mirror of
https://github.com/ROCm/jax.git
synced 2025-04-16 03:46:06 +00:00
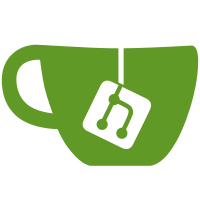
... in preparation for paring down `jax.interpreters.batching`'s exported symbols. PiperOrigin-RevId: 508487887
2727 lines
125 KiB
Python
2727 lines
125 KiB
Python
# Copyright 2021 The JAX Authors.
|
||
#
|
||
# Licensed under the Apache License, Version 2.0 (the "License");
|
||
# you may not use this file except in compliance with the License.
|
||
# You may obtain a copy of the License at
|
||
#
|
||
# https://www.apache.org/licenses/LICENSE-2.0
|
||
#
|
||
# Unless required by applicable law or agreed to in writing, software
|
||
# distributed under the License is distributed on an "AS IS" BASIS,
|
||
# WITHOUT WARRANTIES OR CONDITIONS OF ANY KIND, either express or implied.
|
||
# See the License for the specific language governing permissions and
|
||
# limitations under the License.
|
||
|
||
"""BCOO (Bached coordinate format) matrix object and associated primitives."""
|
||
from __future__ import annotations
|
||
|
||
import functools
|
||
from functools import partial
|
||
import operator
|
||
from typing import Any, List, NamedTuple, Optional, Protocol, Sequence, Tuple, Union
|
||
import warnings
|
||
|
||
import numpy as np
|
||
|
||
import jax
|
||
from jax import core
|
||
from jax import lax
|
||
from jax import tree_util
|
||
from jax import vmap
|
||
from jax.config import config
|
||
from jax.experimental.sparse._base import JAXSparse
|
||
from jax.experimental.sparse.util import (
|
||
nfold_vmap, _count_stored_elements,
|
||
_dot_general_validated_shape, CuSparseEfficiencyWarning,
|
||
SparseEfficiencyError, SparseEfficiencyWarning, Shape,
|
||
SparseInfo)
|
||
from jax.interpreters import partial_eval as pe
|
||
from jax._src.interpreters import mlir
|
||
import jax.numpy as jnp
|
||
from jax.util import safe_zip, unzip2, split_list
|
||
from jax._src import api_util
|
||
from jax._src.interpreters import ad
|
||
from jax._src.interpreters import batching
|
||
from jax._src.lax.lax import (
|
||
_const, ranges_like, remaining, _dot_general_batch_dim_nums, _dot_general_shape_rule,
|
||
DotDimensionNumbers)
|
||
from jax._src.lax.slicing import GatherDimensionNumbers, GatherScatterMode
|
||
from jax._src.lib.mlir import ir
|
||
from jax._src.lib import xla_bridge
|
||
from jax._src.lib import gpu_sparse
|
||
from jax._src.lib.mlir.dialects import hlo
|
||
from jax._src.numpy.setops import _unique
|
||
from jax._src.typing import Array, ArrayLike, DType, DTypeLike
|
||
from jax._src.util import canonicalize_axis
|
||
|
||
|
||
def _bcoo_batch_dims_to_front(batched_args, batch_dims, spinfo, batch_size=None):
|
||
data, indices = batched_args
|
||
data_bdim, indices_bdim = batch_dims
|
||
n_batch = indices.ndim - 2 + bool(indices_bdim is None)
|
||
if not all(b is None or 0 <= b < n_batch for b in batch_dims):
|
||
raise NotImplementedError("batch_dims must be None or satisfy 0 < dim < n_batch. "
|
||
f"Got {batch_dims=} for {n_batch=}.")
|
||
batched_data, batched_indices = [
|
||
lax.expand_dims(arg, [0]) if bdim is None else jnp.moveaxis(arg, bdim, 0)
|
||
for arg, bdim in [(data, data_bdim), (indices, indices_bdim)]]
|
||
if batch_size is None:
|
||
batch_size = max(arg.shape[dim] for arg, dim in zip((data, indices), batch_dims) if dim is not None)
|
||
batched_spinfo = SparseInfo((batch_size, *spinfo.shape),
|
||
indices_sorted=spinfo.indices_sorted,
|
||
unique_indices=spinfo.unique_indices)
|
||
return batched_data, batched_indices, batched_spinfo
|
||
|
||
|
||
#----------------------------------------------------------------------
|
||
# BCOO primitives: batched extension of COO.
|
||
|
||
def _bcoo_set_nse(mat: BCOO, nse: int) -> BCOO:
|
||
"""Return a copy of `mat` with the specified nse.
|
||
Note that if nse < mat.nse, this will potentially discard data.
|
||
"""
|
||
nse = operator.index(nse)
|
||
assert nse >= 0
|
||
if mat.nse == nse:
|
||
return mat
|
||
if nse <= mat.nse:
|
||
data = mat.data[(*(slice(None) for i in range(mat.n_batch)), slice(nse))]
|
||
indices = mat.indices[..., :nse, :]
|
||
else:
|
||
data = jnp.zeros_like(mat.data, shape=(*mat.data.shape[:mat.n_batch], nse, *mat.data.shape[mat.n_batch + 1:]))
|
||
data = data.at[(*(slice(None) for i in range(mat.n_batch)), slice(mat.nse))].set(mat.data)
|
||
indices = jnp.zeros_like(mat.indices, shape=(*mat.indices.shape[:-2], nse, mat.indices.shape[-1]))
|
||
indices = indices.at[..., :mat.nse, :].set(mat.indices)
|
||
indices = indices.at[..., mat.nse:, :].set(jnp.array(mat.shape[mat.n_batch:mat.n_batch + mat.n_sparse],
|
||
dtype=indices.dtype))
|
||
return BCOO((data, indices), shape=mat.shape,
|
||
indices_sorted=mat.indices_sorted,
|
||
unique_indices=mat.unique_indices)
|
||
|
||
# TODO(jakevdp) this can be problematic when used with autodiff; see
|
||
# https://github.com/google/jax/issues/10163. Should this be a primitive?
|
||
# Alternatively, maybe roll this into bcoo_sum_duplicates as an optional argument.
|
||
def bcoo_eliminate_zeros(mat: BCOO, nse: Optional[int] = None) -> BCOO:
|
||
data, indices, shape = mat.data, mat.indices, mat.shape
|
||
props = _validate_bcoo(data, indices, shape)
|
||
mask = (data == 0).all(tuple(range(props.n_batch + 1, data.ndim)))
|
||
dims_to_contract = tuple(i for i, s in enumerate(indices.shape[:props.n_batch]) if s == 1)
|
||
mask = mask.all(dims_to_contract, keepdims=True)
|
||
fill_value = jnp.array(shape[props.n_batch:props.n_batch + props.n_sparse], dtype=indices.dtype)
|
||
f = lambda i, m: jnp.where(m[:, None], fill_value[None, :], i)
|
||
indices = nfold_vmap(f, props.n_batch)(indices, mask)
|
||
return bcoo_sum_duplicates(BCOO((data, indices), shape=shape), nse=nse)
|
||
|
||
|
||
class BCOOProperties(NamedTuple):
|
||
n_batch: int
|
||
n_sparse: int
|
||
n_dense: int
|
||
nse: int
|
||
|
||
class Buffer(Protocol):
|
||
@property
|
||
def shape(self) -> Shape: ...
|
||
@property
|
||
def dtype(self) -> Any: ...
|
||
|
||
|
||
def _validate_bcoo(data: Buffer, indices: Buffer, shape: Sequence[int]) -> BCOOProperties:
|
||
props = _validate_bcoo_indices(indices, shape)
|
||
n_batch, n_sparse, n_dense, nse = props
|
||
shape = tuple(shape)
|
||
if any(s1 not in (1, s2) for s1, s2 in safe_zip(data.shape[:n_batch], shape[:n_batch])):
|
||
raise ValueError(f"data batch dimensions not compatible for {data.shape=}, {shape=}")
|
||
if data.shape[n_batch:] != (nse,) + shape[n_batch + n_sparse:]:
|
||
raise ValueError(f"Invalid {data.shape=} for {nse=}, {n_batch=}, {n_dense=}")
|
||
return props
|
||
|
||
|
||
def _validate_bcoo_indices(indices: Buffer, shape: Sequence[int]) -> BCOOProperties:
|
||
assert jnp.issubdtype(indices.dtype, jnp.integer)
|
||
shape = tuple(shape)
|
||
nse, n_sparse = indices.shape[-2:]
|
||
n_batch = len(indices.shape) - 2
|
||
n_dense = len(shape) - n_batch - n_sparse
|
||
assert n_dense >= 0
|
||
if any(s1 not in (1, s2) for s1, s2 in safe_zip(indices.shape[:n_batch], shape[:n_batch])):
|
||
raise ValueError(f"indices batch dimensions not compatible for {indices.shape=}, {shape=}")
|
||
if indices.shape[n_batch:] != (nse, n_sparse):
|
||
raise ValueError(f"Invalid ={indices.shape=} for {nse=}, {n_batch=}, {n_dense=}")
|
||
return BCOOProperties(n_batch=n_batch, n_sparse=n_sparse, n_dense=n_dense, nse=nse)
|
||
|
||
|
||
#----------------------------------------------------------------------
|
||
# bcoo_todense
|
||
|
||
bcoo_todense_p = core.Primitive('bcoo_todense')
|
||
|
||
def bcoo_todense(mat: BCOO) -> Array:
|
||
"""Convert batched sparse matrix to a dense matrix.
|
||
|
||
Args:
|
||
mat: BCOO matrix.
|
||
|
||
Returns:
|
||
mat_dense: dense version of ``mat``.
|
||
"""
|
||
return _bcoo_todense(mat.data, mat.indices, spinfo=mat._info)
|
||
|
||
def _bcoo_todense(data: Array, indices: Array, *, spinfo: SparseInfo
|
||
) -> Array:
|
||
"""Convert batched sparse matrix to a dense matrix.
|
||
|
||
Args:
|
||
data : array of shape ``batch_dims + (nse,) + block_dims``.
|
||
indices : array of shape ``batch_dims + (n_sparse, nse)``
|
||
spinfo : SparseInfo. In particular, this includes the shape
|
||
of the matrix, which is equal to ``batch_dims + sparse_dims + block_dims``
|
||
where ``len(sparse_dims) == n_sparse``
|
||
|
||
Returns:
|
||
mat : array with specified shape and dtype matching ``data``
|
||
"""
|
||
return bcoo_todense_p.bind(jnp.asarray(data), jnp.asarray(indices), spinfo=spinfo)
|
||
|
||
@bcoo_todense_p.def_impl
|
||
def _bcoo_todense_impl(data, indices, *, spinfo):
|
||
shape = spinfo.shape
|
||
n_batch, n_sparse, _, _ = _validate_bcoo(data, indices, shape)
|
||
|
||
ind_slices = tuple(np.zeros(s, int) if i_s == 1 else np.arange(s)
|
||
for s, i_s in zip(shape[:n_batch], indices.shape[:n_batch]))
|
||
grid = tuple(np.meshgrid(*ind_slices, indexing='ij', sparse=True))
|
||
sparse_ind = tuple(indices[grid + (slice(None), i)] for i in range(n_sparse))
|
||
|
||
batch_slices = tuple(np.arange(s) for s in shape[:n_batch])
|
||
grid = np.meshgrid(*batch_slices, np.arange(1), indexing='ij', sparse=True)
|
||
batch_ind = tuple(grid)[:-1]
|
||
|
||
if not sparse_ind:
|
||
data = data.sum(n_batch, keepdims=bool(batch_ind), dtype=data.dtype)
|
||
return jnp.zeros(shape, data.dtype).at[batch_ind + sparse_ind].add(data)
|
||
|
||
@bcoo_todense_p.def_abstract_eval
|
||
def _bcoo_todense_abstract_eval(data, indices, *, spinfo):
|
||
shape = spinfo.shape
|
||
_validate_bcoo(data, indices, shape)
|
||
return core.ShapedArray(shape, data.dtype)
|
||
|
||
def _bcoo_todense_jvp(data_dot, data, indices, *, spinfo):
|
||
return _bcoo_todense(data_dot, indices, spinfo=spinfo)
|
||
|
||
def _bcoo_todense_transpose(ct, data, indices, *, spinfo):
|
||
shape = spinfo.shape
|
||
assert ad.is_undefined_primal(data)
|
||
if ad.is_undefined_primal(indices):
|
||
raise ValueError("Cannot transpose with respect to sparse indices")
|
||
assert ct.shape == shape
|
||
assert ct.dtype == data.aval.dtype
|
||
return _bcoo_extract(indices, ct), indices
|
||
|
||
def _bcoo_todense_batching_rule(batched_args, batch_dims, *, spinfo):
|
||
data, indices, spinfo = _bcoo_batch_dims_to_front(batched_args, batch_dims, spinfo)
|
||
return _bcoo_todense(data, indices, spinfo=spinfo), 0
|
||
|
||
ad.defjvp(bcoo_todense_p, _bcoo_todense_jvp, None)
|
||
ad.primitive_transposes[bcoo_todense_p] = _bcoo_todense_transpose
|
||
batching.primitive_batchers[bcoo_todense_p] = _bcoo_todense_batching_rule
|
||
mlir.register_lowering(bcoo_todense_p, mlir.lower_fun(
|
||
_bcoo_todense_impl, multiple_results=False))
|
||
|
||
#--------------------------------------------------------------------
|
||
# bcoo_fromdense
|
||
|
||
bcoo_fromdense_p = core.Primitive('bcoo_fromdense')
|
||
bcoo_fromdense_p.multiple_results = True
|
||
|
||
_TRACED_NSE_ERROR = """
|
||
The error arose for the nse argument of bcoo_fromdense. In order for
|
||
BCOO.fromdense() to be used in traced/compiled code, you must pass a concrete
|
||
value to the nse (number of stored elements) argument.
|
||
"""
|
||
|
||
def bcoo_fromdense(mat: Array, *, nse: Optional[int] = None, n_batch: int = 0,
|
||
n_dense: int = 0, index_dtype: DTypeLike = jnp.int32) -> BCOO:
|
||
"""Create BCOO-format sparse matrix from a dense matrix.
|
||
|
||
Args:
|
||
mat : array to be converted to BCOO.
|
||
nse : number of specified elements in each batch
|
||
n_batch : number of batch dimensions (default: 0)
|
||
n_dense : number of block_dimensions (default: 0)
|
||
index_dtype : dtype of sparse indices (default: int32)
|
||
|
||
Returns:
|
||
mat_bcoo: BCOO representation of the matrix.
|
||
"""
|
||
mat = jnp.asarray(mat)
|
||
if nse is None:
|
||
nse = _count_stored_elements(mat, n_batch, n_dense)
|
||
nse_int = core.concrete_or_error(operator.index, nse, _TRACED_NSE_ERROR)
|
||
return BCOO(_bcoo_fromdense(mat, nse=nse_int, n_batch=n_batch, n_dense=n_dense,
|
||
index_dtype=index_dtype),
|
||
shape=mat.shape, indices_sorted=True, unique_indices=True)
|
||
|
||
def _bcoo_fromdense(mat: Array, *, nse: int, n_batch: int = 0, n_dense: int = 0,
|
||
index_dtype: DTypeLike = jnp.int32) -> Tuple[Array, Array]:
|
||
"""Create BCOO-format sparse matrix from a dense matrix.
|
||
|
||
Args:
|
||
mat : array to be converted to BCOO, with ``ndim = n_batch + n_sparse + n_dense``.
|
||
nse : number of specified elements in each batch
|
||
n_batch : number of batch dimensions (default: 0)
|
||
n_dense : number of block_dimensions (default: 0)
|
||
index_dtype : dtype of sparse indices (default: int32)
|
||
|
||
Returns:
|
||
data : array of shape ``mat.shape[:n_batch] + (nse,) + mat.shape[mat.ndim - n_dense:]``
|
||
and dtype ``mat.dtype``
|
||
indices : array of shape ``mat.shape[:n_batch] + (n_sparse, nse)``
|
||
"""
|
||
mat = jnp.asarray(mat)
|
||
nse = core.concrete_or_error(operator.index, nse, _TRACED_NSE_ERROR)
|
||
return bcoo_fromdense_p.bind(mat, nse=nse, n_batch=n_batch, n_dense=n_dense,
|
||
index_dtype=index_dtype)
|
||
|
||
@bcoo_fromdense_p.def_impl
|
||
def _bcoo_fromdense_impl(mat, *, nse, n_batch, n_dense, index_dtype):
|
||
mat = jnp.asarray(mat)
|
||
n_sparse = mat.ndim - n_dense - n_batch
|
||
mask = (mat != 0)
|
||
if n_dense > 0:
|
||
mask = mask.any([-(i + 1) for i in range(n_dense)])
|
||
@partial(nfold_vmap, N=n_batch, broadcasted=False)
|
||
def _nonzero(a):
|
||
if a.ndim:
|
||
return jnp.nonzero(a, size=nse, fill_value=a.shape[:n_sparse])
|
||
return ()
|
||
indices = _nonzero(mask)
|
||
if not indices:
|
||
indices = jnp.zeros(mask.shape[:n_batch] + (nse, 0), index_dtype)
|
||
else:
|
||
indices = jnp.moveaxis(jnp.array(indices, index_dtype), 0, n_batch + 1)
|
||
data = _bcoo_extract(indices, mat)
|
||
|
||
true_nse = mask.sum(list(range(n_batch, mask.ndim)))[..., None]
|
||
true_nonzeros = lax.broadcasted_iota(true_nse.dtype, (1,) * n_batch + (nse,), n_batch) < true_nse
|
||
true_nonzeros = true_nonzeros[(n_batch + 1) * (slice(None),) + n_dense * (None,)]
|
||
data = jnp.where(true_nonzeros, data, 0)
|
||
|
||
return data, indices
|
||
|
||
@bcoo_fromdense_p.def_abstract_eval
|
||
def _bcoo_fromdense_abstract_eval(mat, *, nse, n_batch, n_dense, index_dtype):
|
||
n_sparse = mat.ndim - n_batch - n_dense
|
||
data_shape = mat.shape[:n_batch] + (nse,) + mat.shape[n_batch + n_sparse:]
|
||
index_shape = mat.shape[:n_batch] + (nse, n_sparse)
|
||
return core.ShapedArray(data_shape, mat.dtype), core.ShapedArray(index_shape, index_dtype)
|
||
|
||
def _bcoo_fromdense_jvp(primals, tangents, *, nse, n_batch, n_dense, index_dtype):
|
||
M, = primals
|
||
Mdot, = tangents
|
||
|
||
primals_out = _bcoo_fromdense(M, nse=nse, n_batch=n_batch, n_dense=n_dense, index_dtype=index_dtype)
|
||
data, indices = primals_out
|
||
|
||
if type(Mdot) is ad.Zero:
|
||
data_dot = ad.Zero.from_value(data)
|
||
else:
|
||
data_dot = _bcoo_extract(indices, Mdot)
|
||
|
||
tangents_out = (data_dot, ad.Zero.from_value(indices))
|
||
|
||
return primals_out, tangents_out
|
||
|
||
def _bcoo_fromdense_transpose(ct, M, *, nse, n_batch, n_dense, index_dtype):
|
||
data, indices = ct
|
||
n_sparse = M.ndim - n_batch - n_dense
|
||
assert data.shape == M.shape[:n_batch] + (nse,) + M.shape[n_batch + n_sparse:]
|
||
assert indices.shape == M.shape[:n_batch] + (n_sparse, nse)
|
||
assert indices.dtype == index_dtype
|
||
if isinstance(indices, ad.Zero):
|
||
raise ValueError("Cannot transpose with respect to sparse indices")
|
||
assert ad.is_undefined_primal(M)
|
||
return _bcoo_todense(data, indices, spinfo=SparseInfo(M.aval.shape))
|
||
|
||
def _bcoo_fromdense_batching_rule(batched_args, batch_dims, *, nse, n_batch, n_dense, index_dtype):
|
||
M, = batched_args
|
||
bdim, = batch_dims
|
||
if not (0 <= bdim <= n_batch):
|
||
raise ValueError(f"Expected 0 < bdim <= n_batch; got {bdim=}, {n_batch=}")
|
||
return _bcoo_fromdense(M, nse=nse, n_batch=n_batch + 1, n_dense=n_dense, index_dtype=index_dtype), (bdim, bdim)
|
||
|
||
ad.primitive_jvps[bcoo_fromdense_p] = _bcoo_fromdense_jvp
|
||
ad.primitive_transposes[bcoo_fromdense_p] = _bcoo_fromdense_transpose
|
||
batching.primitive_batchers[bcoo_fromdense_p] = _bcoo_fromdense_batching_rule
|
||
mlir.register_lowering(bcoo_fromdense_p, mlir.lower_fun(
|
||
_bcoo_fromdense_impl, multiple_results=True))
|
||
|
||
#----------------------------------------------------------------------
|
||
# bcoo_extract
|
||
|
||
bcoo_extract_p = core.Primitive('bcoo_extract')
|
||
|
||
|
||
def bcoo_extract(sparr: BCOO, arr: ArrayLike, *, assume_unique: Optional[bool] = None) -> BCOO:
|
||
"""Extract values from a dense array according to the sparse array's indices.
|
||
|
||
Args:
|
||
sparr : BCOO array whose indices will be used for the output.
|
||
arr : ArrayLike with shape equal to self.shape
|
||
assume_unique : bool, defaults to sparr.unique_indices
|
||
If True, extract values for every index, even if index contains duplicates.
|
||
If False, duplicate indices will have their values summed and returned in
|
||
the position of the first index.
|
||
|
||
Returns:
|
||
extracted : a BCOO array with the same sparsity pattern as self.
|
||
"""
|
||
if not isinstance(sparr, BCOO):
|
||
raise ValueError(f"First argument to bcoo_extract should be a BCOO array. Got {type(sparr)=}")
|
||
arr = jnp.asarray(arr)
|
||
if arr.shape != sparr.shape:
|
||
raise ValueError(f"shape mismatch: {sparr.shape=} {arr.shape=}")
|
||
if assume_unique is None:
|
||
assume_unique = sparr.unique_indices
|
||
data = _bcoo_extract(sparr.indices, arr, assume_unique=assume_unique)
|
||
return BCOO((data, sparr.indices), **sparr._info._asdict())
|
||
|
||
|
||
def _bcoo_extract(indices: Array, arr: Array, *, assume_unique=True) -> Array:
|
||
"""Extract BCOO data values from a dense array at given BCOO indices.
|
||
|
||
Args:
|
||
indices: An ndarray; see BCOO indices.
|
||
arr: A dense array.
|
||
assume_unique: bool, default=True
|
||
If True, then indices will be assumed unique and a value will be extracted
|
||
from arr for each index. Otherwise, extra work will be done to de-duplicate
|
||
indices to zero-out duplicate extracted values.
|
||
|
||
Returns:
|
||
An ndarray; see BCOO data.
|
||
"""
|
||
return bcoo_extract_p.bind(indices, arr, assume_unique=assume_unique)
|
||
|
||
@bcoo_extract_p.def_impl
|
||
def _bcoo_extract_impl(indices, arr, *, assume_unique):
|
||
arr = jnp.asarray(arr)
|
||
props = _validate_bcoo_indices(indices, arr.shape)
|
||
if not assume_unique:
|
||
indices, sort_ind = _unique_indices(indices, shape=arr.shape, return_index=True)
|
||
original_props = props
|
||
props = _validate_bcoo_indices(indices, arr.shape)
|
||
|
||
ind_slices = tuple(np.zeros(s, int) if i_s == 1 else np.arange(s)
|
||
for s, i_s in zip(arr.shape[:props.n_batch], indices.shape[:props.n_batch]))
|
||
grid = tuple(np.meshgrid(*ind_slices, indexing='ij', sparse=True))
|
||
sparse_ind = tuple(indices[grid + (slice(None), i)] for i in range(props.n_sparse))
|
||
|
||
batch_slices = tuple(np.arange(s) for s in arr.shape[:props.n_batch])
|
||
grid = np.meshgrid(*batch_slices, np.arange(1), indexing='ij', sparse=True)
|
||
batch_ind = tuple(grid)[:-1]
|
||
|
||
if not sparse_ind + batch_ind:
|
||
result = arr[None]
|
||
else:
|
||
result = arr.at[batch_ind + sparse_ind].get(mode='fill', fill_value=0)
|
||
if props.n_sparse == 0 and props.nse != 1:
|
||
if assume_unique:
|
||
result = lax.broadcast_in_dim(
|
||
result, _tuple_replace(result.shape, props.n_batch, props.nse), range(result.ndim))
|
||
else:
|
||
out_shape = _tuple_replace(result.shape, props.n_batch, original_props.nse)
|
||
ind = props.n_batch * (slice(None),) + (slice(1),)
|
||
result = jnp.zeros_like(result, shape=out_shape).at[ind].set(result)
|
||
if not assume_unique:
|
||
unbatched_out_shape = (original_props.nse, *result.shape[props.n_batch + 1:])
|
||
def f(r, i):
|
||
return jnp.zeros_like(r, shape=unbatched_out_shape).at[i].add(r)
|
||
for _ in range(props.n_batch):
|
||
f = vmap(f)
|
||
result = f(result, sort_ind)
|
||
return result
|
||
|
||
@bcoo_extract_p.def_abstract_eval
|
||
def _bcoo_extract_abstract_eval(indices, arr, *, assume_unique):
|
||
_ = bool(assume_unique)
|
||
n_batch, _, n_dense, nse = _validate_bcoo_indices(indices, arr.shape)
|
||
out_shape = arr.shape[:n_batch] + (nse,) + arr.shape[arr.ndim - n_dense:]
|
||
return core.ShapedArray(out_shape, arr.dtype)
|
||
|
||
def _bcoo_extract_jvp(arr_dot, indices, arr, *, assume_unique):
|
||
assert arr_dot.shape == arr.shape
|
||
return _bcoo_extract(indices, arr_dot, assume_unique=assume_unique)
|
||
|
||
def _bcoo_extract_transpose(ct, indices, arr, *, assume_unique):
|
||
if not assume_unique:
|
||
raise NotImplementedError("transpose of bcoo_extract with assume_unique=False")
|
||
assert ad.is_undefined_primal(arr)
|
||
if ad.is_undefined_primal(indices):
|
||
raise ValueError("Cannot transpose with respect to sparse indices")
|
||
assert ct.dtype == arr.aval.dtype
|
||
return indices, _bcoo_todense(ct, indices, spinfo=SparseInfo(arr.aval.shape))
|
||
|
||
def _bcoo_extract_batching_rule(batched_args, batch_dims, *, assume_unique):
|
||
indices, arr = batched_args
|
||
assert any(b is not None for b in batch_dims)
|
||
if batch_dims[0] is None:
|
||
bdim = batch_dims[1]
|
||
indices = lax.expand_dims(indices, (bdim,))
|
||
elif batch_dims[1] is None:
|
||
# TODO(jakevdp) can we handle this case without explicit broadcasting?
|
||
bdim = batch_dims[0]
|
||
result_shape = list(arr.shape)
|
||
result_shape.insert(bdim, indices.shape[bdim])
|
||
arr = lax.broadcast_in_dim(arr, result_shape, (bdim,))
|
||
else:
|
||
if batch_dims[0] != batch_dims[1]:
|
||
raise NotImplementedError("bcoo_extract with unequal batch dimensions.")
|
||
bdim = batch_dims[0]
|
||
n_batch = indices.ndim - 2
|
||
if bdim >= n_batch:
|
||
raise ValueError(f"{batch_dims=} out of range for indices with {n_batch=}")
|
||
return _bcoo_extract(indices, arr, assume_unique=assume_unique), bdim
|
||
|
||
ad.defjvp(bcoo_extract_p, None, _bcoo_extract_jvp)
|
||
ad.primitive_transposes[bcoo_extract_p] = _bcoo_extract_transpose
|
||
batching.primitive_batchers[bcoo_extract_p] = _bcoo_extract_batching_rule
|
||
mlir.register_lowering(bcoo_extract_p, mlir.lower_fun(
|
||
_bcoo_extract_impl, multiple_results=False))
|
||
|
||
#----------------------------------------------------------------------
|
||
# bcoo_transpose
|
||
# transpose of a BCOO array
|
||
|
||
bcoo_transpose_p = core.Primitive('bcoo_transpose')
|
||
bcoo_transpose_p.multiple_results = True
|
||
|
||
def bcoo_transpose(mat: BCOO, *, permutation: Sequence[int]) -> BCOO:
|
||
"""Transpose a BCOO-format array.
|
||
|
||
Args:
|
||
mat: A BCOO-format array.
|
||
permutation: A tuple or list or ndarray which contains a permutation of
|
||
[0,1,..,N-1] where N is the number of axes of ``mat`` in the order of
|
||
batch, sparse, and dense dimensions. The i’th axis of the returned array
|
||
corresponds to the axis numbered permutation[i] of ``mat``. Transpose
|
||
permutation currently does not support permuting batch axes with non-batch
|
||
axes nor permutating dense axes with non-dense axes.
|
||
|
||
Returns:
|
||
A BCOO-format array.
|
||
"""
|
||
buffers = _bcoo_transpose(mat.data, mat.indices, permutation=permutation, spinfo=mat._info)
|
||
out_shape = tuple(mat.shape[p] for p in permutation)
|
||
return BCOO(buffers, shape=out_shape, unique_indices=mat.unique_indices)
|
||
|
||
def _bcoo_transpose(data: Array, indices: Array, *,
|
||
permutation: Sequence[int], spinfo: SparseInfo) -> Tuple[Array, Array]:
|
||
permutation = tuple(permutation)
|
||
if permutation == tuple(range(len(spinfo.shape))):
|
||
return data, indices
|
||
else:
|
||
return bcoo_transpose_p.bind(data, indices, permutation=permutation,
|
||
spinfo=spinfo)
|
||
|
||
def _validate_permutation(data, indices, permutation, shape):
|
||
if not isinstance(permutation, (tuple, list, np.ndarray)):
|
||
raise TypeError(f"transpose permutation must be a tuple/list/ndarray, got {type(permutation)}.")
|
||
if tuple(sorted(permutation)) != tuple(range(len(shape))):
|
||
raise TypeError("transpose permutation isn't a permutation of operand dimensions, "
|
||
f"got permutation {permutation} for shape {shape}.")
|
||
n_batch, n_sparse, n_dense, _ = _validate_bcoo(data, indices, shape)
|
||
batch_perm = permutation[:n_batch]
|
||
sparse_perm = [p - n_batch for p in permutation[n_batch: n_batch + n_sparse]]
|
||
dense_perm = [p - n_sparse - n_batch for p in permutation[n_batch + n_sparse:]]
|
||
if n_batch and tuple(sorted(batch_perm)) != tuple(range(n_batch)):
|
||
raise NotImplementedError("transpose permutation cannot permute batch axes with non-batch axes; "
|
||
f"got permutation {permutation}, with {n_batch=}.")
|
||
if n_dense and tuple(sorted(dense_perm)) != tuple(range(n_dense)):
|
||
raise NotImplementedError("transpose permutation cannot permute dense axes with non-dense axes; "
|
||
f"got permutation {permutation}, with {n_dense=}.")
|
||
return batch_perm, sparse_perm, dense_perm
|
||
|
||
@bcoo_transpose_p.def_impl
|
||
def _bcoo_transpose_impl(data, indices, *, permutation: Sequence[int], spinfo: SparseInfo):
|
||
batch_perm, sparse_perm, dense_perm = _validate_permutation(data, indices, permutation, spinfo.shape)
|
||
n_batch = len(batch_perm)
|
||
indices = indices[..., sparse_perm].transpose(*batch_perm, n_batch, n_batch + 1)
|
||
data = data.transpose(*batch_perm, n_batch, *(d + n_batch + 1 for d in dense_perm))
|
||
return data, indices
|
||
|
||
@bcoo_transpose_p.def_abstract_eval
|
||
def _bcoo_transpose_abstract_eval(data, indices, *, permutation: Sequence[int], spinfo: SparseInfo):
|
||
batch_perm, _, dense_perm = _validate_permutation(data, indices, permutation, spinfo.shape)
|
||
n_batch = len(batch_perm)
|
||
indices_shape = np.array(indices.shape)[[*batch_perm, n_batch, n_batch + 1]]
|
||
data_shape = np.array(data.shape)[[*batch_perm, n_batch, *(d + n_batch + 1 for d in dense_perm)]]
|
||
return core.ShapedArray(data_shape, data.dtype), core.ShapedArray(indices_shape, indices.dtype)
|
||
|
||
def _bcoo_transpose_jvp(primals, tangents, *, permutation: Sequence[int], spinfo: SparseInfo):
|
||
data, indices = primals
|
||
data_dot, _ = tangents
|
||
primals_out = _bcoo_transpose(data, indices, permutation=permutation, spinfo=spinfo)
|
||
data_dot_out, _ = _bcoo_transpose(data_dot, indices, permutation=permutation, spinfo=spinfo)
|
||
return primals_out, (data_dot_out, ad.Zero.from_value(indices))
|
||
|
||
def _bcoo_transpose_transpose(ct, data, indices, *, permutation: Sequence[int], spinfo: SparseInfo):
|
||
data_ct, indices_ct = ct
|
||
assert isinstance(indices_ct, ad.Zero)
|
||
if ad.is_undefined_primal(indices):
|
||
raise ValueError("Cannot transpose with respect to sparse indices")
|
||
assert data_ct.dtype == data.aval.dtype
|
||
ct_spinfo = SparseInfo(tuple(spinfo.shape[p] for p in permutation))
|
||
rev_permutation = list(np.argsort(permutation))
|
||
# TODO(jakevdp) avoid dummy indices?
|
||
dummy_indices = jnp.zeros([1 for i in range(indices.ndim - 2)] + list(indices.shape[-2:]), dtype=int)
|
||
data_trans, _ = _bcoo_transpose(data_ct, dummy_indices, permutation=rev_permutation, spinfo=ct_spinfo)
|
||
return data_trans, indices_ct
|
||
|
||
def _bcoo_transpose_batch_rule(batched_args, batch_dims, *, permutation: Sequence[int], spinfo: SparseInfo):
|
||
data, indices, spinfo = _bcoo_batch_dims_to_front(batched_args, batch_dims, spinfo)
|
||
batched_permutation = (0, *(p + 1 for p in permutation))
|
||
data, indices = _bcoo_transpose(data, indices, permutation=batched_permutation, spinfo=spinfo)
|
||
batch_dims_out = [None if bdim is None else 0 for bdim in batch_dims]
|
||
args_out = [lax.squeeze(arg, [0]) if bdim is None else arg
|
||
for arg, bdim in zip((data, indices), batch_dims_out)]
|
||
return args_out, batch_dims_out
|
||
|
||
ad.primitive_jvps[bcoo_transpose_p] = _bcoo_transpose_jvp
|
||
ad.primitive_transposes[bcoo_transpose_p] = _bcoo_transpose_transpose
|
||
batching.primitive_batchers[bcoo_transpose_p] = _bcoo_transpose_batch_rule
|
||
mlir.register_lowering(bcoo_transpose_p, mlir.lower_fun(
|
||
_bcoo_transpose_impl, multiple_results=True))
|
||
|
||
#----------------------------------------------------------------------
|
||
# bcoo_dot_general
|
||
# (batched) general dot product of a BCOO sparse ND array and a dense ND array,
|
||
# returning a dense ND array.
|
||
|
||
bcoo_dot_general_p = core.Primitive('bcoo_dot_general')
|
||
|
||
def bcoo_dot_general(lhs: Union[BCOO, Array], rhs: Union[BCOO, Array], *, dimension_numbers: DotDimensionNumbers,
|
||
precision: None = None, preferred_element_type: None = None) -> Union[BCOO, Array]:
|
||
"""A general contraction operation.
|
||
|
||
Args:
|
||
lhs: An ndarray or BCOO-format sparse array.
|
||
rhs: An ndarray or BCOO-format sparse array..
|
||
dimension_numbers: a tuple of tuples of the form
|
||
`((lhs_contracting_dims, rhs_contracting_dims),
|
||
(lhs_batch_dims, rhs_batch_dims))`.
|
||
precision: unused
|
||
preferred_element_type: unused
|
||
|
||
Returns:
|
||
An ndarray or BCOO-format sparse array containing the result. If both inputs
|
||
are sparse, the result will be sparse, of type BCOO. If either input is dense,
|
||
the result will be dense, of type ndarray.
|
||
"""
|
||
# TODO(jakevdp) make use of these?
|
||
del precision, preferred_element_type # unused
|
||
if isinstance(lhs, BCOO) and isinstance(rhs, BCOO):
|
||
shape = _dot_general_validated_shape(lhs.shape, rhs.shape,
|
||
dimension_numbers)
|
||
bufs = _bcoo_spdot_general(lhs.data, lhs.indices, rhs.data, rhs.indices,
|
||
lhs_spinfo=lhs._info, rhs_spinfo=rhs._info,
|
||
dimension_numbers=dimension_numbers)
|
||
return BCOO(bufs, shape=shape)
|
||
elif isinstance(lhs, BCOO):
|
||
return _bcoo_dot_general(lhs.data, lhs.indices, rhs, dimension_numbers=dimension_numbers, # type: ignore[arg-type]
|
||
lhs_spinfo=lhs._info)
|
||
elif isinstance(rhs, BCOO):
|
||
return _bcoo_rdot_general(lhs, rhs.data, rhs.indices, dimension_numbers=dimension_numbers, # type: ignore[arg-type]
|
||
rhs_spinfo=rhs._info)
|
||
else:
|
||
return lax.dot_general(lhs, rhs, dimension_numbers=dimension_numbers)
|
||
|
||
def _bcoo_dot_general(lhs_data: Array, lhs_indices: Array, rhs: Array, *,
|
||
dimension_numbers: DotDimensionNumbers, lhs_spinfo: SparseInfo) -> Array:
|
||
(lhs_contract, rhs_contract), (lhs_batch, rhs_batch) = dimension_numbers
|
||
cdims = (api_util._ensure_index_tuple(lhs_contract),
|
||
api_util._ensure_index_tuple(rhs_contract))
|
||
bdims = (api_util._ensure_index_tuple(lhs_batch),
|
||
api_util._ensure_index_tuple(rhs_batch))
|
||
return bcoo_dot_general_p.bind(jnp.asarray(lhs_data), jnp.asarray(lhs_indices), jnp.asarray(rhs),
|
||
dimension_numbers=(cdims, bdims),
|
||
lhs_spinfo=lhs_spinfo)
|
||
|
||
def _bcoo_rdot_general(lhs: Array, rhs_data: Array, rhs_indices: Array, *,
|
||
dimension_numbers: DotDimensionNumbers, rhs_spinfo: SparseInfo) -> Array:
|
||
# TODO(jakevdp): perhaps this should be part of the bcoo_dot_general primitive?
|
||
dimension_numbers_reversed: DotDimensionNumbers = tuple(d[::-1] for d in dimension_numbers) # type: ignore[assignment]
|
||
result = _bcoo_dot_general(rhs_data, rhs_indices, lhs, lhs_spinfo=rhs_spinfo,
|
||
dimension_numbers=dimension_numbers_reversed)
|
||
n_contract, n_batch = (len(d[0]) for d in dimension_numbers)
|
||
n_swap = len(rhs_spinfo.shape) - n_contract
|
||
permutation = tuple([*range(n_batch), *range(n_swap, result.ndim), *range(n_batch, n_swap)])
|
||
return lax.transpose(result, permutation)
|
||
|
||
@bcoo_dot_general_p.def_impl
|
||
def _bcoo_dot_general_impl(lhs_data, lhs_indices, rhs, *, dimension_numbers, lhs_spinfo: SparseInfo):
|
||
lhs_data = jnp.asarray(lhs_data)
|
||
lhs_indices = jnp.asarray(lhs_indices)
|
||
rhs = jnp.asarray(rhs)
|
||
# Validate all inputs via abstract_eval
|
||
out_aval = _bcoo_dot_general_abstract_eval(lhs_data.aval, lhs_indices.aval, rhs.aval,
|
||
dimension_numbers=dimension_numbers,
|
||
lhs_spinfo=lhs_spinfo)
|
||
n_sparse = lhs_indices.shape[-1]
|
||
n_batch = lhs_indices.ndim - 2
|
||
|
||
(lhs_contracting, rhs_contracting), (lhs_batch, rhs_batch) = dimension_numbers
|
||
lhs_contracting_b, rhs_contracting_b = unzip2([
|
||
(l, r) for l, r in safe_zip(lhs_contracting, rhs_contracting) if l < n_batch])
|
||
lhs_contracting_s, rhs_contracting_s = unzip2([
|
||
(l, r) for l, r in safe_zip(lhs_contracting, rhs_contracting) if l >= n_batch])
|
||
|
||
# Reorder lhs batch dimensions
|
||
if lhs_batch or lhs_contracting_b:
|
||
batch_perm = [*lhs_batch, *remaining(range(n_batch), lhs_batch, lhs_contracting_b), *lhs_contracting_b]
|
||
lhs_data = lhs_data.transpose([*batch_perm, *range(n_batch, lhs_data.ndim)])
|
||
lhs_indices = lhs_indices.transpose([*batch_perm, *range(n_batch, lhs_indices.ndim)])
|
||
|
||
# Reorder lhs sparse dimensions
|
||
if lhs_contracting_s:
|
||
lhs_contracting_s = tuple(d - n_batch for d in lhs_contracting_s)
|
||
sparse_perm = jnp.array([*lhs_contracting_s, *remaining(range(n_sparse), lhs_contracting_s)])
|
||
lhs_indices = lhs_indices[..., sparse_perm]
|
||
|
||
# Reorder rhs dimensions
|
||
rhs_perm = [*rhs_batch, *rhs_contracting_b, *rhs_contracting_s,
|
||
*remaining(range(rhs.ndim), rhs_batch, rhs_contracting)]
|
||
rhs = rhs.transpose(rhs_perm)
|
||
|
||
def result(out_array, lhs_data, lhs_indices, rhs):
|
||
idx = tuple(lhs_indices[..., i] for i in range(n_sparse))
|
||
idx_right = idx[:len(lhs_contracting_s)]
|
||
idx_out = idx[len(lhs_contracting_s):]
|
||
if idx_right and lhs_indices.ndim > 2:
|
||
idx_batch = jnp.meshgrid(
|
||
*(jnp.arange(n) for n in lhs_indices.shape[:-1]),
|
||
indexing='ij')[:lhs_indices.ndim - 2]
|
||
idx_right = (*idx_batch, *idx_right)
|
||
batch_dims = list(range(len(lhs_contracting_b) + bool(lhs_contracting_s)))
|
||
prod = lax.dot_general(lhs_data, rhs.at[idx_right].get(mode='fill', fill_value=0),
|
||
(([], []), (batch_dims, batch_dims)))
|
||
if idx_out:
|
||
return out_array.at[idx_out].add(prod)
|
||
else:
|
||
return prod.sum(tuple(range(prod.ndim - out_array.ndim)), dtype=out_array.dtype)
|
||
result = nfold_vmap(result, n_batch - len(lhs_contracting_b))
|
||
rhs = lax.expand_dims(rhs, range(len(rhs_batch), n_batch - len(lhs_contracting_b)))
|
||
out_array = jnp.zeros(out_aval.shape, out_aval.dtype)
|
||
return result(out_array, lhs_data, lhs_indices, rhs)
|
||
|
||
@bcoo_dot_general_p.def_abstract_eval
|
||
def _bcoo_dot_general_abstract_eval(lhs_data, lhs_indices, rhs, *, dimension_numbers, lhs_spinfo: SparseInfo):
|
||
if lhs_data.dtype != rhs.dtype:
|
||
raise ValueError("bcoo_dot_general requires arguments to have matching dtypes; "
|
||
f"got lhs.dtype={lhs_data.dtype}, rhs.dtype={rhs.dtype}")
|
||
|
||
(lhs_contracting, _), (lhs_batch, _) = dimension_numbers
|
||
n_batch, n_sparse, _, _ = _validate_bcoo(lhs_data, lhs_indices, lhs_spinfo.shape)
|
||
out_shape = _dot_general_validated_shape(lhs_spinfo.shape, rhs.shape,
|
||
dimension_numbers)
|
||
|
||
if lhs_batch and max(lhs_batch) >= n_batch:
|
||
raise NotImplementedError(
|
||
"bcoo_dot_general batch dimensions must be among the batch dimensions in the sparse representtaion.\n"
|
||
f"got {lhs_batch=}, {n_batch=}")
|
||
|
||
# TODO: support contraction of dense dimensions?
|
||
if any(d >= n_batch + n_sparse for d in lhs_contracting):
|
||
raise NotImplementedError("bcoo_dot_general: contracting over dense dimensions.")
|
||
|
||
return core.ShapedArray(out_shape, lhs_data.dtype)
|
||
|
||
_bcoo_dot_general_default_lowering = mlir.lower_fun(
|
||
_bcoo_dot_general_impl, multiple_results=False)
|
||
|
||
def _collapse_hlo(x, start, end):
|
||
x_type = ir.RankedTensorType(x.type)
|
||
shape = x_type.shape
|
||
shape = (shape[:start]
|
||
+ [functools.reduce(operator.mul, shape[start:end + 1])]
|
||
+ shape[end + 1:])
|
||
return hlo.ReshapeOp(
|
||
ir.RankedTensorType.get(shape, x_type.element_type), x).result
|
||
|
||
def _bcoo_dot_general_cuda_lowering(
|
||
coo_matvec_lowering, coo_matmat_lowering, ctx, lhs_data, lhs_indices, rhs,
|
||
*, dimension_numbers, lhs_spinfo: SparseInfo):
|
||
(lhs_contract, rhs_contract), (lhs_batch, rhs_batch) = dimension_numbers
|
||
lhs_data_aval, lhs_indices_aval, rhs_aval, = ctx.avals_in
|
||
props = _validate_bcoo_indices(lhs_indices_aval, lhs_spinfo.shape)
|
||
rhs_ndim = len(ir.RankedTensorType(rhs.type).shape)
|
||
|
||
# Checks the shapes of lhs and rhs.
|
||
assert props.n_dense == 0
|
||
assert (props.n_batch, props.n_sparse, rhs_ndim) in [
|
||
(0, 1, 1), (0, 1, 2), (0, 2, 1), (0, 2, 2), (1, 2, 2)]
|
||
|
||
# Checks the operation dimensions.
|
||
assert len(lhs_batch) == 0
|
||
assert len(rhs_batch) == 0
|
||
assert len(lhs_contract) == 1
|
||
|
||
# Checks the dtype.
|
||
assert lhs_data_aval.dtype in [np.float32, np.float64, np.complex64,
|
||
np.complex128]
|
||
assert lhs_data_aval.dtype == rhs_aval.dtype
|
||
assert lhs_indices_aval.dtype == np.int32
|
||
|
||
if rhs_ndim == 1:
|
||
bcoo_dot_general_fn = coo_matvec_lowering
|
||
elif rhs_ndim == 2:
|
||
bcoo_dot_general_fn = coo_matmat_lowering
|
||
if rhs_contract[0] == 1:
|
||
rhs = hlo.TransposeOp(
|
||
rhs, permutation=mlir.dense_int_elements([1, 0])).result
|
||
else:
|
||
raise ValueError(f"rhs has to be 1d or 2d; get {rhs_ndim}d.")
|
||
|
||
if props.n_batch == 0:
|
||
# non-batch mode.
|
||
lhs_transpose = False
|
||
if props.n_sparse == 1:
|
||
# Converts lhs to a row vector.
|
||
col = _collapse_hlo(lhs_indices, start=0, end=1)
|
||
row = mlir.full_like_aval(
|
||
ctx, 0, core.ShapedArray(ir.RankedTensorType(col.type).shape,
|
||
np.dtype(np.int32)))
|
||
lhs_shape = (1, lhs_spinfo.shape[0])
|
||
dot_product = bcoo_dot_general_fn(
|
||
lhs_data, row, col, rhs, shape=lhs_shape, transpose=lhs_transpose,
|
||
data_dtype=lhs_data_aval.dtype, index_dtype=lhs_indices_aval.dtype,
|
||
x_dtype=rhs_aval.dtype)
|
||
|
||
if rhs_ndim == 1:
|
||
# Transforms a single-element array to a scalar.
|
||
return [hlo.ReshapeOp(
|
||
ir.RankedTensorType.get(
|
||
[], ir.RankedTensorType(dot_product.type).element_type),
|
||
dot_product).result]
|
||
else:
|
||
return [_collapse_hlo(dot_product, start=0, end=1)]
|
||
elif props.n_sparse == 2:
|
||
lhs_indices_shape = ir.RankedTensorType(lhs_indices.type).shape
|
||
row = _collapse_hlo(
|
||
hlo.SliceOp(
|
||
lhs_indices,
|
||
start_indices=mlir.dense_int_elements([0, 0]),
|
||
limit_indices=mlir.dense_int_elements([lhs_indices_shape[0], 1]),
|
||
strides=mlir.dense_int_elements([1, 1])).result,
|
||
start=0, end=1)
|
||
col = _collapse_hlo(
|
||
hlo.SliceOp(
|
||
lhs_indices,
|
||
start_indices=mlir.dense_int_elements([0, 1]),
|
||
limit_indices=mlir.dense_int_elements([lhs_indices_shape[0], 2]),
|
||
strides=mlir.dense_int_elements([1, 1])).result,
|
||
start=0, end=1)
|
||
|
||
if lhs_contract[0] == 0:
|
||
lhs_transpose = True
|
||
|
||
return [bcoo_dot_general_fn(
|
||
lhs_data, row, col, rhs, shape=lhs_spinfo.shape,
|
||
transpose=lhs_transpose, data_dtype=lhs_data_aval.dtype,
|
||
index_dtype=lhs_indices_aval.dtype,
|
||
x_dtype=rhs_aval.dtype)]
|
||
else:
|
||
raise ValueError(f"lhs has to be 1d or 2d; get {props.n_sparse}d.")
|
||
elif props.n_batch == 1:
|
||
# batch mode.
|
||
lhs_indices_shape = ir.RankedTensorType(lhs_indices.type).shape
|
||
lhs_data_shape = ir.RankedTensorType(lhs_data.type).shape
|
||
batch_count, _, _ = lhs_indices_shape
|
||
rhs_shape = ir.RankedTensorType(rhs.type).shape
|
||
|
||
# Squeeze the batch dimension for both indices and data.
|
||
lhs_indices_2d_shape = (np.prod(np.array(lhs_indices_shape)[:-1]),
|
||
lhs_indices_shape[-1])
|
||
lhs_data_1d_shape = (np.prod(np.array(lhs_data_shape)), )
|
||
|
||
lhs_indices_2d = hlo.ReshapeOp(
|
||
ir.RankedTensorType.get(
|
||
lhs_indices_2d_shape,
|
||
ir.RankedTensorType(lhs_indices.type).element_type),
|
||
lhs_indices).result
|
||
|
||
lhs_data_1d = hlo.ReshapeOp(
|
||
ir.RankedTensorType.get(
|
||
lhs_data_1d_shape,
|
||
ir.RankedTensorType(lhs_data.type).element_type),
|
||
lhs_data).result
|
||
|
||
row = _collapse_hlo(
|
||
hlo.SliceOp(
|
||
lhs_indices_2d,
|
||
start_indices=mlir.dense_int_elements([0, 0]),
|
||
limit_indices=mlir.dense_int_elements([lhs_indices_2d_shape[0], 1]),
|
||
strides=mlir.dense_int_elements([1, 1])).result,
|
||
start=0, end=1)
|
||
|
||
col = _collapse_hlo(
|
||
hlo.SliceOp(
|
||
lhs_indices_2d,
|
||
start_indices=mlir.dense_int_elements([0, 1]),
|
||
limit_indices=mlir.dense_int_elements([lhs_indices_2d_shape[0], 2]),
|
||
strides=mlir.dense_int_elements([1, 1])).result,
|
||
start=0, end=1)
|
||
|
||
# Broadcast rhs to have the same batch size as lhs.
|
||
# TODO(tianjianlu): remove broadcasting.
|
||
# Use batch_stride = 0 for non-batch.
|
||
# The issue (https://github.com/NVIDIA/CUDALibrarySamples/issues/81#issuecomment-1205562643)
|
||
# in cusparse library does not allow batch_stride = 0 for a non-batched rhs.
|
||
batched_rhs_shape = (batch_count,) + tuple(rhs_shape)
|
||
batched_rhs = hlo.BroadcastInDimOp(
|
||
ir.RankedTensorType.get(batched_rhs_shape,
|
||
ir.RankedTensorType(rhs.type).element_type),
|
||
rhs,
|
||
broadcast_dimensions=mlir.dense_int_elements([1, 2])).result
|
||
batched_rhs_2d_shape = (np.prod(np.array(batched_rhs_shape)[:-1]), batched_rhs_shape[-1])
|
||
batched_rhs_2d = hlo.ReshapeOp(
|
||
ir.RankedTensorType.get(
|
||
batched_rhs_2d_shape,
|
||
ir.RankedTensorType(batched_rhs.type).element_type),
|
||
batched_rhs).result
|
||
|
||
lhs_transpose = True if lhs_contract[0] == props.n_batch else False
|
||
|
||
return [bcoo_dot_general_fn(
|
||
lhs_data_1d, row, col, batched_rhs_2d, shape=lhs_spinfo.shape,
|
||
transpose=lhs_transpose, data_dtype=lhs_data_aval.dtype,
|
||
index_dtype=lhs_indices_aval.dtype,
|
||
x_dtype=rhs_aval.dtype)]
|
||
else:
|
||
raise ValueError(f"n_batch has to be 0 or 1; get {props.n_batch}.")
|
||
|
||
def _bcoo_dot_general_gpu_lowering(
|
||
coo_matvec_lowering, coo_matmat_lowering,
|
||
ctx, lhs_data, lhs_indices, rhs, *, dimension_numbers,
|
||
lhs_spinfo: SparseInfo):
|
||
|
||
if not config.jax_bcoo_cusparse_lowering:
|
||
return _bcoo_dot_general_default_lowering(
|
||
ctx, lhs_data, lhs_indices, rhs,
|
||
dimension_numbers=dimension_numbers, lhs_spinfo=lhs_spinfo)
|
||
|
||
(lhs_contract, rhs_contract), (lhs_batch, rhs_batch) = dimension_numbers
|
||
lhs_data_aval, lhs_indices_aval, rhs_aval, = ctx.avals_in
|
||
n_batch, n_sparse, n_dense, _ = _validate_bcoo(
|
||
lhs_data_aval, lhs_indices_aval, lhs_spinfo.shape)
|
||
|
||
dtype = lhs_data_aval.dtype
|
||
if dtype not in [np.float32, np.float64, np.complex64, np.complex128]:
|
||
warnings.warn(f'bcoo_dot_general cusparse/hipsparse lowering not available '
|
||
f'for {dtype=}. Falling back to default implementation.',
|
||
CuSparseEfficiencyWarning)
|
||
return _bcoo_dot_general_default_lowering(
|
||
ctx, lhs_data, lhs_indices, rhs,
|
||
dimension_numbers=dimension_numbers, lhs_spinfo=lhs_spinfo)
|
||
|
||
if (n_batch > 1 or n_dense or
|
||
n_sparse not in [1, 2] or rhs_aval.ndim not in [1, 2] or
|
||
lhs_batch or rhs_batch or len(lhs_contract) != 1):
|
||
return _bcoo_dot_general_default_lowering(
|
||
ctx, lhs_data, lhs_indices, rhs,
|
||
dimension_numbers=dimension_numbers, lhs_spinfo=lhs_spinfo)
|
||
else:
|
||
if not lhs_spinfo.indices_sorted:
|
||
warnings.warn("bcoo_dot_general GPU lowering requires matrices with "
|
||
"sorted indices. To sort the rows in your matrix, use e.g. "
|
||
"mat = mat.sort_indices(). Falling back to the default "
|
||
"implementation.", CuSparseEfficiencyWarning)
|
||
return _bcoo_dot_general_default_lowering(
|
||
ctx, lhs_data, lhs_indices, rhs,
|
||
dimension_numbers=dimension_numbers, lhs_spinfo=lhs_spinfo)
|
||
|
||
if n_batch == 1:
|
||
# The support for batched computation in cusparseSpMM COO was added in
|
||
# 11.6.1: https://docs.nvidia.com/cuda/cuda-toolkit-release-notes/index.html#cusparse-11.6.1
|
||
cuda_version = int(xla_bridge.get_backend().platform_version.split()[-1])
|
||
|
||
# TODO(tianjianlu): enable the batch mode of cusparseSpMv.
|
||
cuda_supported_batch_mode = (
|
||
n_sparse == 2 and rhs_aval.ndim == 2 and
|
||
len(lhs_contract) == 1 and lhs_contract[0] in [1, 2] and
|
||
len(rhs_contract) == 1 and rhs_contract[0] in [0, 1] and
|
||
cuda_version >= 11061)
|
||
if not cuda_supported_batch_mode:
|
||
warnings.warn("bcoo_dot_general GPU lowering currently does not "
|
||
"support this batch-mode computation. Falling back to "
|
||
"the default implementation.", CuSparseEfficiencyWarning)
|
||
return _bcoo_dot_general_default_lowering(
|
||
ctx, lhs_data, lhs_indices, rhs,
|
||
dimension_numbers=dimension_numbers, lhs_spinfo=lhs_spinfo)
|
||
|
||
return _bcoo_dot_general_cuda_lowering(
|
||
coo_matvec_lowering, coo_matmat_lowering, ctx, lhs_data, lhs_indices, rhs,
|
||
dimension_numbers=dimension_numbers, lhs_spinfo=lhs_spinfo)
|
||
|
||
def _bcoo_dot_general_jvp_lhs(lhs_data_dot, lhs_data, lhs_indices, rhs, *, dimension_numbers, lhs_spinfo: SparseInfo):
|
||
return _bcoo_dot_general(lhs_data_dot, lhs_indices, rhs, dimension_numbers=dimension_numbers, lhs_spinfo=lhs_spinfo)
|
||
|
||
def _bcoo_dot_general_jvp_rhs(rhs_dot, lhs_data, lhs_indices, rhs, *, dimension_numbers, lhs_spinfo: SparseInfo):
|
||
return _bcoo_dot_general(lhs_data, lhs_indices, rhs_dot, dimension_numbers=dimension_numbers, lhs_spinfo=lhs_spinfo)
|
||
|
||
def _bcoo_dot_general_transpose(ct, lhs_data, lhs_indices, rhs, *, dimension_numbers, lhs_spinfo: SparseInfo):
|
||
assert not ad.is_undefined_primal(lhs_indices)
|
||
if type(ct) is ad.Zero:
|
||
return ad.Zero
|
||
(lhs_contract, rhs_contract), (lhs_batch, rhs_batch) = dimension_numbers
|
||
lhs_ndim = len(lhs_spinfo.shape)
|
||
rhs_ndim = rhs.aval.ndim if ad.is_undefined_primal(rhs) else rhs.ndim
|
||
lhs_kept = remaining(range(lhs_ndim), lhs_contract, lhs_batch)
|
||
rhs_kept = remaining(range(rhs_ndim), rhs_contract, rhs_batch)
|
||
ans_batch, ans_lhs, ans_rhs = map(list, ranges_like(lhs_batch, lhs_kept, rhs_kept))
|
||
if ad.is_undefined_primal(lhs_data):
|
||
dims: DotDimensionNumbers = ((ans_rhs, rhs_kept), (ans_batch, rhs_batch)) # type: ignore[assignment]
|
||
lhs_contract_sorted_by_rhs = list(np.take(lhs_contract, np.argsort(rhs_contract)))
|
||
permutation = list(lhs_batch) + lhs_kept + lhs_contract_sorted_by_rhs
|
||
out_axes = list(np.argsort(permutation))
|
||
|
||
# Determine whether efficient approach is possible:
|
||
placeholder_data = jnp.empty((lhs_indices.ndim - 2) * (1,) + (lhs_indices.shape[-2],))
|
||
placeholder_shape = tuple(lhs_indices.shape[:-2]) + lhs_indices.shape[-1] * (1,)
|
||
try:
|
||
_validate_permutation(placeholder_data, lhs_indices, permutation, placeholder_shape)
|
||
except NotImplementedError:
|
||
indices_can_be_untransposed = False
|
||
else:
|
||
indices_can_be_untransposed = True
|
||
|
||
# TODO(jakevdp): explore implementing the efficient approach without actually un-transposing
|
||
# the indices. Could this be done by un-permuting ct, rhs, and dims?
|
||
|
||
if indices_can_be_untransposed:
|
||
# Efficient approach: (1) un-transpose indices, (2) compute SDDMM, (3) re-transpose result.
|
||
_, lhs_indices_T = _bcoo_transpose(placeholder_data, lhs_indices, permutation=permutation,
|
||
spinfo=SparseInfo(placeholder_shape))
|
||
result_T_shape = tuple(placeholder_shape[i] for i in permutation)
|
||
result_T = bcoo_dot_general_sampled(ct, rhs, lhs_indices_T, dimension_numbers=dims)
|
||
result, _ = _bcoo_transpose(result_T, lhs_indices_T, permutation=out_axes,
|
||
spinfo=SparseInfo(result_T_shape))
|
||
else:
|
||
# Fallback to direct approach when above is not possible.
|
||
out_dense_T = lax.dot_general(ct, rhs, dimension_numbers=dims)
|
||
out_dense = lax.transpose(out_dense_T, out_axes)
|
||
result = _bcoo_extract(lhs_indices, out_dense)
|
||
return result, lhs_indices, rhs
|
||
else:
|
||
dims = ((lhs_kept, ans_lhs), (lhs_batch, ans_batch)) # type: ignore[assignment]
|
||
rhs_contract_sorted_by_lhs = list(np.take(rhs_contract, np.argsort(lhs_contract)))
|
||
out_axes = list(np.argsort(list(rhs_batch) + rhs_contract_sorted_by_lhs + rhs_kept))
|
||
result = _bcoo_dot_general(lhs_data, lhs_indices, ct, lhs_spinfo=lhs_spinfo, dimension_numbers=dims)
|
||
return lhs_data, lhs_indices, lax.transpose(result, out_axes)
|
||
|
||
def _bcoo_dot_general_batch_rule(batched_args, batch_dims, *, dimension_numbers, lhs_spinfo: SparseInfo):
|
||
_, _, rhs = batched_args
|
||
_, _, rhs_bdim = batch_dims
|
||
new_lhs_data, new_lhs_indices, new_lhs_spinfo = _bcoo_batch_dims_to_front(
|
||
batched_args[:2], batch_dims[:2], lhs_spinfo,
|
||
batch_size=None if rhs_bdim is None else rhs.shape[rhs_bdim])
|
||
new_dimension_numbers, result_batch_dim = _dot_general_batch_dim_nums(
|
||
(len(lhs_spinfo.shape), rhs.ndim), (0, rhs_bdim), dimension_numbers)
|
||
batched_out = _bcoo_dot_general(new_lhs_data, new_lhs_indices, rhs, lhs_spinfo=new_lhs_spinfo,
|
||
dimension_numbers=new_dimension_numbers)
|
||
return batched_out, result_batch_dim
|
||
|
||
ad.defjvp(bcoo_dot_general_p, _bcoo_dot_general_jvp_lhs, None, _bcoo_dot_general_jvp_rhs)
|
||
ad.primitive_transposes[bcoo_dot_general_p] = _bcoo_dot_general_transpose
|
||
batching.primitive_batchers[bcoo_dot_general_p] = _bcoo_dot_general_batch_rule
|
||
|
||
mlir.register_lowering(
|
||
bcoo_dot_general_p, _bcoo_dot_general_default_lowering)
|
||
|
||
if gpu_sparse.cuda_is_supported:
|
||
mlir.register_lowering(bcoo_dot_general_p,
|
||
partial(_bcoo_dot_general_gpu_lowering,
|
||
gpu_sparse.cuda_coo_matvec,
|
||
gpu_sparse.cuda_coo_matmat),
|
||
platform='cuda')
|
||
if gpu_sparse.rocm_is_supported:
|
||
mlir.register_lowering(bcoo_dot_general_p,
|
||
partial(_bcoo_dot_general_gpu_lowering,
|
||
gpu_sparse.rocm_coo_matvec,
|
||
gpu_sparse.rocm_coo_matmat),
|
||
platform='rocm')
|
||
|
||
#----------------------------------------------------------------------
|
||
# bcoo_dot_general_sampled
|
||
# (batched) general sampled dot product of two dense ND arrays, with
|
||
# output computed only at a given set of sparse indices.
|
||
|
||
bcoo_dot_general_sampled_p = core.Primitive("bcoo_dot_general_sampled")
|
||
|
||
def bcoo_dot_general_sampled(A: Array, B: Array, indices: Array, *, dimension_numbers: DotDimensionNumbers) -> Array:
|
||
"""A contraction operation with output computed at given sparse indices.
|
||
|
||
Args:
|
||
lhs: An ndarray.
|
||
rhs: An ndarray.
|
||
indices: BCOO indices.
|
||
dimension_numbers: a tuple of tuples of the form
|
||
`((lhs_contracting_dims, rhs_contracting_dims),
|
||
(lhs_batch_dims, rhs_batch_dims))`.
|
||
|
||
Returns:
|
||
BCOO data, an ndarray containing the result.
|
||
"""
|
||
(lhs_contract, rhs_contract), (lhs_batch, rhs_batch) = dimension_numbers
|
||
cdims = (api_util._ensure_index_tuple(lhs_contract),
|
||
api_util._ensure_index_tuple(rhs_contract))
|
||
bdims = (api_util._ensure_index_tuple(lhs_batch),
|
||
api_util._ensure_index_tuple(rhs_batch))
|
||
return bcoo_dot_general_sampled_p.bind(A, B, indices,
|
||
dimension_numbers=(cdims, bdims))
|
||
|
||
@bcoo_dot_general_sampled_p.def_impl
|
||
def _bcoo_dot_general_sampled_impl(A, B, indices, *, dimension_numbers):
|
||
# TODO(jakevdp): use a more efficient implementation that avoids the full dot product.
|
||
dense_result = lax.dot_general(A, B, dimension_numbers=dimension_numbers)
|
||
return _bcoo_extract(indices, dense_result)
|
||
|
||
@bcoo_dot_general_sampled_p.def_abstract_eval
|
||
def _bcoo_dot_general_sampled_abstract_eval(A, B, indices, *, dimension_numbers):
|
||
dense_result, = pe.abstract_eval_fun(lambda *args: [lax.dot_general(*args, dimension_numbers=dimension_numbers)], A, B)
|
||
sparse_result, = pe.abstract_eval_fun(lambda *args: [_bcoo_extract(*args)], indices, dense_result)
|
||
return sparse_result
|
||
|
||
def _bcoo_dot_general_sampled_transpose(ct, A, B, indices, *, dimension_numbers):
|
||
A_shape = A.aval.shape if hasattr(A, 'aval') else A.shape
|
||
B_shape = B.aval.shape if hasattr(B, 'aval') else B.shape
|
||
mat_shape = _dot_general_validated_shape(A_shape, B_shape, dimension_numbers)
|
||
mat = ad.UndefinedPrimal(core.ShapedArray(mat_shape, ct.dtype))
|
||
indices, ct = _bcoo_extract_transpose(ct, indices, mat, assume_unique=True)
|
||
kwds = {'dimension_numbers': dimension_numbers,
|
||
'precision': None,
|
||
'preferred_element_type': None}
|
||
A, B = ad.get_primitive_transpose(lax.dot_general_p)(ct, A, B, **kwds)
|
||
return A, B, indices
|
||
|
||
def _bcoo_dot_general_sampled_jvp_A(A_dot, A, B, indices, *, dimension_numbers):
|
||
return bcoo_dot_general_sampled(A_dot, B, indices, dimension_numbers=dimension_numbers)
|
||
|
||
def _bcoo_dot_general_sampled_jvp_B(B_dot, A, B, indices, *, dimension_numbers):
|
||
return bcoo_dot_general_sampled(A, B_dot, indices, dimension_numbers=dimension_numbers)
|
||
|
||
def _bcoo_dot_general_sampled_batch_rule(batched_args, batch_dims, *, dimension_numbers):
|
||
def impl(A, B, indices):
|
||
return _bcoo_dot_general_sampled_impl(A, B, indices, dimension_numbers=dimension_numbers)
|
||
return vmap(impl, in_axes=batch_dims, out_axes=0)(*batched_args), 0
|
||
|
||
ad.defjvp(bcoo_dot_general_sampled_p, _bcoo_dot_general_sampled_jvp_A,
|
||
_bcoo_dot_general_sampled_jvp_B, None)
|
||
ad.primitive_transposes[bcoo_dot_general_sampled_p] = _bcoo_dot_general_sampled_transpose
|
||
batching.primitive_batchers[bcoo_dot_general_sampled_p] = _bcoo_dot_general_sampled_batch_rule
|
||
mlir.register_lowering(
|
||
bcoo_dot_general_sampled_p,
|
||
mlir.lower_fun(_bcoo_dot_general_sampled_impl, multiple_results=False))
|
||
|
||
#----------------------------------------------------------------------
|
||
# bcoo_spdot_general
|
||
# (batched) general dot product of two BCOO sparse arrays returning a
|
||
# Dense ND array.
|
||
|
||
bcoo_spdot_general_p = core.Primitive('bcoo_spdot_general')
|
||
bcoo_spdot_general_p.multiple_results = True
|
||
|
||
def _bcoo_spdot_general(lhs_data: Array, lhs_indices: Array, rhs_data: Array, rhs_indices: Array, *,
|
||
lhs_spinfo: SparseInfo, rhs_spinfo: SparseInfo, dimension_numbers: DotDimensionNumbers) -> Tuple[Array, Array]:
|
||
(lhs_contract, rhs_contract), (lhs_batch, rhs_batch) = dimension_numbers
|
||
cdims = (api_util._ensure_index_tuple(lhs_contract),
|
||
api_util._ensure_index_tuple(rhs_contract))
|
||
bdims = (api_util._ensure_index_tuple(lhs_batch),
|
||
api_util._ensure_index_tuple(rhs_batch))
|
||
return bcoo_spdot_general_p.bind(lhs_data, lhs_indices, rhs_data, rhs_indices,
|
||
lhs_spinfo=lhs_spinfo, rhs_spinfo=rhs_spinfo,
|
||
dimension_numbers=(cdims, bdims))
|
||
|
||
def _bcoo_spdot_general_unbatched(lhs_data, lhs_indices, rhs_data, rhs_indices, *, lhs_spinfo, rhs_spinfo, lhs_contracting, rhs_contracting):
|
||
lhs_shape = lhs_spinfo.shape
|
||
rhs_shape = rhs_spinfo.shape
|
||
|
||
lhs = _validate_bcoo(lhs_data, lhs_indices, lhs_shape)
|
||
rhs = _validate_bcoo(rhs_data, rhs_indices, rhs_shape)
|
||
|
||
assert lhs.n_batch == rhs.n_batch == 0
|
||
assert lhs.n_dense == rhs.n_dense == 0
|
||
assert [lhs_shape[d] for d in lhs_contracting] == [rhs_shape[d] for d in rhs_contracting]
|
||
assert max(lhs_contracting, default=-1) < lhs.n_sparse
|
||
assert max(rhs_contracting, default=-1) < rhs.n_sparse
|
||
|
||
out_shape = (
|
||
*(s for i, s in enumerate(lhs_shape) if i not in lhs_contracting),
|
||
*(s for i, s in enumerate(rhs_shape) if i not in rhs_contracting))
|
||
|
||
lhs_i = lhs_indices[:, jnp.array(lhs_contracting, dtype=int)]
|
||
rhs_i = rhs_indices[:, jnp.array(rhs_contracting, dtype=int)]
|
||
lhs_j = lhs_indices[:, jnp.array(remaining(range(lhs.n_sparse), lhs_contracting), dtype=int)]
|
||
rhs_j = rhs_indices[:, jnp.array(remaining(range(rhs.n_sparse), rhs_contracting), dtype=int)]
|
||
|
||
# TODO(jakevdp): can we do this more efficiently than using an outer product? Note that
|
||
# jnp.isin() currently doesn't help much, because it also does all() over an outer
|
||
# comparison.
|
||
overlap = (lhs_i[:, None] == rhs_i[None, :]).all(-1)
|
||
lhs_fill_value = jnp.expand_dims(
|
||
jnp.array([lhs_shape[d] for d in lhs_contracting], dtype=lhs_i.dtype),
|
||
range(lhs_i.ndim - 1))
|
||
rhs_fill_value = jnp.expand_dims(
|
||
jnp.array([rhs_shape[d] for d in rhs_contracting], dtype=rhs_i.dtype),
|
||
range(rhs_i.ndim - 1))
|
||
lhs_valid = (lhs_i < lhs_fill_value).all(-1)
|
||
rhs_valid = (rhs_i < rhs_fill_value).all(-1)
|
||
out_data = jnp.where(overlap & lhs_valid[:, None] & rhs_valid[None, :],
|
||
lhs_data[:, None] * rhs_data[None, :], 0).ravel()
|
||
|
||
out_indices = jnp.empty([lhs.nse, rhs.nse, lhs_j.shape[-1] + rhs_j.shape[-1]],
|
||
dtype=jnp.result_type(lhs_indices, rhs_indices))
|
||
out_indices = out_indices.at[:, :, :lhs_j.shape[-1]].set(lhs_j[:, None])
|
||
out_indices = out_indices.at[:, :, lhs_j.shape[-1]:].set(rhs_j[None, :])
|
||
out_indices = out_indices.reshape(len(out_data), out_indices.shape[-1])
|
||
out_nse = (lhs.nse if lhs_j.shape[1] else 1) * (rhs.nse if rhs_j.shape[1] else 1)
|
||
# Note: we do not eliminate zeros here, because it can cause issues with autodiff.
|
||
# See https://github.com/google/jax/issues/10163.
|
||
return _bcoo_sum_duplicates(out_data, out_indices, spinfo=SparseInfo(shape=out_shape), nse=out_nse)
|
||
|
||
@bcoo_spdot_general_p.def_impl
|
||
def _bcoo_spdot_general_impl(lhs_data, lhs_indices, rhs_data, rhs_indices, *, lhs_spinfo: SparseInfo, rhs_spinfo: SparseInfo, dimension_numbers):
|
||
lhs_shape = lhs_spinfo.shape
|
||
rhs_shape = rhs_spinfo.shape
|
||
|
||
lhs = _validate_bcoo(lhs_data, lhs_indices, lhs_shape)
|
||
rhs = _validate_bcoo(rhs_data, rhs_indices, rhs_shape)
|
||
assert lhs.n_dense == rhs.n_dense == 0
|
||
data_aval, indices_aval = _bcoo_spdot_general_abstract_eval(
|
||
lhs_data.aval, lhs_indices.aval, rhs_data.aval, rhs_indices.aval,
|
||
lhs_spinfo=lhs_spinfo, rhs_spinfo=rhs_spinfo, dimension_numbers=dimension_numbers)
|
||
out_shape = _dot_general_validated_shape(lhs_shape, rhs_shape,
|
||
dimension_numbers)
|
||
_validate_bcoo(data_aval, indices_aval, out_shape)
|
||
|
||
(lhs_contracting, rhs_contracting), (lhs_batch, rhs_batch) = dimension_numbers
|
||
|
||
# Move batch dimensions to front of each array.
|
||
lhs_batch_perm = [*lhs_batch, *remaining(range(lhs.n_batch), lhs_batch)]
|
||
rhs_batch_perm = [*rhs_batch, *remaining(range(rhs.n_batch), rhs_batch)]
|
||
lhs_data = lhs_data.transpose([*lhs_batch_perm, *range(lhs.n_batch, lhs_data.ndim)])
|
||
rhs_data = rhs_data.transpose([*rhs_batch_perm, *range(rhs.n_batch, rhs_data.ndim)])
|
||
lhs_indices = lhs_indices.transpose([*lhs_batch_perm, *range(lhs.n_batch, lhs_indices.ndim)])
|
||
rhs_indices = rhs_indices.transpose([*rhs_batch_perm, *range(rhs.n_batch, rhs_indices.ndim)])
|
||
|
||
# Implement batched dot product via vmap
|
||
func = functools.partial(_bcoo_spdot_general_unbatched,
|
||
lhs_spinfo=SparseInfo(lhs_shape[lhs.n_batch:]),
|
||
rhs_spinfo=SparseInfo(rhs_shape[rhs.n_batch:]),
|
||
lhs_contracting=[d - lhs.n_batch for d in lhs_contracting],
|
||
rhs_contracting=[d - rhs.n_batch for d in rhs_contracting])
|
||
|
||
func = nfold_vmap(func, rhs.n_batch - len(rhs_batch), in_axes=(None, None, 0, 0))
|
||
func = nfold_vmap(func, lhs.n_batch - len(lhs_batch), in_axes=(0, 0, None, None))
|
||
func = nfold_vmap(func, len(lhs_batch))
|
||
return func(lhs_data, lhs_indices, rhs_data, rhs_indices)
|
||
|
||
@bcoo_spdot_general_p.def_abstract_eval
|
||
def _bcoo_spdot_general_abstract_eval(lhs_data, lhs_indices, rhs_data, rhs_indices, *, lhs_spinfo: SparseInfo, rhs_spinfo: SparseInfo, dimension_numbers):
|
||
lhs_shape = lhs_spinfo.shape
|
||
rhs_shape = rhs_spinfo.shape
|
||
|
||
if lhs_data.dtype != rhs_data.dtype:
|
||
raise ValueError("bcoo_spdot_general requires inputs to have matching dtypes; "
|
||
f"got lhs.dtype={lhs_data.dtype}, rhs.dtype={rhs_data.dtype}")
|
||
lhs = _validate_bcoo(lhs_data, lhs_indices, lhs_shape)
|
||
rhs = _validate_bcoo(rhs_data, rhs_indices, rhs_shape)
|
||
(lhs_contracting, rhs_contracting), (lhs_batch, rhs_batch) = dimension_numbers
|
||
_ = _dot_general_validated_shape(lhs_shape, rhs_shape, dimension_numbers)
|
||
|
||
if lhs.n_dense or rhs.n_dense:
|
||
# TODO(jakevdp): handle dense dimensions
|
||
raise NotImplementedError("bcoo_spdot_general with dense dimensions.")
|
||
|
||
if (lhs_batch and max(lhs_batch) >= lhs.n_batch) or (rhs_batch and max(rhs_batch) >= rhs.n_batch):
|
||
raise NotImplementedError("bcoo_spdot_general: batch_dims must correspond to batch dimensions of the sparse representation.")
|
||
|
||
if lhs_contracting and (min(lhs_contracting) < lhs.n_batch or max(lhs_contracting) >= lhs.n_batch + lhs.n_sparse):
|
||
raise NotImplementedError("bcoo_spdot_general only supports contraction of sparse indices.")
|
||
|
||
if rhs_contracting and (min(rhs_contracting) < rhs.n_batch or max(rhs_contracting) >= rhs.n_batch + rhs.n_sparse):
|
||
raise NotImplementedError("bcoo_spdot_general only supports contraction of sparse indices.")
|
||
|
||
if rhs.n_batch > len(rhs_batch) and lhs.n_sparse > len(lhs_contracting):
|
||
raise ValueError("bcoo_spdot_general: cannot have unused batch dims on rhs with unused sparse dims on lhs.")
|
||
|
||
out_nse = (
|
||
(lhs.nse if lhs.n_sparse > len(lhs_contracting) else 1) *
|
||
(rhs.nse if rhs.n_sparse > len(rhs_contracting) else 1)
|
||
)
|
||
|
||
data_shape = (
|
||
*(lhs_shape[dim] for dim in lhs_batch),
|
||
*(lhs_data.shape[dim] for dim in range(lhs.n_batch) if dim not in lhs_batch),
|
||
*(rhs_data.shape[dim] for dim in range(rhs.n_batch) if dim not in rhs_batch),
|
||
out_nse)
|
||
indices_shape = (
|
||
*(lhs_shape[dim] for dim in lhs_batch),
|
||
*(lhs_indices.shape[dim] for dim in range(lhs.n_batch) if dim not in lhs_batch),
|
||
*(rhs_indices.shape[dim] for dim in range(rhs.n_batch) if dim not in rhs_batch),
|
||
out_nse, lhs.n_sparse + rhs.n_sparse - 2 * len(lhs_contracting))
|
||
return core.ShapedArray(data_shape, lhs_data.dtype), core.ShapedArray(indices_shape, lhs_indices.dtype)
|
||
|
||
def _bcoo_spdot_general_batch_rule(batched_args, batch_dims, *, lhs_spinfo: SparseInfo, rhs_spinfo: SparseInfo, dimension_numbers):
|
||
lhs_ndim = len(lhs_spinfo.shape)
|
||
rhs_ndim = len(rhs_spinfo.shape)
|
||
batch_size = max(arg.shape[dim] for arg, dim in zip(batched_args, batch_dims) if dim is not None)
|
||
lhs_data, lhs_indices, lhs_spinfo = _bcoo_batch_dims_to_front(
|
||
batched_args[:2], batch_dims[:2], lhs_spinfo, batch_size=batch_size)
|
||
rhs_data, rhs_indices, rhs_spinfo = _bcoo_batch_dims_to_front(
|
||
batched_args[2:], batch_dims[2:], rhs_spinfo, batch_size=batch_size)
|
||
dimension_numbers, result_batch_dim = _dot_general_batch_dim_nums(
|
||
(lhs_ndim, rhs_ndim), (0, 0), dimension_numbers)
|
||
batched_out = _bcoo_spdot_general(lhs_data, lhs_indices, rhs_data, rhs_indices,
|
||
dimension_numbers=dimension_numbers,
|
||
lhs_spinfo=lhs_spinfo, rhs_spinfo=rhs_spinfo)
|
||
return batched_out, (result_batch_dim, result_batch_dim)
|
||
|
||
|
||
def _bcoo_spdot_general_jvp(primals, tangents, **kwds):
|
||
lhs_data, lhs_indices, rhs_data, rhs_indices = primals
|
||
lhs_data_dot, lhs_indices_dot, rhs_data_dot, rhs_indices_dot = tangents
|
||
primals_out = _bcoo_spdot_general(*primals, **kwds)
|
||
assert type(lhs_indices_dot) is ad.Zero
|
||
assert type(rhs_indices_dot) is ad.Zero
|
||
data_dot_out = 0
|
||
if type(lhs_data_dot) is not ad.Zero:
|
||
data_dot_out += _bcoo_spdot_general(lhs_data_dot, lhs_indices, rhs_data, rhs_indices, **kwds)[0]
|
||
if type(rhs_data_dot) is not ad.Zero:
|
||
data_dot_out += _bcoo_spdot_general(lhs_data, lhs_indices, rhs_data_dot, rhs_indices, **kwds)[0]
|
||
return primals_out, [data_dot_out, ad.Zero.from_value(primals_out[1])]
|
||
|
||
# TODO(JVP): transpose rule
|
||
batching.primitive_batchers[bcoo_spdot_general_p] = _bcoo_spdot_general_batch_rule
|
||
ad.primitive_jvps[bcoo_spdot_general_p] = _bcoo_spdot_general_jvp
|
||
mlir.register_lowering(bcoo_spdot_general_p, mlir.lower_fun(
|
||
_bcoo_spdot_general_impl, multiple_results=True))
|
||
|
||
|
||
#----------------------------------------------------------------------
|
||
# bcoo_sort_indices
|
||
# Utility to sort the indices of a BCOO representation. This primitive
|
||
# does not support deduplication or removing of zeros; see bcoo_sum_duplicates.
|
||
|
||
bcoo_sort_indices_p = core.Primitive("bcoo_sort_indices")
|
||
bcoo_sort_indices_p.multiple_results = True
|
||
|
||
def bcoo_sort_indices(mat: BCOO) -> BCOO:
|
||
"""Sort indices of a BCOO array.
|
||
|
||
Args:
|
||
mat : BCOO array
|
||
|
||
Returns:
|
||
mat_out : BCOO array with sorted indices.
|
||
"""
|
||
data, indices = bcoo_sort_indices_p.bind(*mat._bufs, spinfo=mat._info)
|
||
return BCOO((data, indices), shape=mat.shape, indices_sorted=True,
|
||
unique_indices=mat.unique_indices)
|
||
|
||
@bcoo_sort_indices_p.def_impl
|
||
def _bcoo_sort_indices_impl(data, indices, *, spinfo):
|
||
props = _validate_bcoo(data, indices, spinfo.shape)
|
||
if props.n_sparse == 0:
|
||
return data, indices
|
||
f = nfold_vmap(_bcoo_sort_indices_unbatched, props.n_batch, broadcasted=False)
|
||
indices, perm = f(indices)
|
||
permute = nfold_vmap(lambda d, p: d[p], props.n_batch)
|
||
data = permute(data, perm)
|
||
return data, indices
|
||
|
||
def _bcoo_sort_indices_unbatched(indices):
|
||
# sort indices without summing duplicates
|
||
nse, N = indices.shape
|
||
idx_cols = (indices[:, i] for i in range(N))
|
||
*indices, perm = lax.sort((*idx_cols, lax.iota(indices.dtype, nse)), num_keys=N)
|
||
return jnp.column_stack(indices), perm
|
||
|
||
@bcoo_sort_indices_p.def_abstract_eval
|
||
def _bcoo_sort_indices_abstract_eval(data, indices, *, spinfo):
|
||
props = _validate_bcoo(data, indices, spinfo.shape)
|
||
if props.n_sparse == 0:
|
||
return data, indices
|
||
data_out = core.ShapedArray(
|
||
(*map(max, indices.shape[:props.n_batch], data.shape[:props.n_batch]),
|
||
props.nse, *data.shape[props.n_batch + 1:]), data.dtype, weak_type=data.weak_type)
|
||
return data_out, indices
|
||
|
||
def _bcoo_sort_indices_batching_rule(batched_args, batch_dims, *, spinfo):
|
||
data, indices, spinfo = _bcoo_batch_dims_to_front(batched_args, batch_dims, spinfo)
|
||
data_out, indices_out = bcoo_sort_indices_p.bind(data, indices, spinfo=spinfo)
|
||
out_axes = (0, 0)
|
||
# Note: if data is unbatched on input, it will be batched on output.
|
||
# However, if indices are unbatched on input, they will be unbatched on output.
|
||
if batch_dims[1] is None:
|
||
indices_out = indices_out[0]
|
||
out_axes = (0, None)
|
||
return (data_out, indices_out), out_axes
|
||
|
||
def _bcoo_sort_indices_jvp(primals, tangents, *, spinfo):
|
||
props = _validate_bcoo(*primals, spinfo.shape)
|
||
if props.n_sparse == 0:
|
||
return primals, tangents
|
||
|
||
data, indices = primals
|
||
data_dot, _ = tangents
|
||
f = nfold_vmap(_bcoo_sort_indices_unbatched, props.n_batch)
|
||
indices_out, perm = f(indices)
|
||
permute = nfold_vmap(lambda d, p: d[p], props.n_batch)
|
||
data_out = permute(data, perm)
|
||
|
||
indices_dot_out = ad.Zero.from_value(indices)
|
||
data_dot_out = ad.Zero.from_value(data_out) if type(data_dot) is ad.Zero else permute(data_dot, perm)
|
||
return (data_out, indices_out), (data_dot_out, indices_dot_out)
|
||
|
||
_bcoo_sort_indices_hlo = mlir.lower_fun(
|
||
_bcoo_sort_indices_impl, multiple_results=True)
|
||
|
||
ad.primitive_jvps[bcoo_sort_indices_p] = _bcoo_sort_indices_jvp
|
||
batching.primitive_batchers[bcoo_sort_indices_p] = _bcoo_sort_indices_batching_rule
|
||
mlir.register_lowering(bcoo_sort_indices_p, _bcoo_sort_indices_hlo)
|
||
|
||
|
||
#----------------------------------------------------------------------
|
||
# bcoo_sum_duplicates
|
||
# Utility to sum duplicate indices in a BCOO array representation.
|
||
|
||
bcoo_sum_duplicates_p = core.Primitive("bcoo_sum_duplicates")
|
||
bcoo_sum_duplicates_p.multiple_results = True
|
||
|
||
def bcoo_sum_duplicates(mat: BCOO, nse: Optional[int] = None) -> BCOO:
|
||
"""Sums duplicate indices within a BCOO array, returning an array with sorted indices.
|
||
|
||
Args:
|
||
mat : BCOO array
|
||
nse : integer (optional). The number of specified elements in the output matrix. This must
|
||
be specified for bcoo_sum_duplicates to be compatible with JIT and other JAX transformations.
|
||
If not specified, the optimal nse will be computed based on the contents of the data and
|
||
index arrays. If specified nse is larger than necessary, data and index arrays will be padded
|
||
with standard fill values. If smaller than necessary, data elements will be dropped from the
|
||
output matrix.
|
||
|
||
Returns:
|
||
mat_out : BCOO array with sorted indices and no duplicate indices.
|
||
"""
|
||
data, indices = _bcoo_sum_duplicates(mat.data, mat.indices, spinfo=mat._info, nse=nse)
|
||
return BCOO((data, indices), shape=mat.shape, indices_sorted=True,
|
||
unique_indices=True)
|
||
|
||
def _bcoo_sum_duplicates(data: Array, indices: Array, *, spinfo: SparseInfo, nse: Optional[int]) -> Tuple[Array, Array]:
|
||
if nse is not None:
|
||
nse = core.concrete_or_error(operator.index, nse, "nse argument of bcoo_sum_duplicates.")
|
||
return bcoo_sum_duplicates_p.bind(data, indices, spinfo=spinfo, nse=nse)
|
||
|
||
@bcoo_sum_duplicates_p.def_impl
|
||
def _bcoo_sum_duplicates_impl(data, indices, *, spinfo, nse):
|
||
props = _validate_bcoo(data, indices, spinfo.shape)
|
||
indices_out, mapping, nse_batched = _unique_indices(
|
||
indices, shape=spinfo.shape, return_inverse=True, return_true_size=True)
|
||
if nse is None:
|
||
nse = 1 if props.n_sparse == 0 else nse_batched.max()
|
||
indices_out = _adjust_indices_nse(indices_out, nse=nse, shape=spinfo.shape)
|
||
if props.n_sparse == 0:
|
||
data = data.sum(props.n_batch, keepdims=True)
|
||
data_out = jnp.empty((*map(max, indices.shape[:props.n_batch], data.shape[:props.n_batch]),
|
||
nse, *data.shape[props.n_batch + 1:]), dtype=data.dtype)
|
||
permute = lambda d_out, m, d: d_out.at[m].add(d, mode='drop')
|
||
permute = nfold_vmap(permute, props.n_batch)
|
||
data_out = permute(data_out, mapping, data)
|
||
return data_out, indices_out
|
||
|
||
def _adjust_indices_nse(indices, *, nse, shape):
|
||
props = _validate_bcoo_indices(indices, shape)
|
||
if nse <= props.nse:
|
||
indices = indices[..., :nse, :]
|
||
else:
|
||
fill = lax.broadcast_in_dim(
|
||
operand=jnp.array(shape[props.n_batch:props.n_batch + props.n_sparse], dtype=indices.dtype),
|
||
shape=(*indices.shape[:-2], nse - props.nse, indices.shape[-1]),
|
||
broadcast_dimensions=(indices.ndim - 1,)
|
||
)
|
||
indices = lax.concatenate([indices, fill], dimension=indices.ndim - 2)
|
||
return indices
|
||
|
||
def _unique_indices(indices, *, shape, return_inverse=False,
|
||
return_index=False, return_true_size=False):
|
||
props = _validate_bcoo_indices(indices, shape)
|
||
f = partial(_unique_indices_unbatched, shape=shape[props.n_batch:],
|
||
return_inverse=return_inverse, return_index=return_index,
|
||
return_true_size=return_true_size)
|
||
f = nfold_vmap(f, props.n_batch, broadcasted=False)
|
||
return f(indices)
|
||
|
||
def _unique_indices_unbatched(indices, *, shape, return_inverse=False,
|
||
return_index=False, return_true_size=False):
|
||
props = _validate_bcoo_indices(indices, shape)
|
||
if props.n_sparse == 0:
|
||
nse = 1
|
||
indices_out = jnp.zeros_like(indices, shape=(nse, 0))
|
||
out = (indices_out,)
|
||
if return_index:
|
||
out = (*out, jnp.zeros(nse, dtype='int32'))
|
||
if return_inverse:
|
||
out = (*out, jnp.zeros(nse, dtype='int32'))
|
||
if return_true_size:
|
||
out = (*out, nse)
|
||
return out[0] if len(out) == 1 else out
|
||
fill_value = jnp.expand_dims(jnp.array(shape[:props.n_sparse], dtype=indices.dtype), (0,))
|
||
out_of_bounds = (indices >= fill_value).any(-1, keepdims=True)
|
||
indices = jnp.where(out_of_bounds, fill_value, indices)
|
||
# TODO: check if `indices_sorted` is True.
|
||
out = _unique(indices, axis=0, return_inverse=return_inverse, return_index=return_index,
|
||
return_true_size=return_true_size, size=props.nse, fill_value=fill_value)
|
||
if return_true_size:
|
||
nse = out[-1]
|
||
nse = nse - (indices == fill_value).any().astype(nse.dtype)
|
||
out = (*out[:-1], nse)
|
||
return out
|
||
|
||
def _fix_oob_indices_unbatched(data, indices, *, shape):
|
||
"""Set out-of-bound (OOB) indices and the corresponding data to zero."""
|
||
n_batch, n_sparse, n_dense, _ = _validate_bcoo_indices(indices, shape)
|
||
assert n_dense == 0, "not implemented"
|
||
assert n_batch == 0
|
||
mask = indices >= jnp.array(shape[:n_sparse])[None, :]
|
||
new_indices = jnp.where(mask, 0, indices)
|
||
new_data = jnp.where(mask.any(-1), 0, data)
|
||
return new_data, new_indices
|
||
|
||
def _fix_oob_indices(data, indices, *, spinfo):
|
||
"""Set out-of-bound (OOB) indices and the corresponding data to zero."""
|
||
props = _validate_bcoo_indices(indices, spinfo.shape)
|
||
f = partial(_fix_oob_indices_unbatched, shape=spinfo.shape[props.n_batch:])
|
||
f = nfold_vmap(f, props.n_batch)
|
||
return f(data, indices)
|
||
|
||
@bcoo_sum_duplicates_p.def_abstract_eval
|
||
def _bcoo_sum_duplicates_abstract_eval(data, indices, *, spinfo, nse):
|
||
if nse is None:
|
||
raise ValueError("bcoo_sum_duplicates: nse must be specified when using the function within "
|
||
"jit, vmap, and other transformations requiring abstract evaluation.")
|
||
props = _validate_bcoo(data, indices, spinfo.shape)
|
||
indices_out = core.ShapedArray((*indices.shape[:props.n_batch], nse, props.n_sparse),
|
||
dtype=indices.dtype, weak_type=indices.weak_type)
|
||
data_out = core.ShapedArray(
|
||
(*map(max, indices.shape[:props.n_batch], data.shape[:props.n_batch]),
|
||
nse, *data.shape[props.n_batch + 1:]), data.dtype, weak_type=data.weak_type)
|
||
return data_out, indices_out
|
||
|
||
def _bcoo_sum_duplicates_batching_rule(batched_args, batch_dims, *, spinfo, nse):
|
||
data, indices, new_spinfo = _bcoo_batch_dims_to_front(batched_args, batch_dims, spinfo)
|
||
data_out, indices_out = bcoo_sum_duplicates_p.bind(data, indices, spinfo=new_spinfo, nse=nse)
|
||
# Note: if data is unbatched on input, it will be batched on output.
|
||
# However, if indices are unbatched on input, they will be unbatched on output.
|
||
if batch_dims[1] is None:
|
||
indices_out = lax.squeeze(indices_out, [0])
|
||
out_axes = (0, None)
|
||
else:
|
||
out_axes = (0, 0)
|
||
return (data_out, indices_out), out_axes
|
||
|
||
def _bcoo_sum_duplicates_jvp(primals, tangents, *, spinfo, nse):
|
||
props = _validate_bcoo(*primals, spinfo.shape)
|
||
|
||
data, indices = primals
|
||
data_dot, _ = tangents
|
||
indices_out, mapping, nse_batched = _unique_indices(
|
||
indices, shape=spinfo.shape, return_inverse=True, return_true_size=True)
|
||
if nse is None:
|
||
nse = jnp.sum(nse_batched)
|
||
try:
|
||
nse = core.concrete_or_error(operator.index, nse, "nse argument of bcoo_sum_duplicates.")
|
||
except core.ConcretizationTypeError:
|
||
raise ValueError("bcoo_sum_duplicates: nse must be specified when using the function within "
|
||
"jit, vmap, and other transformations requiring abstract evaluation.")
|
||
indices_out = _adjust_indices_nse(indices_out, nse=nse, shape=spinfo.shape)
|
||
if props.n_sparse == 0:
|
||
data = data.sum(props.n_batch, keepdims=True)
|
||
data_dot = data_dot.sum(props.n_batch, keepdims=True)
|
||
data_out = jnp.empty((*map(max, indices.shape[:props.n_batch], data.shape[:props.n_batch]),
|
||
nse, *data.shape[props.n_batch + 1:]), dtype=data.dtype)
|
||
data_dot_out = data_out
|
||
# This check is because scatter-add on zero-sized arrays has poorly defined
|
||
# semantics; see https://github.com/google/jax/issues/13656.
|
||
if data_out.size:
|
||
permute = lambda x, i, y: x.at[i].add(y, mode='drop')
|
||
else:
|
||
permute = lambda x, i, y: x
|
||
permute = nfold_vmap(permute, props.n_batch)
|
||
data_out = permute(data_out, mapping, data)
|
||
indices_dot_out = ad.Zero.from_value(indices_out)
|
||
data_dot_out = ad.Zero.from_value(data_out) if type(data_dot) is ad.Zero else permute(data_dot_out, mapping, data_dot)
|
||
return (data_out, indices_out), (data_dot_out, indices_dot_out)
|
||
|
||
_bcoo_sum_duplicates_hlo = mlir.lower_fun(
|
||
_bcoo_sum_duplicates_impl, multiple_results=True)
|
||
|
||
ad.primitive_jvps[bcoo_sum_duplicates_p] = _bcoo_sum_duplicates_jvp
|
||
batching.primitive_batchers[bcoo_sum_duplicates_p] = _bcoo_sum_duplicates_batching_rule
|
||
mlir.register_lowering(bcoo_sum_duplicates_p, _bcoo_sum_duplicates_hlo)
|
||
|
||
#----------------------------------------------------------------------
|
||
# BCOO functions that maybe should be primitives?
|
||
|
||
def bcoo_update_layout(mat: BCOO, *, n_batch: Optional[int] = None, n_dense: Optional[int] = None,
|
||
on_inefficient: Optional[str] = 'error') -> BCOO:
|
||
"""Update the storage layout (i.e. n_batch & n_dense) of a BCOO matrix.
|
||
|
||
In many cases this can be done without introducing undue storage overhead. However,
|
||
increasing ``mat.n_batch`` or ``mat.n_dense`` will lead to very inefficient storage,
|
||
with many explicitly-stored zeros, unless the new batch or dense dimensions have size
|
||
0 or 1. In such cases, ``bcoo_update_layout`` will raise a :class:`SparseEfficiencyError`.
|
||
This can be silenced by specifying the ``on_inefficient`` argument.
|
||
|
||
Args:
|
||
mat : BCOO array
|
||
n_batch : optional(int) the number of batch dimensions in the output matrix. If None,
|
||
then n_batch = mat.n_batch.
|
||
n_dense : optional(int) the number of dense dimensions in the output matrix. If None,
|
||
then n_dense = mat.n_dense.
|
||
on_inefficient : optional(string), one of ``['error', 'warn', None]``. Specify the
|
||
behavior in case of an inefficient reconfiguration. This is defined as a reconfiguration
|
||
where the size of the resulting representation is much larger than the size of the
|
||
input representation.
|
||
|
||
Returns:
|
||
mat_out : BCOO array
|
||
A BCOO array representing the same sparse array as the input, with the specified
|
||
layout. ``mat_out.todense()`` will match ``mat.todense()`` up to appropriate precision.
|
||
"""
|
||
# TODO(jakevdp): allow specification of nse?
|
||
# TODO(jakevdp): there is room for some improvements here:
|
||
# - we could probably do better in the case of converting a dense dim to
|
||
# a batch dim or vice-versa. Worth adding that special case?
|
||
# - we could work to preserve broadcasted batch dimensions when possible.
|
||
# - if indices are known to be unique, we can convert them to batch/dense
|
||
# dimensions more efficiently.
|
||
n_batch = mat.n_batch if n_batch is None else operator.index(n_batch)
|
||
n_dense = mat.n_dense if n_dense is None else operator.index(n_dense)
|
||
|
||
if (n_batch, n_dense) == (mat.n_batch, mat.n_dense):
|
||
return mat
|
||
|
||
n_sparse = mat.ndim - n_batch - n_dense
|
||
if on_inefficient not in ['error', 'warn', None]:
|
||
raise ValueError("on_inefficent={on_inefficient!r}; expected one of ['error', 'warn', None].")
|
||
|
||
if n_batch < 0:
|
||
raise ValueError(f"n_batch must be non-negative; got {n_batch}")
|
||
if n_dense < 0:
|
||
raise ValueError(f"n_dense must be non-negative; got {n_dense}")
|
||
if n_sparse < 0:
|
||
raise ValueError(f"sum of {n_batch=} and {n_dense=} "
|
||
f"cannot be larger than mat.ndim={mat.ndim}.")
|
||
|
||
def _maybe_err_or_warn(msg):
|
||
if on_inefficient == 'error':
|
||
msg += (" To disable this error, set the on_inefficient argument "
|
||
"of bcoo_update_layout to 'warn' or None.")
|
||
raise SparseEfficiencyError(msg)
|
||
elif on_inefficient == 'warn':
|
||
msg += (" To disable this warning, set the on_inefficient argument "
|
||
"of bcoo_update_layout to None.")
|
||
warnings.warn(msg, category=SparseEfficiencyWarning)
|
||
|
||
# TODO(jakevdp): are efficiency warnings necessary when nse is 0 or 1?
|
||
if (n_dense > mat.n_dense and
|
||
any(d > 1 for d in mat.shape[-n_dense:mat.ndim - mat.n_dense])):
|
||
_maybe_err_or_warn(f"For matrix of shape {mat.shape}, increasing n_dense from "
|
||
f"{mat.n_dense} to {n_dense} results in inefficient storage.")
|
||
if n_batch > mat.n_batch and any(d > 1 for d in mat.shape[mat.n_batch:n_batch]):
|
||
_maybe_err_or_warn(f"For matrix of shape {mat.shape}, increasing n_batch from "
|
||
f"{mat.n_batch} to {n_batch} results in inefficient storage.")
|
||
|
||
new_data, new_indices = mat.data, mat.indices
|
||
shape = mat.shape
|
||
current_n_batch = mat.n_batch
|
||
current_n_dense = mat.n_dense
|
||
|
||
if n_dense < current_n_dense:
|
||
n = current_n_dense - n_dense
|
||
@partial(nfold_vmap, N=current_n_batch + 1)
|
||
def _update(d, i):
|
||
new_d = d.reshape(np.prod(d.shape[:n]), *d.shape[n:])
|
||
meshes = jnp.meshgrid(*(jnp.arange(s, dtype=i.dtype) for s in d.shape[:n]),
|
||
indexing='ij')
|
||
new_i = jnp.column_stack([jnp.broadcast_to(i, (new_d.shape[0], i.size)),
|
||
*map(jnp.ravel, meshes)])
|
||
return new_d, new_i
|
||
new_data, new_indices = _update(new_data, new_indices)
|
||
new_data = new_data.reshape(*new_data.shape[:current_n_batch],
|
||
np.prod(new_data.shape[current_n_batch:current_n_batch + 2]),
|
||
*new_data.shape[current_n_batch + 2:])
|
||
new_indices = new_indices.reshape(*new_indices.shape[:current_n_batch],
|
||
np.prod(new_indices.shape[current_n_batch: current_n_batch + 2]),
|
||
*new_indices.shape[current_n_batch + 2:])
|
||
current_n_dense = n_dense
|
||
|
||
if n_batch < current_n_batch:
|
||
n = current_n_batch - n_batch
|
||
@partial(nfold_vmap, N=n_batch)
|
||
def _update(d, i):
|
||
nse = i.shape[-2]
|
||
new_d = d.reshape(np.prod(d.shape[:n + 1]), *d.shape[n + 1:])
|
||
meshes = jnp.meshgrid(*(jnp.arange(d, dtype=i.dtype) for d in (*i.shape[:n], nse)),
|
||
indexing='ij')
|
||
new_i = i.reshape(np.prod(i.shape[:n + 1]), *i.shape[n + 1:])
|
||
new_i = jnp.column_stack((*(m.ravel() for m in meshes[:-1]), new_i))
|
||
return new_d, new_i
|
||
new_data, new_indices = _update(new_data, new_indices)
|
||
current_n_batch = n_batch
|
||
|
||
if n_dense > current_n_dense:
|
||
n = n_dense - current_n_dense
|
||
@partial(nfold_vmap, N=current_n_batch + 1)
|
||
def _update(d, i):
|
||
new_d = jnp.zeros_like(d, shape=shape[-n_dense:]).at[tuple(i[-n:])].set(d)
|
||
new_i = i[:-n]
|
||
return new_d, new_i
|
||
new_data, new_indices = _update(new_data, new_indices)
|
||
current_n_dense = n_dense
|
||
|
||
if n_batch > current_n_batch:
|
||
n = n_batch - current_n_batch
|
||
@partial(nfold_vmap, N=current_n_batch)
|
||
def _update(d, i):
|
||
nse = i.shape[-2]
|
||
idx = tuple(i[:, j] for j in range(n)) + (jnp.arange(nse),)
|
||
new_i_shape = (*shape[current_n_batch:n_batch], nse, i.shape[-1] - n)
|
||
new_i = jnp.broadcast_to(i[:, n:], new_i_shape)
|
||
new_d_shape = (*shape[current_n_batch:n_batch], nse, *d.shape[d.ndim - n_dense:])
|
||
new_d = jnp.zeros_like(d, shape=new_d_shape).at[idx].set(d)
|
||
return new_d, new_i
|
||
new_data, new_indices = _update(new_data, new_indices)
|
||
current_n_batch = n_batch
|
||
|
||
return BCOO((new_data, new_indices), shape=shape)
|
||
|
||
|
||
def bcoo_broadcast_in_dim(mat: BCOO, *, shape: Shape, broadcast_dimensions: Sequence[int]) -> BCOO:
|
||
"""Expand the size and rank of a BCOO array by duplicating the data.
|
||
|
||
A BCOO equivalence to jax.lax.broadcast_in_dim.
|
||
|
||
Args:
|
||
mat: A BCOO-format array.
|
||
shape: The shape of the target array.
|
||
broadcast_dimensions: The dimension in the shape of the target array which
|
||
each dimension of the operand (``mat``) shape corresponds to.
|
||
|
||
Returns:
|
||
A BCOO-format array containing the target array.
|
||
"""
|
||
return BCOO(_bcoo_broadcast_in_dim(mat.data, mat.indices, spinfo=mat._info,
|
||
shape=shape,
|
||
broadcast_dimensions=broadcast_dimensions),
|
||
shape=shape)
|
||
|
||
def _bcoo_broadcast_in_dim(data: Array, indices: Array, *, spinfo: SparseInfo, shape: Shape,
|
||
broadcast_dimensions: Sequence[int]) -> Tuple[Array, Array]:
|
||
"""BCOO equivalent of lax.broadcast_in_dim"""
|
||
if len(spinfo.shape) != len(broadcast_dimensions):
|
||
raise ValueError(f"{spinfo.shape=} and {broadcast_dimensions=} must have the same length")
|
||
props = _validate_bcoo(data, indices, spinfo.shape)
|
||
batch_dims, sparse_dims, dense_dims = split_list(broadcast_dimensions, [props.n_batch, props.n_sparse])
|
||
|
||
if max(batch_dims, default=0) > min(sparse_dims, default=len(shape)):
|
||
raise ValueError("Cannot mix batch and sparse dimensions during broadcast_in_dim")
|
||
if max(sparse_dims, default=0) > min(dense_dims, default=len(shape)):
|
||
raise ValueError("Cannot mix sparse and dense dimensions during broadcast_in_dim")
|
||
|
||
# All new dimensions preceding a sparse or dense dimension are batch dimensions:
|
||
new_n_batch = min(broadcast_dimensions[props.n_batch:], default=len(shape))
|
||
# TODO(jakevdp): Should new trailing dimensions be dense by default?
|
||
new_n_dense = props.n_dense and len(shape) - min(broadcast_dimensions[-props.n_dense:])
|
||
new_n_sparse = len(shape) - new_n_batch - new_n_dense
|
||
|
||
if np.prod(spinfo.shape[props.n_batch: props.n_batch + props.n_sparse]) != np.prod(shape[new_n_batch:new_n_batch + new_n_sparse]):
|
||
raise NotImplementedError("Adding sparse dimensions with lengths != 1")
|
||
new_data, new_indices = data, indices
|
||
|
||
# batch & dense dimensions
|
||
if (new_n_batch, new_n_dense) != (props.n_batch, props.n_dense):
|
||
new_data = lax.broadcast_in_dim(new_data,
|
||
shape=(*shape[:new_n_batch], props.nse, *shape[new_n_batch + new_n_sparse:]),
|
||
broadcast_dimensions=(*batch_dims, new_n_batch, *(b + 1 - new_n_sparse for b in dense_dims)))
|
||
new_indices = lax.broadcast_in_dim(new_indices,
|
||
shape=(*shape[:new_n_batch], props.nse, props.n_sparse),
|
||
broadcast_dimensions=(*batch_dims, new_n_batch, new_n_batch + 1))
|
||
|
||
# sparse dimensions
|
||
if new_n_sparse != props.n_sparse:
|
||
shape = (*shape[:new_n_batch], props.nse, new_n_sparse)
|
||
ind = jnp.array(sparse_dims, int) - new_n_batch
|
||
new_indices = (jnp.zeros_like(new_indices, shape=shape).at[..., ind].set(new_indices))
|
||
|
||
return new_data, new_indices
|
||
|
||
def bcoo_concatenate(operands: Sequence[BCOO], *, dimension: int) -> BCOO:
|
||
"""Sparse implementation of :func:`jax.lax.concatenate`
|
||
|
||
Args:
|
||
operands : Sequence of BCOO arrays to concatenate. The arrays must have equal
|
||
shapes, except in the `dimension` axis. Additionally, the arrays must have
|
||
have equivalent batch, sparse, and dense dimensions.
|
||
dimension : Positive integer specifying the dimension along which to concatenate
|
||
the arrays. The dimension must be among batch or sparse dimensions of the input;
|
||
concatenation along dense dimensions is not supported.
|
||
|
||
Returns:
|
||
A BCOO array containing the concatenation of the inputs.
|
||
"""
|
||
dimension = operator.index(dimension)
|
||
if not all(isinstance(op, BCOO) for op in operands):
|
||
raise ValueError("bcoo_concatenate: expected operands to be a sequence of BCOO arrays. "
|
||
f"Got {operands}")
|
||
# Validate inputs using lax.concatenate abstract evaluation.
|
||
out_aval = jax.eval_shape(
|
||
functools.partial(lax.concatenate, dimension=dimension),
|
||
[core.ShapedArray(op.shape, op.dtype) for op in operands])
|
||
if len({op.n_dense for op in operands}) > 1:
|
||
raise ValueError("bcoo_concatenate requires inputs to have matching nse dimensions.")
|
||
|
||
n_batches = {op.n_batch for op in operands}
|
||
# Correct for the common case, where op[None, :] adds a single batch dimension and we
|
||
# need to align it in order to match the others & concatenate.
|
||
if len(n_batches) != 1 and max(n_batches) == 1:
|
||
if all(op.shape[0] == 1 for op in operands if op.n_batch == 0):
|
||
operands = [bcoo_update_layout(op, n_batch=1) if op.n_batch == 0 else op for op in operands]
|
||
elif all(op.shape[0] == 1 for op in operands if op.n_batch == 1):
|
||
operands = [bcoo_update_layout(op, n_batch=0) if op.n_batch == 1 else op for op in operands]
|
||
n_batches = {op.n_batch for op in operands}
|
||
|
||
if len(n_batches) != 1:
|
||
raise ValueError("bcoo_concatenate requires inputs to have matching batch dimensions.")
|
||
|
||
n_batch, n_sparse = operands[0].n_batch, operands[0].n_sparse
|
||
|
||
index_batches = [op.indices.shape[:n_batch] for op in operands]
|
||
data_batches = [op.data.shape[:n_batch] for op in operands]
|
||
if dimension < n_batch:
|
||
index_batches = [s[:dimension] + s[dimension + 1:] for s in index_batches]
|
||
data_batches = [s[:dimension] + s[dimension + 1:] for s in data_batches]
|
||
if not (len(set(index_batches)) == len(set(data_batches)) == 1):
|
||
raise NotImplementedError("concatenation of arrays with broadcasted batch indices")
|
||
|
||
if dimension < n_batch: # Concatenation along batch axes
|
||
# Ensure nse of operands match.
|
||
nses = {op.nse for op in operands}
|
||
if len(nses) != 1:
|
||
nse = max(nses)
|
||
operands = [_bcoo_set_nse(op, nse) for op in operands]
|
||
new_indices = lax.concatenate([op.indices for op in operands], dimension=dimension)
|
||
new_data = lax.concatenate([op.data for op in operands], dimension=dimension)
|
||
elif dimension < n_batch + n_sparse: # Concatenation along sparse axes
|
||
offsets = np.cumsum([0] + [op.shape[dimension] for op in operands[:-1]],
|
||
dtype=operands[0].indices.dtype)
|
||
new_data = lax.concatenate([op.data for op in operands], dimension=n_batch)
|
||
new_indices = lax.concatenate([op.indices.at[..., dimension - n_batch].add(offset)
|
||
for op, offset in safe_zip(operands, offsets)],
|
||
dimension=n_batch)
|
||
else: # Concatenation along dense axes
|
||
# TODO(jakevdp) should we implement this? In general it results in a wasteful
|
||
# representation because we cannot assume that the indices match.
|
||
raise NotImplementedError("Concatenation along dense dimensions.")
|
||
|
||
return BCOO((new_data, new_indices), shape=out_aval.shape)
|
||
|
||
|
||
def bcoo_reshape(mat: BCOO, *, new_sizes: Sequence[int], dimensions: Sequence[int]) -> BCOO:
|
||
"""Sparse implementation of {func}`jax.lax.reshape`.
|
||
|
||
Args:
|
||
operand: BCOO array to be reshaped.
|
||
new_sizes: sequence of integers specifying the resulting shape. The size
|
||
of the final array must match the size of the input. This must be specified
|
||
such that batch, sparse, and dense dimensions do not mix.
|
||
dimensions: optional sequence of integers specifying the permutation order of
|
||
the input shape. If specified, the length must match ``operand.shape``.
|
||
Additionally, dimensions must only permute among like dimensions of mat:
|
||
batch, sparse, and dense dimensions cannot be permuted.
|
||
|
||
Returns:
|
||
out: reshaped array.
|
||
"""
|
||
if (mat.indices.shape[:mat.n_batch] != mat.data.shape[:mat.n_batch] != mat.shape[:mat.n_batch]):
|
||
# TODO(jakevdp) implement this case via broadcast_in_dim
|
||
raise NotImplementedError("reshape of arrays with broadacsted batch dimensions.")
|
||
|
||
batch_shape, sparse_shape, dense_shape = split_list(mat.shape, [mat.n_batch, mat.n_sparse])
|
||
batch_perm, sparse_perm, dense_perm = _validate_permutation(
|
||
mat.data, mat.indices, dimensions or tuple(range(mat.ndim)), mat.shape)
|
||
batch_size = np.prod(batch_shape, dtype=int)
|
||
sparse_size = np.prod(sparse_shape, dtype=int)
|
||
|
||
cuml_shape = np.cumprod(new_sizes)
|
||
if batch_size != 1 and batch_size not in cuml_shape:
|
||
raise ValueError("bcoo_reshape: new shape cannot mix batch and sparse dimensions; "
|
||
f"got shape={mat.shape} new_shape={new_sizes} with n_batch={mat.n_batch}")
|
||
if sparse_size != 1 and batch_size * sparse_size not in cuml_shape:
|
||
raise ValueError("bcoo_reshape: new shape cannot mix sparse and dense dimensions; "
|
||
f"got shape={mat.shape} new_shape={new_sizes} with n_dense={mat.n_dense}")
|
||
|
||
i1 = cuml_shape.searchsorted(batch_size, side='right')
|
||
i2 = cuml_shape.searchsorted(batch_size * sparse_size, side='right')
|
||
new_batch_shape, new_sparse_shape, new_dense_shape = split_list(new_sizes, [i1, i2])
|
||
|
||
# Reshape batch & dense dimensions: this is accomplished via a standard reshape.
|
||
data = lax.reshape(
|
||
mat.data, new_sizes=(*new_batch_shape, mat.nse, *new_dense_shape),
|
||
dimensions=(*batch_perm, mat.n_batch, *(p + mat.n_batch + 1 for p in dense_perm)))
|
||
indices = lax.reshape(
|
||
mat.indices, new_sizes=(*new_batch_shape, mat.nse, mat.n_sparse),
|
||
dimensions=(*batch_perm, mat.n_batch, mat.n_batch + 1))
|
||
|
||
# Reshape the sparse dimensions: this is accomplished by re-indexing.
|
||
if not new_sparse_shape:
|
||
indices = jnp.empty_like(indices, shape=(*new_batch_shape, mat.nse, 0))
|
||
elif sparse_shape:
|
||
index_cols = tuple(indices[..., i] for i in sparse_perm)
|
||
sparse_shape = [int(mat.shape[mat.n_batch + i]) for i in sparse_perm]
|
||
flat_indices = jnp.ravel_multi_index(index_cols, dims=tuple(sparse_shape), mode='clip')
|
||
with jax.numpy_rank_promotion('allow'):
|
||
oob_indices = (indices >= jnp.array(mat.shape[mat.n_batch: mat.n_batch + mat.n_sparse],
|
||
dtype=indices.dtype)).any(-1, keepdims=True)
|
||
new_index_cols = jnp.unravel_index(flat_indices, new_sparse_shape)
|
||
indices = jnp.concatenate([col[..., None] for col in new_index_cols], axis=-1)
|
||
indices = jnp.where(oob_indices, jnp.array(new_sparse_shape, dtype=indices.dtype), indices)
|
||
|
||
return BCOO((data, indices), shape=new_sizes)
|
||
|
||
|
||
def bcoo_rev(operand, dimensions):
|
||
"""Sparse implementation of {func}`jax.lax.rev`"""
|
||
# Check validity of dimensions via original implementation.
|
||
_ = jax.eval_shape(partial(lax.rev, dimensions=dimensions),
|
||
jax.ShapeDtypeStruct(operand.shape, operand.dtype))
|
||
batch_dims = [d for d in dimensions if d < operand.n_batch]
|
||
sparse_dims = [d for d in dimensions if operand.n_batch <= d < operand.n_batch + operand.n_sparse]
|
||
dense_dims = [d for d in dimensions if d >= operand.n_batch + operand.n_sparse]
|
||
|
||
data, indices = operand.data, operand.indices
|
||
|
||
if batch_dims:
|
||
indices = lax.rev(indices, dimensions=batch_dims)
|
||
if batch_dims or dense_dims:
|
||
data = lax.rev(data, dimensions=batch_dims + [d + 1 - operand.n_sparse for d in dense_dims])
|
||
|
||
if sparse_dims:
|
||
sparse_shape = jnp.array(operand.shape[operand.n_batch: operand.n_batch + operand.n_sparse],
|
||
dtype=indices.dtype)
|
||
spdims = jnp.array([d - operand.n_batch for d in sparse_dims])
|
||
indices = indices.at[..., spdims].mul(-1)
|
||
indices = indices.at[..., spdims].add(sparse_shape[spdims] - 1)
|
||
indices = jnp.where(indices < 0, sparse_shape, indices)
|
||
|
||
return BCOO((data, indices), shape=operand.shape)
|
||
|
||
|
||
def bcoo_squeeze(arr: BCOO, *, dimensions: Sequence[int]) -> BCOO:
|
||
"""Sparse implementation of {func}`jax.lax.squeeze`.
|
||
|
||
Squeeze any number of size 1 dimensions from an array.
|
||
|
||
Args:
|
||
arr: BCOO array to be reshaped.
|
||
dimensions: sequence of integers specifying dimensions to squeeze.
|
||
|
||
Returns:
|
||
out: reshaped array.
|
||
"""
|
||
dimensions = tuple(canonicalize_axis(dim, arr.ndim) for dim in dimensions)
|
||
if any(arr.shape[dim] != 1 for dim in dimensions):
|
||
raise ValueError("cannot select an axis to squeeze out which has size not equal to one, "
|
||
f"got shape={arr.shape} and {dimensions=}")
|
||
batch_dims = tuple(d for d in dimensions if d < arr.n_batch)
|
||
sparse_dims = np.array([i for i in range(arr.n_sparse)
|
||
if i + arr.n_batch not in dimensions], dtype=int)
|
||
dense_dims = tuple(d - arr.n_sparse + 1 for d in dimensions
|
||
if d >= arr.n_batch + arr.n_sparse)
|
||
data_out = lax.squeeze(arr.data, batch_dims + dense_dims)
|
||
indices_out = lax.squeeze(arr.indices[..., sparse_dims], batch_dims)
|
||
out_shape = tuple(s for i, s in enumerate(arr.shape) if i not in dimensions)
|
||
return BCOO((data_out, indices_out), shape=out_shape,
|
||
indices_sorted=arr.indices_sorted, unique_indices=arr.unique_indices)
|
||
|
||
|
||
def bcoo_slice(mat: BCOO, *, start_indices: Sequence[int], limit_indices: Sequence[int],
|
||
strides: Optional[Sequence[int]] = None) -> BCOO:
|
||
"""Sparse implementation of {func}`jax.lax.slice`.
|
||
|
||
Args:
|
||
mat: BCOO array to be reshaped.
|
||
start_indices: sequence of integers of length `mat.ndim` specifying the starting
|
||
indices of each slice.
|
||
limit_indices: sequence of integers of length `mat.ndim` specifying the ending
|
||
indices of each slice
|
||
strides: (not implemented) sequence of integers of length `mat.ndim` specifying
|
||
the stride for each slice
|
||
|
||
Returns:
|
||
out: BCOO array containing the slice.
|
||
"""
|
||
if not isinstance(mat, BCOO):
|
||
raise ValueError(f"bcoo_slice: input should be BCOO array, got type(mat)={type(mat)}")
|
||
start_indices = [operator.index(i) for i in start_indices]
|
||
limit_indices = [operator.index(i) for i in limit_indices]
|
||
if strides is not None:
|
||
strides = [operator.index(i) for i in strides]
|
||
else:
|
||
strides = [1] * mat.ndim
|
||
if len(start_indices) != len(limit_indices) != len(strides) != mat.ndim:
|
||
raise ValueError(f"bcoo_slice: indices must have size mat.ndim={mat.ndim}")
|
||
if len(strides) != mat.ndim:
|
||
raise ValueError(f"len(strides) = {len(strides)}; expected {mat.ndim}")
|
||
if any(s <= 0 for s in strides):
|
||
raise ValueError(f"strides must be a sequence of positive integers; got {strides}")
|
||
|
||
if not all(0 <= start <= end <= size
|
||
for start, end, size in safe_zip(start_indices, limit_indices, mat.shape)):
|
||
raise ValueError(f"bcoo_slice: invalid indices. Got {start_indices=}, "
|
||
f"{limit_indices=} and shape={mat.shape}")
|
||
|
||
start_batch, start_sparse, start_dense = split_list(start_indices, [mat.n_batch, mat.n_sparse])
|
||
end_batch, end_sparse, end_dense = split_list(limit_indices, [mat.n_batch, mat.n_sparse])
|
||
stride_batch, stride_sparse, stride_dense = split_list(strides, [mat.n_batch, mat.n_sparse])
|
||
|
||
data_slices = []
|
||
index_slices = []
|
||
for i, (start, end, stride) in enumerate(zip(start_batch, end_batch, stride_batch)):
|
||
data_slices.append(slice(None) if mat.data.shape[i] != mat.shape[i] else slice(start, end, stride))
|
||
index_slices.append(slice(None) if mat.indices.shape[i] != mat.shape[i] else slice(start, end, stride))
|
||
data_slices.append(slice(None))
|
||
index_slices.extend([slice(None), slice(None)])
|
||
for i, (start, end, stride) in enumerate(zip(start_dense, end_dense, stride_dense)):
|
||
data_slices.append(slice(start, end, stride))
|
||
new_data = mat.data[tuple(data_slices)]
|
||
new_indices = mat.indices[tuple(index_slices)]
|
||
new_shape = tuple(
|
||
(end - start + stride - 1) // stride
|
||
for start, end, stride in safe_zip(start_indices, limit_indices, strides))
|
||
_, new_shape_sparse, _ = split_list(new_shape, [mat.n_batch, mat.n_sparse])
|
||
|
||
if mat.n_sparse:
|
||
starts = jnp.expand_dims(jnp.array(start_sparse, dtype=new_indices.dtype), range(mat.n_batch + 1))
|
||
ends = jnp.expand_dims(jnp.array(end_sparse, dtype=new_indices.dtype), range(mat.n_batch + 1))
|
||
strides_ = jnp.expand_dims(jnp.array(stride_sparse, dtype=new_indices.dtype), range(mat.n_batch + 1))
|
||
|
||
keep = jnp.all((new_indices >= starts) & (new_indices < ends) &
|
||
((new_indices - starts) % strides_ == 0),
|
||
axis=-1, keepdims=True)
|
||
new_indices = jnp.where(keep, (new_indices - starts + strides_ - 1) // strides_,
|
||
(ends - starts + strides_ - 1) // strides_)
|
||
|
||
keep_data = lax.expand_dims(keep[..., 0], range(mat.n_batch + 1, mat.n_batch + 1 + mat.n_dense))
|
||
new_data = jnp.where(keep_data, new_data, 0)
|
||
|
||
new_nse = int(np.prod(new_shape_sparse))
|
||
if mat.nse > new_nse:
|
||
new_data, new_indices = _bcoo_sum_duplicates(
|
||
new_data, new_indices, spinfo=SparseInfo(shape=new_shape), nse=new_nse)
|
||
|
||
return BCOO((new_data, new_indices), shape=new_shape)
|
||
|
||
def bcoo_dynamic_slice(mat: BCOO, start_indices: Sequence[Any], slice_sizes: Sequence[int]) -> BCOO:
|
||
"""Sparse implementation of {func}`jax.lax.dynamic_slice`.
|
||
|
||
Args:
|
||
mat: BCOO array to slice.
|
||
start_indices: a list of scalar indices, one per dimension. These values
|
||
may be dynamic.
|
||
slice_sizes: the size of the slice. Must be a sequence of non-negative
|
||
integers with length equal to `ndim(operand)`. Inside a JIT compiled
|
||
function, only static values are supported (all JAX arrays inside JIT
|
||
must have statically known size).
|
||
|
||
Returns:
|
||
out: BCOO array containing the slice.
|
||
"""
|
||
# Use abstract eval to validate inputs.
|
||
jax.eval_shape(partial(lax.dynamic_slice, slice_sizes=slice_sizes),
|
||
jax.ShapeDtypeStruct(mat.shape, mat.dtype), start_indices)
|
||
if not isinstance(mat, BCOO):
|
||
raise ValueError(f"bcoo_slice: input should be BCOO array, got type(mat)={type(mat)}")
|
||
start_indices = tuple(jnp.asarray(i) for i in start_indices)
|
||
assert all(jnp.issubdtype(i.dtype, np.integer) for i in start_indices)
|
||
assert all(i.shape == () for i in start_indices)
|
||
slice_sizes = tuple(operator.index(i) for i in slice_sizes)
|
||
if len(start_indices) != len(slice_sizes) != mat.ndim:
|
||
raise ValueError(f"bcoo_dynamic_slice: indices must have size mat.ndim={mat.ndim}")
|
||
if not all(0 <= slice_size <= axis_size for slice_size, axis_size in zip(slice_sizes, mat.shape)):
|
||
raise TypeError("slice_sizes must be less than or equal to operand shape, "
|
||
f"got slice_sizes {slice_sizes} for operand shape {mat.shape}")
|
||
|
||
start_batch, start_sparse, start_dense = split_list(start_indices, [mat.n_batch, mat.n_sparse])
|
||
size_batch, size_sparse, size_dense = split_list(slice_sizes, [mat.n_batch, mat.n_sparse])
|
||
|
||
data_start = []
|
||
data_sizes = []
|
||
indices_start = []
|
||
indices_sizes = []
|
||
zero = _const(start_indices[0] if start_indices else np.int32, 0)
|
||
for i, (start, size) in enumerate(zip(start_batch, size_batch)):
|
||
data_is_broadcast = mat.data.shape[i] != mat.shape[i]
|
||
indices_is_broadcast = mat.indices.shape[i] != mat.shape[i]
|
||
data_start.append(zero if data_is_broadcast else start)
|
||
data_sizes.append(1 if data_is_broadcast else size)
|
||
indices_start.append(zero if indices_is_broadcast else start)
|
||
indices_sizes.append(1 if indices_is_broadcast else size)
|
||
data_start.append(zero)
|
||
data_sizes.append(mat.nse)
|
||
indices_start.extend([zero, zero])
|
||
indices_sizes.extend([mat.nse, mat.n_sparse])
|
||
data_start.extend(start_dense)
|
||
data_sizes.extend(size_dense)
|
||
|
||
new_data = lax.dynamic_slice(mat.data, data_start, data_sizes)
|
||
new_indices = lax.dynamic_slice(mat.indices, indices_start, indices_sizes)
|
||
new_shape = slice_sizes
|
||
|
||
if mat.n_sparse:
|
||
starts = jnp.array(start_sparse, dtype=new_indices.dtype)
|
||
sizes = jnp.array(size_sparse, dtype=new_indices.dtype)
|
||
sparse_shape = jnp.array(mat.shape[mat.n_batch: mat.n_batch + mat.n_sparse], dtype=new_indices.dtype)
|
||
starts = jnp.where(starts < 0, starts + sparse_shape, starts)
|
||
starts = jnp.clip(starts, 0, sparse_shape - sizes)
|
||
|
||
starts = jnp.expand_dims(starts, range(mat.n_batch + 1))
|
||
sizes = jnp.expand_dims(sizes, range(mat.n_batch + 1))
|
||
sparse_shape = jnp.expand_dims(sparse_shape, range(mat.n_batch + 1))
|
||
|
||
keep = jnp.all((new_indices >= starts) & (new_indices < starts + sizes), -1, keepdims=True)
|
||
new_indices = jnp.where(keep, new_indices - starts, sizes)
|
||
|
||
keep_data = lax.expand_dims(keep[..., 0], range(mat.n_batch + 1, mat.n_batch + 1 + mat.n_dense))
|
||
new_data = jnp.where(keep_data, new_data, 0)
|
||
|
||
if mat.nse > np.prod(size_sparse):
|
||
new_nse = int(np.prod(size_sparse))
|
||
new_data, new_indices = _bcoo_sum_duplicates(
|
||
new_data, new_indices, spinfo=SparseInfo(shape=new_shape), nse=new_nse)
|
||
|
||
return BCOO((new_data, new_indices), shape=new_shape)
|
||
|
||
|
||
def _tuple_replace(tup, ind, val):
|
||
return tuple(val if i == ind else t for i, t in enumerate(tup))
|
||
|
||
def bcoo_reduce_sum(mat: BCOO, *, axes: Sequence[int]) -> BCOO:
|
||
"""Sum array element over given axes.
|
||
|
||
Args:
|
||
mat: A BCOO-format array.
|
||
shape: The shape of the target array.
|
||
axes: A tuple or list or ndarray which contains axes of ``mat`` over which
|
||
sum is performed.
|
||
|
||
Returns:
|
||
A BCOO-format array containing the result.
|
||
"""
|
||
out_data, out_indices, out_shape = _bcoo_reduce_sum(
|
||
mat.data, mat.indices, spinfo=mat._info, axes=axes)
|
||
return BCOO((out_data, out_indices), shape=out_shape)
|
||
|
||
def _bcoo_reduce_sum(data: Array, indices: Array, *, spinfo: SparseInfo, axes: Sequence[int]) -> Tuple[Array, Array, Shape]:
|
||
shape = spinfo.shape
|
||
assert all(0 <= a < len(shape) for a in axes)
|
||
n_batch, n_sparse, _, nse = _validate_bcoo(data, indices, shape)
|
||
axes = sorted(set(axes))
|
||
|
||
# Sum over dense dimensions -> sum over data
|
||
dense_axes = tuple(ax - n_sparse + 1 for ax in axes if ax >= n_batch + n_sparse)
|
||
data = data.sum(dense_axes)
|
||
if n_sparse:
|
||
# zero-out data corresponding to invalid indices.
|
||
fill_value = jnp.expand_dims(
|
||
jnp.array(shape[n_batch: n_batch + n_sparse], dtype=indices.dtype),
|
||
range(indices.ndim - 1))
|
||
mask = jnp.all(indices < fill_value, -1)
|
||
if data.ndim > mask.ndim:
|
||
mask = lax.expand_dims(mask, tuple(range(mask.ndim, data.ndim)))
|
||
data = jnp.where(mask, data, 0)
|
||
|
||
# Sum over sparse dimensions -> drop index; sum is implicit
|
||
sparse_idx = [i for i in range(n_sparse) if i + n_batch not in axes]
|
||
if not sparse_idx:
|
||
indices = jnp.zeros(_tuple_replace(indices.shape, n_batch + 1, 0), indices.dtype)
|
||
else:
|
||
indices = indices[..., np.array(sparse_idx)]
|
||
|
||
# Sum over batch dimensions -> reshape into nse
|
||
batch_axes = {ax for ax in axes if ax < n_batch}
|
||
|
||
# First handle broadcasted batch dimensions
|
||
for ax in batch_axes:
|
||
if data.shape[ax] == 1:
|
||
if indices.shape[ax] == 1:
|
||
data = data * shape[ax]
|
||
else:
|
||
data = lax.broadcast_in_dim(data, _tuple_replace(data.shape, ax, shape[ax]), tuple(range(data.ndim)))
|
||
else:
|
||
if indices.shape[ax] == 1:
|
||
data = data.sum(ax)
|
||
assert data.shape[ax] == indices.shape[ax]
|
||
|
||
new_batch_dims = tuple(sorted(set(range(n_batch)) - batch_axes))
|
||
new_batch_shape = tuple(data.shape[i] for i in new_batch_dims)
|
||
new_nse = int(nse * np.prod([data.shape[i] for i in batch_axes]))
|
||
|
||
data = lax.reshape(data,
|
||
(*new_batch_shape, new_nse, *data.shape[n_batch + 1:]),
|
||
(*new_batch_dims, *batch_axes, *range(n_batch, data.ndim)))
|
||
indices = lax.reshape(indices,
|
||
(*new_batch_shape, new_nse, *indices.shape[n_batch + 1:]),
|
||
(*new_batch_dims, *batch_axes, *range(n_batch, indices.ndim)))
|
||
|
||
out_shape = tuple(shape[i] for i in range(len(shape)) if i not in axes)
|
||
return data, indices, out_shape
|
||
|
||
def bcoo_multiply_sparse(lhs: BCOO, rhs: BCOO) -> BCOO:
|
||
"""An element-wise multiplication of two sparse arrays.
|
||
|
||
Args:
|
||
lhs: A BCOO-format array.
|
||
rhs: A BCOO-format array.
|
||
|
||
Returns:
|
||
An BCOO-format array containing the result.
|
||
"""
|
||
out_data, out_indices, out_shape = _bcoo_multiply_sparse(
|
||
lhs.data, lhs.indices, rhs.data, rhs.indices, lhs_spinfo=lhs._info,
|
||
rhs_spinfo=rhs._info)
|
||
return BCOO((out_data, out_indices), shape=out_shape)
|
||
|
||
def _bcoo_multiply_sparse(lhs_data: Array, lhs_indices: Array, rhs_data: Array, rhs_indices: Array, *,
|
||
lhs_spinfo: SparseInfo, rhs_spinfo: SparseInfo) -> Tuple[Array, Array, Shape]:
|
||
lhs_shape = lhs_spinfo.shape
|
||
rhs_shape = rhs_spinfo.shape
|
||
|
||
lhs = _validate_bcoo(lhs_data, lhs_indices, lhs_shape)
|
||
rhs = _validate_bcoo(rhs_data, rhs_indices, rhs_shape)
|
||
if len(lhs_shape) != len(rhs_shape):
|
||
# Similar requirement as lax.mul:
|
||
raise TypeError("bcoo_multiply_sparse: arrays must have same number of dimensions, "
|
||
f"got {lhs_shape}, {rhs_shape}")
|
||
if lhs.n_dense != rhs.n_dense:
|
||
raise NotImplementedError("bcoo_multiply_sparse: arrays with differing numbers of "
|
||
f"dense dimensions: {lhs}, {rhs}")
|
||
n_batch = min(lhs.n_batch, rhs.n_batch)
|
||
_mul = functools.partial(_bcoo_multiply_sparse_unbatched,
|
||
lhs_shape=lhs_shape[n_batch:],
|
||
rhs_shape=rhs_shape[n_batch:])
|
||
_mul = nfold_vmap(_mul, n_batch)
|
||
data, indices = _mul(lhs_data, lhs_indices, rhs_data, rhs_indices)
|
||
return data, indices, jnp.broadcast_shapes(lhs_shape, rhs_shape)
|
||
|
||
def _bcoo_multiply_sparse_unbatched(lhs_data, lhs_indices, rhs_data, rhs_indices, *, lhs_shape, rhs_shape):
|
||
lhs = _validate_bcoo(lhs_data, lhs_indices, lhs_shape)
|
||
rhs = _validate_bcoo(rhs_data, rhs_indices, rhs_shape)
|
||
assert (lhs.n_batch == 0) or (rhs.n_batch == 0) # Ensured at call site above
|
||
|
||
# TODO(jakevdp): this can be made more efficient by utilizing batch structure.
|
||
if lhs.n_batch:
|
||
lhs_data, lhs_indices = bcoo_update_layout(BCOO((lhs_data, lhs_indices), shape=lhs_shape), n_batch=0)._bufs
|
||
lhs = _validate_bcoo(lhs_data, lhs_indices, lhs_shape)
|
||
elif rhs.n_batch:
|
||
rhs_data, rhs_indices = bcoo_update_layout(BCOO((rhs_data, rhs_indices), shape=rhs_shape), n_batch=0)._bufs
|
||
rhs = _validate_bcoo(rhs_data, rhs_indices, rhs_shape)
|
||
dims = jnp.array([i for i, (s1, s2) in enumerate(safe_zip(lhs_shape[:lhs.n_sparse], rhs_shape[:rhs.n_sparse]))
|
||
if s1 != 1 and s2 != 1], dtype=int)
|
||
|
||
# TODO(jakevdp): this nse can be tightened to min(lhs.nse, rhs.nse) if there
|
||
# is no broadcasting and indices are unique.
|
||
nse = lhs.nse * rhs.nse
|
||
|
||
# TODO(jakevdp): this is pretty inefficient. Can we do this membership check
|
||
# without constructing the full (lhs.nse, rhs.nse) masking matrix?
|
||
mask = jnp.all(lhs_indices[:, None, dims] == rhs_indices[None, :, dims], -1)
|
||
i_lhs, i_rhs = jnp.nonzero(mask, size=nse, fill_value=(lhs.nse, rhs.nse))
|
||
data = (lhs_data.at[i_lhs].get(mode='fill', fill_value=0) *
|
||
rhs_data.at[i_rhs].get(mode='fill', fill_value=0))
|
||
indices = jnp.maximum(
|
||
lhs_indices.at[i_lhs].get(mode='fill', fill_value=max(lhs_shape, default=0)),
|
||
rhs_indices.at[i_rhs].get(mode='fill', fill_value=max(rhs_shape, default=0)))
|
||
return data, indices
|
||
|
||
def bcoo_multiply_dense(sp_mat: BCOO, v: Array) -> Array:
|
||
"""An element-wise multiplication between a sparse and a dense array.
|
||
|
||
Args:
|
||
lhs: A BCOO-format array.
|
||
rhs: An ndarray.
|
||
|
||
Returns:
|
||
An ndarray containing the result.
|
||
"""
|
||
return _bcoo_multiply_dense(sp_mat.data, sp_mat.indices, v, spinfo=sp_mat._info)
|
||
|
||
def _bcoo_multiply_dense(data: Array, indices: Array, v: Array, *, spinfo: SparseInfo) -> Array:
|
||
"""Broadcasted elementwise multiplication between a BCOO array and a dense array."""
|
||
# TODO(jakevdp): the logic here is similar to bcoo_extract... can we reuse that?
|
||
shape = spinfo.shape
|
||
if v.ndim == 0:
|
||
return lax.mul(data, v)
|
||
if shape == v.shape:
|
||
# Note: due to distributive property, no deduplication necessary!
|
||
return lax.mul(data, _bcoo_extract(indices, v))
|
||
|
||
if lax.broadcast_shapes(v.shape, shape) != shape:
|
||
raise NotImplementedError(
|
||
"multiplication between sparse and dense is only implemented for cases "
|
||
"where the output shape matches the sparse matrix shape. Got "
|
||
f"{shape=}, {v.shape=}")
|
||
v = lax.expand_dims(v, range(len(shape) - v.ndim))
|
||
|
||
props = _validate_bcoo(data, indices, shape)
|
||
|
||
@partial(nfold_vmap, N=props.n_batch)
|
||
def _mul(data, indices, v):
|
||
assert indices.shape[1] == v.ndim - props.n_dense
|
||
ind = tuple(indices[:, i] for i in range(indices.shape[1]))
|
||
ind = tuple(i if s != 1 else 0 for i, s in zip(ind, v.shape))
|
||
return data * v[ind]
|
||
return _mul(data, indices, v)
|
||
|
||
def bcoo_gather(operand: BCOO, start_indices: Array,
|
||
dimension_numbers: GatherDimensionNumbers,
|
||
slice_sizes: Shape, *,
|
||
unique_indices: bool = False,
|
||
indices_are_sorted: bool = False,
|
||
mode: Optional[Union[str, GatherScatterMode]] = None,
|
||
fill_value = None) -> BCOO:
|
||
"""BCOO version of lax.gather."""
|
||
_validate_bcoo(operand.data, operand.indices, operand.shape)
|
||
|
||
# TODO(jakevdp) make use of unique_indices and indices_are_sorted?
|
||
if mode is None:
|
||
mode = GatherScatterMode.PROMISE_IN_BOUNDS
|
||
parsed_mode = GatherScatterMode.from_any(mode)
|
||
if parsed_mode != GatherScatterMode.PROMISE_IN_BOUNDS:
|
||
raise NotImplementedError(f"bcoo_gather: {mode=} not yet supported.")
|
||
|
||
kwds = dict(dimension_numbers=dimension_numbers, slice_sizes=slice_sizes,
|
||
unique_indices=unique_indices, indices_are_sorted=indices_are_sorted,
|
||
mode=mode, fill_value=fill_value)
|
||
|
||
# Abstract eval lax.gather to validate arguments & determine output shape.
|
||
out_aval = jax.eval_shape(partial(lax.gather, **kwds),
|
||
jax.ShapeDtypeStruct(operand.shape, operand.dtype),
|
||
jax.ShapeDtypeStruct(start_indices.shape, start_indices.dtype))
|
||
offset_dims = dimension_numbers.offset_dims
|
||
collapsed_slice_dims = dimension_numbers.collapsed_slice_dims
|
||
start_index_map = dimension_numbers.start_index_map
|
||
|
||
# Expand start_indices & slice_sizes to full rank & use bcoo_dynamic_slice
|
||
full_start_indices: List[ArrayLike] = [_const(start_indices, 0)] * operand.ndim
|
||
in_axes: List[Optional[int]] = [None for i in range(operand.ndim)]
|
||
full_slice_sizes = list(operand.shape)
|
||
for i, j in enumerate(start_index_map):
|
||
full_start_indices[j] = start_indices[..., i].ravel()
|
||
full_slice_sizes[j] = slice_sizes[j]
|
||
in_axes[j] = 0
|
||
def slice_func(indices):
|
||
slc = bcoo_dynamic_slice(operand, indices, slice_sizes=full_slice_sizes)
|
||
return bcoo_squeeze(slc, dimensions=collapsed_slice_dims)
|
||
result = vmap(slice_func, in_axes=(in_axes,))(full_start_indices)
|
||
result = bcoo_reshape(result,
|
||
new_sizes=(*start_indices.shape[:-1], *result.shape[1:]),
|
||
dimensions=tuple(range(result.ndim)))
|
||
|
||
# Use offset_dims to permute result dimensions
|
||
if result.shape:
|
||
batch_dims = tuple(dim for dim in range(len(out_aval.shape))
|
||
if dim not in offset_dims)
|
||
permutation = np.zeros(result.ndim, dtype=int)
|
||
permutation[np.array(batch_dims + offset_dims)] = np.arange(result.ndim)
|
||
if set(permutation[:len(batch_dims)]) != set(range(len(batch_dims))):
|
||
# TODO: jakevdp more granular approach here. Can we do this in a
|
||
# way that preserves the original batch dimensions?
|
||
result = bcoo_update_layout(result, n_batch=0)
|
||
result = bcoo_transpose(result, permutation=tuple(permutation))
|
||
|
||
return result.reshape(out_aval.shape).astype(out_aval.dtype)
|
||
|
||
def bcoo_conv_general_dilated(lhs, rhs, *, window_strides, padding,
|
||
lhs_dilation=None, rhs_dilation=None, dimension_numbers=None,
|
||
feature_group_count=1, batch_group_count=1, precision=None,
|
||
preferred_element_type=None) -> BCOO:
|
||
# Validate and process parameters using lax.conv_general_dilated abstract evaluation.
|
||
func = functools.partial(
|
||
lax.conv_general_dilated,
|
||
window_strides=window_strides, padding=padding,
|
||
lhs_dilation=lhs_dilation, rhs_dilation=rhs_dilation, dimension_numbers=dimension_numbers,
|
||
feature_group_count=feature_group_count, batch_group_count=batch_group_count,
|
||
precision=precision, preferred_element_type=preferred_element_type)
|
||
jaxpr = jax.make_jaxpr(func)(jax.ShapeDtypeStruct(lhs.shape, lhs.dtype),
|
||
jax.ShapeDtypeStruct(rhs.shape, rhs.dtype))
|
||
assert len(jaxpr.eqns) == 1
|
||
params = jaxpr.eqns[0].params
|
||
|
||
if params['lhs_dilation'] != (1,) * (lhs.ndim - 2):
|
||
raise NotImplementedError("bcoo convolution with lhs_dilation.")
|
||
if params['rhs_dilation'] != (1,) * (rhs.ndim - 2):
|
||
raise NotImplementedError("bcoo convolution with lhs_dilation.")
|
||
if params['window_strides'] != (1,) * (lhs.ndim - 2):
|
||
raise NotImplementedError("bcoo convolution with non-unit window_strides.")
|
||
if params['batch_group_count'] != params['feature_group_count'] != 1:
|
||
raise NotImplementedError("bcoo convolution with non-unit group counts.")
|
||
|
||
if lhs.shape[:2] != rhs.shape[:2] != (1, 1):
|
||
raise NotImplementedError("bcoo convolution with leading dimensions other than (1, 1)")
|
||
|
||
index_dtype = (lhs.indices.dtype if hasattr(lhs, 'indices')
|
||
else rhs.indices.dtype if hasattr(rhs, 'indices')
|
||
else 'int32')
|
||
|
||
padding, = params['padding']
|
||
return _bcoo_conv_1d(_convert_to_1d_for_conv(lhs, index_dtype),
|
||
_convert_to_1d_for_conv(rhs, index_dtype),
|
||
padding=padding)
|
||
|
||
def _convert_to_1d_for_conv(mat, index_dtype):
|
||
if isinstance(mat, (jax.Array, np.ndarray)):
|
||
data = lax.squeeze(mat, (0, 1))
|
||
indices = lax.broadcasted_iota(index_dtype, (len(data), 1), 0)
|
||
elif isinstance(mat, BCOO):
|
||
mat = mat.update_layout(n_batch=2, n_dense=0)
|
||
data = lax.squeeze(mat.data, (0, 1))
|
||
indices = lax.squeeze(mat.indices, (0, 1))
|
||
# zero-out data at OOB indices, otherwise strange things happen.
|
||
data = jnp.where(lax.squeeze(indices, (1,)) < mat.shape[-1], data, 0)
|
||
else:
|
||
raise ValueError(f"bcoo_conv_general_dilated: input of type {type(mat)} not recognized.")
|
||
return BCOO((data, indices), shape=mat.shape[2:])
|
||
|
||
def _bcoo_conv_1d(lhs: BCOO, rhs: BCOO, padding: Sequence[int]) -> BCOO:
|
||
assert lhs.ndim == lhs.n_sparse == rhs.ndim == rhs.n_sparse == 1
|
||
assert lhs.dtype == rhs.dtype
|
||
padding = tuple(map(int, padding))
|
||
assert len(padding) == 2
|
||
|
||
new_data = (lhs.data[:, None] * rhs.data[None, :]).ravel()
|
||
|
||
offset = padding[0] - rhs.indices
|
||
new_indices = (lhs.indices[:, None] + offset[None, :]).ravel()
|
||
|
||
mask = (new_indices < 0)
|
||
new_indices = jnp.where(mask, 0, new_indices)
|
||
new_data = jnp.where(mask, 0, new_data)
|
||
dimsize = max(0, lhs.shape[0] + padding[0] + padding[1] - rhs.shape[0] + 1)
|
||
|
||
new_data = lax.expand_dims(new_data, (0, 1))
|
||
new_indices = lax.expand_dims(new_indices, (0, 1, 3))
|
||
return BCOO((new_data, new_indices), shape=(1, 1, dimsize))
|
||
|
||
|
||
@tree_util.register_pytree_node_class
|
||
class BCOO(JAXSparse):
|
||
"""Experimental batched COO matrix implemented in JAX
|
||
|
||
Args:
|
||
(data, indices) : data and indices in batched COO format.
|
||
shape : shape of sparse array.
|
||
|
||
Attributes:
|
||
data : ndarray of shape ``[*batch_dims, nse, *dense_dims]`` containing the
|
||
explicitly stored data within the sparse matrix.
|
||
indices : ndarray of shape ``[*batch_dims, nse, n_sparse]`` containing the
|
||
indices of the explicitly stored data. Duplicate entries will be summed.
|
||
|
||
Examples:
|
||
Create a sparse array from a dense array:
|
||
|
||
>>> M = jnp.array([[0., 2., 0.], [1., 0., 4.]])
|
||
>>> M_sp = BCOO.fromdense(M)
|
||
>>> M_sp
|
||
BCOO(float32[2, 3], nse=3)
|
||
|
||
Examine the internal representation:
|
||
|
||
>>> M_sp.data
|
||
Array([2., 1., 4.], dtype=float32)
|
||
>>> M_sp.indices
|
||
Array([[0, 1],
|
||
[1, 0],
|
||
[1, 2]], dtype=int32)
|
||
|
||
Create a dense array from a sparse array:
|
||
|
||
>>> M_sp.todense()
|
||
Array([[0., 2., 0.],
|
||
[1., 0., 4.]], dtype=float32)
|
||
|
||
Create a sparse array from COO data & indices:
|
||
|
||
>>> data = jnp.array([1., 3., 5.])
|
||
>>> indices = jnp.array([[0, 0],
|
||
... [1, 1],
|
||
... [2, 2]])
|
||
>>> mat = BCOO((data, indices), shape=(3, 3))
|
||
>>> mat
|
||
BCOO(float32[3, 3], nse=3)
|
||
>>> mat.todense()
|
||
Array([[1., 0., 0.],
|
||
[0., 3., 0.],
|
||
[0., 0., 5.]], dtype=float32)
|
||
"""
|
||
# Note: additional BCOO methods are defined in transform.py
|
||
|
||
data: Array
|
||
indices: Array
|
||
shape: Shape
|
||
nse = property(lambda self: self.indices.shape[-2])
|
||
dtype = property(lambda self: self.data.dtype)
|
||
n_batch = property(lambda self: self.indices.ndim - 2)
|
||
n_sparse = property(lambda self: self.indices.shape[-1])
|
||
n_dense = property(lambda self: self.data.ndim - 1 - self.n_batch)
|
||
indices_sorted: bool
|
||
unique_indices: bool
|
||
_info = property(lambda self: SparseInfo(self.shape, self.indices_sorted,
|
||
self.unique_indices))
|
||
_bufs = property(lambda self: (self.data, self.indices))
|
||
|
||
def __init__(self, args: Tuple[Array, Array], *, shape: Sequence[int],
|
||
indices_sorted: bool = False, unique_indices: bool = False):
|
||
self.data, self.indices = map(jnp.asarray, args)
|
||
self.indices_sorted = indices_sorted
|
||
self.unique_indices = unique_indices
|
||
super().__init__(args, shape=tuple(shape))
|
||
_validate_bcoo(self.data, self.indices, self.shape)
|
||
|
||
def __repr__(self):
|
||
name = self.__class__.__name__
|
||
try:
|
||
nse = self.nse
|
||
n_batch = self.n_batch
|
||
n_dense = self.n_dense
|
||
dtype = self.dtype
|
||
shape = list(self.shape)
|
||
except:
|
||
repr_ = f"{name}(<invalid>)"
|
||
else:
|
||
extra = f", {nse=}"
|
||
if n_batch: extra += f", {n_batch=}"
|
||
if n_dense: extra += f", {n_dense=}"
|
||
repr_ = f"{name}({dtype}{shape}{extra})"
|
||
if isinstance(self.data, core.Tracer):
|
||
repr_ = f"{type(self.data).__name__}[{repr_}]"
|
||
return repr_
|
||
|
||
# Stub methods: these are defined in transform.py
|
||
def reshape(self, *args, **kwargs) -> BCOO:
|
||
raise NotImplementedError("BCOO.reshape")
|
||
|
||
def astype(self, *args, **kwargs) -> BCOO:
|
||
raise NotImplementedError("BCOO.astype")
|
||
|
||
def sum(self) -> BCOO:
|
||
raise NotImplementedError("BCOO.sum")
|
||
|
||
@classmethod
|
||
def fromdense(cls, mat: Array, *, nse: Optional[int] = None, index_dtype: DTypeLike = np.int32,
|
||
n_dense: int = 0, n_batch: int = 0) -> BCOO:
|
||
"""Create a BCOO array from a (dense) :class:`DeviceArray`."""
|
||
return bcoo_fromdense(
|
||
mat, nse=nse, index_dtype=index_dtype, n_dense=n_dense, n_batch=n_batch)
|
||
|
||
@classmethod
|
||
def from_scipy_sparse(cls, mat, *, index_dtype: Optional[DTypeLike]=None,
|
||
n_dense: int = 0, n_batch: int = 0) -> BCOO:
|
||
"""Create a BCOO array from a :mod:`scipy.sparse` array."""
|
||
if n_dense != 0 or n_batch != 0:
|
||
raise NotImplementedError("BCOO.fromscipy with nonzero n_dense/n_batch")
|
||
|
||
mat = mat.tocoo()
|
||
data = jnp.asarray(mat.data)
|
||
indices = jnp.column_stack((mat.row, mat.col)).astype(
|
||
index_dtype or jnp.int32)
|
||
# TODO: determines sorted and unique indices for scipy conversion.
|
||
return cls((data, indices), shape=mat.shape, indices_sorted=False,
|
||
unique_indices=False)
|
||
|
||
@classmethod
|
||
def _empty(cls, shape: Shape, *, dtype: Optional[DTypeLike] = None, index_dtype: DTypeLike = 'int32',
|
||
n_dense: int = 0, n_batch: int = 0, nse: int = 0) -> BCOO:
|
||
"""Create an empty BCOO instance. Public method is sparse.empty()."""
|
||
shape = tuple(shape)
|
||
n_sparse = len(shape) - n_dense - n_batch
|
||
if n_sparse < 0 or n_dense < 0 or n_batch < 0 or nse < 0:
|
||
raise ValueError(f"Invalid inputs: {shape=}, {n_dense=}, {n_batch=}, {nse=}")
|
||
batch_shape, sparse_shape, dense_shape = split_list(shape, [n_batch, n_sparse])
|
||
data = jnp.zeros((*batch_shape, nse, *dense_shape), dtype)
|
||
indices = jnp.full((*batch_shape, nse, n_sparse), jnp.array(sparse_shape), index_dtype)
|
||
return cls((data, indices), shape=shape, indices_sorted=True,
|
||
unique_indices=True)
|
||
|
||
@classmethod
|
||
def _eye(cls, N: int, M: int, k: int, *, dtype: Optional[DTypeLike] = None,
|
||
index_dtype: DTypeLike = 'int32', n_batch: int = 0, n_dense: int = 0) -> BCOO:
|
||
n_sparse = 2 - n_batch - n_dense
|
||
if n_sparse < 0 or n_dense < 0 or n_batch < 0:
|
||
raise ValueError(f"Invalid inputs: shape={(N, M)}, {n_dense=}, {n_batch=}")
|
||
|
||
if k > 0:
|
||
diag_size = min(N, M - k)
|
||
else:
|
||
diag_size = min(N + k, M)
|
||
|
||
if diag_size <= 0:
|
||
# if k is out of range, return an empty matrix.
|
||
return cls._empty((N, M), dtype=dtype, index_dtype=index_dtype,
|
||
n_batch=n_batch, n_dense=n_dense)
|
||
|
||
if n_dense > 0 or n_batch > 1:
|
||
# These cases explicitly store all the zeros, so fall back to fromdense.
|
||
return cls.fromdense(jnp.eye(N, M, k, dtype=dtype),
|
||
n_batch=n_batch, n_dense=n_dense,
|
||
index_dtype=index_dtype)
|
||
|
||
if n_batch == 0:
|
||
data = jnp.ones(diag_size, dtype=dtype)
|
||
idx = jnp.arange(diag_size, dtype=index_dtype)
|
||
zero = _const(idx, 0)
|
||
k = _const(idx, k)
|
||
indices = jnp.column_stack([
|
||
lax.sub(idx, lax.cond(k >= 0, lambda: zero, lambda: k)),
|
||
lax.add(idx, lax.cond(k <= 0, lambda: zero, lambda: k))])
|
||
else:
|
||
data = jnp.ones(N, dtype=dtype)
|
||
indices = jnp.arange(N, dtype=index_dtype)
|
||
indices = indices + _const(indices, k)
|
||
if k < 0:
|
||
data = data.at[:abs(k)].set(0)
|
||
indices = indices.at[:abs(k)].set(M)
|
||
elif k > 0:
|
||
data = data.at[M - abs(k):].set(0)
|
||
indices = indices.at[M - abs(k)].set(M)
|
||
data = data[:, None]
|
||
indices = indices[:, None, None]
|
||
return cls((data, indices), shape=(N, M), indices_sorted=True,
|
||
unique_indices=True)
|
||
|
||
def update_layout(self, *, n_batch: Optional[int] = None, n_dense: Optional[int] = None,
|
||
on_inefficient: str = 'error') -> BCOO:
|
||
"""Update the storage layout (i.e. n_batch & n_dense) of a BCOO matrix.
|
||
|
||
In many cases this can be done without introducing undue storage overhead. However,
|
||
increasing ``mat.n_batch`` or ``mat.n_dense`` will lead to very inefficient storage,
|
||
with many explicitly-stored zeros, unless the new batch or dense dimensions have size
|
||
0 or 1. In such cases, ``update_layout`` will raise a :class:`SparseEfficiencyError`.
|
||
This can be silenced by specifying the ``on_inefficient`` argument.
|
||
|
||
Args:
|
||
n_batch : optional(int) the number of batch dimensions in the output matrix. If None,
|
||
then n_batch = mat.n_batch.
|
||
n_dense : optional(int) the number of dense dimensions in the output matrix. If None,
|
||
then n_dense = mat.n_dense.
|
||
on_inefficient : optional(string), one of ``['error', 'warn', None]``. Specify the
|
||
behavior in case of an inefficient reconfiguration. This is defined as a reconfiguration
|
||
where the size of the resulting representation is much larger than the size of the
|
||
input representation.
|
||
|
||
Returns:
|
||
mat_out : BCOO array
|
||
A BCOO array representing the same sparse array as the input, with the specified
|
||
layout. ``mat_out.todense()`` will match ``mat.todense()`` up to appropriate precision.
|
||
"""
|
||
return bcoo_update_layout(self, n_batch=n_batch, n_dense=n_dense, on_inefficient=on_inefficient)
|
||
|
||
def sum_duplicates(self, nse: Optional[int] = None, remove_zeros: bool = True) -> BCOO:
|
||
"""Return a copy of the array with duplicate indices summed.
|
||
|
||
Additionally, this operation will result in explicit zero entries removed, and
|
||
indices being sorted in lexicographic order.
|
||
|
||
Because the size of the resulting representation depends on the values in the
|
||
arrays, this operation is not compatible with JIT or other transforms. To use
|
||
``sum_duplicates`` in such cases, you may pass a value to `nse` to specify the
|
||
desired size of the output representation.
|
||
|
||
Args:
|
||
nse : integer (optional), if specified, gives the number of specified elements in
|
||
the output sparse representation; if it is larger than the number required, data
|
||
will be padded with zeros and indices will be padded with out-of-bounds values.
|
||
If it is smaller than the number required, data will be silently discarded.
|
||
remove_zeros : bool (default=True). If True, remove explicit zeros from the data
|
||
as part of summing duplicates. If False, then explicit zeros at unique indices
|
||
will remain among the specified elements. Note: remove_zeros=True is incompatible
|
||
with autodiff.
|
||
"""
|
||
if remove_zeros:
|
||
return bcoo_eliminate_zeros(self, nse=nse)
|
||
else:
|
||
return bcoo_sum_duplicates(self, nse=nse)
|
||
|
||
def sort_indices(self) -> BCOO:
|
||
"""Return a copy of the matrix with indices sorted."""
|
||
return bcoo_sort_indices(self)
|
||
|
||
def todense(self) -> Array:
|
||
"""Create a dense version of the array."""
|
||
return bcoo_todense(self)
|
||
|
||
def transpose(self, axes: Optional[Sequence[int]] = None) -> BCOO:
|
||
"""Create a new array containing the transpose."""
|
||
perm: List[int] = list(range(self.ndim)[::-1] if axes is None else axes)
|
||
mat_T = bcoo_transpose(self, permutation=perm)
|
||
shape_T = tuple(self.shape[i] for i in perm)
|
||
sparse_perm = [p - self.n_batch
|
||
for p in perm[self.n_batch: self.n_batch + self.n_sparse]]
|
||
if tuple(sparse_perm) == tuple(range(self.n_sparse)):
|
||
is_sorted = self.indices_sorted
|
||
else:
|
||
# TODO: address the corner cases that the transposed indices are sorted.
|
||
# possibly use permutation?
|
||
is_sorted = False
|
||
return BCOO((mat_T.data, mat_T.indices), shape=shape_T,
|
||
indices_sorted=is_sorted, unique_indices=self.unique_indices)
|
||
|
||
def tree_flatten(self):
|
||
return (self.data, self.indices), self._info._asdict()
|
||
|
||
@classmethod
|
||
def tree_unflatten(cls, aux_data, children):
|
||
obj = object.__new__(cls)
|
||
obj.data, obj.indices = children
|
||
if aux_data.keys() != {'shape', 'indices_sorted', 'unique_indices'}:
|
||
raise ValueError(f"BCOO.tree_unflatten: invalid {aux_data=}")
|
||
obj.__dict__.update(**aux_data)
|
||
return obj
|
||
|
||
|
||
# vmappable handlers
|
||
def _bcoo_to_elt(cont, _, val, axis):
|
||
if axis is None:
|
||
return val
|
||
if axis >= val.n_batch:
|
||
raise ValueError(f"Cannot map in_axis={axis} for BCOO array with n_batch={val.n_batch}. "
|
||
"in_axes for batched BCOO operations must correspond to a batch dimension.")
|
||
return BCOO((cont(val.data, axis), cont(val.indices, axis)),
|
||
shape=val.shape[:axis] + val.shape[axis + 1:],
|
||
indices_sorted=val.indices_sorted, unique_indices=val.unique_indices)
|
||
|
||
def _bcoo_from_elt(cont, axis_size, elt, axis):
|
||
if axis is None:
|
||
return elt
|
||
if axis > elt.n_batch:
|
||
raise ValueError(f"BCOO: cannot add out_axis={axis} for BCOO array with n_batch={elt.n_batch}. "
|
||
"BCOO batch axes must be a contiguous block of leading dimensions.")
|
||
return BCOO((cont(axis_size, elt.data, axis), cont(axis_size, elt.indices, axis)),
|
||
shape=elt.shape[:axis] + (axis_size,) + elt.shape[axis:],
|
||
indices_sorted=elt.indices_sorted, unique_indices=elt.unique_indices)
|
||
|
||
batching.register_vmappable(BCOO, int, int, _bcoo_to_elt, _bcoo_from_elt, None)
|