mirror of
https://github.com/ROCm/jax.git
synced 2025-04-19 05:16:06 +00:00
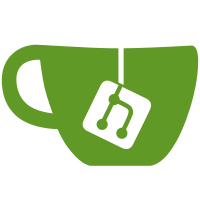
1. Add (limited) precision specifier handling to LSTM Enables differentiating between TF32 and FP32 math. TF32 math had insufficient precision to reliably pass LSTM correctness tests on A100 and H100. 2. Run the test using FP32 TF32 precision is not sufficient for the test to pass reliably on Ampere+ GPUs such as A100 and H100.
133 lines
5.3 KiB
Python
133 lines
5.3 KiB
Python
# Copyright 2022 The JAX Authors.
|
|
#
|
|
# Licensed under the Apache License, Version 2.0 (the "License");
|
|
# you may not use this file except in compliance with the License.
|
|
# You may obtain a copy of the License at
|
|
#
|
|
# https://www.apache.org/licenses/LICENSE-2.0
|
|
#
|
|
# Unless required by applicable law or agreed to in writing, software
|
|
# distributed under the License is distributed on an "AS IS" BASIS,
|
|
# WITHOUT WARRANTIES OR CONDITIONS OF ANY KIND, either express or implied.
|
|
# See the License for the specific language governing permissions and
|
|
# limitations under the License.
|
|
|
|
import jaxlib.mlir.ir as ir
|
|
import jaxlib.mlir.dialects.stablehlo as hlo
|
|
|
|
import numpy as np
|
|
|
|
from jaxlib import xla_client
|
|
from .gpu_common_utils import GpuLibNotLinkedError
|
|
|
|
try:
|
|
from .cuda import _rnn # pytype: disable=import-error
|
|
for _name, _value in _rnn.registrations().items():
|
|
xla_client.register_custom_call_target(_name, _value, platform='CUDA')
|
|
except ImportError:
|
|
_rnn = None
|
|
|
|
if _rnn:
|
|
compute_rnn_workspace_reserve_space_sizes = _rnn.compute_rnn_workspace_reserve_space_sizes
|
|
|
|
|
|
def cudnn_rnn_lowering(ctx, input, h_0, c_0, weights, seq_lengths, *,
|
|
input_size: int, hidden_size: int, num_layers: int,
|
|
dropout: bool, bidirectional: bool,
|
|
cudnn_allow_tf32: bool):
|
|
"""CuDnn RNN."""
|
|
out_dtype = ctx.avals_out[0].dtype
|
|
if out_dtype == np.float32:
|
|
out_type = ir.F32Type.get()
|
|
elif out_dtype == np.float64:
|
|
out_type = ir.F64Type.get()
|
|
elif out_dtype == np.complex64:
|
|
out_type = ir.ComplexType.get(ir.F32Type.get())
|
|
elif out_dtype == np.complex128:
|
|
out_type = ir.ComplexType.get(ir.F64Type.get())
|
|
else:
|
|
raise ValueError(f'Unknown output type {out_dtype}')
|
|
|
|
output_type = ir.RankedTensorType.get(ctx.avals_out[0].shape, out_type)
|
|
batch_size = ctx.avals_in[0].shape[0]
|
|
max_seq_length = ctx.avals_in[0].shape[1]
|
|
# workspace_shape = ctx.avals_out[3].shape
|
|
workspace_size, _ = compute_rnn_workspace_reserve_space_sizes(
|
|
input_size, hidden_size, num_layers, batch_size, max_seq_length,
|
|
dropout, bidirectional, cudnn_allow_tf32)
|
|
workspace_shape = (workspace_size,)
|
|
workspace_type = ir.RankedTensorType.get(workspace_shape, ir.F32Type.get())
|
|
reserve_space_shape = ctx.avals_out[3].shape
|
|
reserve_space_type = ir.RankedTensorType.get(reserve_space_shape,
|
|
ir.F32Type.get())
|
|
if not _rnn:
|
|
raise GpuLibNotLinkedError()
|
|
|
|
opaque = _rnn.build_rnn_descriptor(input_size, hidden_size, num_layers,
|
|
batch_size, max_seq_length, dropout,
|
|
bidirectional, cudnn_allow_tf32,
|
|
workspace_shape[0],
|
|
reserve_space_shape[0])
|
|
|
|
i32_type = ir.IntegerType.get_signless(32)
|
|
|
|
out = hlo.CustomCallOp(
|
|
[output_type, h_0.type, c_0.type, workspace_type, reserve_space_type],
|
|
[input, h_0, c_0, weights, seq_lengths],
|
|
call_target_name=ir.StringAttr.get('cudnn_rnn'),
|
|
has_side_effect=ir.BoolAttr.get(False),
|
|
backend_config=ir.StringAttr.get(opaque),
|
|
api_version=ir.IntegerAttr.get(i32_type, 2),
|
|
called_computations=ir.ArrayAttr.get([]),
|
|
)
|
|
return out.results[:-2] + out.results[-1:] # drop workspace output
|
|
|
|
|
|
def _hlo_zeros_f32(shape):
|
|
return hlo.ConstantOp(
|
|
ir.DenseElementsAttr.get(
|
|
np.zeros(shape, dtype=np.float32), type=ir.F32Type.get())).result
|
|
|
|
|
|
def cudnn_rnn_bwd_lowering(ctx, dy, dhn, dcn, x, h0, c0, w, y,
|
|
reserve_space, seq_lengths, *, input_size: int,
|
|
hidden_size: int, num_layers: int, dropout: bool,
|
|
bidirectional: bool, cudnn_allow_tf32: bool):
|
|
"""CuDnn RNN Backward pass."""
|
|
batch_size = ctx.avals_in[3].shape[0]
|
|
max_seq_length = ctx.avals_in[3].shape[1]
|
|
workspace_size, _ = compute_rnn_workspace_reserve_space_sizes(
|
|
input_size, hidden_size, num_layers, batch_size, max_seq_length,
|
|
dropout, bidirectional, cudnn_allow_tf32)
|
|
workspace_shape = (workspace_size,)
|
|
workspace_type = ir.RankedTensorType.get(workspace_shape, ir.F32Type.get())
|
|
reserve_space_shape = ctx.avals_in[8].shape
|
|
|
|
if _rnn is None:
|
|
raise RuntimeError("cuda couldn't be imported")
|
|
opaque = _rnn.build_rnn_descriptor(input_size, hidden_size, num_layers,
|
|
batch_size, max_seq_length, dropout,
|
|
bidirectional, cudnn_allow_tf32,
|
|
workspace_shape[0],
|
|
reserve_space_shape[0])
|
|
|
|
i32_type = ir.IntegerType.get_signless(32)
|
|
zeroed_dw = _hlo_zeros_f32(ctx.avals_out[3].shape)
|
|
out = hlo.CustomCallOp(
|
|
[x.type, h0.type, c0.type, w.type, workspace_type], [
|
|
dy, dhn, dcn, x, h0, c0, w, y, reserve_space, zeroed_dw,
|
|
seq_lengths
|
|
],
|
|
call_target_name=ir.StringAttr.get('cudnn_rnn_bwd'),
|
|
has_side_effect=ir.BoolAttr.get(False),
|
|
backend_config=ir.StringAttr.get(opaque),
|
|
api_version=ir.IntegerAttr.get(i32_type, 2),
|
|
called_computations=ir.ArrayAttr.get([]),
|
|
output_operand_aliases=ir.ArrayAttr.get([
|
|
hlo.OutputOperandAlias.get(
|
|
output_tuple_indices=[3],
|
|
operand_index=9,
|
|
operand_tuple_indices=[])
|
|
]))
|
|
return out.results[:-1] # drop workspace output
|