mirror of
https://github.com/ROCm/jax.git
synced 2025-04-16 20:06:05 +00:00
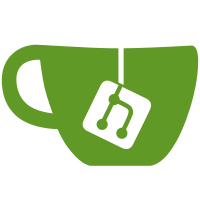
This adds support for shape polymorphism and export for this custom call, and adds the appropriate tests. One of the biggest changes here is to move all the lowing logic for the getrf call into jax (lax/linalg.py) instead of in jaxlib (gpu_solver.py and lapack.py) since the lowering code is now identical for CPU and GPU (the only difference is the handler names). PiperOrigin-RevId: 665829252
581 lines
20 KiB
Python
581 lines
20 KiB
Python
# Copyright 2019 The JAX Authors.
|
|
#
|
|
# Licensed under the Apache License, Version 2.0 (the "License");
|
|
# you may not use this file except in compliance with the License.
|
|
# You may obtain a copy of the License at
|
|
#
|
|
# https://www.apache.org/licenses/LICENSE-2.0
|
|
#
|
|
# Unless required by applicable law or agreed to in writing, software
|
|
# distributed under the License is distributed on an "AS IS" BASIS,
|
|
# WITHOUT WARRANTIES OR CONDITIONS OF ANY KIND, either express or implied.
|
|
# See the License for the specific language governing permissions and
|
|
# limitations under the License.
|
|
|
|
from collections.abc import Sequence
|
|
from functools import partial
|
|
import importlib
|
|
import math
|
|
|
|
import jaxlib.mlir.ir as ir
|
|
import jaxlib.mlir.dialects.stablehlo as hlo
|
|
|
|
import numpy as np
|
|
|
|
from .gpu_common_utils import GpuLibNotLinkedError
|
|
|
|
from jaxlib import xla_client
|
|
|
|
from .hlo_helpers import (
|
|
DimensionSize, ShapeTypePair, mk_result_types_and_shapes,
|
|
custom_call, ensure_hlo_s32, hlo_s32, dense_int_array)
|
|
|
|
try:
|
|
from .cuda import _blas as _cublas # pytype: disable=import-error
|
|
except ImportError:
|
|
for cuda_module_name in ["jax_cuda12_plugin"]:
|
|
try:
|
|
_cublas = importlib.import_module(f"{cuda_module_name}._blas")
|
|
except ImportError:
|
|
_cublas = None
|
|
else:
|
|
break
|
|
|
|
if _cublas:
|
|
for _name, _value in _cublas.registrations().items():
|
|
xla_client.register_custom_call_target(_name, _value, platform="CUDA")
|
|
|
|
for cuda_module_name in [".cuda", "jax_cuda12_plugin"]:
|
|
try:
|
|
_cusolver = importlib.import_module(
|
|
f"{cuda_module_name}._solver", package="jaxlib"
|
|
)
|
|
except ImportError:
|
|
_cusolver = None
|
|
else:
|
|
break
|
|
|
|
if _cusolver:
|
|
for _name, _value in _cusolver.registrations().items():
|
|
# TODO(danfm): Clean up after all legacy custom calls are ported.
|
|
api_version = 1 if _name.endswith("_ffi") else 0
|
|
xla_client.register_custom_call_target(_name, _value, platform="CUDA",
|
|
api_version=api_version)
|
|
|
|
try:
|
|
from .rocm import _blas as _hipblas # pytype: disable=import-error
|
|
except ImportError:
|
|
for rocm_module_name in ["jax_rocm60_plugin"]:
|
|
try:
|
|
_hipblas = importlib.import_module(f"{rocm_module_name}._blas")
|
|
except:
|
|
_hipblas = None
|
|
else:
|
|
break
|
|
|
|
if _hipblas:
|
|
for _name, _value in _hipblas.registrations().items():
|
|
xla_client.register_custom_call_target(_name, _value, platform="ROCM")
|
|
|
|
for rocm_module_name in [".rocm", "jax_rocm60_plugin"]:
|
|
try:
|
|
_hipsolver = importlib.import_module(
|
|
f"{rocm_module_name}._solver", package="jaxlib"
|
|
)
|
|
except ImportError:
|
|
_hipsolver = None
|
|
else:
|
|
break
|
|
|
|
if _hipsolver:
|
|
for _name, _value in _hipsolver.registrations().items():
|
|
# TODO(danfm): Clean up after all legacy custom calls are ported.
|
|
api_version = 1 if _name.endswith("_ffi") else 0
|
|
xla_client.register_custom_call_target(_name, _value, platform="ROCM",
|
|
api_version=api_version)
|
|
|
|
def _real_type(dtype):
|
|
"""Returns the real equivalent of 'dtype'."""
|
|
return np.finfo(dtype).dtype
|
|
|
|
|
|
# TODO(b/357034884): Remove this function after the forward compat window.
|
|
def _getrf_hlo(platform, gpu_blas, gpu_solver, dtype, a):
|
|
"""LU decomposition."""
|
|
a_type = ir.RankedTensorType(a.type)
|
|
dims = a_type.shape
|
|
assert len(dims) >= 2
|
|
m, n = dims[-2:]
|
|
batch_dims = tuple(dims[:-2])
|
|
num_bd = len(batch_dims)
|
|
i32_type = ir.IntegerType.get_signless(32)
|
|
layout = (num_bd, num_bd + 1) + tuple(range(num_bd - 1, -1, -1))
|
|
|
|
if not gpu_blas:
|
|
raise GpuLibNotLinkedError()
|
|
|
|
batch = math.prod(batch_dims)
|
|
if batch > 1 and m == n and m // batch <= 128:
|
|
lwork, opaque = gpu_blas.build_getrf_batched_descriptor(
|
|
np.dtype(dtype), batch, m)
|
|
workspace = ir.RankedTensorType.get([lwork], ir.IntegerType.get_signless(8))
|
|
kernel = f"{platform}blas_getrf_batched"
|
|
else:
|
|
lwork, opaque = gpu_solver.build_getrf_descriptor(
|
|
np.dtype(dtype), batch, m, n)
|
|
workspace = ir.RankedTensorType.get([lwork], a_type.element_type)
|
|
kernel = f"{platform}solver_getrf"
|
|
|
|
out = custom_call(
|
|
kernel,
|
|
result_types=[
|
|
a.type,
|
|
ir.RankedTensorType.get(batch_dims + (min(m, n),), i32_type),
|
|
ir.RankedTensorType.get(batch_dims, i32_type),
|
|
workspace,
|
|
],
|
|
operands=[a],
|
|
backend_config=opaque,
|
|
operand_layouts=[layout],
|
|
result_layouts=[
|
|
layout,
|
|
tuple(range(num_bd, -1, -1)),
|
|
tuple(range(num_bd - 1, -1, -1)),
|
|
[0],
|
|
],
|
|
operand_output_aliases={0: 0}).results
|
|
return out[:3]
|
|
|
|
|
|
cuda_getrf = partial(_getrf_hlo, "cu", _cublas, _cusolver)
|
|
rocm_getrf = partial(_getrf_hlo, "hip", _hipblas, _hipsolver)
|
|
|
|
|
|
def _geqrf_hlo(platform, gpu_solver, dtype, a):
|
|
"""QR decomposition."""
|
|
a_type = ir.RankedTensorType(a.type)
|
|
dims = a_type.shape
|
|
assert len(dims) >= 2
|
|
m, n = dims[-2:]
|
|
batch_dims = tuple(dims[:-2])
|
|
num_bd = len(batch_dims)
|
|
batch = math.prod(batch_dims)
|
|
|
|
lwork, opaque = gpu_solver.build_geqrf_descriptor(
|
|
np.dtype(dtype), batch, m, n)
|
|
|
|
layout = (num_bd, num_bd + 1) + tuple(range(num_bd - 1, -1, -1))
|
|
i32_type = ir.IntegerType.get_signless(32)
|
|
out = custom_call(
|
|
f"{platform}solver_geqrf",
|
|
result_types=[
|
|
a.type,
|
|
ir.RankedTensorType.get(batch_dims + (min(m, n),), a_type.element_type),
|
|
ir.RankedTensorType.get(batch_dims, i32_type),
|
|
ir.RankedTensorType.get([lwork], a_type.element_type),
|
|
],
|
|
operands=[a],
|
|
backend_config=opaque,
|
|
operand_layouts=[layout],
|
|
result_layouts=[
|
|
layout,
|
|
tuple(range(num_bd, -1, -1)),
|
|
tuple(range(num_bd - 1, -1, -1)),
|
|
[0],
|
|
],
|
|
operand_output_aliases={0: 0}).results
|
|
return out[:3]
|
|
|
|
cuda_geqrf = partial(_geqrf_hlo, "cu", _cusolver)
|
|
rocm_geqrf = partial(_geqrf_hlo, "hip", _hipsolver)
|
|
|
|
def _geqrf_batched_hlo(platform, gpu_blas, dtype, a):
|
|
"""Batched QR decomposition."""
|
|
a_type = ir.RankedTensorType(a.type)
|
|
dims = a_type.shape
|
|
assert len(dims) >= 2
|
|
m, n = dims[-2:]
|
|
batch_dims = tuple(dims[:-2])
|
|
num_bd = len(batch_dims)
|
|
batch = math.prod(batch_dims)
|
|
|
|
if not gpu_blas:
|
|
raise GpuLibNotLinkedError()
|
|
|
|
lwork, opaque = gpu_blas.build_geqrf_batched_descriptor(
|
|
np.dtype(dtype), batch, m, n)
|
|
|
|
layout = (num_bd, num_bd + 1) + tuple(range(num_bd - 1, -1, -1))
|
|
out = custom_call(
|
|
f"{platform}blas_geqrf_batched",
|
|
result_types=[
|
|
a.type,
|
|
ir.RankedTensorType.get(batch_dims + (min(m, n),), a_type.element_type),
|
|
ir.RankedTensorType.get([lwork], ir.IntegerType.get_signless(8)),
|
|
ir.RankedTensorType.get([lwork], ir.IntegerType.get_signless(8)),
|
|
],
|
|
operands=[a],
|
|
backend_config=opaque,
|
|
operand_layouts=[layout],
|
|
result_layouts=[
|
|
layout,
|
|
tuple(range(num_bd, -1, -1)),
|
|
[0],
|
|
[0],
|
|
],
|
|
operand_output_aliases={0: 0}
|
|
).results
|
|
return out[:2]
|
|
|
|
cuda_geqrf_batched = partial(_geqrf_batched_hlo, "cu", _cublas)
|
|
rocm_geqrf_batched = partial(_geqrf_batched_hlo, "hip", _hipblas)
|
|
|
|
|
|
def _csrlsvqr_hlo(platform, gpu_solver, dtype, data,
|
|
indices, indptr, b, tol, reorder):
|
|
"""Sparse solver via QR decomposition. CUDA only."""
|
|
b_type = ir.RankedTensorType(b.type)
|
|
data_type = ir.RankedTensorType(data.type)
|
|
|
|
n = b_type.shape[0]
|
|
nnz = data_type.shape[0]
|
|
opaque = gpu_solver.build_csrlsvqr_descriptor(
|
|
np.dtype(dtype), n, nnz, reorder, tol
|
|
)
|
|
|
|
out = custom_call(
|
|
f"{platform}solver_csrlsvqr", # call_target_name
|
|
result_types=[b.type],
|
|
operands=[data, indptr, indices, b],
|
|
backend_config=opaque, # backend_config
|
|
operand_layouts=[(0,), (0,), (0,), (0,)], # operand_layouts
|
|
result_layouts=[(0,)] # result_layouts
|
|
).results
|
|
return out
|
|
|
|
cuda_csrlsvqr = partial(_csrlsvqr_hlo, "cu", _cusolver)
|
|
|
|
|
|
def _orgqr_hlo(platform, gpu_solver, dtype, a, tau):
|
|
"""Product of elementary Householder reflections."""
|
|
a_type = ir.RankedTensorType(a.type)
|
|
dims = a_type.shape
|
|
assert len(dims) >= 2
|
|
m, n = dims[-2:]
|
|
batch_dims = tuple(dims[:-2])
|
|
num_bd = len(batch_dims)
|
|
batch = math.prod(batch_dims)
|
|
|
|
tau_dims = ir.RankedTensorType(tau.type).shape
|
|
assert tau_dims[:-1] == dims[:-2]
|
|
k = tau_dims[-1]
|
|
|
|
lwork, opaque = gpu_solver.build_orgqr_descriptor(
|
|
np.dtype(dtype), batch, m, n, k)
|
|
|
|
layout = (num_bd, num_bd + 1) + tuple(range(num_bd - 1, -1, -1))
|
|
i32_type = ir.IntegerType.get_signless(32)
|
|
out = custom_call(
|
|
f"{platform}solver_orgqr",
|
|
result_types=[
|
|
a.type,
|
|
ir.RankedTensorType.get(batch_dims, i32_type),
|
|
ir.RankedTensorType.get([lwork], a_type.element_type),
|
|
],
|
|
operands=[a, tau],
|
|
backend_config=opaque,
|
|
operand_layouts=[
|
|
layout,
|
|
tuple(range(num_bd, -1, -1)),
|
|
],
|
|
result_layouts=[
|
|
layout,
|
|
tuple(range(num_bd - 1, -1, -1)),
|
|
[0],
|
|
],
|
|
operand_output_aliases={0: 0}).results
|
|
return out[:2]
|
|
|
|
cuda_orgqr = partial(_orgqr_hlo, "cu", _cusolver)
|
|
rocm_orgqr = partial(_orgqr_hlo, "hip", _hipsolver)
|
|
|
|
|
|
def _syevd_hlo(platform, gpu_solver, have_jacobi_solver, dtype, a, *,
|
|
a_shape_vals: tuple[DimensionSize, ...], lower=False):
|
|
"""Symmetric (Hermitian) eigendecomposition."""
|
|
a_type = ir.RankedTensorType(a.type)
|
|
assert len(a_shape_vals) >= 2
|
|
m, n = a_shape_vals[-2:]
|
|
assert type(m) is int and type(n) is int and m == n, a_shape_vals
|
|
batch_dims_vals = a_shape_vals[:-2]
|
|
|
|
num_bd = len(batch_dims_vals)
|
|
layout = (num_bd, num_bd + 1) + tuple(range(num_bd - 1, -1, -1))
|
|
|
|
dynamic_batch_dims = any(type(d) != int for d in batch_dims_vals)
|
|
if dynamic_batch_dims:
|
|
batch_int = -1 # Signals to the kernel that the batch is an operand.
|
|
else:
|
|
batch_int = math.prod(batch_dims_vals)
|
|
|
|
if have_jacobi_solver and n <= 32 and not dynamic_batch_dims:
|
|
# We cannot use syevj for dynamic shapes because the workspace size
|
|
# depends on the batch size.
|
|
kernel = f"{platform}solver_syevj"
|
|
lwork, opaque = gpu_solver.build_syevj_descriptor(
|
|
np.dtype(dtype), lower, batch_int, n)
|
|
else:
|
|
kernel = f"{platform}solver_syevd"
|
|
lwork, opaque = gpu_solver.build_syevd_descriptor(
|
|
np.dtype(dtype), lower, batch_int, n)
|
|
# TODO(Ruturaj4): Currently, hipsolverSsyevd sets lwork to 0 if n==0.
|
|
# Remove if this behavior changes in then new ROCm release.
|
|
if n > 0 or platform != "hip":
|
|
assert lwork > 0
|
|
|
|
if ir.ComplexType.isinstance(a_type.element_type):
|
|
eigvals_type = ir.ComplexType(a_type.element_type).element_type
|
|
else:
|
|
eigvals_type = a_type.element_type
|
|
|
|
i32_type = ir.IntegerType.get_signless(32)
|
|
operands = [a]
|
|
operand_layouts = [layout]
|
|
if dynamic_batch_dims:
|
|
batch_size_val = hlo_s32(1)
|
|
for b_v in batch_dims_vals:
|
|
batch_size_val = hlo.multiply(batch_size_val, ensure_hlo_s32(b_v))
|
|
operands.append(batch_size_val)
|
|
operand_layouts.append(())
|
|
|
|
shape_type_pairs: Sequence[ShapeTypePair] = [
|
|
(a_shape_vals, a_type.element_type),
|
|
(batch_dims_vals + (n,), eigvals_type),
|
|
(batch_dims_vals, i32_type),
|
|
([lwork], a_type.element_type)]
|
|
result_types, result_shapes = mk_result_types_and_shapes(shape_type_pairs)
|
|
out = custom_call(
|
|
kernel,
|
|
result_types=result_types,
|
|
operands=operands,
|
|
backend_config=opaque,
|
|
operand_layouts=operand_layouts,
|
|
result_layouts=[
|
|
layout,
|
|
tuple(range(num_bd, -1, -1)),
|
|
tuple(range(num_bd - 1, -1, -1)),
|
|
[0],
|
|
],
|
|
operand_output_aliases={0: 0},
|
|
result_shapes=result_shapes).results
|
|
return out[:3]
|
|
|
|
cuda_syevd = partial(_syevd_hlo, "cu", _cusolver, True)
|
|
rocm_syevd = partial(_syevd_hlo, "hip", _hipsolver, True)
|
|
|
|
|
|
def _gesvd_hlo(platform, gpu_solver, have_jacobi_solver, dtype, a,
|
|
full_matrices=True, compute_uv=True):
|
|
"""Singular value decomposition."""
|
|
a_type = ir.RankedTensorType(a.type)
|
|
dims = a_type.shape
|
|
assert len(dims) >= 2
|
|
m, n = dims[-2:]
|
|
batch_dims = tuple(dims[:-2])
|
|
num_bd = len(batch_dims)
|
|
b = math.prod(batch_dims)
|
|
if ir.ComplexType.isinstance(a_type.element_type):
|
|
singular_vals_type = ir.ComplexType(a_type.element_type).element_type
|
|
else:
|
|
singular_vals_type = a_type.element_type
|
|
|
|
scalar_layout = tuple(range(num_bd - 1, -1, -1))
|
|
vector_layout = (num_bd,) + tuple(range(num_bd - 1, -1, -1))
|
|
i32_type = ir.IntegerType.get_signless(32)
|
|
|
|
# NVIDIA's batched Jacobi solver supports a maximum matrix size of 32x32, but
|
|
# the unbatched solver has no such limit. The unbatched solver appears to
|
|
# outperform gesvd for small-moderate matrices, e.g., see:
|
|
# https://developer.download.nvidia.com/video/gputechconf/gtc/2019/presentation/s9226-fast-singular-value-decomposition-on-gpus-v2.pdf
|
|
# slide 5.
|
|
if have_jacobi_solver and m <= 1024 and n <= 1024:
|
|
# The gesvdjbatched kernel doesn't support "econ" mode. We will use that
|
|
# kernel only if b > 1 and m <= 32 and n <= 32.
|
|
econ = not full_matrices and (b <= 1 or m > 32 or n > 32)
|
|
lwork, opaque = gpu_solver.build_gesvdj_descriptor(
|
|
np.dtype(dtype), b, m, n, compute_uv, 1 if econ else 0)
|
|
k = min(m, n)
|
|
matrix_layout = (num_bd, num_bd + 1) + tuple(range(num_bd - 1, -1, -1))
|
|
_, s, u, v, info, _ = custom_call(
|
|
f"{platform}solver_gesvdj",
|
|
result_types=[
|
|
a.type,
|
|
ir.RankedTensorType.get(batch_dims + (min(m, n),), singular_vals_type),
|
|
ir.RankedTensorType.get(batch_dims + (m, k if econ else m),
|
|
a_type.element_type),
|
|
ir.RankedTensorType.get(batch_dims + (n, k if econ else n),
|
|
a_type.element_type),
|
|
ir.RankedTensorType.get(batch_dims, i32_type),
|
|
ir.RankedTensorType.get([lwork], a_type.element_type),
|
|
],
|
|
operands=[a],
|
|
backend_config=opaque,
|
|
operand_layouts=[matrix_layout],
|
|
result_layouts=[
|
|
matrix_layout,
|
|
vector_layout,
|
|
matrix_layout,
|
|
matrix_layout,
|
|
scalar_layout,
|
|
[0],
|
|
],
|
|
operand_output_aliases={0: 0}).results
|
|
vt = hlo.transpose(
|
|
v,
|
|
dense_int_array(np.array(tuple(range(num_bd)) + (num_bd + 1, num_bd))))
|
|
if np.issubdtype(dtype, np.complexfloating):
|
|
vt = hlo.complex(hlo.real(vt), hlo.negate(hlo.imag(vt)))
|
|
if not full_matrices and not econ:
|
|
u = hlo.slice(
|
|
u,
|
|
dense_int_array(np.zeros([len(dims)], np.int64)),
|
|
dense_int_array(np.array(batch_dims + (m, min(m, n)))),
|
|
dense_int_array(np.ones([len(dims)], np.int64)))
|
|
vt = hlo.slice(
|
|
vt,
|
|
dense_int_array(np.zeros([len(dims)], np.int64)),
|
|
dense_int_array(np.array(batch_dims + (min(m, n), n))),
|
|
dense_int_array(np.ones([len(dims)], np.int64)))
|
|
elif m < n:
|
|
lwork, opaque = gpu_solver.build_gesvd_descriptor(
|
|
np.dtype(dtype), b, n, m, compute_uv, full_matrices)
|
|
k = n if full_matrices else m
|
|
matrix_layout = (num_bd + 1, num_bd) + tuple(range(num_bd - 1, -1, -1))
|
|
_, s, vt, u, info, _ = custom_call(
|
|
f"{platform}solver_gesvd",
|
|
result_types=[
|
|
a.type,
|
|
ir.RankedTensorType.get(batch_dims + (min(m, n),), singular_vals_type),
|
|
ir.RankedTensorType.get(batch_dims + (k, n), a_type.element_type),
|
|
ir.RankedTensorType.get(batch_dims + (m, m), a_type.element_type),
|
|
ir.RankedTensorType.get(batch_dims, i32_type),
|
|
ir.RankedTensorType.get([lwork], a_type.element_type),
|
|
],
|
|
operands=[a],
|
|
backend_config=opaque,
|
|
operand_layouts=[matrix_layout],
|
|
result_layouts=[
|
|
matrix_layout,
|
|
vector_layout,
|
|
matrix_layout,
|
|
matrix_layout,
|
|
scalar_layout,
|
|
[0],
|
|
],
|
|
operand_output_aliases={0: 0}).results
|
|
else:
|
|
lwork, opaque = gpu_solver.build_gesvd_descriptor(
|
|
np.dtype(dtype), b, m, n, compute_uv, full_matrices)
|
|
k = m if full_matrices else n
|
|
matrix_layout = (num_bd, num_bd + 1) + tuple(range(num_bd - 1, -1, -1))
|
|
_, s, u, vt, info, _ = custom_call(
|
|
f"{platform}solver_gesvd",
|
|
result_types=[
|
|
a.type,
|
|
ir.RankedTensorType.get(batch_dims + (min(m, n),), singular_vals_type),
|
|
ir.RankedTensorType.get(batch_dims + (m, k), a_type.element_type),
|
|
ir.RankedTensorType.get(batch_dims + (n, n), a_type.element_type),
|
|
ir.RankedTensorType.get(batch_dims, i32_type),
|
|
ir.RankedTensorType.get([lwork], a_type.element_type),
|
|
],
|
|
operands=[a],
|
|
backend_config=opaque,
|
|
operand_layouts=[matrix_layout],
|
|
result_layouts=[
|
|
matrix_layout,
|
|
vector_layout,
|
|
matrix_layout,
|
|
matrix_layout,
|
|
scalar_layout,
|
|
[0],
|
|
],
|
|
operand_output_aliases={0: 0}).results
|
|
return s, u, vt, info
|
|
|
|
cuda_gesvd = partial(_gesvd_hlo, "cu", _cusolver, True)
|
|
rocm_gesvd = partial(_gesvd_hlo, "hip", _hipsolver, False)
|
|
|
|
|
|
def _sytrd_hlo(platform, gpu_solver, dtype, a, *, lower):
|
|
"""sytrd: Reduction of a symmetric (Hermitian) matrix to tridiagonal form."""
|
|
a_type = ir.RankedTensorType(a.type)
|
|
dims = a_type.shape
|
|
assert len(dims) >= 2
|
|
m, n = dims[-2:]
|
|
assert m == n, (m, n)
|
|
batch_dims = tuple(dims[:-2])
|
|
num_bd = len(batch_dims)
|
|
b = 1
|
|
for d in batch_dims:
|
|
b *= d
|
|
|
|
lwork, opaque = gpu_solver.build_sytrd_descriptor(dtype, lower, b, n)
|
|
if np.issubdtype(dtype, np.floating):
|
|
diag_type = a_type.element_type
|
|
elif dtype == np.complex64:
|
|
diag_type = ir.F32Type.get()
|
|
elif dtype == np.complex128:
|
|
diag_type = ir.F64Type.get()
|
|
else:
|
|
raise NotImplementedError(f"Unsupported dtype {dtype}")
|
|
|
|
layout = (num_bd, num_bd + 1) + tuple(range(num_bd - 1, -1, -1))
|
|
i32_type = ir.IntegerType.get_signless(32)
|
|
a, d, e, taus, info, _ = custom_call(
|
|
f"{platform}solver_sytrd",
|
|
result_types=[
|
|
a.type,
|
|
ir.RankedTensorType.get(batch_dims + (n,), diag_type),
|
|
ir.RankedTensorType.get(batch_dims + (n - 1,), diag_type),
|
|
ir.RankedTensorType.get(batch_dims + (n - 1,), a_type.element_type),
|
|
ir.RankedTensorType.get(batch_dims, i32_type),
|
|
ir.RankedTensorType.get([lwork], a_type.element_type),
|
|
],
|
|
operands=[a],
|
|
backend_config=opaque,
|
|
operand_layouts=[layout],
|
|
result_layouts=[
|
|
layout,
|
|
(num_bd,) + tuple(range(num_bd - 1, -1, -1)),
|
|
(num_bd,) + tuple(range(num_bd - 1, -1, -1)),
|
|
(num_bd,) + tuple(range(num_bd - 1, -1, -1)),
|
|
tuple(range(num_bd - 1, -1, -1)),
|
|
[0],
|
|
],
|
|
operand_output_aliases={0: 0},
|
|
).results
|
|
# Workaround for NVIDIA partners bug #3865118: sytrd returns an incorrect "1"
|
|
# in the first element of the superdiagonal in the `a` matrix in the
|
|
# lower=False case. The correct result is returned in the `e` vector so we can
|
|
# simply copy it back to where it needs to be:
|
|
intattr = lambda xs: ir.DenseIntElementsAttr.get(np.asarray(xs, np.int64))
|
|
intarrattr = lambda xs: dense_int_array(np.asarray(xs, np.int64))
|
|
if not lower and platform == "cu" and m > 1:
|
|
start = (0,) * len(batch_dims) + (0,)
|
|
end = batch_dims + (1,)
|
|
s = hlo.slice(
|
|
e, intarrattr(start), intarrattr(end), intarrattr([1] * len(start)))
|
|
s_type = ir.RankedTensorType.get(batch_dims + (1, 1), diag_type)
|
|
s = hlo.broadcast_in_dim(s_type, s, intarrattr(range(len(dims) - 1)))
|
|
# The diagonals are always real; convert to complex if needed.
|
|
s = hlo.convert(
|
|
ir.RankedTensorType.get(s_type.shape, a_type.element_type), s)
|
|
offsets = tuple(hlo.constant(intattr(i))
|
|
for i in ((0,) * len(batch_dims) + (0, 1)))
|
|
a = hlo.dynamic_update_slice(a, s, offsets)
|
|
|
|
return a, d, e, taus, info
|
|
|
|
cuda_sytrd = partial(_sytrd_hlo, "cu", _cusolver)
|
|
rocm_sytrd = partial(_sytrd_hlo, "hip", _hipsolver)
|