mirror of
https://github.com/ROCm/jax.git
synced 2025-04-24 15:56:09 +00:00
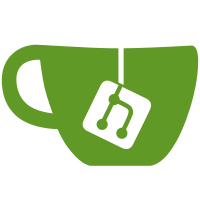
We introduced these shims when migrating from MHLO to StableHLO, and they helped accommodate the version skew between jaxlib and JAX across different environments. Now that a sufficient amount of time has passed, these shims are no longer used anywhere and can be deleted. PiperOrigin-RevId: 510820007
745 lines
23 KiB
Python
745 lines
23 KiB
Python
# Copyright 2018 The JAX Authors.
|
|
#
|
|
# Licensed under the Apache License, Version 2.0 (the "License");
|
|
# you may not use this file except in compliance with the License.
|
|
# You may obtain a copy of the License at
|
|
#
|
|
# https://www.apache.org/licenses/LICENSE-2.0
|
|
#
|
|
# Unless required by applicable law or agreed to in writing, software
|
|
# distributed under the License is distributed on an "AS IS" BASIS,
|
|
# WITHOUT WARRANTIES OR CONDITIONS OF ANY KIND, either express or implied.
|
|
# See the License for the specific language governing permissions and
|
|
# limitations under the License.
|
|
|
|
# Shims that allow the XLA CPU backend to call scipy-provided LAPACK kernels
|
|
# via CustomCallWithLayout.
|
|
|
|
import jaxlib.mlir.ir as ir
|
|
import jaxlib.mlir.dialects.stablehlo as hlo
|
|
|
|
import numpy as np
|
|
from jaxlib import xla_client
|
|
|
|
from .hlo_helpers import custom_call
|
|
from .cpu import _lapack
|
|
|
|
for _name, _value in _lapack.registrations().items():
|
|
xla_client.register_custom_call_target(_name, _value, platform="cpu")
|
|
|
|
# Function that lazily initializes the LAPACK kernels in the runtime on first
|
|
# use.
|
|
_initialize = _lapack.initialize
|
|
|
|
|
|
def _hlo_u8(x):
|
|
return hlo.ConstantOp(
|
|
ir.DenseElementsAttr.get(
|
|
np.array(x, dtype=np.uint8),
|
|
type=ir.IntegerType.get_unsigned(8))).result
|
|
|
|
def _hlo_s32(x):
|
|
return hlo.ConstantOp(
|
|
ir.DenseElementsAttr.get(
|
|
np.array(x, dtype=np.int32),
|
|
type=ir.IntegerType.get_signless(32))).result
|
|
|
|
# TODO(phawkins): it would be nice to avoid duplicating code for each type.
|
|
|
|
# ?trsm(left_side, lower, trans_a, diag, m, n, alpha, a, b):
|
|
# triangular solve
|
|
def trsm_hlo(dtype, alpha, a, b, left_side=False, lower=False, trans_a=False,
|
|
conj_a=False, diag=False):
|
|
_initialize()
|
|
a_type = ir.RankedTensorType(a.type)
|
|
b_type = ir.RankedTensorType(b.type)
|
|
|
|
dims = b_type.shape
|
|
m, n = dims[-2:]
|
|
k = m if left_side else n
|
|
|
|
batch_dims = tuple(dims[:-2])
|
|
num_bd = len(batch_dims)
|
|
num_b = 1
|
|
for d in batch_dims:
|
|
num_b *= d
|
|
|
|
if (batch_dims + (k, k) != tuple(a_type.shape) or
|
|
a_type.element_type != b_type.element_type):
|
|
raise ValueError("Argument mismatch for trsm, got {} and {}".format(
|
|
a_type, b_type))
|
|
|
|
if dtype == np.float32:
|
|
fn = "blas_strsm"
|
|
elif dtype == np.float64:
|
|
fn = "blas_dtrsm"
|
|
elif dtype == np.complex64:
|
|
fn = "blas_ctrsm"
|
|
elif dtype == np.complex128:
|
|
fn = "blas_ztrsm"
|
|
else:
|
|
raise NotImplementedError(f"Unsupported dtype {dtype}")
|
|
|
|
if conj_a and not trans_a:
|
|
raise NotImplementedError("Conjugation without transposition not supported")
|
|
scalar_layout = []
|
|
layout = (num_bd, num_bd + 1) + tuple(range(num_bd - 1, -1, -1))
|
|
return custom_call(
|
|
fn,
|
|
[b.type],
|
|
[_hlo_s32(int(left_side)), _hlo_s32(int(lower)),
|
|
_hlo_s32((2 if conj_a else 1) if trans_a else 0), _hlo_s32(int(diag)),
|
|
_hlo_s32(m), _hlo_s32(n), _hlo_s32(num_b),
|
|
alpha, a, b],
|
|
operand_layouts=[scalar_layout] * 8 + [layout] * 2,
|
|
result_layouts=[layout],
|
|
operand_output_aliases={9: 0},
|
|
)
|
|
|
|
|
|
# # ?getrf: LU decomposition
|
|
|
|
def getrf_hlo(dtype, a):
|
|
_initialize()
|
|
dims = ir.RankedTensorType(a.type).shape
|
|
assert len(dims) >= 2
|
|
m, n = dims[-2:]
|
|
batch_dims = tuple(dims[:-2])
|
|
num_bd = len(batch_dims)
|
|
b = 1
|
|
for d in batch_dims:
|
|
b *= d
|
|
|
|
if dtype == np.float32:
|
|
fn = b"lapack_sgetrf"
|
|
elif dtype == np.float64:
|
|
fn = b"lapack_dgetrf"
|
|
elif dtype == np.complex64:
|
|
fn = b"lapack_cgetrf"
|
|
elif dtype == np.complex128:
|
|
fn = b"lapack_zgetrf"
|
|
else:
|
|
raise NotImplementedError(f"Unsupported dtype {dtype}")
|
|
|
|
scalar_layout = []
|
|
layout = (num_bd, num_bd + 1) + tuple(range(num_bd - 1, -1, -1))
|
|
i32_type = ir.IntegerType.get_signless(32)
|
|
return custom_call(
|
|
fn,
|
|
[
|
|
a.type,
|
|
ir.RankedTensorType.get(batch_dims + (min(m, n),), i32_type),
|
|
ir.RankedTensorType.get(batch_dims, i32_type),
|
|
],
|
|
[_hlo_s32(int(b)), _hlo_s32(m), _hlo_s32(n), a],
|
|
operand_layouts=[scalar_layout] * 3 + [layout],
|
|
result_layouts=[
|
|
layout,
|
|
tuple(range(num_bd, -1, -1)),
|
|
tuple(range(num_bd - 1, -1, -1)),
|
|
],
|
|
operand_output_aliases={3: 0},
|
|
)
|
|
|
|
|
|
# # ?geqrf: QR decomposition
|
|
|
|
def geqrf_hlo(dtype, a):
|
|
_initialize()
|
|
a_type = ir.RankedTensorType(a.type)
|
|
dims = a_type.shape
|
|
assert len(dims) >= 2
|
|
m, n = dims[-2:]
|
|
batch_dims = tuple(dims[:-2])
|
|
num_bd = len(batch_dims)
|
|
b = 1
|
|
for d in batch_dims:
|
|
b *= d
|
|
|
|
if dtype == np.float32:
|
|
fn = b"lapack_sgeqrf"
|
|
lwork = _lapack.lapack_sgeqrf_workspace(m, n)
|
|
elif dtype == np.float64:
|
|
fn = b"lapack_dgeqrf"
|
|
lwork = _lapack.lapack_dgeqrf_workspace(m, n)
|
|
elif dtype == np.complex64:
|
|
fn = b"lapack_cgeqrf"
|
|
lwork = _lapack.lapack_cgeqrf_workspace(m, n)
|
|
elif dtype == np.complex128:
|
|
fn = b"lapack_zgeqrf"
|
|
lwork = _lapack.lapack_zgeqrf_workspace(m, n)
|
|
else:
|
|
raise NotImplementedError(f"Unsupported dtype {dtype}")
|
|
|
|
scalar_layout = []
|
|
layout = (num_bd, num_bd + 1) + tuple(range(num_bd - 1, -1, -1))
|
|
i32_type = ir.IntegerType.get_signless(32)
|
|
out = custom_call(
|
|
fn,
|
|
[
|
|
a.type,
|
|
ir.RankedTensorType.get(batch_dims + (min(m, n),), a_type.element_type),
|
|
ir.RankedTensorType.get(batch_dims, i32_type),
|
|
ir.RankedTensorType.get([lwork], a_type.element_type),
|
|
],
|
|
[_hlo_s32(int(b)), _hlo_s32(m), _hlo_s32(n), _hlo_s32(lwork), a],
|
|
operand_layouts=[scalar_layout] * 4 + [layout],
|
|
result_layouts=[
|
|
layout,
|
|
tuple(range(num_bd, -1, -1)),
|
|
tuple(range(num_bd - 1, -1, -1)),
|
|
[0],
|
|
],
|
|
operand_output_aliases={4: 0},
|
|
)
|
|
return out[:3]
|
|
|
|
|
|
# # ?orgqr: product of elementary Householder reflectors:
|
|
|
|
def orgqr_hlo(dtype, a, tau):
|
|
_initialize()
|
|
a_type = ir.RankedTensorType(a.type)
|
|
dims = a_type.shape
|
|
assert len(dims) >= 2
|
|
m, n = dims[-2:]
|
|
batch_dims = tuple(dims[:-2])
|
|
num_bd = len(batch_dims)
|
|
b = 1
|
|
for d in batch_dims:
|
|
b *= d
|
|
|
|
tau_dims = ir.RankedTensorType(tau.type).shape
|
|
assert tau_dims[:-1] == dims[:-2], (tau.type, a.type)
|
|
k = tau_dims[-1]
|
|
|
|
if dtype == np.float32:
|
|
fn = b"lapack_sorgqr"
|
|
lwork = _lapack.lapack_sorgqr_workspace(m, n, k)
|
|
elif dtype == np.float64:
|
|
fn = b"lapack_dorgqr"
|
|
lwork = _lapack.lapack_dorgqr_workspace(m, n, k)
|
|
elif dtype == np.complex64:
|
|
fn = b"lapack_cungqr"
|
|
lwork = _lapack.lapack_cungqr_workspace(m, n, k)
|
|
elif dtype == np.complex128:
|
|
fn = b"lapack_zungqr"
|
|
lwork = _lapack.lapack_zungqr_workspace(m, n, k)
|
|
else:
|
|
raise NotImplementedError(f"Unsupported dtype {dtype}")
|
|
|
|
scalar_layout = []
|
|
layout = (num_bd, num_bd + 1) + tuple(range(num_bd - 1, -1, -1))
|
|
i32_type = ir.IntegerType.get_signless(32)
|
|
out = custom_call(
|
|
fn,
|
|
[
|
|
a.type,
|
|
ir.RankedTensorType.get(batch_dims, i32_type),
|
|
ir.RankedTensorType.get([lwork], a_type.element_type),
|
|
],
|
|
[_hlo_s32(int(b)), _hlo_s32(m), _hlo_s32(n), _hlo_s32(k),
|
|
_hlo_s32(lwork), a, tau],
|
|
operand_layouts=[scalar_layout] * 5 + [
|
|
layout,
|
|
tuple(range(num_bd, -1, -1)),
|
|
],
|
|
result_layouts=[
|
|
layout,
|
|
tuple(range(num_bd - 1, -1, -1)),
|
|
[0],
|
|
],
|
|
operand_output_aliases={5: 0},
|
|
)
|
|
return out[:2]
|
|
|
|
|
|
# ?potrf: Cholesky decomposition
|
|
|
|
def potrf_hlo(dtype, a, lower=False):
|
|
_initialize()
|
|
a_type = ir.RankedTensorType(a.type)
|
|
dims = a_type.shape
|
|
m, n = dims[-2:]
|
|
if m != n:
|
|
raise ValueError(f"potrf expects a square matrix, got {a_type}")
|
|
if dtype == np.float32:
|
|
fn = b"lapack_spotrf"
|
|
elif dtype == np.float64:
|
|
fn = b"lapack_dpotrf"
|
|
elif dtype == np.complex64:
|
|
fn = b"lapack_cpotrf"
|
|
elif dtype == np.complex128:
|
|
fn = b"lapack_zpotrf"
|
|
else:
|
|
raise NotImplementedError(f"Unsupported dtype {dtype}")
|
|
batch_dims = tuple(dims[:-2])
|
|
num_bd = len(batch_dims)
|
|
b = 1
|
|
for d in batch_dims:
|
|
b *= d
|
|
|
|
scalar_layout = []
|
|
layout = (num_bd, num_bd + 1) + tuple(range(num_bd - 1, -1, -1))
|
|
info_layout = tuple(range(num_bd - 1, -1, -1))
|
|
out = custom_call(
|
|
fn,
|
|
[a.type,
|
|
ir.RankedTensorType.get(batch_dims, ir.IntegerType.get_signless(32))],
|
|
[_hlo_s32(int(lower)), _hlo_s32(b), _hlo_s32(n), a],
|
|
operand_layouts=[scalar_layout] * 3 + [layout],
|
|
result_layouts=[layout, info_layout],
|
|
operand_output_aliases={3: 0},
|
|
)
|
|
return out[:2]
|
|
|
|
|
|
|
|
# # ?gesdd: Singular value decomposition
|
|
|
|
def gesdd_hlo(dtype, a, full_matrices=True, compute_uv=True):
|
|
_initialize()
|
|
a_type = ir.RankedTensorType(a.type)
|
|
dims = a_type.shape
|
|
assert len(dims) >= 2
|
|
m, n = dims[-2:]
|
|
batch_dims = tuple(dims[:-2])
|
|
num_bd = len(batch_dims)
|
|
b = 1
|
|
for d in batch_dims:
|
|
b *= d
|
|
|
|
i32_type = ir.IntegerType.get_signless(32)
|
|
if dtype == np.float32:
|
|
fn = b"lapack_sgesdd"
|
|
singular_vals_type = ir.F32Type.get()
|
|
lwork = _lapack.sgesdd_work_size(m, n, compute_uv, full_matrices)
|
|
workspace = [
|
|
ir.RankedTensorType.get([_lapack.gesdd_iwork_size(m, n)], i32_type),
|
|
ir.RankedTensorType.get([lwork], a_type.element_type),
|
|
]
|
|
workspace_layouts = [[0], [0]]
|
|
elif dtype == np.float64:
|
|
fn = b"lapack_dgesdd"
|
|
singular_vals_type = ir.F64Type.get()
|
|
lwork = _lapack.dgesdd_work_size(m, n, compute_uv, full_matrices)
|
|
workspace = [
|
|
ir.RankedTensorType.get([_lapack.gesdd_iwork_size(m, n)], i32_type),
|
|
ir.RankedTensorType.get([lwork], a_type.element_type),
|
|
]
|
|
workspace_layouts = [[0], [0]]
|
|
elif dtype == np.complex64:
|
|
fn = b"lapack_cgesdd"
|
|
singular_vals_type = ir.F32Type.get()
|
|
lwork = _lapack.cgesdd_work_size(m, n, compute_uv, full_matrices)
|
|
workspace = [
|
|
ir.RankedTensorType.get([_lapack.gesdd_iwork_size(m, n)], i32_type),
|
|
ir.RankedTensorType.get(
|
|
[_lapack.cgesdd_rwork_size(m, n, int(compute_uv))],
|
|
ir.F32Type.get()),
|
|
ir.RankedTensorType.get([lwork], a_type.element_type),
|
|
]
|
|
workspace_layouts = [[0], [0], [0]]
|
|
elif dtype == np.complex128:
|
|
fn = b"lapack_zgesdd"
|
|
singular_vals_type = ir.F64Type.get()
|
|
lwork = _lapack.zgesdd_work_size(m, n, compute_uv, full_matrices)
|
|
workspace = [
|
|
ir.RankedTensorType.get([_lapack.gesdd_iwork_size(m, n)], i32_type),
|
|
ir.RankedTensorType.get(
|
|
[_lapack.cgesdd_rwork_size(m, n, int(compute_uv))],
|
|
ir.F64Type.get()),
|
|
ir.RankedTensorType.get([lwork], a_type.element_type),
|
|
]
|
|
workspace_layouts = [[0], [0], [0]]
|
|
else:
|
|
raise NotImplementedError(f"Unsupported dtype {dtype}")
|
|
|
|
scalar_layout = []
|
|
layout = (num_bd, num_bd + 1) + tuple(range(num_bd - 1, -1, -1))
|
|
out = custom_call(
|
|
fn,
|
|
[
|
|
a.type,
|
|
ir.RankedTensorType.get(batch_dims + (min(m, n),), singular_vals_type),
|
|
ir.RankedTensorType.get(
|
|
batch_dims + (m, m if full_matrices else min(m, n)),
|
|
a_type.element_type),
|
|
ir.RankedTensorType.get(
|
|
batch_dims + (n if full_matrices else min(m, n), n),
|
|
a_type.element_type),
|
|
ir.RankedTensorType.get(batch_dims, i32_type),
|
|
] + workspace,
|
|
[_hlo_s32(int(full_matrices)), _hlo_s32(int(compute_uv)), _hlo_s32(b),
|
|
_hlo_s32(m), _hlo_s32(n), _hlo_s32(lwork), a],
|
|
operand_layouts=[scalar_layout] * 6 + [layout],
|
|
result_layouts=[
|
|
layout,
|
|
(num_bd,) + tuple(range(num_bd - 1, -1, -1)),
|
|
layout,
|
|
layout,
|
|
tuple(range(num_bd - 1, -1, -1)),
|
|
] + workspace_layouts,
|
|
operand_output_aliases={6: 0},
|
|
)
|
|
return out[1:5]
|
|
|
|
|
|
# # syevd: Symmetric eigendecomposition
|
|
|
|
def syevd_hlo(dtype, a, lower=False):
|
|
_initialize()
|
|
a_type = ir.RankedTensorType(a.type)
|
|
dims = a_type.shape
|
|
assert len(dims) >= 2
|
|
m, n = dims[-2:]
|
|
assert m == n
|
|
batch_dims = tuple(dims[:-2])
|
|
num_bd = len(batch_dims)
|
|
b = 1
|
|
for d in batch_dims:
|
|
b *= d
|
|
layout = (num_bd, num_bd + 1) + tuple(range(num_bd - 1, -1, -1))
|
|
|
|
i32_type = ir.IntegerType.get_signless(32)
|
|
if dtype == np.float32:
|
|
fn = b"lapack_ssyevd"
|
|
eigvals_type = ir.F32Type.get()
|
|
workspace = [
|
|
ir.RankedTensorType.get([_lapack.syevd_work_size(n)],
|
|
a_type.element_type),
|
|
ir.RankedTensorType.get([_lapack.syevd_iwork_size(n)], i32_type),
|
|
]
|
|
workspace_layouts = [[0], [0]]
|
|
elif dtype == np.float64:
|
|
fn = b"lapack_dsyevd"
|
|
eigvals_type = ir.F64Type.get()
|
|
workspace = [
|
|
ir.RankedTensorType.get([_lapack.syevd_work_size(n)],
|
|
a_type.element_type),
|
|
ir.RankedTensorType.get([_lapack.syevd_iwork_size(n)], i32_type),
|
|
]
|
|
workspace_layouts = [[0], [0]]
|
|
elif dtype == np.complex64:
|
|
fn = b"lapack_cheevd"
|
|
eigvals_type = ir.F32Type.get()
|
|
workspace = [
|
|
ir.RankedTensorType.get([_lapack.heevd_work_size(n)],
|
|
a_type.element_type),
|
|
ir.RankedTensorType.get([_lapack.heevd_rwork_size(n)], eigvals_type),
|
|
ir.RankedTensorType.get([_lapack.syevd_iwork_size(n)], i32_type),
|
|
]
|
|
workspace_layouts = [[0], [0], [0]]
|
|
elif dtype == np.complex128:
|
|
fn = b"lapack_zheevd"
|
|
eigvals_type = ir.F64Type.get()
|
|
workspace = [
|
|
ir.RankedTensorType.get([_lapack.heevd_work_size(n)],
|
|
a_type.element_type),
|
|
ir.RankedTensorType.get([_lapack.heevd_rwork_size(n)], eigvals_type),
|
|
ir.RankedTensorType.get([_lapack.syevd_iwork_size(n)], i32_type),
|
|
]
|
|
workspace_layouts = [[0], [0], [0]]
|
|
else:
|
|
raise NotImplementedError(f"Unsupported dtype {dtype}")
|
|
|
|
scalar_layout = []
|
|
layout = (num_bd, num_bd + 1) + tuple(range(num_bd - 1, -1, -1))
|
|
out = custom_call(
|
|
fn,
|
|
[
|
|
a.type,
|
|
ir.RankedTensorType.get(batch_dims + (n,), eigvals_type),
|
|
ir.RankedTensorType.get(batch_dims, i32_type),
|
|
] + workspace,
|
|
[_hlo_s32(1 if lower else 0), _hlo_s32(b), _hlo_s32(n), a],
|
|
operand_layouts=[scalar_layout] * 3 + [layout],
|
|
result_layouts=[
|
|
layout,
|
|
tuple(range(num_bd, -1, -1)),
|
|
tuple(range(num_bd - 1, -1, -1)),
|
|
] + workspace_layouts,
|
|
operand_output_aliases={3: 0},
|
|
)
|
|
return out[:3]
|
|
|
|
|
|
# # geev: Nonsymmetric eigendecomposition
|
|
|
|
def geev_hlo(dtype, a, jobvl=True, jobvr=True):
|
|
_initialize()
|
|
dims = ir.RankedTensorType(a.type).shape
|
|
assert len(dims) >= 2
|
|
m, n = dims[-2:]
|
|
assert m == n
|
|
batch_dims = tuple(dims[:-2])
|
|
num_bd = len(batch_dims)
|
|
b = 1
|
|
for d in batch_dims:
|
|
b *= d
|
|
layout = (num_bd, num_bd + 1) + tuple(range(num_bd - 1, -1, -1))
|
|
|
|
jobvl_c = ord('V' if jobvl else 'N')
|
|
jobvr_c = ord('V' if jobvr else 'N')
|
|
|
|
if dtype == np.float32:
|
|
fn = b"lapack_sgeev"
|
|
real = True
|
|
eigvecs_type = ir.ComplexType.get(ir.F32Type.get())
|
|
workspaces = [ir.RankedTensorType.get([n, n], ir.F32Type.get()),
|
|
ir.RankedTensorType.get([n, n], ir.F32Type.get()),
|
|
ir.RankedTensorType.get([n, n], ir.F32Type.get())]
|
|
workspace_layouts = [[0, 1]] * 3
|
|
eigvals = [ir.RankedTensorType.get(batch_dims + (n,), ir.F32Type.get()),
|
|
ir.RankedTensorType.get(batch_dims + (n,), ir.F32Type.get())]
|
|
eigvals_layouts = [tuple(range(num_bd, -1, -1))] * 2
|
|
elif dtype == np.float64:
|
|
fn = b"lapack_dgeev"
|
|
real = True
|
|
eigvecs_type = ir.ComplexType.get(ir.F64Type.get())
|
|
workspaces = [ir.RankedTensorType.get([n, n], ir.F64Type.get()),
|
|
ir.RankedTensorType.get([n, n], ir.F64Type.get()),
|
|
ir.RankedTensorType.get([n, n], ir.F64Type.get())]
|
|
workspace_layouts = [[0, 1]] * 3
|
|
eigvals = [ir.RankedTensorType.get(batch_dims + (n,), ir.F64Type.get()),
|
|
ir.RankedTensorType.get(batch_dims + (n,), ir.F64Type.get())]
|
|
eigvals_layouts = [tuple(range(num_bd, -1, -1))] * 2
|
|
elif dtype == np.complex64:
|
|
fn = b"lapack_cgeev"
|
|
real = False
|
|
eigvecs_type = ir.ComplexType.get(ir.F32Type.get())
|
|
workspaces = [ir.RankedTensorType.get([n, n],
|
|
ir.ComplexType.get(ir.F32Type.get())),
|
|
ir.RankedTensorType.get([2 * n], ir.F32Type.get())]
|
|
workspace_layouts = [[0, 1], [0]]
|
|
eigvals = [ir.RankedTensorType.get(batch_dims + (n,),
|
|
ir.ComplexType.get(ir.F32Type.get()))]
|
|
eigvals_layouts = [tuple(range(num_bd, -1, -1))]
|
|
elif dtype == np.complex128:
|
|
fn = b"lapack_zgeev"
|
|
real = False
|
|
eigvecs_type = ir.ComplexType.get(ir.F64Type.get())
|
|
workspaces = [ir.RankedTensorType.get([n, n],
|
|
ir.ComplexType.get(ir.F64Type.get())),
|
|
ir.RankedTensorType.get([2 * n], ir.F64Type.get())]
|
|
workspace_layouts = [[0, 1], [0]]
|
|
eigvals = [ir.RankedTensorType.get(batch_dims + (n,),
|
|
ir.ComplexType.get(ir.F64Type.get()))]
|
|
eigvals_layouts = [tuple(range(num_bd, -1, -1))]
|
|
else:
|
|
raise NotImplementedError(f"Unsupported dtype {dtype}")
|
|
|
|
i32_type = ir.IntegerType.get_signless(32)
|
|
scalar_layout = []
|
|
info_layout = tuple(range(num_bd - 1, -1, -1))
|
|
out = custom_call(
|
|
fn,
|
|
workspaces + eigvals + [
|
|
ir.RankedTensorType.get(dims, eigvecs_type),
|
|
ir.RankedTensorType.get(dims, eigvecs_type),
|
|
ir.RankedTensorType.get(batch_dims, i32_type),
|
|
],
|
|
[_hlo_s32(b), _hlo_s32(n), _hlo_u8(jobvl_c), _hlo_u8(jobvr_c), a],
|
|
operand_layouts=[scalar_layout] * 4 + [layout],
|
|
result_layouts=(workspace_layouts + eigvals_layouts + [layout] * 2 +
|
|
[info_layout])
|
|
)
|
|
if real:
|
|
return (hlo.ComplexOp(out[3], out[4]).result, out[5], out[6], out[7])
|
|
else:
|
|
return out[2:6]
|
|
|
|
# # gees : Schur factorization
|
|
|
|
def gees_hlo(dtype, a, jobvs=True, sort=False, select=None):
|
|
_initialize()
|
|
a_type = ir.RankedTensorType(a.type)
|
|
etype = a_type.element_type
|
|
dims = a_type.shape
|
|
assert len(dims) >= 2
|
|
m, n = dims[-2:]
|
|
assert m == n
|
|
batch_dims = tuple(dims[:-2])
|
|
num_bd = len(batch_dims)
|
|
b = 1
|
|
for d in batch_dims:
|
|
b *= d
|
|
layout = (num_bd, num_bd + 1) + tuple(range(num_bd - 1, -1, -1))
|
|
|
|
if sort:
|
|
raise NotImplementedError(
|
|
"The sort feature of LAPACK's gees routine is not implemented.")
|
|
|
|
jobvs = ord('V' if jobvs else 'N')
|
|
sort = ord('S' if sort else 'N')
|
|
|
|
if dtype == np.float32:
|
|
fn = "lapack_sgees"
|
|
elif dtype == np.float64:
|
|
fn = "lapack_dgees"
|
|
elif dtype == np.complex64:
|
|
fn = "lapack_cgees"
|
|
elif dtype == np.complex128:
|
|
fn = "lapack_zgees"
|
|
else:
|
|
raise NotImplementedError(f"Unsupported dtype {dtype}")
|
|
|
|
if not np.issubdtype(dtype, np.complexfloating):
|
|
workspaces = [ir.RankedTensorType.get(dims, etype)]
|
|
workspace_layouts = [layout]
|
|
eigvals = [ir.RankedTensorType.get(batch_dims + (n,), etype)] * 2
|
|
eigvals_layouts = [tuple(range(num_bd, -1, -1))] * 2
|
|
else:
|
|
workspaces = [
|
|
ir.RankedTensorType.get(dims, etype),
|
|
ir.RankedTensorType.get([n], ir.ComplexType(etype).element_type),
|
|
]
|
|
workspace_layouts = [layout, [0]]
|
|
eigvals = [ir.RankedTensorType.get(batch_dims + (n,), etype)]
|
|
eigvals_layouts = [tuple(range(num_bd, -1, -1))]
|
|
|
|
i32_type = ir.IntegerType.get_signless(32)
|
|
|
|
scalar_layout = []
|
|
out = custom_call(
|
|
fn,
|
|
workspaces + eigvals + [
|
|
ir.RankedTensorType.get(dims, etype),
|
|
ir.RankedTensorType.get(batch_dims, i32_type),
|
|
ir.RankedTensorType.get(batch_dims, i32_type),
|
|
],
|
|
[
|
|
_hlo_s32(b),
|
|
_hlo_s32(n),
|
|
_hlo_u8(np.uint8(jobvs)),
|
|
_hlo_u8(np.uint8(sort)),
|
|
# TODO: figure out how to put the callable select function here
|
|
a
|
|
],
|
|
operand_layouts=[scalar_layout] * 4 + [layout],
|
|
result_layouts=workspace_layouts + eigvals_layouts + [
|
|
layout,
|
|
tuple(range(num_bd - 1, -1, -1)),
|
|
tuple(range(num_bd - 1, -1, -1)),
|
|
],
|
|
operand_output_aliases={4: 0},
|
|
)
|
|
if sort == ord('S'):
|
|
return (out[0], out[3], out[4], out[5])
|
|
else:
|
|
return (out[0], out[3], out[5])
|
|
|
|
|
|
# gehrd: Reduction of a non-symmetric square matrix to upper Hessenberg form.
|
|
def gehrd_hlo(dtype, a):
|
|
_initialize()
|
|
a_type = ir.RankedTensorType(a.type)
|
|
dims = a_type.shape
|
|
assert len(dims) >= 2
|
|
m, n = dims[-2:]
|
|
assert m == n, (m, n)
|
|
batch_dims = tuple(dims[:-2])
|
|
num_bd = len(batch_dims)
|
|
b = 1
|
|
for d in batch_dims:
|
|
b *= d
|
|
|
|
if dtype == np.float32:
|
|
fn = b"lapack_sgehrd"
|
|
lwork = _lapack.lapack_sgehrd_workspace(n, n, 1, n)
|
|
elif dtype == np.float64:
|
|
fn = b"lapack_dgehrd"
|
|
lwork = _lapack.lapack_dgehrd_workspace(n, n, 1, n)
|
|
elif dtype == np.complex64:
|
|
fn = b"lapack_cgehrd"
|
|
lwork = _lapack.lapack_cgehrd_workspace(n, n, 1, n)
|
|
elif dtype == np.complex128:
|
|
fn = b"lapack_zgehrd"
|
|
lwork = _lapack.lapack_zgehrd_workspace(n, n, 1, n)
|
|
else:
|
|
raise NotImplementedError(f"Unsupported dtype {dtype}")
|
|
|
|
layout = (num_bd, num_bd + 1) + tuple(range(num_bd - 1, -1, -1))
|
|
i32_type = ir.IntegerType.get_signless(32)
|
|
out = custom_call(
|
|
fn,
|
|
[
|
|
a.type,
|
|
ir.RankedTensorType.get(batch_dims + (n - 1,), a_type.element_type),
|
|
ir.RankedTensorType.get(batch_dims, i32_type),
|
|
ir.RankedTensorType.get([lwork], a_type.element_type),
|
|
],
|
|
[_hlo_s32(n), _hlo_s32(1), _hlo_s32(n), _hlo_s32(n), _hlo_s32(b),
|
|
_hlo_s32(lwork), a],
|
|
operand_layouts=[[]] * 6 + [layout],
|
|
result_layouts=[
|
|
layout,
|
|
(num_bd,) + tuple(range(num_bd - 1, -1, -1)),
|
|
tuple(range(num_bd - 1, -1, -1)),
|
|
[0],
|
|
],
|
|
operand_output_aliases={6: 0},
|
|
)
|
|
return out[:3]
|
|
|
|
|
|
# sytrd: Reduction of a symmetric (Hermitian) matrix to tridiagonal form.
|
|
def sytrd_hlo(dtype, a, *, lower):
|
|
_initialize()
|
|
a_type = ir.RankedTensorType(a.type)
|
|
dims = a_type.shape
|
|
assert len(dims) >= 2
|
|
m, n = dims[-2:]
|
|
assert m == n, (m, n)
|
|
batch_dims = tuple(dims[:-2])
|
|
num_bd = len(batch_dims)
|
|
b = 1
|
|
for d in batch_dims:
|
|
b *= d
|
|
|
|
if dtype == np.float32:
|
|
fn = b"lapack_ssytrd"
|
|
lwork = _lapack.lapack_ssytrd_workspace(n, n)
|
|
diag_type = a_type.element_type
|
|
elif dtype == np.float64:
|
|
fn = b"lapack_dsytrd"
|
|
lwork = _lapack.lapack_dsytrd_workspace(n, n)
|
|
diag_type = a_type.element_type
|
|
elif dtype == np.complex64:
|
|
fn = b"lapack_chetrd"
|
|
lwork = _lapack.lapack_chetrd_workspace(n, n)
|
|
diag_type = ir.F32Type.get()
|
|
elif dtype == np.complex128:
|
|
fn = b"lapack_zhetrd"
|
|
lwork = _lapack.lapack_zhetrd_workspace(n, n)
|
|
diag_type = ir.F64Type.get()
|
|
else:
|
|
raise NotImplementedError(f"Unsupported dtype {dtype}")
|
|
|
|
layout = (num_bd, num_bd + 1) + tuple(range(num_bd - 1, -1, -1))
|
|
i32_type = ir.IntegerType.get_signless(32)
|
|
out = custom_call(
|
|
fn,
|
|
[
|
|
a.type,
|
|
ir.RankedTensorType.get(batch_dims + (n,), diag_type),
|
|
ir.RankedTensorType.get(batch_dims + (n - 1,), diag_type),
|
|
ir.RankedTensorType.get(batch_dims + (n - 1,), a_type.element_type),
|
|
ir.RankedTensorType.get(batch_dims, i32_type),
|
|
ir.RankedTensorType.get([lwork], a_type.element_type),
|
|
],
|
|
[_hlo_s32(n), _hlo_s32(1 if lower else 0), _hlo_s32(max(1, n)),
|
|
_hlo_s32(b), _hlo_s32(lwork), a],
|
|
operand_layouts=[[]] * 5 + [layout],
|
|
result_layouts=[
|
|
layout,
|
|
(num_bd,) + tuple(range(num_bd - 1, -1, -1)),
|
|
(num_bd,) + tuple(range(num_bd - 1, -1, -1)),
|
|
(num_bd,) + tuple(range(num_bd - 1, -1, -1)),
|
|
tuple(range(num_bd - 1, -1, -1)),
|
|
[0],
|
|
],
|
|
operand_output_aliases={5: 0},
|
|
)
|
|
return out[:5]
|