mirror of
https://github.com/ROCm/jax.git
synced 2025-04-16 03:46:06 +00:00
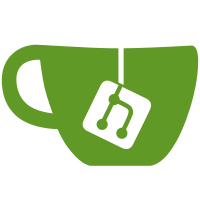
-- 1ecf4f02891cad70cc8f094b49cf2458105ca366 by George Necula <gcnecula@gmail.com>: [jax2tf] Change the conversion of dot_general to use XLA op. Instead of converting the dot_general to a sea of TF ops, when we enable_xla we just use the XLA op. This has the advantage that it also supports the preferred_element_type. Fixed bug with passing the precision parameter to TF. Also improved tests to print the HLO in case of numerical errors. COPYBARA_INTEGRATE_REVIEW=https://github.com/google/jax/pull/6717 from gnecula:tf_dot 1ecf4f02891cad70cc8f094b49cf2458105ca366 PiperOrigin-RevId: 373326655
1048 lines
38 KiB
Python
1048 lines
38 KiB
Python
# Copyright 2020 Google LLC
|
|
#
|
|
# Licensed under the Apache License, Version 2.0 (the "License");
|
|
# you may not use this file except in compliance with the License.
|
|
# You may obtain a copy of the License at
|
|
#
|
|
# https://www.apache.org/licenses/LICENSE-2.0
|
|
#
|
|
# Unless required by applicable law or agreed to in writing, software
|
|
# distributed under the License is distributed on an "AS IS" BASIS,
|
|
# WITHOUT WARRANTIES OR CONDITIONS OF ANY KIND, either express or implied.
|
|
# See the License for the specific language governing permissions and
|
|
# limitations under the License.
|
|
"""Tests for the shape-polymorphic jax2tf conversion."""
|
|
|
|
from absl.testing import absltest
|
|
from typing import Callable, Dict, List, Optional, Sequence, Union
|
|
|
|
import collections
|
|
import functools
|
|
from functools import partial
|
|
import operator
|
|
import re
|
|
|
|
import jax
|
|
from jax import core
|
|
from jax.experimental import jax2tf
|
|
from jax.experimental.jax2tf import shape_poly
|
|
from jax import lax
|
|
import jax.numpy as jnp
|
|
from jax import test_util as jtu
|
|
from jax._src.lax import control_flow as lax_control_flow
|
|
import numpy as np
|
|
|
|
from jax.experimental.jax2tf.tests import tf_test_util
|
|
|
|
import tensorflow as tf # type: ignore[import]
|
|
import unittest
|
|
|
|
from jax.config import config
|
|
|
|
config.parse_flags_with_absl()
|
|
|
|
# Import after parsing flags
|
|
from jax.experimental.jax2tf.tests import primitive_harness
|
|
from jax.experimental.jax2tf.tests.primitive_harness import Harness, CustomArg, RandArg, StaticArg
|
|
from jax.experimental.jax2tf.tests.jax2tf_limitations import Jax2TfLimitation
|
|
|
|
PS = jax2tf.PolyShape
|
|
|
|
|
|
class ShapePolyTest(tf_test_util.JaxToTfTestCase):
|
|
|
|
def test_simple(self):
|
|
"""Test shape polymorphism for a simple case."""
|
|
|
|
def f_jax(x):
|
|
return x + jnp.sin(x)
|
|
|
|
self.CheckShapePolymorphism(
|
|
f_jax,
|
|
input_signature=[tf.TensorSpec([2, 3])],
|
|
polymorphic_shapes=None,
|
|
expected_output_signature=tf.TensorSpec([2, 3]))
|
|
|
|
self.CheckShapePolymorphism(
|
|
f_jax,
|
|
input_signature=[tf.TensorSpec([2, None])],
|
|
polymorphic_shapes=["_, h"],
|
|
expected_output_signature=tf.TensorSpec([2, None]))
|
|
|
|
self.CheckShapePolymorphism(
|
|
f_jax,
|
|
input_signature=[tf.TensorSpec([None, None])],
|
|
polymorphic_shapes=["h, h"],
|
|
expected_output_signature=tf.TensorSpec([None, None]))
|
|
|
|
def test_arg_avals(self):
|
|
"""Test conversion of actual arguments to abstract values."""
|
|
|
|
def check_avals(*, args: Sequence[jax2tf.jax2tf.TfVal],
|
|
polymorphic_shapes: Sequence[Optional[Union[str, PS]]],
|
|
expected_avals: Sequence[core.ShapedArray]):
|
|
avals, shape_env = jax2tf.jax2tf._args_to_avals_and_env(
|
|
args, polymorphic_shapes) # The function under test
|
|
self.assertEqual(expected_avals, avals)
|
|
# TODO: Check the shape_env
|
|
|
|
def shaped_array(shape_spec: str, actual_shape: core.Shape):
|
|
return core.ShapedArray(
|
|
shape_poly.parse_spec(shape_spec, actual_shape), np.float32)
|
|
|
|
def const(shape):
|
|
return np.ones(shape, dtype=np.float32)
|
|
|
|
def tf_const(shape):
|
|
return tf.convert_to_tensor(np.ones(shape, dtype=np.float32))
|
|
|
|
def tf_var(shape, *, initializer_shape=None):
|
|
initializer_shape = initializer_shape or shape
|
|
self.assertEmpty([d for d in initializer_shape if d is None])
|
|
return tf.Variable(
|
|
np.ones(initializer_shape, np.float32), dtype=tf.float32, shape=shape)
|
|
|
|
# Known shapes for the arguments
|
|
check_avals(
|
|
args=[const((2, 3))],
|
|
polymorphic_shapes=[None],
|
|
expected_avals=(shaped_array("2, 3", [2, 3]),))
|
|
|
|
check_avals(
|
|
args=[tf_const((2, 3))],
|
|
polymorphic_shapes=[None],
|
|
expected_avals=(shaped_array("2, 3,", [2, 3]),))
|
|
|
|
check_avals(
|
|
args=[tf_var((2, 3))],
|
|
polymorphic_shapes=[None],
|
|
expected_avals=(shaped_array("(2, 3)", [2, 3]),))
|
|
|
|
check_avals(
|
|
args=[const((2, 3))],
|
|
polymorphic_shapes=["(2, 3)"],
|
|
expected_avals=(shaped_array("2, 3", [2, 3]),))
|
|
|
|
check_avals(
|
|
args=[tf_const((2, 3))],
|
|
polymorphic_shapes=["(_, 3)"],
|
|
expected_avals=(shaped_array("2, 3", [2, 3]),))
|
|
|
|
check_avals(
|
|
args=[tf_const((2, 3))],
|
|
polymorphic_shapes=[PS("_", 3)],
|
|
expected_avals=(shaped_array("2, 3", [2, 3]),))
|
|
|
|
# Partially known shapes for the arguments
|
|
check_avals(
|
|
args=[tf_var([None, 3], initializer_shape=(2, 3))],
|
|
polymorphic_shapes=[PS("b", ...)],
|
|
expected_avals=(shaped_array("(b, 3)", (2, 3)),))
|
|
|
|
check_avals(
|
|
args=[tf_var([None, None], initializer_shape=(2, 3))],
|
|
polymorphic_shapes=["h, h"],
|
|
expected_avals=(shaped_array("(h, h)", (2, 2)),))
|
|
|
|
check_avals(
|
|
args=[tf_var([2, None], initializer_shape=(2, 3))],
|
|
polymorphic_shapes=[("h, h")],
|
|
expected_avals=(shaped_array("(h, h)", (2, 2)),))
|
|
|
|
check_avals(
|
|
args=[tf_var([None, 3, 4], initializer_shape=(2, 3, 4))],
|
|
polymorphic_shapes=["(c, b, a)"],
|
|
expected_avals=(shaped_array("(c, b, a)", (2, 3, 4)),),
|
|
)
|
|
|
|
# Some errors
|
|
with self.assertRaisesRegex(ValueError,
|
|
re.escape("PolyShape ')(' has invalid syntax")):
|
|
check_avals(
|
|
args=[const((2, 3))], polymorphic_shapes=[")("], expected_avals=None)
|
|
|
|
with self.assertRaisesRegex(
|
|
ValueError,
|
|
re.escape("PolyShape '..., 3' can contain Ellipsis only at the end.")):
|
|
check_avals(
|
|
args=[const((2, 3))],
|
|
polymorphic_shapes=["..., 3"],
|
|
expected_avals=None)
|
|
|
|
with self.assertRaisesRegex(
|
|
ValueError,
|
|
re.escape(
|
|
"PolyShape '2, 3, 4, ...' must match the rank of arguments (2, 3).")
|
|
):
|
|
check_avals(
|
|
args=[const((2, 3))],
|
|
polymorphic_shapes=["2, 3, 4, ..."],
|
|
expected_avals=None)
|
|
|
|
with self.assertRaisesRegex(
|
|
ValueError,
|
|
re.escape(
|
|
"PolyShape '(Ellipsis, 3)' can contain Ellipsis only at the end.")):
|
|
check_avals(
|
|
args=[const((2, 3))],
|
|
polymorphic_shapes=[PS(..., 3)],
|
|
expected_avals=None)
|
|
|
|
with self.assertRaisesRegex(
|
|
ValueError,
|
|
re.escape(
|
|
"PolyShape 'None' in axis 1 must contain a shape variable for unknown dimension in argument shape (2, None)"
|
|
)):
|
|
check_avals(
|
|
args=[tf_var([2, None], initializer_shape=(2, 3))],
|
|
polymorphic_shapes=[None],
|
|
expected_avals=None)
|
|
|
|
with self.assertRaisesRegex(
|
|
ValueError,
|
|
re.escape("PolyShape '()' must match the rank of arguments (2, 3)")):
|
|
check_avals(
|
|
args=[const((2, 3))], polymorphic_shapes=["()"], expected_avals=None)
|
|
|
|
with self.assertRaisesRegex(
|
|
ValueError,
|
|
re.escape(
|
|
"PolyShape '(_, _)' in axis 1 must contain a shape variable "
|
|
"for unknown dimension in argument shape (2, None)"
|
|
)):
|
|
check_avals(
|
|
args=[tf_var([2, None], initializer_shape=(2, 3))],
|
|
polymorphic_shapes=["(_, _)"],
|
|
expected_avals=None)
|
|
|
|
with self.assertRaisesRegex(
|
|
ValueError,
|
|
re.escape(
|
|
"PolyShape '(2, 13)' in axis 1 must contain a constant or '_' for "
|
|
"known dimension in argument shape (2, 3)"
|
|
)):
|
|
check_avals(
|
|
args=[const((2, 3))],
|
|
polymorphic_shapes=["(2, 13)"],
|
|
expected_avals=None)
|
|
|
|
with self.assertRaisesRegex(
|
|
ValueError,
|
|
re.escape(
|
|
"PolyShape '(2, 3)' in axis 1 must contain a shape variable for "
|
|
"unknown dimension in argument shape (2, None)"
|
|
)):
|
|
check_avals(
|
|
args=[tf_var([2, None], initializer_shape=(2, 3))],
|
|
polymorphic_shapes=["(2, 3)"],
|
|
expected_avals=None)
|
|
|
|
with self.assertRaisesRegex(
|
|
ValueError,
|
|
re.escape(
|
|
"PolyShape '(a, a)' has dimension variable 'a' corresponding to "
|
|
"multiple values ([2, 3]), for argument shape (2, 3)"
|
|
)):
|
|
check_avals(
|
|
args=[tf_var([2, 3], initializer_shape=(2, 3))],
|
|
polymorphic_shapes=["(a, a)"],
|
|
expected_avals=None)
|
|
|
|
def test_pytree(self):
|
|
"""Arguments and polymorphic_shapes are pytrees."""
|
|
|
|
# Arguments are of the form [([x00, x01], [x10]), dict(a=ya, b=yb)]
|
|
def add_all_jax(x_pair_of_list, y_dict):
|
|
x_list_0, x_list_1 = x_pair_of_list
|
|
return functools.reduce(operator.add,
|
|
x_list_0 + x_list_1 + [y_dict["a"], y_dict["b"]])
|
|
|
|
self.CheckShapePolymorphism(
|
|
add_all_jax,
|
|
input_signature=[([tf.TensorSpec([None]),
|
|
tf.TensorSpec([None])], [tf.TensorSpec([None])]),
|
|
dict(a=tf.TensorSpec([None]),
|
|
b=tf.TensorSpec([None]))],
|
|
polymorphic_shapes=[(["v", "v"], [("v")]),
|
|
dict(a="v", b="v")],
|
|
expected_output_signature=tf.TensorSpec([None]))
|
|
|
|
# Now partial polymorphic_shapes; the parts of the polymorphic_shapes that
|
|
# are not specified must have full input_signatures.
|
|
self.CheckShapePolymorphism(
|
|
add_all_jax,
|
|
input_signature=[([tf.TensorSpec([4]),
|
|
tf.TensorSpec([4])], [tf.TensorSpec([4])]),
|
|
dict(a=tf.TensorSpec([4]), b=tf.TensorSpec([4]))],
|
|
polymorphic_shapes=[(["(4,)", "(_,)"], [("4,")]),
|
|
dict(a="(_,)", b="(4,)")],
|
|
expected_output_signature=tf.TensorSpec([4]))
|
|
|
|
def test_with_custom_vjp(self):
|
|
"""Shape-polymorphic custom VJP."""
|
|
|
|
@jax.custom_vjp
|
|
def f(x):
|
|
# x: [b1, b2, d1, d2] (a batch of matrices)
|
|
# res: [b1, b2, d1, d1]
|
|
return jnp.matmul(x, jnp.transpose(x, axes=(0, 1, 3, 2)))
|
|
|
|
# f_fwd: a -> (b, residual)
|
|
def f_fwd(x):
|
|
# x: [b1, b2, d1, d2]
|
|
# b: [b1, b2, d1, d1]
|
|
# residual: [b1, b2, d1, d2]
|
|
return f(x), 3. * x
|
|
|
|
# f_bwd: (residual, CT b) -> [CT a]
|
|
def f_bwd(residual, ct_b):
|
|
# residual: [b1, b2, d1, d2]
|
|
# ct_b: [b1, b2, d1, d1]
|
|
# ct_a: [b1, b2, d1, d2]
|
|
return jnp.matmul(ct_b, residual),
|
|
|
|
f.defvjp(f_fwd, f_bwd)
|
|
x = np.ones((2, 3, 4, 5), dtype=np.float32)
|
|
res_jax = f(x)
|
|
res_jax_grad = jax.grad(lambda x: jnp.sum(f(x)))(x)
|
|
|
|
f_tf = self.CheckShapePolymorphism(
|
|
f,
|
|
input_signature=[tf.TensorSpec([None, None, None, None])],
|
|
polymorphic_shapes=["(batch1, batch2, d1, d2)"],
|
|
expected_output_signature=tf.TensorSpec([None, None, None, None]))
|
|
|
|
self.assertAllClose(res_jax, f_tf(x))
|
|
|
|
xv = tf.Variable(x, dtype=np.float32)
|
|
|
|
def tf_value_and_grad(xv):
|
|
with tf.GradientTape() as tape:
|
|
tape.watch(xv)
|
|
res_tf = f_tf(xv)
|
|
res_tf_grad = tape.gradient(res_tf, xv)
|
|
return res_tf, res_tf_grad
|
|
|
|
res_tf, res_tf_grad = tf_value_and_grad(xv)
|
|
self.assertAllClose(res_jax, res_tf)
|
|
self.assertAllClose(res_jax_grad, res_tf_grad)
|
|
|
|
# Now use TF tracing for the gradient
|
|
tf_grad = tf.function(
|
|
tf_value_and_grad, autograph=False).get_concrete_function(
|
|
tf.TensorSpec([None, None, 8, 9]))
|
|
|
|
# The shape of the value. This should be (None, None, 8, 8) but the
|
|
# shape inference for XlaDot is broken, and returns too many unknown
|
|
# dimensions.
|
|
self.assertEqual((None, None, None, None), tuple(tf_grad.output_shapes[0]))
|
|
self.assertEqual((None, None, None, None), tuple(tf_grad.output_shapes[1]))
|
|
|
|
def test_gradients_pytree(self):
|
|
"""Shape polymorphism with gradients and pytrees for inputs and outputs."""
|
|
|
|
def f(x):
|
|
# x: dict(x=[b, 3, 4])
|
|
# res: dict(res=[b, 3, 4])
|
|
return dict(res=x["x"] * 2.)
|
|
|
|
f_tf = self.CheckShapePolymorphism(
|
|
f,
|
|
input_signature=[dict(x=tf.TensorSpec([None, 3, 4]))],
|
|
polymorphic_shapes=[dict(x=("b, 3, 4"))],
|
|
expected_output_signature=None)
|
|
|
|
x = dict(x=np.ones((2, 3, 4), dtype=np.float32))
|
|
xv = tf.Variable(x["x"], dtype=np.float32)
|
|
|
|
def tf_value_and_grad(xv):
|
|
# xv: [b, 3, 4]
|
|
# res_value: dict(res=[b, 3, 4])
|
|
# res_grad: dict(grad=[b, 3, 4])
|
|
with tf.GradientTape() as tape:
|
|
tape.watch(xv)
|
|
res_tf = f_tf(dict(x=xv))
|
|
res_tf_grad = tape.gradient(res_tf, xv)
|
|
return res_tf, dict(grad=res_tf_grad)
|
|
|
|
res_tf, res_tf_grad = tf_value_and_grad(xv)
|
|
# Now use TF tracing for the gradient
|
|
tf_grad = tf.function(
|
|
tf_value_and_grad,
|
|
autograph=False).get_concrete_function(tf.TensorSpec([None, 3, 4]))
|
|
# The shape of the value
|
|
self.assertEqual((None, 3, 4), tuple(tf_grad.output_shapes[0]["res"]))
|
|
# The shape of the gradient should match the input
|
|
self.assertEqual((None, 3, 4), tuple(tf_grad.output_shapes[1]["grad"]))
|
|
|
|
def test_cond(self):
|
|
# Test the primitive under conditional
|
|
def f(x, y):
|
|
# x: f32[B, H], y : f32[H]
|
|
return lax.cond(
|
|
jnp.sum(x) > 0.,
|
|
lambda _: x + y,
|
|
lambda _: jnp.zeros_like(x),
|
|
operand=None)
|
|
|
|
x = np.ones((2, 3))
|
|
y = np.ones((3,))
|
|
res_jax = f(x, y)
|
|
self.assertAllClose(
|
|
res_jax,
|
|
jax2tf.convert(f, polymorphic_shapes=["(b, h)", "h"])(x, y))
|
|
|
|
def test_example(self):
|
|
"""Some of the examples from the README."""
|
|
def image_mask_jax(images, mask):
|
|
# images: f32[B, W, W] and mask: f32[W, W]
|
|
return images * mask
|
|
|
|
print(jax.make_jaxpr(image_mask_jax)(np.ones((1024, 28, 28)), np.ones((28, 28))))
|
|
|
|
# will invoke broadcast_in_dim with shape=(1, w, w)
|
|
jax2tf.convert(image_mask_jax, polymorphic_shapes=["(b, w, w)", "(w, w)"])
|
|
|
|
def test_shape_error(self):
|
|
"""Some of the examples from the README."""
|
|
with self.assertRaisesRegex(
|
|
TypeError,
|
|
re.escape("add got incompatible shapes for broadcasting: (v,), (4,)")):
|
|
self.CheckShapePolymorphism(
|
|
lambda x, y: x + y,
|
|
input_signature=[tf.TensorSpec([None]),
|
|
tf.TensorSpec([4])],
|
|
polymorphic_shapes=["(v,)", "(4,)"],
|
|
expected_output_signature=tf.TensorSpec([None]))
|
|
|
|
four_ones = np.ones((4,))
|
|
# We get the error even if we use correct actual arguments
|
|
with self.assertRaisesRegex(
|
|
TypeError,
|
|
re.escape("add got incompatible shapes for broadcasting: (v,), (4,)")):
|
|
jax2tf.convert(
|
|
lambda x, y: x + y, polymorphic_shapes=["(v,)", "(4,)"])(four_ones,
|
|
four_ones)
|
|
|
|
with self.assertRaisesRegex(core.InconclusiveDimensionOperation,
|
|
re.escape("Shape variable comparison v == 4 is inconclusive")):
|
|
jax2tf.convert(lambda x: jnp.matmul(x, x),
|
|
polymorphic_shapes=["(v, 4)"])(np.ones((4, 4)))
|
|
|
|
with self.assertRaisesRegex(TypeError,
|
|
re.escape("unsupported operand type(s) for *: 'DimVar' and 'int'")):
|
|
jax2tf.convert(lambda x: jnp.reshape(x, np.prod(x.shape)),
|
|
polymorphic_shapes=["(b, ...)"])(np.ones((3, 4, 5)))
|
|
|
|
jax2tf.convert(lambda x: jnp.reshape(x, (x.shape[0], np.prod(x.shape[1:]))),
|
|
polymorphic_shapes=["(b, _, _)"])(np.ones((3, 4, 5)))
|
|
|
|
with self.assertRaisesRegex(
|
|
TypeError,
|
|
re.escape("unsupported operand type(s) for /: 'TensorFlowTracer' and 'DimVar'")):
|
|
jax2tf.convert(lambda x: jnp.sum(x, axis=0) / x.shape[0],
|
|
polymorphic_shapes=["(v, _)"])(np.ones((4, 4)))
|
|
|
|
def test_parse_poly_spec(self):
|
|
self.assertEqual((2, 3), shape_poly.parse_spec(None, (2, 3)))
|
|
self.assertEqual((2, 3), shape_poly.parse_spec("2, 3", (2, 3)))
|
|
self.assertEqual((2, 3), shape_poly.parse_spec("2, _", (2, 3)))
|
|
self.assertEqual((2, 3), shape_poly.parse_spec("2, ...", (2, 3)))
|
|
self.assertEqual((2, 3), shape_poly.parse_spec("...", (2, 3)))
|
|
self.assertEqual((2, 3), shape_poly.parse_spec(" ( 2 , 3 ) ", (2, 3)))
|
|
|
|
def test_dim_vars(self):
|
|
"""Unit tests for DimVar."""
|
|
da, db = shape_poly.parse_spec("a, b", (2, 3))
|
|
self.assertEqual(True, da == da)
|
|
self.assertEqual(False, da != da)
|
|
with self.assertRaisesRegex(core.InconclusiveDimensionOperation, ""):
|
|
da == db
|
|
with self.assertRaisesRegex(core.InconclusiveDimensionOperation, ""):
|
|
da != db
|
|
|
|
self.assertLen({da, da}, 1)
|
|
self.assertLen({da, db}, 2)
|
|
self.assertIn(da, {da, db})
|
|
self.assertIn(db, {da, db})
|
|
self.assertIn(da, [da, db])
|
|
with self.assertRaisesRegex(core.InconclusiveDimensionOperation, ""):
|
|
db in [da, db]
|
|
|
|
def test_dim_vars_symbolic_equal(self):
|
|
da, db = shape_poly.parse_spec("a, b", (2, 3))
|
|
self.assertTrue(core.symbolic_equal_dim(da, da))
|
|
self.assertFalse(core.symbolic_equal_dim(da, 1))
|
|
self.assertFalse(core.symbolic_equal_dim(da, db))
|
|
|
|
self.assertTrue(core.symbolic_equal_one_of_dim(da, [2, da]))
|
|
self.assertFalse(core.symbolic_equal_one_of_dim(da, [2, db]))
|
|
self.assertFalse(core.symbolic_equal_one_of_dim(da, []))
|
|
|
|
self.assertTrue(core.symbolic_equal_one_of_dim(2, [da, 3, 2]))
|
|
self.assertFalse(core.symbolic_equal_one_of_dim(1, [2, db]))
|
|
self.assertFalse(core.symbolic_equal_one_of_dim(3, []))
|
|
|
|
self.assertTrue(core.symbolic_equal_dim(1, jnp.add(0, 1))) # A DeviceArray
|
|
with self.assertRaisesRegex(TypeError,
|
|
re.escape("Shapes must be 1D sequences of concrete values of integer type, got (1, 'a').")):
|
|
self.assertTrue(core.symbolic_equal_dim(1, "a"))
|
|
|
|
def test_dim_vars_greater_equal(self):
|
|
da, db = shape_poly.parse_spec("a, b", (2, 3))
|
|
self.assertTrue(core.greater_equal_dim(da, da))
|
|
self.assertTrue(core.greater_equal_dim(da, 0))
|
|
self.assertTrue(core.greater_equal_dim(da, 1))
|
|
|
|
self.assertTrue(core.greater_equal_shape((da, 2), (1, 1)))
|
|
|
|
with self.assertRaisesRegex(core.InconclusiveDimensionOperation,
|
|
"Shape variable comparison .* is inconclusive"):
|
|
core.greater_equal_dim(da, 2)
|
|
|
|
with self.assertRaisesRegex(core.InconclusiveDimensionOperation,
|
|
"Shape variable comparison .* is inconclusive"):
|
|
core.greater_equal_dim(da, db)
|
|
|
|
def test_dilate_shape(self):
|
|
da, = shape_poly.parse_spec("a,", (2,))
|
|
|
|
self.assertEqual((4, 7), core.dilate_shape((2, 3), (3, 3)))
|
|
self.assertEqual((0, 7), core.dilate_shape((0, 3), (3, 3)))
|
|
self.assertEqual((da, 7), core.dilate_shape((da, 3), (1, 3)))
|
|
|
|
with self.assertRaisesRegex(core.InconclusiveDimensionOperation,
|
|
re.escape("Only dilation == 1 is supported for shape variables (var = a, dilation = 2)")):
|
|
core.dilate_shape((da, 3), (2, 3))
|
|
|
|
def test_stride_shape(self):
|
|
da, = shape_poly.parse_spec("a,", (2,))
|
|
|
|
self.assertEqual((8, 9), core.stride_shape((10, 20), (3, 3), (1, 2)))
|
|
self.assertEqual((da, 9), core.stride_shape((da, 20), (1, 3), (1, 2)))
|
|
|
|
with self.assertRaisesRegex(core.InconclusiveDimensionOperation,
|
|
re.escape("Only striding with window_size == window_stride == 1 is supported for shape variables (var = a, window_size = 2, stride = 1")):
|
|
core.stride_shape((da, 20), (2, 3), (1, 2))
|
|
|
|
with self.assertRaisesRegex(core.InconclusiveDimensionOperation,
|
|
re.escape("Only striding with window_size == window_stride == 1 is supported for shape variables (var = a, window_size = 1, stride = 2")):
|
|
core.stride_shape((da, 20), (1, 3), (2, 2))
|
|
|
|
|
|
class ShapeAsValueTest(tf_test_util.JaxToTfTestCase):
|
|
|
|
def setUp(self):
|
|
raise unittest.SkipTest("shape_as_value not supported anymore. See #6080.")
|
|
|
|
def test_concrete_shapes(self):
|
|
# Test shape_as_value with concrete shapes. All transformations work.
|
|
def f(x):
|
|
return jnp.sum(x, axis=0) * jax2tf.shape_as_value(x)[0]
|
|
|
|
x = np.arange(3.)
|
|
self.assertAllClose(9., f(x))
|
|
self.assertAllClose(9., jax.jit(f)(x))
|
|
|
|
res_primal, res_tangent = jax.jvp(f, (x,), (np.array([0.1, 0.2, 0.3]),))
|
|
self.assertAllClose((9., 1.8), (res_primal, res_tangent))
|
|
|
|
self.assertAllClose(np.array([3., 3., 3.]), jax.grad(f)(x))
|
|
|
|
xv = np.arange(24.).reshape((2, 3, 4))
|
|
res_vmap = jax.vmap(f, in_axes=1)(xv)
|
|
# Implement by iteration
|
|
res_iter = jnp.stack([f(xv[:, i, :]) for i in range(xv.shape[1])])
|
|
self.assertAllClose(res_iter, res_vmap)
|
|
|
|
res_mask2, _ = jax.mask(f, polymorphic_shapes=["(b,)"])([x], dict(b=2))
|
|
self.assertAllClose(2., res_mask2)
|
|
res_mask3, _ = jax.mask(f, polymorphic_shapes=["(b,)"])([x], dict(b=3))
|
|
self.assertAllClose(9., res_mask3)
|
|
|
|
def test_dynamic_shapes(self):
|
|
# Test shape_as_value with dynamic shapes. All transformations work.
|
|
def f(x):
|
|
return jnp.sum(x, axis=0) * jax2tf.shape_as_value(x)[0]
|
|
|
|
x = np.arange(3.)
|
|
self.assertAllClose(9., jax2tf.convert(f, polymorphic_shapes=["(b,)"])(x))
|
|
self.assertAllClose(
|
|
9.,
|
|
jax2tf.convert(jax.jit(f), polymorphic_shapes=["(b,)"])(x))
|
|
self.assertAllClose(
|
|
9.,
|
|
tf.function(jax2tf.convert(f, polymorphic_shapes=["(b,)"]))(x))
|
|
|
|
res_primal, res_tangent = jax2tf.convert(
|
|
lambda x, xt: jax.jvp(f, (x,), (xt,)),
|
|
polymorphic_shapes=["b", "b"])(x, np.array([0.1, 0.2, 0.3]))
|
|
self.assertAllClose((9., 1.8), (res_primal, res_tangent))
|
|
|
|
self.assertAllClose(
|
|
np.array([3., 3., 3.]),
|
|
jax2tf.convert(jax.grad(f), polymorphic_shapes=["b"])(x))
|
|
|
|
xv = np.arange(24.).reshape((2, 3, 4))
|
|
res_vmap = jax.vmap(f, in_axes=1)(xv)
|
|
# Implement by iteration
|
|
res_iter = jnp.stack([f(xv[:, i, :]) for i in range(xv.shape[1])])
|
|
self.assertAllClose(res_iter, res_vmap)
|
|
|
|
res_mask2, _ = jax.mask(f, polymorphic_shapes=["(b,)"])([x], dict(b=2))
|
|
self.assertAllClose(2., res_mask2)
|
|
res_mask3, _ = jax.mask(f, polymorphic_shapes=["(b,)"])([x], dict(b=3))
|
|
self.assertAllClose(9., res_mask3)
|
|
|
|
def test_cond(self):
|
|
# Test the primitive under conditional
|
|
def f(x):
|
|
return lax.cond(
|
|
jnp.sum(x) > 0.,
|
|
lambda _: jnp.sum(x) / functools.reduce(lax.mul,
|
|
jax2tf.shape_as_value(x)),
|
|
lambda _: 0.,
|
|
operand=None)
|
|
|
|
x = np.ones((2, 3, 4))
|
|
self.assertAllClose(1., f(x))
|
|
self.assertAllClose(1.,
|
|
jax2tf.convert(f, polymorphic_shapes=["(a, b, 4)"])(x))
|
|
|
|
def test_mean0(self):
|
|
|
|
def f_jax(x):
|
|
return jnp.sum(x, axis=0) / jax2tf.shape_as_value(x)[0]
|
|
|
|
x = np.arange(12.).reshape((3, 4))
|
|
f_tf = self.CheckShapePolymorphism(
|
|
f_jax,
|
|
input_signature=[tf.TensorSpec([None, 4], dtype=x.dtype)],
|
|
polymorphic_shapes=[("batch, _")],
|
|
expected_output_signature=tf.TensorSpec([4]))
|
|
self.assertAllClose(np.array([4., 5., 6., 7.]), f_tf(x))
|
|
|
|
def test_mean_all_axes(self):
|
|
|
|
def f_jax(x):
|
|
return jnp.sum(x) / np.prod(jax2tf.shape_as_value(x))
|
|
|
|
x = np.arange(12.).reshape((3, 4))
|
|
f_tf = self.CheckShapePolymorphism(
|
|
f_jax,
|
|
input_signature=[tf.TensorSpec([None, 4], dtype=x.dtype)],
|
|
polymorphic_shapes=[("batch, _")],
|
|
expected_output_signature=tf.TensorSpec([]))
|
|
|
|
self.assertAllClose(jnp.mean(x), f_tf(x))
|
|
|
|
|
|
###
|
|
### We define primitive harnesses for which we will test shape-polymorphic
|
|
### conversion.
|
|
def _make_harness(group_name: str, name: str,
|
|
func: Callable,
|
|
args: primitive_harness.ArgDescriptor,
|
|
*,
|
|
poly_axes: Sequence[Optional[int]],
|
|
check_result=True,
|
|
tol=None,
|
|
**params) -> Harness:
|
|
"""The `poly_axes` must correspond to the non-static arguments, and for each
|
|
one it must specify which axes are: None, or an int.
|
|
|
|
The name of the harness within the group will include `poly_axes`.
|
|
You can add an additional `name`.
|
|
|
|
`check_result` specifies if we want to check that the result of the shape
|
|
polymorphic conversion produces the same result and the JAX function.
|
|
"""
|
|
poly_axes_name = "poly_axes=" + "_".join(map(str, poly_axes))
|
|
if name:
|
|
name = f"{name}_{poly_axes_name}"
|
|
else:
|
|
name = poly_axes_name
|
|
return Harness(group_name,
|
|
name,
|
|
func, args,
|
|
dtype=np.float32,
|
|
poly_axes=poly_axes,
|
|
check_result=check_result,
|
|
tol=tol,
|
|
**params)
|
|
|
|
|
|
_f32 = np.float32
|
|
|
|
_POLY_SHAPE_TEST_HARNESSES = [
|
|
_make_harness("jnp_add", "",
|
|
jnp.add,
|
|
[RandArg((3, 4), _f32), RandArg((2, 3, 4), _f32)],
|
|
poly_axes=[0, 1]),
|
|
|
|
_make_harness("jnp_broadcast_to", "",
|
|
lambda x: jnp.broadcast_to(x, [x.shape[0], x.shape[0], 4]),
|
|
[RandArg((3, 4), _f32)],
|
|
poly_axes=[0]),
|
|
|
|
_make_harness("clamp", "",
|
|
lax.clamp,
|
|
[RandArg((3, 4, 5), _f32), RandArg((3, 4, 5), _f32),
|
|
RandArg((3, 4, 5), _f32)],
|
|
poly_axes=[0, 0, 0]),
|
|
|
|
_make_harness("conv_general_dilated", "",
|
|
lambda lhs, rhs: lax.conv_general_dilated(lhs, rhs,
|
|
window_strides=(2, 3),
|
|
padding=((0, 0), (0, 0)),
|
|
lhs_dilation=(1, 1),
|
|
rhs_dilation=(1, 2),
|
|
dimension_numbers=("NCHW", "OIHW", "NCHW"),
|
|
feature_group_count=1,
|
|
batch_group_count=1,
|
|
precision=None),
|
|
[RandArg((7, 3, 9, 10), _f32), RandArg((3, 3, 4, 5), _f32)],
|
|
poly_axes=[0, None]),
|
|
|
|
_make_harness("cummax", "",
|
|
lambda x: lax_control_flow.cummax(x, axis=1, reverse=False),
|
|
[RandArg((3, 4, 5), _f32)],
|
|
poly_axes=[0]),
|
|
|
|
_make_harness("dot_general", "",
|
|
lambda lhs, rhs: lax.dot_general(lhs, rhs,
|
|
dimension_numbers=(((2,), (1,)), ((0,), (0,)))),
|
|
[RandArg((3, 4, 4), _f32), RandArg((3, 4), _f32)],
|
|
poly_axes=[0, 0]),
|
|
|
|
_make_harness("dynamic_slice", "",
|
|
# x:shape: (b, 4)
|
|
lambda x: lax.dynamic_slice(x, (0, 1), (x.shape[0], 2)),
|
|
[RandArg((3, 4), _f32)],
|
|
poly_axes=[0]),
|
|
|
|
_make_harness("jnp_take", "",
|
|
lambda a, i: jnp.take(a, i, axis=1),
|
|
[RandArg((3, 4, 5), _f32), np.array([1, 2], np.int32)],
|
|
poly_axes=[0, None]),
|
|
|
|
_make_harness("jnp_getitem", "",
|
|
lambda a, i: a[i],
|
|
[RandArg((3, 4), _f32), np.array([2, 2], np.int32)],
|
|
poly_axes=[None, 0]),
|
|
|
|
# TODO(necula): not supported yet
|
|
# _make_harness("jnp_getitem", "",
|
|
# lambda a, i: a[i],
|
|
# [RandArg((3, 4), _f32), np.array([2, 2], np.int32)],
|
|
# poly_axes=[0, 0]),
|
|
|
|
_make_harness("iota", "",
|
|
lambda x: x + lax.iota(_f32, x.shape[0]),
|
|
[RandArg((3,), _f32)],
|
|
poly_axes=[0]),
|
|
|
|
_make_harness("jnp_matmul", "0",
|
|
jnp.matmul,
|
|
[RandArg((7, 8, 4), _f32), RandArg((7, 4, 5), _f32)],
|
|
poly_axes=[0, 0],
|
|
tol=1e-5),
|
|
|
|
_make_harness("jnp_matmul", "1",
|
|
jnp.matmul,
|
|
[RandArg((7, 8, 4), _f32), RandArg((4, 5), _f32)],
|
|
poly_axes=[0, None],
|
|
tol=1e-5),
|
|
|
|
_make_harness("jnp_where", "",
|
|
jnp.where,
|
|
[RandArg((2,), np.bool_), RandArg((), _f32), RandArg((2,), _f32)],
|
|
poly_axes=[0, None, 0]),
|
|
|
|
_make_harness("pad", "",
|
|
lax.pad,
|
|
[RandArg((3, 2, 5), _f32), np.float32(5.),
|
|
StaticArg(((0, 0, 0), (0, 0, 0), (1, 1, 1)))],
|
|
poly_axes=[0, None]),
|
|
|
|
_make_harness("jnp_ones", "",
|
|
lambda x: jnp.ones(x.shape, dtype=_f32),
|
|
[RandArg((3, 2, 4), _f32)],
|
|
poly_axes=[0]),
|
|
|
|
# TODO: random_gamma does not work yet.
|
|
# _make_harness("random_gamma", "",
|
|
# lambda key, a: jax.random.gamma(key, a),
|
|
# [RandArg((3, 2), np.uint32), RandArg((3, 3), _f32)],
|
|
# poly_axes=[0, 0]),
|
|
|
|
_make_harness("reshape", "",
|
|
lambda x: x.reshape([x.shape[0], -1]),
|
|
[RandArg((3, 2, 3), _f32)],
|
|
poly_axes=[0]),
|
|
|
|
# TODO: support multiple poly axes
|
|
# _make_harness("reshape", "multi",
|
|
# lambda x: x.reshape([x.shape[0], -1, x.shape[3], x.shape[2]]),
|
|
# [RandArg((3, 4, 5, 6, 7), _f32)],
|
|
# poly_axes=[(0, 2, 3)]),
|
|
|
|
_make_harness("jnp_squeeze", "axis=None",
|
|
jnp.squeeze,
|
|
[RandArg((5,), _f32), StaticArg(())],
|
|
poly_axes=[0]),
|
|
|
|
_make_harness("jnp_squeeze", "axis=1",
|
|
jnp.squeeze,
|
|
[RandArg((4, 1), _f32), StaticArg((1,))],
|
|
poly_axes=[0]),
|
|
|
|
_make_harness("scatter_add", "",
|
|
partial(lax.scatter_add, indices_are_sorted=False, unique_indices=True),
|
|
[RandArg((7, 4), _f32),
|
|
np.array([[1], [2]], np.int32), # indices
|
|
RandArg((7, 2), _f32), # upd
|
|
StaticArg(lax.ScatterDimensionNumbers((0,), (1,), (1,)))],
|
|
poly_axes=[0, None, 0]),
|
|
|
|
_make_harness("slice", "entire_axis",
|
|
lambda x: lax.slice(x, start_indices=(0, 1), limit_indices=(x.shape[0], 3)),
|
|
[RandArg((7, 3), _f32)],
|
|
poly_axes=[0]),
|
|
|
|
_make_harness("select", "0",
|
|
# x.shape = (b, 3)
|
|
lambda x: lax.select(x > 5., x, x),
|
|
[RandArg((7, 3), _f32)],
|
|
poly_axes=[0]),
|
|
|
|
_make_harness("select", "1",
|
|
# x.shape = (b, 3); y.shape = (3,)
|
|
jax.vmap(lambda x, y: lax.select(x > 5., x, y), in_axes=[0, None]),
|
|
[RandArg((7, 3), _f32), RandArg((3,), _f32)],
|
|
poly_axes=[0, None]),
|
|
|
|
_make_harness("squeeze", "axis=1_2",
|
|
jnp.squeeze,
|
|
[RandArg((4, 1, 1), _f32), StaticArg((1, 2))],
|
|
poly_axes=[0]),
|
|
]
|
|
|
|
for reduce_op in [jnp.all, jnp.any, jnp.max, jnp.min, jnp.prod, jnp.sum]:
|
|
_POLY_SHAPE_TEST_HARNESSES.append(
|
|
_make_harness("reduce", reduce_op.__name__,
|
|
lambda x: reduce_op(x, axis=-1, keepdims=True),
|
|
[RandArg((3, 5), _f32)],
|
|
poly_axes=[0])
|
|
)
|
|
|
|
|
|
### We add to the test harnesses some that are obtained from the
|
|
### primitive harnesses by applying vmap to the function and then asserting
|
|
### that we can convert shape polymorphically the result.
|
|
|
|
def _add_vmap_primitive_harnesses():
|
|
"""For each harness group, pick a single dtype.
|
|
|
|
Ignore harnesses that fail in graph mode in jax2tf.
|
|
"""
|
|
all_h = primitive_harness.all_harnesses
|
|
|
|
# Index by group
|
|
harness_groups: Dict[
|
|
str, Sequence[primitive_harness.Harness]] = collections.defaultdict(list)
|
|
device = jtu.device_under_test()
|
|
|
|
for h in all_h:
|
|
# Drop the the JAX limitations
|
|
if not h.filter(device_under_test=device, include_jax_unimpl=False):
|
|
continue
|
|
# And the jax2tf limitations that are known to result in TF error.
|
|
if any(l.expect_tf_error for l in _get_jax2tf_limitations(device, h)):
|
|
continue
|
|
harness_groups[h.group_name].append(h)
|
|
|
|
selected_harnesses = []
|
|
for group_name, hlist in harness_groups.items():
|
|
# Pick the dtype with the most harnesses in this group. Some harness
|
|
# groups only test different use cases at a few dtypes.
|
|
c = collections.Counter([h.dtype for h in hlist])
|
|
(dtype, _), = c.most_common(1)
|
|
selected_harnesses.extend([h for h in hlist if h.dtype == dtype])
|
|
|
|
# We do not yet support shape polymorphism for vmap for some primitives
|
|
_NOT_SUPPORTED_YET = frozenset([
|
|
# In the random._gamma_impl we do reshape(-1, 2) for the keys
|
|
"random_gamma",
|
|
|
|
# In linalg._lu_python we do reshape(-1, ...)
|
|
"lu",
|
|
"custom_linear_solve",
|
|
|
|
# We do *= shapes in the batching rule for conv_general_dilated
|
|
"conv_general_dilated",
|
|
|
|
# vmap(clamp) fails in JAX
|
|
"clamp",
|
|
|
|
"iota", # vmap does not make sense for 0-argument functions
|
|
])
|
|
|
|
batch_size = 3
|
|
for h in selected_harnesses:
|
|
if h.group_name in _NOT_SUPPORTED_YET:
|
|
continue
|
|
|
|
def make_batched_arg_descriptor(
|
|
ad: primitive_harness.ArgDescriptor) -> Optional[primitive_harness.ArgDescriptor]:
|
|
if isinstance(ad, RandArg):
|
|
return RandArg((batch_size,) + ad.shape, ad.dtype)
|
|
elif isinstance(ad, CustomArg):
|
|
def wrap_custom(rng):
|
|
arg = ad.make(rng)
|
|
return np.stack([arg] * batch_size)
|
|
|
|
return CustomArg(wrap_custom)
|
|
else:
|
|
assert isinstance(ad, np.ndarray), ad
|
|
return np.stack([ad] * batch_size)
|
|
|
|
new_args = [make_batched_arg_descriptor(ad)
|
|
for ad in h.arg_descriptors
|
|
if not isinstance(ad, StaticArg)]
|
|
|
|
# We do not check the result of harnesses that require custom assertions.
|
|
check_result = all(not l.custom_assert and not l.skip_comparison and l.tol is None
|
|
for l in _get_jax2tf_limitations(device, h))
|
|
vmap_harness = _make_harness(h.group_name, f"vmap_{h.name}",
|
|
jax.vmap(h.dyn_fun, in_axes=0, out_axes=0),
|
|
new_args,
|
|
poly_axes=[0] * len(new_args),
|
|
check_result=check_result,
|
|
**h.params)
|
|
_POLY_SHAPE_TEST_HARNESSES.append(vmap_harness)
|
|
|
|
|
|
def _get_jax2tf_limitations(
|
|
device, h: primitive_harness.Harness) -> Sequence[Jax2TfLimitation]:
|
|
# And the jax2tf limitations
|
|
def applicable_jax2tf_limitation(l: Jax2TfLimitation) -> bool:
|
|
# The CheckShapePolymorphism uses tf.function, so we care about "graph"
|
|
return l.filter(device=device, dtype=h.dtype, mode="graph")
|
|
|
|
limitations = Jax2TfLimitation.limitations_for_harness(h)
|
|
return tuple(filter(applicable_jax2tf_limitation, limitations))
|
|
|
|
|
|
_add_vmap_primitive_harnesses()
|
|
|
|
|
|
class ShapePolyPrimitivesTest(tf_test_util.JaxToTfTestCase):
|
|
"""Tests for primitives that take shape values as parameters."""
|
|
|
|
# This test runs for all _POLY_SHAPE_PRIMITIVE_HARNESSES.
|
|
# For each primitive "xxx" the
|
|
# test will be called "test_prim_xxx_...".
|
|
# If you want to run this test for only one harness that includes "foo"
|
|
# in the name, add parameter `one_containing="foo"` to parameterized below.
|
|
@primitive_harness.parameterized(_POLY_SHAPE_TEST_HARNESSES)
|
|
def test_prim(self, harness: Harness):
|
|
args = harness.dyn_args_maker(self.rng())
|
|
poly_axes = harness.params["poly_axes"]
|
|
assert len(args) == len(poly_axes)
|
|
# Make the polymorphic_shapes and input_signature
|
|
polymorphic_shapes: List[Optional[str]] = []
|
|
input_signature: List[tf.TensorSpec] = []
|
|
for arg, poly_axis in zip(args, poly_axes):
|
|
if poly_axis is None:
|
|
polymorphic_shapes.append(None)
|
|
input_signature.append(tf.TensorSpec(np.shape(arg), arg.dtype))
|
|
else:
|
|
polymorphic_shapes.append(
|
|
", ".join([str(d) if i != poly_axis else "b"
|
|
for i, d in enumerate(arg.shape)]))
|
|
input_signature.append(tf.TensorSpec([d if i != poly_axis else None
|
|
for i, d in enumerate(arg.shape)],
|
|
arg.dtype))
|
|
|
|
res_jax = harness.dyn_fun(*args)
|
|
f_tf = self.CheckShapePolymorphism(
|
|
harness.dyn_fun,
|
|
input_signature=input_signature,
|
|
polymorphic_shapes=polymorphic_shapes,
|
|
expected_output_signature=None)
|
|
|
|
if harness.params["check_result"]:
|
|
tol = harness.params["tol"]
|
|
self.assertAllClose(res_jax, f_tf(*args), atol=tol, rtol=tol)
|
|
|
|
def test_reshape_error(self):
|
|
with self.assertRaisesRegex(
|
|
core.InconclusiveDimensionOperation,
|
|
re.escape("Shapes (batch, 2, batch, height, 3) and (batch, -1, batch) "
|
|
"must have the same set of shape variables")):
|
|
self.CheckShapePolymorphism(
|
|
lambda x: x.reshape([x.shape[0], -1, x.shape[2]]),
|
|
input_signature=[tf.TensorSpec([None, 2, None, None, 3])],
|
|
polymorphic_shapes=["batch, 2, batch, height, 3"],
|
|
expected_output_signature=tf.TensorSpec([None, 6, None]))
|
|
|
|
with self.assertRaisesRegex(
|
|
core.InconclusiveDimensionOperation,
|
|
re.escape(
|
|
"Cannot divide evenly the sizes of shapes (2, 4) and (-1, 3)")):
|
|
self.CheckShapePolymorphism(
|
|
lambda x: x.reshape([x.shape[0], -1, 3]),
|
|
input_signature=[tf.TensorSpec([None, 2, 4])],
|
|
polymorphic_shapes=[PS("batch", ...)],
|
|
expected_output_signature=tf.TensorSpec([None, 1]))
|
|
|
|
def test_reshape_compiled(self):
|
|
# We compile the result of conversion for two shapes, hence we need to
|
|
# involve the TF compiler twice, but we trace only once with shape polymorphism
|
|
traced = False
|
|
|
|
def f_jax(x):
|
|
nonlocal traced
|
|
traced = True
|
|
y = jnp.sin(x)
|
|
return y.reshape([x.shape[0], -1])
|
|
|
|
x = np.random.rand(4, 2, 3)
|
|
res_jax = f_jax(x)
|
|
|
|
traced = False
|
|
# If we get_concrete_function we trace once
|
|
f_tf = tf.function(
|
|
jax2tf.convert(f_jax, polymorphic_shapes=[PS("b", ...)]),
|
|
autograph=False,
|
|
jit_compile=True).get_concrete_function(
|
|
tf.TensorSpec([None, 2, 3], x.dtype))
|
|
self.assertTrue(traced)
|
|
traced = False
|
|
self.assertAllClose(res_jax, f_tf(x))
|
|
self.assertFalse(traced) # We are not tracing again
|
|
|
|
x = np.random.rand(6, 2, 3)
|
|
res_jax = f_jax(x)
|
|
traced = False
|
|
|
|
self.assertAllClose(res_jax, f_tf(x))
|
|
self.assertFalse(traced) # We are not tracing again
|
|
|
|
def test_squeeze_error(self):
|
|
|
|
with self.assertRaisesRegex(
|
|
core.InconclusiveDimensionOperation,
|
|
re.escape("Shape variable comparison b2 == 1 is inconclusive")):
|
|
# Trace with unknown dimension to squeeze
|
|
self.CheckShapePolymorphism(
|
|
lambda x: jnp.squeeze(x, axis=1),
|
|
input_signature=[tf.TensorSpec([None, None])],
|
|
polymorphic_shapes=[PS("b1", "b2")],
|
|
expected_output_signature=tf.TensorSpec([None]))
|
|
|
|
|
|
if __name__ == "__main__":
|
|
absltest.main(testLoader=jtu.JaxTestLoader())
|