mirror of
https://github.com/ROCm/jax.git
synced 2025-04-24 14:56:05 +00:00
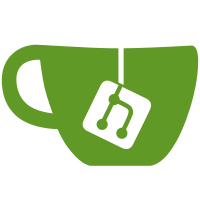
Some folks want to be able to run JAX-generated HLO computations from C++, and those computations may refer to JAX's custom kernels. This change splits the custom kernels into separate modules that may be used independently of Python. The general pattern is that each extension now has two parts: * xyz_kernels.{cc, h} — the C++ parts * xyz.cc — Python bindings around the C++ parts, including code to build any descriptor objects. There's also a new (minimally supported) module named "gpu_kernels.cc" which registers JAX's GPU kernels with the XLA C++ custom kernel registry. PiperOrigin-RevId: 394460343
56 lines
1.8 KiB
Python
56 lines
1.8 KiB
Python
# Copyright 2019 Google LLC
|
|
#
|
|
# Licensed under the Apache License, Version 2.0 (the "License");
|
|
# you may not use this file except in compliance with the License.
|
|
# You may obtain a copy of the License at
|
|
#
|
|
# https://www.apache.org/licenses/LICENSE-2.0
|
|
#
|
|
# Unless required by applicable law or agreed to in writing, software
|
|
# distributed under the License is distributed on an "AS IS" BASIS,
|
|
# WITHOUT WARRANTIES OR CONDITIONS OF ANY KIND, either express or implied.
|
|
# See the License for the specific language governing permissions and
|
|
# limitations under the License.
|
|
|
|
|
|
import functools
|
|
import itertools
|
|
import operator
|
|
|
|
import numpy as np
|
|
|
|
from jaxlib import xla_client
|
|
|
|
try:
|
|
from . import _cuda_prng
|
|
for _name, _value in _cuda_prng.registrations().items():
|
|
xla_client.register_custom_call_target(_name, _value, platform="CUDA")
|
|
except ImportError:
|
|
pass
|
|
|
|
_prod = lambda xs: functools.reduce(operator.mul, xs, 1)
|
|
|
|
def threefry2x32(c, keys, data):
|
|
"""ThreeFry2x32 kernel for GPU."""
|
|
assert len(keys) == 2, keys
|
|
assert len(data) == 2, data
|
|
dims = c.get_shape(keys[0]).dimensions()
|
|
dtype = np.dtype(np.uint32)
|
|
for x in itertools.chain(keys, data):
|
|
x_shape = c.get_shape(x)
|
|
assert x_shape.element_type() == dtype
|
|
assert dims == x_shape.dimensions(), (dims, x_shape)
|
|
ndims = len(dims)
|
|
|
|
opaque = _cuda_prng.cuda_threefry2x32_descriptor(_prod(dims))
|
|
layout = tuple(range(ndims - 1, -1, -1))
|
|
shape = xla_client.Shape.array_shape(dtype, dims, layout)
|
|
return xla_client.ops.CustomCallWithLayout(
|
|
c, b"cuda_threefry2x32",
|
|
operands=(keys[0], keys[1], data[0], data[1]),
|
|
shape_with_layout=xla_client.Shape.tuple_shape([shape, shape]),
|
|
operand_shapes_with_layout=(shape,) * 4,
|
|
opaque=opaque,
|
|
api_version=xla_client.ops.CustomCallApiVersion
|
|
.API_VERSION_STATUS_RETURNING)
|