum.Enum):
|
|
EQ = 1,
|
|
GEQ = 2
|
|
|
|
def sampled_assertion(self,
|
|
e: DimSize,
|
|
fun: Callable,
|
|
*operands_sym: shape_poly.DimSize,
|
|
assertion: AssertionType = AssertionType.EQ
|
|
):
|
|
"""Checks `assertion(e, fun(*operands))` symbolically and concretely.
|
|
|
|
For the concrete check, it will sample the space of dimension variable
|
|
assignments for the dimension variables in `e`.
|
|
|
|
This is useful when `fun` can operate both with symbolic and with
|
|
concrete values, and we want to check that the behavior is sound.
|
|
"""
|
|
computed_sym = fun(*operands_sym)
|
|
assertion_fun = {
|
|
DimExprTest.AssertionType.EQ: self.assertEqual,
|
|
DimExprTest.AssertionType.GEQ: self.assertGreaterEqual}[assertion]
|
|
assertion_fun(e, computed_sym)
|
|
dim_vars: set[str] = set()
|
|
for a in (e, computed_sym, *operands_sym):
|
|
if core.is_symbolic_dim(a):
|
|
dim_vars = dim_vars.union(a._get_vars())
|
|
if not dim_vars:
|
|
return
|
|
dim_vars_tuple = tuple(dim_vars)
|
|
# All combinations of values
|
|
for dim_values in itertools.product(*([(1, 2, 5, 10)] * len(dim_vars_tuple))):
|
|
env = dict(zip(dim_vars_tuple, dim_values))
|
|
def eval(d: shape_poly.DimSize):
|
|
return d._evaluate(env) if core.is_symbolic_dim(d) else d # type: ignore
|
|
|
|
compute_concrete = fun(*map(eval, operands_sym))
|
|
expected_concrete = eval(e)
|
|
assertion_fun(
|
|
expected_concrete, compute_concrete,
|
|
f"{e=} {expected_concrete=} {compute_concrete=} {env=}")
|
|
|
|
def test_parse_shape(self):
|
|
self.assertEqual((), shape_poly.symbolic_shape("", like=()))
|
|
self.assertEqual((), shape_poly.symbolic_shape("()", like=()))
|
|
self.assertEqual((2, 3), shape_poly.symbolic_shape(None, like=(2, 3)))
|
|
self.assertEqual((2, 3), shape_poly.symbolic_shape("2, 3,", like=(2, 3)))
|
|
self.assertEqual((2, 3), shape_poly.symbolic_shape("2, _", like=(2, 3)))
|
|
self.assertEqual((2, 3), shape_poly.symbolic_shape("2, ...", like=(2, 3)))
|
|
self.assertEqual((2,), shape_poly.symbolic_shape("2, ...", like=(2,)))
|
|
self.assertEqual((2, 3), shape_poly.symbolic_shape("...", like=(2, 3)))
|
|
self.assertEqual((2, 3), shape_poly.symbolic_shape(" ( 2 , 3 ) "))
|
|
|
|
a, b = shape_poly.symbolic_shape("a, b")
|
|
self.assertEqual((a, 3), shape_poly.symbolic_shape("(a, ...) ", like=(None, 3),
|
|
scope=a.scope))
|
|
|
|
a, b = shape_poly.symbolic_shape("a, b")
|
|
|
|
@jtu.parameterized_filterable(
|
|
kwargs=[
|
|
dict(testcase_name=f"_{dim_spec}",
|
|
dim_spec=dim_spec, dim_poly=dim_poly, expected_str=expected_str)
|
|
for dim_spec, dim_poly, expected_str in [
|
|
("2*b*a", 2 * a * b, "2*a*b"),
|
|
("-2 * b * a^2 + b^2", -2 * a * a * b + b * b,
|
|
"- 2*a^2*b + b^2"),
|
|
("-2 * a^2 * b + -1 *b^2*a", -2 * a * a * b - a * b * b,
|
|
"- a*b^2 - 2*a^2*b"),
|
|
("3 * a * b * a - 2", 3 * a * b * a - 2,
|
|
"3*a^2*b - 2"),
|
|
("a - b", a - b, "- b + a"),
|
|
("b - a", b - a, "b - a"),
|
|
("-1 * a", -a, "- a"),
|
|
("-2*a", -2 * a, "- 2*a"),
|
|
("a + -1", a - 1, "a - 1"),
|
|
("a + 1 ,", a + 1, "a + 1"),
|
|
("a - 1", a - 1, "a - 1"),
|
|
("+ a - b", a - b, "- b + a"),
|
|
("+ 2", 2, "2"),
|
|
("3 * a * mod(a + 2, b + 2)", 3 * a * ((a + 2) % (b + 2)),
|
|
"3*a*mod(a + 2, b + 2)"),
|
|
("3 * floordiv(a + 2, b + 2) * 2", 3 * ((a + 2) // (b + 2)) * 2,
|
|
"6*floordiv(a + 2, b + 2)"),
|
|
("max(a, b)", "build_inside", "max(a, b)"),
|
|
("min(a, b)", "build_inside", "min(a, b)"),
|
|
]])
|
|
def test_parse_dim(self, dim_spec, dim_poly, expected_str):
|
|
if dim_spec == "max(a, b)":
|
|
dim_poly = core.max_dim(DimExprTest.a, DimExprTest.b)
|
|
elif dim_spec == "min(a, b)":
|
|
dim_poly = core.min_dim(DimExprTest.a, DimExprTest.b)
|
|
|
|
self.assertEqual((dim_poly,), shape_poly.symbolic_shape(dim_spec,
|
|
scope=DimExprTest.a.scope))
|
|
self.assertEqual(expected_str, str(dim_poly))
|
|
self.assertEqual((dim_poly,), shape_poly.symbolic_shape(expected_str,
|
|
scope=DimExprTest.a.scope))
|
|
|
|
@jtu.parameterized_filterable(
|
|
kwargs=[
|
|
dict(dim_spec=dim_spec)
|
|
for dim_spec in [
|
|
"b + a",
|
|
"b - a",
|
|
"b + 3*a",
|
|
"a*b + a^2 + b + a",
|
|
"mod(a, 4) + floordiv(a, 4) + a",
|
|
"2*a^2 - 3*a - 1",
|
|
"a^2 + 3*a - 1",
|
|
"- a + 3",
|
|
"- mod(a, 4) + 3",
|
|
"- 2*a + 3",
|
|
"a*floordiv(b, 8)*mod(b, 4)",
|
|
]
|
|
]
|
|
)
|
|
def test_print_dim(self, *, dim_spec: str):
|
|
e, = shape_poly.symbolic_shape(dim_spec)
|
|
self.assertEqual(str(e), dim_spec)
|
|
|
|
@jtu.parameterized_filterable(
|
|
kwargs=[
|
|
# sanitized shape_spec sometimes collide
|
|
dict(testcase_name=(
|
|
f"_shape_spec={shape_spec}" +
|
|
{"...": "name=ellipsis",
|
|
")(": "name=bad_parens",
|
|
"a ;": "name=a_semicolon",
|
|
"'a'": "name=a_quotes"}.get(shape_spec, "")),
|
|
shape_spec=shape_spec)
|
|
for shape_spec in [
|
|
"2.5", "a + a a", "a ^ a", "a; a",
|
|
"_", "...", "a ;", ")(", "2a", "a@", "'a'", "('a', ...)",
|
|
"mod(a)", "floordiv(a, b, c)", "..., 3"
|
|
]])
|
|
def test_parse_error(self,
|
|
shape_spec="a + a a"):
|
|
with self.assertRaisesRegex(ValueError,
|
|
"syntax error in symbolic shape"):
|
|
shape_poly.symbolic_shape(shape_spec)
|
|
|
|
@jtu.parameterized_filterable(
|
|
kwargs=[
|
|
dict(testcase_name=f"_{shape_spec=}",
|
|
shape_spec=shape_spec, arg_shape=arg_shape, error_msg=error_msg)
|
|
for shape_spec, arg_shape, error_msg in [
|
|
("3", (4,), "different size"),
|
|
("b, 3", (None, 4), "different size"),
|
|
("b, ...", None, "no 'like' shape was given"),
|
|
("b, 5, _", None, "no 'like' shape was given"),
|
|
("b, 6, c, _", (4, 6, 7),
|
|
"because we parsed 3 already and 'like' shape has only 3 dimensions"),
|
|
("b, 7, c, ...", (4, 7),
|
|
"because we parsed 3 already and 'like' shape has only 2 dimensions"),
|
|
]])
|
|
def test_parse_mismatch_error(self, *,
|
|
shape_spec, arg_shape, error_msg):
|
|
with self.assertRaisesRegex(ValueError,
|
|
"syntax error in symbolic shape .*" + error_msg):
|
|
shape_poly.symbolic_shape(shape_spec, like=arg_shape)
|
|
|
|
def test_dim_vars(self):
|
|
a, b, a1 = shape_poly.symbolic_shape("a, b, a")
|
|
self.assertEqual(True, a == a)
|
|
self.assertEqual(True, a == a1)
|
|
self.assertEqual(False, a != a)
|
|
|
|
self.assertNotEqual(a, b)
|
|
|
|
self.assertLen({a, a}, 1)
|
|
self.assertLen({a, b}, 2)
|
|
self.assertIn(a, {a, b})
|
|
self.assertIn(b, {a, b})
|
|
self.assertIn(a, [a, b])
|
|
self.assertIn(b, [a, b])
|
|
|
|
def test_sizes(self):
|
|
a, b = shape_poly.symbolic_shape("a, b")
|
|
self.assertEqual(a._size, b._size)
|
|
self.assertGreater(a._size, shape_poly._ensure_poly(1, "", a.scope)._size)
|
|
self.assertGreater((a + 1)._size, a._size)
|
|
self.assertGreater((a + b)._size, a._size)
|
|
self.assertGreater((a + b)._size, (a + 1)._size)
|
|
self.assertGreater((2 * a)._size, a._size)
|
|
self.assertGreater((2 * a)._size, (a + 1)._size)
|
|
self.assertGreater((a * a)._size, a._size)
|
|
self.assertGreater((a * a * a)._size, (a * a)._size)
|
|
self.assertGreater((a * b)._size, a._size)
|
|
|
|
def test_get_vars(self):
|
|
a, b = shape_poly.symbolic_shape("a, b")
|
|
|
|
self.assertEqual({"a"}, a._get_vars())
|
|
self.assertEqual({"a", "b"}, (a * b * a)._get_vars())
|
|
|
|
def test_evaluate(self):
|
|
a, b = shape_poly.symbolic_shape("a, b")
|
|
|
|
self.assertEqual(1, (a * a - b)._evaluate(dict(a=2, b=3)))
|
|
self.assertEqual(1, ((a * a) // b)._evaluate(dict(a=2, b=3)))
|
|
self.assertEqual(4, ((a * a) % b)._evaluate(dict(a=5, b=7)))
|
|
|
|
def test_dim_vars_definitely_equal(self):
|
|
a, b = shape_poly.symbolic_shape("a, b")
|
|
self.assertTrue(core.definitely_equal(a, a))
|
|
self.assertFalse(core.definitely_equal(a, 1))
|
|
self.assertFalse(core.definitely_equal(a, b))
|
|
|
|
self.assertTrue(core.definitely_equal_one_of_dim(a, [2, a]))
|
|
self.assertFalse(core.definitely_equal_one_of_dim(a, [2, b]))
|
|
self.assertFalse(core.definitely_equal_one_of_dim(a, []))
|
|
|
|
self.assertTrue(core.definitely_equal_one_of_dim(2, [a, 3, 2]))
|
|
self.assertFalse(core.definitely_equal_one_of_dim(1, [2, b]))
|
|
self.assertFalse(core.definitely_equal_one_of_dim(3, []))
|
|
|
|
self.assertTrue(core.definitely_equal(1, jnp.add(0, 1))) # An Array
|
|
self.assertFalse(core.definitely_equal(1, "a"))
|
|
|
|
def test_factors_ordering(self):
|
|
a, b = shape_poly.symbolic_shape("a, b")
|
|
|
|
self.assertTrue(a._to_factor() < b._to_factor())
|
|
self.assertFalse(a._to_factor() >= b._to_factor())
|
|
self.assertTrue(a._to_factor() <= b._to_factor())
|
|
self.assertTrue(a._to_factor() != b._to_factor())
|
|
|
|
self.assertTrue(a._to_factor() < (a % 4)._to_factor())
|
|
self.assertFalse(a._to_factor() > (a % 4)._to_factor())
|
|
# FLOORDIV comes before MON because we compare operations alphabetically
|
|
self.assertTrue((a // 4)._to_factor() < (a % 4)._to_factor())
|
|
|
|
self.assertEqual(hash((a // 4)._to_factor()), hash((a // 4)._to_factor()))
|
|
|
|
def test_term_ordering(self):
|
|
a, b = shape_poly.symbolic_shape("a, b")
|
|
self.assertTrue(a._to_term() < b._to_term())
|
|
self.assertTrue(a._to_term() <= b._to_term())
|
|
self.assertTrue(b._to_term() >= a._to_term())
|
|
self.assertTrue(b._to_term() > a._to_term())
|
|
|
|
self.assertTrue(((3 * b) // a)._to_term() >= ((2 * b) // a)._to_term())
|
|
self.assertTrue(((3 * b) // a)._to_term() <= ((4 * a) // b)._to_term())
|
|
self.assertTrue(a._to_term() < (a * a)._to_term())
|
|
self.assertTrue(b._to_term() < (a * a)._to_term())
|
|
self.assertTrue((a * a * b)._to_term() < (a * b * b)._to_term())
|
|
e1 = 2 + a * a * b + a * b * b + a * b + a * a + a + b
|
|
|
|
sorted_e1 = [shape_poly._DimExpr._from_term(m, m_count, a.scope)
|
|
for m, m_count in e1._sorted_terms]
|
|
self.assertSequenceEqual(sorted_e1,
|
|
[a * b * b, a * a * b, a * b, a * a, b, a, 2])
|
|
|
|
e2 = a * (a // 4) + (a // 4) + b * (a // 4) + b * (a % 4) + a * a + b + 15
|
|
sorted_e2 = [shape_poly._DimExpr._from_term(m, m_count, a.scope)
|
|
for m, m_count in e2._sorted_terms]
|
|
self.assertSequenceEqual(sorted_e2,
|
|
[b * (a % 4), b * (a // 4), a * (a // 4), a // 4,
|
|
a * a, b, 15])
|
|
|
|
def test_bounds_arithmetic(self):
|
|
a, b, c = shape_poly.symbolic_shape("a, b, c")
|
|
bounded_le4 = 5 - a
|
|
bounded_ge2 = b + 1
|
|
bounded_ge0_le4 = a % 5
|
|
self.assertEqual(_bounds(a), (1, np.inf))
|
|
self.assertEqual(_bounds(- a), (-np.inf, -1))
|
|
self.assertEqual(_bounds(bounded_le4), (-np.inf, 4))
|
|
self.assertEqual(_bounds(bounded_ge2), (2, np.inf))
|
|
self.assertEqual(_bounds(bounded_ge0_le4), (0, 4))
|
|
|
|
self.assertEqual(_bounds(b + 1 - b), (1, 1))
|
|
self.assertEqual(_bounds(a), (1, np.inf))
|
|
self.assertEqual(_bounds(a - 1), (0, np.inf))
|
|
self.assertEqual(_bounds(5*a - 2), (3, np.inf))
|
|
self.assertEqual(_bounds(-5*a - 2), (-np.inf, -7))
|
|
self.assertEqual(_bounds(2*a + 3*b + 5*c - 1), (9, np.inf))
|
|
self.assertEqual(_bounds(2*a - 3*b + 5*c - 1), (-np.inf, np.inf))
|
|
self.assertEqual(_bounds(-2*a + -3*b + -5*c + 20), (-np.inf, 10))
|
|
|
|
# Additions
|
|
self.assertEqual(_bounds(bounded_ge0_le4 + bounded_le4), (-np.inf, 8))
|
|
self.assertEqual(_bounds(bounded_ge0_le4 + bounded_ge2), (2, np.inf))
|
|
self.assertEqual(_bounds(bounded_le4 + bounded_ge2), (-np.inf, np.inf))
|
|
|
|
# Subtractions
|
|
self.assertEqual(_bounds(bounded_ge0_le4 - bounded_le4), (-4, np.inf))
|
|
self.assertEqual(_bounds(- bounded_ge0_le4 + bounded_le4), (-np.inf, 4))
|
|
self.assertEqual(_bounds(bounded_ge0_le4 - bounded_ge2), (-np.inf, 2))
|
|
self.assertEqual(_bounds(- bounded_ge0_le4 + bounded_ge2), (-2, np.inf))
|
|
self.assertEqual(_bounds(bounded_le4 - bounded_ge2), (-np.inf, 2))
|
|
self.assertEqual(_bounds(- bounded_le4 + bounded_ge2), (-2, np.inf))
|
|
|
|
# Multiplications
|
|
self.assertEqual(_bounds(2 * a - 3), (-1, np.inf))
|
|
self.assertEqual(_bounds(-2 * a - 3), (-np.inf, -5))
|
|
self.assertEqual(_bounds(3 * a * b * b + 5 * a - 7), (1, np.inf))
|
|
self.assertEqual(_bounds(3 * a * b * b - 5 * a - 7), (-np.inf, np.inf))
|
|
self.assertEqual(_bounds(a + b - a * b + a * b * a), (-np.inf, np.inf))
|
|
self.assertEqual(_bounds(a + 2 * b - a), (2, np.inf))
|
|
self.assertEqual(_bounds(a + 2 * b - a), (2, np.inf))
|
|
|
|
# Higher order polynomial
|
|
self.assertEqual(_bounds(a*a + b - 2), (0, np.inf))
|
|
self.assertEqual(_bounds(-2*a*b - b - 2), (-np.inf, -5))
|
|
|
|
def test_bounds_mod(self):
|
|
a, b = shape_poly.symbolic_shape("a, b")
|
|
|
|
# self.assertEqual(_bounds(5 - a % 5), (1, 5))
|
|
self.assertEqual(_bounds(-5 - a % (-5)), (-5, -1))
|
|
self.assertEqual(_bounds(a - 5 % a), (1, np.inf))
|
|
self.assertEqual(_bounds(a - 5 % a), (1, np.inf))
|
|
self.assertEqual(_bounds(3 * (a + b) - 5 % (3 * (a + b))), (1, np.inf))
|
|
self.assertEqual(_bounds(- a + (b - 5) % a), (-np.inf, -1))
|
|
|
|
# mod
|
|
self.assertEqual(_bounds((b + 1) % 2), (0, 1))
|
|
self.assertEqual(_bounds((b + 1) % -2), (-1, 0))
|
|
self.assertEqual(_bounds((b - 4) % 2), (0, 1))
|
|
self.assertEqual(_bounds((b + 1) % a), (0, np.inf))
|
|
self.assertEqual(_bounds(11 % (a + 1)), (0, np.inf))
|
|
self.assertEqual(_bounds(-11 % (a + 1)), (0, np.inf))
|
|
self.assertEqual(_bounds(b % (a - 2)), (-np.inf, np.inf))
|
|
|
|
# This arises in convolutions, because we use "-2 * div(-b, 2)" to get
|
|
# the "2*ceil(b / 2)".
|
|
self.assertGreaterEqual(-2 * ((- b) // 2), b)
|
|
|
|
def test_bounds_floordiv(self):
|
|
a, b = shape_poly.symbolic_shape("a, b")
|
|
self.assertEqual(_bounds((a + 4) // 2), (2, np.inf))
|
|
self.assertEqual(_bounds((a + 4) // -2), (-np.inf, -3))
|
|
self.assertEqual(_bounds((a + 5) // 2), (3, np.inf))
|
|
self.assertEqual(_bounds((a + 5) // -2), (-np.inf, -3))
|
|
self.assertEqual(_bounds(11 // (a + 1)), (0, 5))
|
|
self.assertEqual(_bounds(-11 // (a + 1)), (-6, -1))
|
|
self.assertEqual(_bounds(-11 // (- a)), (0, 11)) # finite negative dividend, infinite divisor
|
|
self.assertEqual(_bounds((b + 1) // (a + 1)), (0, np.inf))
|
|
self.assertEqual(_bounds(-b // (a + 1)), (-np.inf, -1))
|
|
|
|
self.assertEqual(_bounds(a - a // 2), (1, np.inf))
|
|
self.assertEqual(_bounds((a + 3) - (a + 3) // 2), (2, np.inf))
|
|
self.assertEqual(_bounds((a + 6) - 1 * (a + 6) // 4), (6, np.inf))
|
|
self.assertEqual(_bounds((a + 6) - 2 * ((a + 6) // 4)), (4, np.inf))
|
|
self.assertEqual(_bounds((a + 6) - 3 * ((a + 6) // 4)), (2, np.inf))
|
|
self.assertEqual(_bounds(a - 2 * (a // 2)), (0, 1))
|
|
with self.assertRaisesRegex(core.InconclusiveDimensionOperation,
|
|
"Possible division by 0"):
|
|
_bounds(a // (a - 3))
|
|
|
|
def test_bounds_floordiv_against_concrete_evaluation(self):
|
|
a, b = shape_poly.symbolic_shape("a, b")
|
|
# Generate test cases for floordiv and mod: (a + N) // +-2, (N - a) // +-2
|
|
# and then evaluate them for a = 1, 5, 10000
|
|
div_mod_factors = [
|
|
operation(op1 + n, div)
|
|
for op1 in (a, a + 10, a + 11, -a, -a + 10, -a + 11)
|
|
for n in (-3, -1, 0, 1, 3)
|
|
for div in (-2, 2, a + 4, -4 - a) # Either negative, or positive
|
|
for operation in (op.floordiv, op.mod)
|
|
]
|
|
for fact in div_mod_factors:
|
|
lb, ub = _bounds(fact)
|
|
self.assertLessEqual(lb, ub)
|
|
for a_val in (1, 5, 10000):
|
|
fact_val = fact._evaluate(dict(a=a_val))
|
|
self.assertGreaterEqual(fact_val, lb)
|
|
self.assertLessEqual(fact_val, ub)
|
|
|
|
def test_max_dim(self):
|
|
a, b, c, d = shape_poly.symbolic_shape("a, b, c, d")
|
|
|
|
self.assertEqual(_bounds(core.max_dim(a, b)), (1, np.inf))
|
|
self.assertEqual(_bounds(core.max_dim(2, b)), (2, np.inf))
|
|
self.assertEqual(_bounds(core.max_dim(a, 2)), (2, np.inf))
|
|
self.assertEqual(_bounds(core.max_dim(a - 5, 1)), (1, np.inf))
|
|
self.assertEqual(_bounds(core.max_dim(15 - a, 0)), (0, 14))
|
|
self.assertEqual(_bounds(core.max_dim(15 - a, 0) // 3), (0, 4))
|
|
|
|
self.assertEqual(a + 2, core.max_dim(a, a + 2))
|
|
self.assertEqual(a , core.max_dim(a, a - 2))
|
|
self.assertEqual(core.max_dim(a % 2 - 1, 0), 0)
|
|
self.assertEqual(core.max_dim(0, a % 2 - 1), 0)
|
|
self.assertGreaterEqual(core.max_dim(a, b), a)
|
|
self.assertGreaterEqual(core.max_dim(a, b) + c - 1, a)
|
|
self.assertGreaterEqual(core.max_dim(a, b), b)
|
|
self.assertGreaterEqual(core.max_dim(a, b) + c - 1, b)
|
|
|
|
self.assertGreaterEqual(core.max_dim(a, b), core.min_dim(a, b))
|
|
|
|
self.assertEqual(_bounds(b - core.max_dim(b - a, 0)), (1, np.inf))
|
|
self.assertEqual(_bounds(a - core.min_dim(a, b)), (0, np.inf))
|
|
self.assertEqual(_bounds(b - core.min_dim(a, b)), (0, np.inf))
|
|
|
|
self.assertEqual(_bounds(core.max_dim(a, b) - a), (0, np.inf))
|
|
self.assertEqual(_bounds(core.max_dim(a, b) - b), (0, np.inf))
|
|
self.assertEqual((0, 0), _bounds(core.max_dim(1 - b, 0)))
|
|
self.assertEqual(_bounds(core.max_dim(a, b) - a - core.max_dim(b - a, 0)),
|
|
_expect(best=(0, 0), current=(0, np.inf)))
|
|
self.assertEqual(_bounds(core.max_dim(a, b) + core.max_dim(c, d) -
|
|
core.max_dim(a + core.max_dim(c, d),
|
|
b + core.max_dim(c, d))),
|
|
_expect(best=(0, 0), current=(0, np.inf)))
|
|
self.assertEqual(_bounds(core.max_dim(a, b) + core.min_dim(c, d) -
|
|
core.max_dim(a + core.min_dim(c, d),
|
|
b + core.min_dim(c, d))),
|
|
_expect(best=(0, 0), current=(0, np.inf)))
|
|
|
|
self.sampled_assertion(core.max_dim(a, 5), core.max_dim, a, 5)
|
|
self.sampled_assertion(core.max_dim(5, a), core.max_dim, 5, a)
|
|
|
|
def test_min_dim(self):
|
|
a, b, c = shape_poly.symbolic_shape("a, b, c")
|
|
|
|
self.assertEqual(_bounds(core.min_dim(a, b)), (1, np.inf))
|
|
self.assertEqual(_bounds(core.min_dim(2, b)), (1, 2))
|
|
self.assertEqual(core.min_dim(a, -2), -2)
|
|
self.assertEqual(_bounds(core.min_dim(a - 5, 1)), (-4, 1))
|
|
self.assertEqual(_bounds(core.min_dim(15 - a, 10)), (-np.inf, 10))
|
|
self.assertEqual(_bounds(core.min_dim(15 - a, 20)), (-np.inf, 14))
|
|
|
|
# Test simplification during construction
|
|
self.assertEqual(a, core.min_dim(a, a + 2))
|
|
self.assertEqual(a - 2, core.min_dim(a, a - 2))
|
|
self.assertEqual(core.min_dim(a % 2 - 1, -1), -1)
|
|
self.assertEqual(core.min_dim(-1, a % 2 - 1), -1)
|
|
self.assertGreaterEqual(a, core.min_dim(a, b))
|
|
self.assertGreaterEqual(a + c - 1, core.min_dim(a, b))
|
|
self.assertGreaterEqual(b, core.min_dim(a, b))
|
|
self.assertGreaterEqual(b + c - 1, core.min_dim(a, b))
|
|
|
|
self.sampled_assertion(core.min_dim(a, 5), core.min_dim, a, 5)
|
|
self.sampled_assertion(core.min_dim(5, a), core.min_dim, 5, a)
|
|
|
|
def test_min_max_type_check(self):
|
|
a, = shape_poly.symbolic_shape("a")
|
|
for i, f in enumerate([lambda x: core.max_dim(x, a),
|
|
lambda x: core.max_dim(a, x),
|
|
lambda x: core.min_dim(x, a),
|
|
lambda x: core.min_dim(a, x)]):
|
|
with self.subTest(f"jit_{i}"):
|
|
with self.assertRaisesRegex(core.ConcretizationTypeError, ""):
|
|
jax.jit(f)(1)
|
|
|
|
arr = jnp.array([1], dtype=np.int32)
|
|
for i, f in enumerate([lambda: core.max_dim(arr, a),
|
|
lambda: core.max_dim(a, arr),
|
|
lambda: core.min_dim(arr, a),
|
|
lambda: core.min_dim(a, arr)]):
|
|
with self.subTest(f"array_{i}"):
|
|
with self.assertRaisesRegex(
|
|
TypeError,
|
|
"Only integer scalar arrays can be converted to a scalar index"):
|
|
f()
|
|
|
|
def test_clamp_dim(self):
|
|
a, b = shape_poly.symbolic_shape("a, b")
|
|
# Clamping b <= a <= b + 10
|
|
clamp = core.max_dim(core.min_dim(a, b + 10), b)
|
|
self.assertLessEqual(b, clamp)
|
|
self.assertLessEqual(clamp, b + 10)
|
|
|
|
def test_bounds_complex(self):
|
|
a, b = shape_poly.symbolic_shape("a, b")
|
|
min_a_b = b - core.max_dim(0, b - a)
|
|
# This comes up in slicing with stride
|
|
self.assertGreaterEqual(min_a_b // 2, 0)
|
|
|
|
# Comes up in slice[-2:-2b-2:-2]
|
|
self.assertGreaterEqual((-1 * core.max_dim(-1, a - 2 * b - 2) + core.max_dim(-1, a - 2) + 1),
|
|
0)
|
|
self.assertGreaterEqual((-1 * core.max_dim(-1, a - 2 * b - 2) + core.max_dim(-1, a - 2) + 1) // 2,
|
|
0)
|
|
self.assertEqual((0, 0),
|
|
_bounds(core.max_dim(-1*b + 1, 0) // 2))
|
|
|
|
def test_equal(self):
|
|
a, b = shape_poly.symbolic_shape("a, b")
|
|
poly3 = a + 3 - a
|
|
self.assertEqual(poly3, 3)
|
|
self.assertEqual(poly3, np.array(3, np.int64))
|
|
self.assertEqual(poly3, np.array(3, np.int64)[()])
|
|
self.assertNotEqual(poly3 + 1, 3)
|
|
self.assertNotEqual(poly3, poly3 + 1)
|
|
self.assertTrue((2 * a * b * a + 3)._eq(1 + b * a * a + a * a * b + 2))
|
|
self.assertFalse((2 * a * b * a + 3)._eq(a * b * a + 3))
|
|
|
|
self.assertFalse((a * b * a + 3)._eq(a * b * a + 4))
|
|
self.assertFalse((2 * a * b * a)._eq(a * b * a))
|
|
self.assertFalse((2 * a * b * a + 1)._eq(a * b * a))
|
|
self.assertFalse((3 * a * b * a - 1)._eq(a * b * a))
|
|
|
|
self.assertFalse((3 * a * b * a - 2)._eq(a * b * a))
|
|
|
|
self.sampled_assertion(a % b,
|
|
lambda x: x, a % b)
|
|
self.sampled_assertion(a % b - a % b,
|
|
lambda x: x, 0)
|
|
self.sampled_assertion(a // b,
|
|
lambda x: x, a // b)
|
|
self.sampled_assertion(a // b - a // b,
|
|
lambda x: x, 0)
|
|
|
|
self.sampled_assertion(a % b,
|
|
lambda x: x, (2 * a // 2) % (a + b - a))
|
|
self.sampled_assertion(a // b,
|
|
lambda x: x, (2 * a // 2) // (a + b - a))
|
|
|
|
self.sampled_assertion(a, lambda x: x,
|
|
a + (a + b) // b - (b + a) // b)
|
|
|
|
# Test the normalization (a // b) * b == a - a % b
|
|
# We sacrifice this with a faster implementation of equality.
|
|
# We could fix this by adding: `core.expensive_eq()`
|
|
# self.sampled_assertion((a // 2) * 2,
|
|
# lambda x: x, a - a % 2)
|
|
# self.sampled_assertion((a // 2) + (a // 2),
|
|
# lambda x: x, a - a % 2)
|
|
# self.sampled_assertion((a // 2) * 6,
|
|
# lambda x: x, 3 * a - 3 * (a % 2))
|
|
|
|
def test_compare_ge(self):
|
|
a, b = shape_poly.symbolic_shape("a, b")
|
|
poly = 4 * a + b + 3
|
|
self.assertGreaterEqual(a, a)
|
|
self.assertGreaterEqual(a, 0)
|
|
self.assertGreaterEqual(a, 1)
|
|
|
|
with self.assertRaisesRegex(core.InconclusiveDimensionOperation, "inconclusive"):
|
|
a >= 2
|
|
|
|
self.assertGreaterEqual(poly, 0)
|
|
self.assertGreaterEqual(poly, 8)
|
|
self.assertGreaterEqual(poly, poly)
|
|
self.assertGreaterEqual(poly, poly - 1)
|
|
|
|
with self.assertRaisesRegex(
|
|
core.InconclusiveDimensionOperation,
|
|
re.escape("comparison 'b + 4*a + 3' >= '9' is inconclusive")):
|
|
poly >= 9
|
|
|
|
with self.assertRaisesRegex(
|
|
core.InconclusiveDimensionOperation,
|
|
re.escape("comparison 'b + 4*a + 3' > '9' is inconclusive")):
|
|
poly > 9
|
|
|
|
with self.assertRaisesRegex(core.InconclusiveDimensionOperation,
|
|
"inconclusive"):
|
|
(4 * a - b) >= 0
|
|
|
|
# LHS is an integer
|
|
self.assertLessEqual(8, poly)
|
|
self.assertLess(7, poly)
|
|
self.assertGreaterEqual(-8, -poly)
|
|
self.assertGreater(-7, -poly)
|
|
|
|
|
|
def test_int_results(self):
|
|
# Whenever the result is an integer, it should be represented as a
|
|
# Python integer, not a symbolic dimension.
|
|
a, b = shape_poly.symbolic_shape("a, b")
|
|
self.assertEqual(a + 2 - a, 2)
|
|
self.assertIsInstance(a + 2 - a, int)
|
|
self.assertEqual(a + (2 - a), 2)
|
|
self.assertIsInstance(a + (2 - a), int)
|
|
self.assertEqual(a * 2 // a, 2)
|
|
self.assertIsInstance(a * 2 // a, int)
|
|
self.assertEqual((4*a)*0, 0)
|
|
self.assertEqual(0*(4*a), 0)
|
|
|
|
@jtu.parameterized_filterable(
|
|
kwargs=[
|
|
dict(testcase_name=f"_D={dividend}_d={divisor}_q={quotient}_r={remainder}",
|
|
dividend=dividend, divisor=divisor, quotient=quotient,
|
|
remainder=remainder)
|
|
for dividend, divisor, quotient, remainder in [
|
|
(a, 1, a, 0),
|
|
(3 * a, 3, a, 0),
|
|
(3 * a + 3, 3, a + 1, 0),
|
|
(3 * a + 2, 3, a, 2),
|
|
(3 * a + 5, 3, a + 1, 2),
|
|
(3 * a - 2, 3, a - 1, 1),
|
|
(3 * a * a * b + 2 * b * b * a, a * b, 3 * a + 2 * b, 0),
|
|
(a * a - b * b, a + b, a - b, 0),
|
|
(256 * a * b, 32, 8 * a * b, 0),
|
|
(0, b, 0, 0),
|
|
(a, b, "floordiv(a, b)", "mod(a, b)"),
|
|
(3 * a, 2, "floordiv(3*a, 2)", "mod(3*a, 2)"),
|
|
(2 * a * b + b * b, a + b, "floordiv(2*a*b + b^2, b + a)", "mod(2*a*b + b^2, b + a)"),
|
|
(3, a, "floordiv(3, a)", "mod(3, a)"),
|
|
]])
|
|
def test_divmod(self, *, dividend, quotient, divisor, remainder):
|
|
if isinstance(quotient, str):
|
|
d1, d2 = divmod(dividend, divisor)
|
|
self.assertEqual((quotient, remainder), (str(d1), str(d2)))
|
|
else:
|
|
self.sampled_assertion(quotient, lambda *args: divmod(*args)[0],
|
|
dividend, divisor)
|
|
self.sampled_assertion(remainder, lambda *args: divmod(*args)[1],
|
|
dividend, divisor)
|
|
|
|
|
|
def test_unit_combine_term_with_constraints(self):
|
|
a, b, c, d, e = shape_poly.symbolic_shape("a, b, c, d, e",
|
|
constraints=[
|
|
"a >= 4",
|
|
"b <= 5",
|
|
"c + 3*d >= 10", "c - 2*d >= -4", # -> 5c >= 8 -> c >= 2
|
|
])
|
|
scope = a.scope
|
|
def _m(e: shape_poly._DimExpr) -> shape_poly._DimTerm:
|
|
return e._to_term()
|
|
Comparator = shape_poly.Comparator
|
|
decision = shape_poly_decision._DecisionByElimination(scope).initialize()
|
|
|
|
self.assertSetEqual(
|
|
set(),
|
|
set(decision.combine_term_with_existing(_m(e), 2, scope=scope)))
|
|
|
|
self.assertSetEqual(
|
|
{(Comparator.GEQ, a - 4, 2, -1)},
|
|
set(decision.combine_term_with_existing(_m(a), 2, scope=scope)))
|
|
|
|
self.assertSetEqual(
|
|
{(Comparator.GEQ, a - 4, 2, 1)},
|
|
set(decision.combine_term_with_existing(_m(a), -2, scope=scope)))
|
|
|
|
self.assertSetEqual(
|
|
{(Comparator.GEQ, b - 1, 2, -1),
|
|
(Comparator.GEQ, -b + 5, 2, 1)},
|
|
set(decision.combine_term_with_existing(_m(b), 2, scope=scope)))
|
|
|
|
self.assertSetEqual(
|
|
{(Comparator.GEQ, b - 1, 2, 1),
|
|
(Comparator.GEQ, -b + 5, 2, -1)},
|
|
set(decision.combine_term_with_existing(_m(b), -2, scope=scope)))
|
|
|
|
self.assertSetEqual(
|
|
{(Comparator.GEQ, c - 2, 2, -1),
|
|
(Comparator.GEQ, -2*d + c + 4, 2, -1),
|
|
(Comparator.GEQ, 3*d + c - 10, 2, -1)},
|
|
set(decision.combine_term_with_existing(_m(c), 2, scope=scope,
|
|
only_smaller_than_t=False)))
|
|
|
|
self.assertSetEqual(
|
|
{(Comparator.GEQ, c - 2, 2, 1),
|
|
(Comparator.GEQ, -2*d + c + 4, 2, 1),
|
|
(Comparator.GEQ, 3*d + c - 10, 2, 1)},
|
|
set(decision.combine_term_with_existing(_m(c), -2, scope=scope,
|
|
only_smaller_than_t=False)))
|
|
|
|
self.assertSetEqual(
|
|
{(Comparator.GEQ, c - 2, 2, -1)},
|
|
set(decision.combine_term_with_existing(_m(c), 2, scope=scope,
|
|
only_smaller_than_t=True)))
|
|
|
|
self.assertSetEqual(
|
|
{(Comparator.GEQ, d - 1, 2, -1),
|
|
(Comparator.GEQ, -2*d + c + 4, 2, 2),
|
|
(Comparator.GEQ, 3*d + c - 10, 2, -3)},
|
|
set(decision.combine_term_with_existing(_m(d), 2, scope=scope,
|
|
only_smaller_than_t=False)))
|
|
|
|
self.assertSetEqual(
|
|
{(Comparator.GEQ, d - 1, 2, -1),
|
|
(Comparator.GEQ, -2*d + c + 4, 2, 2),
|
|
(Comparator.GEQ, 3*d + c - 10, 2, -3)},
|
|
set(decision.combine_term_with_existing(_m(d), 2, scope=scope,
|
|
only_smaller_than_t=True)))
|
|
|
|
|
|
def test_dilate_dim(self):
|
|
"""0 if d == 0 else 1 + dilation * (d - 1))"""
|
|
a, = shape_poly.symbolic_shape("a,")
|
|
|
|
self.sampled_assertion(4, core.dilate_dim, 2, 3)
|
|
self.sampled_assertion(7, core.dilate_dim, 3, 3)
|
|
self.sampled_assertion(0, core.dilate_dim, 0, 3)
|
|
self.sampled_assertion(a, core.dilate_dim, a, 1)
|
|
self.sampled_assertion(2 * a - 1, core.dilate_dim, a, 2)
|
|
self.sampled_assertion(core.max_dim(2 * a - 3, 0),
|
|
core.dilate_dim, a - 1, 2)
|
|
|
|
def test_stride_dim(self):
|
|
"""(d - window_size) // window_stride + 1
|
|
|
|
If d < window_size, returns 0.
|
|
"""
|
|
a, stride = shape_poly.symbolic_shape("a, s")
|
|
self.sampled_assertion(8, core.stride_dim, 10, 3, 1)
|
|
self.sampled_assertion(9, core.stride_dim, 20, 3, 2)
|
|
self.sampled_assertion(9, core.stride_dim, 20, 4, 2)
|
|
self.sampled_assertion(a, core.stride_dim, a, 1, 1)
|
|
|
|
self.sampled_assertion(a - 1, core.stride_dim, a, 2, 1)
|
|
self.sampled_assertion(a + 1, core.stride_dim, a * stride + 2, 2, stride)
|
|
self.sampled_assertion((a - 1) // 2 + 1, core.stride_dim, a, 1, 2)
|
|
self.sampled_assertion(core.max_dim((a - 4) // 2 + 1, 0),
|
|
core.stride_dim, a, 4, 2)
|
|
|
|
def test_constraints_ge_basic(self):
|
|
a, b = shape_poly.symbolic_shape("a, b",
|
|
constraints=("a >= 5", "b <= 16"))
|
|
self.assertEqual(_bounds(a), (5, np.inf))
|
|
self.assertEqual(_bounds(b), (1, 16))
|
|
|
|
def test_constraints_ge_trivial(self):
|
|
a, = shape_poly.symbolic_shape("a",
|
|
# Trivial, is dropped
|
|
constraints=("a <= a + 1",))
|
|
self.assertSequenceEqual(a.scope._explicit_constraints, ())
|
|
with self.assertRaisesRegex(ValueError,
|
|
"Unsatisfiable.*a <= a - 1"):
|
|
_ = shape_poly.symbolic_shape("a, b",
|
|
constraints=("a <= a - 1",))
|
|
|
|
with self.assertRaisesRegex(ValueError,
|
|
"Unsatisfiable.*a <= 0"):
|
|
a, b = shape_poly.symbolic_shape("a, b",
|
|
# Contradicts the default a >= 1
|
|
constraints=("a <= 0",))
|
|
a >= b
|
|
|
|
with self.assertRaisesRegex(ValueError,
|
|
"Unsatisfiable.*a <= 0"):
|
|
a, b = shape_poly.symbolic_shape("a, b",
|
|
# Contradicts the default a >= 1
|
|
constraints=("a <= 0",))
|
|
a >= b
|
|
|
|
def test_constraints_ge_smallest_first(self):
|
|
# Since we process the smaller constraints first, they take effect for the
|
|
# processing of the remaining constraints.
|
|
a, = shape_poly.symbolic_shape("a",
|
|
# max(a, 2) = a
|
|
constraints=("a >= 4", "max(a, 2) >= 6"))
|
|
self.assertEqual(core.max_dim(a, 2), a)
|
|
self.assertEqual(_bounds(a), (6, np.inf))
|
|
|
|
def test_constraints_ge_smallest_first_caching(self):
|
|
# When we process the constraint with max(a, 5) we will compute the bounds
|
|
# of (a, 5) and at that time we will cache that "-1 <= a - 5". We should
|
|
# not cache that, because later we learn that a >= 5
|
|
a, = shape_poly.symbolic_shape("a",
|
|
# max(a, 2) = a
|
|
constraints=("a >= 4", "max(a, 5) >= 5",
|
|
"max(a, 2) >= 5"))
|
|
self.assertEqual(core.max_dim(a, 2), a)
|
|
self.assertEqual(core.max_dim(a, 5), a)
|
|
|
|
def test_constraints_ge_a_minus_4d(self):
|
|
# simulates d = div(a, 4) and m = mod(a, 4)
|
|
assumptions = ["a >= 4*d + m ",
|
|
"a <= 4*d + m",
|
|
"m >= 0", "m <= 3"]
|
|
scope = shape_poly.SymbolicScope(assumptions)
|
|
a, d = shape_poly.symbolic_shape("a, d", scope=scope)
|
|
self.assertEqual(_bounds(a - 4*d), (1, 3)) # a - 4d = m >= 1
|
|
self.assertEqual(_bounds(a - 2*d), (3, np.inf)) # a - 2d = m + 2d >= 3
|
|
# TODO: The incompleteness is due to the way we combine external constraints
|
|
self.assertEqual(_bounds(a),
|
|
_expect(best=(5, np.inf), current=(4, np.inf))) # a >= 4d + m >= 5
|
|
|
|
def test_constraints_errors(self):
|
|
with self.assertRaisesRegex(ValueError,
|
|
"The symbolic constraints should be a sequence of strings"):
|
|
_ = shape_poly.symbolic_shape("a",
|
|
constraints="a <= a - 1")
|
|
|
|
with self.assertRaisesRegex(ValueError,
|
|
"Constraint parsing error: must contain one of '==' or '>=' or '<='"):
|
|
_ = shape_poly.symbolic_shape("a",
|
|
constraints=("a != 0",))
|
|
|
|
with self.assertRaisesRegex(ValueError,
|
|
re.escape("Unsatisfiable explicit constraint: a == a + 1")):
|
|
_ = shape_poly.symbolic_shape("a",
|
|
# Contradicts the default a >= 1
|
|
constraints=("a == a + 1",))
|
|
|
|
def test_constraints_ge_term(self):
|
|
a, b = shape_poly.symbolic_shape(
|
|
"a, b",
|
|
constraints=("max(a, b) >= 8", "min(a, b) <= 2",
|
|
"floordiv(a, 4) >= 2",
|
|
"mod(a, b) >= 2",
|
|
"mod(max(a, b), 4) >= 2"))
|
|
self.assertGreaterEqual(core.max_dim(a, b), 6)
|
|
self.assertLessEqual(core.min_dim(a, b), 6)
|
|
self.assertGreaterEqual(a // 4, 1)
|
|
self.assertGreaterEqual(a % b, 1)
|
|
self.assertGreaterEqual(core.max_dim(a, b) % 4, 2)
|
|
|
|
def test_constraints_ge_term_derived(self):
|
|
a, b = shape_poly.symbolic_shape(
|
|
"a, b",
|
|
constraints=("a >= 4", "3 >= b"))
|
|
self.assertGreaterEqual(a, b)
|
|
self.assertEqual(core.max_dim(a, b), a)
|
|
self.assertEqual(core.min_dim(a, b), b)
|
|
self.assertGreaterEqual(a // 3, 1)
|
|
|
|
self.assertEqual(_bounds(a + b), (5, np.inf))
|
|
self.assertEqual(_bounds(a - b), (1, np.inf))
|
|
|
|
def test_constraints_ge_not_term(self):
|
|
a, b = shape_poly.symbolic_shape("a, b",
|
|
constraints=("a >= b",))
|
|
self.assertGreaterEqual(a, b)
|
|
|
|
def test_constraints_ge_complex(self):
|
|
a, b, c = shape_poly.symbolic_shape(
|
|
"a, b, c",
|
|
constraints=("a + 2 <= b", "b <= a + 5", "a + b >= c"))
|
|
self.assertEqual(_bounds(b), (3, np.inf))
|
|
self.assertEqual(_bounds(b - a), (2, 5))
|
|
self.assertEqual(_bounds(b - a - 7), (-5, -2))
|
|
self.assertEqual(_bounds(c - 2*a - 5), (-np.inf, 0))
|
|
|
|
def test_constraints_ge_fractional(self):
|
|
a, = shape_poly.symbolic_shape("a",
|
|
constraints=("2 * a >= 5", "3 * a <= 10",))
|
|
self.assertEqual(_bounds(5*a - 2), (13, 13))
|
|
|
|
@jtu.parameterized_filterable(
|
|
kwargs=[
|
|
dict(constraint="2*a + 3*b >= 10", exp="a + b - 2",
|
|
bounds=(2, np.inf)),
|
|
dict(constraint="-2*a + 3*b >= 10", exp="a + 2*b",
|
|
bounds=(9, np.inf)),
|
|
dict(constraint="-2*a + -3*b >= -10", exp="-1*a + 2*b",
|
|
bounds=(-1, 3)),
|
|
dict(constraint="2*a + -3*b >= 10", exp="-1*a + 2*b", bounds=(-np.inf, np.inf)),
|
|
]
|
|
)
|
|
def test_constraints_ge_complex_gen(self,
|
|
constraint: str, exp: str,
|
|
bounds: tuple[float, float]):
|
|
a, b, exp = shape_poly.symbolic_shape(
|
|
"a, b, " + exp,
|
|
constraints=(constraint,))
|
|
self.assertEqual(bounds, _bounds(exp))
|
|
|
|
def test_constraints_ge_override(self):
|
|
# Some constaints override other
|
|
a, b = shape_poly.symbolic_shape("a, b",
|
|
constraints=("a >= 5", "b <= 16",
|
|
"a >= 10", "b <= 10"))
|
|
self.assertEqual(_bounds(a), (10, np.inf))
|
|
self.assertEqual(_bounds(b), (1, 10))
|
|
|
|
def test_constraint_eq_0(self):
|
|
a, b, c, d = shape_poly.symbolic_shape(
|
|
"a, b, c, d",
|
|
constraints=("b == a", "c == a + b", "d == 5"))
|
|
# Check that we have already applied the normalizaton rules
|
|
self.assertEqual(a._to_var(), "a")
|
|
self.assertEqual(b._to_var(), "a")
|
|
self.assertEqual(c._to_single_term(), (0, 2, a._to_term()))
|
|
self.assertIs(d, 5)
|
|
|
|
def test_constraints_eq_1(self):
|
|
# Some constaints override other
|
|
a, b, c = shape_poly.symbolic_shape("a, b, c",
|
|
constraints=("max(a, b) == c",))
|
|
self.assertEqual(_bounds(core.max_dim(a, b) - c + 3), (3, 3))
|
|
|
|
def test_constraints_eq_2(self):
|
|
a, b = shape_poly.symbolic_shape("a, b",
|
|
constraints=("max(a, b) == 5",
|
|
"min(a, b) == 3"))
|
|
self.assertEqual(_bounds(core.max_dim(a, b)), (5, 5))
|
|
self.assertEqual(_bounds(core.min_dim(a, b)), (3, 3))
|
|
self.assertEqual(_bounds(a), (3, 5))
|
|
|
|
def test_constraints_eq_3(self):
|
|
a, b = shape_poly.symbolic_shape(
|
|
"a, b",
|
|
# The constraint implies `b >= 2` thus `min(b, 2)` gets normalized
|
|
# to `2`
|
|
constraints=("min(a, b) == b - min(b, 2)",))
|
|
self.assertEqual(core.min_dim(b, 2), 2)
|
|
self.assertEqual(_bounds(b), (2, np.inf))
|
|
# TODO: the following ought to work, but the way we wrote the equality
|
|
# constraint, `min(b, 2)` gets rewritten to `2`.
|
|
#self.assertEqual(core.min_dim(a, b), b - core.min_dim(b, 2))
|
|
|
|
def test_constraints_eq_4(self):
|
|
# Equalities of a variable with an expression
|
|
a, b, c, d = shape_poly.symbolic_shape(
|
|
"a, b, c, d",
|
|
constraints=("floordiv(d, 2) == a + 5",
|
|
"floordiv(c, 3) == a + 3",
|
|
"b == a + 1"))
|
|
self.assertEqual(b, a + 1)
|
|
self.assertEqual(d // 2, a + 5)
|
|
self.assertEqual(c // 3, b + 2)
|
|
|
|
def test_constraints_eq_5(self):
|
|
# Constraints involving both inequalities and equalities
|
|
a, b, c, d = shape_poly.symbolic_shape(
|
|
"a, b, c, d",
|
|
constraints=(# max(c, a) == c because c >= b + 3 = a + 3
|
|
"b == a",
|
|
"c >= b + 3",
|
|
"floordiv(d, 2) == floordiv(max(c, a), 3) + 2",
|
|
))
|
|
self.assertEqual(core.max_dim(c, a), c)
|
|
# d // 2 = c // 3 + 2 = b + 5
|
|
self.assertEqual(_bounds(d // 2 - c // 3), (2, 2))
|
|
|
|
def test_constraints_eq_6(self):
|
|
# Constraints involving both inequalities and equalities
|
|
a, b, c, d = shape_poly.symbolic_shape(
|
|
"a, b, c, d",
|
|
constraints=("b == a",
|
|
"c >= b + 3",
|
|
"max(d, a) == b + 3",))
|
|
self.assertEqual(core.max_dim(c, a), c)
|
|
# d <= max(d, a) == a + 3, thus d - a <= 3
|
|
self.assertEqual(_bounds(d - b), (-np.inf, 3))
|
|
|
|
def test_constraints_eq_7(self):
|
|
# This came up in b/324989597
|
|
b, t = shape_poly.symbolic_shape(
|
|
"b, t",
|
|
constraints=(
|
|
"128 * b * floordiv(t + mod(-1*t, 128), 128) == b * t + b * mod(-1*t, 128)",))
|
|
t_ceil = t + (-t) % 128 # A multiple of 128, but hard to tell
|
|
self.assertEqual(128 * b * (t_ceil // 128), b * t_ceil)
|
|
|
|
b1, t1 = shape_poly.symbolic_shape(
|
|
"b1, t1",
|
|
constraints=(
|
|
"128 * floordiv(t1 + mod(-1*t1, 128), 128) == t1 + mod(-1*t1, 128)",))
|
|
t1_ceil = t1 + (-t1) % 128 # A multiple of 128, but hard to tell
|
|
self.assertEqual(128 * (t1_ceil // 128), t1_ceil)
|
|
self.assertEqual(128 * b1 * (t1_ceil // 128), b1 * t1_ceil)
|
|
|
|
def test_constraints_eq_bug_23456(self):
|
|
b, = jax.export.symbolic_shape('b', constraints=['b==5'])
|
|
jax.eval_shape(lambda k: jnp.tile(k, 3), jax.ShapeDtypeStruct((b,), jnp.float32))
|
|
|
|
def test_constraints_eq_bug_23437(self):
|
|
def f1(x, y):
|
|
return x + y
|
|
|
|
x = jnp.ones((4,), dtype=jnp.int32)
|
|
y = jnp.ones((4,), dtype=jnp.int32)
|
|
args_specs = jax.export.symbolic_args_specs((x, y), ("a*2", "b*2"), constraints=("a==b",))
|
|
exp = jax.export.export(jax.jit(f1))(*args_specs)
|
|
self.assertEqual(exp.in_avals[0], exp.in_avals[1])
|
|
|
|
def test_constraints_eq_threefry(self):
|
|
# Test equalities that arise out of the threefry lowering
|
|
# x : i32[a] # a may be even or odd
|
|
# x_padded: i32[a + a % 2] = jnp.concat([x, jnp.zeros((a % 2,))])
|
|
# x_reshaped: i32[(a + a % 2) // 2, 2] = x_padded.reshape((-1, 2))
|
|
# x_1: i32[a + a % 2] = x_reshaped.reshape((-1,))
|
|
a, = shape_poly.symbolic_shape(
|
|
"a",
|
|
constraints=("mod(a + mod(a, 2), -2) == 0",))
|
|
|
|
x_reshaped, r = divmod(a + a % 2, -2)
|
|
self.assertEqual(r, 0)
|
|
self.assertEqual(- x_reshaped, -1 * ((a + a % 2) // -2))
|
|
self.assertEqual(-2 * x_reshaped, a + a % 2)
|
|
|
|
def test_constraints_eq_mod_0(self):
|
|
# mod(b, N) == 0 is a common constraint, we need to ensure we can use it
|
|
# to infer things like: N * floordiv(b, N) == b, b >= N.
|
|
b, c, d = shape_poly.symbolic_shape(
|
|
"b, c, d",
|
|
constraints=("mod(b, 4) == 0",))
|
|
|
|
# Inequalities work, because we use more expensive reasoning
|
|
self.assertGreaterEqual(b, 4 * (b // 4))
|
|
self.assertGreaterEqual(4 * (b // 4), b)
|
|
# Equalities used to fail
|
|
self.assertEqual(b, 4 * (b // 4))
|
|
# And an equality that may come up in a reshape
|
|
self.assertEqual(math.prod([b, c, d]), math.prod([b // 4, c, d, 2, 2]))
|
|
|
|
self.assertGreaterEqual(b, b // 4)
|
|
self.assertGreaterEqual(b, 3 * (b // 4))
|
|
|
|
def test_constraints_eq_a_minus_4d(self):
|
|
# simulates d = div(a, 4) and m = mod(a, 4)
|
|
constraints = ["4*d == a - m", "m >= 0", "m <= 3"]
|
|
scope = shape_poly.SymbolicScope(constraints)
|
|
a, d = shape_poly.symbolic_shape("a, d", scope=scope)
|
|
self.assertEqual(_bounds(a - 4*d), (1, 3)) # a - 4d = m >= 1
|
|
# TODO: The incompleteness is due to the way we combine external constraints
|
|
self.assertEqual(_bounds(a - 2*d),
|
|
_expect(best=(3, np.inf), current=(-np.inf, np.inf))) # a - 2d = m + 2d >= 3
|
|
# TODO: The incompleteness is due to the way we combine external constraints
|
|
self.assertEqual(_bounds(a),
|
|
_expect(best=(5, np.inf), current=(4, np.inf))) # a >= 4d + m >= 5
|
|
|
|
# Now with a different order of constraints
|
|
constraints1 = ["m1 >= 0", "m1 <= 3", "a1 == 4*d1 + m1"]
|
|
scope1 = shape_poly.SymbolicScope(constraints1)
|
|
a1, d1, m1 = shape_poly.symbolic_shape("a1, d1, m1", scope=scope1)
|
|
self.assertEqual(_bounds(a1 - 4*d1), (1, 3)) # a - 4d = m >= 1
|
|
self.assertEqual(_bounds(a1 - 2*d1), (3, np.inf)) # a - 2d = m + 2d >= 3
|
|
self.assertEqual(_bounds(a1), (5, np.inf)) # a >= 4d + m >= 5
|
|
|
|
def test_constraints_eq_geq(self):
|
|
# We ensure that an equality constraint it is usable not just for
|
|
# normalization but also for inequality reasoning.
|
|
a, b = export.symbolic_shape(
|
|
"a, b", constraints=["4 * a == b"])
|
|
self.assertGreaterEqual(b, a)
|
|
self.assertGreaterEqual(b, 3*a)
|
|
self.assertGreaterEqual(b, 4 * a)
|
|
self.assertGreaterEqual(5 * a, b)
|
|
self.assertGreaterEqual(9 * a, 2*b)
|
|
|
|
def test_constraints_error_msg(self):
|
|
a, b = shape_poly.symbolic_shape("a, b",
|
|
constraints=("a >= 5",))
|
|
with self.assertRaisesRegex(
|
|
core.InconclusiveDimensionOperation,
|
|
re.compile("with constraints.*"
|
|
r"a >= 5", re.DOTALL)):
|
|
self.assertGreaterEqual(a, 6)
|
|
|
|
def test_scope_sharing(self):
|
|
a, b = shape_poly.symbolic_shape("a, b",
|
|
constraints=("a >= 5", "a <= 10"))
|
|
self.assertIs(a.scope, b.scope)
|
|
self.assertEqual(_bounds(a), (5, 10))
|
|
|
|
def test_constraints_seq(self):
|
|
a1, a2, a3, a4, a5 = shape_poly.symbolic_shape(
|
|
"a1, a2, a3, a4, a5",
|
|
constraints=(
|
|
"a1 >= a2",
|
|
"a2 >= a3",
|
|
"a3 >= a4",
|
|
"a4 >= a5",
|
|
)
|
|
)
|
|
self.assertEqual(_bounds(a1 - a5), (0, np.inf))
|
|
|
|
def test_constraints_rounding_terms(self):
|
|
a1, a2 = shape_poly.symbolic_shape(
|
|
"a1, a2",
|
|
constraints=(
|
|
# a1 >= 2 and a1 <= 2
|
|
"2 * a1 >= 3", "-2 * a1 >= -5"
|
|
)
|
|
)
|
|
self.assertEqual(_bounds(a1), (2, 2))
|
|
|
|
def test_constraints_rounding_not_terms(self):
|
|
a1, a2 = shape_poly.symbolic_shape(
|
|
"a1, a2",
|
|
constraints=(
|
|
# a1 >= a2 + 2 and a1 <= a2 + 2
|
|
"2*a1 >= 2*a2 + 3", "-2*a1 + 2*a2 >= -5"
|
|
)
|
|
)
|
|
self.assertEqual(_bounds(a1 - a2 - 2), (0, 0))
|
|
self.assertEqual(_bounds(a2 - a1 + 2), (0, 0))
|
|
|
|
def test_constraints_unsat_trivial(self):
|
|
with self.assertRaisesRegex(ValueError,
|
|
r"Unsatisfiable explicit constraint: a1 >= a1 \+ 1"):
|
|
_ = shape_poly.symbolic_shape(
|
|
"a1", constraints=("a1 >= a1 + 1",))
|
|
|
|
def test_constraints_unsat_terms(self):
|
|
with self.assertRaisesRegex(ValueError,
|
|
"Unsatisfiable constraint"):
|
|
a1, a2, *_ = shape_poly.symbolic_shape(
|
|
"a1, a2, a3, a4",
|
|
constraints=(
|
|
# The following -> a1 >= 5
|
|
"a1 >= a3 + 1", "a3 >= 4",
|
|
# The following -> a1 <= 4
|
|
"a1 <= a4 + 2", "a4 <= 2"))
|
|
a1 >= a2
|
|
|
|
def test_constraints_unsat_not_terms(self):
|
|
with self.assertRaisesRegex(ValueError,
|
|
"Unsatisfiable constraint"):
|
|
a1, a2, a3, a4 = shape_poly.symbolic_shape(
|
|
"a1, a2, a3, a4",
|
|
constraints=(
|
|
# The following -> a1 >= a2 + 5
|
|
"a1 >= a3 + 1", "a3 >= a2 + 4",
|
|
# The following -> a1 <= a2 + 4
|
|
"a1 <= a4 + 2", "a4 <= a2 + 2"))
|
|
|
|
self.assertGreaterEqual(a1, a2)
|
|
|
|
def test_constraints_sharing(self):
|
|
a, = shape_poly.symbolic_shape("a",
|
|
constraints=("a >= 5", "a <= 10"))
|
|
self.assertEqual(_bounds(a), (5, 10))
|
|
# The constraints order does not matter, and they are canonicalized
|
|
a1, = shape_poly.symbolic_shape("a",
|
|
constraints=("2*a + 5 <= a + 15", "a >= 5"))
|
|
self.assertNotEqual(a, a1) # Two separate scopes are not equal
|
|
self.assertNotEqual(hash(a), hash(a1)) # Different hash because of different scopes
|
|
self.assertIsNot(a.scope, a1.scope)
|
|
|
|
def test_constraints_different_scope(self):
|
|
a, = shape_poly.symbolic_shape("a",
|
|
constraints=("a >= 5", "a <= 10"))
|
|
self.assertEqual(_bounds(a), (5, 10))
|
|
a1, = shape_poly.symbolic_shape("a",
|
|
constraints=("a <= 10",))
|
|
self.assertNotEqual(a, a1)
|
|
self.assertNotEqual(hash(a), hash(a1)) # Hope there is no collision
|
|
self.assertIsNot(a.scope, a1.scope)
|
|
|
|
# Test the error message
|
|
with self.subTest(msg="error_message"):
|
|
with self.assertRaisesRegex(ValueError,
|
|
re.compile(
|
|
"Invalid mixing of symbolic scopes.*"
|
|
"with constraints.*"
|
|
"a >= 5.*a <= 10.*"
|
|
"and found.*with constraints.*"
|
|
"a <= 10",
|
|
re.DOTALL)):
|
|
a + a1
|
|
|
|
for o in (op.add, op.sub, op.mul, op.mod, op.floordiv,
|
|
op.ge, op.gt, op.le, op.lt, core.max_dim, core.min_dim):
|
|
with self.subTest(o=str(o)):
|
|
with self.assertRaisesRegex(ValueError,
|
|
"Invalid mixing of symbolic scopes"):
|
|
o(a, a1)
|
|
|
|
|
|
class PolyHarness(Harness):
|
|
"""Tests a function with shape polymorphism.
|
|
|
|
Exports `fun` with shape polymorphism, then checks that the JAX native and
|
|
the exported function produce the same results.
|
|
"""
|
|
def __init__(self,
|
|
group_name: str, name: str,
|
|
fun: Callable[..., Any],
|
|
*,
|
|
arg_descriptors: Sequence[test_harnesses.ArgDescriptor] = (),
|
|
polymorphic_shapes: Sequence[str | None] = (),
|
|
symbolic_constraints: Sequence[str] = (),
|
|
expect_error: tuple[Any, str] | None = None,
|
|
check_result: bool = True,
|
|
tol: float | None = None,
|
|
limitations: Sequence[test_harnesses.Limitation] = (),
|
|
override_jax_config_flags: dict[str, Any] = {}):
|
|
"""Args:
|
|
|
|
group_name, name: The name for the harness. See `Harness.__init__`.
|
|
fun: the function to be converted. See `Harness.__init__`.
|
|
arg_descriptors: The argument descriptors. See `Harness.__init__`.
|
|
polymorphic_shapes: For `export.args_specs`.
|
|
symbolic_constraints: For `export.args_specs`.
|
|
expect_error: an optional pair of an Exception type and a regular
|
|
expression to match the expected exception string.
|
|
We expect this error during tracing and exporting with shape
|
|
polymorphism.
|
|
check_result: specifies if we want to check that the result of invoking
|
|
the shape polymorphic export produces the same result as the
|
|
native JAX function.
|
|
tol: the tolerance to use for checking results.
|
|
limitations: a sequence of Limitation(s), used for obtaining the default
|
|
tolerance (if `tol` is not specified).
|
|
override_jax_config_flags: jax.config flags to override for the duration
|
|
of the test.
|
|
"""
|
|
super().__init__(group_name, name, fun, arg_descriptors,
|
|
dtype=np.float32)
|
|
self.polymorphic_shapes = polymorphic_shapes
|
|
self.symbolic_constraints = symbolic_constraints
|
|
self.expect_error = expect_error
|
|
self.tol = tol
|
|
self.check_result = check_result
|
|
self.limitations = limitations
|
|
self.override_jax_config_flags = override_jax_config_flags
|
|
|
|
def run_test(self, tst: jtu.JaxTestCase) -> jax.Array | None:
|
|
def log_message(extra: str):
|
|
return f"[{tst._testMethodName}]: {extra}"
|
|
|
|
# Check that we have overridden the jax.config flags
|
|
for fname, fvalue in self.override_jax_config_flags.items():
|
|
tst.assertEqual(getattr(jax.config, fname), fvalue, (
|
|
f"Flag {fname} current value {getattr(jax.config, fname)} != {fvalue}"))
|
|
|
|
f_jax = jax.jit(self.dyn_fun)
|
|
args = self.dyn_args_maker(tst.rng())
|
|
args = jax.tree.map(jnp.array, args)
|
|
args_specs = export.symbolic_args_specs(args, self.polymorphic_shapes,
|
|
constraints=self.symbolic_constraints)
|
|
|
|
if self.expect_error is not None:
|
|
with tst.assertRaisesRegex(self.expect_error[0], self.expect_error[1]):
|
|
export.export(f_jax)(*args_specs)
|
|
return None
|
|
|
|
exp = export.export(f_jax)(*args_specs)
|
|
if not self.check_result:
|
|
return None
|
|
# Run the JAX natively and then the exported function and compare
|
|
res_jax_native = f_jax(*args)
|
|
res_jax_exported = exp.call(*args)
|
|
custom_assert_lims = [
|
|
l for l in self.limitations if l.custom_assert is not None]
|
|
assert len(custom_assert_lims) <= 1, custom_assert_lims
|
|
tol = None
|
|
if self.tol is not None:
|
|
tol = self.tol
|
|
elif self.limitations:
|
|
max_lim = self.limitations[0].get_max_tolerance_limitation(
|
|
self.limitations)
|
|
if max_lim is not None:
|
|
tol = max_lim.tol
|
|
|
|
if not custom_assert_lims:
|
|
tst.assertAllClose(res_jax_native, res_jax_exported,
|
|
atol=tol, rtol=tol)
|
|
else:
|
|
logging.info(log_message(
|
|
f"Running custom_assert with tol={tol} due "
|
|
f"to {custom_assert_lims[0]}"))
|
|
custom_assert_lims[0].custom_assert(tst, res_jax_native,
|
|
res_jax_exported, args=args, # type: ignore
|
|
tol=tol, err_msg=None)
|
|
return res_jax_exported
|
|
|
|
|
|
def check_shape_poly(tst, f_jax: Callable, *,
|
|
arg_descriptors: Sequence[test_harnesses.ArgDescriptor] = (),
|
|
polymorphic_shapes: Sequence[str | None] = (),
|
|
symbolic_constraints: Sequence[str] = (),
|
|
expect_error=None) -> jax.Array | None:
|
|
# Builds a PolyHarness and runs the test. See PolyHarness documentation.
|
|
h = PolyHarness("", "", jax.jit(f_jax),
|
|
arg_descriptors=arg_descriptors,
|
|
polymorphic_shapes=polymorphic_shapes,
|
|
symbolic_constraints=symbolic_constraints,
|
|
expect_error=expect_error)
|
|
return h.run_test(tst)
|
|
|
|
|
|
class ShapePolyTest(jtu.JaxTestCase):
|
|
|
|
def setUp(self):
|
|
_start_profile(self)
|
|
super().setUp()
|
|
|
|
def tearDown(self):
|
|
super().tearDown()
|
|
_stop_profile(self)
|
|
|
|
def test_simple_unary(self):
|
|
"""Test shape polymorphism for a simple case, unary function."""
|
|
|
|
def f_jax(x):
|
|
return x + jnp.sin(x)
|
|
|
|
for polymorphic_shapes in [None, "_, h", "h, h"]:
|
|
with self.subTest(shapes=polymorphic_shapes):
|
|
check_shape_poly(self,
|
|
f_jax,
|
|
arg_descriptors=[RandArg((3, 3), _f32)],
|
|
polymorphic_shapes=polymorphic_shapes)
|
|
|
|
@jtu.parameterized_filterable(
|
|
kwargs=[
|
|
dict(testcase_name=f"expr={name}", expr=expr)
|
|
for name, expr in [
|
|
("d + 2", lambda d: d + 2),
|
|
("2 - d", lambda d: 2 - d),
|
|
("d * 2", lambda d: d * 2),
|
|
("d * d", lambda d: d * d),
|
|
("(- d) * d", lambda d: (- d) * d),
|
|
("d * d - d", lambda d: d * d - d),
|
|
# Division
|
|
("d // 2", lambda d: d // 2),
|
|
("(d + 1) // 2", lambda d: (d + 1) // 2),
|
|
("d // -2", lambda d: d // -2),
|
|
("(d + 1) // -2", lambda d: (d + 1) // -2),
|
|
("(-d) // 2", lambda d: (-d) // 2),
|
|
("(-d - 1) // 2", lambda d: (-d - 1) // 2),
|
|
("(-d) // -2", lambda d: (-d) // -2),
|
|
("(-d - 1) // -2", lambda d: (-d - 1) // -2),
|
|
# Remainder
|
|
("d % 2", lambda d: d % 2),
|
|
("(d + 1) % 2", lambda d: (d + 1) % 2),
|
|
("d % -2", lambda d: d % -2),
|
|
("(d + 1) % -2", lambda d: (d + 1) % -2),
|
|
("(-d) % 2", lambda d: (-d) % 2),
|
|
("(-d - 1) % 2", lambda d: (-d - 1) % 2),
|
|
("(-d) % -2", lambda d: (-d) % -2),
|
|
("(-d - 1) % -2", lambda d: (-d - 1) % -2),
|
|
]
|
|
])
|
|
def test_non_trivial_dim_expr(self, expr=lambda d: d % -2):
|
|
# Check the lowering for shape expressions
|
|
check_shape_poly(
|
|
self,
|
|
lambda x: x[0] * 0 + expr(x.shape[0]),
|
|
arg_descriptors=[RandArg((3,), np.int64)],
|
|
polymorphic_shapes=["b"])
|
|
|
|
@jtu.parameterized_filterable(
|
|
# The function `f` will be called with x: f32[b]
|
|
kwargs=[
|
|
dict(testcase_name="cube", f=lambda x: x.shape[0] ** 3),
|
|
dict(testcase_name="zero", f=lambda x: x.shape[0] ** 0),
|
|
dict(testcase_name="rpow", f=lambda x: 2 ** x.shape[0]),
|
|
dict(testcase_name="negative",
|
|
f=lambda x: x.shape[0] ** -2,
|
|
expect_error=(ValueError, "cannot be raised to negative powers")),
|
|
dict(testcase_name="non_integer",
|
|
f=lambda x: x.shape[0] ** 1.5,
|
|
expect_error=(ValueError, "cannot be raised to non-integer powers")),
|
|
dict(testcase_name="sym_pow",
|
|
f=lambda x: x.shape[0] ** x.shape[0]),
|
|
]
|
|
)
|
|
def test_pow(self, f, expect_error: tuple[Exception, str] | None = None):
|
|
check_shape_poly(self,
|
|
f,
|
|
arg_descriptors=[RandArg((3,), np.float32)],
|
|
polymorphic_shapes=["b"],
|
|
expect_error=expect_error)
|
|
|
|
def test_static_shape_result(self):
|
|
"""The result has static shape."""
|
|
|
|
def f_jax(x):
|
|
return jnp.sum(x + jnp.sin(x), axis=0)
|
|
|
|
check_shape_poly(self,
|
|
f_jax,
|
|
arg_descriptors=[RandArg((2, 3), _f32)],
|
|
polymorphic_shapes=[None])
|
|
|
|
check_shape_poly(self,
|
|
f_jax,
|
|
arg_descriptors=[RandArg((2, 3), _f32)],
|
|
polymorphic_shapes=["b, _"])
|
|
|
|
def test_kwargs(self):
|
|
"""Test shape polymorphism for a function with kwargs."""
|
|
|
|
x = np.ones(3, dtype=np.float32)
|
|
y = np.ones(1, dtype=np.float32)
|
|
def f_jax(x, *, y):
|
|
return x + jnp.sin(y)
|
|
|
|
exp = export.export(jax.jit(f_jax))(
|
|
jax.ShapeDtypeStruct(export.symbolic_shape("b"), x.dtype),
|
|
y=jax.ShapeDtypeStruct(y.shape, y.dtype))
|
|
self.assertAllClose(f_jax(x, y=y), exp.call(x, y=y))
|
|
|
|
def test_arg_avals_errors(self):
|
|
"""Test error reporting for shape polymorphism."""
|
|
def conv_and_run(*, arg_shape: core.Shape,
|
|
polymorphic_shape: str):
|
|
arg = np.arange(math.prod(arg_shape), dtype=np.float32).reshape(arg_shape)
|
|
check_shape_poly(self, lambda x: x,
|
|
arg_descriptors=[arg],
|
|
polymorphic_shapes=[polymorphic_shape])
|
|
|
|
with self.assertRaisesRegex(ValueError,
|
|
re.escape("polymorphic shape spec should be")):
|
|
conv_and_run(arg_shape=(2,), polymorphic_shape=5.)
|
|
|
|
with self.assertRaisesRegex(ValueError,
|
|
re.escape("pytree structure error: different types")):
|
|
conv_and_run(arg_shape=(2,), polymorphic_shape=["a list"])
|
|
|
|
with self.assertRaisesRegex(ValueError,
|
|
re.escape("pytree structure error: different types")):
|
|
conv_and_run(arg_shape=(2,), polymorphic_shape=("a tuple",))
|
|
|
|
with self.assertRaisesRegex(ValueError,
|
|
"Cannot solve for values of dimension variables {'b'}"):
|
|
conv_and_run(arg_shape=(4, 36, 3), polymorphic_shape="b * b, b * d * d, d")
|
|
|
|
with self.assertRaisesRegex(ValueError,
|
|
"Division had remainder 2 when computing the value of 'b'"):
|
|
conv_and_run(arg_shape=(5, 36), polymorphic_shape="3 * b, ...")
|
|
|
|
with self.assertRaisesRegex(ValueError,
|
|
"Expected value >= 1 for dimension variable 'b'"):
|
|
conv_and_run(arg_shape=(10, 3), polymorphic_shape="3 * b + 10, ...")
|
|
|
|
with self.assertRaisesRegex(ValueError,
|
|
"Expected value >= 1 for dimension variable 'b'"):
|
|
conv_and_run(arg_shape=(7, 3), polymorphic_shape="3 * b + 10, ...")
|
|
|
|
with self.assertRaisesRegex(
|
|
ValueError,
|
|
re.escape(
|
|
"Found inconsistency between dimension size "
|
|
"args[0].shape[1] (= 3) and the specification 'a' (= 2)")):
|
|
conv_and_run(arg_shape=(2, 3), polymorphic_shape="(a, a)")
|
|
|
|
def test_pytree(self):
|
|
"""Arguments and polymorphic_shapes are pytrees."""
|
|
|
|
# Arguments are of the form [([x00, x01], [x10]), dict(a=ya, b=yb)]
|
|
def add_all_jax(x_pair_of_list, y_dict):
|
|
x_list_0, x_list_1 = x_pair_of_list
|
|
return functools.reduce(op.add,
|
|
x_list_0 + x_list_1 + [y_dict["a"], y_dict["b"]])
|
|
|
|
x = np.arange(4, dtype=_f32)
|
|
args = (([x, x], [x]), dict(a=x, b=x))
|
|
check_shape_poly(self,
|
|
add_all_jax,
|
|
arg_descriptors=args,
|
|
polymorphic_shapes=[(["v", "v"], ["v"]),
|
|
dict(a="v", b="v")])
|
|
|
|
# Prefix polymorphic shapes
|
|
check_shape_poly(self,
|
|
add_all_jax,
|
|
arg_descriptors=args,
|
|
polymorphic_shapes="v")
|
|
|
|
check_shape_poly(self,
|
|
add_all_jax,
|
|
arg_descriptors=args,
|
|
polymorphic_shapes=["v", "v"])
|
|
|
|
check_shape_poly(self,
|
|
add_all_jax,
|
|
arg_descriptors=args,
|
|
polymorphic_shapes=[("v", "v"), "v"])
|
|
|
|
# Now partial polymorphic_shapes.
|
|
check_shape_poly(self,
|
|
add_all_jax,
|
|
arg_descriptors=args,
|
|
polymorphic_shapes=[(["(4,)", "(_,)"], [("4,")]),
|
|
dict(a="(_,)", b="(4,)")])
|
|
|
|
@jtu.parameterized_filterable(
|
|
kwargs=[
|
|
dict(testcase_name=name, polymorphic_shapes=polymorphic_shapes)
|
|
for name, polymorphic_shapes in [
|
|
("1", ("b", "b", "b")),
|
|
("2", dict(a="b")),
|
|
("3", (dict(a="b"), "b")),
|
|
]]
|
|
)
|
|
def test_pytree_errors(self, polymorphic_shapes=("b", "b", "b")):
|
|
"""Arguments and polymorphic_shapes are not-matching pytrees."""
|
|
|
|
# Arguments are of the form [([x00, x01], [x10]), dict(a=ya, b=yb)]
|
|
x = np.arange(4, dtype=_f32)
|
|
args = (([x, x], [x]), dict(a=x, b=x))
|
|
def add_all_jax(x_pair_of_list, y_dict):
|
|
x_list_0, x_list_1 = x_pair_of_list
|
|
return functools.reduce(op.add,
|
|
x_list_0 + x_list_1 + [y_dict["a"], y_dict["b"]])
|
|
|
|
with self.assertRaisesRegex(ValueError, "pytree structure error"):
|
|
check_shape_poly(self,
|
|
add_all_jax,
|
|
arg_descriptors=args,
|
|
polymorphic_shapes=polymorphic_shapes)
|
|
|
|
def test_with_nested_jit(self):
|
|
def f_jax(x): # x: f32[w, h]
|
|
return jnp.sin(x) + jnp.arange(x.shape[0] * x.shape[1],
|
|
dtype=x.dtype).reshape(x.shape)
|
|
check_shape_poly(self,
|
|
lambda x: x + jax.jit(f_jax)(x),
|
|
arg_descriptors=[RandArg((3, 4), _f32)],
|
|
polymorphic_shapes=["a, b"])
|
|
|
|
@jtu.parameterized_filterable(
|
|
kwargs=[
|
|
dict(testcase_name=str(polymorphic_shapes), polymorphic_shapes=polymorphic_shapes)
|
|
# The polymorphic_shapes should have three comma-separated DimExpr matching
|
|
# 16, 24, 32
|
|
for polymorphic_shapes in [
|
|
"b1+6,b1+14,b2", # b1=10, b2=32
|
|
"2*b1,4*b2,b1+b2+18", # b1=8,b2=6
|
|
"b1+2*b2,4*b2,b1*b1+16", # b1=4,b2=6
|
|
]
|
|
])
|
|
def test_non_trivial_polynomials_spec(self,
|
|
polymorphic_shapes="2*b1,4*b2,b1+b2+18"):
|
|
# We can handle non-trivial polynomials in the input shape,
|
|
# as long as all variables also occur in trivial expressions
|
|
check_shape_poly(self,
|
|
lambda x: 2 * x.shape[0] + 3 * x.shape[1] + 4 * x.shape[2],
|
|
arg_descriptors=[RandArg((16, 24, 32), _f32)],
|
|
polymorphic_shapes=[polymorphic_shapes])
|
|
|
|
def test_constraints_basic(self):
|
|
def f(x): # x: i32[a], with a >= 8
|
|
assert x.shape[0] >= 6
|
|
check_shape_poly(self, f,
|
|
arg_descriptors=[RandArg((16,), _i32)],
|
|
polymorphic_shapes=["a"],
|
|
symbolic_constraints=["a >= 8"])
|
|
|
|
def test_constraints_slice_in_dim(self):
|
|
def f(x): # x: i32[a], with a >= 8
|
|
return lax.dynamic_slice_in_dim(x, 0, 8, 0)
|
|
check_shape_poly(self, f,
|
|
arg_descriptors=[RandArg((16,), _i32)],
|
|
polymorphic_shapes=["a"],
|
|
symbolic_constraints=["a >= 8"])
|
|
|
|
def test_constraints_slice_in_dim_eq(self):
|
|
def f(x, y): # x: i32[a], y: i32[b]
|
|
v1 = x[:y.shape[0]] # i32[min(a, b)]
|
|
v2 = y[2:] # i32[b - min(b, 2)]
|
|
return v1 + v2
|
|
check_shape_poly(self, f,
|
|
arg_descriptors=[RandArg((16,), _i32), # a == 16
|
|
RandArg((18,), _i32)], # b == 18
|
|
polymorphic_shapes=["a", "b"],
|
|
# This is the exact failure we are getting.
|
|
symbolic_constraints=[
|
|
"b >= 2",
|
|
"min(a, b) == b - 2"
|
|
])
|
|
|
|
def test_constraints_eq_threefry(self):
|
|
# This pattern arises in the lowering of threefry
|
|
def f(x): # x: i32[a]
|
|
a, = x.shape
|
|
x_padded = jnp.concatenate([x, jnp.zeros((a % 2,), dtype=x.dtype)])
|
|
x_reshaped = x_padded.reshape((2, -1))
|
|
x_reshaped += 2
|
|
x_padded_1 = x_reshaped.reshape((-1,))
|
|
x_1 = x_padded_1[:a]
|
|
# Ensure x and x_1 have the same shape
|
|
return x + x_1
|
|
|
|
check_shape_poly(self, f,
|
|
arg_descriptors=[RandArg((16,), _i32)], # a == 16
|
|
polymorphic_shapes=["a"],
|
|
# These are the exact failure we are getting.
|
|
symbolic_constraints=[
|
|
"mod(a + mod(a, 2), -2) == 0",
|
|
"-2*floordiv(a + mod(a, 2), -2) == a + mod(a, 2)"])
|
|
|
|
def test_constraints_eq_mod_0(self):
|
|
# mod(b, N) == 0 is a common constraint, we need to ensure we can use it
|
|
# to infer things like: N * floordiv(b, N) == b, b >= N.
|
|
def f(x): # x: f32[b] and b % 4 == 0
|
|
b = x.shape[0]
|
|
y1 = jnp.ones((1, 3, 4, b // 4), dtype=x.dtype)
|
|
y2 = y1.reshape((1, 3, -1)) # : f32[1, 3, b]
|
|
y3 = x.reshape((1, 1, b)) + y2 # : f32[1, 3, b]
|
|
|
|
slice0 = lax.slice(x, (0,), (b // 4,)) # Requires b >= b // 4
|
|
slice1 = lax.slice(x, (0,), (2 * (b // 4),)) # Requires b >= 2 * (b // 4)
|
|
slice2 = lax.slice(x, (0,), (3 * (b // 4),)) # Requires b >= 2 * (b // 4)
|
|
slice3 = lax.slice(x, (0,), (4 * (b // 4),)) # Requires b >= 2 * (b // 4)
|
|
return (jnp.sum(y3) +
|
|
jnp.sum(slice0) + jnp.sum(slice1) +
|
|
jnp.sum(slice2) + jnp.sum(slice3))
|
|
|
|
check_shape_poly(self, f,
|
|
arg_descriptors=[RandArg((16,), _i32)],
|
|
polymorphic_shapes=["b"],
|
|
symbolic_constraints=["mod(b, 4) == 0"])
|
|
|
|
def test_constraints_for_profile(self):
|
|
# A somewhat more involved tests to stress test the correctness and
|
|
# performance
|
|
def f(x): # x: i32[a, b]
|
|
acc = 0
|
|
for start in range(0, 50):
|
|
slice = x[start::2] # exercises floordiv and min
|
|
acc += jnp.sum(slice, axis=0)
|
|
|
|
slice = x[start:(x.shape[0] - x.shape[0] % 2):2] # exercises max and min
|
|
acc += jnp.sum(slice, axis=0)
|
|
return acc
|
|
|
|
_ = export.export(jax.jit(f))(
|
|
jax.ShapeDtypeStruct(export.symbolic_shape("a, b"), np.int32))
|
|
|
|
def test_constraints_ge_compile_time_check(self):
|
|
def f(x): # x: i32[a]
|
|
a = x.shape[0]
|
|
assert _bounds(a) == (2, 4)
|
|
return lax.dynamic_slice_in_dim(x, 1, 2, 0)
|
|
|
|
x_spec = jax.ShapeDtypeStruct(
|
|
export.symbolic_shape("a",
|
|
constraints=["a >= 2", "a <= 4"]), np.int32)
|
|
exp = export.export(jax.jit(f))(x_spec)
|
|
|
|
x_2 = np.arange(2, dtype=np.int32)
|
|
res_2 = exp.call(x_2)
|
|
self.assertAllClose(x_2[0:2], res_2)
|
|
|
|
x_4 = np.arange(4, dtype=np.int32)
|
|
res_4 = exp.call(x_4)
|
|
self.assertAllClose(x_4[1:3], res_4)
|
|
|
|
with self.assertRaisesRegex(
|
|
ValueError,
|
|
re.escape("Expected 'a - 2' to be greater or equal to 0, but found -1")):
|
|
exp.call(np.arange(1, dtype=np.int32))
|
|
|
|
with self.assertRaisesRegex(
|
|
ValueError,
|
|
re.escape("Expected '- a + 4' to be greater or equal to 0, but found -1")):
|
|
exp.call(np.arange(5, dtype=np.int32))
|
|
|
|
def test_constraints_eq_0_compile_time_check(self):
|
|
def f(x): # x: i32[a, b]
|
|
return x
|
|
|
|
x_spec = jax.ShapeDtypeStruct(
|
|
export.symbolic_shape("a, b",
|
|
constraints=["max(a, b) == b"]), np.int32)
|
|
exp = export.export(jax.jit(f))(x_spec)
|
|
with self.assertRaisesRegex(
|
|
ValueError,
|
|
re.escape("Expected 'max(a, b) - b' to be equal to 0, but found 1")):
|
|
exp.call(np.ones((3, 2), dtype=np.int32))
|
|
|
|
def test_constraints_eq_1_compile_time_check(self):
|
|
def f(x): # x: i32[a, b]
|
|
return x
|
|
|
|
x_spec = jax.ShapeDtypeStruct(
|
|
export.symbolic_shape("a, b",
|
|
constraints=["a == b"]), np.int32)
|
|
exp = export.export(jax.jit(f))(x_spec)
|
|
exp.call(np.ones((3, 3), dtype=np.int32))
|
|
|
|
def test_constraints_eq_2_compile_time_check(self):
|
|
def f(x): # x: i32[a, b]
|
|
return x
|
|
|
|
x_spec = jax.ShapeDtypeStruct(
|
|
export.symbolic_shape("a, b",
|
|
constraints=["max(a, b) == 4", "a == b"]), np.int32)
|
|
exp = export.export(jax.jit(f))(x_spec)
|
|
with self.assertRaisesRegex(
|
|
ValueError,
|
|
re.escape("Expected 'max(a, b) - 4' to be equal to 0, but found -1")):
|
|
exp.call(np.ones((3, 3), dtype=np.int32))
|
|
|
|
def test_caching_with_scopes(self):
|
|
f_tracing_count = 0
|
|
expected_a_bounds = (1, np.inf)
|
|
@jax.jit
|
|
def f(x): # x: i32[a]
|
|
nonlocal f_tracing_count
|
|
f_tracing_count += 1
|
|
a = x.shape[0]
|
|
assert _bounds(a) == expected_a_bounds
|
|
|
|
x_spec = jax.ShapeDtypeStruct(export.symbolic_shape("a"), np.int32)
|
|
_ = export.export(f)(x_spec)
|
|
self.assertEqual(1, f_tracing_count)
|
|
_ = export.export(f)(x_spec) # This hits the tracing cache
|
|
self.assertEqual(1, f_tracing_count)
|
|
|
|
# Recreate x_spec, with the same symbolic expressions, and the same scope
|
|
x_spec = jax.ShapeDtypeStruct(export.symbolic_shape("a",
|
|
scope=x_spec.shape[0].scope), np.int32)
|
|
_ = export.export(f)(x_spec) # This hits the tracing cache
|
|
self.assertEqual(1, f_tracing_count)
|
|
|
|
# Recreate x_spec, with the same symbolic expressisions with a fresh scope
|
|
x_spec = jax.ShapeDtypeStruct(export.symbolic_shape("a"), np.int32)
|
|
_ = export.export(f)(x_spec) # This is a cache miss
|
|
self.assertEqual(2, f_tracing_count)
|
|
|
|
# Now with constraints
|
|
x_spec = jax.ShapeDtypeStruct(export.symbolic_shape("a",
|
|
constraints=["a >= 2"]),
|
|
np.int32)
|
|
expected_a_bounds = (2, np.inf)
|
|
_ = export.export(f)(x_spec) # Cache miss
|
|
self.assertEqual(3, f_tracing_count)
|
|
|
|
def test_unused_args(self):
|
|
# Tests with functions that do not use their inputs.
|
|
|
|
# First arg unused, not polymorphic
|
|
check_shape_poly(self,
|
|
lambda x_unused, y: y * 2.0,
|
|
arg_descriptors=[RandArg((2, 3), _f32), RandArg((3,), _f32)],
|
|
polymorphic_shapes=[None, "b"])
|
|
|
|
# Some args unused, not polymorphic
|
|
check_shape_poly(self,
|
|
lambda x_unused, y, z_unused, w: jnp.concatenate([y, w]),
|
|
arg_descriptors=[RandArg((3,), _f32), RandArg((4,), _f32),
|
|
RandArg((5,), _f32), RandArg((6,), _f32)],
|
|
polymorphic_shapes=[None, "b1", None, "b2"])
|
|
|
|
# A polymorphic arg is not used, but the dimension var appears
|
|
# in a used arg also
|
|
check_shape_poly(self,
|
|
lambda x_unused, y: y * 2.0,
|
|
arg_descriptors=[RandArg((3,), _f32), RandArg((3,), _f32)],
|
|
polymorphic_shapes=["b", "b"])
|
|
|
|
# A polymorphic arg is not used, and the dimension var does not appear
|
|
# elsewhere.
|
|
check_shape_poly(self,
|
|
lambda x_unused, y: y * 2.0,
|
|
arg_descriptors=[RandArg((4,), _f32), RandArg((3,), _f32)],
|
|
polymorphic_shapes=["b1", "b2"])
|
|
|
|
# A polymorphic arg is not used, and the dimension var does appear
|
|
# elsewhere but not as a trivial term.
|
|
check_shape_poly(self,
|
|
lambda x_unused, y: y * 2.0,
|
|
arg_descriptors=[RandArg((3,), _f32), RandArg((9,), _f32)],
|
|
polymorphic_shapes=["b1", "b1 * b1"])
|
|
|
|
# It is not sufficient to just use the shape of an input; it is still unused
|
|
check_shape_poly(self,
|
|
lambda x_unused, y: y + x_unused.shape[0],
|
|
arg_descriptors=[RandArg((3,), _f32), RandArg((9,), _f32)],
|
|
polymorphic_shapes=["b1", "b2"])
|
|
|
|
def test_cond(self):
|
|
# Test the primitive under conditional
|
|
def f(x, y):
|
|
# x: f32[B, H], y : f32[H]
|
|
return lax.cond(
|
|
jnp.sum(x) > 0.,
|
|
lambda _: x + jnp.reshape(y, (1, y.shape[0])),
|
|
lambda _: jnp.zeros_like(x),
|
|
operand=None)
|
|
|
|
x = np.ones((2, 3))
|
|
y = np.ones((3,))
|
|
res_jax = f(x, y)
|
|
self.assertAllClose(
|
|
res_jax,
|
|
check_shape_poly(self, f, arg_descriptors=[x, y],
|
|
polymorphic_shapes=["(b, h)", "h"]))
|
|
|
|
def test_while(self):
|
|
def f(x):
|
|
# x: f32[B], iter: i32
|
|
return lax.while_loop(lambda x_iter: x_iter[1] < 5,
|
|
lambda x_iter: (x_iter[0] + jnp.arange(x_iter[0].shape[0], dtype=np.float32), x_iter[1] + 1),
|
|
(x, 0))
|
|
|
|
x = np.ones((3,), dtype=np.float32)
|
|
res_tf = check_shape_poly(self, f, arg_descriptors=[x],
|
|
polymorphic_shapes=["(b,)"])
|
|
self.assertAllClose(f(x), res_tf)
|
|
|
|
@jax.debug_key_reuse(False)
|
|
def test_prng(self):
|
|
# The PRNG implementation uses opaque types, test shape polymorphism
|
|
with config.enable_custom_prng(True):
|
|
|
|
def f_jax(x): # x: f32[b1, b2]
|
|
key = random.PRNGKey(123) # key: key<fry>[]
|
|
# Exercise key operations that have custom lowering rules
|
|
broadcast_keys = lax.broadcast_in_dim(key, x.shape, ()) # key<fry>[b1, b2]
|
|
gather_keys = lax.broadcast_in_dim(broadcast_keys[0], (1, x.shape[1]), (1,)) # : key[1, b2]
|
|
slice_keys1 = lax.slice(broadcast_keys, (0, 0), (1, x.shape[1]), (1, 1)) # key[1, b2]
|
|
slice_keys2 = lax.dynamic_slice(broadcast_keys, (0, 0), slice_sizes=(1, x.shape[1])) # key[1, b2]
|
|
upd1 = lax.dynamic_update_slice(slice_keys2, slice_keys1, start_indices=(0, 0)) # key[1, b2]
|
|
_ = lax.dynamic_update_slice(upd1, gather_keys, start_indices=(0, 0))
|
|
|
|
# We need to test the special case for vmap(while)
|
|
xs = broadcast_keys
|
|
counts = jnp.arange(broadcast_keys.shape[0], dtype=np.int32)
|
|
def f_vmap_jax(counts, xs): # counts: i32[b1], xs: key<fry>[b1, b2]
|
|
def inner(count, x): # count i32, x: key<fry>[b2]
|
|
return lax.fori_loop(0, count, lambda _, acc: acc, x)
|
|
return jax.vmap(inner)(counts, xs)
|
|
|
|
_ = f_vmap_jax(counts, xs)
|
|
return x
|
|
|
|
check_shape_poly(self, f_jax,
|
|
arg_descriptors=[RandArg((3, 4), _f32)],
|
|
polymorphic_shapes=["b1, b2"])
|
|
|
|
def test_dynamic_shapes(self):
|
|
# Test dim_as_value with dynamic shapes.
|
|
def f(x):
|
|
return jnp.sum(x, axis=0) * x.shape[0]
|
|
|
|
x = np.arange(3.)
|
|
self.assertAllClose(9.,
|
|
check_shape_poly(self, f,
|
|
arg_descriptors=[x],
|
|
polymorphic_shapes=["(b,)"]))
|
|
self.assertAllClose(
|
|
9.,
|
|
check_shape_poly(self, jax.jit(f),
|
|
arg_descriptors=[x], polymorphic_shapes=["(b,)"]))
|
|
|
|
res_primal, res_tangent = check_shape_poly(self,
|
|
lambda x, xt: jax.jvp(f, (x,), (xt,)),
|
|
arg_descriptors=[x, np.array([0.1, 0.2, 0.3])],
|
|
polymorphic_shapes=["b", "b"])
|
|
self.assertAllClose((9., 1.8), (res_primal, res_tangent))
|
|
|
|
self.assertAllClose(
|
|
np.array([3., 3., 3.]),
|
|
check_shape_poly(self, jax.grad(f),
|
|
arg_descriptors=[x],
|
|
polymorphic_shapes=["b"]))
|
|
|
|
xv = np.arange(24.).reshape((2, 3, 4))
|
|
res_vmap = jax.vmap(f, in_axes=1)(xv)
|
|
# Implement by iteration
|
|
res_iter = jnp.stack([f(xv[:, i, :]) for i in range(xv.shape[1])])
|
|
self.assertAllClose(res_iter, res_vmap)
|
|
|
|
res_vmap_tf = check_shape_poly(self, jax.vmap(f, in_axes=1),
|
|
arg_descriptors=[xv],
|
|
polymorphic_shapes=["b1, b2, ..."])
|
|
self.assertAllClose(res_iter, res_vmap_tf)
|
|
|
|
def test_with_hash_collision_vmap(self):
|
|
# Batching caches based on Jaxpr, and Jaxpr include _DimExpr. If we have
|
|
# a collision for the hashing of a _DimExpr, then Python will call the
|
|
# equality, which will raise InconclusiveDimensionOperation.
|
|
|
|
def f_jax(x):
|
|
return jnp.reshape(x, (2, -1,))
|
|
orig_hash = None
|
|
try:
|
|
# Override the hashing to create collisions
|
|
orig_hash = getattr(shape_poly._DimExpr, "__hash__")
|
|
def collision_hash(obj):
|
|
return hash(5)
|
|
|
|
setattr(shape_poly._DimExpr, "__hash__", collision_hash)
|
|
xs = [np.ones((3, 5, 6), dtype=np.float32)]
|
|
f_toconvert = jax.vmap(pjit.pjit(f_jax))
|
|
res_1 = check_shape_poly(self, f_toconvert, arg_descriptors=xs,
|
|
polymorphic_shapes=["..."])
|
|
res_2 = check_shape_poly(self, f_toconvert, arg_descriptors=xs,
|
|
polymorphic_shapes=["b1, b2, ..."])
|
|
self.assertAllClose(res_1, res_2)
|
|
finally:
|
|
setattr(shape_poly._DimExpr, "__hash__", orig_hash)
|
|
|
|
@jtu.parameterized_filterable(
|
|
kwargs=[
|
|
dict(testcase_name=op_name, op=op)
|
|
for op, op_name in [
|
|
(jnp.array, "array"),
|
|
(jnp.sin, "sin"),
|
|
(lambda x: x, "id"),
|
|
(core.dimension_as_value, "dimension_as_value"),
|
|
]])
|
|
def test_poly_unary_op(self, *, op=jnp.array):
|
|
def f_jax(x): # x: f32[b]
|
|
poly = 2 * x.shape[0]
|
|
return (op(poly), x) # Make sure we are using x
|
|
|
|
check_shape_poly(self,
|
|
f_jax,
|
|
arg_descriptors=[RandArg((3,), _f32)],
|
|
polymorphic_shapes=["b"])
|
|
|
|
@jtu.parameterized_filterable(
|
|
kwargs=[
|
|
dict(testcase_name=f"_{op.__name__}_other={other}:{type(other)}{'_other_jnp_array' if other_jnp_array else ''}{'_swap' if swap else ''}",
|
|
op=op, other=other,
|
|
other_jnp_array=other_jnp_array, swap=swap)
|
|
for op in [op.add, op.mul, op.sub,
|
|
op.mod, op.floordiv, op.truediv]
|
|
for other in [
|
|
2, np.int32(2), 2., np.float32(2),
|
|
np.array(2, dtype=np.int32), np.arange(1, 5, dtype=np.int32),
|
|
np.array(2., dtype=np.float32), np.arange(1., 7., dtype=np.float32)
|
|
]
|
|
for other_jnp_array in (
|
|
[True, False] if np.shape(other) == (7,) else [False]) # type: ignore
|
|
for swap in [False, True] # The poly is the left op by default
|
|
])
|
|
def test_poly_binary_op(self, *, op=op.add,
|
|
other=np.arange(2, dtype=np.int32),
|
|
other_jnp_array=False,
|
|
swap=True):
|
|
# Test arithmetic operations with poly and a variety of other operand types
|
|
def f_jax(x): # x: f32[b]
|
|
poly = 2 * x.shape[0] # This will allow divisions with 2
|
|
other_wrapped = jnp.array(other) if other_jnp_array else other
|
|
ops = (poly, other_wrapped) if not swap else (other_wrapped, poly)
|
|
res = op(*ops)
|
|
|
|
# If the other op is an integer then the result is a symbolic dim
|
|
try:
|
|
op.index(other)
|
|
other_isint = True
|
|
except Exception:
|
|
other_isint = False
|
|
|
|
if (hasattr(poly, "dimension_as_value") and
|
|
other_isint and
|
|
op.__name__ != "truediv"):
|
|
# If we running under jax2tf and "other" is an integer the result
|
|
# should be a symbolic dimension
|
|
self.assertTrue(isinstance(res, int) or hasattr(res, "dimension_as_value"))
|
|
|
|
if config.enable_x64.value:
|
|
# Outside jax2tf, x.shape[0] is a Python (64-bit) integer and for most
|
|
# operations here JAX is not involved at all because the other operand
|
|
# is a Python or NumPy constant. So the result will be 64-bits. But under
|
|
# jax2tf, x.shape[0] is rewritten to jnp.array(x.shape[0]) which when
|
|
# used with int32 or float32 values will produce 32-bit values.
|
|
return (lax.convert_element_type(res, np.float32), x)
|
|
return (res, x) # Make sure we are using x
|
|
|
|
check_shape_poly(self,
|
|
f_jax,
|
|
arg_descriptors=[RandArg((3,), np.int32)],
|
|
polymorphic_shapes=["b"])
|
|
|
|
|
|
def test_shape_as_array(self):
|
|
def f_jax(x):
|
|
# The entire x.shape is passed to jnp.array
|
|
return x + jnp.sum(jnp.array(x.shape)).astype(np.int32)
|
|
|
|
check_shape_poly(self,
|
|
f_jax,
|
|
arg_descriptors=[RandArg((3, 4), _i32)],
|
|
polymorphic_shapes=["b, _"])
|
|
|
|
def test_dim_as_value_weak_type(self):
|
|
def f_jax(x): # x: f32[b]
|
|
d0 = jnp.array(x.shape[0]) # in JAX should have weak_type=True
|
|
if isinstance(d0, core.Tracer):
|
|
self.assertTrue(d0.aval.weak_type)
|
|
|
|
# And an implicit conversion to array
|
|
d1 = x.shape[0] + jnp.array(4)
|
|
if isinstance(d1, core.Tracer):
|
|
self.assertTrue(d1.aval.weak_type)
|
|
return d0 + np.array(5., dtype=np.float32) + d1 + x[0]
|
|
|
|
with config.numpy_dtype_promotion("strict"):
|
|
# strict type promotion is sensitive to weak_types
|
|
check_shape_poly(self,
|
|
f_jax,
|
|
arg_descriptors=[RandArg((3,), _f32)],
|
|
polymorphic_shapes=["b"])
|
|
|
|
def test_vmap_while(self):
|
|
def cond_func(x): # x: f32[3]
|
|
return jnp.sum(x) >= 0.
|
|
def body_func(x): # x: f32[3]
|
|
return x - 1.
|
|
def f_jax(x):
|
|
return lax.while_loop(cond_func, body_func, x)
|
|
|
|
check_shape_poly(self,
|
|
jax.vmap(f_jax),
|
|
arg_descriptors=[RandArg((5, 3), _f32)],
|
|
polymorphic_shapes=["b, ..."])
|
|
|
|
def test_vmap_error(self):
|
|
# vmap is careful to give nice error messages when mapped axes have
|
|
# different sizes, but this can be foiled by InconsistentDimensionOperation
|
|
x = y = np.ones((3, 5), dtype=np.float32)
|
|
with self.assertRaisesRegex(ValueError,
|
|
"vmap got inconsistent sizes for array axes to be mapped"):
|
|
check_shape_poly(self, jax.vmap(lambda x, y: x + y),
|
|
arg_descriptors=[x, y],
|
|
polymorphic_shapes=["b, ...", None])
|
|
|
|
z = x
|
|
with self.assertRaisesRegex(ValueError,
|
|
"vmap got inconsistent sizes for array axes to be mapped"):
|
|
check_shape_poly(self, jax.vmap(lambda x, y, z: x + y + z),
|
|
arg_descriptors=[x, y, z],
|
|
polymorphic_shapes=["b, ...", "c, ...", None])
|
|
|
|
|
|
@jtu.parameterized_filterable(
|
|
kwargs=[
|
|
dict(slc=slc)
|
|
for slc in [
|
|
slice(None, None, None),
|
|
slice(2, 5),
|
|
]
|
|
])
|
|
def test_stateful(self, slc: slice):
|
|
w, = export.symbolic_shape("w", constraints=["w >= 3"])
|
|
def f(x_ref):
|
|
ones = jnp.ones_like(x_ref)[slc]
|
|
ref_primitives.ref_addupdate(x_ref, slc, ones)
|
|
x1 = ref_primitives.ref_get(x_ref, slc)
|
|
x2 = x1 + ones
|
|
ref_primitives.ref_set(x_ref, slc, x2)
|
|
|
|
exp = export.export(jax.jit(discharge.run_state(f)))(
|
|
jax.ShapeDtypeStruct((w,), dtype=_f32))
|
|
x = np.ones((32,), dtype=_f32)
|
|
expected = np.copy(x)
|
|
expected[slc] = 3.
|
|
self.assertAllClose(exp.call(x), expected)
|
|
|
|
|
|
# List containing either harnesses, or lists of harnesses
|
|
_POLY_SHAPE_TEST_HARNESSES = [
|
|
PolyHarness("add", "",
|
|
lambda x, y: jnp.add(jnp.expand_dims(x, axis=0), y),
|
|
arg_descriptors=[RandArg((3, 4), _f32), RandArg((2, 3, 4), _f32)],
|
|
polymorphic_shapes=["b, _", "_, b, _"]),
|
|
PolyHarness("add_transpose", "",
|
|
jax.grad(lambda x: jnp.sum(
|
|
jnp.expand_dims(jnp.sum(x, axis=0, keepdims=False), axis=0)
|
|
+ jnp.sin(x))),
|
|
arg_descriptors=[RandArg((3, 4), _f32)],
|
|
polymorphic_shapes=["b, ..."]),
|
|
[ # approx_max_k
|
|
# x: f32[b, {n}, 32] with n being either 8 or the symbol "n"
|
|
# we reduce on dim=1, with size n
|
|
# k is either the constant 4 or the symbol "k"
|
|
PolyHarness("approx_max_k", f"n_{n}_k_{k}_agg={agg}",
|
|
lambda x, x_k, agg: lax.approx_max_k(
|
|
x, k=x_k.shape[0], reduction_dimension=1,
|
|
aggregate_to_topk=agg),
|
|
arg_descriptors=[RandArg((3, 8, 32), _f32),
|
|
RandArg((4,), _f32),
|
|
StaticArg(agg)],
|
|
polymorphic_shapes=[f"b, {n}, 32", f"{k},"],
|
|
# k must be at most the reduction dimension size
|
|
symbolic_constraints=[f"{k} <= {n}"],
|
|
expect_error=(
|
|
(NotImplementedError, "aggregate_to_topk=False") if (
|
|
not agg and (isinstance(k, str) or
|
|
isinstance(n, str))) else
|
|
None
|
|
))
|
|
for n in [8, "n"]
|
|
for k in [4, "k"]
|
|
for agg in [True, False]
|
|
],
|
|
[ # arange
|
|
PolyHarness("arange", name,
|
|
f_jax,
|
|
arg_descriptors=[RandArg((6,), np.float32)],
|
|
polymorphic_shapes=["b"])
|
|
for name, f_jax in [
|
|
# Positive step
|
|
("b", lambda x: jnp.arange(x.shape[0], None, None, None)),
|
|
("0_b+1", lambda x: jnp.arange(0, x.shape[0] + 1, None, None)),
|
|
("0_5b_2", lambda x: jnp.arange(0, 5 * x.shape[0], 2, None)),
|
|
("0_5b+1_2", lambda x: jnp.arange(0, 5 * x.shape[0] + 1, 2, None)),
|
|
("b_5b+2_2", lambda x: jnp.arange(x.shape[0], 5 * x.shape[0] + 2, 2, None)),
|
|
("0_b-1_2", lambda x: jnp.arange(0, x.shape[0] - 1, 2, None)),
|
|
("0_b-2_2", lambda x: jnp.arange(0, x.shape[0] - 2, 2, None)),
|
|
("0_-b_2", lambda x: jnp.arange(0, -x.shape[0], 2, None)),
|
|
("0_1-b_2", lambda x: jnp.arange(0, 1 - x.shape[0], 2, None)),
|
|
("0_b-3_2", lambda x: jnp.arange(0, x.shape[0] - 3, 2, None)),
|
|
# Cannot tell if size >= 0
|
|
# Negative step
|
|
("b_0_-1", lambda x: jnp.arange(x.shape[0], 0, -1, None)),
|
|
("b_1_-2", lambda x: jnp.arange(x.shape[0], 1, -2, None)),
|
|
("b_-1_-1", lambda x: jnp.arange(x.shape[0], -1, -1, None)),
|
|
("5b+1_0_-2", lambda x: jnp.arange(5 * x.shape[0] + 1, 0, -2, None)),
|
|
("5b+2_0_-2", lambda x: jnp.arange(5 * x.shape[0] + 2, 0, -2, None)),
|
|
("b-3_0_-2", lambda x: jnp.arange(x.shape[0] - 3, 0, -2, None)),
|
|
# Cannot tell if size >= 0
|
|
# Symbolic step
|
|
("0_10_b", lambda x: jnp.arange(0, 10, x.shape[0])),
|
|
("0_0_b", lambda x: jnp.arange(0, 0, x.shape[0])),
|
|
("10_0_-b", lambda x: jnp.arange(10, 0, -x.shape[0])),
|
|
("b_1_-b", lambda x: jnp.arange(x.shape[0], 1, -x.shape[0])),
|
|
# Float return type
|
|
("0_b_1_f32", lambda x: jnp.arange(0, x.shape[0], 1, np.float32))
|
|
]
|
|
],
|
|
[ # arange errors
|
|
PolyHarness("arange_error", name,
|
|
# x: i32[b]
|
|
f_jax,
|
|
arg_descriptors=[RandArg((3,), dtype=np.int32)],
|
|
polymorphic_shapes=["b"],
|
|
expect_error=(expect_error, expect_msg))
|
|
for name, f_jax, expect_error, expect_msg in [
|
|
# make_args invoked with op.shape[0]: start, stop, step
|
|
("float_start", lambda x: x[0] + jnp.arange(0., x.shape[0], None),
|
|
ValueError, "must be either dimension expressions or integers"),
|
|
("float_step", lambda x: x[0] + jnp.arange(0, x.shape[0], 0.5),
|
|
ValueError, "must be either dimension expressions or integers"),
|
|
("step_0", lambda x: x[0] + jnp.arange(0, x.shape[0], 0),
|
|
ValueError, "has step == 0"),
|
|
("inconclusive_step_sign", lambda x: x[0] + jnp.arange(0, x.shape[0],
|
|
x.shape[0] - 2),
|
|
core.InconclusiveDimensionOperation,
|
|
"must be resolved statically if it is > 0 or < 0"),
|
|
]
|
|
],
|
|
[ # indexing
|
|
# operand is non-poly, index is poly
|
|
PolyHarness("indexing", "op=static_idx=poly",
|
|
lambda a, i: a[i],
|
|
arg_descriptors=[RandArg((3, 4), _f32),
|
|
np.array([2, 2], np.int32)],
|
|
polymorphic_shapes=[None, "b0, ..."]),
|
|
# operand is poly, index is integer
|
|
PolyHarness("indexing", "op=poly_idx=const",
|
|
lambda a: a[1],
|
|
arg_descriptors=[RandArg((3, 4), _f32)],
|
|
polymorphic_shapes=["b, ..."]),
|
|
# Both the operand and the index are poly
|
|
PolyHarness("indexing", "op=poly_idx=poly",
|
|
lambda a, i: a[i],
|
|
arg_descriptors=[RandArg((3, 4), _f32),
|
|
np.array([1, 2, 0], np.int32)],
|
|
polymorphic_shapes=["b, ...", "b, ..."]),
|
|
],
|
|
[ # indexing with slices
|
|
PolyHarness("indexing", f"start_{start_name}_stop_{stop_name}_step_{step_name}",
|
|
partial(lambda start, stop, step, x: x[slice(start(x.shape[1]),
|
|
stop(x.shape[1]),
|
|
step(x.shape[1]))],
|
|
start, stop, step),
|
|
arg_descriptors=[RandArg((16, 8), np.float32)],
|
|
polymorphic_shapes=["c, b"])
|
|
# start, stop, step are functions that take the argument "b"
|
|
# "b" actual value is 8 and "c" is 16
|
|
for start_name, start in [
|
|
("None", lambda b: None),
|
|
("0", lambda b: 0),
|
|
("2", lambda b: 2),
|
|
("b", lambda b: b),
|
|
("2b2", lambda b: 2*b + 2),
|
|
("-2", lambda b: -2),
|
|
("-b", lambda b: -b),
|
|
("-2b2", lambda b: -2*b - 2),
|
|
]
|
|
for stop_name, stop in [
|
|
("None", lambda b: None),
|
|
("0", lambda b: 0),
|
|
("b", lambda b: b),
|
|
("2b2", lambda b: 2*b + 2),
|
|
("4", lambda b: 4),
|
|
("-4", lambda b: -4),
|
|
("-b", lambda b: -b),
|
|
("-2b2", lambda b: -2*b - 2),
|
|
]
|
|
for step_name, step in [
|
|
("None", lambda b: None),
|
|
("1", lambda b: 1),
|
|
("2", lambda b: 2),
|
|
("b", lambda b: b),
|
|
("-1", lambda b: -1),
|
|
("-2", lambda b: -2),
|
|
("-b", lambda b: -b),
|
|
]
|
|
],
|
|
# Reduce the poly dimension
|
|
PolyHarness("argmax", "0",
|
|
lambda op: lax.argmax(op, axis=0, index_dtype=np.int32),
|
|
arg_descriptors=[RandArg((3, 4, 5), _f32)],
|
|
polymorphic_shapes=["b, ..."]),
|
|
# Reduce the non-poly dimension
|
|
PolyHarness("argmax", "1",
|
|
lambda op: lax.argmax(op, axis=1, index_dtype=np.int32),
|
|
arg_descriptors=[RandArg((3, 4, 5), _f32)],
|
|
polymorphic_shapes=["b, ..."]),
|
|
PolyHarness("jnp.argsort", "",
|
|
jnp.argsort,
|
|
arg_descriptors=[RandArg((3, 4, 5), _f32)],
|
|
polymorphic_shapes=["b, ..."]),
|
|
[
|
|
PolyHarness("average",
|
|
f"{axis=}_weights=None",
|
|
lambda x, axis: jnp.average(x, axis=axis, returned=False, weights=None),
|
|
arg_descriptors=[RandArg((7, 8, 4), _f32), StaticArg(axis)],
|
|
polymorphic_shapes=["b, ..."])
|
|
for axis in [None, 0, 1]
|
|
],
|
|
[
|
|
PolyHarness("average",
|
|
f"{axis=}_weights=Some",
|
|
lambda x, weights, axis: jnp.average(x, axis=axis, returned=False, weights=weights),
|
|
arg_descriptors=[RandArg((7, 8, 4), _f32), RandArg((7, 8, 4), _f32), StaticArg(axis)],
|
|
polymorphic_shapes=["b, ...", "b, ..."])
|
|
for axis in [None, 0, 1]
|
|
],
|
|
PolyHarness("jnp.bincount", "length=constant",
|
|
lambda x: jnp.bincount(x % 2, length=4),
|
|
arg_descriptors=[RandArg((12,), np.int32)],
|
|
polymorphic_shapes=["b, ..."]),
|
|
PolyHarness("jnp.bincount", "length=poly",
|
|
lambda x: jnp.bincount(x % 4, length=x.shape[0]),
|
|
arg_descriptors=[RandArg((12,), np.int32)],
|
|
polymorphic_shapes=["b, ..."]),
|
|
PolyHarness("broadcast_to", "",
|
|
lambda x: jnp.broadcast_to(x, [x.shape[0], x.shape[0], 4]),
|
|
arg_descriptors=[RandArg((3, 4), _f32)],
|
|
polymorphic_shapes=["b, ..."]),
|
|
PolyHarness("broadcast_in_dim", "0",
|
|
lambda x: lax.broadcast_in_dim(x, [x.shape[0], 4, 5, 6],
|
|
broadcast_dimensions=(0, 2, 3)),
|
|
arg_descriptors=[RandArg((3, 1, 6), _f32)],
|
|
polymorphic_shapes=["b, ..."]),
|
|
PolyHarness("broadcast_in_dim", "poly",
|
|
lambda x: lax.broadcast_in_dim(x, [x.shape[0], x.shape[0] + x.shape[0], 4],
|
|
broadcast_dimensions=(0, 1, 2)),
|
|
arg_descriptors=[RandArg((3, 1, 4), _f32)],
|
|
polymorphic_shapes=["b, ..."]),
|
|
PolyHarness("broadcast_in_dim", "poly2",
|
|
lambda x: lax.broadcast_in_dim(x, [x.shape[0], 5, 6, x.shape[2], 4],
|
|
broadcast_dimensions=(0, 2, 3)),
|
|
arg_descriptors=[RandArg((3, 1, 4), _f32)],
|
|
polymorphic_shapes=["b1, _, b2"]),
|
|
PolyHarness("broadcast_in_dim", "transpose",
|
|
jax.grad(lambda x: jnp.sum(
|
|
lax.broadcast_in_dim(jnp.sin(x), [2, x.shape[0], 5, x.shape[2], 4],
|
|
broadcast_dimensions=(1, 2, 3)))),
|
|
arg_descriptors=[RandArg((3, 1, 4), _f32)],
|
|
polymorphic_shapes=["b1, _, b2"]),
|
|
PolyHarness("clamp", "",
|
|
lax.clamp,
|
|
arg_descriptors=[RandArg((3, 4, 5), _f32), RandArg((3, 4, 5), _f32),
|
|
RandArg((3, 4, 5), _f32)],
|
|
polymorphic_shapes=["b, ...", "b, ...", "b, ..."]),
|
|
PolyHarness("collapse", "",
|
|
lambda x: lax.collapse(x, 1, 4),
|
|
arg_descriptors=[RandArg((3, 4, 5, 6, 7), _f32)],
|
|
polymorphic_shapes=["b0, b1, _, b3, ..."]),
|
|
PolyHarness("concatenate", "",
|
|
lambda x: jnp.concatenate([x, x], axis=0),
|
|
arg_descriptors=[RandArg((3, 4, 5), _f32)],
|
|
polymorphic_shapes=["b0, b1, _"]),
|
|
PolyHarness("concatenate", "grad",
|
|
jax.grad(lambda x: jnp.sum(jnp.concatenate([x, jnp.sin(x)], axis=0))),
|
|
arg_descriptors=[RandArg((3, 4, 5), _f32)],
|
|
polymorphic_shapes=["b0, b1, _"]),
|
|
|
|
PolyHarness("conv_general_dilated", "1d_stride=1",
|
|
lambda lhs, rhs: lax.conv_general_dilated(
|
|
lhs, rhs,
|
|
window_strides=(1,),
|
|
padding="SAME",
|
|
rhs_dilation=None,
|
|
dimension_numbers=lax.ConvDimensionNumbers(lhs_spec=(0, 2, 1),
|
|
rhs_spec=(2, 1, 0),
|
|
out_spec=(0, 2, 1))),
|
|
arg_descriptors=[RandArg((1, 12, 16), _f32), RandArg((4, 16, 16), _f32)],
|
|
polymorphic_shapes=["_, b, _", None]),
|
|
# The same example from above, but with stride=2.
|
|
PolyHarness("conv_general_dilated", "1d_stride=2_even",
|
|
lambda lhs, rhs: lax.conv_general_dilated(
|
|
lhs, rhs,
|
|
window_strides=(2,),
|
|
padding="SAME",
|
|
rhs_dilation=None,
|
|
dimension_numbers=lax.ConvDimensionNumbers(lhs_spec=(0, 2, 1),
|
|
rhs_spec=(2, 1, 0),
|
|
out_spec=(0, 2, 1))),
|
|
arg_descriptors=[RandArg((1, 12, 16), _f32), RandArg((4, 16, 16), _f32)],
|
|
polymorphic_shapes=["_, b, _", None]),
|
|
# The same example from above, but with stride=2 and odd input size.
|
|
PolyHarness("conv_general_dilated", "1d_stride=2_odd",
|
|
lambda lhs, rhs: lax.conv_general_dilated(
|
|
lhs, rhs,
|
|
window_strides=(2,),
|
|
padding="SAME",
|
|
rhs_dilation=None,
|
|
dimension_numbers=lax.ConvDimensionNumbers(lhs_spec=(0, 2, 1),
|
|
rhs_spec=(2, 1, 0),
|
|
out_spec=(0, 2, 1))),
|
|
arg_descriptors=[RandArg((1, 13, 16), _f32), RandArg((4, 16, 16), _f32)],
|
|
polymorphic_shapes=["_, b, _", None]),
|
|
PolyHarness("conv_general_dilated", "1d_stride=2_zero_output",
|
|
lambda lhs, rhs: lax.conv_general_dilated(
|
|
lhs, rhs,
|
|
window_strides=(2,),
|
|
padding="VALID",
|
|
rhs_dilation=None,
|
|
dimension_numbers=lax.ConvDimensionNumbers(lhs_spec=(0, 2, 1),
|
|
rhs_spec=(2, 1, 0),
|
|
out_spec=(0, 2, 1))
|
|
).shape[1], # should be 0 in JAX native
|
|
arg_descriptors=[RandArg((1, 4, 16), _f32),
|
|
RandArg((8, 16, 16), _f32)],
|
|
polymorphic_shapes=["_, b, _",
|
|
None]),
|
|
# Issue #11402
|
|
PolyHarness("conv_general_dilated", "1d_2",
|
|
lambda lhs, rhs: lax.conv_transpose(lhs, rhs,
|
|
strides=(2,),
|
|
padding="SAME",
|
|
rhs_dilation=None,
|
|
transpose_kernel=False),
|
|
arg_descriptors=[RandArg((5, 12, 16), _f32), RandArg((4, 16, 16), _f32)],
|
|
polymorphic_shapes=["b, _, _", None],
|
|
tol=5e-5),
|
|
# Issue #11402
|
|
PolyHarness("conv_general_dilated", "1d_3",
|
|
lambda lhs, rhs: lax.conv_transpose(lhs, rhs,
|
|
strides=(2,),
|
|
padding="SAME",
|
|
rhs_dilation=None,
|
|
transpose_kernel=False),
|
|
arg_descriptors=[RandArg((5, 12, 16), _f32), RandArg((4, 16, 16), _f32)],
|
|
polymorphic_shapes=["_, b, _", None],
|
|
tol=5e-5),
|
|
PolyHarness("conv_general_dilated", "",
|
|
lambda lhs, rhs: lax.conv_general_dilated(
|
|
lhs, rhs,
|
|
window_strides=(2, 3),
|
|
padding=((0, 0), (0, 0)),
|
|
lhs_dilation=(1, 1),
|
|
rhs_dilation=(1, 2),
|
|
dimension_numbers=("NCHW", "OIHW", "NCHW"),
|
|
feature_group_count=1,
|
|
batch_group_count=1,
|
|
precision=None),
|
|
arg_descriptors=[RandArg((7, 3, 9, 10), _f32), RandArg((3, 3, 4, 5), _f32)],
|
|
polymorphic_shapes=["b, ...", None]),
|
|
[
|
|
[
|
|
PolyHarness(cum_name, "reduce_axis_poly",
|
|
lambda x: cum_func(x, axis=0),
|
|
arg_descriptors=[RandArg((3, 5), _f32)],
|
|
polymorphic_shapes=["b, ..."]),
|
|
PolyHarness(cum_name, "reduce_axis_static",
|
|
lambda x: cum_func(x, axis=1),
|
|
arg_descriptors=[RandArg((3, 5), _f32)],
|
|
polymorphic_shapes=["b, ..."])
|
|
]
|
|
for cum_name, cum_func in [
|
|
("cumlogsumexp", lax_control_flow.cumlogsumexp),
|
|
("cummax", lax_control_flow.cummax),
|
|
("cummin", lax_control_flow.cummin),
|
|
("cumsum", lax_control_flow.cumsum),
|
|
("cumprod", lax_control_flow.cumprod)
|
|
]
|
|
],
|
|
PolyHarness("delta", "0",
|
|
lambda x: lax_internal._delta(_f32, x.shape, axes=(0, 1)) + x,
|
|
arg_descriptors=[RandArg((3, 1), _f32)],
|
|
polymorphic_shapes=["b, ..."]),
|
|
PolyHarness("dot_general", "",
|
|
lambda lhs, rhs: lax.dot_general(lhs, rhs,
|
|
dimension_numbers=(((2,), (1,)), ((0,), (0,)))),
|
|
arg_descriptors=[RandArg((3, 4, 4), _f32), RandArg((3, 4), _f32)],
|
|
polymorphic_shapes=["b, ...", "b, ..."]),
|
|
PolyHarness("dynamic_slice", "idx=tuple_int",
|
|
# x:shape: (b, 4)
|
|
lambda x: lax.dynamic_slice(x, (0, 1), (x.shape[0], 2)),
|
|
arg_descriptors=[RandArg((3, 4), _f32)],
|
|
polymorphic_shapes=["b, ..."]),
|
|
PolyHarness("dynamic_slice", "idx=tuple_arg",
|
|
# x:shape: (b, 4)
|
|
lambda x, i0: lax.dynamic_slice(x, (i0, np.int32(1)), (x.shape[0], 2)),
|
|
arg_descriptors=[RandArg((3, 4), _f32), np.array(-2, dtype=np.int32)],
|
|
polymorphic_shapes=["b, ...", None]),
|
|
PolyHarness("dynamic_slice", "idx=array",
|
|
# x:shape: (b, 4)
|
|
lambda x, idx: lax.dynamic_slice(x, idx, (x.shape[0], 2)),
|
|
arg_descriptors=[RandArg((3, 4), _f32), np.array([-2, -1], dtype=np.int32)],
|
|
polymorphic_shapes=["b, ...", None]),
|
|
PolyHarness("dynamic_slice", "idx=tuple_int_start_oob_large",
|
|
# x:shape: (b, 4)
|
|
lambda x: lax.dynamic_slice(x, (1, 1), (x.shape[0], 2)),
|
|
arg_descriptors=[RandArg((3, 4), _f32)],
|
|
polymorphic_shapes=["b, ..."]),
|
|
PolyHarness("dynamic_slice", "idx=tuple_int_start_oob_small",
|
|
# x:shape: (b, 4)
|
|
lambda x: lax.dynamic_slice(x, (-1, 1), (x.shape[0] - 1, 2)),
|
|
arg_descriptors=[RandArg((3, 4), _f32)],
|
|
polymorphic_shapes=["b, ..."]),
|
|
PolyHarness("dynamic_slice_in_dim", "idx=0",
|
|
# x:shape: (b, 4)
|
|
lambda x: lax.dynamic_slice_in_dim(x, 0, x.shape[0], axis=0),
|
|
arg_descriptors=[RandArg((3, 4), _f32)],
|
|
polymorphic_shapes=["b, ..."]),
|
|
PolyHarness("dynamic_update_slice", "idx=tuple_int",
|
|
# x:shape: (b, 4)
|
|
lambda x: lax.dynamic_update_slice(x, x, (0, 0)),
|
|
arg_descriptors=[RandArg((3, 4), _f32)],
|
|
polymorphic_shapes=["b, ..."]),
|
|
PolyHarness("dynamic_update_slice", "idx=tuple_arg",
|
|
# x:shape: (b, 4)
|
|
lambda x, i0: lax.dynamic_update_slice(x, x, (i0, np.int32(0))),
|
|
arg_descriptors=[RandArg((3, 4), _f32), np.array(-2, dtype=np.int32)],
|
|
polymorphic_shapes=["b, ...", None]),
|
|
PolyHarness("dynamic_update_slice", "idx=array",
|
|
# x:shape: (b, 4)
|
|
lambda x, idx: lax.dynamic_update_slice(x, x, idx),
|
|
arg_descriptors=[RandArg((3, 4), _f32), np.array([-2, -1], dtype=np.int32)],
|
|
polymorphic_shapes=["b, _", None]),
|
|
[
|
|
PolyHarness("eig", f"shape={jtu.format_shape_dtype_string((3, 5, 5), dtype)}_poly={poly}_{left=}_{right=}",
|
|
lambda x, left, right: lax.linalg.eig(x, compute_left_eigenvectors=left, compute_right_eigenvectors=right),
|
|
arg_descriptors=[RandArg((3, 5, 5), dtype),
|
|
StaticArg(left), StaticArg(right)],
|
|
polymorphic_shapes=[poly])
|
|
for dtype in {np.float32, np.float64, np.complex64, np.complex128} & jtu.supported_dtypes()
|
|
for poly in ["b, ...", "b, w, w"]
|
|
for left in ([True, False] if dtype == np.float32 else [True])
|
|
for right in ([True, False] if dtype == np.float32 else [False])
|
|
],
|
|
PolyHarness("einsum", "0",
|
|
lambda x: jnp.einsum("...i->...", x),
|
|
arg_descriptors=[RandArg((3, 4), _f32)],
|
|
polymorphic_shapes=["b, ..."]),
|
|
PolyHarness("einsum", "0_alt",
|
|
lambda x: jnp.einsum(x, (..., 1), [...]),
|
|
arg_descriptors=[RandArg((3, 4), _f32)],
|
|
polymorphic_shapes=["b, ..."]),
|
|
PolyHarness("einsum", "1",
|
|
lambda x, y: jnp.einsum("...ij,...jk->...ik", x, y),
|
|
arg_descriptors=[RandArg((3, 4, 5), _f32), RandArg((3, 5, 6), _f32)],
|
|
polymorphic_shapes=["b, ...", "b, ..."]),
|
|
PolyHarness("einsum", "1_alt",
|
|
lambda x, y: jnp.einsum(x, [..., 0, 1], y, (..., 1, 2), [..., 0, 2]),
|
|
arg_descriptors=[RandArg((3, 4, 5), _f32), RandArg((3, 5, 6), _f32)],
|
|
polymorphic_shapes=["b, ...", "b, ..."]),
|
|
PolyHarness("einsum", "2",
|
|
lambda x, y: jnp.einsum("...ij,jk->...ik", x, y),
|
|
arg_descriptors=[RandArg((3, 4, 5), _f32), RandArg((5, 6), _f32)],
|
|
polymorphic_shapes=["b, ...", None]),
|
|
PolyHarness("einsum", "2_alt",
|
|
lambda x, y: jnp.einsum(x, [..., 0, 1], y, [1, 2], [..., 0, 2]),
|
|
arg_descriptors=[RandArg((3, 4, 5), _f32), RandArg((5, 6), _f32)],
|
|
polymorphic_shapes=["b, ...", None]),
|
|
PolyHarness("einsum", "3",
|
|
# Reduced dimension is polymorphic
|
|
lambda x, y: jnp.einsum("ij,jk->ik", x, y),
|
|
arg_descriptors=[RandArg((3, 4), _f32), RandArg((4, 5), _f32)],
|
|
polymorphic_shapes=["_, b", "b, ..."]),
|
|
PolyHarness("einsum", "3_alt",
|
|
# Reduced dimension is polymorphic
|
|
lambda x, y: jnp.einsum(x, [0, 1], y, [1, 2], [0, 2]),
|
|
arg_descriptors=[RandArg((3, 4), _f32), RandArg((4, 5), _f32)],
|
|
polymorphic_shapes=["_, b", "b, ..."]),
|
|
PolyHarness("einsum", "4",
|
|
# Reduced dimension is polymorphic, and is 2*b
|
|
lambda x, y: jnp.einsum("ij,jk->ik",
|
|
jnp.concatenate([x, x], axis=1),
|
|
jnp.concatenate([y, y], axis=0)),
|
|
arg_descriptors=[RandArg((3, 4), _f32), RandArg((4, 5), _f32)],
|
|
polymorphic_shapes=["_, b", "b, ..."]),
|
|
PolyHarness("einsum", "4_alt",
|
|
# Reduced dimension is polymorphic, and is 2*b
|
|
lambda x, y: jnp.einsum(jnp.concatenate([x, x], axis=1), [0, 1],
|
|
jnp.concatenate([y, y], axis=0), [1, 2],
|
|
[0, 2]),
|
|
arg_descriptors=[RandArg((3, 4), _f32), RandArg((4, 5), _f32)],
|
|
polymorphic_shapes=["_, b", "b, ..."]),
|
|
PolyHarness("einsum", "multiple_contractions",
|
|
lambda x, y, z: jnp.einsum("ab,bc,cd->ad", x, y, z),
|
|
arg_descriptors=[RandArg((3, 2), _f32), RandArg((2, 3), _f32), RandArg((3, 4), _f32)],
|
|
polymorphic_shapes=["b, ...", None, None]),
|
|
PolyHarness("einsum", "incompatible_contractions_error",
|
|
lambda x, y: jnp.einsum("ab,cb->ac", x, y),
|
|
arg_descriptors=[RandArg((2, 3), _f32), RandArg((2, 3), _f32)],
|
|
polymorphic_shapes=["(2, b0)", "(2, b1)"],
|
|
expect_error=(AssertionError,
|
|
"Incompatible reduction dimensions")),
|
|
PolyHarness("eye", "N=poly_M=None",
|
|
lambda x: jnp.eye(x.shape[0], dtype=_f32) + x,
|
|
arg_descriptors=[RandArg((3, 1), _f32)],
|
|
polymorphic_shapes=["b, ..."]),
|
|
PolyHarness("eye", "N=poly_M=poly",
|
|
lambda x: jnp.eye(x.shape[0], M=x.shape[0] + 2, dtype=_f32) + x,
|
|
arg_descriptors=[RandArg((3, 1), _f32)],
|
|
polymorphic_shapes=["b, ..."]),
|
|
[
|
|
PolyHarness("fft", f"{fft_type=}_{nr_fft_lengths=}",
|
|
lambda x, fft_type, nr_fft_lengths: lax.fft_p.bind(
|
|
x, fft_type=fft_type,
|
|
fft_lengths=tuple(
|
|
x.shape[-nr_fft_lengths:] if fft_type != lax.FftType.IRFFT else
|
|
[(x.shape[-1] - 1) * 2])),
|
|
arg_descriptors=[
|
|
RandArg((3, 4, 5, 6),
|
|
np.float32 if fft_type == lax.FftType.RFFT else np.complex64),
|
|
StaticArg(fft_type),
|
|
StaticArg(nr_fft_lengths)],
|
|
# All axes but the last one are dynamic. This means that the test
|
|
# with nr_fft_lengths==1 will not have dynamic fft_lengths.
|
|
polymorphic_shapes=["b0, b1, b2, ..."],
|
|
tol=1e-4)
|
|
|
|
for fft_type in (lax.FftType.FFT, lax.FftType.IFFT,
|
|
lax.FftType.RFFT, lax.FftType.IRFFT)
|
|
for nr_fft_lengths in (1, 2)
|
|
],
|
|
PolyHarness("full", "",
|
|
lambda x: lax.full((x.shape[0], 2), 3.) + x,
|
|
arg_descriptors=[RandArg((3, 1), _f32)],
|
|
polymorphic_shapes=["b, ..."]),
|
|
PolyHarness("gather", "1d",
|
|
lambda operand, start_indices, x: lax.gather(
|
|
operand,
|
|
start_indices,
|
|
dimension_numbers=lax.GatherDimensionNumbers(
|
|
offset_dims=(1,),
|
|
collapsed_slice_dims=(),
|
|
start_index_map=(0,)),
|
|
slice_sizes=x.shape,
|
|
mode="promise_in_bounds"),
|
|
arg_descriptors=[
|
|
RandArg((10,), np.float32),
|
|
np.random.randint(0, high=10, size=(3, 1),
|
|
dtype=np.int32),
|
|
np.zeros((10,), dtype=jnp.int32),
|
|
],
|
|
polymorphic_shapes=["(t, )", "(3, 1)", "(t)"]),
|
|
PolyHarness("image_resize", "linear_0",
|
|
lambda x: jax.image.resize(x, (x.shape[0], 2 * x.shape[1], 2 * x.shape[2], x.shape[3]),
|
|
method="linear"),
|
|
arg_descriptors=[RandArg((3, 16, 32, 3), _f32)],
|
|
polymorphic_shapes=["_, b1, b2, ..."]),
|
|
PolyHarness("image_resize", "linear_to_fixed_dim",
|
|
lambda x: jax.image.resize(x, (x.shape[0], 64, 64, x.shape[3]),
|
|
method="linear"),
|
|
arg_descriptors=[RandArg((3, 16, 32, 3), _f32)],
|
|
polymorphic_shapes=["_, b1, b2, ..."]),
|
|
PolyHarness("image_resize", "nearest_0",
|
|
lambda x: jax.image.resize(x, (x.shape[0], 2 * x.shape[1], 2 * x.shape[2], x.shape[3]),
|
|
method="nearest"),
|
|
arg_descriptors=[RandArg((3, 5, 7, 3), _f32)],
|
|
polymorphic_shapes=["_, b1, b2, ..."]),
|
|
PolyHarness("index_in_dim", "0",
|
|
lambda x: lax.index_in_dim(x, -1, axis=0, keepdims=False),
|
|
arg_descriptors=[RandArg((3, 4), _f32)],
|
|
polymorphic_shapes=["b, ..."]),
|
|
PolyHarness("index_in_dim", "idx=neg",
|
|
lambda x: lax.index_in_dim(x, -1, axis=0, keepdims=False),
|
|
arg_descriptors=[RandArg((3, 4), _f32)],
|
|
polymorphic_shapes=["b, ..."]),
|
|
PolyHarness("index_in_dim", "idx=last",
|
|
lambda x: lax.index_in_dim(x, x.shape[0] - 1, axis=0, keepdims=False),
|
|
arg_descriptors=[RandArg((3, 4), _f32)],
|
|
polymorphic_shapes=["b, ..."]),
|
|
PolyHarness("jnp.insert", "insert=constant",
|
|
lambda x: jnp.insert(x, jnp.arange(3, dtype=_i32), np.array([3, 4, 5], dtype=_i32)),
|
|
arg_descriptors=[RandArg((12,), _i32)],
|
|
polymorphic_shapes=["b, ..."],
|
|
expect_error=expect_error_associative_scan),
|
|
PolyHarness("jnp.insert", "insert=poly",
|
|
lambda x: jnp.insert(x, jnp.arange(x.shape[0], dtype=_i32), x, axis=0),
|
|
arg_descriptors=[RandArg((12, 3), _i32)],
|
|
polymorphic_shapes=["b0, b1, ..."],
|
|
expect_error=expect_error_associative_scan),
|
|
PolyHarness("iota", "",
|
|
lambda x: x + lax.iota(_f32, x.shape[0]),
|
|
arg_descriptors=[RandArg((3,), _f32)],
|
|
polymorphic_shapes=["b, ..."]),
|
|
PolyHarness("linspace", "",
|
|
lambda x: jnp.linspace(0, x.shape[0], 4),
|
|
arg_descriptors=[RandArg((30,), _f32)],
|
|
polymorphic_shapes=["b, ..."]),
|
|
PolyHarness("linspace", "num_poly",
|
|
lambda x: jnp.linspace(0, 100, x.shape[0]),
|
|
arg_descriptors=[RandArg((30,), _f32)],
|
|
polymorphic_shapes=["b, ..."],
|
|
symbolic_constraints=["b >= 2"]),
|
|
PolyHarness("matmul", "0",
|
|
jnp.matmul,
|
|
arg_descriptors=[RandArg((7, 8, 4), _f32), RandArg((7, 4, 5), _f32)],
|
|
polymorphic_shapes=["b, ...", "b, ..."],
|
|
tol=1e-5),
|
|
PolyHarness("matmul", "1",
|
|
jnp.matmul,
|
|
arg_descriptors=[RandArg((7, 8, 4), _f32), RandArg((4, 5), _f32)],
|
|
polymorphic_shapes=["b, ...", None],
|
|
tol=1e-5),
|
|
[
|
|
PolyHarness("mean",
|
|
f"{axis=}_{keepdims=}_where=None",
|
|
lambda x, axis, keepdims: jnp.mean(x, axis=axis, keepdims=keepdims, where=None),
|
|
arg_descriptors=[RandArg((7, 8, 4), _f32), StaticArg(axis), StaticArg(keepdims)],
|
|
polymorphic_shapes=["b, ..."])
|
|
for keepdims in [False, True]
|
|
for axis in [None, (0,), (0, 1), (1,)]
|
|
],
|
|
[
|
|
PolyHarness("mean",
|
|
f"{axis=}_{keepdims=}_where=Some",
|
|
lambda x, where, axis, keepdims: jnp.mean(x, axis=axis, keepdims=keepdims, where=where),
|
|
arg_descriptors=[RandArg((7, 8, 4), _f32), RandArg((7, 8, 4), np.bool_),
|
|
StaticArg(axis), StaticArg(keepdims)],
|
|
polymorphic_shapes=["b, ...", "b, ..."])
|
|
for keepdims in [False, True]
|
|
for axis in [None, (0,), (0, 1), (1,)]
|
|
],
|
|
PolyHarness("jnp.nonzero", "size=constant",
|
|
lambda x: jnp.nonzero(x % 3, size=10, fill_value=100),
|
|
arg_descriptors=[RandArg((3, 2, 4), _i32)],
|
|
polymorphic_shapes=["b, ..."],
|
|
expect_error=expect_error_associative_scan),
|
|
PolyHarness("jnp.nonzero", "size=poly",
|
|
lambda x: jnp.nonzero(x % 3, size=x.shape[0] * 2, fill_value=100),
|
|
arg_descriptors=[RandArg((3, 2, 4), _i32)],
|
|
polymorphic_shapes=["b, ..."],
|
|
expect_error=expect_error_associative_scan),
|
|
PolyHarness("one_hot", "poly_num_classes",
|
|
lambda x, y: jax.nn.one_hot(x, y.shape[0]),
|
|
arg_descriptors=[np.arange(16, dtype=_i32), RandArg((16,), _f32)],
|
|
polymorphic_shapes=[None, "b0, ..."]),
|
|
PolyHarness("one_hot", "all_poly",
|
|
lambda x, y: jax.nn.one_hot(x, y.shape[0]),
|
|
arg_descriptors=[np.arange(16, dtype=_i32), RandArg((16,), _f32)],
|
|
polymorphic_shapes=["b, ...", "b, ..."]),
|
|
PolyHarness("ones", "",
|
|
lambda x: jnp.ones(x.shape, dtype=_f32) + x,
|
|
arg_descriptors=[RandArg((3, 2, 4), _f32)],
|
|
polymorphic_shapes=["b, ..."]),
|
|
PolyHarness("pad", "",
|
|
lax.pad,
|
|
arg_descriptors=[RandArg((3, 2, 5), _f32), np.float32(5.),
|
|
StaticArg(((0, 0, 0), (0, 0, 0), (1, 1, 1)))],
|
|
polymorphic_shapes=["b, ...", None]),
|
|
PolyHarness("pad", "poly_padding_config",
|
|
lambda x: lax.pad(x, _f32(0.),
|
|
((x.shape[0], x.shape[1], x.shape[0]),
|
|
(0, 0, 0))),
|
|
arg_descriptors=[RandArg((3, 2), _f32)],
|
|
polymorphic_shapes=["b, ..."]),
|
|
PolyHarness("jnp.pad", "mode=constant",
|
|
lambda x: jnp.pad(x, [[x.shape[0], 0], [x.shape[1], 1]],
|
|
mode="constant"),
|
|
arg_descriptors=[RandArg((3, 5), _f32)],
|
|
polymorphic_shapes=["b, ..."]),
|
|
PolyHarness("jnp.pad", "mode=constant_bminus1",
|
|
# We slice first the unknown dimension to make it of size b - 1
|
|
# which may be 0.
|
|
lambda x: jnp.pad(lax.dynamic_slice_in_dim(x, 1, x.shape[0] - 1,
|
|
axis=0),
|
|
[[x.shape[0], 0], [x.shape[1], 1]],
|
|
mode="constant"),
|
|
arg_descriptors=[RandArg((3, 5), _f32)],
|
|
polymorphic_shapes=["b, ..."]),
|
|
PolyHarness("jnp.pad", "mode=edge",
|
|
lambda x: jnp.pad(x, [[x.shape[0], 0], [x.shape[1], 1]],
|
|
mode="edge"),
|
|
arg_descriptors=[RandArg((3, 5), _f32)],
|
|
polymorphic_shapes=["b, ..."]),
|
|
PolyHarness("jnp.pad", "mode=maximum",
|
|
lambda x: jnp.pad(x, [[x.shape[0], 0], [x.shape[1], 1]],
|
|
mode="maximum"),
|
|
arg_descriptors=[RandArg((3, 5), _f32)],
|
|
polymorphic_shapes=["b, ..."]),
|
|
PolyHarness("jnp.pad", "mode=maximum_stat_length=b",
|
|
lambda x: jnp.pad(x, [[x.shape[0], 0], [x.shape[1], 1]],
|
|
mode="maximum", stat_length=((x.shape[0] // 2, 2), (2, 2))),
|
|
arg_descriptors=[RandArg((3, 5), _f32)],
|
|
polymorphic_shapes=["b, ..."],
|
|
symbolic_constraints=["b >= 2"]),
|
|
PolyHarness("jnp.pad", "mode=linear_ramp",
|
|
lambda x: jnp.pad(x, [[x.shape[0], 0], [x.shape[1], 1]],
|
|
mode="linear_ramp"),
|
|
arg_descriptors=[RandArg((3, 5), _f32)],
|
|
polymorphic_shapes=["b, ..."],
|
|
symbolic_constraints=["b >= 2"]),
|
|
PolyHarness("jnp.pad", "mode=reflect_odd",
|
|
lambda x: jnp.pad(x, [[x.shape[0] - 1, 0], [x.shape[1], 1]],
|
|
mode="reflect", reflect_type="odd"),
|
|
arg_descriptors=[RandArg((3, 5), _f32)],
|
|
polymorphic_shapes=["b, ..."],
|
|
symbolic_constraints=["b >= 2"]),
|
|
PolyHarness("jnp.pad", "mode=reflect_odd_error",
|
|
lambda x: jnp.pad(x, [[x.shape[0] - 1, 0], [x.shape[1], 1]],
|
|
mode="reflect", reflect_type="odd"),
|
|
arg_descriptors=[RandArg((3, 5), _f32)],
|
|
polymorphic_shapes=["b, ..."],
|
|
expect_error=(ValueError, "Shape polymorphism is supported for jnp.pad")),
|
|
PolyHarness("jnp.pad", "mode=reflect_even",
|
|
lambda x: jnp.pad(x, [[x.shape[0] - 1, 0], [x.shape[1], 1]],
|
|
mode="reflect", reflect_type="even"),
|
|
arg_descriptors=[RandArg((3, 5), _f32)],
|
|
polymorphic_shapes=["b, ..."],
|
|
symbolic_constraints=["b >= 2"]),
|
|
PolyHarness("jnp.pad", "mode=symmetric_odd",
|
|
lambda x: jnp.pad(x, [[x.shape[0], 0], [x.shape[1], 1]],
|
|
mode="symmetric", reflect_type="odd"),
|
|
arg_descriptors=[RandArg((3, 5), _f32)],
|
|
polymorphic_shapes=["b, ..."],
|
|
symbolic_constraints=["b >= 2"]),
|
|
PolyHarness("jnp.pad", "mode=symmetric_even",
|
|
lambda x: jnp.pad(x, [[x.shape[0], 0], [x.shape[1], 1]],
|
|
mode="symmetric", reflect_type="even"),
|
|
arg_descriptors=[RandArg((3, 5), _f32)],
|
|
polymorphic_shapes=["b, ..."],
|
|
symbolic_constraints=["b >= 2"]),
|
|
PolyHarness("jnp.pad", "mode=wrap",
|
|
lambda x: jnp.pad(x, [[x.shape[0], 0], [x.shape[1], 1]],
|
|
mode="wrap"),
|
|
arg_descriptors=[RandArg((3, 5), _f32)],
|
|
polymorphic_shapes=["b, ..."]),
|
|
PolyHarness("percentile", "axis=None",
|
|
lambda x: jnp.percentile(x, 50, axis=None),
|
|
arg_descriptors=[RandArg((3, 5), _f32)],
|
|
polymorphic_shapes=["b, ..."]),
|
|
PolyHarness("nanquantile", "axis=None",
|
|
lambda x: jnp.nanquantile(x, .5, axis=None),
|
|
arg_descriptors=[RandArg((3, 5), _f32)],
|
|
polymorphic_shapes=["b, ..."]),
|
|
PolyHarness("percentile", "axis=0",
|
|
lambda x: jnp.percentile(x, 50, axis=0),
|
|
arg_descriptors=[RandArg((3, 5), _f32)],
|
|
polymorphic_shapes=["b, ..."]),
|
|
PolyHarness("nanquantile", "axis=0",
|
|
lambda x: jnp.nanquantile(x, .5, axis=0),
|
|
arg_descriptors=[RandArg((3, 5), _f32)],
|
|
polymorphic_shapes=["b, ..."]),
|
|
PolyHarness("inv", "",
|
|
lambda x: jnp.linalg.inv(jnp.eye(x.shape[0])),
|
|
arg_descriptors=[RandArg((3, 3), _f32)],
|
|
polymorphic_shapes=["b, b, ..."],
|
|
override_jax_config_flags={"jax_export_ignore_forward_compatibility": True}),
|
|
[
|
|
PolyHarness( # pylint: disable=g-complex-comprehension
|
|
"qr", f"shape={jtu.format_shape_dtype_string(shape, dtype)}_poly={poly}_{full_matrices=}",
|
|
lambda x, full_matrices: lax.linalg.qr(x, full_matrices=full_matrices),
|
|
arg_descriptors=[RandArg(shape, dtype), StaticArg(full_matrices)],
|
|
polymorphic_shapes=[poly],
|
|
symbolic_constraints=constraints)
|
|
for dtype in {np.float32, np.float64, np.complex64, np.complex128} & jtu.supported_dtypes()
|
|
for shape, poly, full_matrices, constraints in [
|
|
((2, 0, 4), "b, ...", False, ()), # m = 0
|
|
((2, 4, 0), "b, ...", False, ()), # n = 0
|
|
((2, 3, 4, 4), "b1, b2, ...", False, ()), # m == n
|
|
((2, 3, 4, 4), "b1, b2, ...", True, ()),
|
|
((2, 3, 4, 5), "b1, b2, ...", False, ()), # m < n
|
|
((2, 3, 4, 5), "b1, b2, ...", True, ()),
|
|
((2, 3, 8, 4), "b1, b2, ...", False, ()), # m > n
|
|
((2, 3, 8, 4), "b1, b2, ...", True, ()),
|
|
# Dynamic shapes are also supported for non-batch dimensions with
|
|
# some constraints.
|
|
((2, 3, 4, 4), "b1, b2, m, m", False, ()), # m == n
|
|
((2, 3, 4, 4), "b1, b2, m, m", True, ()),
|
|
((2, 3, 4, 5), "b1, b2, m, n", False, ["m + 1 <= n"]), # m < n
|
|
((2, 3, 4, 5), "b1, b2, m, n", True, ["m + 1 <= n"]),
|
|
((2, 3, 8, 4), "b1, b2, m, n", False, ["n <= m"]), # m > n
|
|
((2, 3, 8, 4), "b1, b2, m, n", True, ["n <= m"]),
|
|
]
|
|
],
|
|
[
|
|
PolyHarness(
|
|
"lu_pivots_to_permutation",
|
|
f"shape={jtu.format_shape_dtype_string(shape, np.int32)}_poly={poly}_{permutation_size=}",
|
|
lax.linalg.lu_pivots_to_permutation,
|
|
arg_descriptors=[RandArg(shape, np.int32), StaticArg(permutation_size)],
|
|
polymorphic_shapes=[poly],
|
|
symbolic_constraints=constraints,
|
|
)
|
|
for shape, poly, permutation_size, constraints in [
|
|
((4,), None, 8, ()),
|
|
((2, 3, 4), "b1, b2, ...", 8, ()),
|
|
((4,), "b", 8, ["b <= 8"]),
|
|
((2, 3, 4), "b1, b2, b3", 8, ["b3 <= 8"]),
|
|
]
|
|
],
|
|
[
|
|
# Tracing errors are only thrown when the trailing dimension of pivots
|
|
# is static. Otherwise, the error is thrown at runtime.
|
|
PolyHarness(
|
|
"lu_pivots_to_permutation_error",
|
|
f"shape={jtu.format_shape_dtype_string(shape, np.int32)}_poly={poly}_{permutation_size=}",
|
|
lax.linalg.lu_pivots_to_permutation,
|
|
arg_descriptors=[RandArg(shape, np.int32), StaticArg(permutation_size)],
|
|
polymorphic_shapes=[poly],
|
|
symbolic_constraints=constraints,
|
|
expect_error=(ValueError, "Output permutation size"),
|
|
)
|
|
for shape, poly, permutation_size, constraints in [
|
|
((4,), None, 3, ()),
|
|
((2, 3, 4), "b1, b2, ...", 3, ()),
|
|
((4,), "b", 8, ["b >= 9"]),
|
|
((2, 3, 4), "b1, b2, b3", 8, ["b3 >= 9"]),
|
|
]
|
|
],
|
|
[
|
|
PolyHarness( # pylint: disable=g-complex-comprehension
|
|
"lu", f"shape={jtu.format_shape_dtype_string(shape, dtype)}_poly={poly}",
|
|
lax.linalg.lu,
|
|
arg_descriptors=[RandArg(shape, dtype)],
|
|
polymorphic_shapes=[poly])
|
|
for dtype in {np.float32, np.float64, np.complex64, np.complex128} & jtu.supported_dtypes()
|
|
for shape, poly in [
|
|
((5, 4), "m, n"),
|
|
((2, 0, 4), "b, ..."),
|
|
((2, 4, 0), "b, ..."),
|
|
((2, 3, 4, 4), "b1, b2, ..."),
|
|
((2, 3, 4, 5), "b1, b2, ..."),
|
|
((2, 3, 8, 4), "b1, b2, ..."),
|
|
((2, 3, 4, 5), "b1, b2, m, n"),
|
|
]
|
|
],
|
|
[
|
|
PolyHarness( # pylint: disable=g-complex-comprehension
|
|
"eigh", f"shape={jtu.format_shape_dtype_string(shape, dtype)}_poly={poly}_{lower=}",
|
|
lambda x, lower: lax.linalg.eigh(x, lower=lower),
|
|
arg_descriptors=[RandArg(shape, dtype), StaticArg(lower)],
|
|
polymorphic_shapes=[poly])
|
|
for dtype in {np.float32, np.float64, np.complex64, np.complex128} & jtu.supported_dtypes()
|
|
for lower in [True, False]
|
|
for shape, poly in [
|
|
((4, 4), "n, n"),
|
|
((2, 3, 4, 4), "b1, b2, ..."),
|
|
((2, 3, 4, 4), "b1, b2, n, n"),
|
|
]
|
|
],
|
|
[
|
|
PolyHarness( # pylint: disable=g-complex-comprehension
|
|
"eigh_shape_error", f"shape={jtu.format_shape_dtype_string(shape, dtype)}_poly={poly}",
|
|
lambda x: lax.linalg.eigh(x, symmetrize_input=False),
|
|
arg_descriptors=[RandArg(shape, dtype)],
|
|
polymorphic_shapes=[poly],
|
|
expect_error=(ValueError, "Argument to symmetric eigendecomposition"))
|
|
for dtype in {np.float32, np.float64, np.complex64, np.complex128} & jtu.supported_dtypes()
|
|
for shape, poly in [
|
|
((4, 5), "m, n"),
|
|
((2, 3, 4, 5), "b1, b2, ..."),
|
|
((2, 3, 4, 5), "b1, b2, m, n"),
|
|
]
|
|
],
|
|
[
|
|
PolyHarness( # pylint: disable=g-complex-comprehension
|
|
"svd", f"shape={jtu.format_shape_dtype_string(shape, dtype)}_poly={poly}_{full_matrices=}_{compute_uv=}",
|
|
lambda x, full_matrices, compute_uv: lax.linalg.svd(x, full_matrices=full_matrices, compute_uv=compute_uv),
|
|
arg_descriptors=[RandArg(shape, dtype), StaticArg(full_matrices), StaticArg(compute_uv)],
|
|
polymorphic_shapes=[poly],
|
|
symbolic_constraints=constraints)
|
|
for dtype in {np.float32, np.float64, np.complex64, np.complex128} & jtu.supported_dtypes()
|
|
for compute_uv in [True, False]
|
|
for full_matrices in ([True, False] if compute_uv else [True])
|
|
for shape, poly, constraints in [
|
|
((2, 0, 4), "b, ...", ()),
|
|
((2, 4, 0), "b, ...", ()),
|
|
((2, 3, 4, 4), "b1, b2, ...", ()),
|
|
((2, 3, 4, 5), "b1, b2, ...", ()),
|
|
((2, 3, 8, 4), "b1, b2, ...", ()),
|
|
# The constraints listed here are only for the GPU implementation
|
|
# which selects an algorithm based on the size of the matrix.
|
|
((5, 4), "m, n", ["n <= m", "m <= 32", "n <= 32"]),
|
|
((2, 3, 4, 5), "b1, b2, m, n", ["m <= n", "m <= 32", "n <= 32"]),
|
|
]
|
|
],
|
|
[
|
|
# The random primitive tests, with threefry (both partitionable and
|
|
# non-partitionable), and unsafe_rbg.
|
|
[
|
|
PolyHarness("random_gamma", f"{flags_name}",
|
|
lambda key, a: jax.vmap(jax.random.gamma)(
|
|
jax.random.wrap_key_data(key), a),
|
|
arg_descriptors=[RandArg((3, key_size), np.uint32),
|
|
RandArg((3, 4, 5), _f32)],
|
|
polymorphic_shapes=["b, ...", "b, w, ..."], tol=1E-5,
|
|
override_jax_config_flags=override_jax_config_flags), # type: ignore
|
|
PolyHarness("random_categorical", f"axis=0_{flags_name}",
|
|
lambda key, a: jax.random.categorical(
|
|
jax.random.wrap_key_data(key), a, axis=0),
|
|
arg_descriptors=[RandArg((key_size,), np.uint32),
|
|
RandArg((3, 8), _f32)],
|
|
polymorphic_shapes=[None, "b0, b1"],
|
|
override_jax_config_flags=override_jax_config_flags), # type: ignore
|
|
PolyHarness("random_categorical", f"axis=1_{flags_name}",
|
|
lambda key, a: jax.random.categorical(
|
|
jax.random.wrap_key_data(key), a, axis=1),
|
|
arg_descriptors=[RandArg((key_size,), np.uint32),
|
|
RandArg((3, 5, 8), _f32)],
|
|
polymorphic_shapes=[None, "b0, b1, b2"],
|
|
override_jax_config_flags=override_jax_config_flags), # type: ignore
|
|
PolyHarness("random_categorical", f"axis=1_then_reshape_{flags_name}",
|
|
lambda key, a: jax.random.categorical(
|
|
jax.random.wrap_key_data(key), a, axis=1).reshape(-1),
|
|
arg_descriptors=[RandArg((key_size,), np.uint32),
|
|
RandArg((3, 5, 8), _f32)],
|
|
polymorphic_shapes=[None, "b0, b1, b2"],
|
|
override_jax_config_flags=override_jax_config_flags), # type: ignore
|
|
PolyHarness("random_categorical", f"0_dim_{flags_name}", # One axis has 0 size
|
|
lambda key, a: jax.random.categorical(
|
|
jax.random.wrap_key_data(key), a, axis=1),
|
|
arg_descriptors=[RandArg((key_size,), np.uint32),
|
|
RandArg((3, 5, 0), _f32)],
|
|
polymorphic_shapes=[None, "b0, b1, ..."],
|
|
override_jax_config_flags=override_jax_config_flags), # type: ignore
|
|
[
|
|
PolyHarness("random_choice", f"{flags_name}_arr_poly={arr_poly}_shape_poly={shape_poly}_replace={replace}_use_p={use_p}",
|
|
lambda key, a, res_shape, use_p: jax.random.choice(
|
|
jax.random.wrap_key_data(key),
|
|
a,
|
|
shape=res_shape.shape,
|
|
p=jnp.full((a.shape[1],), 0.1, dtype=_f32) if use_p else None,
|
|
axis=1,
|
|
replace=replace),
|
|
arg_descriptors=[RandArg((key_size,), np.uint32),
|
|
RandArg((64, 12, 4), _f32), # sample on axis=1
|
|
RandArg((3, 4), _f32),
|
|
StaticArg(use_p)],
|
|
polymorphic_shapes=[None,
|
|
"b0, b1, b2" if arr_poly else None,
|
|
"b3, b4" if shape_poly else None],
|
|
# The array sampled dimension must be larger than res_shape.size
|
|
symbolic_constraints=[
|
|
"b1 >= 12" if arr_poly else "1 >= 0",
|
|
"b1 >= b3*b4" if arr_poly and shape_poly else "1 >= 0",
|
|
"12 >= b3*b4" if shape_poly else "1 >= 0"
|
|
],
|
|
override_jax_config_flags=override_jax_config_flags,
|
|
expect_error=(
|
|
(NotImplementedError, "permutation")
|
|
if arr_poly and not use_p else None)) # type: ignore
|
|
# np.insert used in random.choice tries to coerce shape_poly to
|
|
# integer arrays, but only when the arr_poly is False.
|
|
for arr_poly in [True, False]
|
|
for shape_poly in [True, False]
|
|
for replace in [True, False]
|
|
for use_p in [True, False]
|
|
],
|
|
PolyHarness("random_split", f"{flags_name}",
|
|
lambda key, a: jax.random.key_data(
|
|
jax.random.split(jax.random.wrap_key_data(key),
|
|
2 * a.shape[0])),
|
|
arg_descriptors=[RandArg((key_size,), np.uint32),
|
|
RandArg((3, 4), _f32)],
|
|
polymorphic_shapes=[None, "b0, ..."],
|
|
override_jax_config_flags=override_jax_config_flags), # type: ignore
|
|
# Works when the known dimensions are known to be even or odd.
|
|
PolyHarness("random_uniform", f"even_1_{flags_name}",
|
|
lambda key, a: jax.random.uniform(jax.random.wrap_key_data(key),
|
|
a.shape, dtype=_f32),
|
|
arg_descriptors=[RandArg((key_size,), np.uint32), RandArg((3, 4, 5), _f32)],
|
|
polymorphic_shapes=[None, "b0, 4, 5"],
|
|
override_jax_config_flags=override_jax_config_flags), # type: ignore
|
|
PolyHarness("random_uniform", f"even_2_{flags_name}",
|
|
lambda key, a: jax.random.uniform(jax.random.wrap_key_data(key),
|
|
a.shape, dtype=_f32),
|
|
arg_descriptors=[RandArg((key_size,), np.uint32), RandArg((3, 4), _f32)],
|
|
polymorphic_shapes=[None, "b0, 2*b1"],
|
|
override_jax_config_flags=override_jax_config_flags), # type: ignore
|
|
PolyHarness("random_uniform", f"error_unknown_evenness_{flags_name}",
|
|
lambda key, a: jax.random.uniform(jax.random.wrap_key_data(key),
|
|
a.shape, dtype=_f32),
|
|
arg_descriptors=[RandArg((key_size,), np.uint32),
|
|
RandArg((3, 5), _f32)],
|
|
polymorphic_shapes=[None, "b0, b1"],
|
|
override_jax_config_flags=override_jax_config_flags) # type: ignore
|
|
]
|
|
for key_size, flags_name, override_jax_config_flags in [
|
|
(2, "threefry_non_partitionable",
|
|
dict(jax_default_prng_impl="threefry2x32", jax_threefry_partitionable=False)),
|
|
(2, "threefry_partitionable",
|
|
dict(jax_default_prng_impl="threefry2x32", jax_threefry_partitionable=True)),
|
|
(4, "unsafe_rbg",
|
|
dict(jax_default_prng_impl="unsafe_rbg"))
|
|
]
|
|
],
|
|
# For reduce_window we have a variant with one reduction axis of
|
|
# non-static shape, and one with additionally the dimension window
|
|
# non-static.
|
|
PolyHarness("reduce_window", "min_window_size=static",
|
|
# x: f32[b, 8]
|
|
lambda x: lax.reduce_window(x, np.array(1., _f32), lax.min,
|
|
(2, 2), (1, 1), "VALID"),
|
|
arg_descriptors=[RandArg((3, 8), _f32)],
|
|
polymorphic_shapes=["b, ..."]),
|
|
PolyHarness("reduce_window", "min_window_size=dynamic",
|
|
# x: f32[b, 8]
|
|
lambda x: lax.reduce_window(x, np.array(1., _f32), lax.min,
|
|
(2, x.shape[0]), (1, 1), "VALID"),
|
|
arg_descriptors=[RandArg((3, 8), _f32)],
|
|
polymorphic_shapes=["b, ..."]),
|
|
PolyHarness("reduce_window", "min_plus_max_window_size=static",
|
|
# x: f32[b, 8]
|
|
lambda x: (
|
|
# Test that we don't get confusion for the reducer name.
|
|
lax.reduce_window(x, np.array(1., _f32), lax.min,
|
|
(2, 2), (1, 1), "VALID") +
|
|
lax.reduce_window(x, np.array(1., _f32), lax.max,
|
|
(2, 2), (1, 1), "VALID")),
|
|
arg_descriptors=[RandArg((3, 8), _f32)],
|
|
polymorphic_shapes=["b, ..."]),
|
|
PolyHarness("reduce_window", "min_plus_max_window_size=dynamic",
|
|
# x: f32[b, 8]
|
|
lambda x: (
|
|
# Test that we don't get confusion for the reducer name.
|
|
lax.reduce_window(x, np.array(1., _f32), lax.min,
|
|
(2, x.shape[0]), (1, 1), "VALID") +
|
|
lax.reduce_window(x, np.array(1., _f32), lax.max,
|
|
(2, x.shape[0]), (1, 1), "VALID")),
|
|
arg_descriptors=[RandArg((3, 8), _f32)],
|
|
polymorphic_shapes=["b, ..."]),
|
|
PolyHarness("reduce_window", "add_monoid_base_window_size=static",
|
|
# x: f32[b, 8]
|
|
lambda x: lax.reduce_window(x, np.array(0., _f32), lax.add,
|
|
(2, 2), (1, 1), "VALID"),
|
|
arg_descriptors=[RandArg((3, 8), _f32)],
|
|
polymorphic_shapes=["b, ..."]),
|
|
PolyHarness("reduce_window", "add_monoid_base_window_size=dynamic",
|
|
# x: f32[b, 8]
|
|
lambda x: lax.reduce_window(x, np.array(0., _f32), lax.add,
|
|
(2, x.shape[0]), (1, 1), "VALID"),
|
|
arg_descriptors=[RandArg((3, 8), _f32)],
|
|
polymorphic_shapes=["b, ..."]),
|
|
# https://github.com/jax-ml/jax/issues/11804
|
|
# Use the reshape trick to simulate a polymorphic dimension of 16*b.
|
|
# (See test "conv_general_dilated.1d_1" above for more details.)
|
|
PolyHarness("reduce_window", "add_monoid_strides_window_size=static",
|
|
# x: f32[1, 16*b, 1]
|
|
lambda x: lax.reduce_window(
|
|
jnp.reshape(x, (1, -1, 1)),
|
|
np.array(0., _f32), lax.add, (1, 4, 1), (1, 2, 1), "SAME"),
|
|
arg_descriptors=[RandArg((1, 128, 16), _f32)],
|
|
polymorphic_shapes=["_, b1, ..."]),
|
|
PolyHarness("reduce_window", "add_generic_window_size=static",
|
|
# x: f32[1, 16*b, 1]
|
|
# Use an initial value of 1. to trigger the generic reduction path
|
|
lambda x: lax.reduce_window(
|
|
jnp.reshape(x, (1, -1, 1)),
|
|
np.array(1., _f32), lax.add, (1, 4, 1), (1, 2, 1), "SAME"),
|
|
arg_descriptors=[RandArg((1, 128, 16), _f32)],
|
|
polymorphic_shapes=["_, b1, ..."]),
|
|
PolyHarness("reduce_window", "variadic_generic_window_size=static",
|
|
# x: f32[b, 8] y: f32[b, 8]
|
|
lambda x, y: lax.reduce_window(
|
|
(x, y), (np.array(1., _f32), np.array(2, _i32)),
|
|
lambda xy0, xy1: (lax.add(xy0[0], xy1[0]),
|
|
lax.sub(xy0[1], xy1[1])),
|
|
(2, 2), (1, 1), "VALID"),
|
|
arg_descriptors=[RandArg((3, 8), _f32), RandArg((3, 8), _i32)],
|
|
polymorphic_shapes=["b, ...", "b, ..."]),
|
|
PolyHarness("reduce_window", "variadic_generic_window_size=dynamic",
|
|
# x: f32[b, 8] y: f32[b, 8]
|
|
lambda x, y: lax.reduce_window(
|
|
(x, y), (np.array(1., _f32), np.array(2, _i32)),
|
|
lambda xy0, xy1: (lax.add(xy0[0], xy1[0]),
|
|
lax.sub(xy0[1], xy1[1])),
|
|
(2, x.shape[0]), (1, 1), "VALID"),
|
|
arg_descriptors=[RandArg((3, 8), _f32), RandArg((3, 8), _i32)],
|
|
polymorphic_shapes=["b, ...", "b, ..."]),
|
|
# TODO(necula): not yet supported, but also unlikely to come up.
|
|
# PolyHarness("random_uniform", "odd",
|
|
# lambda key, a: jax.random.uniform(key, (2 * a.shape[0] + 1, a.shape[1]),
|
|
# dtype=_f32),
|
|
# [RandArg((2,), np.uint32), RandArg((3, 5), _f32)],
|
|
# polymorphic_shapes=[None, "b0, ..."]),
|
|
[
|
|
PolyHarness("reduce", reduce_op.__name__,
|
|
lambda x: reduce_op(x, axis=-1, keepdims=True), # type: ignore
|
|
arg_descriptors=[RandArg((3, 5), _f32)],
|
|
polymorphic_shapes=["b, ..."])
|
|
for reduce_op in [jnp.all, jnp.any, jnp.max, jnp.min, jnp.prod, jnp.sum]
|
|
],
|
|
# Repeat f32[b, 2] * 3
|
|
PolyHarness("repeat", "repeats=int_axis=0",
|
|
lambda x: jnp.repeat(x, repeats=3, axis=0),
|
|
arg_descriptors=[RandArg((3, 2), _f32)],
|
|
polymorphic_shapes=["b, ..."]),
|
|
# Repeat f32[b, 2] * b
|
|
PolyHarness("repeat", "repeats=poly_axis=0",
|
|
lambda x: jnp.repeat(x, repeats=x.shape[0], axis=0),
|
|
arg_descriptors=[RandArg((3, 2), _f32)],
|
|
polymorphic_shapes=["b, ..."]),
|
|
# Repeat f32[b, 2] * b
|
|
PolyHarness("repeat", "repeats=poly_axis=None",
|
|
lambda x: jnp.repeat(x, repeats=x.shape[0], axis=None),
|
|
arg_descriptors=[RandArg((3, 2), _f32)],
|
|
polymorphic_shapes=["b, ..."]),
|
|
# Repeat f32 * b
|
|
PolyHarness("repeat", "repeats=poly_axis=None_scalar",
|
|
lambda x, y: jnp.expand_dims(jnp.repeat(x, repeats=y.shape[0], axis=None), axis=1) + y,
|
|
arg_descriptors=[RandArg((), _f32), RandArg((3, 1), _f32)],
|
|
polymorphic_shapes=[None, "b0, ..."]),
|
|
PolyHarness("repeat", "repeats=poly_axis=None_total_repeat_length1",
|
|
lambda x: jnp.repeat(x, repeats=x.shape[0], axis=None, total_repeat_length=8),
|
|
arg_descriptors=[RandArg((3, 2), _f32)],
|
|
polymorphic_shapes=["b, ..."],
|
|
expect_error=(ValueError, "jnp.repeat with a non-constant `repeats` is supported only .*")),
|
|
PolyHarness("reshape", "0",
|
|
lambda x: x.reshape([x.shape[0], -1]),
|
|
arg_descriptors=[RandArg((3, 2, 3), _f32)],
|
|
polymorphic_shapes=["b, ..."]),
|
|
PolyHarness("reshape", "1",
|
|
lambda x: x.reshape([x.shape[0], -1]),
|
|
arg_descriptors=[RandArg((3, 2, 3), _f32)],
|
|
polymorphic_shapes=["b0, b1, ..."]),
|
|
PolyHarness("reshape", "2",
|
|
lambda x: x.reshape([x.shape[0], -1, x.shape[3], x.shape[2]]),
|
|
arg_descriptors=[RandArg((3, 4, 5, 6, 7), _f32)],
|
|
polymorphic_shapes=["b0, _, b2, b3, ..."]),
|
|
PolyHarness("reshape", "3",
|
|
lambda x: jnp.reshape(x, [2, -1]),
|
|
arg_descriptors=[RandArg((3, 4, 5, 6, 7), _f32)],
|
|
polymorphic_shapes=["b0, _, b2, ..."]),
|
|
PolyHarness("reshape", "_issue_9975",
|
|
# The newshape is a scalar
|
|
lambda x: jnp.reshape(x, x.shape[0] * x.shape[1]),
|
|
arg_descriptors=[RandArg((3, 4), _f32)],
|
|
polymorphic_shapes=["b, ..."]),
|
|
PolyHarness("reshape", "error",
|
|
lambda x: x.reshape([x.shape[0], -1, 3]),
|
|
arg_descriptors=[RandArg((3, 2, 4), _f32)],
|
|
polymorphic_shapes=["b, ..."],
|
|
expect_error=(core.InconclusiveDimensionOperation,
|
|
re.escape(
|
|
"Cannot divide evenly the sizes of shapes (b, 2, 4) and (b, -1, 3)"))),
|
|
PolyHarness("roll", "axis=0",
|
|
lambda x: jnp.roll(x, 2, axis=0),
|
|
arg_descriptors=[RandArg((3, 4), _f32)],
|
|
polymorphic_shapes=["b, ..."]),
|
|
PolyHarness("roll", "axis=None",
|
|
lambda x: jnp.roll(x, 2),
|
|
arg_descriptors=[RandArg((3, 4), _f32)],
|
|
polymorphic_shapes=["b, ..."]),
|
|
PolyHarness("scatter_add", "",
|
|
partial(lax.scatter_add, indices_are_sorted=False, unique_indices=True),
|
|
arg_descriptors=[RandArg((7, 4), _f32), # op: [b, 4]
|
|
np.array([[1], [2]], np.int32), # indices: [2, 1]
|
|
RandArg((7, 2), _f32), # updates: [b, 2]
|
|
StaticArg(lax.ScatterDimensionNumbers((0,), (1,), (1,)))],
|
|
polymorphic_shapes=["b, ...", None, "b, ..."]),
|
|
PolyHarness("scatter_add", "clip0",
|
|
partial(lax.scatter_add, indices_are_sorted=False, unique_indices=True, mode=lax.GatherScatterMode.CLIP),
|
|
arg_descriptors=[RandArg((7, 4), _f32), # op: [b, 4]
|
|
np.array([[1], [2]], np.int32), # indices: [2, 1]
|
|
RandArg((7, 2), _f32), # updates: [b, 2]
|
|
StaticArg(lax.ScatterDimensionNumbers((0,), (1,), (1,)))],
|
|
polymorphic_shapes=["b, ...", None, "b, ..."]),
|
|
PolyHarness("scatter_add", "clip1",
|
|
partial(lax.scatter_add, indices_are_sorted=False, unique_indices=True, mode=lax.GatherScatterMode.CLIP),
|
|
arg_descriptors=[RandArg((7, 4), _f32), # op: [b, 4]
|
|
# indices: [b, 2]
|
|
np.array([[1, 2], [-2, 0], [6, 4], [7, -1], [1, 0], [3, 0], [0, 5]], np.int32),
|
|
RandArg((7, 1), _f32), # updates: [b, 1]
|
|
StaticArg(lax.ScatterDimensionNumbers((1,), (0,), (0, 1,)))],
|
|
polymorphic_shapes=["b, ...", "b, ...", "b, ..."]),
|
|
PolyHarness("scatter_grad", "",
|
|
lambda *args: jax.grad(
|
|
lambda *args:
|
|
jnp.sum(lax.scatter( # type: ignore
|
|
*args,
|
|
indices_are_sorted=False,
|
|
unique_indices=False,
|
|
))
|
|
)(*args),
|
|
arg_descriptors=[RandArg((7, 4), _f32), # : [b, 4]
|
|
np.array([[1], [2]], np.int32), # indices: [2, 1]
|
|
RandArg((7, 2), _f32), # updates: [b, 2]
|
|
StaticArg(lax.ScatterDimensionNumbers((0,), (1,), (1,))),
|
|
],
|
|
polymorphic_shapes=["b, ...", None, "b, ..."]),
|
|
PolyHarness("scatter_grad", "poly_indices",
|
|
lambda *args: jax.grad(
|
|
lambda *args:
|
|
jnp.sum(lax.scatter( # type: ignore
|
|
*args,
|
|
indices_are_sorted=False,
|
|
unique_indices=False))
|
|
)(*args),
|
|
arg_descriptors=[RandArg((7, 4), _f32), # op: [b, 4]
|
|
# indices: [b, 2]
|
|
np.array(
|
|
[[1, 2], [-2, 0], [6, 4], [7, -1], [1, 0],
|
|
[3, 0], [0, 5]], np.int32),
|
|
RandArg((7, 1), _f32), # updates: [b, 1]
|
|
StaticArg(lax.ScatterDimensionNumbers((1,), (0,), (0, 1))),
|
|
],
|
|
polymorphic_shapes=["b, ...", "b, ...", "b, ..."]),
|
|
[
|
|
PolyHarness("segment", f"{name}_bucket_size_{bucket_size}",
|
|
lambda x, segment_ids: op(
|
|
x, # f32[b, s, buckets]
|
|
segment_ids, # i32[b]
|
|
num_segments=x.shape[1],
|
|
bucket_size=(x.shape[2]
|
|
if bucket_size == "poly" else bucket_size)),
|
|
arg_descriptors=[RandArg((8, 5, 2), _f32),
|
|
np.array([0, 0, 0, 1, 1, 3, 3, 3],
|
|
dtype=np.int32)
|
|
],
|
|
polymorphic_shapes=["b, s, buckets", "b"])
|
|
for name, op in [
|
|
("max", ops.segment_max),
|
|
("min", ops.segment_min),
|
|
("sum", ops.segment_sum),
|
|
("prod", ops.segment_prod),
|
|
]
|
|
for bucket_size in [None, 2, "poly"]
|
|
],
|
|
[
|
|
PolyHarness("schur",
|
|
f"shape={jtu.format_shape_dtype_string(shape, dtype)}_{poly=}_{compute_schur_vectors=}",
|
|
lambda a, compute_schur_vectors: lax.linalg.schur(
|
|
a, compute_schur_vectors=compute_schur_vectors),
|
|
arg_descriptors=[RandArg(shape, dtype),
|
|
StaticArg(compute_schur_vectors)],
|
|
polymorphic_shapes=[poly])
|
|
for dtype in {np.float32, np.float64, np.complex64, np.complex128} & jtu.supported_dtypes()
|
|
for compute_schur_vectors in [True, False]
|
|
for (shape, poly) in [
|
|
((3, 3), "w, w"),
|
|
((3, 4, 4), "b, w, w"),
|
|
]
|
|
],
|
|
PolyHarness("select", "0",
|
|
# x.shape = (b, 3)
|
|
lambda x: lax.select(x > 5., x, x),
|
|
arg_descriptors=[RandArg((7, 3), _f32)],
|
|
polymorphic_shapes=["b, ..."]),
|
|
PolyHarness("select", "1",
|
|
# x.shape = (b, 3); y.shape = (3,)
|
|
jax.vmap(lambda x, y: lax.select(x > 5., x, y), in_axes=[0, None]),
|
|
arg_descriptors=[RandArg((7, 3), _f32), RandArg((3,), _f32)],
|
|
polymorphic_shapes=["b, ...", None]),
|
|
PolyHarness("slice", "entire_axis",
|
|
lambda x: lax.slice(x, start_indices=(0, 1), limit_indices=(x.shape[0], 3)),
|
|
arg_descriptors=[RandArg((7, 3), _f32)],
|
|
polymorphic_shapes=["b, ..."]),
|
|
PolyHarness("slice_in_dim", "entire_axis",
|
|
lambda x: lax.slice_in_dim(x, 0, x.shape[0], stride=1, axis=0),
|
|
arg_descriptors=[RandArg((3, 4), _f32)],
|
|
polymorphic_shapes=["b, ..."]),
|
|
PolyHarness("slice_in_dim", "start=neg",
|
|
lambda x: lax.slice_in_dim(x, -1, x.shape[0], stride=1, axis=0),
|
|
arg_descriptors=[RandArg((3, 4), _f32)],
|
|
polymorphic_shapes=["b, ..."]),
|
|
PolyHarness("slice_in_dim", "limit=neg",
|
|
lambda x: lax.slice_in_dim(x, 0, -1, stride=1, axis=0),
|
|
arg_descriptors=[RandArg((3, 4), _f32)],
|
|
polymorphic_shapes=["b, ..."]),
|
|
PolyHarness("slice_in_dim", "stride=2_even",
|
|
lambda x: lax.slice_in_dim(x, 0, x.shape[0], stride=2, axis=0),
|
|
arg_descriptors=[RandArg((12, 4), _f32)],
|
|
polymorphic_shapes=["b, ..."]),
|
|
PolyHarness("slice_in_dim", "stride=2_odd",
|
|
lambda x: lax.slice_in_dim(x, 0, x.shape[0], stride=2, axis=0),
|
|
arg_descriptors=[RandArg((13, 4), _f32)],
|
|
polymorphic_shapes=["b, ..."]),
|
|
PolyHarness("slice_in_dim", "stride_sym",
|
|
lambda x: lax.slice_in_dim(x, 0, x.shape[0], stride=1 + x.shape[0] // 4, axis=0),
|
|
arg_descriptors=[RandArg((13, 4), _f32)],
|
|
polymorphic_shapes=["b, ..."]),
|
|
PolyHarness("sort", "",
|
|
lambda a: lax.sort(a),
|
|
arg_descriptors=[RandArg((16,), _f32)],
|
|
polymorphic_shapes=["b"]),
|
|
PolyHarness("jvp_sort", "",
|
|
lambda a: jax.jvp(lax.sort, (a,), (a,)),
|
|
arg_descriptors=[RandArg((16,), _f32)],
|
|
polymorphic_shapes=["b"]),
|
|
PolyHarness("jnp_split", "idx_tuple_ct",
|
|
# The indices are a tuple with constants
|
|
lambda a: jnp.split(a, (2,)),
|
|
arg_descriptors=[RandArg((16,), _f32)],
|
|
polymorphic_shapes=["b + 4"]),
|
|
PolyHarness("jnp_split", "idx_tuple_poly",
|
|
# The indices are a tuple with poly expressions
|
|
lambda a: jnp.split(a, (a.shape[0] - 2,), axis=0),
|
|
arg_descriptors=[RandArg((16,), _f32)],
|
|
polymorphic_shapes=["b + 4"]),
|
|
PolyHarness("jnp_split", "idx_ct",
|
|
# A constant num_sections
|
|
lambda a: jnp.split(a, 2, axis=0),
|
|
arg_descriptors=[RandArg((16,), _f32)],
|
|
polymorphic_shapes=["2*b + 4"]),
|
|
PolyHarness("jnp_split", "idx_poly",
|
|
# A poly expression as num_sections
|
|
lambda a: jnp.split(a, a.shape[0] // 2, axis=0),
|
|
arg_descriptors=[RandArg((16,), _f32)],
|
|
polymorphic_shapes=["b + 4"],
|
|
expect_error=(ValueError,
|
|
"jax.numpy.split with a symbolic number of sections is not supported")),
|
|
PolyHarness("squeeze", "axis=empty",
|
|
jnp.squeeze,
|
|
arg_descriptors=[RandArg((5,), _f32), StaticArg(())],
|
|
polymorphic_shapes=["b, ..."]),
|
|
PolyHarness("squeeze", "axis=None",
|
|
jnp.squeeze,
|
|
arg_descriptors=[RandArg((5,), _f32), StaticArg(None)],
|
|
polymorphic_shapes=["b, ..."],
|
|
expect_error=(ValueError, "jnp.squeeze with axis=None is not supported with shape polymorphism")),
|
|
PolyHarness("squeeze", "axis=1",
|
|
jnp.squeeze,
|
|
arg_descriptors=[RandArg((4, 1), _f32), StaticArg((1,))],
|
|
polymorphic_shapes=["b, ..."]),
|
|
PolyHarness("squeeze", "axis=1_2",
|
|
jnp.squeeze,
|
|
arg_descriptors=[RandArg((4, 1, 1), _f32), StaticArg((1, 2))],
|
|
polymorphic_shapes=["b, ..."]),
|
|
PolyHarness("squeeze", "error",
|
|
jnp.squeeze,
|
|
arg_descriptors=[RandArg((3, 33), _f32), StaticArg(-1)],
|
|
polymorphic_shapes=["b0, b1"],
|
|
expect_error=(ValueError,
|
|
re.escape(
|
|
"cannot select an axis to squeeze out which has size not equal to one, got shape=(b0, b1) and dimensions=(1,)"))
|
|
),
|
|
PolyHarness("take", "",
|
|
lambda a, i: jnp.take(a, i, axis=1),
|
|
arg_descriptors=[RandArg((3, 4, 5), _f32), np.array([1, 2], np.int32)],
|
|
polymorphic_shapes=["b, ...", None]),
|
|
PolyHarness("take_along_axis", "0",
|
|
lambda x, y: jnp.take_along_axis(x, y, axis=0),
|
|
arg_descriptors=[RandArg((5, 2), _f32), RandArg((5, 1), np.int32)],
|
|
polymorphic_shapes=["b, ...", "b, ..."]),
|
|
PolyHarness("take_along_axis", "1",
|
|
lambda x, y: jnp.take_along_axis(x, y, axis=1),
|
|
arg_descriptors=[RandArg((5, 2), _f32), RandArg((5, 1), np.int32)],
|
|
polymorphic_shapes=["b, ...", "b, ..."]),
|
|
PolyHarness("tile", "0",
|
|
lambda x: jnp.tile(x, (1, 2)),
|
|
arg_descriptors=[RandArg((4, 3), _f32)],
|
|
polymorphic_shapes=["b, ..."]),
|
|
PolyHarness("tile", "1",
|
|
# The repetitions are polys
|
|
lambda x: jnp.tile(x, (1, x.shape[0])),
|
|
arg_descriptors=[RandArg((4, 2), _f32)],
|
|
polymorphic_shapes=["b, ..."]),
|
|
PolyHarness("lax_top_k", "",
|
|
lambda x: jax.lax.top_k(x, x.shape[-1] - 1),
|
|
arg_descriptors=[RandArg((16,), _f32)],
|
|
polymorphic_shapes=["b, ..."]),
|
|
PolyHarness("tri", "N=poly_M=None",
|
|
lambda x: jnp.tri(x.shape[0]) + x,
|
|
arg_descriptors=[RandArg((3, 1), _f32)],
|
|
polymorphic_shapes=["b, ..."]),
|
|
PolyHarness("tri", "N=poly_M=poly",
|
|
lambda x: jnp.tri(x.shape[0], M=x.shape[0] + 2) + x,
|
|
arg_descriptors=[RandArg((3, 1), _f32)],
|
|
polymorphic_shapes=["b, ..."]),
|
|
PolyHarness("tril", "",
|
|
lambda x: jnp.tril(jnp.ones((x.shape[0], x.shape[0] + x.shape[1]),
|
|
dtype=_f32),
|
|
k=x.shape[1]),
|
|
arg_descriptors=[RandArg((3, 4), _f32)],
|
|
polymorphic_shapes=["m, n"]),
|
|
[
|
|
PolyHarness("triangular_solve",
|
|
f"shape={jtu.format_shape_dtype_string(a_shape, dtype)}_{left_side=}_{a_poly=}_{b_poly=}",
|
|
lambda a, b, left_side: lax.linalg.triangular_solve(
|
|
jnp.tril(a) + 5 * jnp.eye(a.shape[-1], dtype=a.dtype),
|
|
b, left_side=left_side,
|
|
lower=True, transpose_a=False, conjugate_a=False,
|
|
unit_diagonal=False),
|
|
arg_descriptors=[RandArg(a_shape, dtype),
|
|
RandArg(b_shape, dtype),
|
|
StaticArg(left_side)],
|
|
polymorphic_shapes=[a_poly, b_poly],
|
|
# Some of the cases have batch dimensions for `a`, so the
|
|
# "+" needs rank promotion.
|
|
override_jax_config_flags={"jax_numpy_rank_promotion": "allow"})
|
|
|
|
for dtype in {np.float32, np.float64, np.complex64, np.complex128} & jtu.supported_dtypes()
|
|
for (left_side, a_shape, b_shape, a_poly, b_poly) in [
|
|
(True, (3, 4, 4), (3, 4, 5), "b, ...", "b, ..."),
|
|
(True, (3, 4, 4), (3, 4, 5), "b, k, k", "b, k, m"),
|
|
(False, (3, 4, 4), (3, 5, 4), "b, ...", "b, ..."),
|
|
(False, (3, 4, 4), (3, 5, 4), "b, k, k", "b, m, k"),
|
|
# We use custom calls on CPU if not batched
|
|
(True, (4, 4), (4, 5), "k, k", "k, m"),
|
|
(False, (4, 4), (5, 4), "k, k", "m, k"),
|
|
]
|
|
],
|
|
[
|
|
PolyHarness("var",
|
|
f"{axis=}_{keepdims=}_where=None",
|
|
lambda x, axis, keepdims: jnp.var(x, axis=axis, keepdims=keepdims, where=None),
|
|
arg_descriptors=[RandArg((7, 8, 4), _f32),
|
|
StaticArg(axis),
|
|
StaticArg(keepdims)],
|
|
polymorphic_shapes=["b, ..."])
|
|
for keepdims in [False, True]
|
|
for axis in [None, (0,), (0, 1), (1,)]
|
|
],
|
|
[
|
|
PolyHarness("var",
|
|
f"{axis=}_{keepdims=}_where=Some",
|
|
lambda x, where, axis, keepdims: jnp.var(x, axis=axis, keepdims=keepdims, where=where),
|
|
arg_descriptors=[RandArg((7, 8, 4), _f32),
|
|
RandArg((7, 8, 4), np.bool_),
|
|
StaticArg(axis),
|
|
StaticArg(keepdims)],
|
|
polymorphic_shapes=["b, ...", "b, ..."])
|
|
for keepdims in [False, True]
|
|
for axis in [None, (0,), (0, 1), (1,)]
|
|
],
|
|
PolyHarness("where", "",
|
|
jnp.where,
|
|
arg_descriptors=[RandArg((2,), np.bool_), RandArg((), _f32), RandArg((2,), _f32)],
|
|
polymorphic_shapes=["b, ...", None, "b, ..."]),
|
|
]
|
|
|
|
|
|
### We add to the test harnesses some that are obtained from the
|
|
### primitive harnesses by applying vmap to the function and then asserting
|
|
### that we can convert shape polymorphically the result.
|
|
def _make_vmap_primitive_harnesses() -> Sequence[PolyHarness]:
|
|
"""For each harness group, pick a single dtype.
|
|
|
|
See PolyHarness for documentation.
|
|
"""
|
|
all_h = test_harnesses.all_harnesses
|
|
res = []
|
|
|
|
# Index by group
|
|
harness_groups: dict[
|
|
str, Sequence[test_harnesses.Harness]] = collections.defaultdict(list)
|
|
device = jtu.device_under_test()
|
|
|
|
for h in all_h:
|
|
# Drop the JAX limitations
|
|
if not h.filter(device_under_test=device, include_jax_unimpl=False):
|
|
continue
|
|
harness_groups[h.group_name].append(h)
|
|
|
|
selected_harnesses = []
|
|
for _, hlist in harness_groups.items():
|
|
# Pick the dtype with the most harnesses in this group. Some harness
|
|
# groups only test different use cases at a few dtypes.
|
|
c = collections.Counter([h.dtype for h in hlist])
|
|
(_, max_count), = c.most_common(1)
|
|
# Pick the first alphabetically among those with max_count, to ensure
|
|
# that we generate deterministic tests.
|
|
dtypes_with_max_count = (dtype for dtype, count in c.items()
|
|
if count == max_count)
|
|
dtype, *_ = sorted(dtypes_with_max_count, key=str)
|
|
selected_harnesses.extend([h for h in hlist if h.dtype == dtype])
|
|
|
|
batch_size = 3
|
|
for h in selected_harnesses:
|
|
if h.group_name in [
|
|
"tridiagonal_solve", # batching not implemented in JAX
|
|
]:
|
|
continue
|
|
|
|
def make_batched_arg_descriptor(
|
|
ad: test_harnesses.ArgDescriptor) -> test_harnesses.ArgDescriptor | None:
|
|
if isinstance(ad, RandArg):
|
|
return RandArg((batch_size,) + ad.shape, ad.dtype)
|
|
elif isinstance(ad, CustomArg):
|
|
def wrap_custom(rng):
|
|
arg = ad.make(rng)
|
|
return np.stack([arg] * batch_size)
|
|
|
|
return CustomArg(wrap_custom)
|
|
else:
|
|
assert isinstance(ad, np.ndarray), ad
|
|
return np.stack([ad] * batch_size)
|
|
|
|
new_args = [make_batched_arg_descriptor(ad)
|
|
for ad in h.arg_descriptors
|
|
if not isinstance(ad, StaticArg)]
|
|
|
|
# This test does not make sense for nullary functions
|
|
if not new_args:
|
|
continue
|
|
|
|
vmap_harness = PolyHarness("vmap_" + h.group_name, h.name,
|
|
jax.vmap(h.dyn_fun, in_axes=0, out_axes=0),
|
|
arg_descriptors=new_args,
|
|
polymorphic_shapes=["b, ..."] * len(new_args))
|
|
vmap_harness.original_harness = h
|
|
res.append(vmap_harness)
|
|
return res
|
|
|
|
_POLY_SHAPE_TEST_HARNESSES.append(_make_vmap_primitive_harnesses())
|
|
|
|
def _flatten_harnesses(harnesses):
|
|
res = []
|
|
for h in harnesses:
|
|
if isinstance(h, Sequence):
|
|
res.extend(_flatten_harnesses(h))
|
|
else:
|
|
res.append(h)
|
|
return res
|
|
|
|
|
|
class ShapePolyHarnessesTest(jtu.JaxTestCase):
|
|
"""This test runs for all _POLY_SHAPE_PRIMITIVE_HARNESSES."""
|
|
|
|
def setUp(self):
|
|
_start_profile(self)
|
|
super().setUp()
|
|
|
|
def tearDown(self):
|
|
super().tearDown()
|
|
_stop_profile(self)
|
|
|
|
# For each primitive "xxx" the test will be called "test_harness_xxx_...".
|
|
# If you want to run this test for only one harness that includes "foo"
|
|
# in the name (after test_harness), add parameter `one_containing="foo"`
|
|
# to parameterized below.
|
|
@test_harnesses.parameterized(
|
|
_flatten_harnesses(_POLY_SHAPE_TEST_HARNESSES),
|
|
#one_containing="",
|
|
)
|
|
def test_harness(self, harness: PolyHarness):
|
|
# We do not expect the associative scan error on TPUs
|
|
if harness.expect_error == expect_error_associative_scan and jtu.test_device_matches(["tpu"]):
|
|
harness.expect_error = None
|
|
|
|
if harness.group_name == "schur" and not jtu.test_device_matches(["cpu"]):
|
|
raise unittest.SkipTest("schur decomposition is only implemented on CPU.")
|
|
|
|
if "fft_fft_type" in harness.fullname:
|
|
if "nr_fft_lengths_2" in harness.fullname:
|
|
raise unittest.SkipTest("native serialization with shape polymorphism not implemented for fft with non-constant fft_lengths on GPU and TPU")
|
|
|
|
if harness.group_name == "vmap_eigh":
|
|
raise unittest.SkipTest(
|
|
"Should not compare eigendecompositions for equality directly"
|
|
"because eigenvalues are sorted.")
|
|
|
|
if harness.group_name == "vmap_tan":
|
|
# Tan (b/274462307) require support for custom call stablehlo.tan.
|
|
raise unittest.SkipTest(
|
|
"native lowering with shape polymorphism requires additional StableHLO feature support")
|
|
|
|
if (jtu.test_device_matches(["cpu", "gpu"]) and
|
|
harness.fullname in [
|
|
"cumsum_reduce_axis_poly", "cumprod_reduce_axis_poly",
|
|
"cummin_reduce_axis_poly", "cummax_reduce_axis_poly",
|
|
"cumlogsumexp_reduce_axis_poly",
|
|
"jnp_insert_insert_constant", "jnp_insert_insert_poly",
|
|
"jnp_nonzero_size_constant", "jnp_nonzero_size_poly"]):
|
|
# Need associative scan reductions on CPU and GPU. On TPU we use the
|
|
# reduce_window HLO, but on CPU and GPU (with axis size >= 32) we use
|
|
# a recursive associative scan that we cannot express with shape
|
|
# polymorphism.
|
|
raise unittest.SkipTest(
|
|
"native serialization with shape polymorphism not implemented for window_reductions on CPU and GPU")
|
|
|
|
if harness.group_name == "vmap_conv_general_dilated":
|
|
# https://github.com/openxla/stablehlo/issues/1268
|
|
raise unittest.SkipTest("Need more dynamism for DynamicConvOp")
|
|
|
|
if harness.group_name == "eig" and not jtu.test_device_matches(["cpu"]):
|
|
raise unittest.SkipTest("JAX implements eig only on CPU.")
|
|
|
|
if (harness.group_name in ("eigh", "svd") and
|
|
not harness.polymorphic_shapes[0].endswith("...") and
|
|
jtu.test_device_matches(["tpu"])):
|
|
raise unittest.SkipTest(
|
|
"Shape polymorphism for Eigh and Svd is only supported for batch dimensions on TPU.")
|
|
|
|
config_flags = harness.override_jax_config_flags
|
|
# Update this here rather than in harness object because vmap_random_gamma is derived
|
|
# from test_harnesses.all_harnesses, which strips override_jax_config_flags.
|
|
if "random_gamma" in harness.group_name:
|
|
config_flags = {**config_flags, "jax_debug_key_reuse": False}
|
|
|
|
# TPU precision is a little lower since we swap the order of matmul operands.
|
|
if "cholesky" in harness.group_name and jtu.test_device_matches(["tpu"]):
|
|
harness.tol = 5e-5
|
|
|
|
with jtu.thread_local_config_context(**config_flags):
|
|
harness.run_test(self)
|
|
|
|
|
|
if __name__ == "__main__":
|
|
absltest.main(testLoader=jtu.JaxTestLoader())
|