mirror of
https://github.com/ROCm/jax.git
synced 2025-04-17 20:36:05 +00:00
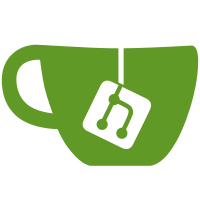
In https://github.com/jax-ml/jax/pull/23574, we added a new `algorithm` parameter to `lax.dot_general` with the goal of giving users explicit control over the specific algorithm used to control dot product accumulation. When using this feature in real use cases, we have found that the API is both too conservative (it required the user to pass the appropriate input types) and too restrictive for common use cases. In this change, I simplify the API to bring it more in line with user expectations, and generalize it to support a broader range of use cases. The core change is to update the dot_general lowering rule to add explicit type casts to the inputs, making sure that they always have the appropriate storage types going into the `DotGeneral` StableHLO op. Before this change, some backends would implicitly cast for some algorithms (e.g. f32 -> bf16), but error for others. It seems more user friendly to include automatic casts in all cases where a specific algorithm is requested. Another change in behavior is to (if needed) cast the result of the `DotGeneral` op (which is defined by the algorithm's `accumulation_type`) to match the input types. This means that, regardless of the algorithm choice, the output type will match the value that a user would expect from past use of `lax.dot_general`. The `preferred_element_type` parameter can now be used to control the output type, even when an algorithm is selected. To summarize, the updated version of `dot_general` accepts _any_ input dtypes, and the output will always match the inputs (under the existing promotion rules if the LHS and RHS don't match) unless `preferred_element_type` is used to select a specific output type. The specified "algorithm" is now more of an implementation detail, rather than the defining feature of the API, and JAX will do whatever it can to satisfy the user's request. (If an algorithm is not supported on the current device, we will still get a compile time error.) With the above changes in mind, it's no longer really necessary to have a `transpose_algorithm` parameter, because we can now use the same algorithm for the backwards pass. For users who need to customize the algorithm on the backwards pass, that is still possible using `custom_vjp`. Given the above changes, @sbodenstein made the excellent point that we don't really need the `algorithm` parameter anymore: just accept `DotAlgorithm` inputs to `precision`. I think this is a really nice suggestion, so I have updated the interface to implement this. One minor negative of this approach is that `preferred_element_type` isn't a great name for what that parameter does when it is used in conjunction with an algorithm. In the long run, I'd like to rename this parameter, but keeping it as is for now seems like the best short term approach. PiperOrigin-RevId: 683302687
486 lines
18 KiB
Python
486 lines
18 KiB
Python
# Copyright 2022 The JAX Authors.
|
|
#
|
|
# Licensed under the Apache License, Version 2.0 (the "License");
|
|
# you may not use this file except in compliance with the License.
|
|
# You may obtain a copy of the License at
|
|
#
|
|
# https://www.apache.org/licenses/LICENSE-2.0
|
|
#
|
|
# Unless required by applicable law or agreed to in writing, software
|
|
# distributed under the License is distributed on an "AS IS" BASIS,
|
|
# WITHOUT WARRANTIES OR CONDITIONS OF ANY KIND, either express or implied.
|
|
# See the License for the specific language governing permissions and
|
|
# limitations under the License.
|
|
"""`jax.experimental.rnn`: GPU accelerated RNN
|
|
|
|
----------------------------------------------
|
|
|
|
This module provides experimental support to CUDNN-backed LSTM.
|
|
|
|
Currently, the only supported RNN flavor is LSTM with double-bias. We use
|
|
notations and variable names similar to
|
|
https://pytorch.org/docs/stable/generated/torch.nn.LSTM.html#torch.nn.LSTM
|
|
|
|
and CUDNN_LSTM entry in
|
|
https://docs.nvidia.com/deeplearning/cudnn/api/index.html#cudnnRNNMode_t.
|
|
|
|
Note that a bidirectional LSTM is treated as having twice the number of layers,
|
|
where a forward layer i is followed by a reverse layer i. Each direction has
|
|
its own associated weights. We use pseudo-layer to denote such layers
|
|
following CUDNN documentation
|
|
https://docs.nvidia.com/deeplearning/cudnn/api/index.html#cudnnGetRNNWeightParams.
|
|
|
|
CUDNN takes an opaque 1D weight array that densely packs all the weight arrays
|
|
in a sparsely documented layout. Through trial-and-error and testing, we believe
|
|
the layout is the following. Assume 2-layer bi-LSTM with double-bias, so 4
|
|
pseudo-layers in total (forward-0, reverse-0, forward-1, reverse-1).
|
|
|
|
There are 4 kinds of weights: W_ih, W_hh, b_ih and b_hh, where
|
|
|
|
W_ih = (W_ii, W_if, W_ig, W_io) concatenated on leading axis,
|
|
W_hh = (W_hi, W_hf, W_hg, W_ho) concatenated on leading axis,
|
|
b_ih = (b_ii, b_if, b_ig, b_io) concatenated on leading axis,
|
|
b_hh = (b_hi, b_hf, b_hg, b_ho) concatenated on leading axis.
|
|
|
|
Say W_ih^0 denotates W_ih from pseudo-layer 0. The linear weights are packed
|
|
together from all pseudo-layers followed by bias weights from all pseudo-layers.
|
|
In particular, for each layer, W_ih is followed by W_hh and b_ih by b_hh.
|
|
|
|
(W_ih^0, W_hh^0, W_ih^1, W_hh^1, W_ih^2, W_hh^2, W_ih^3, W_hh^3,
|
|
b_ih^0, b_hh^0, b_ih^1, b_hh^1, b_ih^2, b_hh^2, b_ih^3, b_hh^3)
|
|
|
|
See `get_params_shapes_in_lstm`.
|
|
|
|
Example usage:
|
|
```
|
|
x = jax.random.normal(
|
|
k1, (batch_size, seq_len, input_size), dtype=jnp.float32)
|
|
h_0 = jax.random.normal(
|
|
k2, (num_directions * num_layers, batch_size, hidden_size),
|
|
dtype=jnp.float32)
|
|
c_0 = jax.random.normal(
|
|
k3, (num_directions * num_layers, batch_size, hidden_size),
|
|
dtype=jnp.float32)
|
|
seq_lengths = jnp.ones((batch_size,), dtype=jnp.int32) * seq_len
|
|
weights = rnn.init_lstm_weight(k4, input_size, hidden_size, num_layers,
|
|
bidirectional)
|
|
y, h_n, c_n = rnn.lstm(
|
|
x,
|
|
h_0,
|
|
c_0,
|
|
weights,
|
|
seq_lengths=seq_lengths,
|
|
input_size=input_size,
|
|
hidden_size=hidden_size,
|
|
num_layers=num_layers,
|
|
dropout=False,
|
|
bidirectional=bidirectional)
|
|
```
|
|
|
|
TODO:
|
|
- Add support for input and weight dtypes other than float32.
|
|
- Support ragged inputs.
|
|
- Support RNNs other than LSTM.
|
|
"""
|
|
from functools import partial
|
|
import math
|
|
from typing import cast, Any
|
|
|
|
import jax
|
|
import numpy as np
|
|
from jax._src import core
|
|
from jax.interpreters import mlir
|
|
from jax.interpreters import xla
|
|
from jax._src.custom_derivatives import custom_vjp
|
|
from jax._src.typing import Array, Shape
|
|
from jax._src.lax import lax
|
|
import jax.numpy as jnp
|
|
try:
|
|
from jax._src.lib import gpu_rnn
|
|
except ImportError:
|
|
gpu_rnn = None # type: ignore[assignment]
|
|
|
|
PRNGKeyArray = Any
|
|
sigmoid = jax.nn.sigmoid
|
|
tanh = jax.nn.tanh
|
|
|
|
|
|
def _W_ih_l(layer_i: int, input_size: int, hidden_size: int,
|
|
bidirectional: bool) -> Shape:
|
|
"""Shape of W_ii|W_if|W_ig|W_io.
|
|
|
|
Note that layer_i is an index of pseudo-layers.
|
|
"""
|
|
if layer_i == 0 or (layer_i == 1 and bidirectional):
|
|
return (4 * hidden_size, input_size)
|
|
else:
|
|
num_directions = 2 if bidirectional else 1
|
|
return (4 * hidden_size, num_directions * hidden_size)
|
|
|
|
|
|
def _W_hh_l(layer_i: int, input_size: int, hidden_size: int,
|
|
bidirectional: bool) -> Shape:
|
|
"""Shape of W_hi|W_hf|W_hg|W_ho."""
|
|
return (4 * hidden_size, hidden_size)
|
|
|
|
|
|
def _b_ih_l(layer_i: int, input_size: int, hidden_size: int,
|
|
bidirectional: bool) -> Shape:
|
|
"""Shape of b_ii|b_if|b_ig|b_io."""
|
|
return (4 * hidden_size,)
|
|
|
|
|
|
def _b_hh_l(layer_i: int, input_size: int, hidden_size: int,
|
|
bidirectional: bool) -> Shape:
|
|
"""Shape of b_hi|b_hf|b_hg|b_ho."""
|
|
return (4 * hidden_size,)
|
|
|
|
|
|
def _get_params_shapes_in_lstm(input_size: int, hidden_size: int,
|
|
num_layers: int,
|
|
bidirectional: bool) -> list[Shape]:
|
|
"""Get flat param shapes in LSTM. See module docstring for layout."""
|
|
layer_shapes = []
|
|
num_directions = 2 if bidirectional else 1
|
|
num_pseudo_layers = num_layers * num_directions
|
|
linear_weights = [_W_ih_l, _W_hh_l]
|
|
for i in range(num_pseudo_layers):
|
|
for w_kind in linear_weights:
|
|
layer_shape = w_kind(i, input_size, hidden_size, bidirectional)
|
|
layer_shapes.append(layer_shape)
|
|
|
|
bias_weights = [_b_ih_l, _b_hh_l]
|
|
for i in range(num_pseudo_layers):
|
|
for w_kind in bias_weights:
|
|
layer_shape = w_kind(i, input_size, hidden_size, bidirectional)
|
|
layer_shapes.append(layer_shape)
|
|
return layer_shapes
|
|
|
|
|
|
def get_num_params_in_lstm(input_size: int, hidden_size: int, num_layers: int,
|
|
bidirectional: bool) -> int:
|
|
"""Get param count in LSTM."""
|
|
layer_shapes = _get_params_shapes_in_lstm(input_size, hidden_size, num_layers,
|
|
bidirectional)
|
|
param_count = sum(math.prod(shape) for shape in layer_shapes)
|
|
return param_count
|
|
|
|
|
|
def init_lstm_weight(rng: PRNGKeyArray, input_size: int, hidden_size: int,
|
|
num_layers: int, bidirectional: bool):
|
|
"""Random initialize LSTM weights from U(-k, k), k=sqrt(1/hidden_size)."""
|
|
param_count = get_num_params_in_lstm(input_size, hidden_size, num_layers,
|
|
bidirectional)
|
|
k = np.sqrt(1.0 / hidden_size)
|
|
return jax.random.uniform(
|
|
rng, shape=(param_count,), dtype=jnp.float32, minval=-k, maxval=k)
|
|
|
|
|
|
def unpack_lstm_weights(
|
|
weights: Array, input_size: int, hidden_size: int, num_layers: int,
|
|
bidirectional: bool
|
|
) -> tuple[dict[int, Array], dict[int, Array], dict[int, Array], dict[int,
|
|
Array]]:
|
|
"""Unpack cudnn LSTM weights into individual weights.
|
|
|
|
CUDNN LSTM weight layout: (num_layers, num_directions, W_ih, W_hh, b_ih, b_hh)
|
|
Returns W_ih, W_hh, b_ih, b_hh. e.g. W_ih[2][1] is the concat weights of
|
|
4 weights (W_ii, W_if, W_ig, W_io), each of shape (hidden_size, input_size)
|
|
at 2nd layer for the reverse direction. See notations from
|
|
https://pytorch.org/docs/stable/generated/torch.nn.LSTM.html#torch.nn.LSTM.
|
|
"""
|
|
flat_shapes = _get_params_shapes_in_lstm(input_size, hidden_size, num_layers,
|
|
bidirectional)
|
|
flat_shapes_offset = 0
|
|
w_offsets = 0
|
|
num_directions = 2 if bidirectional else 1
|
|
num_pseudo_layers = num_layers * num_directions
|
|
|
|
W_ih: dict[int, Array] = {}
|
|
W_hh: dict[int, Array] = {}
|
|
for l in range(num_pseudo_layers):
|
|
for w_kind in [W_ih, W_hh]:
|
|
shape = flat_shapes[flat_shapes_offset]
|
|
flat_shapes_offset += 1
|
|
num_elems = math.prod(shape)
|
|
w_kind[l] = weights[w_offsets:w_offsets + num_elems].reshape(shape)
|
|
w_offsets += num_elems
|
|
|
|
b_ih: dict[int, Array] = {}
|
|
b_hh: dict[int, Array] = {}
|
|
for l in range(num_pseudo_layers):
|
|
for w_kind in [b_ih, b_hh]:
|
|
shape = flat_shapes[flat_shapes_offset]
|
|
flat_shapes_offset += 1
|
|
num_elems = math.prod(shape)
|
|
w_kind[l] = weights[w_offsets:w_offsets + num_elems].reshape(shape)
|
|
w_offsets += num_elems
|
|
return W_ih, W_hh, b_ih, b_hh
|
|
|
|
|
|
def _lstm_cudnn_allow_tf32(precision: lax.PrecisionLike) -> bool:
|
|
# the logic from canonicalize_precision that we require here boils down to:
|
|
#
|
|
# if precision is None and config.jax_default_matmul_precision is not None:
|
|
# precision = Precision(config.jax_default_matmul_precision)
|
|
# else:
|
|
# precision = None
|
|
#
|
|
# but we prefer to still invoke it here for consistency
|
|
precision = lax.canonicalize_precision(precision)
|
|
if precision is None or not (isinstance(precision, tuple) and len(precision) == 2):
|
|
return True
|
|
# cuDNN allows only one precision specifier per RNN op
|
|
precision, _ = cast(tuple[lax.Precision, lax.Precision], precision)
|
|
if precision == lax.Precision.HIGHEST:
|
|
return False
|
|
elif precision == lax.Precision.HIGH:
|
|
return True
|
|
elif precision == lax.Precision.DEFAULT: # bfloat16
|
|
raise NotImplementedError("bfloat16 support not implemented for LSTM")
|
|
else:
|
|
raise ValueError(f"Unexpected precision specifier value {precision}")
|
|
|
|
|
|
@partial(custom_vjp, nondiff_argnums=(5, 6, 7, 8, 9, 10))
|
|
def lstm(x: Array, h_0: Array, c_0: Array, weights: Array, seq_lengths: Array,
|
|
input_size: int, hidden_size: int, num_layers: int, dropout: float,
|
|
bidirectional: bool, precision: lax.PrecisionLike = None) -> tuple[Array, Array, Array]:
|
|
"""LSTM via CuDNN or HIPDNN (not-yet-supported).
|
|
|
|
Assume batch-first inputs.
|
|
|
|
Arguments:
|
|
x: (batch_size, max_seq_length, input_size)
|
|
h_0: (num_directions * num_layers, batch_size, hidden_size)
|
|
c_0: (num_directions * num_layers, batch_size, hidden_size)
|
|
weights: (num_params,) where num_params = get_num_params_in_lstm(...)
|
|
seq_lengths: (batch_size,)
|
|
Returns: (y, h_n, c_n, reserve_space).
|
|
y: (batch_size, max_seq_length, hidden_size * num_directions)
|
|
h_n: (num_directions * num_layers, batch_size, hidden_size)
|
|
c_n: (num_directions * num_layers, batch_size, hidden_size)
|
|
"""
|
|
(y, h_n, c_n), _ = lstm_fwd(
|
|
x,
|
|
h_0,
|
|
c_0,
|
|
weights,
|
|
seq_lengths,
|
|
input_size=input_size,
|
|
hidden_size=hidden_size,
|
|
num_layers=num_layers,
|
|
dropout=dropout,
|
|
bidirectional=bidirectional,
|
|
precision=precision)
|
|
return y, h_n, c_n
|
|
|
|
|
|
@partial(jax.jit, static_argnums=(8, 9, 10, 11, 12))
|
|
def lstm_ref(x: Array, h_0: Array, c_0: Array, W_ih: dict[int, Array],
|
|
W_hh: dict[int, Array], b_ih: dict[int, Array],
|
|
b_hh: dict[int, Array], seq_lengths: Array, input_size: int,
|
|
hidden_size: int, num_layers: int, dropout: float,
|
|
bidirectional: bool) -> tuple[Array, Array, Array]:
|
|
"""Reference implementation of LSTM.
|
|
|
|
See https://pytorch.org/docs/stable/generated/torch.nn.LSTM.html#lstm
|
|
https://docs.nvidia.com/deeplearning/cudnn/api/index.html#cudnnRNNMode_t
|
|
"""
|
|
if seq_lengths.dtype != jnp.dtype("int32"):
|
|
raise NotImplementedError("`seq_lengths` can only be int32.")
|
|
if dropout != 0.0:
|
|
raise NotImplementedError(
|
|
'Dropout not supported in LSTM reference because we cannot determine CUDNN dropout mask.'
|
|
)
|
|
|
|
# TODO(zhangqiaorjc): Handle ragged seq_lengths.
|
|
# batch_size, max_seq_length = x.shape[0], x.shape[1]
|
|
# assert seq_lengths.shape == (batch_size,)
|
|
# for i in range(batch_size):
|
|
# if int(seq_lengths[i]) != max_seq_length:
|
|
# raise NotImplementedError('Does not yet support ragged sequences.')
|
|
|
|
def lstm_cell(carry, x, *, W_ih, W_hh, b_ih, b_hh):
|
|
h, c = carry
|
|
W_ii, W_if, W_ig, W_io = jnp.split(W_ih, 4, axis=0)
|
|
W_hi, W_hf, W_hg, W_ho = jnp.split(W_hh, 4, axis=0)
|
|
b_ii, b_if, b_ig, b_io = jnp.split(b_ih, 4, axis=0)
|
|
b_hi, b_hf, b_hg, b_ho = jnp.split(b_hh, 4, axis=0)
|
|
i = sigmoid(x @ W_ii.T + b_ii[None] + h @ W_hi.T + b_hi[None])
|
|
f = sigmoid(x @ W_if.T + b_if[None] + h @ W_hf.T + b_hf[None])
|
|
g = tanh(x @ W_ig.T + b_ig[None] + h @ W_hg.T + b_hg[None])
|
|
o = sigmoid(x @ W_io.T + b_io[None] + h @ W_ho.T + b_ho[None])
|
|
c = f * c + i * g
|
|
h = o * tanh(c)
|
|
return (h, c), h
|
|
|
|
# here we also output the carry so that we can later slice
|
|
# the correct carry according to seq_lengths, while this takes more memory
|
|
# it is faster than using 'jnp.where' inside the scan loop
|
|
def scan_fn(cell, carry, x):
|
|
carry, y = cell(carry, x)
|
|
return carry, (carry, y)
|
|
|
|
seq_first_y = x.transpose(1, 0, 2)
|
|
if not bidirectional:
|
|
final_h = []
|
|
final_c = []
|
|
for l in range(num_layers):
|
|
cell = partial(
|
|
lstm_cell, W_ih=W_ih[l], W_hh=W_hh[l], b_ih=b_ih[l], b_hh=b_hh[l])
|
|
cell_fn = partial(scan_fn, cell)
|
|
out = jax.lax.scan(cell_fn, (h_0[l], c_0[l]),
|
|
seq_first_y)
|
|
(h_t, c_t), seq_first_y = _extract_output(seq_lengths, out)
|
|
final_h.append(h_t)
|
|
final_c.append(c_t)
|
|
h_n = jnp.stack(final_h)
|
|
c_n = jnp.stack(final_c)
|
|
return seq_first_y.transpose(1, 0, 2), h_n, c_n
|
|
|
|
# bidirectional
|
|
final_h = []
|
|
final_c = []
|
|
for l in range(num_layers * 2):
|
|
cell = partial(
|
|
lstm_cell, W_ih=W_ih[l], W_hh=W_hh[l], b_ih=b_ih[l], b_hh=b_hh[l])
|
|
cell_fn = partial(scan_fn, cell)
|
|
if l % 2 == 0:
|
|
out = jax.lax.scan(cell_fn, (h_0[l], c_0[l]),
|
|
seq_first_y)
|
|
(h_t, c_t), seq_first_y_fwd = _extract_output(seq_lengths, out)
|
|
else:
|
|
# reverse sequence while keeping padding at the end
|
|
seq_first_y_reversed = _flip_sequence(seq_first_y, seq_lengths)
|
|
out = jax.lax.scan(
|
|
cell_fn, (h_0[l], c_0[l]), seq_first_y_reversed)
|
|
(h_t, c_t), seq_first_y_bwd = _extract_output(seq_lengths, out)
|
|
# align reversed sequence with original sequence
|
|
seq_first_y_bwd = _flip_sequence(seq_first_y_bwd, seq_lengths)
|
|
# Inputs to next layer are concat'ed from fwd and bwd.
|
|
seq_first_y = jnp.concatenate([seq_first_y_fwd, seq_first_y_bwd], axis=-1) # pytype: disable=name-error
|
|
final_h.append(h_t)
|
|
final_c.append(c_t)
|
|
h_n = jnp.stack(final_h)
|
|
c_n = jnp.stack(final_c)
|
|
return seq_first_y.transpose(1, 0, 2), h_n, c_n
|
|
|
|
def _extract_output(seq_lengths: Array, out) -> tuple[tuple[Array, Array], Array]:
|
|
_, ((hs, cs), seq_first_y) = out
|
|
h_t = _select_last_carry(hs, seq_lengths)
|
|
c_t = _select_last_carry(cs, seq_lengths)
|
|
|
|
# [seq_len, batch] [1, batch] [seq_len, 1]
|
|
mask = seq_lengths[None] > jnp.arange(seq_first_y.shape[0], dtype=jnp.int32)[:, None]
|
|
# [batch, seq_len, hidden_size]
|
|
seq_first_y = jnp.where(
|
|
mask[..., None], # [seq_len, batch, 1]
|
|
seq_first_y, # [seq_len, batch, hidden_size]
|
|
0)
|
|
return (h_t, c_t), seq_first_y
|
|
|
|
def _select_last_carry(carry_seq: Array, seq_lengths: Array):
|
|
return carry_seq[seq_lengths - 1, jnp.arange(carry_seq.shape[1])]
|
|
|
|
def _flip_sequence(sequences: Array, seq_lengths: Array) -> Array:
|
|
max_steps = sequences.shape[0]
|
|
roll_amounts = max_steps - seq_lengths
|
|
# roll initially puts padding at the front so when the sequence is reversed
|
|
# (via [::-1]) the padding stays at the end
|
|
return jax.vmap(partial(jnp.roll, axis=0), in_axes=(1, 0),
|
|
out_axes=1)(sequences, roll_amounts)[::-1]
|
|
|
|
def lstm_fwd(x: Array, h_0: Array, c_0: Array, w: Array, seq_lengths: Array,
|
|
input_size: int, hidden_size: int, num_layers: int, dropout: float,
|
|
bidirectional: bool, precision: lax.PrecisionLike):
|
|
if seq_lengths.dtype != jnp.dtype("int32"):
|
|
raise NotImplementedError("`seq_lengths` can only be int32.")
|
|
cudnn_allow_tf32 = _lstm_cudnn_allow_tf32(precision)
|
|
y, h_n, c_n, reserve_space = rnn_fwd_p.bind(
|
|
x,
|
|
h_0,
|
|
c_0,
|
|
w,
|
|
seq_lengths,
|
|
input_size=input_size,
|
|
hidden_size=hidden_size,
|
|
num_layers=num_layers,
|
|
dropout=dropout,
|
|
bidirectional=bidirectional,
|
|
cudnn_allow_tf32=cudnn_allow_tf32)
|
|
return (y, h_n, c_n), (x, h_0, c_0, w, seq_lengths, y, reserve_space)
|
|
|
|
|
|
def rnn_abstract_eval(x_aval, h_0_aval, c_0_aval, w_aval, seq_lengths_aval,
|
|
input_size: int, hidden_size: int, num_layers: int,
|
|
dropout: float, bidirectional: bool,
|
|
cudnn_allow_tf32: bool):
|
|
batch_size, max_seq_length = x_aval.shape[0], x_aval.shape[1]
|
|
num_directions = 2 if bidirectional else 1
|
|
output_shape = (batch_size, max_seq_length, num_directions * hidden_size)
|
|
output_aval = core.ShapedArray(output_shape, x_aval.dtype)
|
|
_, reserve_space_size = (
|
|
gpu_rnn.compute_rnn_workspace_reserve_space_sizes( # pytype: disable=attribute-error
|
|
input_size, hidden_size, num_layers, batch_size, max_seq_length,
|
|
dropout, bidirectional, cudnn_allow_tf32))
|
|
reserve_space_aval = core.ShapedArray((reserve_space_size,), jnp.float32)
|
|
return output_aval, h_0_aval, c_0_aval, reserve_space_aval
|
|
|
|
|
|
def _gpu_lowering_strip_tf32(fn, *args, cudnn_allow_tf32, **kw):
|
|
del cudnn_allow_tf32
|
|
return fn(*args, **kw)
|
|
|
|
rnn_fwd_p = core.Primitive('rnn_fwd')
|
|
rnn_fwd_p.multiple_results = True
|
|
rnn_fwd_p.def_impl(partial(xla.apply_primitive, rnn_fwd_p))
|
|
rnn_fwd_p.def_abstract_eval(rnn_abstract_eval)
|
|
if gpu_rnn:
|
|
mlir.register_lowering(rnn_fwd_p, gpu_rnn.cudnn_rnn_lowering, platform='cuda')
|
|
|
|
|
|
def lstm_bwd(input_size: int, hidden_size: int, num_layers: int, dropout: float,
|
|
bidirectional: bool, precision: lax.PrecisionLike,
|
|
residuals, gradients):
|
|
cudnn_allow_tf32 = _lstm_cudnn_allow_tf32(precision)
|
|
x, h_0, c_0, w, seq_lengths, y, reserve_space = residuals
|
|
dy, dh_n, dc_n = gradients
|
|
dx, dh_0, dc_0, dw = rnn_bwd_p.bind(
|
|
dy,
|
|
dh_n,
|
|
dc_n,
|
|
x,
|
|
h_0,
|
|
c_0,
|
|
w,
|
|
y,
|
|
reserve_space,
|
|
seq_lengths,
|
|
input_size=input_size,
|
|
hidden_size=hidden_size,
|
|
num_layers=num_layers,
|
|
dropout=dropout,
|
|
bidirectional=bidirectional,
|
|
cudnn_allow_tf32=cudnn_allow_tf32)
|
|
return (dx, dh_0, dc_0, dw, jnp.zeros_like(seq_lengths))
|
|
|
|
|
|
def rnn_bwd_abstract_eval(dy_aval, dhn_aval, dcn_aval, x_aval, h0_aval, c0_aval,
|
|
w_aval, y_aval, reserve_space_aval,
|
|
seq_lengths_aval, input_size: int, hidden_size: int,
|
|
num_layers: int, dropout: float, bidirectional: bool,
|
|
cudnn_allow_tf32: bool):
|
|
return x_aval, h0_aval, c0_aval, w_aval
|
|
|
|
|
|
rnn_bwd_p = core.Primitive('rnn_bwd')
|
|
rnn_bwd_p.multiple_results = True
|
|
rnn_bwd_p.def_impl(partial(xla.apply_primitive, rnn_bwd_p))
|
|
rnn_bwd_p.def_abstract_eval(rnn_bwd_abstract_eval)
|
|
if gpu_rnn:
|
|
mlir.register_lowering(
|
|
rnn_bwd_p, gpu_rnn.cudnn_rnn_bwd_lowering, platform='cuda')
|
|
|
|
lstm.defvjp(lstm_fwd, lstm_bwd)
|