mirror of
https://github.com/ROCm/jax.git
synced 2025-04-16 03:46:06 +00:00
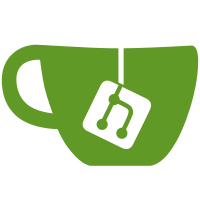
Also removes a bunch of dead version guards (0.4.7 has xla_extension_version 144 and mlir_api_version 47)
2655 lines
117 KiB
Python
2655 lines
117 KiB
Python
# Copyright 2020 The JAX Authors.
|
|
#
|
|
# Licensed under the Apache License, Version 2.0 (the "License");
|
|
# you may not use this file except in compliance with the License.
|
|
# You may obtain a copy of the License at
|
|
#
|
|
# https://www.apache.org/licenses/LICENSE-2.0
|
|
#
|
|
# Unless required by applicable law or agreed to in writing, software
|
|
# distributed under the License is distributed on an "AS IS" BASIS,
|
|
# WITHOUT WARRANTIES OR CONDITIONS OF ANY KIND, either express or implied.
|
|
# See the License for the specific language governing permissions and
|
|
# limitations under the License.
|
|
"""Tests for the shape-polymorphic jax2tf conversion."""
|
|
import contextlib
|
|
import unittest
|
|
|
|
from absl.testing import absltest, parameterized
|
|
from typing import Any, Callable, Dict, List, Optional, Sequence, Tuple, Union
|
|
|
|
import collections
|
|
import functools
|
|
from functools import partial
|
|
import operator as op
|
|
import re
|
|
|
|
import jax
|
|
from jax import core
|
|
from jax.experimental import jax2tf
|
|
from jax.experimental.jax2tf import shape_poly
|
|
from jax.experimental import pjit
|
|
from jax import lax
|
|
import jax.numpy as jnp
|
|
from jax import random
|
|
from jax._src import test_util as jtu
|
|
from jax._src import util
|
|
from jax._src.lax import lax as lax_internal
|
|
from jax._src.lax import control_flow as lax_control_flow
|
|
from jax._src.lib import xla_client
|
|
import numpy as np
|
|
|
|
from jax.experimental.jax2tf.tests import tf_test_util
|
|
|
|
import tensorflow as tf # type: ignore[import]
|
|
|
|
from jax.config import config
|
|
from jax._src.config import numpy_dtype_promotion
|
|
|
|
config.parse_flags_with_absl()
|
|
|
|
# Import after parsing flags
|
|
from jax.experimental.jax2tf.tests import primitive_harness
|
|
from jax.experimental.jax2tf.tests.primitive_harness import Harness, CustomArg, RandArg, StaticArg
|
|
from jax.experimental.jax2tf.tests.jax2tf_limitations import Jax2TfLimitation
|
|
|
|
PS = jax2tf.PolyShape
|
|
_f32 = np.float32
|
|
_i32 = np.int32
|
|
|
|
expect_error_associative_scan = (
|
|
(None, None) if (not config.jax2tf_default_native_serialization or
|
|
jtu.device_under_test() == "tpu") else
|
|
(NotImplementedError,
|
|
"associative scan over axis of non-constant size"))
|
|
|
|
|
|
class DimExprTest(tf_test_util.JaxToTfTestCase):
|
|
|
|
def test_parse_poly_spec(self):
|
|
self.assertEqual((2, 3), shape_poly._parse_spec(None, (2, 3)))
|
|
self.assertEqual((2, 3), shape_poly._parse_spec("2, 3", (2, 3)))
|
|
self.assertEqual((2, 3), shape_poly._parse_spec("2, _", (2, 3)))
|
|
self.assertEqual((2, 3), shape_poly._parse_spec("2, ...", (2, 3)))
|
|
self.assertEqual((2, 3), shape_poly._parse_spec("...", (2, 3)))
|
|
self.assertEqual((2, 3), shape_poly._parse_spec(" ( 2 , 3 ) ", (2, 3)))
|
|
|
|
a, b = shape_poly._parse_spec("a, b", (2, 3))
|
|
self.assertEqual((a, 3), shape_poly._parse_spec("(a, ...) ", (None, 3)))
|
|
tshape = tf.TensorShape([None, 3])
|
|
self.assertEqual((a, 3), shape_poly._parse_spec("(a, ...) ", tshape))
|
|
|
|
a, b = shape_poly._parse_spec("a, b", (2, 3))
|
|
@parameterized.named_parameters(
|
|
dict(testcase_name=f"_{dim_spec=}",
|
|
dim_spec=dim_spec, dim_poly=dim_poly)
|
|
for dim_spec, dim_poly in [
|
|
("2*a*b", 2 * a * b),
|
|
("-2 * a^2 * b + b^2", -2 * a * a * b + b * b),
|
|
("-2 * a^2 * b + -1 *b^2*a", -2 * a * a * b - a * b * b),
|
|
("3 * a * b * a + -2", 3 * a * b * a - 2),
|
|
("a + 1", a + 1),
|
|
("a + -1", a - 1),
|
|
])
|
|
def test_parse_poly_spec_poly(self,
|
|
dim_spec="3 * a * b * a + -2",
|
|
dim_poly=3 * a * b * a - 2):
|
|
# For internal usage only (the polymorphic_shapes of VJP) we need to
|
|
# parse polynomials.
|
|
self.assertEqual((dim_poly,), shape_poly._parse_spec(dim_spec, (2,)))
|
|
self.assertEqual((dim_poly,), shape_poly._parse_spec(str(dim_poly), (2,)))
|
|
|
|
@parameterized.named_parameters(
|
|
dict(testcase_name=f"_{dim_spec=}",
|
|
dim_spec=dim_spec, dim_poly=dim_poly)
|
|
for dim_spec, dim_poly in [
|
|
("2*a*b", 2 * a * b),
|
|
("-2 * a^2 * b + b^2", -2 * a * a * b + b * b),
|
|
("-2 * a^2 * b + -1 *b^2*a", -2 * a * a * b - a * b * b),
|
|
("3 * a * b * a + -2", 3 * a * b * a - 2),
|
|
("a + 1", a + 1),
|
|
("a + -1", a - 1),
|
|
])
|
|
def test_parse_poly_spec_shapeenv(self,
|
|
dim_spec="3 * a * b * a + -2",
|
|
dim_poly=3 * a * b * a - 2):
|
|
# For internal usage only (the polymorphic_shapes of VJP) we need to
|
|
# parse polynomials.
|
|
self.assertEqual((dim_poly,), shape_poly._parse_spec(dim_spec, (2,)))
|
|
self.assertEqual((dim_poly,), shape_poly._parse_spec(str(dim_poly), (2,)))
|
|
|
|
def test_dim_vars(self):
|
|
a, b, a1 = shape_poly._parse_spec("a, b, a", (2, 3, 2))
|
|
self.assertEqual(True, a == a)
|
|
self.assertEqual(True, a == a1)
|
|
self.assertEqual(False, a != a)
|
|
|
|
self.assertFalse(a == b)
|
|
self.assertTrue(a != b)
|
|
|
|
self.assertLen({a, a}, 1)
|
|
self.assertLen({a, b}, 2)
|
|
self.assertIn(a, {a, b})
|
|
self.assertIn(b, {a, b})
|
|
self.assertIn(a, [a, b])
|
|
self.assertIn(b, [a, b])
|
|
|
|
def test_get_vars(self):
|
|
a, b = shape_poly._parse_spec("a, b", (2, 3))
|
|
|
|
self.assertEqual({"a"}, a.get_vars())
|
|
self.assertEqual({"a", "b"}, (a * b * a).get_vars())
|
|
|
|
def test_evaluate(self):
|
|
a, b = shape_poly._parse_spec("a, b", (2, 3))
|
|
|
|
self.assertEqual(1, (a * a - b).evaluate(dict(a=2, b=3)))
|
|
self.assertEqual(1, ((a * a) // b).evaluate(dict(a=2, b=3)))
|
|
self.assertEqual(4, ((a * a) % b).evaluate(dict(a=5, b=7)))
|
|
|
|
def test_dim_vars_symbolic_equal(self):
|
|
a, b = shape_poly._parse_spec("a, b", (2, 3))
|
|
self.assertTrue(core.symbolic_equal_dim(a, a))
|
|
self.assertFalse(core.symbolic_equal_dim(a, 1))
|
|
self.assertFalse(core.symbolic_equal_dim(a, b))
|
|
|
|
self.assertTrue(core.symbolic_equal_one_of_dim(a, [2, a]))
|
|
self.assertFalse(core.symbolic_equal_one_of_dim(a, [2, b]))
|
|
self.assertFalse(core.symbolic_equal_one_of_dim(a, []))
|
|
|
|
self.assertTrue(core.symbolic_equal_one_of_dim(2, [a, 3, 2]))
|
|
self.assertFalse(core.symbolic_equal_one_of_dim(1, [2, b]))
|
|
self.assertFalse(core.symbolic_equal_one_of_dim(3, []))
|
|
|
|
self.assertTrue(core.symbolic_equal_dim(1, jnp.add(0, 1))) # A DeviceArray
|
|
with self.assertRaisesRegex(TypeError,
|
|
re.escape("Shapes must be 1D sequences of concrete values of integer type, got (1, 'a').")):
|
|
self.assertTrue(core.symbolic_equal_dim(1, "a"))
|
|
|
|
def test_poly_bounds(self):
|
|
a, b = shape_poly._parse_spec("a, b", (2, 3))
|
|
bounded_le4 = 5 - a
|
|
bounded_ge2 = b + 1
|
|
bounded_ge0_le4 = a % 5
|
|
self.assertEqual(a.bounds(), (1, np.PINF))
|
|
self.assertEqual(bounded_le4.bounds(), (np.NINF, 4))
|
|
self.assertEqual(bounded_ge2.bounds(), (2, np.PINF))
|
|
self.assertEqual(bounded_ge0_le4.bounds(), (0, 4))
|
|
|
|
# Additions
|
|
self.assertEqual((bounded_ge0_le4 + bounded_le4).bounds(), (np.NINF, 8))
|
|
self.assertEqual((bounded_ge0_le4 + bounded_ge2).bounds(), (2, np.PINF))
|
|
self.assertEqual((bounded_le4 + bounded_ge2).bounds(), (np.NINF, np.PINF))
|
|
|
|
# Subtractions
|
|
self.assertEqual((bounded_ge0_le4 - bounded_le4).bounds(), (-4, np.PINF))
|
|
self.assertEqual((- bounded_ge0_le4 + bounded_le4).bounds(), (np.NINF, 4))
|
|
self.assertEqual((bounded_ge0_le4 - bounded_ge2).bounds(), (np.NINF, 2))
|
|
self.assertEqual((- bounded_ge0_le4 + bounded_ge2).bounds(), (-2, np.PINF))
|
|
self.assertEqual((bounded_le4 - bounded_ge2).bounds(), (np.NINF, 2))
|
|
self.assertEqual((- bounded_le4 + bounded_ge2).bounds(), (-2, np.PINF))
|
|
|
|
# Multiplications
|
|
self.assertEqual((2 * a - 3).bounds(), (-1, np.PINF))
|
|
self.assertEqual((-2 * a - 3).bounds(), (np.NINF, -5))
|
|
self.assertEqual((3 * a * b * b + 5 * a - 7).bounds(), (1, np.PINF))
|
|
self.assertEqual((3 * a * b * b - 5 * a - 7).bounds(), (np.NINF, np.PINF))
|
|
self.assertEqual((a + b - a * b + a * b * a).bounds(), (np.NINF, np.PINF))
|
|
self.assertEqual((a + 2 * b - a).bounds(), (2, np.PINF))
|
|
self.assertEqual((a + 2 * b - a).bounds(), (2, np.PINF))
|
|
|
|
# mod
|
|
self.assertEqual(((b + 1) % 2).bounds(), (0, 1))
|
|
self.assertEqual(((b + 1) % -2).bounds(), (-1, 0))
|
|
self.assertEqual(((b - 4) % 2).bounds(), (0, 1))
|
|
self.assertEqual(((b + 1) % a).bounds(), (0, np.PINF))
|
|
self.assertEqual((11 % (a + 1)).bounds(), (0, np.PINF))
|
|
self.assertEqual((-11 % (a + 1)).bounds(), (0, np.PINF))
|
|
self.assertEqual((b % (a - 2)).bounds(), (np.NINF, np.PINF))
|
|
|
|
# floordiv
|
|
self.assertEqual(((a + 4) // 2).bounds(), (2, np.PINF))
|
|
self.assertEqual(((a + 4) // -2).bounds(), (np.NINF, -3))
|
|
self.assertEqual(((a + 5) // 2).bounds(), (3, np.PINF))
|
|
self.assertEqual(((a + 5) // -2).bounds(), (np.NINF, -3))
|
|
self.assertEqual((11 // (a + 1)).bounds(), (0, 5))
|
|
self.assertEqual((-11 // (a + 1)).bounds(), (-6, -1))
|
|
self.assertEqual((-11 // (- a)).bounds(), (0, 11)) # finite negative dividend, infinite divisor
|
|
self.assertEqual(((b + 1) // (a + 1)).bounds(), (0, np.PINF))
|
|
self.assertEqual((-b // (a + 1)).bounds(), (np.NINF, -1))
|
|
|
|
|
|
|
|
|
|
# Generate test cases for floordiv and mod: (a + N) // +-2, (N - a) // +-2
|
|
# and then evaluate them for a = 1, 5, 10000
|
|
div_mod_atoms = [
|
|
operation(op1 + n, div)
|
|
for op1 in (a, a + 10, a + 11, -a, -a + 10, -a + 11)
|
|
for n in (-3, -1, 0, 1, 3)
|
|
for div in (-2, 2, a + 4, -4 - a) # Either negative, or positive
|
|
for operation in (op.floordiv, op.mod)
|
|
]
|
|
for atom in div_mod_atoms:
|
|
lb, ub = atom.bounds()
|
|
self.assertLessEqual(lb, ub)
|
|
for a_val in (1, 5, 10000):
|
|
atom_val = atom.evaluate(dict(a=a_val))
|
|
self.assertGreaterEqual(atom_val, lb)
|
|
self.assertLessEqual(atom_val, ub)
|
|
|
|
def test_poly_equal(self):
|
|
a, b = shape_poly._parse_spec("a, b", (2, 3))
|
|
poly3 = a + 3 - a
|
|
self.assertTrue(poly3 == 3)
|
|
self.assertTrue(poly3 == np.array(3, np.int64))
|
|
self.assertTrue(poly3 == np.array(3, np.int64)[()])
|
|
self.assertFalse((poly3 + 1) == 3)
|
|
self.assertFalse(poly3 == poly3 + 1)
|
|
self.assertTrue((2 * a * b * a + 3).eq(1 + b * a * a + a * a * b + 2))
|
|
self.assertFalse((2 * a * b * a + 3).eq(a * b * a + 3))
|
|
|
|
self.assertFalse((a * b * a + 3).eq(a * b * a + 4))
|
|
self.assertFalse((2 * a * b * a).eq(a * b * a))
|
|
self.assertFalse((2 * a * b * a + 1).eq(a * b * a))
|
|
self.assertFalse((3 * a * b * a - 1).eq(a * b * a))
|
|
|
|
self.assertFalse((3 * a * b * a - 2).eq(a * b * a))
|
|
|
|
self.assertTrue(a % b == a % b)
|
|
self.assertTrue(a % b - a % b == 0)
|
|
self.assertTrue(a // b == a // b)
|
|
self.assertTrue(a // b - a // b == 0)
|
|
|
|
self.assertTrue(a % b == (2 * a // 2) % (a + b - a))
|
|
self.assertTrue(a // b == (2 * a // 2) // (a + b - a))
|
|
|
|
self.assertTrue(a, a + (a + b) // b - (b + a) // b)
|
|
|
|
# Test the normalization (a // b) * b == a - a % b
|
|
self.assertTrue((a // 2) * 2 == a - a % 2)
|
|
self.assertTrue((a // 2) + (a // 2) == a - a % 2)
|
|
self.assertTrue((a // 2) * 6 == 3 * a - 3 * (a % 2))
|
|
self.assertTrue((a // b) * b == a - a % b)
|
|
self.assertTrue(2 * (a // b) * b * b == 2 * b * a - 2 * b * (a % b))
|
|
self.assertTrue(a // (2 * b) * 2 * b == a - a % (2 * b))
|
|
|
|
def test_poly_compare(self):
|
|
a, b = shape_poly._parse_spec("a, b", (2, 3))
|
|
poly = 4 * a + b + 3
|
|
self.assertTrue(poly.ge(0))
|
|
self.assertTrue(poly.ge(8))
|
|
self.assertTrue(poly.ge(poly))
|
|
self.assertTrue(poly.ge(poly - 1))
|
|
|
|
with self.assertRaisesRegex(core.InconclusiveDimensionOperation, "inconclusive"):
|
|
poly.ge(9)
|
|
|
|
with self.assertRaisesRegex(core.InconclusiveDimensionOperation, "inconclusive"):
|
|
(4 * a - b).ge(0)
|
|
|
|
def test_poly_compare_overload(self):
|
|
a, b = shape_poly._parse_spec("a, b", (2, 3))
|
|
poly = 4 * a + b + 3
|
|
self.assertTrue(poly >= 0)
|
|
self.assertTrue(poly >= 8)
|
|
self.assertTrue(poly > 7)
|
|
self.assertTrue(poly >= poly)
|
|
self.assertTrue(poly >= poly - 1)
|
|
|
|
with self.assertRaisesRegex(core.InconclusiveDimensionOperation, "inconclusive"):
|
|
poly >= 9
|
|
|
|
with self.assertRaisesRegex(core.InconclusiveDimensionOperation, "inconclusive"):
|
|
(4 * a - b) >= 0
|
|
|
|
def test_core_greater_equal(self):
|
|
a, b = shape_poly._parse_spec("a, b", (2, 3))
|
|
self.assertTrue(core.greater_equal_dim(a, a))
|
|
self.assertTrue(core.greater_equal_dim(a, 0))
|
|
self.assertTrue(core.greater_equal_dim(a, 1))
|
|
|
|
self.assertTrue(core.greater_equal_shape((a, 2), (1, 1)))
|
|
|
|
with self.assertRaisesRegex(core.InconclusiveDimensionOperation,
|
|
"Symbolic dimension comparison .* is inconclusive"):
|
|
core.greater_equal_dim(a, 2)
|
|
|
|
with self.assertRaisesRegex(core.InconclusiveDimensionOperation,
|
|
"Symbolic dimension comparison .* is inconclusive"):
|
|
core.greater_equal_dim(a, b)
|
|
|
|
def test_poly_int_results(self):
|
|
# Whenever the result is an integer, it should be represented as an
|
|
# Python integer, not a symbolic dimension.
|
|
a, b = shape_poly._parse_spec("a, b", (2, 3))
|
|
self.assertEqual(a + 2 - a, 2)
|
|
self.assertIsInstance(a + 2 - a, int)
|
|
self.assertEqual(a + (2 - a), 2)
|
|
self.assertIsInstance(a + (2 - a), int)
|
|
self.assertEqual(a * 2 // a, 2)
|
|
self.assertIsInstance(a * 2 // a, int)
|
|
|
|
@parameterized.named_parameters(
|
|
dict(testcase_name=f"_D={dividend}_d={divisor}_q={quotient}_r={remainder}",
|
|
dividend=dividend, divisor=divisor, quotient=quotient,
|
|
remainder=remainder)
|
|
for dividend, divisor, quotient, remainder in [
|
|
(a, 1, a, 0),
|
|
(3 * a, 3, a, 0),
|
|
(3 * a + 3, 3, a + 1, 0),
|
|
(3 * a + 2, 3, a, 2),
|
|
(3 * a + 5, 3, a + 1, 2),
|
|
(3 * a - 2, 3, a - 1, 1),
|
|
(3 * a * a * b + 2 * b * b * a, a * b, 3 * a + 2 * b, 0),
|
|
(a * a - b * b, a + b, a - b, 0),
|
|
(a, b, "floordiv(a, b)", "mod(a, b)"),
|
|
(3 * a, 2, "floordiv(3*a, 2)", "mod(3*a, 2)"),
|
|
(2 * a * b + b * b, a + b, "floordiv(2*a*b + b^2, a + b)", "mod(2*a*b + b^2, a + b)"),
|
|
(3, a, "floordiv(3, a)", "mod(3, a)"),
|
|
])
|
|
def test_poly_divmod(self, *, dividend, quotient, divisor, remainder):
|
|
if isinstance(quotient, str):
|
|
d1, d2 = divmod(dividend, divisor)
|
|
self.assertEqual((quotient, remainder), (str(d1), str(d2)))
|
|
else:
|
|
self.assertEqual((quotient, remainder), divmod(dividend, divisor))
|
|
|
|
def test_dilate_shape(self):
|
|
"""0 if d == 0 else 1 + dilation * (d - 1))"""
|
|
a, = shape_poly._parse_spec("a,", (2,))
|
|
|
|
self.assertEqual((4, 7), core.dilate_shape((2, 3), (3, 3)))
|
|
self.assertEqual((0, 7), core.dilate_shape((0, 3), (3, 3)))
|
|
self.assertEqual((a, 7), core.dilate_shape((a, 3), (1, 3)))
|
|
self.assertEqual((2 * a - 1, 7), core.dilate_shape((a, 3), (2, 3)))
|
|
|
|
def test_stride_shape(self):
|
|
"""(s - window_size) // window_stride + 1"""
|
|
a, stride = shape_poly._parse_spec("a, s", (2, 3))
|
|
|
|
self.assertEqual((8, 9), core.stride_shape((10, 20), window_size=(3, 3), window_stride=(1, 2)))
|
|
self.assertEqual((a, 9), core.stride_shape((a, 20), (1, 3), (1, 2)))
|
|
|
|
self.assertEqual((a - 1, 9), core.stride_shape((a, 20), (2, 3), (1, 2)))
|
|
self.assertEqual((a + 1, 9), core.stride_shape((a * stride + 2, 20), (2, 3), (stride, 2)))
|
|
|
|
(stride0, stride1) = core.stride_shape((a, 20), (1, 3), (2, 2))
|
|
self.assertEqual("floordiv(a + -1, 2) + 1", str(stride0))
|
|
self.assertEqual(9, stride1)
|
|
|
|
|
|
class PolyHarness(Harness):
|
|
"""Tests a function with shape polymorphism.
|
|
|
|
Converts `fun` with shape polymorphism, creates a `tf.ConcreteFunction`
|
|
given `input_signature` and checks the inferred output shapes to match
|
|
`expected_output_shapes`, then checks that the JAX and the TF functions
|
|
produce the same results.
|
|
"""
|
|
def __init__(self,
|
|
group_name: str, name: str,
|
|
fun: Callable,
|
|
*,
|
|
arg_descriptors: Sequence[primitive_harness.ArgDescriptor] = (),
|
|
polymorphic_shapes: Optional[Sequence[Any]] = None,
|
|
input_signature: Optional[Sequence[tf.TensorSpec]] = None,
|
|
poly_axes: Optional[Sequence[Optional[Union[int, Sequence[int]]]]] = None,
|
|
expected_output_signature: Optional[tf.TensorSpec] = None,
|
|
enable_xla: bool = True,
|
|
expect_error: Tuple[Optional[Any], Optional[str]] = (None, None),
|
|
skip_jax_run: bool = False,
|
|
check_result: bool = True,
|
|
tol: Optional[float] = None):
|
|
"""Args:
|
|
|
|
group_name, name: The name for the harness. See `Harness.__init__`.
|
|
fun: the function to be converted, possbily after partial application to
|
|
static arguments from `arg_descriptors`. See `Harness.__init__`.
|
|
arg_descriptors: The argument descriptors. See `Harness.__init__`. May
|
|
be missing, in which case `skip_jax_run` should be `True` and
|
|
`poly_axes` cannot be used.
|
|
polymorphic_shapes: For `jax2tf.convert`. If missing, generated from
|
|
`poly_axes`.
|
|
input_signature: For `tf.function.get_concrete_function`. If missing,
|
|
generated from `poly_axes`.
|
|
poly_axes: If present, used to generate `polymorphic_shapes` and
|
|
`input_signature`. Must correspond to the non-static arguments, and for
|
|
each one it must specify which axes are polymorphic: None, or an int
|
|
(for the index of the polymorphic axis), or a tuple of ints
|
|
(for multiple polymorphic axes). For each argument, we use its
|
|
`poly_axes` entry to generate the polymorphic_shapes specification,
|
|
creating dimension variables `b0`, `b1, ..., for each of its polymorphic
|
|
axes. This means that separate arguments will share the same dimension
|
|
variable names, in the order in which the axes are listed in
|
|
`poly_axes`. We also generate the input_signature from `poly_axes`.
|
|
expected_output_signature: the expected inferred output shape.
|
|
enable_xla: For `jax2tf.convert`.
|
|
expect_error: a pair of an Exception type and a regular expression to
|
|
match the expected exception string.
|
|
skip_jax_run: If True, then neither the JAX nor the TF functions are
|
|
executed.
|
|
check_result: specifies if we want to check that the result of the shape
|
|
polymorphic conversion produces the same result and the JAX function.
|
|
tol: the tolerance to use for checking results.
|
|
"""
|
|
super().__init__(group_name, name, fun, arg_descriptors,
|
|
dtype=np.float32)
|
|
self.poly_axes = poly_axes
|
|
self.polymorphic_shapes = polymorphic_shapes
|
|
self.input_signature = input_signature
|
|
self.expected_output_signature = expected_output_signature
|
|
self.skip_jax_run = skip_jax_run
|
|
self.expect_error = expect_error
|
|
self.enable_xla = enable_xla
|
|
self.tol = tol
|
|
self.check_result = check_result
|
|
|
|
# Replicate the harness for both enable and disable xla
|
|
def both_enable_and_disable_xla(self) -> Tuple["PolyHarness", "PolyHarness"]:
|
|
assert self.enable_xla
|
|
other = PolyHarness(self.group_name,
|
|
f"{self.name}_enable_xla=False",
|
|
self.fun,
|
|
arg_descriptors=self.arg_descriptors,
|
|
poly_axes=self.poly_axes,
|
|
polymorphic_shapes=self.polymorphic_shapes,
|
|
input_signature=self.input_signature,
|
|
expected_output_signature=self.expected_output_signature,
|
|
expect_error=self.expect_error,
|
|
tol=self.tol,
|
|
enable_xla=False)
|
|
self.name = f"{self.name}_enable_xla=True"
|
|
return (self, other)
|
|
|
|
def run_test(self, tst: tf_test_util.JaxToTfTestCase):
|
|
# Make polymorphic_shapes and input_signature from poly_axes.
|
|
if self.poly_axes is None:
|
|
polymorphic_shapes = self.polymorphic_shapes
|
|
input_signature = self.input_signature
|
|
assert input_signature is not None
|
|
if not self.skip_jax_run:
|
|
args = self.dyn_args_maker(tst.rng())
|
|
|
|
else:
|
|
assert isinstance(self.poly_axes, Sequence)
|
|
# Make poly_axes: Sequence[Sequence[int]], one top-level element for each argument
|
|
poly_axes = tuple(map(lambda pa: pa if isinstance(pa, Sequence) or pa is None else (pa,),
|
|
self.poly_axes))
|
|
args = self.dyn_args_maker(tst.rng())
|
|
|
|
assert self.polymorphic_shapes is None
|
|
assert self.input_signature is None
|
|
assert args is not None and len(args) == len(poly_axes)
|
|
# Make the polymorphic_shapes and input_signature
|
|
polymorphic_shapes = []
|
|
input_signature = []
|
|
for arg, poly_axis in zip(args, poly_axes):
|
|
if poly_axis is None:
|
|
polymorphic_shapes.append(None)
|
|
input_signature.append(tf.TensorSpec(np.shape(arg), arg.dtype))
|
|
else:
|
|
def make_arg_polymorphic_shapes(poly_axis: Sequence[int]) -> Tuple[str, tf.TensorSpec]:
|
|
idx = -1
|
|
dims = []
|
|
tensorspec_dims: List[Optional[int]] = []
|
|
for i, d in enumerate(arg.shape):
|
|
if i in poly_axis:
|
|
idx += 1
|
|
dims.append(f"b{idx}")
|
|
tensorspec_dims.append(None)
|
|
else:
|
|
dims.append(str(d))
|
|
tensorspec_dims.append(d)
|
|
return ", ".join(dims), tf.TensorSpec(tensorspec_dims, arg.dtype)
|
|
|
|
arg_polymorphic_shapes, arg_tensorspec = make_arg_polymorphic_shapes(poly_axis)
|
|
polymorphic_shapes.append(arg_polymorphic_shapes)
|
|
input_signature.append(arg_tensorspec)
|
|
|
|
expect_error_type, expect_error_regex = self.expect_error
|
|
if self.skip_jax_run and self.arg_descriptors == ():
|
|
f_jax = self.fun
|
|
else:
|
|
f_jax = self.dyn_fun
|
|
|
|
with contextlib.ExitStack() as stack:
|
|
if expect_error_type is not None:
|
|
stack.enter_context(tst.assertRaisesRegex(expect_error_type, expect_error_regex))
|
|
|
|
f_tf = jax2tf.convert(f_jax, polymorphic_shapes=polymorphic_shapes,
|
|
enable_xla=self.enable_xla)
|
|
# Run in tf.Eager mode first, because it is friendlier to debuggers
|
|
res_tf = f_tf(*args) if not self.skip_jax_run else None
|
|
f_tf_func = tf.function(
|
|
f_tf, autograph=False, input_signature=input_signature)
|
|
# Create tf.ConcreteFunction and check inferred output signature
|
|
concrete_f_tf = f_tf_func.get_concrete_function(*input_signature)
|
|
|
|
if expect_error_type is not None:
|
|
return
|
|
|
|
if self.expected_output_signature:
|
|
# Strangely, output_shapes can be a single shape for a function with a
|
|
# single result, or a list/tuple of shapes.
|
|
expected_output_signature = self.expected_output_signature
|
|
concrete_output_tf_shape = concrete_f_tf.output_shapes
|
|
if not isinstance(concrete_output_tf_shape, (tuple, list)): # Single result
|
|
assert not isinstance(self.expected_output_signature, (tuple, list))
|
|
expected_output_signature = [self.expected_output_signature]
|
|
concrete_output_tf_shape = [concrete_output_tf_shape]
|
|
for expected, found in util.safe_zip(expected_output_signature,
|
|
concrete_output_tf_shape):
|
|
tst.assertEqual(tuple(expected.shape), tuple(found))
|
|
|
|
# Run the JAX and the TF functions and compare the results
|
|
if not self.skip_jax_run:
|
|
res_jax = f_jax(*args)
|
|
if self.check_result:
|
|
tst.assertAllClose(res_jax, res_tf, atol=self.tol, rtol=self.tol)
|
|
|
|
|
|
def check_shape_poly(tst, f_jax: Callable, *,
|
|
arg_descriptors: Sequence[primitive_harness.ArgDescriptor] = (),
|
|
skip_jax_run: bool = False,
|
|
poly_axes = None,
|
|
polymorphic_shapes: Optional[Sequence[Any]] = None,
|
|
input_signature: Optional[Sequence[tf.TensorSpec]] = None,
|
|
expected_output_signature: Optional[tf.TensorSpec] = None,
|
|
expect_error=(None, None)):
|
|
# Makes and tests a harness. See PolyHarness documentation.
|
|
h = PolyHarness("", "", f_jax,
|
|
arg_descriptors=arg_descriptors,
|
|
skip_jax_run=skip_jax_run, poly_axes=poly_axes,
|
|
polymorphic_shapes=polymorphic_shapes,
|
|
input_signature=input_signature,
|
|
expected_output_signature=expected_output_signature,
|
|
expect_error=expect_error)
|
|
h.run_test(tst)
|
|
|
|
|
|
class ShapePolyTest(tf_test_util.JaxToTfTestCase):
|
|
|
|
def test_simple_unary(self):
|
|
"""Test shape polymorphism for a simple case, unary function."""
|
|
|
|
def f_jax(x):
|
|
return x + jnp.sin(x)
|
|
|
|
check_shape_poly(self,
|
|
f_jax,
|
|
arg_descriptors=[RandArg((2, 3), _f32)],
|
|
input_signature=[tf.TensorSpec([2, 3])],
|
|
polymorphic_shapes=None,
|
|
expected_output_signature=tf.TensorSpec([2, 3]))
|
|
|
|
check_shape_poly(self,
|
|
f_jax,
|
|
arg_descriptors=[RandArg((2, 3), _f32)],
|
|
input_signature=[tf.TensorSpec([2, None])],
|
|
polymorphic_shapes=["_, h"],
|
|
expected_output_signature=tf.TensorSpec([2, None]))
|
|
|
|
check_shape_poly(self,
|
|
f_jax,
|
|
arg_descriptors=[RandArg((3, 3), _f32)],
|
|
input_signature=[tf.TensorSpec([None, None])],
|
|
polymorphic_shapes=["h, h"],
|
|
expected_output_signature=tf.TensorSpec([None, None]))
|
|
|
|
check_shape_poly(self,
|
|
f_jax,
|
|
arg_descriptors=[RandArg((3, 3), _f32)],
|
|
input_signature=[tf.TensorSpec([None, None])],
|
|
polymorphic_shapes="h, h",
|
|
expected_output_signature=tf.TensorSpec([None, None]))
|
|
|
|
def test_simple_binary(self):
|
|
"""Test shape polymorphism for a simple case, binary function."""
|
|
|
|
def f_jax(x, y):
|
|
return x + jnp.sin(y)
|
|
|
|
check_shape_poly(self,
|
|
f_jax,
|
|
arg_descriptors=[RandArg((2, 3), _f32), RandArg((2, 3), _f32)],
|
|
input_signature=[tf.TensorSpec([2, 3]), tf.TensorSpec([2, 3])],
|
|
polymorphic_shapes=None,
|
|
expected_output_signature=tf.TensorSpec([2, 3]))
|
|
|
|
check_shape_poly(self,
|
|
f_jax,
|
|
arg_descriptors=[RandArg((2, 3), _f32), RandArg((2, 3), _f32)],
|
|
input_signature=[tf.TensorSpec([2, None]), tf.TensorSpec([2, 3])],
|
|
polymorphic_shapes="_, h",
|
|
expected_output_signature=(
|
|
# for native serialization we cannot refine the inferred shape of the
|
|
# output if the input is more specific than polymorphic_shapes.
|
|
tf.TensorSpec([2, 3]) if not config.jax2tf_default_native_serialization
|
|
else tf.TensorSpec([2, None])))
|
|
|
|
check_shape_poly(self,
|
|
f_jax,
|
|
arg_descriptors=[RandArg((3, 3), _f32), RandArg((3, 3), _f32)],
|
|
input_signature=[tf.TensorSpec([None, None]), tf.TensorSpec([None, None])],
|
|
polymorphic_shapes=PS("h", "h"),
|
|
expected_output_signature=tf.TensorSpec([None, None]))
|
|
|
|
def test_arange(self):
|
|
def f_jax(x):
|
|
return x + jnp.arange(x.shape[0], dtype=np.float32)
|
|
x = np.ones((3,), dtype=np.float32)
|
|
self.assertAllClose(jax2tf.convert(f_jax, polymorphic_shapes="b")(x),
|
|
f_jax(x))
|
|
|
|
def test_argmax(self):
|
|
def f_jax(x): # x: f32[b, 4, 5]
|
|
return lax.argmax(x, axis=1, index_dtype=np.int32)
|
|
x = np.arange(3 * 4 * 5, dtype=np.float32).reshape((3, 4, 5))
|
|
self.assertAllClose(jax2tf.convert(f_jax, polymorphic_shapes="(b, _, _)")(x),
|
|
f_jax(x))
|
|
|
|
@parameterized.named_parameters([
|
|
dict(testcase_name=f"_expr={name}", expr=expr)
|
|
for name, expr in [
|
|
("d + 2", lambda d: d + 2),
|
|
("2 - d", lambda d: 2 - d),
|
|
("d * 2", lambda d: d * 2),
|
|
("d * d", lambda d: d * d),
|
|
("(- d) * d", lambda d: (- d) * d),
|
|
("d * d - d", lambda d: d * d - d),
|
|
# Division
|
|
("d // 2", lambda d: d // 2),
|
|
("(d + 1) // 2", lambda d: (d + 1) // 2),
|
|
("d // -2", lambda d: d // -2),
|
|
("(d + 1) // -2", lambda d: (d + 1) // -2),
|
|
("(-d) // 2", lambda d: (-d) // 2),
|
|
("(-d - 1) // 2", lambda d: (-d - 1) // 2),
|
|
("(-d) // -2", lambda d: (-d) // -2),
|
|
("(-d - 1) // -2", lambda d: (-d - 1) // -2),
|
|
# Remainder
|
|
("d % 2", lambda d: d % 2),
|
|
("(d + 1) % 2", lambda d: (d + 1) % 2),
|
|
("d % -2", lambda d: d % -2),
|
|
("(d + 1) % -2", lambda d: (d + 1) % -2),
|
|
("(-d) % 2", lambda d: (-d) % 2),
|
|
("(-d - 1) % 2", lambda d: (-d - 1) % 2),
|
|
("(-d) % -2", lambda d: (-d) % -2),
|
|
("(-d - 1) % -2", lambda d: (-d - 1) % -2),
|
|
]
|
|
])
|
|
def test_non_trivial_dim_expr(self, expr=lambda d: d % -2):
|
|
# Check the lowering for shape expressions
|
|
check_shape_poly(
|
|
self,
|
|
lambda x: x[0] * 0 + expr(x.shape[0]),
|
|
arg_descriptors=[RandArg((3,), np.int64)],
|
|
poly_axes=[0])
|
|
|
|
def test_static_shape_result(self):
|
|
"""The result has static shape."""
|
|
|
|
def f_jax(x):
|
|
return jnp.sum(x + jnp.sin(x), axis=0)
|
|
|
|
check_shape_poly(self,
|
|
f_jax,
|
|
arg_descriptors=[RandArg((2, 3), _f32)],
|
|
input_signature=[tf.TensorSpec([2, 3])],
|
|
polymorphic_shapes=None,
|
|
expected_output_signature=tf.TensorSpec([3]))
|
|
|
|
check_shape_poly(self,
|
|
f_jax,
|
|
arg_descriptors=[RandArg((2, 3), _f32)],
|
|
input_signature=[tf.TensorSpec([None, 3])],
|
|
polymorphic_shapes="b, _",
|
|
expected_output_signature=tf.TensorSpec([3]))
|
|
|
|
def test_forgot_polymorphic_shapes_error(self):
|
|
msg_re = "polymorphic shape None in axis .* must contain a dimension variable for unknown dimension in argument shape .*. Perhaps you forgot to add the polymorphic_shapes"
|
|
with self.assertRaisesRegex(ValueError, msg_re):
|
|
check_shape_poly(self,
|
|
jnp.sin,
|
|
arg_descriptors=[RandArg((1, 3,), _f32)],
|
|
input_signature=[tf.TensorSpec([1, None])],
|
|
polymorphic_shapes=None)
|
|
|
|
def test_kwargs(self):
|
|
"""Test shape polymorphism for a function with kwargs."""
|
|
|
|
x = np.ones(3, dtype=np.float32)
|
|
y = np.ones(1, dtype=np.float32)
|
|
def f_jax(x, *, y):
|
|
return x + jnp.sin(y)
|
|
|
|
f_tf: Callable[..., Any] = jax2tf.convert(f_jax, polymorphic_shapes=["b, ..."])
|
|
self.assertAllClose(f_jax(x, y=y), f_tf(x, y=y))
|
|
|
|
def test_arg_avals(self):
|
|
"""Test conversion of actual arguments to abstract values."""
|
|
|
|
def check_avals(*, arg_shapes: Sequence[Sequence[Optional[int]]],
|
|
polymorphic_shapes: Sequence[Optional[Union[str, PS]]],
|
|
expected_avals: Optional[Sequence[core.ShapedArray]] = None,
|
|
expected_shapeenv: Optional[Dict[str, int]] = None,
|
|
eager_mode: bool = False):
|
|
# Use eager mode only for when all arg_shapes are known, in order to
|
|
# check expected_shapeenv.
|
|
arg_dtypes = (_f32,) * len(arg_shapes)
|
|
def f_tf(*args_tf):
|
|
avals = tuple(map(shape_poly.arg_aval, arg_shapes, arg_dtypes, polymorphic_shapes))
|
|
dim_vars, get_dim_values_jax = shape_poly.prepare_dim_var_env(avals)
|
|
dim_values, _ = jax2tf.jax2tf._interpret_fun_jax(get_dim_values_jax,
|
|
args_tf, avals, "")
|
|
if expected_avals is not None:
|
|
self.assertEqual(expected_avals, avals)
|
|
return dict(zip(dim_vars, dim_values))
|
|
if eager_mode:
|
|
# If we want to check the shape_env then all arg_shapes must be known
|
|
assert all(all(d is not None for d in a_s)
|
|
for a_s in arg_shapes)
|
|
shape_env = f_tf(*[tf.ones(a_s, dtype=_f32) for a_s in arg_shapes])
|
|
if expected_shapeenv is not None:
|
|
for v, val in expected_shapeenv.items():
|
|
self.assertEqual(val, shape_env.get(v))
|
|
else:
|
|
f_tf = tf.function(autograph=False)(f_tf)
|
|
f_tf.get_concrete_function(*[tf.TensorSpec(a_s, _f32)
|
|
for a_s in arg_shapes])
|
|
assert not expected_shapeenv, "Should use eager_mode=True"
|
|
|
|
def shaped_array(shape_spec: str, actual_shape: core.Shape):
|
|
return core.ShapedArray(
|
|
shape_poly._parse_spec(shape_spec, actual_shape), np.float32)
|
|
|
|
# Known shapes for the arguments
|
|
check_avals(
|
|
arg_shapes=[(2, 3)],
|
|
polymorphic_shapes=[None],
|
|
expected_avals=(shaped_array("2, 3", [2, 3]),))
|
|
|
|
check_avals(
|
|
arg_shapes=[(2, 3)],
|
|
polymorphic_shapes=["(2, 3)"],
|
|
expected_avals=(shaped_array("2, 3", [2, 3]),))
|
|
|
|
check_avals(
|
|
arg_shapes=[(2, 3)],
|
|
polymorphic_shapes=["(_, 3)"],
|
|
expected_avals=(shaped_array("2, 3", [2, 3]),))
|
|
|
|
check_avals(
|
|
arg_shapes=[(2, 3)],
|
|
polymorphic_shapes=[PS("_", 3)],
|
|
expected_avals=(shaped_array("2, 3", [2, 3]),))
|
|
|
|
check_avals(
|
|
arg_shapes=[(2, 3)],
|
|
polymorphic_shapes=["..."],
|
|
expected_avals=(shaped_array("2, 3", [2, 3]),))
|
|
|
|
check_avals(
|
|
arg_shapes=[(2, 3)],
|
|
polymorphic_shapes=[PS(...)],
|
|
expected_avals=(shaped_array("2, 3", [2, 3]),))
|
|
|
|
# Partially known shapes for the arguments
|
|
check_avals(
|
|
arg_shapes=[(None, 3)],
|
|
polymorphic_shapes=[PS("b", ...)],
|
|
expected_avals=(shaped_array("(b, 3)", (2, 3)),))
|
|
|
|
check_avals(
|
|
arg_shapes=[(None, None)],
|
|
polymorphic_shapes=["h, h"],
|
|
expected_avals=(shaped_array("(h, h)", (2, 2)),))
|
|
|
|
check_avals(
|
|
arg_shapes=[(2, None)],
|
|
polymorphic_shapes=["h, h"],
|
|
expected_avals=(shaped_array("(h, h)", (2, 2)),))
|
|
|
|
check_avals(
|
|
arg_shapes=[(None, 3, 4)],
|
|
polymorphic_shapes=["(c, b, a)"],
|
|
expected_avals=(shaped_array("(c, b, a)", (2, 3, 4)),),
|
|
)
|
|
|
|
# Check cases when the specifications are polynomials
|
|
check_avals(
|
|
arg_shapes=[(2, 3)],
|
|
polymorphic_shapes=[PS("a + 1", "b + 2")],
|
|
eager_mode=True,
|
|
expected_shapeenv=dict(a=1, b=1))
|
|
|
|
check_avals(
|
|
arg_shapes=[(7, 5)],
|
|
polymorphic_shapes=[PS("2 * a + b", "b + 2")],
|
|
eager_mode=True,
|
|
expected_shapeenv=dict(a=2, b=3))
|
|
|
|
check_avals(
|
|
arg_shapes=[(7, 11, 4)],
|
|
polymorphic_shapes=[PS("2 * a + b", "b * b + 2", "b + 1")],
|
|
eager_mode=True,
|
|
expected_shapeenv=dict(a=2, b=3))
|
|
|
|
check_avals(
|
|
arg_shapes=[(7, 11, 19, 7)],
|
|
polymorphic_shapes=[PS("2 * a + b", "b * b + 2", "b + c * c", "2 * c + -1")],
|
|
eager_mode=True,
|
|
expected_shapeenv=dict(a=2, b=3, c=4))
|
|
|
|
with self.assertRaisesRegex(ValueError,
|
|
"Cannot solve for values of dimension variables {'b'}"):
|
|
check_avals(
|
|
arg_shapes=[(4, 36, 3)],
|
|
polymorphic_shapes=[PS("b * b", "b * d * d", "d")])
|
|
|
|
with self.assertRaisesRegex(ValueError,
|
|
"Dimension variable b must have integer value >= 1"):
|
|
check_avals(
|
|
arg_shapes=[(5, 36)],
|
|
polymorphic_shapes=[PS("3 * b", ...)],
|
|
eager_mode=True)
|
|
|
|
with self.assertRaisesRegex(ValueError,
|
|
"Dimension variable b must have integer value >= 1"):
|
|
check_avals(
|
|
arg_shapes=[(10, 3)],
|
|
polymorphic_shapes=[PS("3 * b + 10", ...)],
|
|
eager_mode=True)
|
|
|
|
with self.assertRaisesRegex(ValueError,
|
|
"Dimension variable b must have integer value >= 1"):
|
|
check_avals(
|
|
arg_shapes=[(7, 3)],
|
|
polymorphic_shapes=[PS("3 * b + 10", ...)],
|
|
eager_mode=True)
|
|
|
|
|
|
for invalid_syntax in [")(", "2a", "a@", "a - 2", "'a'", "('a', ...)"]:
|
|
with self.assertRaisesRegex(ValueError,
|
|
re.escape("has invalid syntax")):
|
|
check_avals(
|
|
arg_shapes=[(2,)], polymorphic_shapes=[invalid_syntax])
|
|
|
|
for invalid_syntax in [5.0, ["a list"], ("a tuple",), re.compile(".")]:
|
|
with self.assertRaisesRegex(ValueError,
|
|
re.escape("Invalid polymorphic shape element")):
|
|
check_avals(
|
|
arg_shapes=[(2,)], polymorphic_shapes=[PS([invalid_syntax])])
|
|
|
|
with self.assertRaisesRegex(
|
|
ValueError,
|
|
re.escape("polymorphic shape '..., 3' can contain Ellipsis only at the end.")):
|
|
check_avals(
|
|
arg_shapes=[(2, 3)],
|
|
polymorphic_shapes=["..., 3"])
|
|
|
|
with self.assertRaisesRegex(
|
|
ValueError,
|
|
re.escape(
|
|
"polymorphic shape '2, 3, 4, ...' of rank 3 must match the rank 2 of argument shape (2, 3).")
|
|
):
|
|
check_avals(
|
|
arg_shapes=[(2, 3)],
|
|
polymorphic_shapes=["2, 3, 4, ..."])
|
|
|
|
with self.assertRaisesRegex(
|
|
ValueError,
|
|
re.escape(
|
|
"polymorphic shape (Ellipsis, 3) can contain Ellipsis only at the end.")):
|
|
check_avals(
|
|
arg_shapes=[(2, 3)],
|
|
polymorphic_shapes=[PS(..., 3)])
|
|
|
|
with self.assertRaisesRegex(
|
|
ValueError,
|
|
re.escape(
|
|
"polymorphic shape None in axis 1 must contain a dimension variable for unknown dimension in argument shape (2, None)"
|
|
)):
|
|
check_avals(
|
|
arg_shapes=[(2, None)],
|
|
polymorphic_shapes=[None])
|
|
|
|
with self.assertRaisesRegex(
|
|
ValueError,
|
|
re.escape("polymorphic shape '()' of rank 0 must match the rank 2 of argument shape (2, 3)")):
|
|
check_avals(
|
|
arg_shapes=[(2, 3)], polymorphic_shapes=["()"])
|
|
|
|
with self.assertRaisesRegex(
|
|
ValueError,
|
|
re.escape(
|
|
"polymorphic shape '(_, _)' in axis 1 must contain a dimension variable "
|
|
"for unknown dimension in argument shape (2, None)"
|
|
)):
|
|
check_avals(
|
|
arg_shapes=[(2, None)],
|
|
polymorphic_shapes=["(_, _)"])
|
|
|
|
with self.assertRaisesRegex(
|
|
ValueError,
|
|
re.escape(
|
|
"polymorphic shape '(2, 13)' in axis 1 must match the known dimension size 3 "
|
|
"for argument shape (2, 3)"
|
|
)):
|
|
check_avals(
|
|
arg_shapes=[(2, 3)],
|
|
polymorphic_shapes=["(2, 13)"])
|
|
|
|
with self.assertRaisesRegex(
|
|
ValueError,
|
|
re.escape(
|
|
"polymorphic shape '(2, 3)' in axis 1 must contain a dimension variable for "
|
|
"unknown dimension in argument shape (2, None)"
|
|
)):
|
|
check_avals(
|
|
arg_shapes=[(2, None)],
|
|
polymorphic_shapes=["(2, 3)"])
|
|
|
|
with self.assertRaisesRegex(
|
|
ValueError,
|
|
"Found inconsistency when solving.*"):
|
|
check_avals(
|
|
arg_shapes=[(2, 3)],
|
|
polymorphic_shapes=["(a, a)"],
|
|
eager_mode=True)
|
|
|
|
# Same error across multiple arguments
|
|
with self.assertRaisesRegex(
|
|
ValueError,
|
|
"Found inconsistency when solving.*"):
|
|
check_avals(
|
|
arg_shapes=[(2, 3), (5,)],
|
|
polymorphic_shapes=["a, ...", "a"],
|
|
eager_mode=True)
|
|
|
|
def test_pytree(self):
|
|
"""Arguments and polymorphic_shapes are pytrees."""
|
|
|
|
# Arguments are of the form [([x00, x01], [x10]), dict(a=ya, b=yb)]
|
|
def add_all_jax(x_pair_of_list, y_dict):
|
|
x_list_0, x_list_1 = x_pair_of_list
|
|
return functools.reduce(op.add,
|
|
x_list_0 + x_list_1 + [y_dict["a"], y_dict["b"]])
|
|
|
|
check_shape_poly(self,
|
|
add_all_jax,
|
|
skip_jax_run=True,
|
|
input_signature=[([tf.TensorSpec([None]), tf.TensorSpec([None])],
|
|
[tf.TensorSpec([None])]),
|
|
dict(a=tf.TensorSpec([None]),
|
|
b=tf.TensorSpec([None]))],
|
|
polymorphic_shapes=[(["v", "v"], ["v"]),
|
|
dict(a="v", b="v")],
|
|
expected_output_signature=tf.TensorSpec([None]))
|
|
|
|
# Now partial polymorphic_shapes; the parts of the polymorphic_shapes that
|
|
# are not specified must have full input_signatures.
|
|
check_shape_poly(self,
|
|
add_all_jax,
|
|
skip_jax_run=True,
|
|
input_signature=[([tf.TensorSpec([4]), tf.TensorSpec([4])], [tf.TensorSpec([4])]),
|
|
dict(a=tf.TensorSpec([4]), b=tf.TensorSpec([4]))],
|
|
polymorphic_shapes=[(["(4,)", "(_,)"], [("4,")]),
|
|
dict(a="(_,)", b="(4,)")],
|
|
expected_output_signature=tf.TensorSpec([4]))
|
|
|
|
def test_with_nested_jit(self):
|
|
def f_jax(x): # x: f32[w, h]
|
|
# x + (np.sin(x) + np.broadcast_to(np.arange(x.shape[1]), x.shape))
|
|
return jnp.sin(x) + jnp.arange(x.shape[1], dtype=x.dtype)
|
|
check_shape_poly(self,
|
|
lambda x: x + jax.jit(f_jax)(x),
|
|
arg_descriptors=[RandArg((3, 4), _f32)],
|
|
poly_axes=[(0, 1)])
|
|
|
|
def test_non_trivial_polynomials_spec(self):
|
|
if config.jax_dynamic_shapes:
|
|
raise unittest.SkipTest("--jax_dynamic_shapes supports only trivial polynomials")
|
|
# We can handle non-trivial polynomials in the input shape,
|
|
# as long as all variables also occur in trivial polynoamials
|
|
check_shape_poly(self,
|
|
lambda x, y: x + y.reshape((-1,)),
|
|
arg_descriptors=[RandArg((9,), _f32), RandArg((3, 3), _f32)],
|
|
input_signature=[tf.TensorSpec([None]), tf.TensorSpec([None, None])],
|
|
polymorphic_shapes=["b * b", "b, b"])
|
|
|
|
def test_unused_args(self):
|
|
# Tests with functions that do not use their inputs.
|
|
|
|
# First arg unused, not polymorphic
|
|
check_shape_poly(self,
|
|
lambda x_unused, y: y * 2.0,
|
|
arg_descriptors=[RandArg((2, 3), _f32), RandArg((3,), _f32)],
|
|
input_signature=[tf.TensorSpec([]), tf.TensorSpec([None])],
|
|
polymorphic_shapes=[None, "b"])
|
|
|
|
# Some args unused, not polymorphic
|
|
check_shape_poly(self,
|
|
lambda x_unused, y, z_unused, w: jnp.concatenate([y, w]),
|
|
arg_descriptors=[RandArg((3,), _f32), RandArg((4,), _f32),
|
|
RandArg((5,), _f32), RandArg((6,), _f32)],
|
|
input_signature=[tf.TensorSpec([]), tf.TensorSpec([None]),
|
|
tf.TensorSpec([]), tf.TensorSpec([None])],
|
|
polymorphic_shapes=[None, "b1", None, "b2"])
|
|
|
|
# A polymorphic arg is not used, but the dimension var appears
|
|
# in a used arg also
|
|
check_shape_poly(self,
|
|
lambda x_unused, y: y * 2.0,
|
|
arg_descriptors=[RandArg((3,), _f32), RandArg((3,), _f32)],
|
|
input_signature=[tf.TensorSpec([None]), tf.TensorSpec([None])],
|
|
polymorphic_shapes=["b", "b"])
|
|
|
|
# A polymorphic arg is not used, and the dimension var does not appear
|
|
# elsewhere.
|
|
check_shape_poly(self,
|
|
lambda x_unused, y: y * 2.0,
|
|
arg_descriptors=[RandArg((4,), _f32), RandArg((3,), _f32)],
|
|
input_signature=[tf.TensorSpec([None]), tf.TensorSpec([None])],
|
|
polymorphic_shapes=["b1", "b2"])
|
|
|
|
# A polymorphic arg is not used, and the dimension var does appear
|
|
# elsewhere but not as a trivial monomial.
|
|
check_shape_poly(self,
|
|
lambda x_unused, y: y * 2.0,
|
|
arg_descriptors=[RandArg((3,), _f32), RandArg((9,), _f32)],
|
|
input_signature=[tf.TensorSpec([None]), tf.TensorSpec([None])],
|
|
polymorphic_shapes=["b1", "b1 * b1"])
|
|
|
|
# It is not sufficient to just use the shape of an input; it is still unused
|
|
check_shape_poly(self,
|
|
lambda x_unused, y: y + x_unused.shape[0],
|
|
arg_descriptors=[RandArg((3,), _f32), RandArg((9,), _f32)],
|
|
input_signature=[tf.TensorSpec([None]), tf.TensorSpec([None])],
|
|
polymorphic_shapes=["b1", "b2"])
|
|
|
|
def test_with_custom_vjp(self):
|
|
"""Shape-polymorphic custom VJP."""
|
|
|
|
@jax.custom_vjp
|
|
def f(x):
|
|
# x: [b1, b2, d1, d2] (a batch of matrices)
|
|
# res: [b1, b2, d1, d1]
|
|
return jnp.matmul(x, jnp.transpose(x, axes=(0, 1, 3, 2)))
|
|
|
|
# f_fwd: a -> (b, residual)
|
|
def f_fwd(x):
|
|
# x: [b1, b2, d1, d2]
|
|
# b: [b1, b2, d1, d1]
|
|
# res: [b1, b2, d1, d1]
|
|
# residual: [b1, b2, d1, d2]
|
|
return f(x), 3. * x
|
|
|
|
# f_bwd: (residual, CT b) -> [CT a]
|
|
def f_bwd(residual, ct_b):
|
|
# residual: [b1, b2, d1, d2]
|
|
# ct_b: [b1, b2, d1, d1]
|
|
# ct_a: [b1, b2, d1, d2]
|
|
return jnp.matmul(ct_b, residual),
|
|
|
|
f.defvjp(f_fwd, f_bwd)
|
|
x = np.ones((2, 3, 4, 5), dtype=np.float32)
|
|
res_jax = f(x)
|
|
res_jax_grad = jax.grad(lambda x: jnp.sum(f(x)))(x)
|
|
|
|
f_tf = jax2tf.convert(f, polymorphic_shapes=["(batch1, batch2, d1, d2)"])
|
|
self.assertAllClose(res_jax, f_tf(x))
|
|
|
|
xv = tf.Variable(x, dtype=np.float32)
|
|
|
|
def tf_value_and_grad(xv):
|
|
with tf.GradientTape() as tape:
|
|
tape.watch(xv)
|
|
res_tf = f_tf(xv)
|
|
res_tf_grad = tape.gradient(res_tf, xv)
|
|
return res_tf, res_tf_grad
|
|
|
|
res_tf, res_tf_grad = tf_value_and_grad(xv)
|
|
self.assertAllClose(res_jax, res_tf)
|
|
self.assertAllClose(res_jax_grad, res_tf_grad)
|
|
|
|
# Now use TF tracing for the gradient
|
|
tf_grad = tf.function(
|
|
tf_value_and_grad, autograph=False).get_concrete_function(
|
|
tf.TensorSpec([3, 4, 8, 9]))
|
|
|
|
# for native serialization we cannot refine the inferred shape of the
|
|
# output if the input is more specific than polymorphic_shapes.
|
|
if config.jax2tf_default_native_serialization:
|
|
self.assertEqual((None, None, None, None), tuple(tf_grad.output_shapes[0]))
|
|
self.assertEqual((None, None, None, None), tuple(tf_grad.output_shapes[1]))
|
|
else:
|
|
self.assertEqual((3, 4, 8, 8), tuple(tf_grad.output_shapes[0]))
|
|
self.assertEqual((3, 4, 8, 9), tuple(tf_grad.output_shapes[1]))
|
|
|
|
def test_gradients_pytree(self):
|
|
"""Shape polymorphism with gradients and pytrees for inputs and outputs."""
|
|
|
|
def f(x):
|
|
# x: dict(x=[b, 3, 4])
|
|
# res: dict(res=[b, 3, 4])
|
|
return dict(res=x["x"] * 2.)
|
|
|
|
check_shape_poly(self,
|
|
f,
|
|
skip_jax_run=True,
|
|
input_signature=[dict(x=tf.TensorSpec([None, 3, 4]))],
|
|
polymorphic_shapes=[dict(x=("b, 3, 4"))])
|
|
|
|
f_tf = jax2tf.convert(f, polymorphic_shapes=[dict(x=("b, 3, 4"))])
|
|
x = dict(x=np.ones((2, 3, 4), dtype=np.float32))
|
|
xv = tf.Variable(x["x"], dtype=np.float32)
|
|
|
|
def tf_value_and_grad(xv):
|
|
# xv: [b, 3, 4]
|
|
# res_value: dict(res=[b, 3, 4])
|
|
# res_grad: dict(grad=[b, 3, 4])
|
|
with tf.GradientTape() as tape:
|
|
tape.watch(xv)
|
|
res_tf = f_tf(dict(x=xv))
|
|
res_tf_grad = tape.gradient(res_tf, xv)
|
|
return res_tf, dict(grad=res_tf_grad)
|
|
|
|
res_tf, res_tf_grad = tf_value_and_grad(xv)
|
|
# Now use TF tracing for the gradient
|
|
tf_grad = tf.function(
|
|
tf_value_and_grad,
|
|
autograph=False).get_concrete_function(tf.TensorSpec([None, 3, 4]))
|
|
# The shape of the value
|
|
self.assertEqual((None, 3, 4), tuple(tf_grad.output_shapes[0]["res"]))
|
|
# The shape of the gradient should match the input
|
|
self.assertEqual((None, 3, 4), tuple(tf_grad.output_shapes[1]["grad"]))
|
|
|
|
def test_grad_not_var_output(self):
|
|
# Output of the function has poly shapes, non-variable
|
|
if config.jax2tf_default_native_serialization:
|
|
raise unittest.SkipTest("Not supported with native serialization")
|
|
def f_jax(x): # :[b, 3]
|
|
return jnp.reshape(x, (-1,)) # : [3b]
|
|
x = np.arange(12, dtype=np.float32).reshape((4, 3))
|
|
xv = tf.Variable(x)
|
|
|
|
f_tf = jax2tf.convert(f_jax, with_gradient=True,
|
|
polymorphic_shapes=["b, ..."])
|
|
|
|
with tf.GradientTape() as tape:
|
|
res_tf = f_tf(xv)
|
|
grad_tf = tape.gradient(res_tf, xv)
|
|
self.assertAllClose(np.ones(x.shape, dtype=np.float32), grad_tf.numpy())
|
|
|
|
|
|
def test_cond(self):
|
|
# Test the primitive under conditional
|
|
def f(x, y):
|
|
# x: f32[B, H], y : f32[H]
|
|
return lax.cond(
|
|
jnp.sum(x) > 0.,
|
|
lambda _: x + y,
|
|
lambda _: jnp.zeros_like(x),
|
|
operand=None)
|
|
|
|
x = np.ones((2, 3))
|
|
y = np.ones((3,))
|
|
res_jax = f(x, y)
|
|
self.assertAllClose(
|
|
res_jax,
|
|
jax2tf.convert(f, polymorphic_shapes=["(b, h)", "h"])(x, y))
|
|
|
|
def test_while(self):
|
|
def f(x):
|
|
# x: f32[B], iter: i32
|
|
return lax.while_loop(lambda x_iter: x_iter[1] < 5,
|
|
lambda x_iter: (x_iter[0] + jnp.arange(x_iter[0].shape[0], dtype=np.float32), x_iter[1] + 1),
|
|
(x, 0))
|
|
|
|
x = np.ones((3,), dtype=np.float32)
|
|
res_tf = jax2tf.convert(f, polymorphic_shapes=["(b,)"])(x)
|
|
self.assertAllClose(f(x), res_tf)
|
|
|
|
@jtu.sample_product(with_function=[False, True])
|
|
def test_grad_int(self, with_function=True):
|
|
# https://github.com/google/jax/issues/7093
|
|
# Also issue #6975.
|
|
x_shape = (2, 3, 4)
|
|
xi = np.arange(np.prod(x_shape), dtype=np.int16).reshape(x_shape)
|
|
yf = xi.astype(np.float32)
|
|
xi_yf = (xi, yf)
|
|
zb = np.array([True, False], dtype=np.bool_)
|
|
def f_jax(xi_yf, zb): # xi: s16[2, 3, 4], yf: f32[2, 3, 4], zb: bool[2]
|
|
xi, yf = xi_yf
|
|
# Return a tuple:
|
|
# (1) float constant, with 0 tangent;
|
|
# (2) a tuple with:
|
|
# (2.1) the integer input;
|
|
# (2.2) the boolean input;
|
|
# (2.3) a float depending on both inputs.
|
|
# TODO: there is a problem if we add a None output
|
|
return (jnp.zeros(xi.shape, dtype=jnp.float32),
|
|
(xi, zb, xi.astype(np.float32) * 2. * yf))
|
|
|
|
args = (xi_yf, zb)
|
|
|
|
f_tf = jax2tf.convert(f_jax, polymorphic_shapes=[("b1, b2, 4", "b1, b2, 4"), "b1"])
|
|
if with_function:
|
|
f_tf = tf.function(f_tf, autograph=False)
|
|
|
|
res_tf, g_tf = tf_test_util.ComputeTfValueAndGrad(f_tf, args)
|
|
self.assertAllClose(g_tf[0][0], np.zeros_like(xi))
|
|
self.assertAllClose(g_tf[0][1], (xi * 2).astype(yf.dtype))
|
|
self.assertAllClose(g_tf[1], np.zeros_like(zb))
|
|
|
|
def test_prng(self):
|
|
# The PRNG implementation uses opaque types, test shape polymorphism
|
|
try:
|
|
prev_custom_prng = config.jax_enable_custom_prng
|
|
config.update("jax_enable_custom_prng", True)
|
|
|
|
def f_jax(x): # x: f32[b1, b2]
|
|
key = random.PRNGKey(123) # key
|
|
# Exercise key operations that have custom lowering rules
|
|
broadcast_keys = lax.broadcast_in_dim(key, x.shape, ()) # key[b1, b2]
|
|
gather_keys = lax.broadcast_in_dim(broadcast_keys[0], (1, x.shape[1]), (1,)) # : key[1, b2]
|
|
slice_keys1 = lax.slice(broadcast_keys, (0, 0), (1, x.shape[1]), (1, 1)) # key[1, b2]
|
|
slice_keys2 = lax.dynamic_slice(broadcast_keys, (0, 0), slice_sizes=(1, x.shape[1])) # key[1, b2]
|
|
upd1 = lax.dynamic_update_slice(slice_keys2, slice_keys1, start_indices=(0, 0)) # key[1, b2]
|
|
_ = lax.dynamic_update_slice(upd1, gather_keys, start_indices=(0, 0))
|
|
return x
|
|
|
|
check_shape_poly(self, f_jax,
|
|
arg_descriptors=[RandArg((3, 4), _f32)],
|
|
input_signature=[tf.TensorSpec([None, None], dtype=tf.float32)],
|
|
polymorphic_shapes=["b1, b2"])
|
|
finally:
|
|
config.update("jax_enable_custom_prng", prev_custom_prng)
|
|
|
|
|
|
def test_saved_model(self):
|
|
f_jax = jnp.sin
|
|
f_tf = jax2tf.convert(f_jax, polymorphic_shapes=["(b, ...)"])
|
|
x = np.array([0.7, 0.8], dtype=np.float32)
|
|
restored_f, _ = tf_test_util.SaveAndLoadFunction(
|
|
f_tf, input_signature=[tf.TensorSpec([None], x.dtype)])
|
|
self.assertAllClose(f_jax(x), restored_f(x))
|
|
# Ensure that restored_f works at other batch size as well
|
|
y = np.concatenate([x, x])
|
|
self.assertAllClose(f_jax(y), restored_f(y))
|
|
|
|
def test_saved_model_int_function(self):
|
|
# TODO(https://github.com/google/jax/issues/14437)
|
|
if config.jax2tf_default_native_serialization:
|
|
raise unittest.SkipTest("Gradient function does not use the dimension variables")
|
|
|
|
def f_jax(x): # x:s32[b, 3, 4]
|
|
return jnp.reshape(x, (-1,)) # : s32[b * 12]
|
|
f_tf = jax2tf.convert(f_jax, polymorphic_shapes=["(b, ...)"])
|
|
f_tf = tf.function(f_tf, autograph=False)
|
|
x_shape = (2, 3, 4)
|
|
x = np.arange(np.prod(x_shape), dtype=np.int32).reshape(x_shape)
|
|
|
|
# When saving the model with gradients, we trace the gradient function
|
|
# and we used to get an error when creating zeros_like_aval for a
|
|
# polymorphic shape
|
|
restored_f, _ = tf_test_util.SaveAndLoadFunction(
|
|
f_tf, input_signature=[tf.TensorSpec((None,) + x.shape[1:], x.dtype)])
|
|
f_jax_rt = jax2tf.call_tf(restored_f)
|
|
res_jax_rt = f_jax_rt(x)
|
|
self.assertAllClose(f_jax(x), res_jax_rt)
|
|
|
|
def test_saved_model_constant_gradient(self):
|
|
# TODO(https://github.com/google/jax/issues/14437)
|
|
if config.jax2tf_default_native_serialization:
|
|
raise unittest.SkipTest("Gradient function does not use the dimension variables")
|
|
|
|
def f_jax(x): # A function whose gradient is a constant
|
|
return x
|
|
|
|
f_tf = jax2tf.convert(f_jax, polymorphic_shapes=["(b, ...)"])
|
|
x = np.array([0.7, 0.8], dtype=np.float32)
|
|
restored_f, _ = tf_test_util.SaveAndLoadFunction(
|
|
f_tf, input_signature=[tf.TensorSpec([None], x.dtype)])
|
|
self.assertAllClose(f_jax(x), restored_f(x))
|
|
|
|
|
|
def test_readme_examples(self):
|
|
"""Some of the examples from the README."""
|
|
|
|
jax2tf.convert(lambda x: jnp.reshape(x, (x.shape[0] * x.shape[1],)),
|
|
polymorphic_shapes=["(b, 4)"])(np.ones((3, 4)))
|
|
|
|
jax2tf.convert(lambda x: jnp.reshape(x, (np.prod(x.shape),)),
|
|
polymorphic_shapes=["(b, 4)"])(np.ones((3, 4)))
|
|
|
|
jax2tf.convert(lambda x: x + x.shape[0] + jnp.sin(x.shape[0]),
|
|
polymorphic_shapes=["b"])(np.ones(3))
|
|
|
|
jax2tf.convert(lambda x: jnp.sum(x, axis=0) / x.shape[0],
|
|
polymorphic_shapes=["(v, _)"])(np.ones((3, 4)))
|
|
|
|
with self.assertRaisesRegex(TypeError,
|
|
"prod requires ndarray or scalar arguments"):
|
|
jax2tf.convert(lambda x: jnp.prod(x.shape) + x,
|
|
polymorphic_shapes=["(b, 4)"])(np.ones((3, 4)))
|
|
|
|
jax2tf.convert(lambda x: jnp.prod(jnp.array(x.shape)) + x,
|
|
polymorphic_shapes=["(b, 4)"])(np.ones((3, 4)))
|
|
|
|
four_ones = np.ones((4,))
|
|
with self.assertRaisesRegex(
|
|
TypeError,
|
|
re.escape("add got incompatible shapes for broadcasting: (v,), (4,)")):
|
|
jax2tf.convert(lambda x, y: x + y,
|
|
polymorphic_shapes=["(v,)", "(4,)"])(four_ones, four_ones)
|
|
|
|
# We get the error even if we use correct actual arguments
|
|
with self.assertRaisesRegex(
|
|
TypeError,
|
|
re.escape("add got incompatible shapes for broadcasting: (v,), (4,)")):
|
|
jax2tf.convert(
|
|
lambda x, y: x + y, polymorphic_shapes=["(v,)", "(4,)"])(four_ones,
|
|
four_ones)
|
|
|
|
with self.assertRaisesRegex(TypeError,
|
|
re.escape("dot_general requires contracting dimensions to have the same shape, got (4,) and (v,)")):
|
|
jax2tf.convert(lambda x: jnp.matmul(x, x),
|
|
polymorphic_shapes=["(v, 4)"])(np.ones((4, 4)))
|
|
|
|
with self.assertRaisesRegex(core.InconclusiveDimensionOperation,
|
|
re.compile("Cannot divide evenly the sizes of shapes \\(b, 5, 7\\) and \\(2, -1\\)",
|
|
re.DOTALL)):
|
|
jax2tf.convert(lambda x: jnp.reshape(x, (2, -1)),
|
|
polymorphic_shapes=["(b, _, _)"])(np.ones((4, 5, 7)))
|
|
|
|
jax2tf.convert(lambda x: jnp.reshape(x, (2, -1)),
|
|
polymorphic_shapes=["(b, _, _)"])(np.ones((4, 5, 6)))
|
|
jax2tf.convert(lambda x: jnp.reshape(x, (-1, x.shape[0])),
|
|
polymorphic_shapes=["(b1, b2, ...)"])(np.ones((4, 5, 6)))
|
|
|
|
if not config.jax2tf_default_native_serialization:
|
|
# Does not support 2*b constraints
|
|
jax2tf.convert(lambda x: jnp.reshape(x, (2, -1)),
|
|
polymorphic_shapes=["(2*b, ...)"])(np.ones((4, 5, 7)))
|
|
|
|
with self.assertRaisesRegex(
|
|
core.InconclusiveDimensionOperation,
|
|
re.escape("Symbolic dimension comparison 'a + 1' >= 'b' is inconclusive")):
|
|
jax2tf.convert(lambda x: 0 if x.shape[0] + 1 >= x.shape[1] else 1,
|
|
polymorphic_shapes=["(a, b)"])(np.ones((4, 4)))
|
|
|
|
# Unsoundness: not checking that the dimension variable is 0
|
|
def f1_jax(x): # f32[b]
|
|
# We have to use "x"
|
|
return jnp.concatenate([x, jnp.array([0. if x.shape[0] == 0 else 1.],
|
|
dtype=np.float32)])
|
|
|
|
x0 = np.array([], np.float32)
|
|
# JAX with static shapes sees that the x.shape[0] == 0
|
|
self.assertEqual(jnp.array([0.], dtype=np.float32), f1_jax(x0))
|
|
|
|
# jax2tf catches the broken assumption b >= 1 if the converted function is executed
|
|
# eagerly.
|
|
with self.assertRaisesRegex(
|
|
ValueError,
|
|
"Dimension variable b must have integer value >= 1. Found value 0 when solving .*"):
|
|
jax2tf.convert(f1_jax, polymorphic_shapes=["b"],
|
|
native_serialization=False)(x0)
|
|
|
|
# TODO(https://github.com/google/jax/issues/14437)
|
|
# In native serialization, or if we trace to a TF graph, we miss this
|
|
res1_tf = jax2tf.convert(f1_jax, polymorphic_shapes=["b"],
|
|
native_serialization=True)(x0)
|
|
self.assertEqual(jnp.array([1.], dtype=np.float32), res1_tf)
|
|
|
|
f1_tf = tf.function(
|
|
jax2tf.convert(f1_jax, polymorphic_shapes=["b"],
|
|
native_serialization=False)
|
|
).get_concrete_function(tf.TensorSpec([None], dtype=np.float32))
|
|
self.assertEqual(jnp.array([1.], dtype=np.float32), f1_tf(x0))
|
|
|
|
# Unsoundness: not checking that the actual dimensions denoted by the same
|
|
# dimension variables have equal sizes.
|
|
def f2_jax(x): # f32[b, b]
|
|
# We have to use "x"
|
|
return jnp.sum(x) + (0. if x.shape[0] != x.shape[1] else 1.)
|
|
|
|
x45 = np.ones((4, 5), dtype=np.float32)
|
|
# JAX with static shapes sees that x.shape[0] != x.shape[1]
|
|
self.assertEqual(jnp.sum(x45), f2_jax(x45))
|
|
|
|
# jax2tf catches the broken assumption b >= 1 if the converted function is executed
|
|
# eagerly.
|
|
with self.assertRaisesRegex(ValueError,
|
|
"Found inconsistency when solving b == .*"):
|
|
jax2tf.convert(f2_jax, polymorphic_shapes=["b, b"],
|
|
native_serialization=False)(x45)
|
|
|
|
# TODO(https://github.com/google/jax/issues/14437)
|
|
# In native serialization, or if we trace to a TF graph, we miss this
|
|
res2_tf = jax2tf.convert(f2_jax, polymorphic_shapes=["b, b"],
|
|
native_serialization=True)(x45)
|
|
self.assertEqual(1. + jnp.sum(x45), res2_tf)
|
|
|
|
f2_tf = tf.function(
|
|
jax2tf.convert(f2_jax, polymorphic_shapes=["b, b"],
|
|
native_serialization=False)
|
|
).get_concrete_function(tf.TensorSpec([None, None], dtype=np.float32))
|
|
self.assertEqual(1. + jnp.sum(x45), f2_tf(x45))
|
|
|
|
x = np.ones((5,), dtype=np.float32)
|
|
with self.assertRaisesRegex(
|
|
ValueError,
|
|
"Cannot solve for values of dimension variables"):
|
|
jax2tf.convert(lambda x: jnp.sum(x), polymorphic_shapes=["a + b"],
|
|
native_serialization=False)(x)
|
|
with self.assertRaisesRegex(
|
|
ValueError,
|
|
"dimension variables cannot be computed from the static shapes of the array arguments"):
|
|
jax2tf.convert(lambda x: jnp.sum(x), polymorphic_shapes=["a + b"],
|
|
native_serialization=True)(x)
|
|
|
|
def test_dynamic_shapes(self):
|
|
# Test dim_as_value with dynamic shapes.
|
|
def f(x):
|
|
return jnp.sum(x, axis=0) * x.shape[0]
|
|
|
|
x = np.arange(3.)
|
|
self.assertAllClose(9., jax2tf.convert(f, polymorphic_shapes=["(b,)"])(x))
|
|
self.assertAllClose(
|
|
9.,
|
|
jax2tf.convert(jax.jit(f), polymorphic_shapes=["(b,)"])(x))
|
|
self.assertAllClose(
|
|
9.,
|
|
tf.function(jax2tf.convert(f, polymorphic_shapes=["(b,)"]))(x))
|
|
|
|
res_primal, res_tangent = jax2tf.convert(
|
|
lambda x, xt: jax.jvp(f, (x,), (xt,)),
|
|
polymorphic_shapes=["b", "b"])(x, np.array([0.1, 0.2, 0.3]))
|
|
self.assertAllClose((9., 1.8), (res_primal, res_tangent))
|
|
|
|
# TODO(https://github.com/google/jax/issues/14437)
|
|
if not config.jax2tf_default_native_serialization:
|
|
self.assertAllClose(
|
|
np.array([3., 3., 3.]),
|
|
jax2tf.convert(jax.grad(f), polymorphic_shapes=["b"])(x))
|
|
|
|
xv = np.arange(24.).reshape((2, 3, 4))
|
|
res_vmap = jax.vmap(f, in_axes=1)(xv)
|
|
# Implement by iteration
|
|
res_iter = jnp.stack([f(xv[:, i, :]) for i in range(xv.shape[1])])
|
|
self.assertAllClose(res_iter, res_vmap)
|
|
|
|
res_vmap_tf = jax2tf.convert(jax.vmap(f, in_axes=1),
|
|
polymorphic_shapes=["b1, b2, ..."])(xv)
|
|
self.assertAllClose(res_iter, res_vmap_tf.numpy())
|
|
|
|
def test_with_hash_collision_vmap(self):
|
|
# Batching caches based on Jaxpr, and Jaxpr include _DimExpr. If we have
|
|
# a collision for the hashing of a _DimExpr, then Python will call the
|
|
# equality, which will raise InconclusiveDimensionOperation.
|
|
|
|
def f_jax(x):
|
|
return jnp.reshape(x, (2, -1,))
|
|
try:
|
|
# Override the hashing to create collisions
|
|
orig_hash = getattr(shape_poly._DimExpr, "__hash__")
|
|
def collision_hash(obj):
|
|
return hash(5)
|
|
|
|
setattr(shape_poly._DimExpr, "__hash__", collision_hash)
|
|
xs = np.ones((3, 5, 6), dtype=np.float32)
|
|
f_toconvert = jax.vmap(pjit.pjit(f_jax))
|
|
res_1 = jax2tf.convert(f_toconvert)(xs)
|
|
res_2 = jax2tf.convert(f_toconvert,
|
|
polymorphic_shapes = "b1, b2, ...")(xs)
|
|
self.assertAllClose(res_1, res_2)
|
|
finally:
|
|
setattr(shape_poly._DimExpr, "__hash__", orig_hash)
|
|
|
|
@parameterized.named_parameters([
|
|
dict(testcase_name=f"_{op_name}",
|
|
op=op)
|
|
for op, op_name in [
|
|
(jnp.array, "array"),
|
|
(jnp.sin, "sin"),
|
|
(lambda x: x, "id"),
|
|
(core.dimension_as_value, "dimension_as_value"),
|
|
]
|
|
])
|
|
def test_poly_unary_op(self, *, op=jnp.array):
|
|
def f_jax(x): # x: f32[b]
|
|
poly = 2 * x.shape[0]
|
|
return (op(poly), x) # Make sure we are using x
|
|
|
|
check_shape_poly(self,
|
|
f_jax,
|
|
arg_descriptors=[RandArg((3,), _f32)],
|
|
poly_axes=[0],
|
|
expected_output_signature=(tf.TensorSpec([]), tf.TensorSpec((None,), _f32)))
|
|
|
|
@parameterized.named_parameters([
|
|
dict(testcase_name=f"_{op.__name__}_other={other}:{type(other)}{'_other_jnp_array' if other_jnp_array else ''}{'_swap' if swap else ''}",
|
|
op=op, other=other,
|
|
other_jnp_array=other_jnp_array, swap=swap)
|
|
for op in [op.add, op.mul, op.sub,
|
|
op.mod, op.floordiv, op.truediv]
|
|
for other in [
|
|
2, np.int32(2), 2., np.float32(2),
|
|
np.array(2, dtype=np.int32), np.arange(1, 5, dtype=np.int32),
|
|
np.array(2., dtype=np.float32), np.arange(1., 7., dtype=np.float32)
|
|
]
|
|
for other_jnp_array in (
|
|
[True, False] if np.shape(other) == (7,) else [False]) # type: ignore
|
|
for swap in [False, True] # The poly is the left op by default
|
|
])
|
|
def test_poly_binary_op(self, *, op=op.add,
|
|
other=np.arange(2, dtype=np.int32),
|
|
other_jnp_array=False,
|
|
swap=True):
|
|
# Test arithmetic operations with poly and a variety of other operand types
|
|
def f_jax(x): # x: f32[b]
|
|
poly = 2 * x.shape[0] # This will allow divisions with 2
|
|
other_wrapped = jnp.array(other) if other_jnp_array else other
|
|
ops = (poly, other_wrapped) if not swap else (other_wrapped, poly)
|
|
res = op(*ops)
|
|
|
|
# If the other op is an integer then the result is a symbolic dim
|
|
try:
|
|
op.index(other)
|
|
other_isint = True
|
|
except Exception:
|
|
other_isint = False
|
|
|
|
if (hasattr(poly, "dimension_as_value") and
|
|
other_isint and
|
|
op.__name__ != "truediv"):
|
|
# If we running under jax2tf and "other" is an integer the result
|
|
# should be a symbolic dimension
|
|
self.assertTrue(isinstance(res, int) or hasattr(res, "dimension_as_value"))
|
|
|
|
if config.jax_enable_x64:
|
|
# Outside jax2tf, x.shape[0] is a Python (64-bit) integer and for most
|
|
# operations here JAX is not involved at all because the other operand
|
|
# is a Python or NumPy constant. So the result will be 64-bits. But under
|
|
# jax2tf, x.shape[0] is rewritten to jnp.array(x.shape[0]) which when
|
|
# used with int32 or float32 values will produce 32-bit values.
|
|
return (lax.convert_element_type(res, np.float32), x)
|
|
return (res, x) # Make sure we are using x
|
|
|
|
check_shape_poly(self,
|
|
f_jax,
|
|
arg_descriptors=[RandArg((3,), np.int32)],
|
|
poly_axes=[0])
|
|
|
|
def test_mean0(self):
|
|
def f_jax(x): # x: f32[b, 4]
|
|
return jnp.sum(x, axis=0) / x.shape[0]
|
|
check_shape_poly(self,
|
|
f_jax,
|
|
arg_descriptors=[RandArg((3, 4), _f32)],
|
|
poly_axes=[0],
|
|
expected_output_signature=tf.TensorSpec([4]))
|
|
|
|
|
|
def test_shape_as_array(self):
|
|
def f_jax(x):
|
|
# The entire x.shape is passed to jnp.array
|
|
return x + jnp.sum(jnp.array(x.shape)).astype(np.int32)
|
|
|
|
check_shape_poly(self,
|
|
f_jax,
|
|
arg_descriptors=[RandArg((3, 4), _f32)],
|
|
poly_axes=[0])
|
|
|
|
def test_dim_as_value_weak_type(self):
|
|
def f_jax(x): # x: f32[b]
|
|
d0 = jnp.array(x.shape[0]) # in JAX should have weak_type=True
|
|
if isinstance(d0, core.Tracer):
|
|
self.assertTrue(d0.aval.weak_type), d0
|
|
|
|
# And an implicit conversion to array
|
|
d1 = x.shape[0] + jnp.array(4)
|
|
if isinstance(d1, core.Tracer):
|
|
self.assertTrue(d1.aval.weak_type), d1
|
|
return d0 + np.array(5., dtype=np.float32) + d1 + x[0]
|
|
|
|
with numpy_dtype_promotion("strict"):
|
|
# strict type promotion is sensitive to weak_types
|
|
check_shape_poly(self,
|
|
f_jax,
|
|
arg_descriptors=[RandArg((3,), _f32)],
|
|
poly_axes=[0])
|
|
|
|
def test_vmap_while(self):
|
|
def cond_func(x): # x: f32[3]
|
|
return jnp.sum(x) >= 0.
|
|
def body_func(x): # x: f32[3]
|
|
return x - 1.
|
|
def f_jax(x):
|
|
return lax.while_loop(cond_func, body_func, x)
|
|
|
|
check_shape_poly(self,
|
|
jax.vmap(f_jax),
|
|
arg_descriptors=[RandArg((3,), _f32)],
|
|
input_signature=[tf.TensorSpec((None, 3), dtype=tf.float32)],
|
|
polymorphic_shapes=["b, ..."],
|
|
expected_output_signature=tf.TensorSpec((None, 3), dtype=tf.float32)
|
|
)
|
|
|
|
def test_vmap_error(self):
|
|
# vmap is careful to give nice error messages when mapped axes have
|
|
# different sizes, but this can be foiled by InconsistentDimensionOperation
|
|
x = y = np.ones((3, 5), dtype=np.float32)
|
|
with self.assertRaisesRegex(ValueError,
|
|
"vmap got inconsistent sizes for array axes to be mapped"):
|
|
jax2tf.convert(jax.vmap(lambda x, y: x + y),
|
|
polymorphic_shapes=["b, ...", None])(x, y)
|
|
|
|
z = x
|
|
with self.assertRaisesRegex(ValueError,
|
|
"vmap got inconsistent sizes for array axes to be mapped"):
|
|
jax2tf.convert(jax.vmap(lambda x, y, z: x + y + z),
|
|
polymorphic_shapes=["b, ...", "c, ...", None])(x, y, z)
|
|
|
|
def test_reshape_compiled(self):
|
|
# We compile the result of conversion for two shapes, hence we need to
|
|
# involve the TF compiler twice, but we trace only once with shape polymorphism
|
|
traced = False
|
|
|
|
def f_jax(x):
|
|
nonlocal traced
|
|
traced = True
|
|
y = jnp.sin(x)
|
|
return y.reshape([x.shape[0], -1])
|
|
|
|
x = self.rng().rand(4, 2, 3)
|
|
res_jax = f_jax(x)
|
|
|
|
traced = False
|
|
# If we get_concrete_function we trace once
|
|
f_tf = tf.function(
|
|
jax2tf.convert(f_jax, polymorphic_shapes=[PS("b", ...)]),
|
|
autograph=False,
|
|
jit_compile=True).get_concrete_function(
|
|
tf.TensorSpec([None, 2, 3], x.dtype))
|
|
self.assertTrue(traced)
|
|
traced = False
|
|
self.assertAllClose(res_jax, f_tf(x))
|
|
self.assertFalse(traced) # We are not tracing again
|
|
|
|
x = self.rng().rand(6, 2, 3)
|
|
res_jax = f_jax(x)
|
|
traced = False
|
|
|
|
self.assertAllClose(res_jax, f_tf(x))
|
|
self.assertFalse(traced) # We are not tracing again
|
|
|
|
|
|
# List containing either harnesses, or lists of harnesses
|
|
_POLY_SHAPE_TEST_HARNESSES = [
|
|
PolyHarness("add", "",
|
|
jnp.add,
|
|
arg_descriptors=[RandArg((3, 4), _f32), RandArg((2, 3, 4), _f32)],
|
|
poly_axes=[0, 1]),
|
|
PolyHarness("add_transpose", "",
|
|
jax.grad(lambda x: jnp.sum(jnp.sum(x, axis=0, keepdims=False) + jnp.sin(x))),
|
|
arg_descriptors=[RandArg((3, 4), _f32)],
|
|
poly_axes=[0]),
|
|
PolyHarness("arange", "start",
|
|
lambda op: jnp.arange(2 * op.shape[0], dtype=_f32) + op[0],
|
|
arg_descriptors=[RandArg((3,), _f32)],
|
|
poly_axes=[0]).both_enable_and_disable_xla(),
|
|
PolyHarness("arange", "start_no_dtype",
|
|
lambda op: jnp.arange(op.shape[0]) + op[0],
|
|
arg_descriptors=[RandArg((3,), _f32)],
|
|
poly_axes=[0]),
|
|
PolyHarness("arange", "error1",
|
|
lambda op: jnp.arange(op.shape[0], 10),
|
|
arg_descriptors=[RandArg((3,), _f32)],
|
|
poly_axes=[0],
|
|
expect_error=(ValueError, "jax.numpy.arange supports non-constant arguments only in single-argument form")),
|
|
PolyHarness("arange", "error2",
|
|
lambda op: jnp.arange(1, op.shape[0]),
|
|
arg_descriptors=[RandArg((3,), _f32)],
|
|
poly_axes=[0],
|
|
expect_error=(ValueError, "jax.numpy.arange supports non-constant arguments only in single-argument form")),
|
|
PolyHarness("arange", "error3",
|
|
lambda op: jnp.arange(1, 5, op.shape[0]),
|
|
arg_descriptors=[RandArg((3,), _f32)],
|
|
poly_axes=[0],
|
|
expect_error=(ValueError, "jax.numpy.arange supports non-constant arguments only in single-argument form")),
|
|
# Reduce the poly dimension
|
|
PolyHarness("argmax", "0",
|
|
lambda op: lax.argmax(op, axis=0, index_dtype=np.int32),
|
|
arg_descriptors=[RandArg((3, 4, 5), _f32)],
|
|
poly_axes=[0]).both_enable_and_disable_xla(),
|
|
# Reduce the non-poly dimension
|
|
PolyHarness("argmax", "1",
|
|
lambda op: lax.argmax(op, axis=1, index_dtype=np.int32),
|
|
arg_descriptors=[RandArg((3, 4, 5), _f32)],
|
|
poly_axes=[0]).both_enable_and_disable_xla(),
|
|
PolyHarness("jnp.argsort", "",
|
|
lambda op: jnp.argsort(op),
|
|
arg_descriptors=[RandArg((3, 4, 5), _f32)],
|
|
poly_axes=[0]),
|
|
[
|
|
PolyHarness("average",
|
|
f"{axis=}_weights=None",
|
|
lambda x, axis: jnp.average(x, axis=axis, returned=False, weights=None),
|
|
arg_descriptors=[RandArg((7, 8, 4), _f32), StaticArg(axis)],
|
|
poly_axes=[0])
|
|
for axis in [None, 0, 1]
|
|
],
|
|
[
|
|
PolyHarness("average",
|
|
f"{axis=}_weights=Some",
|
|
lambda x, weights, axis: jnp.average(x, axis=axis, returned=False, weights=weights),
|
|
arg_descriptors=[RandArg((7, 8, 4), _f32), RandArg((7, 8, 4), _f32), StaticArg(axis)],
|
|
poly_axes=[0, 0])
|
|
for axis in [None, 0, 1]
|
|
],
|
|
PolyHarness("jnp.bincount", "length=constant",
|
|
lambda x: jnp.bincount(x % 2, length=4),
|
|
arg_descriptors=[RandArg((12,), np.int32)],
|
|
poly_axes=[0]),
|
|
PolyHarness("jnp.bincount", "length=poly",
|
|
lambda x: jnp.bincount(x % 4, length=x.shape[0]),
|
|
arg_descriptors=[RandArg((12,), np.int32)],
|
|
poly_axes=[0]),
|
|
PolyHarness("broadcast_to", "",
|
|
lambda x: jnp.broadcast_to(x, [x.shape[0], x.shape[0], 4]),
|
|
arg_descriptors=[RandArg((3, 4), _f32)],
|
|
poly_axes=[0]),
|
|
PolyHarness("broadcast_in_dim", "0",
|
|
lambda x: lax.broadcast_in_dim(x, [x.shape[0], 4, 5, 6],
|
|
broadcast_dimensions=(0, 2, 3)),
|
|
arg_descriptors=[RandArg((3, 1, 6), _f32)],
|
|
poly_axes=[0]),
|
|
PolyHarness("broadcast_in_dim", "poly",
|
|
lambda x: lax.broadcast_in_dim(x, [x.shape[0], x.shape[0] + x.shape[0], 4],
|
|
broadcast_dimensions=(0, 1, 2)),
|
|
arg_descriptors=[RandArg((3, 1, 4), _f32)],
|
|
poly_axes=[0]),
|
|
PolyHarness("broadcast_in_dim", "poly2",
|
|
lambda x: lax.broadcast_in_dim(x, [x.shape[0], 5, 6, x.shape[2], 4],
|
|
broadcast_dimensions=(0, 2, 3)),
|
|
arg_descriptors=[RandArg((3, 1, 4), _f32)],
|
|
poly_axes=[(0, 2)]),
|
|
PolyHarness("broadcast_in_dim", "transpose",
|
|
jax.grad(lambda x: jnp.sum(
|
|
lax.broadcast_in_dim(jnp.sin(x), [2, x.shape[0], 5, x.shape[2], 4],
|
|
broadcast_dimensions=(1, 2, 3)))),
|
|
arg_descriptors=[RandArg((3, 1, 4), _f32)],
|
|
poly_axes=[(0, 2)]),
|
|
PolyHarness("clamp", "",
|
|
lax.clamp,
|
|
arg_descriptors=[RandArg((3, 4, 5), _f32), RandArg((3, 4, 5), _f32),
|
|
RandArg((3, 4, 5), _f32)],
|
|
poly_axes=[0, 0, 0]),
|
|
PolyHarness("collapse", "",
|
|
lambda x: lax.collapse(x, 1, 4),
|
|
arg_descriptors=[RandArg((3, 4, 5, 6, 7), _f32)],
|
|
poly_axes=[(0, 1, 3)]),
|
|
PolyHarness("concatenate", "",
|
|
lambda x: jnp.concatenate([x, x], axis=0),
|
|
arg_descriptors=[RandArg((3, 4, 5), _f32)],
|
|
poly_axes=[(0, 1)]),
|
|
PolyHarness("concatenate", "grad",
|
|
jax.grad(lambda x: jnp.sum(jnp.concatenate([x, jnp.sin(x)], axis=0))),
|
|
arg_descriptors=[RandArg((3, 4, 5), _f32)],
|
|
poly_axes=[(0, 1)]),
|
|
|
|
PolyHarness("conv_general_dilated", "1d_stride=1",
|
|
lambda lhs, rhs: lax.conv_general_dilated(
|
|
lhs, rhs,
|
|
window_strides=(1,),
|
|
padding="SAME",
|
|
rhs_dilation=None,
|
|
dimension_numbers=lax.ConvDimensionNumbers(lhs_spec=(0, 2, 1),
|
|
rhs_spec=(2, 1, 0),
|
|
out_spec=(0, 2, 1))),
|
|
arg_descriptors=[RandArg((1, 12, 16), _f32), RandArg((4, 16, 16), _f32)],
|
|
poly_axes=[1, None]).both_enable_and_disable_xla(),
|
|
# The same example from above, but with stride=2.
|
|
PolyHarness("conv_general_dilated", "1d_stride=2_even",
|
|
lambda lhs, rhs: lax.conv_general_dilated(
|
|
lhs, rhs,
|
|
window_strides=(2,),
|
|
padding="SAME",
|
|
rhs_dilation=None,
|
|
dimension_numbers=lax.ConvDimensionNumbers(lhs_spec=(0, 2, 1),
|
|
rhs_spec=(2, 1, 0),
|
|
out_spec=(0, 2, 1))),
|
|
arg_descriptors=[RandArg((1, 12, 16), _f32), RandArg((4, 16, 16), _f32)],
|
|
poly_axes=[1, None],
|
|
).both_enable_and_disable_xla(),
|
|
# The same example from above, but with stride=2 and odd input size.
|
|
PolyHarness("conv_general_dilated", "1d_stride=2_odd",
|
|
lambda lhs, rhs: lax.conv_general_dilated(
|
|
lhs, rhs,
|
|
window_strides=(2,),
|
|
padding="SAME",
|
|
rhs_dilation=None,
|
|
dimension_numbers=lax.ConvDimensionNumbers(lhs_spec=(0, 2, 1),
|
|
rhs_spec=(2, 1, 0),
|
|
out_spec=(0, 2, 1))),
|
|
arg_descriptors=[RandArg((1, 13, 16), _f32), RandArg((4, 16, 16), _f32)],
|
|
poly_axes=[1, None],
|
|
).both_enable_and_disable_xla(),
|
|
# Issue #11402
|
|
PolyHarness("conv_general_dilated", "1d_2",
|
|
lambda lhs, rhs: lax.conv_transpose(lhs, rhs,
|
|
strides=(2,),
|
|
padding="SAME",
|
|
rhs_dilation=None,
|
|
transpose_kernel=False),
|
|
arg_descriptors=[RandArg((5, 12, 16), _f32), RandArg((4, 16, 16), _f32)],
|
|
poly_axes=[0, None],
|
|
tol=1e-5).both_enable_and_disable_xla(),
|
|
# Issue #11402
|
|
PolyHarness("conv_general_dilated", "1d_3",
|
|
lambda lhs, rhs: lax.conv_transpose(lhs, rhs,
|
|
strides=(2,),
|
|
padding="SAME",
|
|
rhs_dilation=None,
|
|
transpose_kernel=False),
|
|
arg_descriptors=[RandArg((5, 12, 16), _f32), RandArg((4, 16, 16), _f32)],
|
|
poly_axes=[1, None],
|
|
tol=1e-5).both_enable_and_disable_xla(),
|
|
PolyHarness("conv_general_dilated", "",
|
|
lambda lhs, rhs: lax.conv_general_dilated(
|
|
lhs, rhs,
|
|
window_strides=(2, 3),
|
|
padding=((0, 0), (0, 0)),
|
|
lhs_dilation=(1, 1),
|
|
rhs_dilation=(1, 2),
|
|
dimension_numbers=("NCHW", "OIHW", "NCHW"),
|
|
feature_group_count=1,
|
|
batch_group_count=1,
|
|
precision=None),
|
|
arg_descriptors=[RandArg((7, 3, 9, 10), _f32), RandArg((3, 3, 4, 5), _f32)],
|
|
poly_axes=[0, None]).both_enable_and_disable_xla(),
|
|
PolyHarness("cummax", "",
|
|
lambda x: lax_control_flow.cummax(x, axis=1, reverse=False),
|
|
arg_descriptors=[RandArg((3, 4, 5), _f32)],
|
|
poly_axes=[0]),
|
|
PolyHarness("jnp.cumsum", "reduce_axis=poly",
|
|
lambda x: jnp.cumsum(x, axis=0),
|
|
arg_descriptors=[RandArg((3, 5), _f32)],
|
|
poly_axes=[0],
|
|
expect_error=(
|
|
(None, None) if (not config.jax2tf_default_native_serialization or
|
|
jtu.device_under_test() == "tpu") else
|
|
(NotImplementedError,
|
|
"associative scan over axis of non-constant size"))),
|
|
PolyHarness("jnp.cumsum", "reduce_axis=static",
|
|
lambda x: jnp.cumsum(x, axis=1),
|
|
arg_descriptors=[RandArg((3, 5), _f32)],
|
|
poly_axes=[0]),
|
|
PolyHarness("delta", "0",
|
|
lambda x: lax_internal._delta(_f32, x.shape, axes=(0, 1)) + x,
|
|
arg_descriptors=[RandArg((3, 1), _f32)],
|
|
poly_axes=[0]),
|
|
PolyHarness("dot_general", "",
|
|
lambda lhs, rhs: lax.dot_general(lhs, rhs,
|
|
dimension_numbers=(((2,), (1,)), ((0,), (0,)))),
|
|
arg_descriptors=[RandArg((3, 4, 4), _f32), RandArg((3, 4), _f32)],
|
|
poly_axes=[0, 0]),
|
|
PolyHarness("dynamic_slice", "idx=tuple_int",
|
|
# x:shape: (b, 4)
|
|
lambda x: lax.dynamic_slice(x, (0, 1), (x.shape[0], 2)),
|
|
arg_descriptors=[RandArg((3, 4), _f32)],
|
|
poly_axes=[0]).both_enable_and_disable_xla(),
|
|
PolyHarness("dynamic_slice", "idx=tuple_arg",
|
|
# x:shape: (b, 4)
|
|
lambda x, i0: lax.dynamic_slice(x, (i0, np.int32(1)), (x.shape[0], 2)),
|
|
arg_descriptors=[RandArg((3, 4), _f32), np.array(-2, dtype=np.int32)],
|
|
poly_axes=[0, None]).both_enable_and_disable_xla(),
|
|
PolyHarness("dynamic_slice", "idx=array",
|
|
# x:shape: (b, 4)
|
|
lambda x, idx: lax.dynamic_slice(x, idx, (x.shape[0], 2)),
|
|
arg_descriptors=[RandArg((3, 4), _f32), np.array([-2, -1], dtype=np.int32)],
|
|
poly_axes=[0, None]).both_enable_and_disable_xla(),
|
|
PolyHarness("dynamic_slice_in_dim", "idx=0",
|
|
# x:shape: (b, 4)
|
|
lambda x: lax.dynamic_slice_in_dim(x, 0, x.shape[0], axis=0),
|
|
arg_descriptors=[RandArg((3, 4), _f32)],
|
|
poly_axes=[0]).both_enable_and_disable_xla(),
|
|
PolyHarness("dynamic_update_slice", "idx=tuple_int",
|
|
# x:shape: (b, 4)
|
|
lambda x: lax.dynamic_update_slice(x, x, (0, 0)),
|
|
arg_descriptors=[RandArg((3, 4), _f32)],
|
|
poly_axes=[0]).both_enable_and_disable_xla(),
|
|
PolyHarness("dynamic_update_slice", "idx=tuple_arg",
|
|
# x:shape: (b, 4)
|
|
lambda x, i0: lax.dynamic_update_slice(x, x, (i0, np.int32(0))),
|
|
arg_descriptors=[RandArg((3, 4), _f32), np.array(-2, dtype=np.int32)],
|
|
poly_axes=[0, None]).both_enable_and_disable_xla(),
|
|
PolyHarness("dynamic_update_slice", "idx=array",
|
|
# x:shape: (b, 4)
|
|
lambda x, idx: lax.dynamic_update_slice(x, x, idx),
|
|
arg_descriptors=[RandArg((3, 4), _f32), np.array([-2, -1], dtype=np.int32)],
|
|
poly_axes=[0, None]).both_enable_and_disable_xla(),
|
|
PolyHarness("einsum", "0",
|
|
lambda x: jnp.einsum("...i->...", x),
|
|
arg_descriptors=[RandArg((3, 4), _f32)],
|
|
poly_axes=[0]),
|
|
PolyHarness("einsum", "0_alt",
|
|
lambda x: jnp.einsum(x, (..., 1), [...]),
|
|
arg_descriptors=[RandArg((3, 4), _f32)],
|
|
poly_axes=[0]),
|
|
PolyHarness("einsum", "1",
|
|
lambda x, y: jnp.einsum("...ij,...jk->...ik", x, y),
|
|
arg_descriptors=[RandArg((3, 4, 5), _f32), RandArg((3, 5, 6), _f32)],
|
|
poly_axes=[0, 0]),
|
|
PolyHarness("einsum", "1_alt",
|
|
lambda x, y: jnp.einsum(x, [..., 0, 1], y, (..., 1, 2), [..., 0, 2]),
|
|
arg_descriptors=[RandArg((3, 4, 5), _f32), RandArg((3, 5, 6), _f32)],
|
|
poly_axes=[0, 0]),
|
|
PolyHarness("einsum", "2",
|
|
lambda x, y: jnp.einsum("...ij,jk->...ik", x, y),
|
|
arg_descriptors=[RandArg((3, 4, 5), _f32), RandArg((5, 6), _f32)],
|
|
poly_axes=[0, None]),
|
|
PolyHarness("einsum", "2_alt",
|
|
lambda x, y: jnp.einsum(x, [..., 0, 1], y, [1, 2], [..., 0, 2]),
|
|
arg_descriptors=[RandArg((3, 4, 5), _f32), RandArg((5, 6), _f32)],
|
|
poly_axes=[0, None]),
|
|
PolyHarness("einsum", "3",
|
|
# Reduced dimension is polymorphic
|
|
lambda x, y: jnp.einsum("ij,jk->ik", x, y),
|
|
arg_descriptors=[RandArg((3, 4), _f32), RandArg((4, 5), _f32)],
|
|
poly_axes=[1, 0]),
|
|
PolyHarness("einsum", "3_alt",
|
|
# Reduced dimension is polymorphic
|
|
lambda x, y: jnp.einsum(x, [0, 1], y, [1, 2], [0, 2]),
|
|
arg_descriptors=[RandArg((3, 4), _f32), RandArg((4, 5), _f32)],
|
|
poly_axes=[1, 0]),
|
|
PolyHarness("einsum", "4",
|
|
# Reduced dimension is polymorphic, and is 2*b
|
|
lambda x, y: jnp.einsum("ij,jk->ik",
|
|
jnp.concatenate([x, x], axis=1),
|
|
jnp.concatenate([y, y], axis=0)),
|
|
arg_descriptors=[RandArg((3, 4), _f32), RandArg((4, 5), _f32)],
|
|
poly_axes=[1, 0]),
|
|
PolyHarness("einsum", "4_alt",
|
|
# Reduced dimension is polymorphic, and is 2*b
|
|
lambda x, y: jnp.einsum(jnp.concatenate([x, x], axis=1), [0, 1],
|
|
jnp.concatenate([y, y], axis=0), [1, 2],
|
|
[0, 2]),
|
|
arg_descriptors=[RandArg((3, 4), _f32), RandArg((4, 5), _f32)],
|
|
poly_axes=[1, 0]),
|
|
PolyHarness("einsum", "multiple_contractions",
|
|
lambda x, y, z: jnp.einsum("ab,bc,cd->ad", x, y, z),
|
|
arg_descriptors=[RandArg((3, 2), _f32), RandArg((2, 3), _f32), RandArg((3, 4), _f32)],
|
|
poly_axes=[0, None, None]),
|
|
PolyHarness("einsum", "incompatible_contractions_error",
|
|
lambda x, y: jnp.einsum("ab,cb->ac", x, y),
|
|
arg_descriptors=[RandArg((2, 3), _f32), RandArg((2, 3), _f32)],
|
|
polymorphic_shapes=["(2, b0)", "(2, b1)"],
|
|
input_signature=[tf.TensorSpec((2, None)), tf.TensorSpec((2, None))],
|
|
expect_error=(AssertionError,
|
|
"Incompatible reduction dimensions")),
|
|
PolyHarness("eye", "N=poly_M=None",
|
|
lambda x: jnp.eye(x.shape[0]) + x,
|
|
arg_descriptors=[RandArg((3, 1), _f32)],
|
|
poly_axes=[0]),
|
|
PolyHarness("eye", "N=poly_M=poly",
|
|
lambda x: jnp.eye(x.shape[0], M=x.shape[0] + 2) + x,
|
|
arg_descriptors=[RandArg((3, 1), _f32)],
|
|
poly_axes=[0]),
|
|
PolyHarness("full", "",
|
|
lambda x: lax.full((x.shape[0], 2), 3.) + x,
|
|
arg_descriptors=[RandArg((3, 1), _f32)],
|
|
poly_axes=[0]),
|
|
# operand is non-poly, index is poly
|
|
PolyHarness("getitem", "op=static_idx=poly",
|
|
lambda a, i: a[i],
|
|
arg_descriptors=[RandArg((3, 4), _f32), np.array([2, 2], np.int32)],
|
|
poly_axes=[None, 0]).both_enable_and_disable_xla(),
|
|
# operand is poly, index is integer
|
|
PolyHarness("getitem", "op=poly_idx=const",
|
|
lambda a: a[1],
|
|
arg_descriptors=[RandArg((3, 4), _f32)],
|
|
poly_axes=[0]).both_enable_and_disable_xla(),
|
|
# operand is poly, index is dim poly
|
|
PolyHarness("getitem", "op=poly_idx=dim",
|
|
lambda a: a[jnp.array(a.shape[0] - 2)],
|
|
arg_descriptors=[RandArg((3, 4), _f32)],
|
|
poly_axes=[0]).both_enable_and_disable_xla(),
|
|
# Both the operand and the index are poly
|
|
PolyHarness("getitem", "op=poly_idx=poly",
|
|
lambda a, i: a[i],
|
|
arg_descriptors=[RandArg((3, 4), _f32), np.array([1, 2, 0], np.int32)],
|
|
poly_axes=[0, 0]).both_enable_and_disable_xla(),
|
|
# op is poly and index is an entire slice
|
|
PolyHarness("getitem", "op=poly_idx=slice-all",
|
|
lambda a: a[:],
|
|
arg_descriptors=[RandArg((3, 4), _f32)],
|
|
poly_axes=[0]).both_enable_and_disable_xla(),
|
|
# op is poly and index is a partial slice
|
|
PolyHarness("getitem", "op=poly_idx=slice-ct-1",
|
|
lambda a: a[:2],
|
|
arg_descriptors=[RandArg((3, 4), _f32)],
|
|
poly_axes=[0],
|
|
expect_error=(IndexError, "Cannot use NumPy slice indexing on an array dimension")
|
|
).both_enable_and_disable_xla(),
|
|
PolyHarness("getitem", "op=poly_idx=slice-ct-2",
|
|
lambda a: a[:, :2],
|
|
arg_descriptors=[RandArg((3, 4), _f32)],
|
|
poly_axes=[0]).both_enable_and_disable_xla(),
|
|
PolyHarness("getitem", "op=poly_idx=slice-None-1",
|
|
lambda a: a[:a.shape[0]],
|
|
arg_descriptors=[RandArg((3, 4), _f32)],
|
|
poly_axes=[0]).both_enable_and_disable_xla(),
|
|
PolyHarness("getitem", "op=poly_idx=slice-poly",
|
|
lambda a: a[:a.shape[0] - 1],
|
|
arg_descriptors=[RandArg((3, 4), _f32)],
|
|
poly_axes=[0],
|
|
expect_error=(IndexError, "Array slice indices must have static")).both_enable_and_disable_xla(),
|
|
PolyHarness("image_resize", "linear_0",
|
|
lambda x: jax.image.resize(x, (x.shape[0], 2 * x.shape[1], 2 * x.shape[2], x.shape[3]),
|
|
method="linear"),
|
|
arg_descriptors=[RandArg((3, 16, 32, 3), _f32)],
|
|
poly_axes=[(1, 2)]),
|
|
PolyHarness("image_resize", "linear_to_fixed_dim",
|
|
lambda x: jax.image.resize(x, (x.shape[0], 64, 64, x.shape[3]),
|
|
method="linear"),
|
|
arg_descriptors=[RandArg((3, 16, 32, 3), _f32)],
|
|
poly_axes=[(1, 2)]),
|
|
PolyHarness("image_resize", "nearest_0",
|
|
lambda x: jax.image.resize(x, (x.shape[0], 2 * x.shape[1], 2 * x.shape[2], x.shape[3]),
|
|
method="nearest"),
|
|
arg_descriptors=[RandArg((3, 5, 7, 3), _f32)],
|
|
poly_axes=[(1, 2)]),
|
|
PolyHarness("index_in_dim", "0",
|
|
lambda x: lax.index_in_dim(x, -1, axis=0, keepdims=False),
|
|
arg_descriptors=[RandArg((3, 4), _f32)],
|
|
poly_axes=[0]),
|
|
PolyHarness("index_in_dim", "idx=neg",
|
|
lambda x: lax.index_in_dim(x, -1, axis=0, keepdims=False),
|
|
arg_descriptors=[RandArg((3, 4), _f32)],
|
|
poly_axes=[0]),
|
|
PolyHarness("index_in_dim", "idx=last",
|
|
lambda x: lax.index_in_dim(x, x.shape[0] - 1, axis=0, keepdims=False),
|
|
arg_descriptors=[RandArg((3, 4), _f32)],
|
|
poly_axes=[0]),
|
|
PolyHarness("jnp.insert", "insert=constant",
|
|
lambda x: jnp.insert(x, jnp.arange(3, dtype=_i32), np.array([3, 4, 5], dtype=_i32)),
|
|
arg_descriptors=[RandArg((12,), _i32)],
|
|
poly_axes=[0],
|
|
expect_error=expect_error_associative_scan),
|
|
PolyHarness("jnp.insert", "insert=poly",
|
|
lambda x: jnp.insert(x, jnp.arange(x.shape[0], dtype=_i32), x, axis=0),
|
|
arg_descriptors=[RandArg((12, 3), _i32)],
|
|
poly_axes=[(0, 1)],
|
|
expect_error=expect_error_associative_scan),
|
|
PolyHarness("iota", "",
|
|
lambda x: x + lax.iota(_f32, x.shape[0]),
|
|
arg_descriptors=[RandArg((3,), _f32)],
|
|
poly_axes=[0]),
|
|
PolyHarness("matmul", "0",
|
|
jnp.matmul,
|
|
arg_descriptors=[RandArg((7, 8, 4), _f32), RandArg((7, 4, 5), _f32)],
|
|
poly_axes=[0, 0],
|
|
tol=1e-5),
|
|
PolyHarness("matmul", "1",
|
|
jnp.matmul,
|
|
arg_descriptors=[RandArg((7, 8, 4), _f32), RandArg((4, 5), _f32)],
|
|
poly_axes=[0, None],
|
|
tol=1e-5),
|
|
[
|
|
PolyHarness("mean",
|
|
f"{axis=}_{keepdims=}_where=None",
|
|
lambda x, axis, keepdims: jnp.mean(x, axis=axis, keepdims=keepdims, where=None),
|
|
arg_descriptors=[RandArg((7, 8, 4), _f32), StaticArg(axis), StaticArg(keepdims)],
|
|
poly_axes=[0])
|
|
for keepdims in [False, True]
|
|
for axis in [None, (0,), (0, 1), (1,)]
|
|
],
|
|
[
|
|
PolyHarness("mean",
|
|
f"{axis=}_{keepdims=}_where=Some",
|
|
lambda x, where, axis, keepdims: jnp.mean(x, axis=axis, keepdims=keepdims, where=where),
|
|
arg_descriptors=[RandArg((7, 8, 4), _f32), RandArg((7, 8, 4), np.bool_),
|
|
StaticArg(axis), StaticArg(keepdims)],
|
|
poly_axes=[0, 0])
|
|
for keepdims in [False, True]
|
|
for axis in [None, (0,), (0, 1), (1,)]
|
|
],
|
|
PolyHarness("jnp.nonzero", "size=constant",
|
|
lambda x: jnp.nonzero(x % 3, size=10, fill_value=100),
|
|
arg_descriptors=[RandArg((3, 2, 4), _i32)],
|
|
poly_axes=[0],
|
|
expect_error=expect_error_associative_scan),
|
|
PolyHarness("jnp.nonzero", "size=poly",
|
|
lambda x: jnp.nonzero(x % 3, size=x.shape[0] * 2, fill_value=100),
|
|
arg_descriptors=[RandArg((3, 2, 4), _i32)],
|
|
poly_axes=[0],
|
|
expect_error=expect_error_associative_scan),
|
|
PolyHarness("ones", "",
|
|
lambda x: jnp.ones(x.shape, dtype=_f32) + x,
|
|
arg_descriptors=[RandArg((3, 2, 4), _f32)],
|
|
poly_axes=[0]),
|
|
PolyHarness("pad", "",
|
|
lax.pad,
|
|
arg_descriptors=[RandArg((3, 2, 5), _f32), np.float32(5.),
|
|
StaticArg(((0, 0, 0), (0, 0, 0), (1, 1, 1)))],
|
|
poly_axes=[0, None]),
|
|
PolyHarness("pad", "poly_padding_config",
|
|
lambda x: lax.pad(x, _f32(0.),
|
|
((x.shape[0], x.shape[1], x.shape[0]),
|
|
(0, 0, 0))),
|
|
arg_descriptors=[RandArg((3, 2), _f32)],
|
|
poly_axes=[0]),
|
|
PolyHarness("jnp.pad", "mode=constant",
|
|
lambda x: jnp.pad(x, [[x.shape[0], 0], [x.shape[1], 1]],
|
|
mode="constant"),
|
|
arg_descriptors=[RandArg((3, 5), _f32)],
|
|
poly_axes=[0]),
|
|
PolyHarness("jnp.pad", "mode=constant_bminus1",
|
|
# We slice first the unknown dimension to make it of size b - 1
|
|
# which may be 0.
|
|
lambda x: jnp.pad(lax.dynamic_slice_in_dim(x, 1, x.shape[0] - 1,
|
|
axis=0),
|
|
[[x.shape[0], 0], [x.shape[1], 1]],
|
|
mode="constant"),
|
|
arg_descriptors=[RandArg((3, 5), _f32)],
|
|
poly_axes=[0]),
|
|
PolyHarness("jnp.pad", "mode=edge",
|
|
lambda x: jnp.pad(x, [[x.shape[0], 0], [x.shape[1], 1]],
|
|
mode="edge"),
|
|
arg_descriptors=[RandArg((3, 5), _f32)],
|
|
poly_axes=[0]),
|
|
PolyHarness("percentile", "axis=None",
|
|
lambda x: jnp.percentile(x, 50, axis=None),
|
|
arg_descriptors=[RandArg((3, 5), _f32)],
|
|
poly_axes=[0]),
|
|
PolyHarness("nanquantile", "axis=None",
|
|
lambda x: jnp.nanquantile(x, .5, axis=None),
|
|
arg_descriptors=[RandArg((3, 5), _f32)],
|
|
poly_axes=[0]),
|
|
PolyHarness("percentile", "axis=0",
|
|
lambda x: jnp.percentile(x, 50, axis=0),
|
|
arg_descriptors=[RandArg((3, 5), _f32)],
|
|
poly_axes=[0]),
|
|
PolyHarness("nanquantile", "axis=0",
|
|
lambda x: jnp.nanquantile(x, .5, axis=0),
|
|
arg_descriptors=[RandArg((3, 5), _f32)],
|
|
poly_axes=[0]),
|
|
PolyHarness("random_gamma", "",
|
|
lambda key, a: jax.random.gamma(key, a),
|
|
arg_descriptors=[RandArg((3, 2), np.uint32), RandArg((3, 3), _f32)],
|
|
poly_axes=[0, 0]),
|
|
# The known dimensions product must be even.
|
|
PolyHarness("random_categorical", "axis=0",
|
|
lambda key, a: jax.random.categorical(key, a, axis=0),
|
|
arg_descriptors=[RandArg((2,), np.uint32), RandArg((3, 8), _f32)],
|
|
poly_axes=[None, 0]),
|
|
PolyHarness("random_categorical", "axis=1",
|
|
lambda key, a: jax.random.categorical(key, a, axis=1),
|
|
arg_descriptors=[RandArg((2,), np.uint32), RandArg((3, 5, 8), _f32)],
|
|
poly_axes=[None, (0, 1)]),
|
|
PolyHarness("random_categorical", "axis=1_then_reshape",
|
|
lambda key, a: jax.random.categorical(key, a, axis=1).reshape((-1)),
|
|
arg_descriptors=[RandArg((2,), np.uint32), RandArg((3, 5, 8), _f32)],
|
|
poly_axes=[None, (0, 1)]),
|
|
PolyHarness("random_categorical", "0_dim", # One axis has 0 size
|
|
lambda key, a: jax.random.categorical(key, a, axis=1),
|
|
arg_descriptors=[RandArg((2,), np.uint32), RandArg((3, 5, 0), _f32)],
|
|
poly_axes=[None, (0, 1)]),
|
|
# Works when the known dimensions are known to be even or odd.
|
|
PolyHarness("random_uniform", "even_1",
|
|
lambda key, a: jax.random.uniform(key, a.shape, dtype=_f32) + a,
|
|
arg_descriptors=[RandArg((2,), np.uint32), RandArg((3, 4), _f32)],
|
|
poly_axes=[None, 0]),
|
|
PolyHarness("random_uniform", "even_2",
|
|
lambda key, a: jax.random.uniform(key, (2 * a.shape[0], a.shape[1]),
|
|
dtype=_f32) + jnp.concatenate([a, a], axis=0),
|
|
arg_descriptors=[RandArg((2,), np.uint32), RandArg((3, 1), _f32)],
|
|
poly_axes=[None, 0]),
|
|
PolyHarness("random_uniform", "error_not_even",
|
|
lambda key, a: jax.random.uniform(key, a.shape, dtype=_f32),
|
|
arg_descriptors=[RandArg((2,), np.uint32), RandArg((3, 5), _f32)],
|
|
poly_axes=[None, 0],
|
|
expect_error=(core.InconclusiveDimensionOperation,
|
|
"the product of the known dimensions must be even")),
|
|
PolyHarness("reduce_window", "min",
|
|
# x.shape = (b, 8)
|
|
lambda x: lax.reduce_window(x, np.array(1., _f32), lax.min,
|
|
(2, 2), (1, 1), "VALID"),
|
|
arg_descriptors=[RandArg((3, 8), _f32)],
|
|
poly_axes=[0]).both_enable_and_disable_xla(),
|
|
PolyHarness("reduce_window", "add_0",
|
|
# x.shape = (b, 8)
|
|
lambda x: lax.reduce_window(x, 0, lax.add, (2, 2), (1, 1),
|
|
"VALID"),
|
|
arg_descriptors=[RandArg((3, 8), _f32)],
|
|
poly_axes=[0]).both_enable_and_disable_xla(),
|
|
# https://github.com/google/jax/issues/11804
|
|
# Use the reshape trick to simulate a polymorphic dimension of 16*b.
|
|
# (See test "conv_general_dilated.1d_1" above for more details.)
|
|
PolyHarness("reduce_window", "add_1",
|
|
# x.shape = (1, 16*b, 1)
|
|
lambda x: lax.reduce_window(
|
|
jnp.reshape(x, (1, -1, 1)),
|
|
0., lax.add, (1, 4, 1), (1, 2, 1), "SAME"),
|
|
arg_descriptors=[RandArg((1, 128, 16), _f32)],
|
|
poly_axes=[1]).both_enable_and_disable_xla(),
|
|
# TODO(necula): not yet supported, but also unlikely to come up.
|
|
# PolyHarness("random_uniform", "odd",
|
|
# lambda key, a: jax.random.uniform(key, (2 * a.shape[0] + 1, a.shape[1]),
|
|
# dtype=_f32),
|
|
# [RandArg((2,), np.uint32), RandArg((3, 5), _f32)],
|
|
# poly_axes=[None, 0]),
|
|
[
|
|
PolyHarness("reduce", reduce_op.__name__,
|
|
lambda x: reduce_op(x, axis=-1, keepdims=True), # type: ignore
|
|
arg_descriptors=[RandArg((3, 5), _f32)],
|
|
poly_axes=[0])
|
|
for reduce_op in [jnp.all, jnp.any, jnp.max, jnp.min, jnp.prod, jnp.sum]
|
|
],
|
|
# Repeat f32[b, 2] * 3
|
|
PolyHarness("repeat", "repeats=int_axis=0",
|
|
lambda x: jnp.repeat(x, repeats=3, axis=0),
|
|
arg_descriptors=[RandArg((3, 2), _f32)],
|
|
poly_axes=[0]),
|
|
# Repeat f32[b, 2] * b
|
|
PolyHarness("repeat", "repeats=poly_axis=0",
|
|
lambda x: jnp.repeat(x, repeats=x.shape[0], axis=0),
|
|
arg_descriptors=[RandArg((3, 2), _f32)],
|
|
poly_axes=[0]),
|
|
# Repeat f32[b, 2] * b
|
|
PolyHarness("repeat", "repeats=poly_axis=None",
|
|
lambda x: jnp.repeat(x, repeats=x.shape[0], axis=None),
|
|
arg_descriptors=[RandArg((3, 2), _f32)],
|
|
poly_axes=[0]),
|
|
# Repeat f32 * b
|
|
PolyHarness("repeat", "repeats=poly_axis=None_scalar",
|
|
lambda x, y: jnp.repeat(x, repeats=y.shape[0], axis=None) + y,
|
|
arg_descriptors=[RandArg((), _f32), RandArg((3, 1), _f32)],
|
|
poly_axes=[None, 0]),
|
|
PolyHarness("repeat", "repeats=poly_axis=None_total_repeat_length1",
|
|
lambda x: jnp.repeat(x, repeats=x.shape[0], axis=None, total_repeat_length=8),
|
|
arg_descriptors=[RandArg((3, 2), _f32)],
|
|
poly_axes=[0],
|
|
expect_error=(ValueError, "jnp.repeat with a non-constant `repeats` is supported only .*")),
|
|
PolyHarness("reshape", "0",
|
|
lambda x: x.reshape([x.shape[0], -1]),
|
|
arg_descriptors=[RandArg((3, 2, 3), _f32)],
|
|
poly_axes=[0]),
|
|
PolyHarness("reshape", "1",
|
|
lambda x: x.reshape([x.shape[0], -1]),
|
|
arg_descriptors=[RandArg((3, 2, 3), _f32)],
|
|
poly_axes=[(0, 1)]),
|
|
PolyHarness("reshape", "2",
|
|
lambda x: x.reshape([x.shape[0], -1, x.shape[3], x.shape[2]]),
|
|
arg_descriptors=[RandArg((3, 4, 5, 6, 7), _f32)],
|
|
poly_axes=[(0, 2, 3)]),
|
|
PolyHarness("reshape", "3",
|
|
lambda x: jnp.reshape(x, [2, -1]),
|
|
arg_descriptors=[RandArg((3, 4, 5, 6, 7), _f32)],
|
|
poly_axes=[(0, 2)]),
|
|
PolyHarness("reshape", "_issue_9975",
|
|
# The newshape is a scalar
|
|
lambda x: jnp.reshape(x, x.shape[0] * x.shape[1]),
|
|
arg_descriptors=[RandArg((3, 4), _f32)],
|
|
poly_axes=[0]),
|
|
PolyHarness("reshape", "error",
|
|
lambda x: x.reshape([x.shape[0], -1, 3]),
|
|
arg_descriptors=[RandArg((3, 2, 4), _f32)],
|
|
poly_axes=[0],
|
|
skip_jax_run=True,
|
|
expect_error=(core.InconclusiveDimensionOperation,
|
|
re.escape(
|
|
"Cannot divide evenly the sizes of shapes (b0, 2, 4) and (b0, -1, 3)"))),
|
|
PolyHarness("roll", "axis=0",
|
|
lambda x: jnp.roll(x, 2, axis=0),
|
|
arg_descriptors=[RandArg((3, 4), _f32)],
|
|
poly_axes=[0]),
|
|
PolyHarness("roll", "axis=None",
|
|
lambda x: jnp.roll(x, 2),
|
|
arg_descriptors=[RandArg((3, 4), _f32)],
|
|
poly_axes=[0]),
|
|
PolyHarness("scatter_add", "",
|
|
partial(lax.scatter_add, indices_are_sorted=False, unique_indices=True),
|
|
arg_descriptors=[RandArg((7, 4), _f32),
|
|
np.array([[1], [2]], np.int32), # indices: [2, 1]
|
|
RandArg((7, 2), _f32), # updates: [7, 2]
|
|
StaticArg(lax.ScatterDimensionNumbers((0,), (1,), (1,)))],
|
|
poly_axes=[0, None, 0]),
|
|
PolyHarness("scatter_add", "clip0",
|
|
partial(lax.scatter_add, indices_are_sorted=False, unique_indices=True, mode=lax.GatherScatterMode.CLIP),
|
|
arg_descriptors=[RandArg((7, 4), _f32), # [b, 4]
|
|
np.array([[1], [2]], np.int32), # indices: [2, 1]
|
|
RandArg((7, 2), _f32), # updates: [b, 2]
|
|
StaticArg(lax.ScatterDimensionNumbers((0,), (1,), (1,)))],
|
|
poly_axes=[0, None, 0]),
|
|
PolyHarness("scatter_add", "clip1",
|
|
partial(lax.scatter_add, indices_are_sorted=False, unique_indices=True, mode=lax.GatherScatterMode.CLIP),
|
|
arg_descriptors=[RandArg((7, 4), _f32), # [b, 4]
|
|
np.array([[1, 2], [-2, 0], [6, 4], [7, -1], [1, 0], [3, 0], [0, 5]], np.int32), # indices: [b, 2]
|
|
RandArg((7, 1), _f32), # updates: [b, 1]
|
|
StaticArg(lax.ScatterDimensionNumbers((1,), (0,), (0, 1,)))],
|
|
poly_axes=[0, 0, 0]),
|
|
PolyHarness("select", "0",
|
|
# x.shape = (b, 3)
|
|
lambda x: lax.select(x > 5., x, x),
|
|
arg_descriptors=[RandArg((7, 3), _f32)],
|
|
poly_axes=[0]),
|
|
PolyHarness("select", "1",
|
|
# x.shape = (b, 3); y.shape = (3,)
|
|
jax.vmap(lambda x, y: lax.select(x > 5., x, y), in_axes=[0, None]),
|
|
arg_descriptors=[RandArg((7, 3), _f32), RandArg((3,), _f32)],
|
|
poly_axes=[0, None]),
|
|
PolyHarness("slice", "entire_axis",
|
|
lambda x: lax.slice(x, start_indices=(0, 1), limit_indices=(x.shape[0], 3)),
|
|
arg_descriptors=[RandArg((7, 3), _f32)],
|
|
poly_axes=[0]),
|
|
PolyHarness("slice_in_dim", "entire_axis",
|
|
lambda x: lax.slice_in_dim(x, 0, x.shape[0], stride=1, axis=0),
|
|
arg_descriptors=[RandArg((3, 4), _f32)],
|
|
poly_axes=[0]),
|
|
PolyHarness("slice_in_dim", "start=neg",
|
|
lambda x: lax.slice_in_dim(x, -1, x.shape[0], stride=1, axis=0),
|
|
arg_descriptors=[RandArg((3, 4), _f32)],
|
|
poly_axes=[0]),
|
|
PolyHarness("slice_in_dim", "limit=neg",
|
|
lambda x: lax.slice_in_dim(x, 0, -1, stride=1, axis=0),
|
|
arg_descriptors=[RandArg((3, 4), _f32)],
|
|
poly_axes=[0]),
|
|
PolyHarness("slice_in_dim", "stride=2_even",
|
|
lambda x: lax.slice_in_dim(x, 0, x.shape[0], stride=2, axis=0),
|
|
arg_descriptors=[RandArg((12, 4), _f32)],
|
|
poly_axes=[0]),
|
|
PolyHarness("slice_in_dim", "stride=2_odd",
|
|
lambda x: lax.slice_in_dim(x, 0, x.shape[0], stride=2, axis=0),
|
|
arg_descriptors=[RandArg((13, 4), _f32)],
|
|
poly_axes=[0]),
|
|
# Not yet, the slice_in_dim does int(stride)
|
|
# PolyHarness("slice_in_dim", "stride=sym",
|
|
# lambda x: lax.slice_in_dim(x, 0, x.shape[0], stride=x.shape[0] // 4, axis=0),
|
|
# arg_descriptors=[RandArg((13, 4), _f32)],
|
|
# poly_axes=[0]),
|
|
PolyHarness("squeeze", "axis=empty",
|
|
jnp.squeeze,
|
|
arg_descriptors=[RandArg((5,), _f32), StaticArg(())],
|
|
poly_axes=[0]),
|
|
PolyHarness("squeeze", "axis=None",
|
|
jnp.squeeze,
|
|
arg_descriptors=[RandArg((5,), _f32), StaticArg(None)],
|
|
poly_axes=[0],
|
|
expect_error=(ValueError, "jnp.squeeze with axis=None is not supported with shape polymorphism")),
|
|
PolyHarness("squeeze", "axis=1",
|
|
jnp.squeeze,
|
|
arg_descriptors=[RandArg((4, 1), _f32), StaticArg((1,))],
|
|
poly_axes=[0]),
|
|
PolyHarness("squeeze", "axis=1_2",
|
|
jnp.squeeze,
|
|
arg_descriptors=[RandArg((4, 1, 1), _f32), StaticArg((1, 2))],
|
|
poly_axes=[0]),
|
|
PolyHarness("squeeze", "error",
|
|
jnp.squeeze,
|
|
arg_descriptors=[RandArg((3, 33), _f32), StaticArg(-1)],
|
|
poly_axes=[(0, 1)],
|
|
skip_jax_run=True,
|
|
expect_error=(ValueError,
|
|
re.escape(
|
|
"cannot select an axis to squeeze out which has size not equal to one, got shape=(b0, b1) and dimensions=(1,)"))
|
|
),
|
|
PolyHarness("take", "",
|
|
lambda a, i: jnp.take(a, i, axis=1),
|
|
arg_descriptors=[RandArg((3, 4, 5), _f32), np.array([1, 2], np.int32)],
|
|
poly_axes=[0, None]).both_enable_and_disable_xla(),
|
|
PolyHarness("take_along_axis", "0",
|
|
lambda x, y: jnp.take_along_axis(x, y, axis=0),
|
|
arg_descriptors=[RandArg((5, 2), _f32), RandArg((5, 1), np.int32)],
|
|
poly_axes=[0, 0]),
|
|
PolyHarness("take_along_axis", "1",
|
|
lambda x, y: jnp.take_along_axis(x, y, axis=1),
|
|
arg_descriptors=[RandArg((5, 2), _f32), RandArg((5, 1), np.int32)],
|
|
poly_axes=[0, 0]),
|
|
PolyHarness("tile", "0",
|
|
lambda x: jnp.tile(x, (1, 2)),
|
|
arg_descriptors=[RandArg((4, 3), _f32)],
|
|
poly_axes=[0]),
|
|
PolyHarness("tile", "1",
|
|
# The repetitions are polys
|
|
lambda x: jnp.tile(x, (1, x.shape[0])),
|
|
arg_descriptors=[RandArg((4, 2), _f32)],
|
|
poly_axes=[0]),
|
|
PolyHarness("tri", "N=poly_M=None",
|
|
lambda x: jnp.tri(x.shape[0]) + x,
|
|
arg_descriptors=[RandArg((3, 1), _f32)],
|
|
poly_axes=[0]),
|
|
PolyHarness("tri", "N=poly_M=poly",
|
|
lambda x: jnp.tri(x.shape[0], M=x.shape[0] + 2) + x,
|
|
arg_descriptors=[RandArg((3, 1), _f32)],
|
|
poly_axes=[0]),
|
|
[
|
|
PolyHarness("var",
|
|
f"{axis=}_{keepdims=}_where=None",
|
|
lambda x, axis, keepdims: jnp.var(x, axis=axis, keepdims=keepdims, where=None),
|
|
arg_descriptors=[RandArg((7, 8, 4), _f32), StaticArg(axis), StaticArg(keepdims)],
|
|
poly_axes=[0])
|
|
for keepdims in [False, True]
|
|
for axis in [None, (0,), (0, 1), (1,)]
|
|
],
|
|
[
|
|
PolyHarness("var",
|
|
f"{axis=}_{keepdims=}_where=Some",
|
|
lambda x, where, axis, keepdims: jnp.var(x, axis=axis, keepdims=keepdims, where=where),
|
|
arg_descriptors=[RandArg((7, 8, 4), _f32), RandArg((7, 8, 4), np.bool_), StaticArg(axis), StaticArg(keepdims)],
|
|
poly_axes=[0, 0])
|
|
for keepdims in [False, True]
|
|
for axis in [None, (0,), (0, 1), (1,)]
|
|
],
|
|
PolyHarness("where", "",
|
|
jnp.where,
|
|
arg_descriptors=[RandArg((2,), np.bool_), RandArg((), _f32), RandArg((2,), _f32)],
|
|
poly_axes=[0, None, 0]),
|
|
]
|
|
|
|
def _get_jax2tf_limitations(
|
|
device, h: primitive_harness.Harness) -> Sequence[Jax2TfLimitation]:
|
|
# And the jax2tf limitations
|
|
def applicable_jax2tf_limitation(l: Jax2TfLimitation) -> bool:
|
|
# The CheckShapePolymorphism uses tf.function, so we care about "graph"
|
|
return l.filter(device=device, dtype=h.dtype, mode="graph")
|
|
|
|
limitations = Jax2TfLimitation.limitations_for_harness(h)
|
|
return tuple(filter(applicable_jax2tf_limitation, limitations))
|
|
|
|
### We add to the test harnesses some that are obtained from the
|
|
### primitive harnesses by applying vmap to the function and then asserting
|
|
### that we can convert shape polymorphically the result.
|
|
|
|
def _make_vmap_primitive_harnesses() -> Sequence[PolyHarness]:
|
|
"""For each harness group, pick a single dtype.
|
|
|
|
See PolyHarness for documentation.
|
|
|
|
Ignore harnesses that fail in graph mode in jax2tf.
|
|
"""
|
|
all_h = primitive_harness.all_harnesses
|
|
res = []
|
|
|
|
# Index by group
|
|
harness_groups: Dict[
|
|
str, Sequence[primitive_harness.Harness]] = collections.defaultdict(list)
|
|
device = jtu.device_under_test()
|
|
|
|
for h in all_h:
|
|
# Drop the JAX limitations
|
|
if not h.filter(device_under_test=device, include_jax_unimpl=False):
|
|
continue
|
|
# And the jax2tf limitations that are known to result in TF error.
|
|
if any(l.expect_tf_error for l in _get_jax2tf_limitations(device, h)):
|
|
continue
|
|
# TODO(marcvanzee): We currently exclude tests with enable_xla=False because
|
|
# this doesn't work with vmap due to a call to lax.gather. We should include
|
|
# them once vmap works with enable_xla=False.
|
|
if not h.params.get("enable_xla", True):
|
|
continue
|
|
harness_groups[h.group_name].append(h)
|
|
|
|
selected_harnesses = []
|
|
for group_name, hlist in harness_groups.items():
|
|
# Pick the dtype with the most harnesses in this group. Some harness
|
|
# groups only test different use cases at a few dtypes.
|
|
c = collections.Counter([h.dtype for h in hlist])
|
|
(dtype, _), = c.most_common(1)
|
|
selected_harnesses.extend([h for h in hlist if h.dtype == dtype])
|
|
|
|
# We do not yet support shape polymorphism for vmap for some primitives
|
|
_NOT_SUPPORTED_YET = frozenset([
|
|
# In linalg._lu_python we do reshape(-1, ...)
|
|
"lu",
|
|
"custom_linear_solve",
|
|
|
|
# We do *= shapes in the batching rule for conv_general_dilated
|
|
"conv_general_dilated",
|
|
|
|
"tridiagonal_solve", # batching not implemented in JAX
|
|
"iota", # vmap does not make sense for 0-argument functions
|
|
"rng_bit_generator", # vmap not implemented
|
|
])
|
|
|
|
batch_size = 3
|
|
for h in selected_harnesses:
|
|
if h.group_name in _NOT_SUPPORTED_YET:
|
|
continue
|
|
|
|
def make_batched_arg_descriptor(
|
|
ad: primitive_harness.ArgDescriptor) -> Optional[primitive_harness.ArgDescriptor]:
|
|
if isinstance(ad, RandArg):
|
|
return RandArg((batch_size,) + ad.shape, ad.dtype)
|
|
elif isinstance(ad, CustomArg):
|
|
def wrap_custom(rng):
|
|
arg = ad.make(rng)
|
|
return np.stack([arg] * batch_size)
|
|
|
|
return CustomArg(wrap_custom)
|
|
else:
|
|
assert isinstance(ad, np.ndarray), ad
|
|
return np.stack([ad] * batch_size)
|
|
|
|
new_args = [make_batched_arg_descriptor(ad)
|
|
for ad in h.arg_descriptors
|
|
if not isinstance(ad, StaticArg)]
|
|
|
|
# This test does not make sense for nullary functions
|
|
if not new_args:
|
|
continue
|
|
|
|
# We do not check the result of harnesses that require custom assertions.
|
|
check_result = all(not l.custom_assert and not l.skip_comparison and l.tol is None
|
|
for l in _get_jax2tf_limitations(device, h))
|
|
if h.group_name == "cumsum":
|
|
# TODO(necula): why do we need to adjust the cumsum tolerance?
|
|
tol = 1e-5
|
|
else:
|
|
tol = None
|
|
vmap_harness = PolyHarness("vmap_" + h.group_name, h.name,
|
|
jax.vmap(h.dyn_fun, in_axes=0, out_axes=0),
|
|
arg_descriptors=new_args,
|
|
poly_axes=[0] * len(new_args),
|
|
check_result=check_result,
|
|
tol=tol)
|
|
vmap_harness.original_harness = h
|
|
res.append(vmap_harness)
|
|
return res
|
|
|
|
_POLY_SHAPE_TEST_HARNESSES.append(_make_vmap_primitive_harnesses())
|
|
|
|
def _flatten_harnesses(harnesses):
|
|
res = []
|
|
for h in harnesses:
|
|
if isinstance(h, Sequence):
|
|
res.extend(h)
|
|
else:
|
|
res.append(h)
|
|
return res
|
|
|
|
|
|
class ShapePolyPrimitivesTest(tf_test_util.JaxToTfTestCase):
|
|
"""Tests for primitives that take shape values as parameters."""
|
|
|
|
# This test runs for all _POLY_SHAPE_PRIMITIVE_HARNESSES.
|
|
|
|
# For each primitive "xxx" the test will be called "test_harness_xxx_...".
|
|
# If you want to run this test for only one harness that includes "foo"
|
|
# in the name (after test_harness), add parameter `one_containing="foo"`
|
|
# to parameterized below.
|
|
@primitive_harness.parameterized(
|
|
_flatten_harnesses(_POLY_SHAPE_TEST_HARNESSES),
|
|
#one_containing="",
|
|
)
|
|
def test_harness(self, harness: PolyHarness):
|
|
# Exclude some harnesses that are known to fail for native serialization
|
|
if config.jax2tf_default_native_serialization:
|
|
if not harness.enable_xla:
|
|
raise unittest.SkipTest("disabled for native_serialization and enable_xla=False")
|
|
|
|
# Set of harness.group_name:platform that are implemented with custom call
|
|
custom_call_harnesses = {
|
|
"vmap_cholesky:cpu", "vmap_cholesky:gpu", "vmap_eig:cpu",
|
|
"vmap_eigh:cpu", "vmap_eigh:gpu", "vmap_fft:cpu",
|
|
"householder_product:cpu", "householder_product:gpu",
|
|
"vmap_geqrf:cpu", "vmap_geqrf:gpu",
|
|
"vmap_lu:cpu", "vmap_lu:gpu", "vmap_qr:cpu", "vmap_qr:gpu",
|
|
"vmap_svd:cpu", "vmap_svd:gpu",
|
|
"random_gamma:gpu", "vmap_random_gamma:gpu",
|
|
"random_categorical:gpu", "vmap_random_categorical:gpu",
|
|
"random_randint:gpu", "vmap_random_randint:gpu",
|
|
"random_uniform:gpu", "vmap_random_uniform:gpu",
|
|
"vmap_random_split:gpu"}
|
|
if f"{harness.group_name}:{jtu.device_under_test()}" in custom_call_harnesses:
|
|
raise unittest.SkipTest("native serialization with shape polymorphism not implemented for custom calls; b/261671778")
|
|
|
|
# Set of harness.group_name or harness.group_name:platform that are implemented with HLO fallback lowering rules
|
|
fallback_lowering_harnesses = {
|
|
"vmap_approx_top_k", "vmap_bessel_i0e", "vmap_eigh:tpu",
|
|
"vmap_erf_inv", "vmap_igamma", "vmap_igammac", "vmap_lu",
|
|
"vmap_regularized_incomplete_beta", "vmap_qr:tpu",
|
|
"vmap_random_gamma:cpu", "random_gamma:tpu",
|
|
"vmap_random_gamma:tpu", "vmap_svd:tpu"}
|
|
if (harness.group_name in fallback_lowering_harnesses or
|
|
f"{harness.group_name}:{jtu.device_under_test()}" in fallback_lowering_harnesses):
|
|
raise unittest.SkipTest(
|
|
"native serialization with shape polymorphism not implemented for JAX primitives still using HLO fallback lowering; b/261682623")
|
|
|
|
# Set of harness.group_name that are unsupported in serialization
|
|
require_stablehlo_feature_support = {
|
|
# Tan (b/274462307) and TopK (openxla/stablehlo#1255) require support.
|
|
"vmap_tan", "vmap_top_k",
|
|
# Crash due to openxla/stablehlo#1328
|
|
"vmap_random_randint", "vmap_random_uniform"
|
|
}
|
|
if harness.group_name in require_stablehlo_feature_support:
|
|
raise unittest.SkipTest(
|
|
"native lowering with shape polymorphism requires additional StableHLO feature support")
|
|
if (jtu.device_under_test() == "tpu" and
|
|
harness.fullname in [
|
|
"jnp.cumsum_reduce_axis=poly",
|
|
"jnp.insert_insert=constant", "jnp.insert_insert=poly",
|
|
"jnp.nonzero_size=constant", "jnp.nonzero_size=poly"]):
|
|
# https://github.com/openxla/stablehlo/issues/1258
|
|
raise unittest.SkipTest(
|
|
"native serialization with shape polymorphism not implemented for window_reductions on TPU")
|
|
if (jtu.device_under_test() == "gpu" and
|
|
harness.fullname in [
|
|
"jnp.cumsum_reduce_axis=poly",
|
|
"jnp.insert_insert=constant", "jnp.insert_insert=poly",
|
|
"jnp.nonzero_size=constant", "jnp.nonzero_size=poly"]):
|
|
raise unittest.SkipTest(
|
|
"TODO(b/271645610): investigate inconclusive dimension operation for cumsum on gpu")
|
|
|
|
harness.run_test(self)
|
|
|
|
|
|
if __name__ == "__main__":
|
|
absltest.main(testLoader=jtu.JaxTestLoader())
|