mirror of
https://github.com/ROCm/jax.git
synced 2025-04-14 10:56:06 +00:00
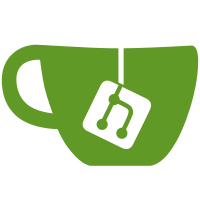
Move the common jaxlib/gpu_plugin_extension into jaxlib/gpu/ Cleanup only, no functional changes intended. PiperOrigin-RevId: 738183402
10148 lines
350 KiB
Python
10148 lines
350 KiB
Python
# Copyright 2018 The JAX Authors.
|
||
#
|
||
# Licensed under the Apache License, Version 2.0 (the "License");
|
||
# you may not use this file except in compliance with the License.
|
||
# You may obtain a copy of the License at
|
||
#
|
||
# https://www.apache.org/licenses/LICENSE-2.0
|
||
#
|
||
# Unless required by applicable law or agreed to in writing, software
|
||
# distributed under the License is distributed on an "AS IS" BASIS,
|
||
# WITHOUT WARRANTIES OR CONDITIONS OF ANY KIND, either express or implied.
|
||
# See the License for the specific language governing permissions and
|
||
# limitations under the License.
|
||
|
||
# pytype: skip-file
|
||
"""
|
||
Implements the NumPy API, using the primitives in :mod:`jax.lax`.
|
||
|
||
NumPy operations are implemented in Python in terms of the primitive operations
|
||
in :mod:`jax.lax`. Since NumPy operations are not primitive and instead are
|
||
implemented in terms of :mod:`jax.lax` operations, we do not need to define
|
||
transformation rules such as gradient or batching rules. Instead,
|
||
transformations for NumPy primitives can be derived from the transformation
|
||
rules for the underlying :code:`lax` primitives.
|
||
"""
|
||
from __future__ import annotations
|
||
|
||
import builtins
|
||
from collections.abc import Callable, Sequence
|
||
from functools import partial
|
||
import importlib
|
||
import math
|
||
import operator
|
||
import os
|
||
from typing import (Any, IO, Literal, Protocol, TypeVar, Union, overload)
|
||
import warnings
|
||
|
||
import jax
|
||
from jax import jit
|
||
from jax import lax
|
||
from jax._src import config
|
||
from jax._src import core
|
||
from jax._src import deprecations
|
||
from jax._src import dtypes
|
||
from jax._src import xla_bridge
|
||
from jax._src.api_util import _ensure_index_tuple
|
||
from jax._src.custom_derivatives import custom_jvp
|
||
from jax._src.lax import lax as lax_internal
|
||
from jax._src.lax.lax import (PrecisionLike,_array_copy,
|
||
_sort_le_comparator, _sort_lt_comparator)
|
||
from jax._src.lib import xla_client as xc
|
||
from jax._src.numpy.array_creation import (empty, empty_like, full,
|
||
ones, ones_like, zeros, zeros_like)
|
||
from jax._src.numpy import indexing
|
||
from jax._src.numpy import reductions
|
||
from jax._src.numpy import tensor_contractions
|
||
from jax._src.numpy import ufuncs
|
||
from jax._src.numpy import util
|
||
from jax._src.numpy.sorting import argsort, sort
|
||
from jax._src.numpy.vectorize import vectorize
|
||
from jax._src.typing import (
|
||
Array, ArrayLike, DType, DTypeLike, DeprecatedArg, DimSize, Shape
|
||
)
|
||
from jax._src.util import (
|
||
NumpyComplexWarning, canonicalize_axis as _canonicalize_axis,
|
||
ceil_of_ratio, safe_zip, set_module, unzip2)
|
||
from jax.sharding import Sharding
|
||
from jax._src.sharding_impls import SingleDeviceSharding
|
||
from jax.tree_util import tree_leaves, tree_map
|
||
import numpy as np
|
||
|
||
export = set_module('jax.numpy')
|
||
|
||
for pkg_name in ['jax_cuda12_plugin', 'jax.jaxlib.cuda']:
|
||
try:
|
||
cuda_plugin_extension = importlib.import_module(
|
||
f'{pkg_name}.cuda_plugin_extension'
|
||
)
|
||
except ImportError:
|
||
cuda_plugin_extension = None # type: ignore
|
||
else:
|
||
break
|
||
|
||
T = TypeVar('T')
|
||
|
||
# Wrappers for NumPy printoptions
|
||
|
||
def get_printoptions():
|
||
"""Alias of :func:`numpy.get_printoptions`.
|
||
|
||
JAX arrays are printed via NumPy, so NumPy's `printoptions`
|
||
configurations will apply to printed JAX arrays.
|
||
|
||
See the :func:`numpy.set_printoptions` documentation for details
|
||
on the available options and their meanings.
|
||
"""
|
||
return np.get_printoptions()
|
||
|
||
def printoptions(*args, **kwargs):
|
||
"""Alias of :func:`numpy.printoptions`.
|
||
|
||
JAX arrays are printed via NumPy, so NumPy's `printoptions`
|
||
configurations will apply to printed JAX arrays.
|
||
|
||
See the :func:`numpy.set_printoptions` documentation for details
|
||
on the available options and their meanings.
|
||
"""
|
||
return np.printoptions(*args, **kwargs)
|
||
|
||
def set_printoptions(*args, **kwargs):
|
||
"""Alias of :func:`numpy.set_printoptions`.
|
||
|
||
JAX arrays are printed via NumPy, so NumPy's `printoptions`
|
||
configurations will apply to printed JAX arrays.
|
||
|
||
See the :func:`numpy.set_printoptions` documentation for details
|
||
on the available options and their meanings.
|
||
"""
|
||
return np.set_printoptions(*args, **kwargs)
|
||
|
||
@export
|
||
def iscomplexobj(x: Any) -> bool:
|
||
"""Check if the input is a complex number or an array containing complex elements.
|
||
|
||
JAX implementation of :func:`numpy.iscomplexobj`.
|
||
|
||
The function evaluates based on input type rather than value.
|
||
Inputs with zero imaginary parts are still considered complex.
|
||
|
||
Args:
|
||
x: input object to check.
|
||
|
||
Returns:
|
||
True if ``x`` is a complex number or an array containing at least one complex element,
|
||
False otherwise.
|
||
|
||
See Also:
|
||
- :func:`jax.numpy.isrealobj`
|
||
- :func:`jax.numpy.iscomplex`
|
||
|
||
Examples:
|
||
>>> jnp.iscomplexobj(True)
|
||
False
|
||
>>> jnp.iscomplexobj(0)
|
||
False
|
||
>>> jnp.iscomplexobj(jnp.array([1, 2]))
|
||
False
|
||
>>> jnp.iscomplexobj(1+2j)
|
||
True
|
||
>>> jnp.iscomplexobj(jnp.array([0, 1+2j]))
|
||
True
|
||
"""
|
||
if x is None:
|
||
return False
|
||
try:
|
||
typ = x.dtype.type
|
||
except AttributeError:
|
||
typ = asarray(x).dtype.type
|
||
return issubdtype(typ, np.complexfloating)
|
||
|
||
|
||
def _dtype(x: Any) -> DType:
|
||
return dtypes.dtype(x, canonicalize=True)
|
||
|
||
# Dtype-related functions
|
||
iinfo = dtypes.iinfo
|
||
finfo = dtypes.finfo
|
||
|
||
can_cast = dtypes.can_cast
|
||
promote_types = dtypes.promote_types
|
||
|
||
ComplexWarning = NumpyComplexWarning
|
||
|
||
_lax_const = lax_internal._const
|
||
|
||
|
||
def _convert_and_clip_integer(val: ArrayLike, dtype: DType) -> Array:
|
||
"""
|
||
Convert integer-typed val to specified integer dtype, clipping to dtype
|
||
range rather than wrapping.
|
||
|
||
Args:
|
||
val: value to be converted
|
||
dtype: dtype of output
|
||
|
||
Returns:
|
||
equivalent of val in new dtype
|
||
|
||
Examples
|
||
--------
|
||
Normal integer type conversion will wrap:
|
||
|
||
>>> val = jnp.uint32(0xFFFFFFFF)
|
||
>>> val.astype('int32')
|
||
Array(-1, dtype=int32)
|
||
|
||
This function clips to the values representable in the new type:
|
||
|
||
>>> _convert_and_clip_integer(val, 'int32')
|
||
Array(2147483647, dtype=int32)
|
||
"""
|
||
val = val if isinstance(val, Array) else asarray(val)
|
||
dtype = dtypes.canonicalize_dtype(dtype)
|
||
if not (issubdtype(dtype, np.integer) and issubdtype(val.dtype, np.integer)):
|
||
raise TypeError("_convert_and_clip_integer only accepts integer dtypes.")
|
||
|
||
val_dtype = dtypes.canonicalize_dtype(val.dtype)
|
||
if val_dtype != val.dtype:
|
||
# TODO(jakevdp): this is a weird corner case; need to figure out how to handle it.
|
||
# This happens in X32 mode and can either come from a jax value created in another
|
||
# context, or a Python integer converted to int64.
|
||
pass
|
||
min_val = _lax_const(val, max(iinfo(dtype).min, iinfo(val_dtype).min))
|
||
max_val = _lax_const(val, min(iinfo(dtype).max, iinfo(val_dtype).max))
|
||
return clip(val, min_val, max_val).astype(dtype)
|
||
|
||
|
||
@export
|
||
def load(file: IO[bytes] | str | os.PathLike[Any], *args: Any, **kwargs: Any) -> Array:
|
||
"""Load JAX arrays from npy files.
|
||
|
||
JAX wrapper of :func:`numpy.load`.
|
||
|
||
This function is a simple wrapper of :func:`numpy.load`, but in the case of
|
||
``.npy`` files created with :func:`numpy.save` or :func:`jax.numpy.save`,
|
||
the output will be returned as a :class:`jax.Array`, and ``bfloat16`` data
|
||
types will be restored. For ``.npz`` files, results will be returned as
|
||
normal NumPy arrays.
|
||
|
||
This function requires concrete array inputs, and is not compatible with
|
||
transformations like :func:`jax.jit` or :func:`jax.vmap`.
|
||
|
||
Args:
|
||
file: string, bytes, or path-like object containing the array data.
|
||
args, kwargs: for additional arguments, see :func:`numpy.load`
|
||
|
||
Returns:
|
||
the array stored in the file.
|
||
|
||
See also:
|
||
- :func:`jax.numpy.save`: save an array to a file.
|
||
|
||
Examples:
|
||
>>> import io
|
||
>>> f = io.BytesIO() # use an in-memory file-like object.
|
||
>>> x = jnp.array([2, 4, 6, 8], dtype='bfloat16')
|
||
>>> jnp.save(f, x)
|
||
>>> f.seek(0)
|
||
0
|
||
>>> jnp.load(f)
|
||
Array([2, 4, 6, 8], dtype=bfloat16)
|
||
"""
|
||
# The main purpose of this wrapper is to recover bfloat16 data types.
|
||
# Note: this will only work for files created via np.save(), not np.savez().
|
||
out = np.load(file, *args, **kwargs)
|
||
if isinstance(out, np.ndarray):
|
||
# numpy does not recognize bfloat16, so arrays are serialized as void16
|
||
if out.dtype == 'V2':
|
||
out = out.view(dtypes.bfloat16)
|
||
try:
|
||
out = asarray(out)
|
||
except (TypeError, AssertionError): # Unsupported dtype
|
||
pass
|
||
return out
|
||
|
||
### implementations of numpy functions in terms of lax
|
||
|
||
@export
|
||
@jit
|
||
def fmin(x1: ArrayLike, x2: ArrayLike) -> Array:
|
||
"""Return element-wise minimum of the input arrays.
|
||
|
||
JAX implemtentation of :func:`numpy.fmin`.
|
||
|
||
Args:
|
||
x1: input array or scalar.
|
||
x2: input array or scalar. x1 and x2 must either have same shape or be
|
||
broadcast compatible.
|
||
|
||
Returns:
|
||
An array containing the element-wise minimum of x1 and x2.
|
||
|
||
Note:
|
||
For each pair of elements, ``jnp.fmin`` returns:
|
||
- the smaller of the two if both elements are finite numbers.
|
||
- finite number if one element is ``nan``.
|
||
- ``-inf`` if one element is ``-inf`` and the other is finite or ``nan``.
|
||
- ``inf`` if one element is ``inf`` and the other is ``nan``.
|
||
- ``nan`` if both elements are ``nan``.
|
||
|
||
Examples:
|
||
>>> jnp.fmin(2, 3)
|
||
Array(2, dtype=int32, weak_type=True)
|
||
>>> jnp.fmin(2, jnp.array([1, 4, 2, -1]))
|
||
Array([ 1, 2, 2, -1], dtype=int32)
|
||
|
||
>>> x1 = jnp.array([1, 3, 2])
|
||
>>> x2 = jnp.array([2, 1, 4])
|
||
>>> jnp.fmin(x1, x2)
|
||
Array([1, 1, 2], dtype=int32)
|
||
|
||
>>> x3 = jnp.array([1, 5, 3])
|
||
>>> x4 = jnp.array([[2, 3, 1],
|
||
... [5, 6, 7]])
|
||
>>> jnp.fmin(x3, x4)
|
||
Array([[1, 3, 1],
|
||
[1, 5, 3]], dtype=int32)
|
||
|
||
>>> nan = jnp.nan
|
||
>>> x5 = jnp.array([jnp.inf, 5, nan])
|
||
>>> x6 = jnp.array([[2, 3, nan],
|
||
... [nan, 6, 7]])
|
||
>>> jnp.fmin(x5, x6)
|
||
Array([[ 2., 3., nan],
|
||
[inf, 5., 7.]], dtype=float32)
|
||
"""
|
||
return where(ufuncs.less(x1, x2) | ufuncs.isnan(x2), x1, x2)
|
||
|
||
|
||
@export
|
||
@jit
|
||
def fmax(x1: ArrayLike, x2: ArrayLike) -> Array:
|
||
"""Return element-wise maximum of the input arrays.
|
||
|
||
JAX implementation of :func:`numpy.fmax`.
|
||
|
||
Args:
|
||
x1: input array or scalar
|
||
x2: input array or scalar. x1 and x1 must either have same shape or be
|
||
broadcast compatible.
|
||
|
||
Returns:
|
||
An array containing the element-wise maximum of x1 and x2.
|
||
|
||
Note:
|
||
For each pair of elements, ``jnp.fmax`` returns:
|
||
- the larger of the two if both elements are finite numbers.
|
||
- finite number if one element is ``nan``.
|
||
- ``nan`` if both elements are ``nan``.
|
||
- ``inf`` if one element is ``inf`` and the other is finite or ``nan``.
|
||
- ``-inf`` if one element is ``-inf`` and the other is ``nan``.
|
||
|
||
Examples:
|
||
>>> jnp.fmax(3, 7)
|
||
Array(7, dtype=int32, weak_type=True)
|
||
>>> jnp.fmax(5, jnp.array([1, 7, 9, 4]))
|
||
Array([5, 7, 9, 5], dtype=int32)
|
||
|
||
>>> x1 = jnp.array([1, 3, 7, 8])
|
||
>>> x2 = jnp.array([-1, 4, 6, 9])
|
||
>>> jnp.fmax(x1, x2)
|
||
Array([1, 4, 7, 9], dtype=int32)
|
||
|
||
>>> x3 = jnp.array([[2, 3, 5, 10],
|
||
... [11, 9, 7, 5]])
|
||
>>> jnp.fmax(x1, x3)
|
||
Array([[ 2, 3, 7, 10],
|
||
[11, 9, 7, 8]], dtype=int32)
|
||
|
||
>>> x4 = jnp.array([jnp.inf, 6, -jnp.inf, nan])
|
||
>>> x5 = jnp.array([[3, 5, 7, nan],
|
||
... [nan, 9, nan, -1]])
|
||
>>> jnp.fmax(x4, x5)
|
||
Array([[ inf, 6., 7., nan],
|
||
[ inf, 9., -inf, -1.]], dtype=float32)
|
||
"""
|
||
return where(ufuncs.greater(x1, x2) | ufuncs.isnan(x2), x1, x2)
|
||
|
||
|
||
@export
|
||
def issubdtype(arg1: DTypeLike, arg2: DTypeLike) -> bool:
|
||
"""Return True if arg1 is equal or lower than arg2 in the type hierarchy.
|
||
|
||
JAX implementation of :func:`numpy.issubdtype`.
|
||
|
||
The main difference in JAX's implementation is that it properly handles
|
||
dtype extensions such as :code:`bfloat16`.
|
||
|
||
Args:
|
||
arg1: dtype-like object. In typical usage, this will be a dtype specifier,
|
||
such as ``"float32"`` (i.e. a string), ``np.dtype('int32')`` (i.e. an
|
||
instance of :class:`numpy.dtype`), ``jnp.complex64`` (i.e. a JAX scalar
|
||
constructor), or ``np.uint8`` (i.e. a NumPy scalar type).
|
||
arg2: dtype-like object. In typical usage, this will be a generic scalar
|
||
type, such as ``jnp.integer``, ``jnp.floating``, or ``jnp.complexfloating``.
|
||
|
||
Returns:
|
||
True if arg1 represents a dtype that is equal or lower in the type
|
||
hierarchy than arg2.
|
||
|
||
See also:
|
||
- :func:`jax.numpy.isdtype`: similar function aligning with the array API standard.
|
||
|
||
Examples:
|
||
>>> jnp.issubdtype('uint32', jnp.unsignedinteger)
|
||
True
|
||
>>> jnp.issubdtype(np.int32, jnp.integer)
|
||
True
|
||
>>> jnp.issubdtype(jnp.bfloat16, jnp.floating)
|
||
True
|
||
>>> jnp.issubdtype(np.dtype('complex64'), jnp.complexfloating)
|
||
True
|
||
>>> jnp.issubdtype('complex64', jnp.integer)
|
||
False
|
||
|
||
Be aware that while this is very similar to :func:`numpy.issubdtype`, the
|
||
results of these differ in the case of JAX's custom floating point types:
|
||
|
||
>>> np.issubdtype('bfloat16', np.floating)
|
||
False
|
||
>>> jnp.issubdtype('bfloat16', jnp.floating)
|
||
True
|
||
"""
|
||
return dtypes.issubdtype(arg1, arg2)
|
||
|
||
|
||
@export
|
||
def isscalar(element: Any) -> bool:
|
||
"""Return True if the input is a scalar.
|
||
|
||
JAX implementation of :func:`numpy.isscalar`. JAX's implementation differs
|
||
from NumPy's in that it considers zero-dimensional arrays to be scalars; see
|
||
the *Note* below for more details.
|
||
|
||
Args:
|
||
element: input object to check; any type is valid input.
|
||
|
||
Returns:
|
||
True if ``element`` is a scalar value or an array-like object with zero
|
||
dimensions, False otherwise.
|
||
|
||
Note:
|
||
JAX and NumPy differ in their representation of scalar values. NumPy has
|
||
special scalar objects (e.g. ``np.int32(0)``) which are distinct from
|
||
zero-dimensional arrays (e.g. ``np.array(0)``), and :func:`numpy.isscalar`
|
||
returns ``True`` for the former and ``False`` for the latter.
|
||
|
||
JAX does not define special scalar objects, but rather represents scalars as
|
||
zero-dimensional arrays. As such, :func:`jax.numpy.isscalar` returns ``True``
|
||
for both scalar objects (e.g. ``0.0`` or ``np.float32(0.0)``) and array-like
|
||
objects with zero dimensions (e.g. ``jnp.array(0.0)``, ``np.array(0.0)``).
|
||
|
||
One reason for the different conventions in ``isscalar`` is to maintain
|
||
JIT-invariance: i.e. the property that the result of a function should not
|
||
change when it is JIT-compiled. Because scalar inputs are cast to
|
||
zero-dimensional JAX arrays at JIT boundaries, the semantics of
|
||
:func:`numpy.isscalar` are such that the result changes under JIT:
|
||
|
||
>>> np.isscalar(1.0)
|
||
True
|
||
>>> jax.jit(np.isscalar)(1.0)
|
||
Array(False, dtype=bool)
|
||
|
||
By treating zero-dimensional arrays as scalars, :func:`jax.numpy.isscalar`
|
||
avoids this issue:
|
||
|
||
>>> jnp.isscalar(1.0)
|
||
True
|
||
>>> jax.jit(jnp.isscalar)(1.0)
|
||
Array(True, dtype=bool)
|
||
|
||
Examples:
|
||
In JAX, both scalars and zero-dimensional array-like objects are considered
|
||
scalars:
|
||
|
||
>>> jnp.isscalar(1.0)
|
||
True
|
||
>>> jnp.isscalar(1 + 1j)
|
||
True
|
||
>>> jnp.isscalar(jnp.array(1)) # zero-dimensional JAX array
|
||
True
|
||
>>> jnp.isscalar(jnp.int32(1)) # JAX scalar constructor
|
||
True
|
||
>>> jnp.isscalar(np.array(1.0)) # zero-dimensional NumPy array
|
||
True
|
||
>>> jnp.isscalar(np.int32(1)) # NumPy scalar type
|
||
True
|
||
|
||
Arrays with one or more dimension are not considered scalars:
|
||
|
||
>>> jnp.isscalar(jnp.array([1]))
|
||
False
|
||
>>> jnp.isscalar(np.array([1]))
|
||
False
|
||
|
||
Compare this to :func:`numpy.isscalar`, which returns ``True`` for
|
||
scalar-typed objects, and ``False`` for *all* arrays, even those with
|
||
zero dimensions:
|
||
|
||
>>> np.isscalar(np.int32(1)) # scalar object
|
||
True
|
||
>>> np.isscalar(np.array(1)) # zero-dimensional array
|
||
False
|
||
|
||
In JAX, as in NumPy, objects which are not array-like are not considered
|
||
scalars:
|
||
|
||
>>> jnp.isscalar(None)
|
||
False
|
||
>>> jnp.isscalar([1])
|
||
False
|
||
>>> jnp.isscalar(tuple())
|
||
False
|
||
>>> jnp.isscalar(slice(10))
|
||
False
|
||
"""
|
||
if np.isscalar(element):
|
||
return True
|
||
elif isinstance(element, (np.ndarray, jax.Array)):
|
||
return element.ndim == 0
|
||
elif hasattr(element, '__jax_array__'):
|
||
return asarray(element).ndim == 0
|
||
return False
|
||
|
||
|
||
@export
|
||
def result_type(*args: Any) -> DType:
|
||
"""Return the result of applying JAX promotion rules to the inputs.
|
||
|
||
JAX implementation of :func:`numpy.result_type`.
|
||
|
||
JAX's dtype promotion behavior is described in :ref:`type-promotion`.
|
||
|
||
Args:
|
||
args: one or more arrays or dtype-like objects.
|
||
|
||
Returns:
|
||
A :class:`numpy.dtype` instance representing the result of type
|
||
promotion for the inputs.
|
||
|
||
Examples:
|
||
Inputs can be dtype specifiers:
|
||
|
||
>>> jnp.result_type('int32', 'float32')
|
||
dtype('float32')
|
||
>>> jnp.result_type(np.uint16, np.dtype('int32'))
|
||
dtype('int32')
|
||
|
||
Inputs may also be scalars or arrays:
|
||
|
||
>>> jnp.result_type(1.0, jnp.bfloat16(2))
|
||
dtype(bfloat16)
|
||
>>> jnp.result_type(jnp.arange(4), jnp.zeros(4))
|
||
dtype('float32')
|
||
|
||
Be aware that the result type will be canonicalized based on the state
|
||
of the ``jax_enable_x64`` configuration flag, meaning that 64-bit types
|
||
may be downcast to 32-bit:
|
||
|
||
>>> jnp.result_type('float64')
|
||
dtype('float32')
|
||
|
||
For details on 64-bit values, refer to `Sharp bits - double precision`_:
|
||
|
||
.. _Sharp bits - double precision: https://jax.readthedocs.io/en/latest/notebooks/Common_Gotchas_in_JAX.html#double-64bit-precision
|
||
"""
|
||
return dtypes.result_type(*args)
|
||
|
||
|
||
@export
|
||
@jit
|
||
def trunc(x: ArrayLike) -> Array:
|
||
"""Round input to the nearest integer towards zero.
|
||
|
||
JAX implementation of :func:`numpy.trunc`.
|
||
|
||
Args:
|
||
x: input array or scalar.
|
||
|
||
Returns:
|
||
An array with same shape and dtype as ``x`` containing the rounded values.
|
||
|
||
See also:
|
||
- :func:`jax.numpy.fix`: Rounds the input to the nearest integer towards zero.
|
||
- :func:`jax.numpy.ceil`: Rounds the input up to the nearest integer.
|
||
- :func:`jax.numpy.floor`: Rounds the input down to the nearest integer.
|
||
|
||
Examples:
|
||
>>> key = jax.random.key(42)
|
||
>>> x = jax.random.uniform(key, (3, 3), minval=-10, maxval=10)
|
||
>>> with jnp.printoptions(precision=2, suppress=True):
|
||
... print(x)
|
||
[[-0.23 3.6 2.33]
|
||
[ 1.22 -0.99 1.72]
|
||
[-8.5 5.5 3.98]]
|
||
>>> jnp.trunc(x)
|
||
Array([[-0., 3., 2.],
|
||
[ 1., -0., 1.],
|
||
[-8., 5., 3.]], dtype=float32)
|
||
"""
|
||
x = util.ensure_arraylike('trunc', x)
|
||
if dtypes.isdtype(dtypes.dtype(x), ('integral', 'bool')):
|
||
return x
|
||
return where(lax.lt(x, _lax_const(x, 0)), ufuncs.ceil(x), ufuncs.floor(x))
|
||
|
||
|
||
@partial(jit, static_argnames=['mode', 'op', 'precision', 'preferred_element_type'])
|
||
def _conv(x: Array, y: Array, mode: str, op: str, precision: PrecisionLike,
|
||
preferred_element_type: DTypeLike | None = None) -> Array:
|
||
if np.ndim(x) != 1 or np.ndim(y) != 1:
|
||
raise ValueError(f"{op}() only support 1-dimensional inputs.")
|
||
if preferred_element_type is None:
|
||
# if unspecified, promote to inexact following NumPy's default for convolutions.
|
||
x, y = util.promote_dtypes_inexact(x, y)
|
||
else:
|
||
# otherwise cast to same type but otherwise preserve input dtypes
|
||
x, y = util.promote_dtypes(x, y)
|
||
if len(x) == 0 or len(y) == 0:
|
||
raise ValueError(f"{op}: inputs cannot be empty, got shapes {x.shape} and {y.shape}.")
|
||
|
||
out_order = slice(None)
|
||
if op == 'correlate':
|
||
y = ufuncs.conj(y)
|
||
if len(x) < len(y):
|
||
x, y = y, x
|
||
out_order = slice(None, None, -1)
|
||
elif op == 'convolve':
|
||
if len(x) < len(y):
|
||
x, y = y, x
|
||
y = flip(y)
|
||
|
||
if mode == 'valid':
|
||
padding = [(0, 0)]
|
||
elif mode == 'same':
|
||
padding = [(y.shape[0] // 2, y.shape[0] - y.shape[0] // 2 - 1)]
|
||
elif mode == 'full':
|
||
padding = [(y.shape[0] - 1, y.shape[0] - 1)]
|
||
else:
|
||
raise ValueError("mode must be one of ['full', 'same', 'valid']")
|
||
|
||
result = lax.conv_general_dilated(x[None, None, :], y[None, None, :], (1,),
|
||
padding, precision=precision,
|
||
preferred_element_type=preferred_element_type)
|
||
return result[0, 0, out_order]
|
||
|
||
|
||
@export
|
||
@partial(jit, static_argnames=('mode', 'precision', 'preferred_element_type'))
|
||
def convolve(a: ArrayLike, v: ArrayLike, mode: str = 'full', *,
|
||
precision: PrecisionLike = None,
|
||
preferred_element_type: DTypeLike | None = None) -> Array:
|
||
r"""Convolution of two one dimensional arrays.
|
||
|
||
JAX implementation of :func:`numpy.convolve`.
|
||
|
||
Convolution of one dimensional arrays is defined as:
|
||
|
||
.. math::
|
||
|
||
c_k = \sum_j a_{k - j} v_j
|
||
|
||
Args:
|
||
a: left-hand input to the convolution. Must have ``a.ndim == 1``.
|
||
v: right-hand input to the convolution. Must have ``v.ndim == 1``.
|
||
mode: controls the size of the output. Available operations are:
|
||
|
||
* ``"full"``: (default) output the full convolution of the inputs.
|
||
* ``"same"``: return a centered portion of the ``"full"`` output which
|
||
is the same size as ``a``.
|
||
* ``"valid"``: return the portion of the ``"full"`` output which do not
|
||
depend on padding at the array edges.
|
||
|
||
precision: Specify the precision of the computation. Refer to
|
||
:class:`jax.lax.Precision` for a description of available values.
|
||
|
||
preferred_element_type: A datatype, indicating to accumulate results to and
|
||
return a result with that datatype. Default is ``None``, which means the
|
||
default accumulation type for the input types.
|
||
|
||
Returns:
|
||
Array containing the convolved result.
|
||
|
||
See Also:
|
||
- :func:`jax.scipy.signal.convolve`: ND convolution
|
||
- :func:`jax.numpy.correlate`: 1D correlation
|
||
|
||
Examples:
|
||
A few 1D convolution examples:
|
||
|
||
>>> x = jnp.array([1, 2, 3, 2, 1])
|
||
>>> y = jnp.array([4, 1, 2])
|
||
|
||
``jax.numpy.convolve``, by default, returns full convolution using implicit
|
||
zero-padding at the edges:
|
||
|
||
>>> jnp.convolve(x, y)
|
||
Array([ 4., 9., 16., 15., 12., 5., 2.], dtype=float32)
|
||
|
||
Specifying ``mode = 'same'`` returns a centered convolution the same size
|
||
as the first input:
|
||
|
||
>>> jnp.convolve(x, y, mode='same')
|
||
Array([ 9., 16., 15., 12., 5.], dtype=float32)
|
||
|
||
Specifying ``mode = 'valid'`` returns only the portion where the two arrays
|
||
fully overlap:
|
||
|
||
>>> jnp.convolve(x, y, mode='valid')
|
||
Array([16., 15., 12.], dtype=float32)
|
||
|
||
For complex-valued inputs:
|
||
|
||
>>> x1 = jnp.array([3+1j, 2, 4-3j])
|
||
>>> y1 = jnp.array([1, 2-3j, 4+5j])
|
||
>>> jnp.convolve(x1, y1)
|
||
Array([ 3. +1.j, 11. -7.j, 15.+10.j, 7. -8.j, 31. +8.j], dtype=complex64)
|
||
"""
|
||
a, v = util.ensure_arraylike("convolve", a, v)
|
||
return _conv(a, v, mode=mode, op='convolve',
|
||
precision=precision, preferred_element_type=preferred_element_type)
|
||
|
||
|
||
@export
|
||
@partial(jit, static_argnames=('mode', 'precision', 'preferred_element_type'))
|
||
def correlate(a: ArrayLike, v: ArrayLike, mode: str = 'valid', *,
|
||
precision: PrecisionLike = None,
|
||
preferred_element_type: DTypeLike | None = None) -> Array:
|
||
r"""Correlation of two one dimensional arrays.
|
||
|
||
JAX implementation of :func:`numpy.correlate`.
|
||
|
||
Correlation of one dimensional arrays is defined as:
|
||
|
||
.. math::
|
||
|
||
c_k = \sum_j a_{k + j} \overline{v_j}
|
||
|
||
where :math:`\overline{v_j}` is the complex conjugate of :math:`v_j`.
|
||
|
||
Args:
|
||
a: left-hand input to the correlation. Must have ``a.ndim == 1``.
|
||
v: right-hand input to the correlation. Must have ``v.ndim == 1``.
|
||
mode: controls the size of the output. Available operations are:
|
||
|
||
* ``"full"``: output the full correlation of the inputs.
|
||
* ``"same"``: return a centered portion of the ``"full"`` output which
|
||
is the same size as ``a``.
|
||
* ``"valid"``: (default) return the portion of the ``"full"`` output which do not
|
||
depend on padding at the array edges.
|
||
|
||
precision: Specify the precision of the computation. Refer to
|
||
:class:`jax.lax.Precision` for a description of available values.
|
||
|
||
preferred_element_type: A datatype, indicating to accumulate results to and
|
||
return a result with that datatype. Default is ``None``, which means the
|
||
default accumulation type for the input types.
|
||
|
||
Returns:
|
||
Array containing the cross-correlation result.
|
||
|
||
See Also:
|
||
- :func:`jax.scipy.signal.correlate`: ND correlation
|
||
- :func:`jax.numpy.convolve`: 1D convolution
|
||
|
||
Examples:
|
||
>>> x = jnp.array([1, 2, 3, 2, 1])
|
||
>>> y = jnp.array([4, 5, 6])
|
||
|
||
Since default ``mode = 'valid'``, ``jax.numpy.correlate`` returns only the
|
||
portion of correlation where the two arrays fully overlap:
|
||
|
||
>>> jnp.correlate(x, y)
|
||
Array([32., 35., 28.], dtype=float32)
|
||
|
||
Specifying ``mode = 'full'`` returns full correlation using implicit
|
||
zero-padding at the edges.
|
||
|
||
>>> jnp.correlate(x, y, mode='full')
|
||
Array([ 6., 17., 32., 35., 28., 13., 4.], dtype=float32)
|
||
|
||
Specifying ``mode = 'same'`` returns a centered correlation the same size
|
||
as the first input:
|
||
|
||
>>> jnp.correlate(x, y, mode='same')
|
||
Array([17., 32., 35., 28., 13.], dtype=float32)
|
||
|
||
If both the inputs arrays are real-valued and symmetric then the result will
|
||
also be symmetric and will be equal to the result of ``jax.numpy.convolve``.
|
||
|
||
>>> x1 = jnp.array([1, 2, 3, 2, 1])
|
||
>>> y1 = jnp.array([4, 5, 4])
|
||
>>> jnp.correlate(x1, y1, mode='full')
|
||
Array([ 4., 13., 26., 31., 26., 13., 4.], dtype=float32)
|
||
>>> jnp.convolve(x1, y1, mode='full')
|
||
Array([ 4., 13., 26., 31., 26., 13., 4.], dtype=float32)
|
||
|
||
For complex-valued inputs:
|
||
|
||
>>> x2 = jnp.array([3+1j, 2, 2-3j])
|
||
>>> y2 = jnp.array([4, 2-5j, 1])
|
||
>>> jnp.correlate(x2, y2, mode='full')
|
||
Array([ 3. +1.j, 3.+17.j, 18.+11.j, 27. +4.j, 8.-12.j], dtype=complex64)
|
||
"""
|
||
a, v = util.ensure_arraylike("correlate", a, v)
|
||
return _conv(a, v, mode=mode, op='correlate',
|
||
precision=precision, preferred_element_type=preferred_element_type)
|
||
|
||
|
||
@export
|
||
def histogram_bin_edges(a: ArrayLike, bins: ArrayLike = 10,
|
||
range: None | Array | Sequence[ArrayLike] = None,
|
||
weights: ArrayLike | None = None) -> Array:
|
||
"""Compute the bin edges for a histogram.
|
||
|
||
JAX implementation of :func:`numpy.histogram_bin_edges`.
|
||
|
||
Args:
|
||
a: array of values to be binned
|
||
bins: Specify the number of bins in the histogram (default: 10).
|
||
range: tuple of scalars. Specifies the range of the data. If not specified,
|
||
the range is inferred from the data.
|
||
weights: unused by JAX.
|
||
|
||
Returns:
|
||
An array of bin edges for the histogram.
|
||
|
||
See also:
|
||
- :func:`jax.numpy.histogram`: compute a 1D histogram.
|
||
- :func:`jax.numpy.histogram2d`: compute a 2D histogram.
|
||
- :func:`jax.numpy.histogramdd`: compute an N-dimensional histogram.
|
||
|
||
Examples:
|
||
>>> a = jnp.array([2, 5, 3, 6, 4, 1])
|
||
>>> jnp.histogram_bin_edges(a, bins=5)
|
||
Array([1., 2., 3., 4., 5., 6.], dtype=float32)
|
||
>>> jnp.histogram_bin_edges(a, bins=5, range=(-10, 10)) # doctest: +SKIP
|
||
Array([-10., -6., -2., 2., 6., 10.], dtype=float32)
|
||
"""
|
||
del weights # unused, because string bins is not supported.
|
||
if isinstance(bins, str):
|
||
raise NotImplementedError("string values for `bins` not implemented.")
|
||
util.check_arraylike("histogram_bin_edges", a, bins)
|
||
arr = asarray(a)
|
||
dtype = dtypes.to_inexact_dtype(arr.dtype)
|
||
if np.ndim(bins) == 1:
|
||
return asarray(bins, dtype=dtype)
|
||
|
||
bins_int = core.concrete_or_error(operator.index, bins,
|
||
"bins argument of histogram_bin_edges")
|
||
if range is None:
|
||
range = [arr.min(), arr.max()]
|
||
range = asarray(range, dtype=dtype)
|
||
if np.shape(range) != (2,):
|
||
raise ValueError(f"`range` must be either None or a sequence of scalars, got {range}")
|
||
range = (where(reductions.ptp(range) == 0, range[0] - 0.5, range[0]),
|
||
where(reductions.ptp(range) == 0, range[1] + 0.5, range[1]))
|
||
assert range is not None
|
||
return linspace(range[0], range[1], bins_int + 1, dtype=dtype)
|
||
|
||
|
||
@export
|
||
def histogram(a: ArrayLike, bins: ArrayLike = 10,
|
||
range: Sequence[ArrayLike] | None = None,
|
||
weights: ArrayLike | None = None,
|
||
density: bool | None = None) -> tuple[Array, Array]:
|
||
"""Compute a 1-dimensional histogram.
|
||
|
||
JAX implementation of :func:`numpy.histogram`.
|
||
|
||
Args:
|
||
a: array of values to be binned. May be any size or dimension.
|
||
bins: Specify the number of bins in the histogram (default: 10). ``bins``
|
||
may also be an array specifying the locations of the bin edges.
|
||
range: tuple of scalars. Specifies the range of the data. If not specified,
|
||
the range is inferred from the data.
|
||
weights: An optional array specifying the weights of the data points.
|
||
Should be broadcast-compatible with ``a``. If not specified, each
|
||
data point is weighted equally.
|
||
density: If True, return the normalized histogram in units of counts
|
||
per unit length. If False (default) return the (weighted) counts per bin.
|
||
|
||
Returns:
|
||
A tuple of arrays ``(histogram, bin_edges)``, where ``histogram`` contains
|
||
the aggregated data, and ``bin_edges`` specifies the boundaries of the bins.
|
||
|
||
See Also:
|
||
- :func:`jax.numpy.bincount`: Count the number of occurrences of each value in an array.
|
||
- :func:`jax.numpy.histogram2d`: Compute the histogram of a 2D array.
|
||
- :func:`jax.numpy.histogramdd`: Compute the histogram of an N-dimensional array.
|
||
- :func:`jax.numpy.histogram_bin_edges`: Compute the bin edges for a histogram.
|
||
|
||
Examples:
|
||
>>> a = jnp.array([1, 2, 3, 10, 11, 15, 19, 25])
|
||
>>> counts, bin_edges = jnp.histogram(a, bins=8)
|
||
>>> print(counts)
|
||
[3. 0. 0. 2. 1. 0. 1. 1.]
|
||
>>> print(bin_edges)
|
||
[ 1. 4. 7. 10. 13. 16. 19. 22. 25.]
|
||
|
||
Specifying the bin range:
|
||
|
||
>>> counts, bin_edges = jnp.histogram(a, range=(0, 25), bins=5)
|
||
>>> print(counts)
|
||
[3. 0. 2. 2. 1.]
|
||
>>> print(bin_edges)
|
||
[ 0. 5. 10. 15. 20. 25.]
|
||
|
||
Specifying the bin edges explicitly:
|
||
|
||
>>> bin_edges = jnp.array([0, 10, 20, 30])
|
||
>>> counts, _ = jnp.histogram(a, bins=bin_edges)
|
||
>>> print(counts)
|
||
[3. 4. 1.]
|
||
|
||
Using ``density=True`` returns a normalized histogram:
|
||
|
||
>>> density, bin_edges = jnp.histogram(a, density=True)
|
||
>>> dx = jnp.diff(bin_edges)
|
||
>>> normed_sum = jnp.sum(density * dx)
|
||
>>> jnp.allclose(normed_sum, 1.0)
|
||
Array(True, dtype=bool)
|
||
"""
|
||
if weights is None:
|
||
util.check_arraylike("histogram", a, bins)
|
||
a, = util.promote_dtypes_inexact(a)
|
||
weights = ones_like(a)
|
||
else:
|
||
util.check_arraylike("histogram", a, bins, weights)
|
||
if np.shape(a) != np.shape(weights):
|
||
raise ValueError("weights should have the same shape as a.")
|
||
a, weights = util.promote_dtypes_inexact(a, weights)
|
||
|
||
bin_edges = histogram_bin_edges(a, bins, range, weights)
|
||
bin_idx = searchsorted(bin_edges, a, side='right')
|
||
bin_idx = where(a == bin_edges[-1], len(bin_edges) - 1, bin_idx)
|
||
counts = zeros(len(bin_edges), weights.dtype).at[bin_idx].add(weights)[1:]
|
||
if density:
|
||
bin_widths = diff(bin_edges)
|
||
counts = counts / bin_widths / counts.sum()
|
||
return counts, bin_edges
|
||
|
||
|
||
@export
|
||
def histogram2d(x: ArrayLike, y: ArrayLike, bins: ArrayLike | list[ArrayLike] = 10,
|
||
range: Sequence[None | Array | Sequence[ArrayLike]] | None = None,
|
||
weights: ArrayLike | None = None,
|
||
density: bool | None = None) -> tuple[Array, Array, Array]:
|
||
"""Compute a 2-dimensional histogram.
|
||
|
||
JAX implementation of :func:`numpy.histogram2d`.
|
||
|
||
Args:
|
||
x: one-dimensional array of x-values for points to be binned.
|
||
y: one-dimensional array of y-values for points to be binned.
|
||
bins: Specify the number of bins in the histogram (default: 10). ``bins``
|
||
may also be an array specifying the locations of the bin edges, or a pair
|
||
of integers or pair of arrays specifying the number of bins in each
|
||
dimension.
|
||
range: Pair of arrays or lists of the form ``[[xmin, xmax], [ymin, ymax]]``
|
||
specifying the range of the data in each dimension. If not specified, the
|
||
range is inferred from the data.
|
||
weights: An optional array specifying the weights of the data points.
|
||
Should be the same shape as ``x`` and ``y``. If not specified, each
|
||
data point is weighted equally.
|
||
density: If True, return the normalized histogram in units of counts
|
||
per unit area. If False (default) return the (weighted) counts per bin.
|
||
|
||
Returns:
|
||
A tuple of arrays ``(histogram, x_edges, y_edges)``, where ``histogram``
|
||
contains the aggregated data, and ``x_edges`` and ``y_edges`` specify the
|
||
boundaries of the bins.
|
||
|
||
See Also:
|
||
- :func:`jax.numpy.histogram`: Compute the histogram of a 1D array.
|
||
- :func:`jax.numpy.histogramdd`: Compute the histogram of an N-dimensional array.
|
||
- :func:`jax.numpy.histogram_bin_edges`: Compute the bin edges for a histogram.
|
||
|
||
Examples:
|
||
>>> x = jnp.array([1, 2, 3, 10, 11, 15, 19, 25])
|
||
>>> y = jnp.array([2, 5, 6, 8, 13, 16, 17, 18])
|
||
>>> counts, x_edges, y_edges = jnp.histogram2d(x, y, bins=8)
|
||
>>> counts.shape
|
||
(8, 8)
|
||
>>> x_edges
|
||
Array([ 1., 4., 7., 10., 13., 16., 19., 22., 25.], dtype=float32)
|
||
>>> y_edges
|
||
Array([ 2., 4., 6., 8., 10., 12., 14., 16., 18.], dtype=float32)
|
||
|
||
Specifying the bin range:
|
||
|
||
>>> counts, x_edges, y_edges = jnp.histogram2d(x, y, range=[(0, 25), (0, 25)], bins=5)
|
||
>>> counts.shape
|
||
(5, 5)
|
||
>>> x_edges
|
||
Array([ 0., 5., 10., 15., 20., 25.], dtype=float32)
|
||
>>> y_edges
|
||
Array([ 0., 5., 10., 15., 20., 25.], dtype=float32)
|
||
|
||
Specifying the bin edges explicitly:
|
||
|
||
>>> x_edges = jnp.array([0, 10, 20, 30])
|
||
>>> y_edges = jnp.array([0, 10, 20, 30])
|
||
>>> counts, _, _ = jnp.histogram2d(x, y, bins=[x_edges, y_edges])
|
||
>>> counts
|
||
Array([[3, 0, 0],
|
||
[1, 3, 0],
|
||
[0, 1, 0]], dtype=int32)
|
||
|
||
Using ``density=True`` returns a normalized histogram:
|
||
|
||
>>> density, x_edges, y_edges = jnp.histogram2d(x, y, density=True)
|
||
>>> dx = jnp.diff(x_edges)
|
||
>>> dy = jnp.diff(y_edges)
|
||
>>> normed_sum = jnp.sum(density * dx[:, None] * dy[None, :])
|
||
>>> jnp.allclose(normed_sum, 1.0)
|
||
Array(True, dtype=bool)
|
||
"""
|
||
util.check_arraylike("histogram2d", x, y)
|
||
try:
|
||
N = len(bins) # type: ignore[arg-type]
|
||
except TypeError:
|
||
N = 1
|
||
|
||
if N != 1 and N != 2:
|
||
x_edges = y_edges = asarray(bins)
|
||
bins = [x_edges, y_edges]
|
||
|
||
sample = transpose(asarray([x, y]))
|
||
hist, edges = histogramdd(sample, bins, range, weights, density)
|
||
return hist, edges[0], edges[1]
|
||
|
||
|
||
@export
|
||
def histogramdd(sample: ArrayLike, bins: ArrayLike | list[ArrayLike] = 10,
|
||
range: Sequence[None | Array | Sequence[ArrayLike]] | None = None,
|
||
weights: ArrayLike | None = None,
|
||
density: bool | None = None) -> tuple[Array, list[Array]]:
|
||
"""Compute an N-dimensional histogram.
|
||
|
||
JAX implementation of :func:`numpy.histogramdd`.
|
||
|
||
Args:
|
||
sample: input array of shape ``(N, D)`` representing ``N`` points in
|
||
``D`` dimensions.
|
||
bins: Specify the number of bins in each dimension of the histogram.
|
||
(default: 10). May also be a length-D sequence of integers or arrays
|
||
of bin edges.
|
||
range: Length-D sequence of pairs specifying the range for each dimension.
|
||
If not specified, the range is inferred from the data.
|
||
weights: An optional shape ``(N,)`` array specifying the weights of the
|
||
data points.
|
||
Should be the same shape as ``sample``. If not specified, each
|
||
data point is weighted equally.
|
||
density: If True, return the normalized histogram in units of counts
|
||
per unit volume. If False (default) return the (weighted) counts per bin.
|
||
|
||
Returns:
|
||
A tuple of arrays ``(histogram, bin_edges)``, where ``histogram`` contains
|
||
the aggregated data, and ``bin_edges`` specifies the boundaries of the bins.
|
||
|
||
See Also:
|
||
- :func:`jax.numpy.histogram`: Compute the histogram of a 1D array.
|
||
- :func:`jax.numpy.histogram2d`: Compute the histogram of a 2D array.
|
||
- :func:`jax.numpy.histogram_bin_edges`: Compute the bin edges for a histogram.
|
||
|
||
Examples:
|
||
A histogram over 100 points in three dimensions
|
||
|
||
>>> key = jax.random.key(42)
|
||
>>> a = jax.random.normal(key, (100, 3))
|
||
>>> counts, bin_edges = jnp.histogramdd(a, bins=6,
|
||
... range=[(-3, 3), (-3, 3), (-3, 3)])
|
||
>>> counts.shape
|
||
(6, 6, 6)
|
||
>>> bin_edges # doctest: +SKIP
|
||
[Array([-3., -2., -1., 0., 1., 2., 3.], dtype=float32),
|
||
Array([-3., -2., -1., 0., 1., 2., 3.], dtype=float32),
|
||
Array([-3., -2., -1., 0., 1., 2., 3.], dtype=float32)]
|
||
|
||
Using ``density=True`` returns a normalized histogram:
|
||
|
||
>>> density, bin_edges = jnp.histogramdd(a, density=True)
|
||
>>> bin_widths = map(jnp.diff, bin_edges)
|
||
>>> dx, dy, dz = jnp.meshgrid(*bin_widths, indexing='ij')
|
||
>>> normed = jnp.sum(density * dx * dy * dz)
|
||
>>> jnp.allclose(normed, 1.0)
|
||
Array(True, dtype=bool)
|
||
"""
|
||
if weights is None:
|
||
util.check_arraylike("histogramdd", sample)
|
||
sample, = util.promote_dtypes_inexact(sample)
|
||
else:
|
||
util.check_arraylike("histogramdd", sample, weights)
|
||
if np.shape(weights) != np.shape(sample)[:1]:
|
||
raise ValueError("should have one weight for each sample.")
|
||
sample, weights = util.promote_dtypes_inexact(sample, weights)
|
||
N, D = np.shape(sample)
|
||
|
||
if range is not None and (
|
||
len(range) != D or any(r is not None and np.shape(r)[0] != 2 for r in range)): # type: ignore[arg-type]
|
||
raise ValueError(f"For sample.shape={(N, D)}, range must be a sequence "
|
||
f"of {D} pairs or Nones; got {range=}")
|
||
|
||
try:
|
||
num_bins = len(bins) # type: ignore[arg-type]
|
||
except TypeError:
|
||
# when bin_size is integer, the same bin is used for each dimension
|
||
bins_per_dimension: list[ArrayLike] = D * [bins] # type: ignore[assignment]
|
||
else:
|
||
if num_bins != D:
|
||
raise ValueError("should be a bin for each dimension.")
|
||
bins_per_dimension = list(bins) # type: ignore[arg-type]
|
||
|
||
bin_idx_by_dim: list[Array] = []
|
||
bin_edges_by_dim: list[Array] = []
|
||
|
||
for i in builtins.range(D):
|
||
range_i = None if range is None else range[i]
|
||
bin_edges = histogram_bin_edges(sample[:, i], bins_per_dimension[i], range_i, weights)
|
||
bin_idx = searchsorted(bin_edges, sample[:, i], side='right')
|
||
bin_idx = where(sample[:, i] == bin_edges[-1], bin_idx - 1, bin_idx)
|
||
bin_idx_by_dim.append(bin_idx)
|
||
bin_edges_by_dim.append(bin_edges)
|
||
|
||
nbins = tuple(len(bin_edges) + 1 for bin_edges in bin_edges_by_dim)
|
||
dedges = [diff(bin_edges) for bin_edges in bin_edges_by_dim]
|
||
|
||
xy = ravel_multi_index(tuple(bin_idx_by_dim), nbins, mode='clip')
|
||
hist = bincount(xy, weights, length=math.prod(nbins))
|
||
hist = reshape(hist, nbins)
|
||
core = D*(slice(1, -1),)
|
||
hist = hist[core]
|
||
|
||
if density:
|
||
hist = hist.astype(sample.dtype)
|
||
hist /= hist.sum()
|
||
for norm in ix_(*dedges):
|
||
hist /= norm
|
||
|
||
return hist, bin_edges_by_dim
|
||
|
||
|
||
@export
|
||
def transpose(a: ArrayLike, axes: Sequence[int] | None = None) -> Array:
|
||
"""Return a transposed version of an N-dimensional array.
|
||
|
||
JAX implementation of :func:`numpy.transpose`, implemented in terms of
|
||
:func:`jax.lax.transpose`.
|
||
|
||
Args:
|
||
a: input array
|
||
axes: optionally specify the permutation using a length-`a.ndim` sequence of integers
|
||
``i`` satisfying ``0 <= i < a.ndim``. Defaults to ``range(a.ndim)[::-1]``, i.e.
|
||
reverses the order of all axes.
|
||
|
||
Returns:
|
||
transposed copy of the array.
|
||
|
||
See Also:
|
||
- :func:`jax.Array.transpose`: equivalent function via an :class:`~jax.Array` method.
|
||
- :attr:`jax.Array.T`: equivalent function via an :class:`~jax.Array` property.
|
||
- :func:`jax.numpy.matrix_transpose`: transpose the last two axes of an array. This is
|
||
suitable for working with batched 2D matrices.
|
||
- :func:`jax.numpy.swapaxes`: swap any two axes in an array.
|
||
- :func:`jax.numpy.moveaxis`: move an axis to another position in the array.
|
||
|
||
Note:
|
||
Unlike :func:`numpy.transpose`, :func:`jax.numpy.transpose` will return a copy rather
|
||
than a view of the input array. However, under JIT, the compiler will optimize-away
|
||
such copies when possible, so this doesn't have performance impacts in practice.
|
||
|
||
Examples:
|
||
For a 1D array, the transpose is the identity:
|
||
|
||
>>> x = jnp.array([1, 2, 3, 4])
|
||
>>> jnp.transpose(x)
|
||
Array([1, 2, 3, 4], dtype=int32)
|
||
|
||
For a 2D array, the transpose is a matrix transpose:
|
||
|
||
>>> x = jnp.array([[1, 2],
|
||
... [3, 4]])
|
||
>>> jnp.transpose(x)
|
||
Array([[1, 3],
|
||
[2, 4]], dtype=int32)
|
||
|
||
For an N-dimensional array, the transpose reverses the order of the axes:
|
||
|
||
>>> x = jnp.zeros(shape=(3, 4, 5))
|
||
>>> jnp.transpose(x).shape
|
||
(5, 4, 3)
|
||
|
||
The ``axes`` argument can be specified to change this default behavior:
|
||
|
||
>>> jnp.transpose(x, (0, 2, 1)).shape
|
||
(3, 5, 4)
|
||
|
||
Since swapping the last two axes is a common operation, it can be done
|
||
via its own API, :func:`jax.numpy.matrix_transpose`:
|
||
|
||
>>> jnp.matrix_transpose(x).shape
|
||
(3, 5, 4)
|
||
|
||
For convenience, transposes may also be performed using the :meth:`jax.Array.transpose`
|
||
method or the :attr:`jax.Array.T` property:
|
||
|
||
>>> x = jnp.array([[1, 2],
|
||
... [3, 4]])
|
||
>>> x.transpose()
|
||
Array([[1, 3],
|
||
[2, 4]], dtype=int32)
|
||
>>> x.T
|
||
Array([[1, 3],
|
||
[2, 4]], dtype=int32)
|
||
"""
|
||
util.check_arraylike("transpose", a)
|
||
axes_ = list(range(np.ndim(a))[::-1]) if axes is None else axes
|
||
axes_ = [_canonicalize_axis(i, np.ndim(a)) for i in axes_]
|
||
return lax.transpose(a, axes_)
|
||
|
||
|
||
@export
|
||
def permute_dims(a: ArrayLike, /, axes: tuple[int, ...]) -> Array:
|
||
"""Permute the axes/dimensions of an array.
|
||
|
||
JAX implementation of :func:`array_api.permute_dims`.
|
||
|
||
Args:
|
||
a: input array
|
||
axes: tuple of integers in range ``[0, a.ndim)`` specifying the
|
||
axes permutation.
|
||
|
||
Returns:
|
||
a copy of ``a`` with axes permuted.
|
||
|
||
See also:
|
||
- :func:`jax.numpy.transpose`
|
||
- :func:`jax.numpy.matrix_transpose`
|
||
|
||
Examples:
|
||
>>> a = jnp.array([[1, 2, 3],
|
||
... [4, 5, 6]])
|
||
>>> jnp.permute_dims(a, (1, 0))
|
||
Array([[1, 4],
|
||
[2, 5],
|
||
[3, 6]], dtype=int32)
|
||
"""
|
||
util.check_arraylike("permute_dims", a)
|
||
return lax.transpose(a, axes)
|
||
|
||
|
||
@export
|
||
def matrix_transpose(x: ArrayLike, /) -> Array:
|
||
"""Transpose the last two dimensions of an array.
|
||
|
||
JAX implementation of :func:`numpy.matrix_transpose`, implemented in terms of
|
||
:func:`jax.lax.transpose`.
|
||
|
||
Args:
|
||
x: input array, Must have ``x.ndim >= 2``
|
||
|
||
Returns:
|
||
matrix-transposed copy of the array.
|
||
|
||
See Also:
|
||
- :attr:`jax.Array.mT`: same operation accessed via an :func:`~jax.Array` property.
|
||
- :func:`jax.numpy.transpose`: general multi-axis transpose
|
||
|
||
Note:
|
||
Unlike :func:`numpy.matrix_transpose`, :func:`jax.numpy.matrix_transpose` will return a
|
||
copy rather than a view of the input array. However, under JIT, the compiler will
|
||
optimize-away such copies when possible, so this doesn't have performance impacts in practice.
|
||
|
||
Examples:
|
||
Here is a 2x2x2 matrix representing a batched 2x2 matrix:
|
||
|
||
>>> x = jnp.array([[[1, 2],
|
||
... [3, 4]],
|
||
... [[5, 6],
|
||
... [7, 8]]])
|
||
>>> jnp.matrix_transpose(x)
|
||
Array([[[1, 3],
|
||
[2, 4]],
|
||
<BLANKLINE>
|
||
[[5, 7],
|
||
[6, 8]]], dtype=int32)
|
||
|
||
For convenience, you can perform the same transpose via the :attr:`~jax.Array.mT`
|
||
property of :class:`jax.Array`:
|
||
|
||
>>> x.mT
|
||
Array([[[1, 3],
|
||
[2, 4]],
|
||
<BLANKLINE>
|
||
[[5, 7],
|
||
[6, 8]]], dtype=int32)
|
||
"""
|
||
util.check_arraylike("matrix_transpose", x)
|
||
ndim = np.ndim(x)
|
||
if ndim < 2:
|
||
raise ValueError(f"x must be at least two-dimensional for matrix_transpose; got {ndim=}")
|
||
axes = (*range(ndim - 2), ndim - 1, ndim - 2)
|
||
return lax.transpose(x, axes)
|
||
|
||
|
||
@export
|
||
@partial(jit, static_argnames=('k', 'axes'))
|
||
def rot90(m: ArrayLike, k: int = 1, axes: tuple[int, int] = (0, 1)) -> Array:
|
||
"""Rotate an array by 90 degrees counterclockwise in the plane specified by axes.
|
||
|
||
JAX implementation of :func:`numpy.rot90`.
|
||
|
||
Args:
|
||
m: input array. Must have ``m.ndim >= 2``.
|
||
k: int, optional, default=1. Specifies the number of times the array is rotated.
|
||
For negative values of ``k``, the array is rotated in clockwise direction.
|
||
axes: tuple of 2 integers, optional, default= (0, 1). The axes define the plane
|
||
in which the array is rotated. Both the axes must be different.
|
||
|
||
Returns:
|
||
An array containing the copy of the input, ``m`` rotated by 90 degrees.
|
||
|
||
See also:
|
||
- :func:`jax.numpy.flip`: reverse the order along the given axis
|
||
- :func:`jax.numpy.fliplr`: reverse the order along axis 1 (left/right)
|
||
- :func:`jax.numpy.flipud`: reverse the order along axis 0 (up/down)
|
||
|
||
Examples:
|
||
>>> m = jnp.array([[1, 2, 3],
|
||
... [4, 5, 6]])
|
||
>>> jnp.rot90(m)
|
||
Array([[3, 6],
|
||
[2, 5],
|
||
[1, 4]], dtype=int32)
|
||
>>> jnp.rot90(m, k=2)
|
||
Array([[6, 5, 4],
|
||
[3, 2, 1]], dtype=int32)
|
||
|
||
``jnp.rot90(m, k=1, axes=(1, 0))`` is equivalent to
|
||
``jnp.rot90(m, k=-1, axes(0,1))``.
|
||
|
||
>>> jnp.rot90(m, axes=(1, 0))
|
||
Array([[4, 1],
|
||
[5, 2],
|
||
[6, 3]], dtype=int32)
|
||
>>> jnp.rot90(m, k=-1, axes=(0, 1))
|
||
Array([[4, 1],
|
||
[5, 2],
|
||
[6, 3]], dtype=int32)
|
||
|
||
when input array has ``ndim>2``:
|
||
|
||
>>> m1 = jnp.array([[[1, 2, 3],
|
||
... [4, 5, 6]],
|
||
... [[7, 8, 9],
|
||
... [10, 11, 12]]])
|
||
>>> jnp.rot90(m1, k=1, axes=(2, 1))
|
||
Array([[[ 4, 1],
|
||
[ 5, 2],
|
||
[ 6, 3]],
|
||
<BLANKLINE>
|
||
[[10, 7],
|
||
[11, 8],
|
||
[12, 9]]], dtype=int32)
|
||
"""
|
||
util.check_arraylike("rot90", m)
|
||
if np.ndim(m) < 2:
|
||
raise ValueError("rot90 requires its first argument to have ndim at least "
|
||
f"two, but got first argument of shape {np.shape(m)}, "
|
||
f"which has ndim {np.ndim(m)}")
|
||
ax1, ax2 = axes
|
||
ax1 = _canonicalize_axis(ax1, np.ndim(m))
|
||
ax2 = _canonicalize_axis(ax2, np.ndim(m))
|
||
if ax1 == ax2:
|
||
raise ValueError("Axes must be different") # same as numpy error
|
||
k = k % 4
|
||
if k == 0:
|
||
return asarray(m)
|
||
elif k == 2:
|
||
return flip(flip(m, ax1), ax2)
|
||
else:
|
||
perm = list(range(np.ndim(m)))
|
||
perm[ax1], perm[ax2] = perm[ax2], perm[ax1]
|
||
if k == 1:
|
||
return transpose(flip(m, ax2), perm)
|
||
else:
|
||
return flip(transpose(m, perm), ax2)
|
||
|
||
|
||
@export
|
||
def flip(m: ArrayLike, axis: int | Sequence[int] | None = None) -> Array:
|
||
"""Reverse the order of elements of an array along the given axis.
|
||
|
||
JAX implementation of :func:`numpy.flip`.
|
||
|
||
Args:
|
||
m: Array.
|
||
axis: integer or sequence of integers. Specifies along which axis or axes
|
||
should the array elements be reversed. Default is ``None``, which flips
|
||
along all axes.
|
||
|
||
Returns:
|
||
An array with the elements in reverse order along ``axis``.
|
||
|
||
See Also:
|
||
- :func:`jax.numpy.fliplr`: reverse the order along axis 1 (left/right)
|
||
- :func:`jax.numpy.flipud`: reverse the order along axis 0 (up/down)
|
||
|
||
Examples:
|
||
>>> x1 = jnp.array([[1, 2],
|
||
... [3, 4]])
|
||
>>> jnp.flip(x1)
|
||
Array([[4, 3],
|
||
[2, 1]], dtype=int32)
|
||
|
||
If ``axis`` is specified with an integer, then ``jax.numpy.flip`` reverses
|
||
the array along that particular axis only.
|
||
|
||
>>> jnp.flip(x1, axis=1)
|
||
Array([[2, 1],
|
||
[4, 3]], dtype=int32)
|
||
|
||
>>> x2 = jnp.arange(1, 9).reshape(2, 2, 2)
|
||
>>> x2
|
||
Array([[[1, 2],
|
||
[3, 4]],
|
||
<BLANKLINE>
|
||
[[5, 6],
|
||
[7, 8]]], dtype=int32)
|
||
>>> jnp.flip(x2)
|
||
Array([[[8, 7],
|
||
[6, 5]],
|
||
<BLANKLINE>
|
||
[[4, 3],
|
||
[2, 1]]], dtype=int32)
|
||
|
||
When ``axis`` is specified with a sequence of integers, then
|
||
``jax.numpy.flip`` reverses the array along the specified axes.
|
||
|
||
>>> jnp.flip(x2, axis=[1, 2])
|
||
Array([[[4, 3],
|
||
[2, 1]],
|
||
<BLANKLINE>
|
||
[[8, 7],
|
||
[6, 5]]], dtype=int32)
|
||
"""
|
||
arr = util.ensure_arraylike("flip", m)
|
||
return _flip(arr, reductions._ensure_optional_axes(axis))
|
||
|
||
@partial(jit, static_argnames=('axis',))
|
||
def _flip(m: Array, axis: int | tuple[int, ...] | None = None) -> Array:
|
||
if axis is None:
|
||
return lax.rev(m, list(range(len(np.shape(m)))))
|
||
axis = _ensure_index_tuple(axis)
|
||
return lax.rev(m, [_canonicalize_axis(ax, np.ndim(m)) for ax in axis])
|
||
|
||
|
||
@export
|
||
def fliplr(m: ArrayLike) -> Array:
|
||
"""Reverse the order of elements of an array along axis 1.
|
||
|
||
JAX implementation of :func:`numpy.fliplr`.
|
||
|
||
Args:
|
||
m: Array with at least two dimensions.
|
||
|
||
Returns:
|
||
An array with the elements in reverse order along axis 1.
|
||
|
||
See Also:
|
||
- :func:`jax.numpy.flip`: reverse the order along the given axis
|
||
- :func:`jax.numpy.flipud`: reverse the order along axis 0
|
||
|
||
Examples:
|
||
>>> x = jnp.array([[1, 2],
|
||
... [3, 4]])
|
||
>>> jnp.fliplr(x)
|
||
Array([[2, 1],
|
||
[4, 3]], dtype=int32)
|
||
"""
|
||
arr = util.ensure_arraylike("fliplr", m)
|
||
return _flip(arr, 1)
|
||
|
||
|
||
@export
|
||
def flipud(m: ArrayLike) -> Array:
|
||
"""Reverse the order of elements of an array along axis 0.
|
||
|
||
JAX implementation of :func:`numpy.flipud`.
|
||
|
||
Args:
|
||
m: Array with at least one dimension.
|
||
|
||
Returns:
|
||
An array with the elements in reverse order along axis 0.
|
||
|
||
See Also:
|
||
- :func:`jax.numpy.flip`: reverse the order along the given axis
|
||
- :func:`jax.numpy.fliplr`: reverse the order along axis 1
|
||
|
||
Examples:
|
||
>>> x = jnp.array([[1, 2],
|
||
... [3, 4]])
|
||
>>> jnp.flipud(x)
|
||
Array([[3, 4],
|
||
[1, 2]], dtype=int32)
|
||
"""
|
||
arr = util.ensure_arraylike("flipud", m)
|
||
return _flip(arr, 0)
|
||
|
||
|
||
@export
|
||
@jit
|
||
def iscomplex(x: ArrayLike) -> Array:
|
||
"""Return boolean array showing where the input is complex.
|
||
|
||
JAX implementation of :func:`numpy.iscomplex`.
|
||
|
||
Args:
|
||
x: Input array to check.
|
||
|
||
Returns:
|
||
A new array containing boolean values indicating complex elements.
|
||
|
||
See Also:
|
||
- :func:`jax.numpy.iscomplexobj`
|
||
- :func:`jax.numpy.isrealobj`
|
||
|
||
Examples:
|
||
>>> jnp.iscomplex(jnp.array([True, 0, 1, 2j, 1+2j]))
|
||
Array([False, False, False, True, True], dtype=bool)
|
||
"""
|
||
i = ufuncs.imag(x)
|
||
return lax.ne(i, _lax_const(i, 0))
|
||
|
||
|
||
@export
|
||
@jit
|
||
def isreal(x: ArrayLike) -> Array:
|
||
"""Return boolean array showing where the input is real.
|
||
|
||
JAX implementation of :func:`numpy.isreal`.
|
||
|
||
Args:
|
||
x: input array to check.
|
||
|
||
Returns:
|
||
A new array containing boolean values indicating real elements.
|
||
|
||
See Also:
|
||
- :func:`jax.numpy.iscomplex`
|
||
- :func:`jax.numpy.isrealobj`
|
||
|
||
Examples:
|
||
>>> jnp.isreal(jnp.array([False, 0j, 1, 2.1, 1+2j]))
|
||
Array([ True, True, True, True, False], dtype=bool)
|
||
"""
|
||
i = ufuncs.imag(x)
|
||
return lax.eq(i, _lax_const(i, 0))
|
||
|
||
|
||
@export
|
||
@partial(jit, static_argnames=['deg'])
|
||
def angle(z: ArrayLike, deg: bool = False) -> Array:
|
||
"""Return the angle of a complex valued number or array.
|
||
|
||
JAX implementation of :func:`numpy.angle`.
|
||
|
||
Args:
|
||
z: A complex number or an array of complex numbers.
|
||
deg: Boolean. If ``True``, returns the result in degrees else returns
|
||
in radians. Default is ``False``.
|
||
|
||
Returns:
|
||
An array of counterclockwise angle of each element of ``z``, with the same
|
||
shape as ``z`` of dtype float.
|
||
|
||
Examples:
|
||
|
||
If ``z`` is a number
|
||
|
||
>>> z1 = 2+3j
|
||
>>> jnp.angle(z1)
|
||
Array(0.98279375, dtype=float32, weak_type=True)
|
||
|
||
If ``z`` is an array
|
||
|
||
>>> z2 = jnp.array([[1+3j, 2-5j],
|
||
... [4-3j, 3+2j]])
|
||
>>> with jnp.printoptions(precision=2, suppress=True):
|
||
... print(jnp.angle(z2))
|
||
[[ 1.25 -1.19]
|
||
[-0.64 0.59]]
|
||
|
||
If ``deg=True``.
|
||
|
||
>>> with jnp.printoptions(precision=2, suppress=True):
|
||
... print(jnp.angle(z2, deg=True))
|
||
[[ 71.57 -68.2 ]
|
||
[-36.87 33.69]]
|
||
"""
|
||
re = ufuncs.real(z)
|
||
im = ufuncs.imag(z)
|
||
dtype = _dtype(re)
|
||
if not issubdtype(dtype, np.inexact) or (
|
||
issubdtype(_dtype(z), np.floating) and np.ndim(z) == 0):
|
||
dtype = dtypes.canonicalize_dtype(dtypes.float_)
|
||
re = lax.convert_element_type(re, dtype)
|
||
im = lax.convert_element_type(im, dtype)
|
||
result = lax.atan2(im, re)
|
||
return ufuncs.degrees(result) if deg else result
|
||
|
||
|
||
@export
|
||
@partial(jit, static_argnames=('n', 'axis'))
|
||
def diff(a: ArrayLike, n: int = 1, axis: int = -1,
|
||
prepend: ArrayLike | None = None,
|
||
append: ArrayLike | None = None) -> Array:
|
||
"""Calculate n-th order difference between array elements along a given axis.
|
||
|
||
JAX implementation of :func:`numpy.diff`.
|
||
|
||
The first order difference is computed by ``a[i+1] - a[i]``, and the n-th order
|
||
difference is computed ``n`` times recursively.
|
||
|
||
Args:
|
||
a: input array. Must have ``a.ndim >= 1``.
|
||
n: int, optional, default=1. Order of the difference. Specifies the number
|
||
of times the difference is computed. If n=0, no difference is computed and
|
||
input is returned as is.
|
||
axis: int, optional, default=-1. Specifies the axis along which the difference
|
||
is computed. The difference is computed along ``axis -1`` by default.
|
||
prepend: scalar or array, optional, default=None. Specifies the values to be
|
||
prepended along ``axis`` before computing the difference.
|
||
append: scalar or array, optional, default=None. Specifies the values to be
|
||
appended along ``axis`` before computing the difference.
|
||
|
||
Returns:
|
||
An array containing the n-th order difference between the elements of ``a``.
|
||
|
||
See also:
|
||
- :func:`jax.numpy.ediff1d`: Computes the differences between consecutive
|
||
elements of an array.
|
||
- :func:`jax.numpy.cumsum`: Computes the cumulative sum of the elements of
|
||
the array along a given axis.
|
||
- :func:`jax.numpy.gradient`: Computes the gradient of an N-dimensional array.
|
||
|
||
Examples:
|
||
``jnp.diff`` computes the first order difference along ``axis``, by default.
|
||
|
||
>>> a = jnp.array([[1, 5, 2, 9],
|
||
... [3, 8, 7, 4]])
|
||
>>> jnp.diff(a)
|
||
Array([[ 4, -3, 7],
|
||
[ 5, -1, -3]], dtype=int32)
|
||
|
||
When ``n = 2``, second order difference is computed along ``axis``.
|
||
|
||
>>> jnp.diff(a, n=2)
|
||
Array([[-7, 10],
|
||
[-6, -2]], dtype=int32)
|
||
|
||
When ``prepend = 2``, it is prepended to ``a`` along ``axis`` before computing
|
||
the difference.
|
||
|
||
>>> jnp.diff(a, prepend=2)
|
||
Array([[-1, 4, -3, 7],
|
||
[ 1, 5, -1, -3]], dtype=int32)
|
||
|
||
When ``append = jnp.array([[3],[1]])``, it is appended to ``a`` along ``axis``
|
||
before computing the difference.
|
||
|
||
>>> jnp.diff(a, append=jnp.array([[3],[1]]))
|
||
Array([[ 4, -3, 7, -6],
|
||
[ 5, -1, -3, -3]], dtype=int32)
|
||
"""
|
||
arr = util.ensure_arraylike("diff", a)
|
||
n = core.concrete_or_error(operator.index, n, "'n' argument of jnp.diff")
|
||
axis = core.concrete_or_error(operator.index, axis, "'axis' argument of jnp.diff")
|
||
if n == 0:
|
||
return arr
|
||
if n < 0:
|
||
raise ValueError(f"order must be non-negative but got {n}")
|
||
if arr.ndim == 0:
|
||
raise ValueError(f"diff requires input that is at least one dimensional; got {a}")
|
||
|
||
nd = arr.ndim
|
||
axis = _canonicalize_axis(axis, nd)
|
||
|
||
combined: list[Array] = []
|
||
if prepend is not None:
|
||
prepend = util.ensure_arraylike("diff", prepend)
|
||
if not np.ndim(prepend):
|
||
shape = list(arr.shape)
|
||
shape[axis] = 1
|
||
prepend = broadcast_to(prepend, tuple(shape))
|
||
combined.append(prepend)
|
||
|
||
combined.append(arr)
|
||
|
||
if append is not None:
|
||
append = util.ensure_arraylike("diff", append)
|
||
if not np.ndim(append):
|
||
shape = list(arr.shape)
|
||
shape[axis] = 1
|
||
append = broadcast_to(append, tuple(shape))
|
||
combined.append(append)
|
||
|
||
if len(combined) > 1:
|
||
arr = concatenate(combined, axis)
|
||
|
||
slice1 = [slice(None)] * nd
|
||
slice2 = [slice(None)] * nd
|
||
slice1[axis] = slice(1, None)
|
||
slice2[axis] = slice(None, -1)
|
||
slice1_tuple = tuple(slice1)
|
||
slice2_tuple = tuple(slice2)
|
||
|
||
op = operator.ne if arr.dtype == np.bool_ else operator.sub
|
||
for _ in range(n):
|
||
arr = op(arr[slice1_tuple], arr[slice2_tuple])
|
||
|
||
return arr
|
||
|
||
|
||
@export
|
||
@jit
|
||
def ediff1d(ary: ArrayLike, to_end: ArrayLike | None = None,
|
||
to_begin: ArrayLike | None = None) -> Array:
|
||
"""Compute the differences of the elements of the flattened array.
|
||
|
||
JAX implementation of :func:`numpy.ediff1d`.
|
||
|
||
Args:
|
||
ary: input array or scalar.
|
||
to_end: scalar or array, optional, default=None. Specifies the numbers to
|
||
append to the resulting array.
|
||
to_begin: scalar or array, optional, default=None. Specifies the numbers to
|
||
prepend to the resulting array.
|
||
|
||
Returns:
|
||
An array containing the differences between the elements of the input array.
|
||
|
||
Note:
|
||
Unlike NumPy's implementation of ediff1d, :py:func:`jax.numpy.ediff1d` will
|
||
not issue an error if casting ``to_end`` or ``to_begin`` to the type of
|
||
``ary`` loses precision.
|
||
|
||
See also:
|
||
- :func:`jax.numpy.diff`: Computes the n-th order difference between elements
|
||
of the array along a given axis.
|
||
- :func:`jax.numpy.cumsum`: Computes the cumulative sum of the elements of
|
||
the array along a given axis.
|
||
- :func:`jax.numpy.gradient`: Computes the gradient of an N-dimensional array.
|
||
|
||
Examples:
|
||
>>> a = jnp.array([2, 3, 5, 9, 1, 4])
|
||
>>> jnp.ediff1d(a)
|
||
Array([ 1, 2, 4, -8, 3], dtype=int32)
|
||
>>> jnp.ediff1d(a, to_begin=-10)
|
||
Array([-10, 1, 2, 4, -8, 3], dtype=int32)
|
||
>>> jnp.ediff1d(a, to_end=jnp.array([20, 30]))
|
||
Array([ 1, 2, 4, -8, 3, 20, 30], dtype=int32)
|
||
>>> jnp.ediff1d(a, to_begin=-10, to_end=jnp.array([20, 30]))
|
||
Array([-10, 1, 2, 4, -8, 3, 20, 30], dtype=int32)
|
||
|
||
For array with ``ndim > 1``, the differences are computed after flattening
|
||
the input array.
|
||
|
||
>>> a1 = jnp.array([[2, -1, 4, 7],
|
||
... [3, 5, -6, 9]])
|
||
>>> jnp.ediff1d(a1)
|
||
Array([ -3, 5, 3, -4, 2, -11, 15], dtype=int32)
|
||
>>> a2 = jnp.array([2, -1, 4, 7, 3, 5, -6, 9])
|
||
>>> jnp.ediff1d(a2)
|
||
Array([ -3, 5, 3, -4, 2, -11, 15], dtype=int32)
|
||
"""
|
||
arr = util.ensure_arraylike("ediff1d", ary).ravel()
|
||
result = lax.sub(arr[1:], arr[:-1])
|
||
if to_begin is not None:
|
||
to_begin = util.ensure_arraylike("ediff1d", to_begin)
|
||
result = concatenate((ravel(to_begin.astype(arr.dtype)), result))
|
||
if to_end is not None:
|
||
to_end = util.ensure_arraylike("ediff1d", to_end)
|
||
result = concatenate((result, ravel(to_end.astype(arr.dtype))))
|
||
return result
|
||
|
||
|
||
@export
|
||
@partial(jit, static_argnames=("axis", "edge_order"))
|
||
def gradient(
|
||
f: ArrayLike,
|
||
*varargs: ArrayLike,
|
||
axis: int | Sequence[int] | None = None,
|
||
edge_order: int | None = None,
|
||
) -> Array | list[Array]:
|
||
"""Compute the numerical gradient of a sampled function.
|
||
|
||
JAX implementation of :func:`numpy.gradient`.
|
||
|
||
The gradient in ``jnp.gradient`` is computed using second-order finite
|
||
differences across the array of sampled function values. This should not
|
||
be confused with :func:`jax.grad`, which computes a precise gradient of
|
||
a callable function via :ref:`automatic differentiation <automatic-differentiation>`.
|
||
|
||
Args:
|
||
f: *N*-dimensional array of function values.
|
||
varargs: optional list of scalars or arrays specifying spacing of
|
||
function evaluations. Options are:
|
||
|
||
- not specified: unit spacing in all dimensions.
|
||
- a single scalar: constant spacing in all dimensions.
|
||
- *N* values: specify different spacing in each dimension:
|
||
|
||
- scalar values indicate constant spacing in that dimension.
|
||
- array values must match the length of the corresponding dimension,
|
||
and specify the coordinates at which ``f`` is evaluated.
|
||
|
||
edge_order: not implemented in JAX
|
||
axis: integer or tuple of integers specifying the axis along which
|
||
to compute the gradient. If None (default) calculates the gradient
|
||
along all axes.
|
||
|
||
Returns:
|
||
an array or tuple of arrays containing the numerical gradient along
|
||
each specified axis.
|
||
|
||
See also:
|
||
- :func:`jax.grad`: automatic differentiation of a function with a single output.
|
||
|
||
Examples:
|
||
Comparing numerical and automatic differentiation of a simple function:
|
||
|
||
>>> def f(x):
|
||
... return jnp.sin(x) * jnp.exp(-x / 4)
|
||
...
|
||
>>> def gradf_exact(x):
|
||
... # exact analytical gradient of f(x)
|
||
... return -f(x) / 4 + jnp.cos(x) * jnp.exp(-x / 4)
|
||
...
|
||
>>> x = jnp.linspace(0, 5, 10)
|
||
|
||
>>> with jnp.printoptions(precision=2, suppress=True):
|
||
... print("numerical gradient:", jnp.gradient(f(x), x))
|
||
... print("automatic gradient:", jax.vmap(jax.grad(f))(x))
|
||
... print("exact gradient: ", gradf_exact(x))
|
||
...
|
||
numerical gradient: [ 0.83 0.61 0.18 -0.2 -0.43 -0.49 -0.39 -0.21 -0.02 0.08]
|
||
automatic gradient: [ 1. 0.62 0.17 -0.23 -0.46 -0.51 -0.41 -0.21 -0.01 0.15]
|
||
exact gradient: [ 1. 0.62 0.17 -0.23 -0.46 -0.51 -0.41 -0.21 -0.01 0.15]
|
||
|
||
Notice that, as expected, the numerical gradient has some approximation error
|
||
compared to the automatic gradient computed via :func:`jax.grad`.
|
||
"""
|
||
|
||
if edge_order is not None:
|
||
raise NotImplementedError(
|
||
"The 'edge_order' argument to jnp.gradient is not supported."
|
||
)
|
||
a, *spacing = util.promote_dtypes_inexact(f, *varargs)
|
||
|
||
def gradient_along_axis(a, h, axis):
|
||
sliced = partial(lax.slice_in_dim, a, axis=axis)
|
||
upper_edge = sliced(1, 2) - sliced(0, 1)
|
||
lower_edge = sliced(-1, None) - sliced(-2, -1)
|
||
|
||
if np.ndim(h) == 0:
|
||
inner = (sliced(2, None) - sliced(None, -2)) * 0.5 / h
|
||
lower_edge /= h
|
||
upper_edge /= h
|
||
|
||
elif np.ndim(h) == 1:
|
||
if len(h) != a.shape[axis]:
|
||
raise ValueError(
|
||
"Spacing arrays must have the same length as the "
|
||
"dimension along which the gradient is calculated."
|
||
)
|
||
h_shape = [1] * a.ndim
|
||
h_shape[axis] = len(h)
|
||
h = h.reshape(h_shape)
|
||
sliced_x = partial(lax.slice_in_dim, h, axis=axis)
|
||
|
||
upper_edge /= sliced_x(1, 2) - sliced_x(0, 1)
|
||
lower_edge /= sliced_x(-1, None) - sliced_x(-2, -1)
|
||
dx1 = sliced_x(1, -1) - sliced_x(0, -2)
|
||
dx2 = sliced_x(2, None) - sliced_x(1, -1)
|
||
a = -(dx2) / (dx1 * (dx1 + dx2))
|
||
b = (dx2 - dx1) / (dx1 * dx2)
|
||
c = dx1 / (dx2 * (dx1 + dx2))
|
||
inner = a * sliced(0, -2) + b * sliced(1, -1) + c * sliced(2, None)
|
||
else:
|
||
raise ValueError("Spacing arrays must be 1D arrays or scalars.")
|
||
|
||
return concatenate((upper_edge, inner, lower_edge), axis=axis)
|
||
|
||
if axis is None:
|
||
axis_tuple = tuple(range(a.ndim))
|
||
else:
|
||
axis_tuple = tuple(_canonicalize_axis(i, a.ndim) for i in _ensure_index_tuple(axis))
|
||
if len(axis_tuple) == 0:
|
||
return []
|
||
|
||
if min(s for i, s in enumerate(a.shape) if i in axis_tuple) < 2:
|
||
raise ValueError("Shape of array too small to calculate "
|
||
"a numerical gradient, "
|
||
"at least 2 elements are required.")
|
||
if len(spacing) == 0:
|
||
dx: Sequence[ArrayLike] = [1.0] * len(axis_tuple)
|
||
elif len(spacing) == 1:
|
||
dx = list(spacing) * len(axis_tuple)
|
||
elif len(spacing) == len(axis_tuple):
|
||
dx = list(spacing)
|
||
else:
|
||
TypeError(f"Invalid number of spacing arguments {len(spacing)} for {axis=}")
|
||
|
||
a_grad = [gradient_along_axis(a, h, ax) for ax, h in zip(axis_tuple, dx)]
|
||
return a_grad[0] if len(axis_tuple) == 1 else a_grad
|
||
|
||
|
||
@export
|
||
def isrealobj(x: Any) -> bool:
|
||
"""Check if the input is not a complex number or an array containing complex elements.
|
||
|
||
JAX implementation of :func:`numpy.isrealobj`.
|
||
|
||
The function evaluates based on input type rather than value.
|
||
Inputs with zero imaginary parts are still considered complex.
|
||
|
||
Args:
|
||
x: input object to check.
|
||
|
||
Returns:
|
||
False if ``x`` is a complex number or an array containing at least one complex element,
|
||
True otherwise.
|
||
|
||
See Also:
|
||
- :func:`jax.numpy.iscomplexobj`
|
||
- :func:`jax.numpy.isreal`
|
||
|
||
Examples:
|
||
>>> jnp.isrealobj(0)
|
||
True
|
||
>>> jnp.isrealobj(1.2)
|
||
True
|
||
>>> jnp.isrealobj(jnp.array([1, 2]))
|
||
True
|
||
>>> jnp.isrealobj(1+2j)
|
||
False
|
||
>>> jnp.isrealobj(jnp.array([0, 1+2j]))
|
||
False
|
||
"""
|
||
return not iscomplexobj(x)
|
||
|
||
|
||
@export
|
||
def reshape(
|
||
a: ArrayLike, shape: DimSize | Shape | None = None, order: str = "C", *,
|
||
newshape: DimSize | Shape | DeprecatedArg = DeprecatedArg(),
|
||
copy: bool | None = None) -> Array:
|
||
"""Return a reshaped copy of an array.
|
||
|
||
JAX implementation of :func:`numpy.reshape`, implemented in terms of
|
||
:func:`jax.lax.reshape`.
|
||
|
||
Args:
|
||
a: input array to reshape
|
||
shape: integer or sequence of integers giving the new shape, which must match the
|
||
size of the input array. If any single dimension is given size ``-1``, it will be
|
||
replaced with a value such that the output has the correct size.
|
||
order: ``'F'`` or ``'C'``, specifies whether the reshape should apply column-major
|
||
(fortran-style, ``"F"``) or row-major (C-style, ``"C"``) order; default is ``"C"``.
|
||
JAX does not support ``order="A"``.
|
||
copy: unused by JAX; JAX always returns a copy, though under JIT the compiler
|
||
may optimize such copies away.
|
||
newshape: deprecated alias of the ``shape`` argument. Will result in a
|
||
:class:`DeprecationWarning` if used.
|
||
|
||
Returns:
|
||
reshaped copy of input array with the specified shape.
|
||
|
||
Notes:
|
||
Unlike :func:`numpy.reshape`, :func:`jax.numpy.reshape` will return a copy rather
|
||
than a view of the input array. However, under JIT, the compiler will optimize-away
|
||
such copies when possible, so this doesn't have performance impacts in practice.
|
||
|
||
See Also:
|
||
- :meth:`jax.Array.reshape`: equivalent functionality via an array method.
|
||
- :func:`jax.numpy.ravel`: flatten an array into a 1D shape.
|
||
- :func:`jax.numpy.squeeze`: remove one or more length-1 axes from an array's shape.
|
||
|
||
Examples:
|
||
>>> x = jnp.array([[1, 2, 3],
|
||
... [4, 5, 6]])
|
||
>>> jnp.reshape(x, 6)
|
||
Array([1, 2, 3, 4, 5, 6], dtype=int32)
|
||
>>> jnp.reshape(x, (3, 2))
|
||
Array([[1, 2],
|
||
[3, 4],
|
||
[5, 6]], dtype=int32)
|
||
|
||
You can use ``-1`` to automatically compute a shape that is consistent with
|
||
the input size:
|
||
|
||
>>> jnp.reshape(x, -1) # -1 is inferred to be 6
|
||
Array([1, 2, 3, 4, 5, 6], dtype=int32)
|
||
>>> jnp.reshape(x, (-1, 2)) # -1 is inferred to be 3
|
||
Array([[1, 2],
|
||
[3, 4],
|
||
[5, 6]], dtype=int32)
|
||
|
||
The default ordering of axes in the reshape is C-style row-major ordering.
|
||
To use Fortran-style column-major ordering, specify ``order='F'``:
|
||
|
||
>>> jnp.reshape(x, 6, order='F')
|
||
Array([1, 4, 2, 5, 3, 6], dtype=int32)
|
||
>>> jnp.reshape(x, (3, 2), order='F')
|
||
Array([[1, 5],
|
||
[4, 3],
|
||
[2, 6]], dtype=int32)
|
||
|
||
For convenience, this functionality is also available via the
|
||
:meth:`jax.Array.reshape` method:
|
||
|
||
>>> x.reshape(3, 2)
|
||
Array([[1, 2],
|
||
[3, 4],
|
||
[5, 6]], dtype=int32)
|
||
"""
|
||
del copy # unused
|
||
|
||
__tracebackhide__ = True
|
||
util.check_arraylike("reshape", a)
|
||
|
||
# TODO(jakevdp): finalized 2024-12-2; remove argument after JAX v0.4.40.
|
||
if not isinstance(newshape, DeprecatedArg):
|
||
raise TypeError("The newshape argument to jnp.reshape was removed in JAX v0.4.36."
|
||
" Use shape instead.")
|
||
if shape is None:
|
||
raise TypeError(
|
||
"jnp.shape requires passing a `shape` argument, but none was given."
|
||
)
|
||
try:
|
||
# forward to method for ndarrays
|
||
return a.reshape(shape, order=order) # type: ignore[call-overload,union-attr]
|
||
except AttributeError:
|
||
pass
|
||
return asarray(a).reshape(shape, order=order)
|
||
|
||
|
||
@export
|
||
@partial(jit, static_argnames=('order',), inline=True)
|
||
def ravel(a: ArrayLike, order: str = "C") -> Array:
|
||
"""Flatten array into a 1-dimensional shape.
|
||
|
||
JAX implementation of :func:`numpy.ravel`, implemented in terms of
|
||
:func:`jax.lax.reshape`.
|
||
|
||
``ravel(arr, order=order)`` is equivalent to ``reshape(arr, -1, order=order)``.
|
||
|
||
Args:
|
||
a: array to be flattened.
|
||
order: ``'F'`` or ``'C'``, specifies whether the reshape should apply column-major
|
||
(fortran-style, ``"F"``) or row-major (C-style, ``"C"``) order; default is ``"C"``.
|
||
JAX does not support `order="A"` or `order="K"`.
|
||
|
||
Returns:
|
||
flattened copy of input array.
|
||
|
||
Notes:
|
||
Unlike :func:`numpy.ravel`, :func:`jax.numpy.ravel` will return a copy rather
|
||
than a view of the input array. However, under JIT, the compiler will optimize-away
|
||
such copies when possible, so this doesn't have performance impacts in practice.
|
||
|
||
See Also:
|
||
- :meth:`jax.Array.ravel`: equivalent functionality via an array method.
|
||
- :func:`jax.numpy.reshape`: general array reshape.
|
||
|
||
Examples:
|
||
>>> x = jnp.array([[1, 2, 3],
|
||
... [4, 5, 6]])
|
||
|
||
By default, ravel in C-style, row-major order
|
||
|
||
>>> jnp.ravel(x)
|
||
Array([1, 2, 3, 4, 5, 6], dtype=int32)
|
||
|
||
Optionally ravel in Fortran-style, column-major:
|
||
|
||
>>> jnp.ravel(x, order='F')
|
||
Array([1, 4, 2, 5, 3, 6], dtype=int32)
|
||
|
||
For convenience, the same functionality is available via the :meth:`jax.Array.ravel`
|
||
method:
|
||
|
||
>>> x.ravel()
|
||
Array([1, 2, 3, 4, 5, 6], dtype=int32)
|
||
"""
|
||
util.check_arraylike("ravel", a)
|
||
if order == "K":
|
||
raise NotImplementedError("Ravel not implemented for order='K'.")
|
||
return reshape(a, (np.size(a),), order)
|
||
|
||
|
||
@export
|
||
def ravel_multi_index(multi_index: Sequence[ArrayLike], dims: Sequence[int],
|
||
mode: str = 'raise', order: str = 'C') -> Array:
|
||
"""Convert multi-dimensional indices into flat indices.
|
||
|
||
JAX implementation of :func:`numpy.ravel_multi_index`
|
||
|
||
Args:
|
||
multi_index: sequence of integer arrays containing indices in each dimension.
|
||
dims: sequence of integer sizes; must have ``len(dims) == len(multi_index)``
|
||
mode: how to handle out-of bound indices. Options are
|
||
|
||
- ``"raise"`` (default): raise a ValueError. This mode is incompatible
|
||
with :func:`~jax.jit` or other JAX transformations.
|
||
- ``"clip"``: clip out-of-bound indices to valid range.
|
||
- ``"wrap"``: wrap out-of-bound indices to valid range.
|
||
|
||
order: ``"C"`` (default) or ``"F"``, specify whether to assume C-style
|
||
row-major order or Fortran-style column-major order.
|
||
|
||
Returns:
|
||
array of flattened indices
|
||
|
||
See also:
|
||
:func:`jax.numpy.unravel_index`: inverse of this function.
|
||
|
||
Examples:
|
||
Define a 2-dimensional array and a sequence of indices of even values:
|
||
|
||
>>> x = jnp.array([[2., 3., 4.],
|
||
... [5., 6., 7.]])
|
||
>>> indices = jnp.where(x % 2 == 0)
|
||
>>> indices
|
||
(Array([0, 0, 1], dtype=int32), Array([0, 2, 1], dtype=int32))
|
||
>>> x[indices]
|
||
Array([2., 4., 6.], dtype=float32)
|
||
|
||
Compute the flattened indices:
|
||
|
||
>>> indices_flat = jnp.ravel_multi_index(indices, x.shape)
|
||
>>> indices_flat
|
||
Array([0, 2, 4], dtype=int32)
|
||
|
||
These flattened indices can be used to extract the same values from the
|
||
flattened ``x`` array:
|
||
|
||
>>> x_flat = x.ravel()
|
||
>>> x_flat
|
||
Array([2., 3., 4., 5., 6., 7.], dtype=float32)
|
||
>>> x_flat[indices_flat]
|
||
Array([2., 4., 6.], dtype=float32)
|
||
|
||
The original indices can be recovered with :func:`~jax.numpy.unravel_index`:
|
||
|
||
>>> jnp.unravel_index(indices_flat, x.shape)
|
||
(Array([0, 0, 1], dtype=int32), Array([0, 2, 1], dtype=int32))
|
||
"""
|
||
assert len(multi_index) == len(dims), f"len(multi_index)={len(multi_index)} != len(dims)={len(dims)}"
|
||
dims = tuple(core.concrete_or_error(operator.index, d, "in `dims` argument of ravel_multi_index().") for d in dims)
|
||
util.check_arraylike("ravel_multi_index", *multi_index)
|
||
multi_index_arr = [asarray(i) for i in multi_index]
|
||
for index in multi_index_arr:
|
||
if mode == 'raise':
|
||
core.concrete_or_error(array, index,
|
||
"The error occurred because ravel_multi_index was jit-compiled"
|
||
" with mode='raise'. Use mode='wrap' or mode='clip' instead.")
|
||
if not issubdtype(_dtype(index), np.integer):
|
||
raise TypeError("only int indices permitted")
|
||
if mode == "raise":
|
||
if any(reductions.any((i < 0) | (i >= d)) for i, d in zip(multi_index_arr, dims)):
|
||
raise ValueError("invalid entry in coordinates array")
|
||
elif mode == "clip":
|
||
multi_index_arr = [clip(i, 0, d - 1) for i, d in zip(multi_index_arr, dims)]
|
||
elif mode == "wrap":
|
||
multi_index_arr = [i % d for i, d in zip(multi_index_arr, dims)]
|
||
else:
|
||
raise ValueError(f"invalid mode={mode!r}. Expected 'raise', 'wrap', or 'clip'")
|
||
|
||
if order == "F":
|
||
strides = np.cumprod((1,) + dims[:-1])
|
||
elif order == "C":
|
||
strides = np.cumprod((1,) + dims[1:][::-1])[::-1]
|
||
else:
|
||
raise ValueError(f"invalid order={order!r}. Expected 'C' or 'F'")
|
||
|
||
result = array(0, dtype=(multi_index_arr[0].dtype if multi_index_arr
|
||
else dtypes.canonicalize_dtype(dtypes.int_)))
|
||
for i, s in zip(multi_index_arr, strides):
|
||
result = result + i * int(s)
|
||
return result
|
||
|
||
|
||
@export
|
||
def unravel_index(indices: ArrayLike, shape: Shape) -> tuple[Array, ...]:
|
||
"""Convert flat indices into multi-dimensional indices.
|
||
|
||
JAX implementation of :func:`numpy.unravel_index`. The JAX version differs in
|
||
its treatment of out-of-bound indices: unlike NumPy, negative indices are
|
||
supported, and out-of-bound indices are clipped to the nearest valid value.
|
||
|
||
Args:
|
||
indices: integer array of flat indices
|
||
shape: shape of multidimensional array to index into
|
||
|
||
Returns:
|
||
Tuple of unraveled indices
|
||
|
||
See also:
|
||
:func:`jax.numpy.ravel_multi_index`: Inverse of this function.
|
||
|
||
Examples:
|
||
Start with a 1D array values and indices:
|
||
|
||
>>> x = jnp.array([2., 3., 4., 5., 6., 7.])
|
||
>>> indices = jnp.array([1, 3, 5])
|
||
>>> print(x[indices])
|
||
[3. 5. 7.]
|
||
|
||
Now if ``x`` is reshaped, ``unravel_indices`` can be used to convert
|
||
the flat indices into a tuple of indices that access the same entries:
|
||
|
||
>>> shape = (2, 3)
|
||
>>> x_2D = x.reshape(shape)
|
||
>>> indices_2D = jnp.unravel_index(indices, shape)
|
||
>>> indices_2D
|
||
(Array([0, 1, 1], dtype=int32), Array([1, 0, 2], dtype=int32))
|
||
>>> print(x_2D[indices_2D])
|
||
[3. 5. 7.]
|
||
|
||
The inverse function, ``ravel_multi_index``, can be used to obtain the
|
||
original indices:
|
||
|
||
>>> jnp.ravel_multi_index(indices_2D, shape)
|
||
Array([1, 3, 5], dtype=int32)
|
||
"""
|
||
indices_arr = util.ensure_arraylike("unravel_index", indices)
|
||
# Note: we do not convert shape to an array, because it may be passed as a
|
||
# tuple of weakly-typed values, and asarray() would strip these weak types.
|
||
try:
|
||
shape = list(shape)
|
||
except TypeError:
|
||
# TODO: Consider warning here since shape is supposed to be a sequence, so
|
||
# this should not happen.
|
||
shape = [shape]
|
||
if any(np.ndim(s) != 0 for s in shape):
|
||
raise ValueError("unravel_index: shape should be a scalar or 1D sequence.")
|
||
out_indices: list[ArrayLike] = [0] * len(shape)
|
||
for i, s in reversed(list(enumerate(shape))):
|
||
indices_arr, out_indices[i] = ufuncs.divmod(indices_arr, s)
|
||
oob_pos = indices_arr > 0
|
||
oob_neg = indices_arr < -1
|
||
return tuple(where(oob_pos, s - 1, where(oob_neg, 0, i))
|
||
for s, i in safe_zip(shape, out_indices))
|
||
|
||
|
||
@export
|
||
@partial(jit, static_argnames=('new_shape',))
|
||
def resize(a: ArrayLike, new_shape: Shape) -> Array:
|
||
"""Return a new array with specified shape.
|
||
|
||
JAX implementation of :func:`numpy.resize`.
|
||
|
||
Args:
|
||
a: input array or scalar.
|
||
new_shape: int or tuple of ints. Specifies the shape of the resized array.
|
||
|
||
Returns:
|
||
A resized array with specified shape. The elements of ``a`` are repeated in
|
||
the resized array, if the resized array is larger than the original aray.
|
||
|
||
See also:
|
||
- :func:`jax.numpy.reshape`: Returns a reshaped copy of an array.
|
||
- :func:`jax.numpy.repeat`: Constructs an array from repeated elements.
|
||
|
||
Examples:
|
||
>>> x = jnp.array([1, 2, 3, 4, 5, 6, 7, 8, 9])
|
||
>>> jnp.resize(x, (3, 3))
|
||
Array([[1, 2, 3],
|
||
[4, 5, 6],
|
||
[7, 8, 9]], dtype=int32)
|
||
>>> jnp.resize(x, (3, 4))
|
||
Array([[1, 2, 3, 4],
|
||
[5, 6, 7, 8],
|
||
[9, 1, 2, 3]], dtype=int32)
|
||
>>> jnp.resize(4, (3, 2))
|
||
Array([[4, 4],
|
||
[4, 4],
|
||
[4, 4]], dtype=int32, weak_type=True)
|
||
"""
|
||
util.check_arraylike("resize", a)
|
||
new_shape = _ensure_index_tuple(new_shape)
|
||
|
||
if any(dim_length < 0 for dim_length in new_shape):
|
||
raise ValueError("all elements of `new_shape` must be non-negative")
|
||
|
||
arr = ravel(a)
|
||
|
||
new_size = math.prod(new_shape)
|
||
if arr.size == 0 or new_size == 0:
|
||
return zeros_like(arr, shape=new_shape)
|
||
|
||
repeats = ceil_of_ratio(new_size, arr.size)
|
||
arr = tile(arr, repeats)[:new_size]
|
||
|
||
return reshape(arr, new_shape)
|
||
|
||
|
||
@export
|
||
def squeeze(a: ArrayLike, axis: int | Sequence[int] | None = None) -> Array:
|
||
"""Remove one or more length-1 axes from array
|
||
|
||
JAX implementation of :func:`numpy.sqeeze`, implemented via :func:`jax.lax.squeeze`.
|
||
|
||
Args:
|
||
a: input array
|
||
axis: integer or sequence of integers specifying axes to remove. If any specified
|
||
axis does not have a length of 1, an error is raised. If not specified, squeeze
|
||
all length-1 axes in ``a``.
|
||
|
||
Returns:
|
||
copy of ``a`` with length-1 axes removed.
|
||
|
||
Notes:
|
||
Unlike :func:`numpy.squeeze`, :func:`jax.numpy.squeeze` will return a copy rather
|
||
than a view of the input array. However, under JIT, the compiler will optimize-away
|
||
such copies when possible, so this doesn't have performance impacts in practice.
|
||
|
||
See Also:
|
||
- :func:`jax.numpy.expand_dims`: the inverse of ``squeeze``: add dimensions of length 1.
|
||
- :meth:`jax.Array.squeeze`: equivalent functionality via an array method.
|
||
- :func:`jax.lax.squeeze`: equivalent XLA API.
|
||
- :func:`jax.numpy.ravel`: flatten an array into a 1D shape.
|
||
- :func:`jax.numpy.reshape`: general array reshape.
|
||
|
||
Examples:
|
||
>>> x = jnp.array([[[0]], [[1]], [[2]]])
|
||
>>> x.shape
|
||
(3, 1, 1)
|
||
|
||
Squeeze all length-1 dimensions:
|
||
|
||
>>> jnp.squeeze(x)
|
||
Array([0, 1, 2], dtype=int32)
|
||
>>> _.shape
|
||
(3,)
|
||
|
||
Equivalent while specifying the axes explicitly:
|
||
|
||
>>> jnp.squeeze(x, axis=(1, 2))
|
||
Array([0, 1, 2], dtype=int32)
|
||
|
||
Attempting to squeeze a non-unit axis results in an error:
|
||
|
||
>>> jnp.squeeze(x, axis=0) # doctest: +IGNORE_EXCEPTION_DETAIL
|
||
Traceback (most recent call last):
|
||
...
|
||
ValueError: cannot select an axis to squeeze out which has size not equal to one, got shape=(3, 1, 1) and dimensions=(0,)
|
||
|
||
For convenience, this functionality is also available via the
|
||
:meth:`jax.Array.squeeze` method:
|
||
|
||
>>> x.squeeze()
|
||
Array([0, 1, 2], dtype=int32)
|
||
"""
|
||
arr = util.ensure_arraylike("squeeze", a)
|
||
return _squeeze(arr, _ensure_index_tuple(axis) if axis is not None else None)
|
||
|
||
@partial(jit, static_argnames=('axis',), inline=True)
|
||
def _squeeze(a: Array, axis: tuple[int, ...]) -> Array:
|
||
if axis is None:
|
||
a_shape = np.shape(a)
|
||
if not core.is_constant_shape(a_shape):
|
||
# We do not even know the rank of the output if the input shape is not known
|
||
raise ValueError("jnp.squeeze with axis=None is not supported with shape polymorphism")
|
||
axis = tuple(i for i, d in enumerate(a_shape) if d == 1)
|
||
return lax.squeeze(a, axis)
|
||
|
||
|
||
@export
|
||
def expand_dims(a: ArrayLike, axis: int | Sequence[int]) -> Array:
|
||
"""Insert dimensions of length 1 into array
|
||
|
||
JAX implementation of :func:`numpy.expand_dims`, implemented via
|
||
:func:`jax.lax.expand_dims`.
|
||
|
||
Args:
|
||
a: input array
|
||
axis: integer or sequence of integers specifying positions of axes to add.
|
||
|
||
Returns:
|
||
Copy of ``a`` with added dimensions.
|
||
|
||
Notes:
|
||
Unlike :func:`numpy.expand_dims`, :func:`jax.numpy.expand_dims` will return a copy
|
||
rather than a view of the input array. However, under JIT, the compiler will optimize
|
||
away such copies when possible, so this doesn't have performance impacts in practice.
|
||
|
||
See Also:
|
||
- :func:`jax.numpy.squeeze`: inverse of this operation, i.e. remove length-1 dimensions.
|
||
- :func:`jax.lax.expand_dims`: XLA version of this functionality.
|
||
|
||
Examples:
|
||
>>> x = jnp.array([1, 2, 3])
|
||
>>> x.shape
|
||
(3,)
|
||
|
||
Expand the leading dimension:
|
||
|
||
>>> jnp.expand_dims(x, 0)
|
||
Array([[1, 2, 3]], dtype=int32)
|
||
>>> _.shape
|
||
(1, 3)
|
||
|
||
Expand the trailing dimension:
|
||
|
||
>>> jnp.expand_dims(x, 1)
|
||
Array([[1],
|
||
[2],
|
||
[3]], dtype=int32)
|
||
>>> _.shape
|
||
(3, 1)
|
||
|
||
Expand multiple dimensions:
|
||
|
||
>>> jnp.expand_dims(x, (0, 1, 3))
|
||
Array([[[[1],
|
||
[2],
|
||
[3]]]], dtype=int32)
|
||
>>> _.shape
|
||
(1, 1, 3, 1)
|
||
|
||
Dimensions can also be expanded more succinctly by indexing with ``None``:
|
||
|
||
>>> x[None] # equivalent to jnp.expand_dims(x, 0)
|
||
Array([[1, 2, 3]], dtype=int32)
|
||
>>> x[:, None] # equivalent to jnp.expand_dims(x, 1)
|
||
Array([[1],
|
||
[2],
|
||
[3]], dtype=int32)
|
||
>>> x[None, None, :, None] # equivalent to jnp.expand_dims(x, (0, 1, 3))
|
||
Array([[[[1],
|
||
[2],
|
||
[3]]]], dtype=int32)
|
||
"""
|
||
util.check_arraylike("expand_dims", a)
|
||
axis = _ensure_index_tuple(axis)
|
||
return lax.expand_dims(a, axis)
|
||
|
||
|
||
@export
|
||
@partial(jit, static_argnames=('axis1', 'axis2'), inline=True)
|
||
def swapaxes(a: ArrayLike, axis1: int, axis2: int) -> Array:
|
||
"""Swap two axes of an array.
|
||
|
||
JAX implementation of :func:`numpy.swapaxes`, implemented in terms of
|
||
:func:`jax.lax.transpose`.
|
||
|
||
Args:
|
||
a: input array
|
||
axis1: index of first axis
|
||
axis2: index of second axis
|
||
|
||
Returns:
|
||
Copy of ``a`` with specified axes swapped.
|
||
|
||
Notes:
|
||
Unlike :func:`numpy.swapaxes`, :func:`jax.numpy.swapaxes` will return a copy rather
|
||
than a view of the input array. However, under JIT, the compiler will optimize away
|
||
such copies when possible, so this doesn't have performance impacts in practice.
|
||
|
||
See Also:
|
||
- :func:`jax.numpy.moveaxis`: move a single axis of an array.
|
||
- :func:`jax.numpy.rollaxis`: older API for ``moveaxis``.
|
||
- :func:`jax.lax.transpose`: more general axes permutations.
|
||
- :meth:`jax.Array.swapaxes`: same functionality via an array method.
|
||
|
||
Examples:
|
||
>>> a = jnp.ones((2, 3, 4, 5))
|
||
>>> jnp.swapaxes(a, 1, 3).shape
|
||
(2, 5, 4, 3)
|
||
|
||
Equivalent output via the ``swapaxes`` array method:
|
||
|
||
>>> a.swapaxes(1, 3).shape
|
||
(2, 5, 4, 3)
|
||
|
||
Equivalent output via :func:`~jax.numpy.transpose`:
|
||
|
||
>>> a.transpose(0, 3, 2, 1).shape
|
||
(2, 5, 4, 3)
|
||
"""
|
||
util.check_arraylike("swapaxes", a)
|
||
perm = np.arange(np.ndim(a))
|
||
perm[axis1], perm[axis2] = perm[axis2], perm[axis1]
|
||
return lax.transpose(a, list(perm))
|
||
|
||
|
||
@export
|
||
def moveaxis(a: ArrayLike, source: int | Sequence[int],
|
||
destination: int | Sequence[int]) -> Array:
|
||
"""Move an array axis to a new position
|
||
|
||
JAX implementation of :func:`numpy.moveaxis`, implemented in terms of
|
||
:func:`jax.lax.transpose`.
|
||
|
||
Args:
|
||
a: input array
|
||
source: index or indices of the axes to move.
|
||
destination: index or indices of the axes destinations
|
||
|
||
Returns:
|
||
Copy of ``a`` with axes moved from ``source`` to ``destination``.
|
||
|
||
Notes:
|
||
Unlike :func:`numpy.moveaxis`, :func:`jax.numpy.moveaxis` will return a copy rather
|
||
than a view of the input array. However, under JIT, the compiler will optimize away
|
||
such copies when possible, so this doesn't have performance impacts in practice.
|
||
|
||
See also:
|
||
- :func:`jax.numpy.swapaxes`: swap two axes.
|
||
- :func:`jax.numpy.rollaxis`: older API for moving an axis.
|
||
- :func:`jax.numpy.transpose`: general axes permutation.
|
||
|
||
Examples:
|
||
>>> a = jnp.ones((2, 3, 4, 5))
|
||
|
||
Move axis ``1`` to the end of the array:
|
||
|
||
>>> jnp.moveaxis(a, 1, -1).shape
|
||
(2, 4, 5, 3)
|
||
|
||
Move the last axis to position 1:
|
||
|
||
>>> jnp.moveaxis(a, -1, 1).shape
|
||
(2, 5, 3, 4)
|
||
|
||
Move multiple axes:
|
||
|
||
>>> jnp.moveaxis(a, (0, 1), (-1, -2)).shape
|
||
(4, 5, 3, 2)
|
||
|
||
This can also be accomplished via :func:`~jax.numpy.transpose`:
|
||
|
||
>>> a.transpose(2, 3, 1, 0).shape
|
||
(4, 5, 3, 2)
|
||
"""
|
||
arr = util.ensure_arraylike("moveaxis", a)
|
||
return _moveaxis(arr, _ensure_index_tuple(source),
|
||
_ensure_index_tuple(destination))
|
||
|
||
@partial(jit, static_argnames=('source', 'destination'), inline=True)
|
||
def _moveaxis(a: Array, source: tuple[int, ...], destination: tuple[int, ...]) -> Array:
|
||
source = tuple(_canonicalize_axis(i, np.ndim(a)) for i in source)
|
||
destination = tuple(_canonicalize_axis(i, np.ndim(a)) for i in destination)
|
||
if len(source) != len(destination):
|
||
raise ValueError("Inconsistent number of elements: {} vs {}"
|
||
.format(len(source), len(destination)))
|
||
perm = [i for i in range(np.ndim(a)) if i not in source]
|
||
for dest, src in sorted(zip(destination, source)):
|
||
perm.insert(dest, src)
|
||
return lax.transpose(a, perm)
|
||
|
||
|
||
@export
|
||
@partial(jit, static_argnames=('equal_nan',))
|
||
def isclose(a: ArrayLike, b: ArrayLike, rtol: ArrayLike = 1e-05, atol: ArrayLike = 1e-08,
|
||
equal_nan: bool = False) -> Array:
|
||
r"""Check if the elements of two arrays are approximately equal within a tolerance.
|
||
|
||
JAX implementation of :func:`numpy.allclose`.
|
||
|
||
Essentially this function evaluates the following condition:
|
||
|
||
.. math::
|
||
|
||
|a - b| \le \mathtt{atol} + \mathtt{rtol} * |b|
|
||
|
||
``jnp.inf`` in ``a`` will be considered equal to ``jnp.inf`` in ``b``.
|
||
|
||
Args:
|
||
a: first input array to compare.
|
||
b: second input array to compare.
|
||
rtol: relative tolerance used for approximate equality. Default = 1e-05.
|
||
atol: absolute tolerance used for approximate equality. Default = 1e-08.
|
||
equal_nan: Boolean. If ``True``, NaNs in ``a`` will be considered
|
||
equal to NaNs in ``b``. Default is ``False``.
|
||
|
||
Returns:
|
||
A new array containing boolean values indicating whether the input arrays
|
||
are element-wise approximately equal within the specified tolerances.
|
||
|
||
See Also:
|
||
- :func:`jax.numpy.allclose`
|
||
- :func:`jax.numpy.equal`
|
||
|
||
Examples:
|
||
>>> jnp.isclose(jnp.array([1e6, 2e6, jnp.inf]), jnp.array([1e6, 2e7, jnp.inf]))
|
||
Array([ True, False, True], dtype=bool)
|
||
>>> jnp.isclose(jnp.array([1e6, 2e6, 3e6]),
|
||
... jnp.array([1.00008e6, 2.00008e7, 3.00008e8]), rtol=1e3)
|
||
Array([ True, True, True], dtype=bool)
|
||
>>> jnp.isclose(jnp.array([1e6, 2e6, 3e6]),
|
||
... jnp.array([1.00001e6, 2.00002e6, 3.00009e6]), atol=1e3)
|
||
Array([ True, True, True], dtype=bool)
|
||
>>> jnp.isclose(jnp.array([jnp.nan, 1, 2]),
|
||
... jnp.array([jnp.nan, 1, 2]), equal_nan=True)
|
||
Array([ True, True, True], dtype=bool)
|
||
"""
|
||
a, b = util.promote_args("isclose", a, b)
|
||
dtype = _dtype(a)
|
||
if dtypes.issubdtype(dtype, dtypes.extended):
|
||
return lax.eq(a, b)
|
||
|
||
a, b = util.promote_args_inexact("isclose", a, b)
|
||
dtype = _dtype(a)
|
||
if issubdtype(dtype, np.complexfloating):
|
||
dtype = util._complex_elem_type(dtype)
|
||
rtol = lax.convert_element_type(rtol, dtype)
|
||
atol = lax.convert_element_type(atol, dtype)
|
||
out = lax.le(
|
||
lax.abs(lax.sub(a, b)),
|
||
lax.add(atol, lax.mul(rtol, lax.abs(b))))
|
||
# This corrects the comparisons for infinite and nan values
|
||
a_inf = ufuncs.isinf(a)
|
||
b_inf = ufuncs.isinf(b)
|
||
any_inf = ufuncs.logical_or(a_inf, b_inf)
|
||
both_inf = ufuncs.logical_and(a_inf, b_inf)
|
||
# Make all elements where either a or b are infinite to False
|
||
out = ufuncs.logical_and(out, ufuncs.logical_not(any_inf))
|
||
# Make all elements where both a or b are the same inf to True
|
||
same_value = lax.eq(a, b)
|
||
same_inf = ufuncs.logical_and(both_inf, same_value)
|
||
out = ufuncs.logical_or(out, same_inf)
|
||
|
||
# Make all elements where either a or b is NaN to False
|
||
a_nan = ufuncs.isnan(a)
|
||
b_nan = ufuncs.isnan(b)
|
||
any_nan = ufuncs.logical_or(a_nan, b_nan)
|
||
out = ufuncs.logical_and(out, ufuncs.logical_not(any_nan))
|
||
if equal_nan:
|
||
# Make all elements where both a and b is NaN to True
|
||
both_nan = ufuncs.logical_and(a_nan, b_nan)
|
||
out = ufuncs.logical_or(out, both_nan)
|
||
return out
|
||
|
||
|
||
def _interp(x: ArrayLike, xp: ArrayLike, fp: ArrayLike,
|
||
left: ArrayLike | str | None = None,
|
||
right: ArrayLike | str | None = None,
|
||
period: ArrayLike | None = None) -> Array:
|
||
util.check_arraylike("interp", x, xp, fp)
|
||
if np.shape(xp) != np.shape(fp) or np.ndim(xp) != 1:
|
||
raise ValueError("xp and fp must be one-dimensional arrays of equal size")
|
||
x_arr, xp_arr = util.promote_dtypes_inexact(x, xp)
|
||
fp_arr, = util.promote_dtypes_inexact(fp)
|
||
del x, xp, fp
|
||
|
||
if isinstance(left, str):
|
||
if left != 'extrapolate':
|
||
raise ValueError("the only valid string value of `left` is "
|
||
f"'extrapolate', but got: {left!r}")
|
||
extrapolate_left = True
|
||
else:
|
||
extrapolate_left = False
|
||
if isinstance(right, str):
|
||
if right != 'extrapolate':
|
||
raise ValueError("the only valid string value of `right` is "
|
||
f"'extrapolate', but got: {right!r}")
|
||
extrapolate_right = True
|
||
else:
|
||
extrapolate_right = False
|
||
|
||
if dtypes.issubdtype(x_arr.dtype, np.complexfloating):
|
||
raise ValueError("jnp.interp: complex x values not supported.")
|
||
|
||
if period is not None:
|
||
if np.ndim(period) != 0:
|
||
raise ValueError(f"period must be a scalar; got {period}")
|
||
period = ufuncs.abs(period)
|
||
x_arr = x_arr % period
|
||
xp_arr = xp_arr % period
|
||
xp_arr, fp_arr = lax.sort_key_val(xp_arr, fp_arr)
|
||
xp_arr = concatenate([xp_arr[-1:] - period, xp_arr, xp_arr[:1] + period])
|
||
fp_arr = concatenate([fp_arr[-1:], fp_arr, fp_arr[:1]])
|
||
|
||
i = clip(searchsorted(xp_arr, x_arr, side='right'), 1, len(xp_arr) - 1)
|
||
df = fp_arr[i] - fp_arr[i - 1]
|
||
dx = xp_arr[i] - xp_arr[i - 1]
|
||
delta = x_arr - xp_arr[i - 1]
|
||
|
||
epsilon = np.spacing(np.finfo(xp_arr.dtype).eps)
|
||
dx0 = lax.abs(dx) <= epsilon # Prevent NaN gradients when `dx` is small.
|
||
f = where(dx0, fp_arr[i - 1], fp_arr[i - 1] + (delta / where(dx0, 1, dx)) * df)
|
||
|
||
if not extrapolate_left:
|
||
assert not isinstance(left, str)
|
||
left_arr: ArrayLike = fp_arr[0] if left is None else left
|
||
if period is None:
|
||
f = where(x_arr < xp_arr[0], left_arr, f)
|
||
if not extrapolate_right:
|
||
assert not isinstance(right, str)
|
||
right_arr: ArrayLike = fp_arr[-1] if right is None else right
|
||
if period is None:
|
||
f = where(x_arr > xp_arr[-1], right_arr, f)
|
||
|
||
return f
|
||
|
||
|
||
@export
|
||
def interp(x: ArrayLike, xp: ArrayLike, fp: ArrayLike,
|
||
left: ArrayLike | str | None = None,
|
||
right: ArrayLike | str | None = None,
|
||
period: ArrayLike | None = None) -> Array:
|
||
"""One-dimensional linear interpolation.
|
||
|
||
JAX implementation of :func:`numpy.interp`.
|
||
|
||
Args:
|
||
x: N-dimensional array of x coordinates at which to evaluate the interpolation.
|
||
xp: one-dimensional sorted array of points to be interpolated.
|
||
fp: array of shape ``xp.shape`` containing the function values associated with ``xp``.
|
||
left: specify how to handle points ``x < xp[0]``. Default is to return ``fp[0]``.
|
||
If ``left`` is a scalar value, it will return this value. if ``left`` is the string
|
||
``"extrapolate"``, then the value will be determined by linear extrapolation.
|
||
``left`` is ignored if ``period`` is specified.
|
||
right: specify how to handle points ``x > xp[-1]``. Default is to return ``fp[-1]``.
|
||
If ``right`` is a scalar value, it will return this value. if ``right`` is the string
|
||
``"extrapolate"``, then the value will be determined by linear extrapolation.
|
||
``right`` is ignored if ``period`` is specified.
|
||
period: optionally specify the period for the *x* coordinates, for e.g. interpolation
|
||
in angular space.
|
||
|
||
Returns:
|
||
an array of shape ``x.shape`` containing the interpolated function at values ``x``.
|
||
|
||
Examples:
|
||
>>> xp = jnp.arange(10)
|
||
>>> fp = 2 * xp
|
||
>>> x = jnp.array([0.5, 2.0, 3.5])
|
||
>>> interp(x, xp, fp)
|
||
Array([1., 4., 7.], dtype=float32)
|
||
|
||
Unless otherwise specified, extrapolation will be constant:
|
||
|
||
>>> x = jnp.array([-10., 10.])
|
||
>>> interp(x, xp, fp)
|
||
Array([ 0., 18.], dtype=float32)
|
||
|
||
Use ``"extrapolate"`` mode for linear extrapolation:
|
||
|
||
>>> interp(x, xp, fp, left='extrapolate', right='extrapolate')
|
||
Array([-20., 20.], dtype=float32)
|
||
|
||
For periodic interpolation, specify the ``period``:
|
||
|
||
>>> xp = jnp.array([0, jnp.pi / 2, jnp.pi, 3 * jnp.pi / 2])
|
||
>>> fp = jnp.sin(xp)
|
||
>>> x = 2 * jnp.pi # note: not in input array
|
||
>>> jnp.interp(x, xp, fp, period=2 * jnp.pi)
|
||
Array(0., dtype=float32)
|
||
"""
|
||
static_argnames = []
|
||
if isinstance(left, str) or left is None:
|
||
static_argnames.append('left')
|
||
if isinstance(right, str) or right is None:
|
||
static_argnames.append('right')
|
||
if period is None:
|
||
static_argnames.append('period')
|
||
jitted_interp = jit(_interp, static_argnames=static_argnames)
|
||
return jitted_interp(x, xp, fp, left, right, period)
|
||
|
||
|
||
@overload
|
||
def where(condition: ArrayLike, x: Literal[None] = None,
|
||
y: Literal[None] = None, /, *, size: int | None = None,
|
||
fill_value: None | ArrayLike | tuple[ArrayLike, ...] = None
|
||
) -> tuple[Array, ...]: ...
|
||
|
||
@overload
|
||
def where(condition: ArrayLike, x: ArrayLike, y: ArrayLike, / ,*,
|
||
size: int | None = None,
|
||
fill_value: None | ArrayLike | tuple[ArrayLike, ...] = None
|
||
) -> Array: ...
|
||
|
||
@overload
|
||
def where(condition: ArrayLike, x: ArrayLike | None = None,
|
||
y: ArrayLike | None = None, /, *, size: int | None = None,
|
||
fill_value: None | ArrayLike | tuple[ArrayLike, ...] = None
|
||
) -> Array | tuple[Array, ...]: ...
|
||
|
||
|
||
@export
|
||
def where(condition, x=None, y=None, /, *, size=None, fill_value=None):
|
||
"""Select elements from two arrays based on a condition.
|
||
|
||
JAX implementation of :func:`numpy.where`.
|
||
|
||
.. note::
|
||
when only ``condition`` is provided, ``jnp.where(condition)`` is equivalent
|
||
to ``jnp.nonzero(condition)``. For that case, refer to the documentation of
|
||
:func:`jax.numpy.nonzero`. The docstring below focuses on the case where
|
||
``x`` and ``y`` are specified.
|
||
|
||
The three-term version of ``jnp.where`` lowers to :func:`jax.lax.select`.
|
||
|
||
Args:
|
||
condition: boolean array. Must be broadcast-compatible with ``x`` and ``y`` when
|
||
they are specified.
|
||
x: arraylike. Should be broadcast-compatible with ``condition`` and ``y``, and
|
||
typecast-compatible with ``y``.
|
||
y: arraylike. Should be broadcast-compatible with ``condition`` and ``x``, and
|
||
typecast-compatible with ``x``.
|
||
size: integer, only referenced when ``x`` and ``y`` are ``None``. For details,
|
||
see :func:`jax.numpy.nonzero`.
|
||
fill_value: only referenced when ``x`` and ``y`` are ``None``. For details,
|
||
see :func:`jax.numpy.nonzero`.
|
||
|
||
Returns:
|
||
An array of dtype ``jnp.result_type(x, y)`` with values drawn from ``x`` where ``condition``
|
||
is True, and from ``y`` where condition is ``False``. If ``x`` and ``y`` are ``None``, the
|
||
function behaves differently; see :func:`jax.numpy.nonzero` for a description of the return
|
||
type.
|
||
|
||
See Also:
|
||
- :func:`jax.numpy.nonzero`
|
||
- :func:`jax.numpy.argwhere`
|
||
- :func:`jax.lax.select`
|
||
|
||
Notes:
|
||
Special care is needed when the ``x`` or ``y`` input to :func:`jax.numpy.where` could
|
||
have a value of NaN. Specifically, when a gradient is taken with :func:`jax.grad`
|
||
(reverse-mode differentiation), a NaN in either ``x`` or ``y`` will propagate into the
|
||
gradient, regardless of the value of ``condition``. More information on this behavior
|
||
and workarounds is available in the `JAX FAQ
|
||
<https://jax.readthedocs.io/en/latest/faq.html#gradients-contain-nan-where-using-where>`_.
|
||
|
||
Examples:
|
||
When ``x`` and ``y`` are not provided, ``where`` behaves equivalently to
|
||
:func:`jax.numpy.nonzero`:
|
||
|
||
>>> x = jnp.arange(10)
|
||
>>> jnp.where(x > 4)
|
||
(Array([5, 6, 7, 8, 9], dtype=int32),)
|
||
>>> jnp.nonzero(x > 4)
|
||
(Array([5, 6, 7, 8, 9], dtype=int32),)
|
||
|
||
When ``x`` and ``y`` are provided, ``where`` selects between them based on
|
||
the specified condition:
|
||
|
||
>>> jnp.where(x > 4, x, 0)
|
||
Array([0, 0, 0, 0, 0, 5, 6, 7, 8, 9], dtype=int32)
|
||
"""
|
||
if x is None and y is None:
|
||
util.check_arraylike("where", condition)
|
||
return nonzero(condition, size=size, fill_value=fill_value)
|
||
else:
|
||
util.check_arraylike("where", condition, x, y)
|
||
if size is not None or fill_value is not None:
|
||
raise ValueError("size and fill_value arguments cannot be used in "
|
||
"three-term where function.")
|
||
if x is None or y is None:
|
||
raise ValueError("Either both or neither of the x and y arguments "
|
||
"should be provided to jax.numpy.where, got "
|
||
f"{x} and {y}.")
|
||
return util._where(condition, x, y)
|
||
|
||
|
||
@export
|
||
def select(
|
||
condlist: Sequence[ArrayLike],
|
||
choicelist: Sequence[ArrayLike],
|
||
default: ArrayLike = 0,
|
||
) -> Array:
|
||
"""Select values based on a series of conditions.
|
||
|
||
JAX implementation of :func:`numpy.select`, implemented in terms
|
||
of :func:`jax.lax.select_n`
|
||
|
||
Args:
|
||
condlist: sequence of array-like conditions. All entries must be mutually
|
||
broadcast-compatible.
|
||
choicelist: sequence of array-like values to choose. Must have the same length
|
||
as ``condlist``, and all entries must be broadcast-compatible with entries
|
||
of ``condlist``.
|
||
default: value to return when every condition is False (default: 0).
|
||
|
||
Returns:
|
||
Array of selected values from ``choicelist`` corresponding to the first
|
||
``True`` entry in ``condlist`` at each location.
|
||
|
||
See also:
|
||
- :func:`jax.numpy.where`: select between two values based on a single condition.
|
||
- :func:`jax.lax.select_n`: select between *N* values based on an index.
|
||
|
||
Examples:
|
||
>>> condlist = [
|
||
... jnp.array([False, True, False, False]),
|
||
... jnp.array([True, False, False, False]),
|
||
... jnp.array([False, True, True, False]),
|
||
... ]
|
||
>>> choicelist = [
|
||
... jnp.array([1, 2, 3, 4]),
|
||
... jnp.array([10, 20, 30, 40]),
|
||
... jnp.array([100, 200, 300, 400]),
|
||
... ]
|
||
>>> jnp.select(condlist, choicelist, default=0)
|
||
Array([ 10, 2, 300, 0], dtype=int32)
|
||
|
||
This is logically equivalent to the following nested ``where`` statement:
|
||
|
||
>>> default = 0
|
||
>>> jnp.where(condlist[0],
|
||
... choicelist[0],
|
||
... jnp.where(condlist[1],
|
||
... choicelist[1],
|
||
... jnp.where(condlist[2],
|
||
... choicelist[2],
|
||
... default)))
|
||
Array([ 10, 2, 300, 0], dtype=int32)
|
||
|
||
However, for efficiency it is implemented in terms of :func:`jax.lax.select_n`.
|
||
"""
|
||
if len(condlist) != len(choicelist):
|
||
msg = "condlist must have length equal to choicelist ({} vs {})"
|
||
raise ValueError(msg.format(len(condlist), len(choicelist)))
|
||
if len(condlist) == 0:
|
||
raise ValueError("condlist must be non-empty")
|
||
# Put the default at front with condition False because
|
||
# argmax returns zero for an array of False values.
|
||
choicelist = util.promote_dtypes(default, *choicelist)
|
||
conditions = stack(broadcast_arrays(False, *condlist))
|
||
idx = argmax(conditions.astype(bool), axis=0)
|
||
return lax.select_n(*broadcast_arrays(idx, *choicelist))
|
||
|
||
|
||
@export
|
||
def bincount(x: ArrayLike, weights: ArrayLike | None = None,
|
||
minlength: int = 0, *, length: int | None = None
|
||
) -> Array:
|
||
"""Count the number of occurrences of each value in an integer array.
|
||
|
||
JAX implementation of :func:`numpy.bincount`.
|
||
|
||
For an array of positive integers ``x``, this function returns an array ``counts``
|
||
of size ``x.max() + 1``, such that ``counts[i]`` contains the number of occurrences
|
||
of the value ``i`` in ``x``.
|
||
|
||
The JAX version has a few differences from the NumPy version:
|
||
|
||
- In NumPy, passing an array ``x`` with negative entries will result in an error.
|
||
In JAX, negative values are clipped to zero.
|
||
- JAX adds an optional ``length`` parameter which can be used to statically specify
|
||
the length of the output array so that this function can be used with transformations
|
||
like :func:`jax.jit`. In this case, items larger than `length + 1` will be dropped.
|
||
|
||
Args:
|
||
x : N-dimensional array of positive integers
|
||
weights: optional array of weights associated with ``x``. If not specified, the
|
||
weight for each entry will be ``1``.
|
||
minlength: the minimum length of the output counts array.
|
||
length: the length of the output counts array. Must be specified statically for
|
||
``bincount`` to be used with :func:`jax.jit` and other JAX transformations.
|
||
|
||
Returns:
|
||
An array of counts or summed weights reflecting the number of occurrences of values
|
||
in ``x``.
|
||
|
||
See Also:
|
||
- :func:`jax.numpy.histogram`
|
||
- :func:`jax.numpy.digitize`
|
||
- :func:`jax.numpy.unique_counts`
|
||
|
||
Examples:
|
||
Basic bincount:
|
||
|
||
>>> x = jnp.array([1, 1, 2, 3, 3, 3])
|
||
>>> jnp.bincount(x)
|
||
Array([0, 2, 1, 3], dtype=int32)
|
||
|
||
Weighted bincount:
|
||
|
||
>>> weights = jnp.array([1, 2, 3, 4, 5, 6])
|
||
>>> jnp.bincount(x, weights)
|
||
Array([ 0, 3, 3, 15], dtype=int32)
|
||
|
||
Specifying a static ``length`` makes this jit-compatible:
|
||
|
||
>>> jit_bincount = jax.jit(jnp.bincount, static_argnames=['length'])
|
||
>>> jit_bincount(x, length=5)
|
||
Array([0, 2, 1, 3, 0], dtype=int32)
|
||
|
||
Any negative numbers are clipped to the first bin, and numbers beyond the
|
||
specified ``length`` are dropped:
|
||
|
||
>>> x = jnp.array([-1, -1, 1, 3, 10])
|
||
>>> jnp.bincount(x, length=5)
|
||
Array([2, 1, 0, 1, 0], dtype=int32)
|
||
"""
|
||
util.check_arraylike("bincount", x)
|
||
if _dtype(x) == bool:
|
||
x = lax.convert_element_type(x, 'int32')
|
||
if not issubdtype(_dtype(x), np.integer):
|
||
raise TypeError(f"x argument to bincount must have an integer type; got {_dtype(x)}")
|
||
if np.ndim(x) != 1:
|
||
raise ValueError("only 1-dimensional input supported.")
|
||
minlength = core.concrete_or_error(operator.index, minlength,
|
||
"The error occurred because of argument 'minlength' of jnp.bincount.")
|
||
if length is None:
|
||
x_arr = core.concrete_or_error(asarray, x,
|
||
"The error occurred because of argument 'x' of jnp.bincount. "
|
||
"To avoid this error, pass a static `length` argument.")
|
||
length = max(minlength, x_arr.size and int(max(0, x_arr.max())) + 1)
|
||
else:
|
||
length = core.concrete_dim_or_error(length,
|
||
"The error occurred because of argument 'length' of jnp.bincount.")
|
||
if weights is None:
|
||
weights = np.array(1, dtype=dtypes.int_)
|
||
elif np.shape(x) != np.shape(weights):
|
||
raise ValueError("shape of weights must match shape of x.")
|
||
return zeros(length, _dtype(weights)).at[clip(x, 0)].add(weights, mode='drop')
|
||
|
||
@overload
|
||
def broadcast_shapes(*shapes: Sequence[int]) -> tuple[int, ...]: ...
|
||
|
||
@overload
|
||
def broadcast_shapes(*shapes: Sequence[int | core.Tracer]
|
||
) -> tuple[int | core.Tracer, ...]: ...
|
||
|
||
@export
|
||
def broadcast_shapes(*shapes):
|
||
"""Broadcast input shapes to a common output shape.
|
||
|
||
JAX implementation of :func:`numpy.broadcast_shapes`. JAX uses NumPy-style
|
||
broadcasting rules, which you can read more about at `NumPy broadcasting`_.
|
||
|
||
Args:
|
||
shapes: 0 or more shapes specified as sequences of integers
|
||
|
||
Returns:
|
||
The broadcasted shape as a tuple of integers.
|
||
|
||
See Also:
|
||
- :func:`jax.numpy.broadcast_arrays`: broadcast arrays to a common shape.
|
||
- :func:`jax.numpy.broadcast_to`: broadcast an array to a specified shape.
|
||
|
||
Examples:
|
||
Some compatible shapes:
|
||
|
||
>>> jnp.broadcast_shapes((1,), (4,))
|
||
(4,)
|
||
>>> jnp.broadcast_shapes((3, 1), (4,))
|
||
(3, 4)
|
||
>>> jnp.broadcast_shapes((3, 1), (1, 4), (5, 1, 1))
|
||
(5, 3, 4)
|
||
|
||
Incompatible shapes:
|
||
|
||
>>> jnp.broadcast_shapes((3, 1), (4, 1)) # doctest: +IGNORE_EXCEPTION_DETAIL
|
||
Traceback (most recent call last):
|
||
ValueError: Incompatible shapes for broadcasting: shapes=[(3, 1), (4, 1)]
|
||
|
||
.. _NumPy broadcasting: https://numpy.org/doc/stable/user/basics.broadcasting.html
|
||
"""
|
||
if not shapes:
|
||
return ()
|
||
shapes = [(shape,) if np.ndim(shape) == 0 else tuple(shape) for shape in shapes]
|
||
return lax.broadcast_shapes(*shapes)
|
||
|
||
|
||
@export
|
||
def broadcast_arrays(*args: ArrayLike) -> list[Array]:
|
||
"""Broadcast arrays to a common shape.
|
||
|
||
JAX implementation of :func:`numpy.broadcast_arrays`. JAX uses NumPy-style
|
||
broadcasting rules, which you can read more about at `NumPy broadcasting`_.
|
||
|
||
Args:
|
||
args: zero or more array-like objects to be broadcasted.
|
||
|
||
Returns:
|
||
a list of arrays containing broadcasted copies of the inputs.
|
||
|
||
See also:
|
||
- :func:`jax.numpy.broadcast_shapes`: broadcast input shapes to a common shape.
|
||
- :func:`jax.numpy.broadcast_to`: broadcast an array to a specified shape.
|
||
|
||
Examples:
|
||
|
||
>>> x = jnp.arange(3)
|
||
>>> y = jnp.int32(1)
|
||
>>> jnp.broadcast_arrays(x, y)
|
||
[Array([0, 1, 2], dtype=int32), Array([1, 1, 1], dtype=int32)]
|
||
|
||
>>> x = jnp.array([[1, 2, 3]])
|
||
>>> y = jnp.array([[10],
|
||
... [20]])
|
||
>>> x2, y2 = jnp.broadcast_arrays(x, y)
|
||
>>> x2
|
||
Array([[1, 2, 3],
|
||
[1, 2, 3]], dtype=int32)
|
||
>>> y2
|
||
Array([[10, 10, 10],
|
||
[20, 20, 20]], dtype=int32)
|
||
|
||
.. _NumPy broadcasting: https://numpy.org/doc/stable/user/basics.broadcasting.html
|
||
"""
|
||
return util._broadcast_arrays(*args)
|
||
|
||
|
||
@export
|
||
def broadcast_to(array: ArrayLike, shape: DimSize | Shape) -> Array:
|
||
"""Broadcast an array to a specified shape.
|
||
|
||
JAX implementation of :func:`numpy.broadcast_to`. JAX uses NumPy-style
|
||
broadcasting rules, which you can read more about at `NumPy broadcasting`_.
|
||
|
||
Args:
|
||
array: array to be broadcast.
|
||
shape: shape to which the array will be broadcast.
|
||
|
||
Returns:
|
||
a copy of array broadcast to the specified shape.
|
||
|
||
See also:
|
||
- :func:`jax.numpy.broadcast_arrays`: broadcast arrays to a common shape.
|
||
- :func:`jax.numpy.broadcast_shapes`: broadcast input shapes to a common shape.
|
||
|
||
Examples:
|
||
>>> x = jnp.int32(1)
|
||
>>> jnp.broadcast_to(x, (1, 4))
|
||
Array([[1, 1, 1, 1]], dtype=int32)
|
||
|
||
>>> x = jnp.array([1, 2, 3])
|
||
>>> jnp.broadcast_to(x, (2, 3))
|
||
Array([[1, 2, 3],
|
||
[1, 2, 3]], dtype=int32)
|
||
|
||
>>> x = jnp.array([[2], [4]])
|
||
>>> jnp.broadcast_to(x, (2, 4))
|
||
Array([[2, 2, 2, 2],
|
||
[4, 4, 4, 4]], dtype=int32)
|
||
|
||
.. _NumPy broadcasting: https://numpy.org/doc/stable/user/basics.broadcasting.html
|
||
"""
|
||
return util._broadcast_to(array, shape)
|
||
|
||
|
||
def _split(op: str, ary: ArrayLike,
|
||
indices_or_sections: int | Sequence[int] | ArrayLike,
|
||
axis: int = 0) -> list[Array]:
|
||
ary = util.ensure_arraylike(op, ary)
|
||
axis = core.concrete_or_error(operator.index, axis, f"in jax.numpy.{op} argument `axis`")
|
||
size = ary.shape[axis]
|
||
if (isinstance(indices_or_sections, (tuple, list)) or
|
||
isinstance(indices_or_sections, (np.ndarray, Array)) and
|
||
indices_or_sections.ndim > 0):
|
||
split_indices = np.asarray([0] + [
|
||
core.concrete_dim_or_error(i_s, f"in jax.numpy.{op} argument 1")
|
||
for i_s in indices_or_sections] + [size])
|
||
sizes = list(np.diff(split_indices))
|
||
else:
|
||
if core.is_symbolic_dim(indices_or_sections):
|
||
raise ValueError(f"jax.numpy.{op} with a symbolic number of sections is "
|
||
"not supported")
|
||
num_sections: int = core.concrete_or_error(int, indices_or_sections,
|
||
f"in jax.numpy.{op} argument 1")
|
||
part_size, r = divmod(size, num_sections)
|
||
if r == 0:
|
||
sizes = [part_size] * num_sections
|
||
elif op == "array_split":
|
||
sizes = [(part_size + 1)] * r + [part_size] * (num_sections - r)
|
||
else:
|
||
raise ValueError(f"array split does not result in an equal division: rest is {r}")
|
||
sizes = [i if core.is_symbolic_dim(i) else np.int64(i)
|
||
for i in sizes]
|
||
return list(lax.split(ary, sizes, axis=axis))
|
||
|
||
|
||
@export
|
||
def split(ary: ArrayLike, indices_or_sections: int | Sequence[int] | ArrayLike,
|
||
axis: int = 0) -> list[Array]:
|
||
"""Split an array into sub-arrays.
|
||
|
||
JAX implementation of :func:`numpy.split`.
|
||
|
||
Args:
|
||
ary: N-dimensional array-like object to split
|
||
indices_or_sections: either a single integer or a sequence of indices.
|
||
|
||
- if ``indices_or_sections`` is an integer *N*, then *N* must evenly divide
|
||
``ary.shape[axis]`` and ``ary`` will be divided into *N* equally-sized
|
||
chunks along ``axis``.
|
||
- if ``indices_or_sections`` is a sequence of integers, then these integers
|
||
specify the boundary between unevenly-sized chunks along ``axis``; see
|
||
examples below.
|
||
|
||
axis: the axis along which to split; defaults to 0.
|
||
|
||
Returns:
|
||
A list of arrays. If ``indices_or_sections`` is an integer *N*, then the list is
|
||
of length *N*. If ``indices_or_sections`` is a sequence *seq*, then the list is
|
||
is of length *len(seq) + 1*.
|
||
|
||
Examples:
|
||
Splitting a 1-dimensional array:
|
||
|
||
>>> x = jnp.array([1, 2, 3, 4, 5, 6, 7, 8, 9])
|
||
|
||
Split into three equal sections:
|
||
|
||
>>> chunks = jnp.split(x, 3)
|
||
>>> print(*chunks)
|
||
[1 2 3] [4 5 6] [7 8 9]
|
||
|
||
Split into sections by index:
|
||
|
||
>>> chunks = jnp.split(x, [2, 7]) # [x[0:2], x[2:7], x[7:]]
|
||
>>> print(*chunks)
|
||
[1 2] [3 4 5 6 7] [8 9]
|
||
|
||
Splitting a two-dimensional array along axis 1:
|
||
|
||
>>> x = jnp.array([[1, 2, 3, 4],
|
||
... [5, 6, 7, 8]])
|
||
>>> x1, x2 = jnp.split(x, 2, axis=1)
|
||
>>> print(x1)
|
||
[[1 2]
|
||
[5 6]]
|
||
>>> print(x2)
|
||
[[3 4]
|
||
[7 8]]
|
||
|
||
See also:
|
||
- :func:`jax.numpy.array_split`: like ``split``, but allows ``indices_or_sections``
|
||
to be an integer that does not evenly divide the size of the array.
|
||
- :func:`jax.numpy.vsplit`: split vertically, i.e. along axis=0
|
||
- :func:`jax.numpy.hsplit`: split horizontally, i.e. along axis=1
|
||
- :func:`jax.numpy.dsplit`: split depth-wise, i.e. along axis=2
|
||
"""
|
||
return _split("split", ary, indices_or_sections, axis=axis)
|
||
|
||
|
||
@export
|
||
def vsplit(ary: ArrayLike, indices_or_sections: int | Sequence[int] | ArrayLike) -> list[Array]:
|
||
"""Split an array into sub-arrays vertically.
|
||
|
||
JAX implementation of :func:`numpy.vsplit`.
|
||
|
||
Refer to the documentation of :func:`jax.numpy.split` for details; ``vsplit`` is
|
||
equivalent to ``split`` with ``axis=0``.
|
||
|
||
Examples:
|
||
1D array:
|
||
|
||
>>> x = jnp.array([1, 2, 3, 4, 5, 6])
|
||
>>> x1, x2 = jnp.vsplit(x, 2)
|
||
>>> print(x1, x2)
|
||
[1 2 3] [4 5 6]
|
||
|
||
2D array:
|
||
|
||
>>> x = jnp.array([[1, 2, 3, 4],
|
||
... [5, 6, 7, 8]])
|
||
>>> x1, x2 = jnp.vsplit(x, 2)
|
||
>>> print(x1, x2)
|
||
[[1 2 3 4]] [[5 6 7 8]]
|
||
|
||
See also:
|
||
- :func:`jax.numpy.split`: split an array along any axis.
|
||
- :func:`jax.numpy.hsplit`: split horizontally, i.e. along axis=1
|
||
- :func:`jax.numpy.dsplit`: split depth-wise, i.e. along axis=2
|
||
- :func:`jax.numpy.array_split`: like ``split``, but allows ``indices_or_sections``
|
||
to be an integer that does not evenly divide the size of the array.
|
||
"""
|
||
return _split("vsplit", ary, indices_or_sections, axis=0)
|
||
|
||
|
||
@export
|
||
def hsplit(ary: ArrayLike, indices_or_sections: int | Sequence[int] | ArrayLike) -> list[Array]:
|
||
"""Split an array into sub-arrays horizontally.
|
||
|
||
JAX implementation of :func:`numpy.hsplit`.
|
||
|
||
Refer to the documentation of :func:`jax.numpy.split` for details. ``hsplit`` is
|
||
equivalent to ``split`` with ``axis=1``, or ``axis=0`` for one-dimensional arrays.
|
||
|
||
Examples:
|
||
1D array:
|
||
|
||
>>> x = jnp.array([1, 2, 3, 4, 5, 6])
|
||
>>> x1, x2 = jnp.hsplit(x, 2)
|
||
>>> print(x1, x2)
|
||
[1 2 3] [4 5 6]
|
||
|
||
2D array:
|
||
|
||
>>> x = jnp.array([[1, 2, 3, 4],
|
||
... [5, 6, 7, 8]])
|
||
>>> x1, x2 = jnp.hsplit(x, 2)
|
||
>>> print(x1)
|
||
[[1 2]
|
||
[5 6]]
|
||
>>> print(x2)
|
||
[[3 4]
|
||
[7 8]]
|
||
|
||
See also:
|
||
- :func:`jax.numpy.split`: split an array along any axis.
|
||
- :func:`jax.numpy.vsplit`: split vertically, i.e. along axis=0
|
||
- :func:`jax.numpy.dsplit`: split depth-wise, i.e. along axis=2
|
||
- :func:`jax.numpy.array_split`: like ``split``, but allows ``indices_or_sections``
|
||
to be an integer that does not evenly divide the size of the array.
|
||
"""
|
||
a = util.ensure_arraylike("hsplit", ary)
|
||
return _split("hsplit", a, indices_or_sections, axis=0 if a.ndim == 1 else 1)
|
||
|
||
|
||
@export
|
||
def dsplit(ary: ArrayLike, indices_or_sections: int | Sequence[int] | ArrayLike) -> list[Array]:
|
||
"""Split an array into sub-arrays depth-wise.
|
||
|
||
JAX implementation of :func:`numpy.dsplit`.
|
||
|
||
Refer to the documentation of :func:`jax.numpy.split` for details. ``dsplit`` is
|
||
equivalent to ``split`` with ``axis=2``.
|
||
|
||
Examples:
|
||
|
||
>>> x = jnp.arange(12).reshape(3, 1, 4)
|
||
>>> print(x)
|
||
[[[ 0 1 2 3]]
|
||
<BLANKLINE>
|
||
[[ 4 5 6 7]]
|
||
<BLANKLINE>
|
||
[[ 8 9 10 11]]]
|
||
>>> x1, x2 = jnp.dsplit(x, 2)
|
||
>>> print(x1)
|
||
[[[0 1]]
|
||
<BLANKLINE>
|
||
[[4 5]]
|
||
<BLANKLINE>
|
||
[[8 9]]]
|
||
>>> print(x2)
|
||
[[[ 2 3]]
|
||
<BLANKLINE>
|
||
[[ 6 7]]
|
||
<BLANKLINE>
|
||
[[10 11]]]
|
||
|
||
See also:
|
||
- :func:`jax.numpy.split`: split an array along any axis.
|
||
- :func:`jax.numpy.vsplit`: split vertically, i.e. along axis=0
|
||
- :func:`jax.numpy.hsplit`: split horizontally, i.e. along axis=1
|
||
- :func:`jax.numpy.array_split`: like ``split``, but allows ``indices_or_sections``
|
||
to be an integer that does not evenly divide the size of the array.
|
||
"""
|
||
return _split("dsplit", ary, indices_or_sections, axis=2)
|
||
|
||
|
||
@export
|
||
def array_split(ary: ArrayLike, indices_or_sections: int | Sequence[int] | ArrayLike,
|
||
axis: int = 0) -> list[Array]:
|
||
"""Split an array into sub-arrays.
|
||
|
||
JAX implementation of :func:`numpy.array_split`.
|
||
|
||
Refer to the documentation of :func:`jax.numpy.split` for details; ``array_split``
|
||
is equivalent to ``split``, but allows integer ``indices_or_sections`` which does
|
||
not evenly divide the split axis.
|
||
|
||
Examples:
|
||
>>> x = jnp.array([1, 2, 3, 4, 5, 6, 7, 8, 9])
|
||
>>> chunks = jnp.array_split(x, 4)
|
||
>>> print(*chunks)
|
||
[1 2 3] [4 5] [6 7] [8 9]
|
||
|
||
See also:
|
||
- :func:`jax.numpy.split`: split an array along any axis.
|
||
- :func:`jax.numpy.vsplit`: split vertically, i.e. along axis=0
|
||
- :func:`jax.numpy.hsplit`: split horizontally, i.e. along axis=1
|
||
- :func:`jax.numpy.dsplit`: split depth-wise, i.e. along axis=2
|
||
"""
|
||
return _split("array_split", ary, indices_or_sections, axis=axis)
|
||
|
||
|
||
@export
|
||
@jit
|
||
def clip(
|
||
arr: ArrayLike | None = None,
|
||
/,
|
||
min: ArrayLike | None = None,
|
||
max: ArrayLike | None = None,
|
||
*,
|
||
a: ArrayLike | DeprecatedArg = DeprecatedArg(),
|
||
a_min: ArrayLike | None | DeprecatedArg = DeprecatedArg(),
|
||
a_max: ArrayLike | None | DeprecatedArg = DeprecatedArg()
|
||
) -> Array:
|
||
"""Clip array values to a specified range.
|
||
|
||
JAX implementation of :func:`numpy.clip`.
|
||
|
||
Args:
|
||
arr: N-dimensional array to be clipped.
|
||
min: optional minimum value of the clipped range; if ``None`` (default) then
|
||
result will not be clipped to any minimum value. If specified, it should be
|
||
broadcast-compatible with ``arr`` and ``max``.
|
||
max: optional maximum value of the clipped range; if ``None`` (default) then
|
||
result will not be clipped to any maximum value. If specified, it should be
|
||
broadcast-compatible with ``arr`` and ``min``.
|
||
a: deprecated alias of the ``arr`` argument. Will result in a
|
||
:class:`DeprecationWarning` if used.
|
||
a_min: deprecated alias of the ``min`` argument. Will result in a
|
||
:class:`DeprecationWarning` if used.
|
||
a_max: deprecated alias of the ``max`` argument. Will result in a
|
||
:class:`DeprecationWarning` if used.
|
||
|
||
Returns:
|
||
An array containing values from ``arr``, with values smaller than ``min`` set
|
||
to ``min``, and values larger than ``max`` set to ``max``.
|
||
|
||
See also:
|
||
- :func:`jax.numpy.minimum`: Compute the element-wise minimum value of two arrays.
|
||
- :func:`jax.numpy.maximum`: Compute the element-wise maximum value of two arrays.
|
||
|
||
Examples:
|
||
>>> arr = jnp.array([0, 1, 2, 3, 4, 5, 6, 7])
|
||
>>> jnp.clip(arr, 2, 5)
|
||
Array([2, 2, 2, 3, 4, 5, 5, 5], dtype=int32)
|
||
"""
|
||
# TODO(micky774): deprecated 2024-4-2, remove after deprecation expires.
|
||
arr = a if not isinstance(a, DeprecatedArg) else arr
|
||
if arr is None:
|
||
raise ValueError("No input was provided to the clip function.")
|
||
min = a_min if not isinstance(a_min, DeprecatedArg) else min
|
||
max = a_max if not isinstance(a_max, DeprecatedArg) else max
|
||
if any(not isinstance(t, DeprecatedArg) for t in (a, a_min, a_max)):
|
||
deprecations.warn(
|
||
"jax-numpy-clip-args",
|
||
("Passing arguments 'a', 'a_min' or 'a_max' to jax.numpy.clip is "
|
||
"deprecated. Please use 'arr', 'min' or 'max' respectively instead."),
|
||
stacklevel=2,
|
||
)
|
||
|
||
util.check_arraylike("clip", arr)
|
||
if any(jax.numpy.iscomplexobj(t) for t in (arr, min, max)):
|
||
raise ValueError(
|
||
"Clip received a complex value either through the input or the min/max "
|
||
"keywords. Complex values have no ordering and cannot be clipped. "
|
||
"Please convert to a real value or array by taking the real or "
|
||
"imaginary components via jax.numpy.real/imag respectively.")
|
||
if min is not None:
|
||
arr = ufuncs.maximum(min, arr)
|
||
if max is not None:
|
||
arr = ufuncs.minimum(max, arr)
|
||
return asarray(arr)
|
||
|
||
|
||
@export
|
||
@partial(jit, static_argnames=('decimals',))
|
||
def round(a: ArrayLike, decimals: int = 0, out: None = None) -> Array:
|
||
"""Round input evenly to the given number of decimals.
|
||
|
||
JAX implementation of :func:`numpy.round`.
|
||
|
||
Args:
|
||
a: input array or scalar.
|
||
decimals: int, default=0. Number of decimal points to which the input needs
|
||
to be rounded. It must be specified statically. Not implemented for
|
||
``decimals < 0``.
|
||
out: Unused by JAX.
|
||
|
||
Returns:
|
||
An array containing the rounded values to the specified ``decimals`` with
|
||
same shape and dtype as ``a``.
|
||
|
||
Note:
|
||
``jnp.round`` rounds to the nearest even integer for the values exactly halfway
|
||
between rounded decimal values.
|
||
|
||
See also:
|
||
- :func:`jax.numpy.floor`: Rounds the input to the nearest integer downwards.
|
||
- :func:`jax.numpy.ceil`: Rounds the input to the nearest integer upwards.
|
||
- :func:`jax.numpy.fix` and :func:numpy.trunc`: Rounds the input to the
|
||
nearest integer towards zero.
|
||
|
||
Examples:
|
||
>>> x = jnp.array([1.532, 3.267, 6.149])
|
||
>>> jnp.round(x)
|
||
Array([2., 3., 6.], dtype=float32)
|
||
>>> jnp.round(x, decimals=2)
|
||
Array([1.53, 3.27, 6.15], dtype=float32)
|
||
|
||
For values exactly halfway between rounded values:
|
||
|
||
>>> x1 = jnp.array([10.5, 21.5, 12.5, 31.5])
|
||
>>> jnp.round(x1)
|
||
Array([10., 22., 12., 32.], dtype=float32)
|
||
"""
|
||
a = util.ensure_arraylike("round", a)
|
||
decimals = core.concrete_or_error(operator.index, decimals, "'decimals' argument of jnp.round")
|
||
if out is not None:
|
||
raise NotImplementedError("The 'out' argument to jnp.round is not supported.")
|
||
dtype = _dtype(a)
|
||
if issubdtype(dtype, np.integer):
|
||
if decimals < 0:
|
||
raise NotImplementedError(
|
||
"integer np.round not implemented for decimals < 0")
|
||
return a # no-op on integer types
|
||
|
||
def _round_float(x: ArrayLike) -> Array:
|
||
if decimals == 0:
|
||
return lax.round(x, lax.RoundingMethod.TO_NEAREST_EVEN)
|
||
|
||
# TODO(phawkins): the strategy of rescaling the value isn't necessarily a
|
||
# good one since we may be left with an incorrectly rounded value at the
|
||
# end due to precision problems. As a workaround for float16, convert to
|
||
# float32,
|
||
x = lax.convert_element_type(x, np.float32) if dtype == np.float16 else x
|
||
factor = _lax_const(x, 10 ** decimals)
|
||
out = lax.div(lax.round(lax.mul(x, factor),
|
||
lax.RoundingMethod.TO_NEAREST_EVEN), factor)
|
||
return lax.convert_element_type(out, dtype) if dtype == np.float16 else out
|
||
|
||
if issubdtype(dtype, np.complexfloating):
|
||
return lax.complex(_round_float(lax.real(a)), _round_float(lax.imag(a)))
|
||
else:
|
||
return _round_float(a)
|
||
|
||
|
||
@export
|
||
@partial(jit, static_argnames=('decimals',))
|
||
def around(a: ArrayLike, decimals: int = 0, out: None = None) -> Array:
|
||
"""Alias of :func:`jax.numpy.round`"""
|
||
return round(a, decimals, out)
|
||
|
||
|
||
@export
|
||
@jit
|
||
def fix(x: ArrayLike, out: None = None) -> Array:
|
||
"""Round input to the nearest integer towards zero.
|
||
|
||
JAX implementation of :func:`numpy.fix`.
|
||
|
||
Args:
|
||
x: input array.
|
||
out: unused by JAX.
|
||
|
||
Returns:
|
||
An array with same shape and dtype as ``x`` containing the rounded values.
|
||
|
||
See also:
|
||
- :func:`jax.numpy.trunc`: Rounds the input to nearest integer towards zero.
|
||
- :func:`jax.numpy.ceil`: Rounds the input up to the nearest integer.
|
||
- :func:`jax.numpy.floor`: Rounds the input down to the nearest integer.
|
||
|
||
Examples:
|
||
>>> key = jax.random.key(0)
|
||
>>> x = jax.random.uniform(key, (3, 3), minval=-5, maxval=5)
|
||
>>> with jnp.printoptions(precision=2, suppress=True):
|
||
... print(x)
|
||
[[ 4.48 4.79 -1.68]
|
||
[-0.31 0.7 -3.34]
|
||
[-1.9 1.89 2.47]]
|
||
>>> jnp.fix(x)
|
||
Array([[ 4., 4., -1.],
|
||
[-0., 0., -3.],
|
||
[-1., 1., 2.]], dtype=float32)
|
||
"""
|
||
util.check_arraylike("fix", x)
|
||
if out is not None:
|
||
raise NotImplementedError("The 'out' argument to jnp.fix is not supported.")
|
||
zero = _lax_const(x, 0)
|
||
return where(lax.ge(x, zero), ufuncs.floor(x), ufuncs.ceil(x))
|
||
|
||
|
||
@export
|
||
@jit
|
||
def nan_to_num(x: ArrayLike, copy: bool = True, nan: ArrayLike = 0.0,
|
||
posinf: ArrayLike | None = None,
|
||
neginf: ArrayLike | None = None) -> Array:
|
||
"""Replace NaN and infinite entries in an array.
|
||
|
||
JAX implementation of :func:`numpy.nan_to_num`.
|
||
|
||
Args:
|
||
x: array of values to be replaced. If it does not have an inexact
|
||
dtype it will be returned unmodified.
|
||
copy: unused by JAX
|
||
nan: value to substitute for NaN entries. Defaults to 0.0.
|
||
posinf: value to substitute for positive infinite entries.
|
||
Defaults to the maximum representable value.
|
||
neginf: value to substitute for positive infinite entries.
|
||
Defaults to the minimum representable value.
|
||
|
||
Returns:
|
||
A copy of ``x`` with the requested substitutions.
|
||
|
||
See also:
|
||
- :func:`jax.numpy.isnan`: return True where the array contains NaN
|
||
- :func:`jax.numpy.isposinf`: return True where the array contains +inf
|
||
- :func:`jax.numpy.isneginf`: return True where the array contains -inf
|
||
|
||
Examples:
|
||
>>> x = jnp.array([0, jnp.nan, 1, jnp.inf, 2, -jnp.inf])
|
||
|
||
Default substitution values:
|
||
|
||
>>> jnp.nan_to_num(x)
|
||
Array([ 0.0000000e+00, 0.0000000e+00, 1.0000000e+00, 3.4028235e+38,
|
||
2.0000000e+00, -3.4028235e+38], dtype=float32)
|
||
|
||
Overriding substitutions for ``-inf`` and ``+inf``:
|
||
|
||
>>> jnp.nan_to_num(x, posinf=999, neginf=-999)
|
||
Array([ 0., 0., 1., 999., 2., -999.], dtype=float32)
|
||
|
||
If you only wish to substitute for NaN values while leaving ``inf`` values
|
||
untouched, using :func:`~jax.numpy.where` with :func:`jax.numpy.isnan` is
|
||
a better option:
|
||
|
||
>>> jnp.where(jnp.isnan(x), 0, x)
|
||
Array([ 0., 0., 1., inf, 2., -inf], dtype=float32)
|
||
"""
|
||
del copy
|
||
x = util.ensure_arraylike("nan_to_num", x)
|
||
dtype = _dtype(x)
|
||
if not issubdtype(dtype, np.inexact):
|
||
return x
|
||
if issubdtype(dtype, np.complexfloating):
|
||
return lax.complex(
|
||
nan_to_num(lax.real(x), nan=nan, posinf=posinf, neginf=neginf),
|
||
nan_to_num(lax.imag(x), nan=nan, posinf=posinf, neginf=neginf))
|
||
info = finfo(dtypes.canonicalize_dtype(dtype))
|
||
posinf = info.max if posinf is None else posinf
|
||
neginf = info.min if neginf is None else neginf
|
||
out = where(ufuncs.isnan(x), asarray(nan, dtype=dtype), x)
|
||
out = where(ufuncs.isposinf(out), asarray(posinf, dtype=dtype), out)
|
||
out = where(ufuncs.isneginf(out), asarray(neginf, dtype=dtype), out)
|
||
return out
|
||
|
||
|
||
@export
|
||
@partial(jit, static_argnames=('equal_nan',))
|
||
def allclose(a: ArrayLike, b: ArrayLike, rtol: ArrayLike = 1e-05,
|
||
atol: ArrayLike = 1e-08, equal_nan: bool = False) -> Array:
|
||
r"""Check if two arrays are element-wise approximately equal within a tolerance.
|
||
|
||
JAX implementation of :func:`numpy.allclose`.
|
||
|
||
Essentially this function evaluates the following condition:
|
||
|
||
.. math::
|
||
|
||
|a - b| \le \mathtt{atol} + \mathtt{rtol} * |b|
|
||
|
||
``jnp.inf`` in ``a`` will be considered equal to ``jnp.inf`` in ``b``.
|
||
|
||
Args:
|
||
a: first input array to compare.
|
||
b: second input array to compare.
|
||
rtol: relative tolerance used for approximate equality. Default = 1e-05.
|
||
atol: absolute tolerance used for approximate equality. Default = 1e-08.
|
||
equal_nan: Boolean. If ``True``, NaNs in ``a`` will be considered
|
||
equal to NaNs in ``b``. Default is ``False``.
|
||
|
||
Returns:
|
||
Boolean scalar array indicating whether the input arrays are element-wise
|
||
approximately equal within the specified tolerances.
|
||
|
||
See Also:
|
||
- :func:`jax.numpy.isclose`
|
||
- :func:`jax.numpy.equal`
|
||
|
||
Examples:
|
||
>>> jnp.allclose(jnp.array([1e6, 2e6, 3e6]), jnp.array([1e6, 2e6, 3e7]))
|
||
Array(False, dtype=bool)
|
||
>>> jnp.allclose(jnp.array([1e6, 2e6, 3e6]),
|
||
... jnp.array([1.00008e6, 2.00008e7, 3.00008e8]), rtol=1e3)
|
||
Array(True, dtype=bool)
|
||
>>> jnp.allclose(jnp.array([1e6, 2e6, 3e6]),
|
||
... jnp.array([1.00001e6, 2.00002e6, 3.00009e6]), atol=1e3)
|
||
Array(True, dtype=bool)
|
||
>>> jnp.allclose(jnp.array([jnp.nan, 1, 2]),
|
||
... jnp.array([jnp.nan, 1, 2]), equal_nan=True)
|
||
Array(True, dtype=bool)
|
||
"""
|
||
util.check_arraylike("allclose", a, b)
|
||
return reductions.all(isclose(a, b, rtol, atol, equal_nan))
|
||
|
||
|
||
@export
|
||
def nonzero(a: ArrayLike, *, size: int | None = None,
|
||
fill_value: None | ArrayLike | tuple[ArrayLike, ...] = None
|
||
) -> tuple[Array, ...]:
|
||
"""Return indices of nonzero elements of an array.
|
||
|
||
JAX implementation of :func:`numpy.nonzero`.
|
||
|
||
Because the size of the output of ``nonzero`` is data-dependent, the function
|
||
is not compatible with JIT and other transformations. The JAX version adds
|
||
the optional ``size`` argument which must be specified statically for
|
||
``jnp.nonzero`` to be used within JAX's transformations.
|
||
|
||
Args:
|
||
a: N-dimensional array.
|
||
size: optional static integer specifying the number of nonzero entries to
|
||
return. If there are more nonzero elements than the specified ``size``,
|
||
then indices will be truncated at the end. If there are fewer nonzero
|
||
elements than the specified size, then indices will be padded with
|
||
``fill_value``, which defaults to zero.
|
||
fill_value: optional padding value when ``size`` is specified. Defaults to 0.
|
||
|
||
Returns:
|
||
Tuple of JAX Arrays of length ``a.ndim``, containing the indices of each
|
||
nonzero value.
|
||
|
||
See also:
|
||
- :func:`jax.numpy.flatnonzero`
|
||
- :func:`jax.numpy.where`
|
||
|
||
Examples:
|
||
|
||
One-dimensional array returns a length-1 tuple of indices:
|
||
|
||
>>> x = jnp.array([0, 5, 0, 6, 0, 7])
|
||
>>> jnp.nonzero(x)
|
||
(Array([1, 3, 5], dtype=int32),)
|
||
|
||
Two-dimensional array returns a length-2 tuple of indices:
|
||
|
||
>>> x = jnp.array([[0, 5, 0],
|
||
... [6, 0, 7]])
|
||
>>> jnp.nonzero(x)
|
||
(Array([0, 1, 1], dtype=int32), Array([1, 0, 2], dtype=int32))
|
||
|
||
In either case, the resulting tuple of indices can be used directly to extract
|
||
the nonzero values:
|
||
|
||
>>> indices = jnp.nonzero(x)
|
||
>>> x[indices]
|
||
Array([5, 6, 7], dtype=int32)
|
||
|
||
The output of ``nonzero`` has a dynamic shape, because the number of returned
|
||
indices depends on the contents of the input array. As such, it is incompatible
|
||
with JIT and other JAX transformations:
|
||
|
||
>>> x = jnp.array([0, 5, 0, 6, 0, 7])
|
||
>>> jax.jit(jnp.nonzero)(x) # doctest: +IGNORE_EXCEPTION_DETAIL
|
||
Traceback (most recent call last):
|
||
...
|
||
ConcretizationTypeError: Abstract tracer value encountered where concrete value is expected: traced array with shape int32[].
|
||
The size argument of jnp.nonzero must be statically specified to use jnp.nonzero within JAX transformations.
|
||
|
||
This can be addressed by passing a static ``size`` parameter to specify the
|
||
desired output shape:
|
||
|
||
>>> nonzero_jit = jax.jit(jnp.nonzero, static_argnames='size')
|
||
>>> nonzero_jit(x, size=3)
|
||
(Array([1, 3, 5], dtype=int32),)
|
||
|
||
If ``size`` does not match the true size, the result will be either truncated or padded:
|
||
|
||
>>> nonzero_jit(x, size=2) # size < 3: indices are truncated
|
||
(Array([1, 3], dtype=int32),)
|
||
>>> nonzero_jit(x, size=5) # size > 3: indices are padded with zeros.
|
||
(Array([1, 3, 5, 0, 0], dtype=int32),)
|
||
|
||
You can specify a custom fill value for the padding using the ``fill_value`` argument:
|
||
|
||
>>> nonzero_jit(x, size=5, fill_value=len(x))
|
||
(Array([1, 3, 5, 6, 6], dtype=int32),)
|
||
"""
|
||
arr = util.ensure_arraylike("nonzero", a)
|
||
del a
|
||
if np.ndim(arr) == 0:
|
||
raise ValueError("Calling nonzero on 0d arrays is not allowed. "
|
||
"Use jnp.atleast_1d(scalar).nonzero() instead.")
|
||
mask = arr if arr.dtype == bool else (arr != 0)
|
||
calculated_size_ = mask.sum() if size is None else size
|
||
calculated_size: int = core.concrete_dim_or_error(calculated_size_,
|
||
"The size argument of jnp.nonzero must be statically specified "
|
||
"to use jnp.nonzero within JAX transformations.")
|
||
if arr.size == 0 or calculated_size == 0:
|
||
return tuple(zeros(calculated_size, int) for dim in arr.shape)
|
||
flat_indices = reductions.cumsum(
|
||
bincount(reductions.cumsum(mask), length=calculated_size))
|
||
strides: np.ndarray = (np.cumprod(arr.shape[::-1])[::-1] // arr.shape).astype(dtypes.int_)
|
||
out = tuple((flat_indices // stride) % size for stride, size in zip(strides, arr.shape))
|
||
if fill_value is not None:
|
||
fill_value_tup = fill_value if isinstance(fill_value, tuple) else arr.ndim * (fill_value,)
|
||
if any(np.shape(val) != () for val in fill_value_tup):
|
||
raise ValueError(f"fill_value must be a scalar or a tuple of length {arr.ndim}; got {fill_value}")
|
||
fill_mask = arange(calculated_size) >= mask.sum()
|
||
out = tuple(where(fill_mask, fval, entry) for fval, entry in safe_zip(fill_value_tup, out))
|
||
return out
|
||
|
||
|
||
@export
|
||
def flatnonzero(a: ArrayLike, *, size: int | None = None,
|
||
fill_value: None | ArrayLike | tuple[ArrayLike, ...] = None) -> Array:
|
||
"""Return indices of nonzero elements in a flattened array
|
||
|
||
JAX implementation of :func:`numpy.flatnonzero`.
|
||
|
||
``jnp.flatnonzero(x)`` is equivalent to ``nonzero(ravel(a))[0]``. For a full
|
||
discussion of the parameters to this function, refer to :func:`jax.numpy.nonzero`.
|
||
|
||
Args:
|
||
a: N-dimensional array.
|
||
size: optional static integer specifying the number of nonzero entries to
|
||
return. See :func:`jax.numpy.nonzero` for more discussion of this parameter.
|
||
fill_value: optional padding value when ``size`` is specified. Defaults to 0.
|
||
See :func:`jax.numpy.nonzero` for more discussion of this parameter.
|
||
|
||
Returns:
|
||
Array containing the indices of each nonzero value in the flattened array.
|
||
|
||
See Also:
|
||
- :func:`jax.numpy.nonzero`
|
||
- :func:`jax.numpy.where`
|
||
|
||
Examples:
|
||
>>> x = jnp.array([[0, 5, 0],
|
||
... [6, 0, 8]])
|
||
>>> jnp.flatnonzero(x)
|
||
Array([1, 3, 5], dtype=int32)
|
||
|
||
This is equivalent to calling :func:`~jax.numpy.nonzero` on the flattened
|
||
array, and extracting the first entry in the resulting tuple:
|
||
|
||
>>> jnp.nonzero(x.ravel())[0]
|
||
Array([1, 3, 5], dtype=int32)
|
||
|
||
The returned indices can be used to extract nonzero entries from the
|
||
flattened array:
|
||
|
||
>>> indices = jnp.flatnonzero(x)
|
||
>>> x.ravel()[indices]
|
||
Array([5, 6, 8], dtype=int32)
|
||
"""
|
||
return nonzero(ravel(a), size=size, fill_value=fill_value)[0]
|
||
|
||
|
||
@export
|
||
@partial(jit, static_argnames=('axis',))
|
||
def unwrap(p: ArrayLike, discont: ArrayLike | None = None,
|
||
axis: int = -1, period: ArrayLike = 2 * np.pi) -> Array:
|
||
"""Unwrap a periodic signal.
|
||
|
||
JAX implementation of :func:`numpy.unwrap`.
|
||
|
||
Args:
|
||
p: input array
|
||
discont: the maximum allowable discontinuity in the sequence. The
|
||
default is ``period / 2``
|
||
axis: the axis along which to unwrap; defaults to -1
|
||
period: the period of the signal, which defaults to :math:`2\\pi`
|
||
|
||
Returns:
|
||
An unwrapped copy of ``p``.
|
||
|
||
Examples:
|
||
Consider a situation in which you are making measurements of the position of
|
||
a rotating disk via the ``x`` and ``y`` locations of some point on that disk.
|
||
The underlying variable is an always-increating angle which we'll generate
|
||
this way, using degrees for ease of representation:
|
||
|
||
>>> rng = np.random.default_rng(0)
|
||
>>> theta = rng.integers(0, 90, size=(20,)).cumsum()
|
||
>>> theta
|
||
array([ 76, 133, 179, 203, 230, 233, 239, 240, 255, 328, 386, 468, 513,
|
||
567, 654, 719, 775, 823, 873, 957])
|
||
|
||
Our observations of this angle are the ``x`` and ``y`` coordinates, given by
|
||
the sine and cosine of this underlying angle:
|
||
|
||
>>> x, y = jnp.sin(jnp.deg2rad(theta)), jnp.cos(jnp.deg2rad(theta))
|
||
|
||
Now, say that given these ``x`` and ``y`` coordinates, we wish to recover
|
||
the original angle ``theta``. We might do this via the :func:`atan2` function:
|
||
|
||
>>> theta_out = jnp.rad2deg(jnp.atan2(x, y)).round()
|
||
>>> theta_out
|
||
Array([ 76., 133., 179., -157., -130., -127., -121., -120., -105.,
|
||
-32., 26., 108., 153., -153., -66., -1., 55., 103.,
|
||
153., -123.], dtype=float32)
|
||
|
||
The first few values match the input angle ``theta`` above, but after this the
|
||
values are wrapped because the ``sin`` and ``cos`` observations obscure the phase
|
||
information. The purpose of the :func:`unwrap` function is to recover the original
|
||
signal from this wrapped view of it:
|
||
|
||
>>> jnp.unwrap(theta_out, period=360)
|
||
Array([ 76., 133., 179., 203., 230., 233., 239., 240., 255., 328., 386.,
|
||
468., 513., 567., 654., 719., 775., 823., 873., 957.], dtype=float32)
|
||
|
||
It does this by assuming that the true underlying sequence does not differ by more than
|
||
``discont`` (which defaults to ``period / 2``) within a single step, and when it encounters
|
||
a larger discontinuity it adds factors of the period to the data. For periodic signals
|
||
that satisfy this assumption, :func:`unwrap` can recover the original phased signal.
|
||
"""
|
||
p = util.ensure_arraylike("unwrap", p)
|
||
if issubdtype(p.dtype, np.complexfloating):
|
||
raise ValueError("jnp.unwrap does not support complex inputs.")
|
||
if p.shape[axis] == 0:
|
||
return util.promote_dtypes_inexact(p)[0]
|
||
if discont is None:
|
||
discont = period / 2
|
||
interval = period / 2
|
||
dd = diff(p, axis=axis)
|
||
ddmod = ufuncs.mod(dd + interval, period) - interval
|
||
ddmod = where((ddmod == -interval) & (dd > 0), interval, ddmod)
|
||
|
||
ph_correct = where(ufuncs.abs(dd) < discont, 0, ddmod - dd)
|
||
|
||
up = concatenate((
|
||
lax.slice_in_dim(p, 0, 1, axis=axis),
|
||
lax.slice_in_dim(p, 1, None, axis=axis) + reductions.cumsum(ph_correct, axis=axis)
|
||
), axis=axis)
|
||
|
||
return up
|
||
|
||
|
||
### Padding
|
||
|
||
PadValueLike = Union[T, Sequence[T], Sequence[Sequence[T]]]
|
||
PadValue = tuple[tuple[T, T], ...]
|
||
|
||
class PadStatFunc(Protocol):
|
||
def __call__(self, array: ArrayLike, /, *,
|
||
axis: int | None = None,
|
||
keepdims: bool = False) -> Array: ...
|
||
|
||
|
||
def _broadcast_to_pairs(nvals: PadValueLike, nd: int, name: str) -> PadValue:
|
||
try:
|
||
nvals = np.asarray(tree_map(
|
||
lambda x: core.concrete_or_error(None, x, context=f"{name} argument of jnp.pad"),
|
||
nvals))
|
||
except ValueError as e:
|
||
# In numpy 1.24
|
||
if "array has an inhomogeneous shape" in str(e):
|
||
raise TypeError(f'`{name}` entries must be the same shape: {nvals}') from e
|
||
raise
|
||
|
||
def as_scalar_dim(v):
|
||
if core.is_dim(v) or not np.shape(v):
|
||
return v
|
||
else:
|
||
raise TypeError(f'`{name}` entries must be the same shape: {nvals}')
|
||
|
||
if nvals.shape == (nd, 2):
|
||
# ((before_1, after_1), ..., (before_N, after_N))
|
||
return tuple((as_scalar_dim(nval[0]), as_scalar_dim(nval[1])) for nval in nvals)
|
||
elif nvals.shape == (1, 2):
|
||
# ((before, after),)
|
||
v1_2 = as_scalar_dim(nvals[0, 0]), as_scalar_dim(nvals[0, 1])
|
||
return tuple(v1_2 for i in range(nd))
|
||
elif nvals.shape == (2,):
|
||
# (before, after) (not in the numpy docstring but works anyway)
|
||
v1_2 = as_scalar_dim(nvals[0]), as_scalar_dim(nvals[1])
|
||
return tuple(v1_2 for i in range(nd))
|
||
elif nvals.shape == (1,):
|
||
# (pad,)
|
||
v = as_scalar_dim(nvals[0])
|
||
return tuple((v, v) for i in range(nd))
|
||
elif nvals.shape == ():
|
||
# pad
|
||
v = as_scalar_dim(nvals.flat[0])
|
||
return tuple((v, v) for i in range(nd))
|
||
else:
|
||
raise ValueError(f"jnp.pad: {name} with {nd=} has unsupported shape {nvals.shape}. "
|
||
f"Valid shapes are ({nd}, 2), (1, 2), (2,), (1,), or ().")
|
||
|
||
|
||
def _check_no_padding(axis_padding: tuple[Any, Any], mode: str):
|
||
if (axis_padding[0] > 0 or axis_padding[1] > 0):
|
||
msg = "Cannot apply '{}' padding to empty axis"
|
||
raise ValueError(msg.format(mode))
|
||
|
||
|
||
def _pad_constant(array: Array, pad_width: PadValue[int], constant_values: Array) -> Array:
|
||
nd = np.ndim(array)
|
||
constant_values = lax_internal._convert_element_type(
|
||
constant_values, array.dtype, dtypes.is_weakly_typed(array))
|
||
constant_values_nd = np.ndim(constant_values)
|
||
|
||
if constant_values_nd == 0:
|
||
widths = [(low, high, 0) for (low, high) in pad_width]
|
||
return lax.pad(array, constant_values, widths)
|
||
|
||
if constant_values_nd == 1:
|
||
if constant_values.shape[-1] == 1:
|
||
widths = [(low, high, 0) for (low, high) in pad_width]
|
||
return lax.pad(array, squeeze(constant_values), widths)
|
||
elif constant_values.shape[-1] != 2:
|
||
raise ValueError("jnp.pad: constant_values has unsupported shape "
|
||
f"{constant_values.shape}. If the shape is 1D or 2D, the "
|
||
"last dimension must be of size 1 or 2.")
|
||
|
||
constant_values = broadcast_to(constant_values, (nd, 2))
|
||
for i in range(nd):
|
||
widths = [(0, 0, 0)] * nd
|
||
if pad_width[i][0] != 0:
|
||
widths[i] = (pad_width[i][0], 0, 0)
|
||
array = lax.pad(array, constant_values[i, 0], widths)
|
||
if pad_width[i][1] != 0:
|
||
widths[i] = (0, pad_width[i][1], 0)
|
||
array = lax.pad(array, constant_values[i, 1], widths)
|
||
return array
|
||
|
||
|
||
def _pad_wrap(array: Array, pad_width: PadValue[int]) -> Array:
|
||
for i in range(np.ndim(array)):
|
||
if array.shape[i] == 0:
|
||
_check_no_padding(pad_width[i], "wrap")
|
||
continue
|
||
size = array.shape[i]
|
||
left_repeats, left_remainder = divmod(pad_width[i][0], size)
|
||
right_repeats, right_remainder = divmod(pad_width[i][1], size)
|
||
total_repeats = left_repeats + right_repeats + 1
|
||
parts = []
|
||
if left_remainder > 0:
|
||
parts += [lax.slice_in_dim(array, size - left_remainder, size, axis=i)]
|
||
parts += total_repeats * [array]
|
||
if right_remainder > 0:
|
||
parts += [lax.slice_in_dim(array, 0, right_remainder, axis=i)]
|
||
array = lax.concatenate(parts, dimension=i)
|
||
return array
|
||
|
||
|
||
def _pad_symmetric_or_reflect(array: Array, pad_width: PadValue[int],
|
||
mode: str, reflect_type: str) -> Array:
|
||
assert mode in ("symmetric", "reflect")
|
||
assert reflect_type in ("even", "odd")
|
||
|
||
for i in range(np.ndim(array)):
|
||
if array.shape[i] == 0:
|
||
_check_no_padding(pad_width[i], mode)
|
||
continue
|
||
|
||
axis_size = array.shape[i]
|
||
|
||
def build_padding(array, padding, before):
|
||
if before:
|
||
edge = lax.slice_in_dim(array, 0, 1, axis=i)
|
||
else:
|
||
edge = lax.slice_in_dim(array, -1, None, axis=i)
|
||
|
||
# Try to give nicer error messages for unsupported shape polymorphic uses
|
||
shape_poly_error_msg = lambda: (
|
||
"Shape polymorphism is supported for jnp.pad with 'reflect' or "
|
||
"'symmetric' padding mode only when it is possible to determine "
|
||
f"at lowering time that the axis size (= {axis_size}) is larger than 1 "
|
||
f"and larger or equal than the padding length (= {padding}). "
|
||
f"Error while handling {'left' if before else 'right'} padding on axis {i}.")
|
||
try:
|
||
# We check that we can determine all comparisons.
|
||
offset = 1 if (mode == "reflect" and axis_size > 1) else 0
|
||
has_poly_dim = not core.is_constant_shape((axis_size, padding))
|
||
# For shape polymorphism, ensure the loop below ends after 1 iteration
|
||
if has_poly_dim and not (axis_size > 1 and axis_size - offset >= padding):
|
||
raise ValueError(shape_poly_error_msg())
|
||
except core.InconclusiveDimensionOperation as e:
|
||
raise ValueError(shape_poly_error_msg()) from e
|
||
|
||
while padding > 0:
|
||
curr_pad = min(padding, axis_size - offset)
|
||
padding -= curr_pad
|
||
if has_poly_dim: assert padding == 0
|
||
|
||
if before:
|
||
start = offset
|
||
stop = offset + curr_pad
|
||
else:
|
||
start = -(curr_pad + offset)
|
||
stop = None if (mode == "symmetric" or axis_size == 1) else -1
|
||
|
||
x = lax.slice_in_dim(array, start, stop, axis=i)
|
||
x = flip(x, axis=i)
|
||
|
||
if reflect_type == 'odd':
|
||
x = 2 * edge - x
|
||
if axis_size > 1:
|
||
if before:
|
||
edge = lax.slice_in_dim(x, 0, 1, axis=i)
|
||
else:
|
||
edge = lax.slice_in_dim(x, -1, None, axis=i)
|
||
|
||
if before:
|
||
array = lax.concatenate([x, array], dimension=i)
|
||
else:
|
||
array = lax.concatenate([array, x], dimension=i)
|
||
return array
|
||
|
||
array = build_padding(array, pad_width[i][0], before=True)
|
||
array = build_padding(array, pad_width[i][1], before=False)
|
||
return array
|
||
|
||
|
||
def _pad_edge(array: Array, pad_width: PadValue[int]) -> Array:
|
||
nd = np.ndim(array)
|
||
for i in range(nd):
|
||
if array.shape[i] == 0:
|
||
_check_no_padding(pad_width[i], "edge")
|
||
continue
|
||
|
||
n = array.shape[i]
|
||
npad_before, npad_after = pad_width[i]
|
||
|
||
edge_before = lax.slice_in_dim(array, 0, 1, axis=i)
|
||
pad_before = repeat(edge_before, npad_before, axis=i)
|
||
|
||
edge_after = lax.slice_in_dim(array, n-1, n, axis=i)
|
||
pad_after = repeat(edge_after, npad_after, axis=i)
|
||
|
||
array = lax.concatenate([pad_before, array, pad_after], dimension=i)
|
||
return array
|
||
|
||
|
||
def _pad_linear_ramp(array: Array, pad_width: PadValue[int],
|
||
end_values: PadValue[ArrayLike]) -> Array:
|
||
for axis in range(np.ndim(array)):
|
||
edge_before = lax.slice_in_dim(array, 0, 1, axis=axis)
|
||
edge_after = lax.slice_in_dim(array, -1, None, axis=axis)
|
||
ramp_before = linspace(
|
||
start=end_values[axis][0],
|
||
stop=edge_before.squeeze(axis), # Dimension is replaced by linspace
|
||
num=pad_width[axis][0],
|
||
endpoint=False,
|
||
dtype=array.dtype,
|
||
axis=axis
|
||
)
|
||
ramp_before = lax_internal._convert_element_type(
|
||
ramp_before, weak_type=dtypes.is_weakly_typed(array))
|
||
ramp_after = linspace(
|
||
start=end_values[axis][1],
|
||
stop=edge_after.squeeze(axis), # Dimension is replaced by linspace
|
||
num=pad_width[axis][1],
|
||
endpoint=False,
|
||
dtype=array.dtype,
|
||
axis=axis
|
||
)
|
||
ramp_after = lax_internal._convert_element_type(
|
||
ramp_after, weak_type=dtypes.is_weakly_typed(array))
|
||
|
||
# Reverse linear space in appropriate dimension
|
||
ramp_after = flip(ramp_after, axis)
|
||
|
||
array = lax.concatenate([ramp_before, array, ramp_after], dimension=axis)
|
||
return array
|
||
|
||
|
||
def _pad_stats(array: Array, pad_width: PadValue[int],
|
||
stat_length: PadValue[int] | None,
|
||
stat_func: PadStatFunc) -> Array:
|
||
nd = np.ndim(array)
|
||
for i in range(nd):
|
||
if stat_length is None:
|
||
stat_before = stat_func(array, axis=i, keepdims=True)
|
||
stat_after = stat_before
|
||
else:
|
||
array_length = array.shape[i]
|
||
length_before, length_after = stat_length[i]
|
||
if length_before == 0 or length_after == 0:
|
||
raise ValueError("stat_length of 0 yields no value for padding")
|
||
|
||
# Limit stat_length to length of array.
|
||
length_before = min(length_before, array_length)
|
||
length_after = min(length_after, array_length)
|
||
|
||
slice_before = lax.slice_in_dim(array, 0, length_before, axis=i)
|
||
slice_after = lax.slice_in_dim(array, -length_after, None, axis=i)
|
||
stat_before = stat_func(slice_before, axis=i, keepdims=True)
|
||
stat_after = stat_func(slice_after, axis=i, keepdims=True)
|
||
|
||
if np.issubdtype(array.dtype, np.integer):
|
||
stat_before = round(stat_before)
|
||
stat_after = round(stat_after)
|
||
|
||
stat_before = lax_internal._convert_element_type(
|
||
stat_before, array.dtype, dtypes.is_weakly_typed(array))
|
||
stat_after = lax_internal._convert_element_type(
|
||
stat_after, array.dtype, dtypes.is_weakly_typed(array))
|
||
|
||
npad_before, npad_after = pad_width[i]
|
||
pad_before = repeat(stat_before, npad_before, axis=i)
|
||
pad_after = repeat(stat_after, npad_after, axis=i)
|
||
|
||
array = lax.concatenate([pad_before, array, pad_after], dimension=i)
|
||
return array
|
||
|
||
|
||
def _pad_empty(array: Array, pad_width: PadValue[int]) -> Array:
|
||
# Note: jax.numpy.empty = jax.numpy.zeros
|
||
for i in range(np.ndim(array)):
|
||
shape_before = array.shape[:i] + (pad_width[i][0],) + array.shape[i + 1:]
|
||
pad_before = empty_like(array, shape=shape_before)
|
||
|
||
shape_after = array.shape[:i] + (pad_width[i][1],) + array.shape[i + 1:]
|
||
pad_after = empty_like(array, shape=shape_after)
|
||
array = lax.concatenate([pad_before, array, pad_after], dimension=i)
|
||
return array
|
||
|
||
|
||
def _pad_func(array: Array, pad_width: PadValue[int], func: Callable[..., Any], **kwargs) -> Array:
|
||
pad_width = _broadcast_to_pairs(pad_width, np.ndim(array), "pad_width")
|
||
padded = _pad_constant(array, pad_width, asarray(0))
|
||
for axis in range(np.ndim(padded)):
|
||
padded = apply_along_axis(func, axis, padded, pad_width[axis], axis, kwargs)
|
||
return padded
|
||
|
||
|
||
@partial(jit, static_argnums=(1, 2, 4, 5, 6))
|
||
def _pad(array: ArrayLike, pad_width: PadValueLike[int], mode: str,
|
||
constant_values: ArrayLike, stat_length: PadValueLike[int],
|
||
end_values: PadValueLike[ArrayLike], reflect_type: str):
|
||
array = asarray(array)
|
||
nd = np.ndim(array)
|
||
|
||
if nd == 0:
|
||
return array
|
||
|
||
stat_funcs: dict[str, PadStatFunc] = {
|
||
"maximum": reductions.amax,
|
||
"minimum": reductions.amin,
|
||
"mean": reductions.mean,
|
||
"median": reductions.median
|
||
}
|
||
|
||
pad_width = _broadcast_to_pairs(pad_width, nd, "pad_width")
|
||
pad_width_arr = np.array(pad_width)
|
||
if pad_width_arr.shape != (nd, 2):
|
||
raise ValueError(f"Expected pad_width to have shape {(nd, 2)}; got {pad_width_arr.shape}.")
|
||
|
||
if np.any(pad_width_arr < 0):
|
||
raise ValueError("index can't contain negative values")
|
||
|
||
if mode == "constant":
|
||
return _pad_constant(array, pad_width, asarray(constant_values))
|
||
|
||
elif mode == "wrap":
|
||
return _pad_wrap(array, pad_width)
|
||
|
||
elif mode in ("symmetric", "reflect"):
|
||
return _pad_symmetric_or_reflect(array, pad_width, str(mode), reflect_type)
|
||
|
||
elif mode == "edge":
|
||
return _pad_edge(array, pad_width)
|
||
|
||
elif mode == "linear_ramp":
|
||
end_values = _broadcast_to_pairs(end_values, nd, "end_values")
|
||
return _pad_linear_ramp(array, pad_width, end_values)
|
||
|
||
elif mode in stat_funcs:
|
||
if stat_length is not None:
|
||
stat_length = _broadcast_to_pairs(stat_length, nd, "stat_length")
|
||
return _pad_stats(array, pad_width, stat_length, stat_funcs[str(mode)])
|
||
|
||
elif mode == "empty":
|
||
return _pad_empty(array, pad_width)
|
||
|
||
else:
|
||
assert False, ("Should not be reached since pad already handled unsupported and"
|
||
"not implemented modes")
|
||
|
||
|
||
@export
|
||
def pad(array: ArrayLike, pad_width: PadValueLike[int | Array | np.ndarray],
|
||
mode: str | Callable[..., Any] = "constant", **kwargs) -> Array:
|
||
"""Add padding to an array.
|
||
|
||
JAX implementation of :func:`numpy.pad`.
|
||
|
||
Args:
|
||
array: array to pad.
|
||
pad_width: specify the pad width for each dimension of an array. Padding widths
|
||
may be separately specified for *before* and *after* the array. Options are:
|
||
|
||
- ``int`` or ``(int,)``: pad each array dimension with the same number of values
|
||
both before and after.
|
||
- ``(before, after)``: pad each array with ``before`` elements before, and ``after``
|
||
elements after
|
||
- ``((before_1, after_1), (before_2, after_2), ... (before_N, after_N))``: specify
|
||
distinct ``before`` and ``after`` values for each array dimension.
|
||
|
||
mode: a string or callable. Supported pad modes are:
|
||
|
||
- ``'constant'`` (default): pad with a constant value, which defaults to zero.
|
||
- ``'empty'``: pad with empty values (i.e. zero)
|
||
- ``'edge'``: pad with the edge values of the array.
|
||
- ``'wrap'``: pad by wrapping the array.
|
||
- ``'linear_ramp'``: pad with a linear ramp to specified ``end_values``.
|
||
- ``'maximum'``: pad with the maximum value.
|
||
- ``'mean'``: pad with the mean value.
|
||
- ``'median'``: pad with the median value.
|
||
- ``'minimum'``: pad with the minimum value.
|
||
- ``'reflect'``: pad by reflection.
|
||
- ``'symmetric'``: pad by symmetric reflection.
|
||
- ``<callable>``: a callable function. See Notes below.
|
||
|
||
constant_values: referenced for ``mode = 'constant'``. Specify the constant value
|
||
to pad with.
|
||
stat_length: referenced for ``mode in ['maximum', 'mean', 'median', 'minimum']``.
|
||
An integer or tuple specifying the number of edge values to use when calculating
|
||
the statistic.
|
||
end_values: referenced for ``mode = 'linear_ramp'``. Specify the end values to
|
||
ramp the padding values to.
|
||
reflect_type: referenced for ``mode in ['reflect', 'symmetric']``. Specify whether
|
||
to use even or odd reflection.
|
||
|
||
Returns:
|
||
A padded copy of ``array``.
|
||
|
||
Notes:
|
||
When ``mode`` is callable, it should have the following signature::
|
||
|
||
def pad_func(row: Array, pad_width: tuple[int, int],
|
||
iaxis: int, kwargs: dict) -> Array:
|
||
...
|
||
|
||
Here ``row`` is a 1D slice of the padded array along axis ``iaxis``, with the pad
|
||
values filled with zeros. ``pad_width`` is a tuple specifying the ``(before, after)``
|
||
padding sizes, and ``kwargs`` are any additional keyword arguments passed to the
|
||
:func:`jax.numpy.pad` function.
|
||
|
||
Note that while in NumPy, the function should modify ``row`` in-place, in JAX the
|
||
function should return the modified ``row``. In JAX, the custom padding function
|
||
will be mapped across the padded axis using the :func:`jax.vmap` transformation.
|
||
|
||
See also:
|
||
- :func:`jax.numpy.resize`: resize an array
|
||
- :func:`jax.numpy.tile`: create a larger array by tiling a smaller array.
|
||
- :func:`jax.numpy.repeat`: create a larger array by repeating values of a smaller array.
|
||
|
||
Examples:
|
||
|
||
Pad a 1-dimensional array with zeros:
|
||
|
||
>>> x = jnp.array([10, 20, 30, 40])
|
||
>>> jnp.pad(x, 2)
|
||
Array([ 0, 0, 10, 20, 30, 40, 0, 0], dtype=int32)
|
||
>>> jnp.pad(x, (2, 4))
|
||
Array([ 0, 0, 10, 20, 30, 40, 0, 0, 0, 0], dtype=int32)
|
||
|
||
Pad a 1-dimensional array with specified values:
|
||
|
||
>>> jnp.pad(x, 2, constant_values=99)
|
||
Array([99, 99, 10, 20, 30, 40, 99, 99], dtype=int32)
|
||
|
||
Pad a 1-dimensional array with the mean array value:
|
||
|
||
>>> jnp.pad(x, 2, mode='mean')
|
||
Array([25, 25, 10, 20, 30, 40, 25, 25], dtype=int32)
|
||
|
||
Pad a 1-dimensional array with reflected values:
|
||
|
||
>>> jnp.pad(x, 2, mode='reflect')
|
||
Array([30, 20, 10, 20, 30, 40, 30, 20], dtype=int32)
|
||
|
||
Pad a 2-dimensional array with different paddings in each dimension:
|
||
|
||
>>> x = jnp.array([[1, 2, 3],
|
||
... [4, 5, 6]])
|
||
>>> jnp.pad(x, ((1, 2), (3, 0)))
|
||
Array([[0, 0, 0, 0, 0, 0],
|
||
[0, 0, 0, 1, 2, 3],
|
||
[0, 0, 0, 4, 5, 6],
|
||
[0, 0, 0, 0, 0, 0],
|
||
[0, 0, 0, 0, 0, 0]], dtype=int32)
|
||
|
||
Pad a 1-dimensional array with a custom padding function:
|
||
|
||
>>> def custom_pad(row, pad_width, iaxis, kwargs):
|
||
... # row represents a 1D slice of the zero-padded array.
|
||
... before, after = pad_width
|
||
... before_value = kwargs.get('before_value', 0)
|
||
... after_value = kwargs.get('after_value', 0)
|
||
... row = row.at[:before].set(before_value)
|
||
... return row.at[len(row) - after:].set(after_value)
|
||
>>> x = jnp.array([2, 3, 4])
|
||
>>> jnp.pad(x, 2, custom_pad, before_value=-10, after_value=10)
|
||
Array([-10, -10, 2, 3, 4, 10, 10], dtype=int32)
|
||
"""
|
||
|
||
util.check_arraylike("pad", array)
|
||
pad_width = _broadcast_to_pairs(pad_width, np.ndim(array), "pad_width")
|
||
if pad_width and not all(core.is_dim(p[0]) and core.is_dim(p[1])
|
||
for p in pad_width):
|
||
raise TypeError('`pad_width` must be of integral type.')
|
||
|
||
if callable(mode):
|
||
return _pad_func(asarray(array), pad_width, mode, **kwargs)
|
||
|
||
allowed_kwargs = {
|
||
'empty': [], 'edge': [], 'wrap': [],
|
||
'constant': ['constant_values'],
|
||
'linear_ramp': ['end_values'],
|
||
'maximum': ['stat_length'],
|
||
'mean': ['stat_length'],
|
||
'median': ['stat_length'],
|
||
'minimum': ['stat_length'],
|
||
'reflect': ['reflect_type'],
|
||
'symmetric': ['reflect_type'],
|
||
}
|
||
try:
|
||
unsupported_kwargs = set(kwargs) - set(allowed_kwargs[mode])
|
||
except KeyError:
|
||
msg = "Unimplemented padding mode '{}' for np.pad."
|
||
raise NotImplementedError(msg.format(mode))
|
||
if unsupported_kwargs:
|
||
raise ValueError("unsupported keyword arguments for mode '{}': {}"
|
||
.format(mode, unsupported_kwargs))
|
||
# Set default value if not given.
|
||
constant_values = kwargs.get('constant_values', 0)
|
||
stat_length = kwargs.get('stat_length', None)
|
||
end_values = kwargs.get('end_values', 0)
|
||
reflect_type = kwargs.get('reflect_type', "even")
|
||
|
||
return _pad(array, pad_width, mode, constant_values, stat_length, end_values, reflect_type)
|
||
|
||
### Array-creation functions
|
||
|
||
|
||
@export
|
||
def stack(arrays: np.ndarray | Array | Sequence[ArrayLike],
|
||
axis: int = 0, out: None = None, dtype: DTypeLike | None = None) -> Array:
|
||
"""Join arrays along a new axis.
|
||
|
||
JAX implementation of :func:`numpy.stack`.
|
||
|
||
Args:
|
||
arrays: a sequence of arrays to stack; each must have the same shape. If a
|
||
single array is given it will be treated equivalently to
|
||
`arrays = unstack(arrays)`, but the implementation will avoid explicit
|
||
unstacking.
|
||
axis: specify the axis along which to stack.
|
||
out: unused by JAX
|
||
dtype: optional dtype of the resulting array. If not specified, the dtype
|
||
will be determined via type promotion rules described in :ref:`type-promotion`.
|
||
|
||
Returns:
|
||
the stacked result.
|
||
|
||
See also:
|
||
- :func:`jax.numpy.unstack`: inverse of ``stack``.
|
||
- :func:`jax.numpy.concatenate`: concatenation along existing axes.
|
||
- :func:`jax.numpy.vstack`: stack vertically, i.e. along axis 0.
|
||
- :func:`jax.numpy.hstack`: stack horizontally, i.e. along axis 1.
|
||
- :func:`jax.numpy.dstack`: stack depth-wise, i.e. along axis 2.
|
||
- :func:`jax.numpy.column_stack`: stack columns.
|
||
|
||
Examples:
|
||
>>> x = jnp.array([1, 2, 3])
|
||
>>> y = jnp.array([4, 5, 6])
|
||
>>> jnp.stack([x, y])
|
||
Array([[1, 2, 3],
|
||
[4, 5, 6]], dtype=int32)
|
||
>>> jnp.stack([x, y], axis=1)
|
||
Array([[1, 4],
|
||
[2, 5],
|
||
[3, 6]], dtype=int32)
|
||
|
||
:func:`~jax.numpy.unstack` performs the inverse operation:
|
||
|
||
>>> arr = jnp.stack([x, y], axis=1)
|
||
>>> x, y = jnp.unstack(arr, axis=1)
|
||
>>> x
|
||
Array([1, 2, 3], dtype=int32)
|
||
>>> y
|
||
Array([4, 5, 6], dtype=int32)
|
||
"""
|
||
if not len(arrays):
|
||
raise ValueError("Need at least one array to stack.")
|
||
if out is not None:
|
||
raise NotImplementedError("The 'out' argument to jnp.stack is not supported.")
|
||
if isinstance(arrays, (np.ndarray, Array)):
|
||
axis = _canonicalize_axis(axis, arrays.ndim)
|
||
return concatenate(expand_dims(arrays, axis + 1), axis=axis, dtype=dtype)
|
||
else:
|
||
util.check_arraylike("stack", *arrays)
|
||
shape0 = np.shape(arrays[0])
|
||
axis = _canonicalize_axis(axis, len(shape0) + 1)
|
||
new_arrays = []
|
||
for a in arrays:
|
||
if np.shape(a) != shape0:
|
||
raise ValueError("All input arrays must have the same shape.")
|
||
new_arrays.append(expand_dims(a, axis))
|
||
return concatenate(new_arrays, axis=axis, dtype=dtype)
|
||
|
||
|
||
@export
|
||
@partial(jit, static_argnames="axis")
|
||
def unstack(x: ArrayLike, /, *, axis: int = 0) -> tuple[Array, ...]:
|
||
"""Unstack an array along an axis.
|
||
|
||
JAX implementation of :func:`array_api.unstack`.
|
||
|
||
Args:
|
||
x: array to unstack. Must have ``x.ndim >= 1``.
|
||
axis: integer axis along which to unstack. Must satisfy
|
||
``-x.ndim <= axis < x.ndim``.
|
||
|
||
Returns:
|
||
tuple of unstacked arrays.
|
||
|
||
See also:
|
||
- :func:`jax.numpy.stack`: inverse of ``unstack``
|
||
- :func:`jax.numpy.split`: split array into batches along an axis.
|
||
|
||
Examples:
|
||
>>> arr = jnp.array([[1, 2, 3],
|
||
... [4, 5, 6]])
|
||
>>> arrs = jnp.unstack(arr)
|
||
>>> print(*arrs)
|
||
[1 2 3] [4 5 6]
|
||
|
||
:func:`~jax.numpy.stack` provides the inverse of this:
|
||
|
||
>>> jnp.stack(arrs)
|
||
Array([[1, 2, 3],
|
||
[4, 5, 6]], dtype=int32)
|
||
"""
|
||
x = util.ensure_arraylike("unstack", x)
|
||
if x.ndim == 0:
|
||
raise ValueError(
|
||
"Unstack requires arrays with rank > 0, however a scalar array was "
|
||
"passed."
|
||
)
|
||
dimensions = (axis,)
|
||
return tuple(
|
||
lax.squeeze(t, dimensions)
|
||
for t in lax.split(x, (1,) * x.shape[axis], axis=axis)
|
||
)
|
||
|
||
|
||
@export
|
||
def tile(A: ArrayLike, reps: DimSize | Sequence[DimSize]) -> Array:
|
||
"""Construct an array by repeating ``A`` along specified dimensions.
|
||
|
||
JAX implementation of :func:`numpy.tile`.
|
||
|
||
If ``A`` is an array of shape ``(d1, d2, ..., dn)`` and ``reps`` is a sequence of integers,
|
||
the resulting array will have a shape of ``(reps[0] * d1, reps[1] * d2, ..., reps[n] * dn)``,
|
||
with ``A`` tiled along each dimension.
|
||
|
||
Args:
|
||
A: input array to be repeated. Can be of any shape or dimension.
|
||
reps: specifies the number of repetitions along each axis.
|
||
|
||
Returns:
|
||
a new array where the input array has been repeated according to ``reps``.
|
||
|
||
See also:
|
||
- :func:`jax.numpy.repeat`: Construct an array from repeated elements.
|
||
- :func:`jax.numpy.broadcast_to`: Broadcast an array to a specified shape.
|
||
|
||
Examples:
|
||
>>> arr = jnp.array([1, 2])
|
||
>>> jnp.tile(arr, 2)
|
||
Array([1, 2, 1, 2], dtype=int32)
|
||
>>> arr = jnp.array([[1, 2],
|
||
... [3, 4,]])
|
||
>>> jnp.tile(arr, (2, 1))
|
||
Array([[1, 2],
|
||
[3, 4],
|
||
[1, 2],
|
||
[3, 4]], dtype=int32)
|
||
"""
|
||
util.check_arraylike("tile", A)
|
||
try:
|
||
iter(reps) # type: ignore[arg-type]
|
||
except TypeError:
|
||
reps_tup: tuple[DimSize, ...] = (reps,)
|
||
else:
|
||
reps_tup = tuple(reps) # type: ignore[arg-type]
|
||
reps_tup = tuple(operator.index(rep) if core.is_constant_dim(rep) else rep
|
||
for rep in reps_tup)
|
||
A_shape = (1,) * (len(reps_tup) - np.ndim(A)) + np.shape(A)
|
||
reps_tup = (1,) * (len(A_shape) - len(reps_tup)) + reps_tup
|
||
result = broadcast_to(reshape(A, [j for i in A_shape for j in [1, i]]),
|
||
[k for pair in zip(reps_tup, A_shape) for k in pair])
|
||
return reshape(result, tuple(np.multiply(A_shape, reps_tup)))
|
||
|
||
def _concatenate_array(arr: ArrayLike, axis: int | None,
|
||
dtype: DTypeLike | None = None) -> Array:
|
||
# Fast path for concatenation when the input is an ndarray rather than a list.
|
||
arr = asarray(arr, dtype=dtype)
|
||
if arr.ndim == 0 or arr.shape[0] == 0:
|
||
raise ValueError("Need at least one array to concatenate.")
|
||
if axis is None:
|
||
return lax.reshape(arr, (arr.size,))
|
||
if arr.ndim == 1:
|
||
raise ValueError("Zero-dimensional arrays cannot be concatenated.")
|
||
axis = _canonicalize_axis(axis, arr.ndim - 1)
|
||
shape = arr.shape[1:axis + 1] + (arr.shape[0] * arr.shape[axis + 1],) + arr.shape[axis + 2:]
|
||
dimensions = [*range(1, axis + 1), 0, *range(axis + 1, arr.ndim)]
|
||
return lax.reshape(arr, shape, dimensions)
|
||
|
||
|
||
@export
|
||
def concatenate(arrays: np.ndarray | Array | Sequence[ArrayLike],
|
||
axis: int | None = 0, dtype: DTypeLike | None = None) -> Array:
|
||
"""Join arrays along an existing axis.
|
||
|
||
JAX implementation of :func:`numpy.concatenate`.
|
||
|
||
Args:
|
||
arrays: a sequence of arrays to concatenate; each must have the same shape
|
||
except along the specified axis. If a single array is given it will be
|
||
treated equivalently to `arrays = unstack(arrays)`, but the implementation
|
||
will avoid explicit unstacking.
|
||
axis: specify the axis along which to concatenate.
|
||
dtype: optional dtype of the resulting array. If not specified, the dtype
|
||
will be determined via type promotion rules described in :ref:`type-promotion`.
|
||
|
||
Returns:
|
||
the concatenated result.
|
||
|
||
See also:
|
||
- :func:`jax.lax.concatenate`: XLA concatenation API.
|
||
- :func:`jax.numpy.concat`: Array API version of this function.
|
||
- :func:`jax.numpy.stack`: concatenate arrays along a new axis.
|
||
|
||
Examples:
|
||
One-dimensional concatenation:
|
||
|
||
>>> x = jnp.arange(3)
|
||
>>> y = jnp.zeros(3, dtype=int)
|
||
>>> jnp.concatenate([x, y])
|
||
Array([0, 1, 2, 0, 0, 0], dtype=int32)
|
||
|
||
Two-dimensional concatenation:
|
||
|
||
>>> x = jnp.ones((2, 3))
|
||
>>> y = jnp.zeros((2, 1))
|
||
>>> jnp.concatenate([x, y], axis=1)
|
||
Array([[1., 1., 1., 0.],
|
||
[1., 1., 1., 0.]], dtype=float32)
|
||
"""
|
||
if isinstance(arrays, (np.ndarray, Array)):
|
||
return _concatenate_array(arrays, axis, dtype=dtype)
|
||
util.check_arraylike("concatenate", *arrays)
|
||
if not len(arrays):
|
||
raise ValueError("Need at least one array to concatenate.")
|
||
if axis is None:
|
||
return concatenate([ravel(a) for a in arrays], axis=0, dtype=dtype)
|
||
if np.ndim(arrays[0]) == 0:
|
||
raise ValueError("Zero-dimensional arrays cannot be concatenated.")
|
||
axis = _canonicalize_axis(axis, np.ndim(arrays[0]))
|
||
if dtype is None:
|
||
arrays_out = util.promote_dtypes(*arrays)
|
||
else:
|
||
arrays_out = [asarray(arr, dtype=dtype) for arr in arrays]
|
||
# lax.concatenate can be slow to compile for wide concatenations, so form a
|
||
# tree of concatenations as a workaround especially for op-by-op mode.
|
||
# (https://github.com/jax-ml/jax/issues/653).
|
||
k = 16
|
||
while len(arrays_out) > 1:
|
||
arrays_out = [lax.concatenate(arrays_out[i:i+k], axis)
|
||
for i in range(0, len(arrays_out), k)]
|
||
return arrays_out[0]
|
||
|
||
|
||
@export
|
||
def concat(arrays: Sequence[ArrayLike], /, *, axis: int | None = 0) -> Array:
|
||
"""Join arrays along an existing axis.
|
||
|
||
JAX implementation of :func:`array_api.concat`.
|
||
|
||
Args:
|
||
arrays: a sequence of arrays to concatenate; each must have the same shape
|
||
except along the specified axis. If a single array is given it will be
|
||
treated equivalently to `arrays = unstack(arrays)`, but the implementation
|
||
will avoid explicit unstacking.
|
||
axis: specify the axis along which to concatenate.
|
||
|
||
Returns:
|
||
the concatenated result.
|
||
|
||
See also:
|
||
- :func:`jax.lax.concatenate`: XLA concatenation API.
|
||
- :func:`jax.numpy.concatenate`: NumPy version of this function.
|
||
- :func:`jax.numpy.stack`: concatenate arrays along a new axis.
|
||
|
||
Examples:
|
||
One-dimensional concatenation:
|
||
|
||
>>> x = jnp.arange(3)
|
||
>>> y = jnp.zeros(3, dtype=int)
|
||
>>> jnp.concat([x, y])
|
||
Array([0, 1, 2, 0, 0, 0], dtype=int32)
|
||
|
||
Two-dimensional concatenation:
|
||
|
||
>>> x = jnp.ones((2, 3))
|
||
>>> y = jnp.zeros((2, 1))
|
||
>>> jnp.concat([x, y], axis=1)
|
||
Array([[1., 1., 1., 0.],
|
||
[1., 1., 1., 0.]], dtype=float32)
|
||
"""
|
||
util.check_arraylike("concat", *arrays)
|
||
return jax.numpy.concatenate(arrays, axis=axis)
|
||
|
||
|
||
@export
|
||
def vstack(tup: np.ndarray | Array | Sequence[ArrayLike],
|
||
dtype: DTypeLike | None = None) -> Array:
|
||
"""Vertically stack arrays.
|
||
|
||
JAX implementation of :func:`numpy.vstack`.
|
||
|
||
For arrays of two or more dimensions, this is equivalent to
|
||
:func:`jax.numpy.concatenate` with ``axis=0``.
|
||
|
||
Args:
|
||
tup: a sequence of arrays to stack; each must have the same shape along all
|
||
but the first axis. If a single array is given it will be treated
|
||
equivalently to `tup = unstack(tup)`, but the implementation will avoid
|
||
explicit unstacking.
|
||
dtype: optional dtype of the resulting array. If not specified, the dtype
|
||
will be determined via type promotion rules described in :ref:`type-promotion`.
|
||
|
||
Returns:
|
||
the stacked result.
|
||
|
||
See also:
|
||
- :func:`jax.numpy.stack`: stack along arbitrary axes
|
||
- :func:`jax.numpy.concatenate`: concatenation along existing axes.
|
||
- :func:`jax.numpy.hstack`: stack horizontally, i.e. along axis 1.
|
||
- :func:`jax.numpy.dstack`: stack depth-wise, i.e. along axis 2.
|
||
|
||
Examples:
|
||
Scalar values:
|
||
|
||
>>> jnp.vstack([1, 2, 3])
|
||
Array([[1],
|
||
[2],
|
||
[3]], dtype=int32, weak_type=True)
|
||
|
||
1D arrays:
|
||
|
||
>>> x = jnp.arange(4)
|
||
>>> y = jnp.ones(4)
|
||
>>> jnp.vstack([x, y])
|
||
Array([[0., 1., 2., 3.],
|
||
[1., 1., 1., 1.]], dtype=float32)
|
||
|
||
2D arrays:
|
||
|
||
>>> x = x.reshape(1, 4)
|
||
>>> y = y.reshape(1, 4)
|
||
>>> jnp.vstack([x, y])
|
||
Array([[0., 1., 2., 3.],
|
||
[1., 1., 1., 1.]], dtype=float32)
|
||
"""
|
||
arrs: Array | list[Array]
|
||
if isinstance(tup, (np.ndarray, Array)):
|
||
arrs = jax.vmap(atleast_2d)(tup)
|
||
else:
|
||
# TODO(jakevdp): Non-array input deprecated 2023-09-22; change to error.
|
||
util.check_arraylike("vstack", *tup, emit_warning=True)
|
||
arrs = [atleast_2d(m) for m in tup]
|
||
return concatenate(arrs, axis=0, dtype=dtype)
|
||
|
||
|
||
@export
|
||
def hstack(tup: np.ndarray | Array | Sequence[ArrayLike],
|
||
dtype: DTypeLike | None = None) -> Array:
|
||
"""Horizontally stack arrays.
|
||
|
||
JAX implementation of :func:`numpy.hstack`.
|
||
|
||
For arrays of one or more dimensions, this is equivalent to
|
||
:func:`jax.numpy.concatenate` with ``axis=1``.
|
||
|
||
Args:
|
||
tup: a sequence of arrays to stack; each must have the same shape along all
|
||
but the second axis. Input arrays will be promoted to at least rank 1.
|
||
If a single array is given it will be treated equivalently to
|
||
`tup = unstack(tup)`, but the implementation will avoid explicit unstacking.
|
||
dtype: optional dtype of the resulting array. If not specified, the dtype
|
||
will be determined via type promotion rules described in :ref:`type-promotion`.
|
||
|
||
Returns:
|
||
the stacked result.
|
||
|
||
See also:
|
||
- :func:`jax.numpy.stack`: stack along arbitrary axes
|
||
- :func:`jax.numpy.concatenate`: concatenation along existing axes.
|
||
- :func:`jax.numpy.vstack`: stack vertically, i.e. along axis 0.
|
||
- :func:`jax.numpy.dstack`: stack depth-wise, i.e. along axis 2.
|
||
|
||
Examples:
|
||
Scalar values:
|
||
|
||
>>> jnp.hstack([1, 2, 3])
|
||
Array([1, 2, 3], dtype=int32, weak_type=True)
|
||
|
||
1D arrays:
|
||
|
||
>>> x = jnp.arange(3)
|
||
>>> y = jnp.ones(3)
|
||
>>> jnp.hstack([x, y])
|
||
Array([0., 1., 2., 1., 1., 1.], dtype=float32)
|
||
|
||
2D arrays:
|
||
|
||
>>> x = x.reshape(3, 1)
|
||
>>> y = y.reshape(3, 1)
|
||
>>> jnp.hstack([x, y])
|
||
Array([[0., 1.],
|
||
[1., 1.],
|
||
[2., 1.]], dtype=float32)
|
||
"""
|
||
arrs: Array | list[Array]
|
||
if isinstance(tup, (np.ndarray, Array)):
|
||
arrs = jax.vmap(atleast_1d)(tup)
|
||
arr0_ndim = arrs.ndim - 1
|
||
else:
|
||
# TODO(jakevdp): Non-array input deprecated 2023-09-22; change to error.
|
||
util.check_arraylike("hstack", *tup, emit_warning=True)
|
||
arrs = [atleast_1d(m) for m in tup]
|
||
arr0_ndim = arrs[0].ndim
|
||
return concatenate(arrs, axis=0 if arr0_ndim == 1 else 1, dtype=dtype)
|
||
|
||
|
||
@export
|
||
def dstack(tup: np.ndarray | Array | Sequence[ArrayLike],
|
||
dtype: DTypeLike | None = None) -> Array:
|
||
"""Stack arrays depth-wise.
|
||
|
||
JAX implementation of :func:`numpy.dstack`.
|
||
|
||
For arrays of three or more dimensions, this is equivalent to
|
||
:func:`jax.numpy.concatenate` with ``axis=2``.
|
||
|
||
Args:
|
||
tup: a sequence of arrays to stack; each must have the same shape along all
|
||
but the third axis. Input arrays will be promoted to at least rank 3. If a
|
||
single array is given it will be treated equivalently to `tup = unstack(tup)`,
|
||
but the implementation will avoid explicit unstacking.
|
||
dtype: optional dtype of the resulting array. If not specified, the dtype
|
||
will be determined via type promotion rules described in :ref:`type-promotion`.
|
||
|
||
Returns:
|
||
the stacked result.
|
||
|
||
See also:
|
||
- :func:`jax.numpy.stack`: stack along arbitrary axes
|
||
- :func:`jax.numpy.concatenate`: concatenation along existing axes.
|
||
- :func:`jax.numpy.vstack`: stack vertically, i.e. along axis 0.
|
||
- :func:`jax.numpy.hstack`: stack horizontally, i.e. along axis 1.
|
||
|
||
Examples:
|
||
Scalar values:
|
||
|
||
>>> jnp.dstack([1, 2, 3])
|
||
Array([[[1, 2, 3]]], dtype=int32, weak_type=True)
|
||
|
||
1D arrays:
|
||
|
||
>>> x = jnp.arange(3)
|
||
>>> y = jnp.ones(3)
|
||
>>> jnp.dstack([x, y])
|
||
Array([[[0., 1.],
|
||
[1., 1.],
|
||
[2., 1.]]], dtype=float32)
|
||
|
||
2D arrays:
|
||
|
||
>>> x = x.reshape(1, 3)
|
||
>>> y = y.reshape(1, 3)
|
||
>>> jnp.dstack([x, y])
|
||
Array([[[0., 1.],
|
||
[1., 1.],
|
||
[2., 1.]]], dtype=float32)
|
||
"""
|
||
arrs: Array | list[Array]
|
||
if isinstance(tup, (np.ndarray, Array)):
|
||
arrs = jax.vmap(atleast_3d)(tup)
|
||
else:
|
||
# TODO(jakevdp): Non-array input deprecated 2023-09-22; change to error.
|
||
util.check_arraylike("dstack", *tup, emit_warning=True)
|
||
arrs = [atleast_3d(m) for m in tup]
|
||
return concatenate(arrs, axis=2, dtype=dtype)
|
||
|
||
|
||
@export
|
||
def column_stack(tup: np.ndarray | Array | Sequence[ArrayLike]) -> Array:
|
||
"""Stack arrays column-wise.
|
||
|
||
JAX implementation of :func:`numpy.column_stack`.
|
||
|
||
For arrays of two or more dimensions, this is equivalent to
|
||
:func:`jax.numpy.concatenate` with ``axis=1``.
|
||
|
||
Args:
|
||
tup: a sequence of arrays to stack; each must have the same leading dimension.
|
||
Input arrays will be promoted to at least rank 2. If a single array is given
|
||
it will be treated equivalently to `tup = unstack(tup)`, but the implementation
|
||
will avoid explicit unstacking.
|
||
dtype: optional dtype of the resulting array. If not specified, the dtype
|
||
will be determined via type promotion rules described in :ref:`type-promotion`.
|
||
|
||
Returns:
|
||
the stacked result.
|
||
|
||
See also:
|
||
- :func:`jax.numpy.stack`: stack along arbitrary axes
|
||
- :func:`jax.numpy.concatenate`: concatenation along existing axes.
|
||
- :func:`jax.numpy.vstack`: stack vertically, i.e. along axis 0.
|
||
- :func:`jax.numpy.hstack`: stack horizontally, i.e. along axis 1.
|
||
- :func:`jax.numpy.hstack`: stack depth=wise, i.e. along axis 2.
|
||
|
||
Examples:
|
||
Scalar values:
|
||
|
||
>>> jnp.column_stack([1, 2, 3])
|
||
Array([[1, 2, 3]], dtype=int32, weak_type=True)
|
||
|
||
1D arrays:
|
||
|
||
>>> x = jnp.arange(3)
|
||
>>> y = jnp.ones(3)
|
||
>>> jnp.column_stack([x, y])
|
||
Array([[0., 1.],
|
||
[1., 1.],
|
||
[2., 1.]], dtype=float32)
|
||
|
||
2D arrays:
|
||
|
||
>>> x = x.reshape(3, 1)
|
||
>>> y = y.reshape(3, 1)
|
||
>>> jnp.column_stack([x, y])
|
||
Array([[0., 1.],
|
||
[1., 1.],
|
||
[2., 1.]], dtype=float32)
|
||
"""
|
||
arrs: Array | list[Array] | np.ndarray
|
||
if isinstance(tup, (np.ndarray, Array)):
|
||
arrs = jax.vmap(lambda x: atleast_2d(x).T)(tup) if tup.ndim < 3 else tup
|
||
else:
|
||
# TODO(jakevdp): Non-array input deprecated 2023-09-22; change to error.
|
||
util.check_arraylike("column_stack", *tup, emit_warning=True)
|
||
arrs = [atleast_2d(arr).T if arr.ndim < 2 else arr for arr in map(asarray, tup)]
|
||
return concatenate(arrs, axis=1)
|
||
|
||
|
||
@export
|
||
def choose(a: ArrayLike, choices: Array | np.ndarray | Sequence[ArrayLike],
|
||
out: None = None, mode: str = 'raise') -> Array:
|
||
"""Construct an array by stacking slices of choice arrays.
|
||
|
||
JAX implementation of :func:`numpy.choose`.
|
||
|
||
The semantics of this function can be confusing, but in the simplest case where
|
||
``a`` is a one-dimensional array, ``choices`` is a two-dimensional array, and
|
||
all entries of ``a`` are in-bounds (i.e. ``0 <= a_i < len(choices)``), then the
|
||
function is equivalent to the following::
|
||
|
||
def choose(a, choices):
|
||
return jnp.array([choices[a_i, i] for i, a_i in enumerate(a)])
|
||
|
||
In the more general case, ``a`` may have any number of dimensions and ``choices``
|
||
may be an arbitrary sequence of broadcast-compatible arrays. In this case, again
|
||
for in-bound indices, the logic is equivalent to::
|
||
|
||
def choose(a, choices):
|
||
a, *choices = jnp.broadcast_arrays(a, *choices)
|
||
choices = jnp.array(choices)
|
||
return jnp.array([choices[a[idx], *idx] for idx in np.ndindex(a.shape)])
|
||
|
||
The only additional complexity comes from the ``mode`` argument, which controls
|
||
the behavior for out-of-bound indices in ``a`` as described below.
|
||
|
||
Args:
|
||
a: an N-dimensional array of integer indices.
|
||
choices: an array or sequence of arrays. All arrays in the sequence must be
|
||
mutually broadcast compatible with ``a``.
|
||
out: unused by JAX
|
||
mode: specify the out-of-bounds indexing mode; one of ``'raise'`` (default),
|
||
``'wrap'``, or ``'clip'``. Note that the default mode of ``'raise'`` is
|
||
not compatible with JAX transformations.
|
||
|
||
Returns:
|
||
an array containing stacked slices from ``choices`` at the indices
|
||
specified by ``a``. The shape of the result is
|
||
``broadcast_shapes(a.shape, *(c.shape for c in choices))``.
|
||
|
||
See also:
|
||
- :func:`jax.lax.switch`: choose between N functions based on an index.
|
||
|
||
Examples:
|
||
Here is the simplest case of a 1D index array with a 2D choice array,
|
||
in which case this chooses the indexed value from each column:
|
||
|
||
>>> choices = jnp.array([[ 1, 2, 3, 4],
|
||
... [ 5, 6, 7, 8],
|
||
... [ 9, 10, 11, 12]])
|
||
>>> a = jnp.array([2, 0, 1, 0])
|
||
>>> jnp.choose(a, choices)
|
||
Array([9, 2, 7, 4], dtype=int32)
|
||
|
||
The ``mode`` argument specifies what to do with out-of-bound indices;
|
||
options are to either ``wrap`` or ``clip``:
|
||
|
||
>>> a2 = jnp.array([2, 0, 1, 4]) # last index out-of-bound
|
||
>>> jnp.choose(a2, choices, mode='clip')
|
||
Array([ 9, 2, 7, 12], dtype=int32)
|
||
>>> jnp.choose(a2, choices, mode='wrap')
|
||
Array([9, 2, 7, 8], dtype=int32)
|
||
|
||
In the more general case, ``choices`` may be a sequence of array-like
|
||
objects with any broadcast-compatible shapes.
|
||
|
||
>>> choice_1 = jnp.array([1, 2, 3, 4])
|
||
>>> choice_2 = 99
|
||
>>> choice_3 = jnp.array([[10],
|
||
... [20],
|
||
... [30]])
|
||
>>> a = jnp.array([[0, 1, 2, 0],
|
||
... [1, 2, 0, 1],
|
||
... [2, 0, 1, 2]])
|
||
>>> jnp.choose(a, [choice_1, choice_2, choice_3], mode='wrap')
|
||
Array([[ 1, 99, 10, 4],
|
||
[99, 20, 3, 99],
|
||
[30, 2, 99, 30]], dtype=int32)
|
||
"""
|
||
if out is not None:
|
||
raise NotImplementedError("The 'out' argument to jnp.choose is not supported.")
|
||
util.check_arraylike('choose', a, *choices)
|
||
if not issubdtype(_dtype(a), np.integer):
|
||
raise ValueError("`a` array must be integer typed")
|
||
N = len(choices)
|
||
|
||
if mode == 'raise':
|
||
arr: Array = core.concrete_or_error(asarray, a,
|
||
"The error occurred because jnp.choose was jit-compiled"
|
||
" with mode='raise'. Use mode='wrap' or mode='clip' instead.")
|
||
if reductions.any((arr < 0) | (arr >= N)):
|
||
raise ValueError("invalid entry in choice array")
|
||
elif mode == 'wrap':
|
||
arr = asarray(a) % N
|
||
elif mode == 'clip':
|
||
arr = clip(a, 0, N - 1)
|
||
else:
|
||
raise ValueError(f"mode={mode!r} not understood. Must be 'raise', 'wrap', or 'clip'")
|
||
|
||
arr, *choices = broadcast_arrays(arr, *choices)
|
||
return array(choices)[(arr,) + indices(arr.shape, sparse=True)]
|
||
|
||
|
||
def _atleast_nd(x: ArrayLike, n: int) -> Array:
|
||
m = np.ndim(x)
|
||
return lax.broadcast(x, (1,) * (n - m)) if m < n else asarray(x)
|
||
|
||
def _block(xs: ArrayLike | list[ArrayLike]) -> tuple[Array, int]:
|
||
if isinstance(xs, tuple):
|
||
raise ValueError("jax.numpy.block does not allow tuples, got {}"
|
||
.format(xs))
|
||
elif isinstance(xs, list):
|
||
if len(xs) == 0:
|
||
raise ValueError("jax.numpy.block does not allow empty list arguments")
|
||
xs_tup, depths = unzip2([_block(x) for x in xs])
|
||
if any(d != depths[0] for d in depths[1:]):
|
||
raise ValueError("Mismatched list depths in jax.numpy.block")
|
||
rank = max(depths[0], max(np.ndim(x) for x in xs_tup))
|
||
xs_tup = tuple(_atleast_nd(x, rank) for x in xs_tup)
|
||
return concatenate(xs_tup, axis=-depths[0]), depths[0] + 1
|
||
else:
|
||
return asarray(xs), 1
|
||
|
||
|
||
@export
|
||
@jit
|
||
def block(arrays: ArrayLike | list[ArrayLike]) -> Array:
|
||
"""Create an array from a list of blocks.
|
||
|
||
JAX implementation of :func:`numpy.block`.
|
||
|
||
Args:
|
||
arrays: an array, or nested list of arrays which will be concatenated
|
||
together to form the final array.
|
||
|
||
Returns:
|
||
a single array constructed from the inputs.
|
||
|
||
See also:
|
||
- :func:`concatenate`, :func:`concat`: concatenate arrays along an existing axis.
|
||
- :func:`stack`, :func:`vstack`, :func:`hstack`, :func:`dstack` concatenate
|
||
arrays along a new axis.
|
||
|
||
Examples:
|
||
consider these blocks:
|
||
|
||
>>> zeros = jnp.zeros((2, 2))
|
||
>>> ones = jnp.ones((2, 2))
|
||
>>> twos = jnp.full((2, 2), 2)
|
||
>>> threes = jnp.full((2, 2), 3)
|
||
|
||
Passing a single array to :func:`block` returns the array:
|
||
|
||
>>> jnp.block(zeros)
|
||
Array([[0., 0.],
|
||
[0., 0.]], dtype=float32)
|
||
|
||
Passing a simple list of arrays concatenates them along the last axis:
|
||
|
||
>>> jnp.block([zeros, ones])
|
||
Array([[0., 0., 1., 1.],
|
||
[0., 0., 1., 1.]], dtype=float32)
|
||
|
||
Passing a doubly-nested list of arrays concatenates the inner list along
|
||
the last axis, and the outer list along the second-to-last axis:
|
||
|
||
>>> jnp.block([[zeros, ones],
|
||
... [twos, threes]])
|
||
Array([[0., 0., 1., 1.],
|
||
[0., 0., 1., 1.],
|
||
[2., 2., 3., 3.],
|
||
[2., 2., 3., 3.]], dtype=float32)
|
||
|
||
Note that blocks need not align in all dimensions, though the size along the axis
|
||
of concatenation must match. For example, this is valid because after the inner,
|
||
horizontal concatenation, the resulting blocks have a valid shape for the outer,
|
||
vertical concatenation.
|
||
|
||
>>> a = jnp.zeros((2, 1))
|
||
>>> b = jnp.ones((2, 3))
|
||
>>> c = jnp.full((1, 2), 2)
|
||
>>> d = jnp.full((1, 2), 3)
|
||
>>> jnp.block([[a, b], [c, d]])
|
||
Array([[0., 1., 1., 1.],
|
||
[0., 1., 1., 1.],
|
||
[2., 2., 3., 3.]], dtype=float32)
|
||
|
||
Note also that this logic generalizes to blocks in 3 or more dimensions.
|
||
Here's a 3-dimensional block-wise array:
|
||
|
||
>>> x = jnp.arange(6).reshape((1, 2, 3))
|
||
>>> blocks = [[[x for i in range(3)] for j in range(4)] for k in range(5)]
|
||
>>> jnp.block(blocks).shape
|
||
(5, 8, 9)
|
||
"""
|
||
out, _ = _block(arrays)
|
||
return out
|
||
|
||
|
||
@overload
|
||
def atleast_1d() -> list[Array]:
|
||
...
|
||
@overload
|
||
def atleast_1d(x: ArrayLike, /) -> Array:
|
||
...
|
||
@overload
|
||
def atleast_1d(x: ArrayLike, y: ArrayLike, /, *arys: ArrayLike) -> list[Array]:
|
||
...
|
||
@export
|
||
@jit
|
||
def atleast_1d(*arys: ArrayLike) -> Array | list[Array]:
|
||
"""Convert inputs to arrays with at least 1 dimension.
|
||
|
||
JAX implementation of :func:`numpy.atleast_1d`.
|
||
|
||
Args:
|
||
zero or more arraylike arguments.
|
||
|
||
Returns:
|
||
an array or list of arrays corresponding to the input values. Arrays
|
||
of shape ``()`` are converted to shape ``(1,)``, and arrays with other
|
||
shapes are returned unchanged.
|
||
|
||
See also:
|
||
- :func:`jax.numpy.asarray`
|
||
- :func:`jax.numpy.atleast_2d`
|
||
- :func:`jax.numpy.atleast_3d`
|
||
|
||
Examples:
|
||
Scalar arguments are converted to 1D, length-1 arrays:
|
||
|
||
>>> x = jnp.float32(1.0)
|
||
>>> jnp.atleast_1d(x)
|
||
Array([1.], dtype=float32)
|
||
|
||
Higher dimensional inputs are returned unchanged:
|
||
|
||
>>> y = jnp.arange(4)
|
||
>>> jnp.atleast_1d(y)
|
||
Array([0, 1, 2, 3], dtype=int32)
|
||
|
||
Multiple arguments can be passed to the function at once, in which
|
||
case a list of results is returned:
|
||
|
||
>>> jnp.atleast_1d(x, y)
|
||
[Array([1.], dtype=float32), Array([0, 1, 2, 3], dtype=int32)]
|
||
"""
|
||
util.check_arraylike("atleast_1d", *arys, emit_warning=True)
|
||
if len(arys) == 1:
|
||
return array(arys[0], copy=False, ndmin=1)
|
||
else:
|
||
return [array(arr, copy=False, ndmin=1) for arr in arys]
|
||
|
||
|
||
@overload
|
||
def atleast_2d() -> list[Array]:
|
||
...
|
||
@overload
|
||
def atleast_2d(x: ArrayLike, /) -> Array:
|
||
...
|
||
@overload
|
||
def atleast_2d(x: ArrayLike, y: ArrayLike, /, *arys: ArrayLike) -> list[Array]:
|
||
...
|
||
@export
|
||
@jit
|
||
def atleast_2d(*arys: ArrayLike) -> Array | list[Array]:
|
||
"""Convert inputs to arrays with at least 2 dimensions.
|
||
|
||
JAX implementation of :func:`numpy.atleast_2d`.
|
||
|
||
Args:
|
||
zero or more arraylike arguments.
|
||
|
||
Returns:
|
||
an array or list of arrays corresponding to the input values. Arrays
|
||
of shape ``()`` are converted to shape ``(1, 1)``, 1D arrays of shape
|
||
``(N,)`` are converted to shape ``(1, N)``, and arrays of all other
|
||
shapes are returned unchanged.
|
||
|
||
See also:
|
||
- :func:`jax.numpy.asarray`
|
||
- :func:`jax.numpy.atleast_1d`
|
||
- :func:`jax.numpy.atleast_3d`
|
||
|
||
Examples:
|
||
Scalar arguments are converted to 2D, size-1 arrays:
|
||
|
||
>>> x = jnp.float32(1.0)
|
||
>>> jnp.atleast_2d(x)
|
||
Array([[1.]], dtype=float32)
|
||
|
||
One-dimensional arguments have a unit dimension prepended to the shape:
|
||
|
||
>>> y = jnp.arange(4)
|
||
>>> jnp.atleast_2d(y)
|
||
Array([[0, 1, 2, 3]], dtype=int32)
|
||
|
||
Higher dimensional inputs are returned unchanged:
|
||
|
||
>>> z = jnp.ones((2, 3))
|
||
>>> jnp.atleast_2d(z)
|
||
Array([[1., 1., 1.],
|
||
[1., 1., 1.]], dtype=float32)
|
||
|
||
Multiple arguments can be passed to the function at once, in which
|
||
case a list of results is returned:
|
||
|
||
>>> jnp.atleast_2d(x, y)
|
||
[Array([[1.]], dtype=float32), Array([[0, 1, 2, 3]], dtype=int32)]
|
||
"""
|
||
# TODO(jakevdp): Non-array input deprecated 2023-09-22; change to error.
|
||
util.check_arraylike("atleast_2d", *arys, emit_warning=True)
|
||
if len(arys) == 1:
|
||
return array(arys[0], copy=False, ndmin=2)
|
||
else:
|
||
return [array(arr, copy=False, ndmin=2) for arr in arys]
|
||
|
||
|
||
@overload
|
||
def atleast_3d() -> list[Array]:
|
||
...
|
||
@overload
|
||
def atleast_3d(x: ArrayLike, /) -> Array:
|
||
...
|
||
@overload
|
||
def atleast_3d(x: ArrayLike, y: ArrayLike, /, *arys: ArrayLike) -> list[Array]:
|
||
...
|
||
@export
|
||
@jit
|
||
def atleast_3d(*arys: ArrayLike) -> Array | list[Array]:
|
||
"""Convert inputs to arrays with at least 3 dimensions.
|
||
|
||
JAX implementation of :func:`numpy.atleast_3d`.
|
||
|
||
Args:
|
||
zero or more arraylike arguments.
|
||
|
||
Returns:
|
||
an array or list of arrays corresponding to the input values. Arrays
|
||
of shape ``()`` are converted to shape ``(1, 1, 1)``, 1D arrays of
|
||
shape ``(N,)`` are converted to shape ``(1, N, 1)``, 2D arrays of
|
||
shape ``(M, N)`` are converted to shape ``(M, N, 1)``, and arrays
|
||
of all other shapes are returned unchanged.
|
||
|
||
See also:
|
||
- :func:`jax.numpy.asarray`
|
||
- :func:`jax.numpy.atleast_1d`
|
||
- :func:`jax.numpy.atleast_2d`
|
||
|
||
Examples:
|
||
Scalar arguments are converted to 3D, size-1 arrays:
|
||
|
||
>>> x = jnp.float32(1.0)
|
||
>>> jnp.atleast_3d(x)
|
||
Array([[[1.]]], dtype=float32)
|
||
|
||
1D arrays have a unit dimension prepended and appended:
|
||
|
||
>>> y = jnp.arange(4)
|
||
>>> jnp.atleast_3d(y).shape
|
||
(1, 4, 1)
|
||
|
||
2D arrays have a unit dimension appended:
|
||
|
||
>>> z = jnp.ones((2, 3))
|
||
>>> jnp.atleast_3d(z).shape
|
||
(2, 3, 1)
|
||
|
||
Multiple arguments can be passed to the function at once, in which
|
||
case a list of results is returned:
|
||
|
||
>>> x3, y3 = jnp.atleast_3d(x, y)
|
||
>>> print(x3)
|
||
[[[1.]]]
|
||
>>> print(y3)
|
||
[[[0]
|
||
[1]
|
||
[2]
|
||
[3]]]
|
||
"""
|
||
# TODO(jakevdp): Non-array input deprecated 2023-09-22; change to error.
|
||
util.check_arraylike("atleast_3d", *arys, emit_warning=True)
|
||
if len(arys) == 1:
|
||
arr = asarray(arys[0])
|
||
if arr.ndim == 0:
|
||
arr = lax.expand_dims(arr, dimensions=(0, 1, 2))
|
||
elif arr.ndim == 1:
|
||
arr = lax.expand_dims(arr, dimensions=(0, 2))
|
||
elif arr.ndim == 2:
|
||
arr = lax.expand_dims(arr, dimensions=(2,))
|
||
return arr
|
||
else:
|
||
return [atleast_3d(arr) for arr in arys]
|
||
|
||
|
||
def _supports_buffer_protocol(obj):
|
||
try:
|
||
view = memoryview(obj)
|
||
except TypeError:
|
||
return False
|
||
else:
|
||
return True
|
||
|
||
|
||
def _make_string_array(
|
||
object: np.ndarray,
|
||
dtype: DTypeLike | None = None,
|
||
ndmin: int = 0,
|
||
device: xc.Device | Sharding | None = None,
|
||
) -> Array:
|
||
if not isinstance(object, np.ndarray):
|
||
raise TypeError(
|
||
"Currently, string arrays can only be made from NumPy"
|
||
f" arrays. Got: {type(object)}."
|
||
)
|
||
if dtype is not None and (
|
||
dtypes.is_string_dtype(object.dtype) != dtypes.is_string_dtype(dtype)
|
||
):
|
||
raise TypeError(
|
||
f"Cannot make an array with dtype {dtype} from an object with dtype"
|
||
f" {object.dtype}."
|
||
)
|
||
if ndmin > object.ndim:
|
||
raise TypeError(
|
||
f"ndmin {ndmin} cannot be greater than object's ndims"
|
||
f" {object.ndim} for string arrays."
|
||
)
|
||
|
||
# Just do a device_put since XLA does not support string as a data type.
|
||
return jax.device_put(x=object, device=device)
|
||
|
||
|
||
@export
|
||
def array(object: Any, dtype: DTypeLike | None = None, copy: bool = True,
|
||
order: str | None = "K", ndmin: int = 0,
|
||
*, device: xc.Device | Sharding | None = None) -> Array:
|
||
"""Convert an object to a JAX array.
|
||
|
||
JAX implementation of :func:`numpy.array`.
|
||
|
||
Args:
|
||
object: an object that is convertible to an array. This includes JAX
|
||
arrays, NumPy arrays, Python scalars, Python collections like lists
|
||
and tuples, objects with an ``__array__`` method, and objects
|
||
supporting the Python buffer protocol.
|
||
dtype: optionally specify the dtype of the output array. If not
|
||
specified it will be inferred from the input.
|
||
copy: specify whether to force a copy of the input. Default: True.
|
||
order: not implemented in JAX
|
||
ndmin: integer specifying the minimum number of dimensions in the
|
||
output array.
|
||
device: optional :class:`~jax.Device` or :class:`~jax.sharding.Sharding`
|
||
to which the created array will be committed.
|
||
|
||
Returns:
|
||
A JAX array constructed from the input.
|
||
|
||
See also:
|
||
- :func:`jax.numpy.asarray`: like `array`, but by default only copies
|
||
when necessary.
|
||
- :func:`jax.numpy.from_dlpack`: construct a JAX array from an object
|
||
that implements the dlpack interface.
|
||
- :func:`jax.numpy.frombuffer`: construct a JAX array from an object
|
||
that implements the buffer interface.
|
||
|
||
Examples:
|
||
Constructing JAX arrays from Python scalars:
|
||
|
||
>>> jnp.array(True)
|
||
Array(True, dtype=bool)
|
||
>>> jnp.array(42)
|
||
Array(42, dtype=int32, weak_type=True)
|
||
>>> jnp.array(3.5)
|
||
Array(3.5, dtype=float32, weak_type=True)
|
||
>>> jnp.array(1 + 1j)
|
||
Array(1.+1.j, dtype=complex64, weak_type=True)
|
||
|
||
Constructing JAX arrays from Python collections:
|
||
|
||
>>> jnp.array([1, 2, 3]) # list of ints -> 1D array
|
||
Array([1, 2, 3], dtype=int32)
|
||
>>> jnp.array([(1, 2, 3), (4, 5, 6)]) # list of tuples of ints -> 2D array
|
||
Array([[1, 2, 3],
|
||
[4, 5, 6]], dtype=int32)
|
||
>>> jnp.array(range(5))
|
||
Array([0, 1, 2, 3, 4], dtype=int32)
|
||
|
||
Constructing JAX arrays from NumPy arrays:
|
||
|
||
>>> jnp.array(np.linspace(0, 2, 5))
|
||
Array([0. , 0.5, 1. , 1.5, 2. ], dtype=float32)
|
||
|
||
Constructing a JAX array via the Python buffer interface, using Python's
|
||
built-in :mod:`array` module.
|
||
|
||
>>> from array import array
|
||
>>> pybuffer = array('i', [2, 3, 5, 7])
|
||
>>> jnp.array(pybuffer)
|
||
Array([2, 3, 5, 7], dtype=int32)
|
||
"""
|
||
if order is not None and order != "K":
|
||
raise NotImplementedError("Only implemented for order='K'")
|
||
|
||
# check if the given dtype is compatible with JAX
|
||
dtypes.check_user_dtype_supported(dtype, "array")
|
||
|
||
# Here we make a judgment call: we only return a weakly-typed array when the
|
||
# input object itself is weakly typed. That ensures asarray(x) is a no-op
|
||
# whenever x is weak, but avoids introducing weak types with something like
|
||
# array([1, 2, 3])
|
||
weak_type = dtype is None and dtypes.is_weakly_typed(object)
|
||
if device is None and isinstance(object, core.Tracer):
|
||
sharding = object.aval.sharding
|
||
sharding = None if sharding.mesh.empty else sharding
|
||
else:
|
||
sharding = canonicalize_device_to_sharding(device)
|
||
|
||
# Use device_put to avoid a copy for ndarray inputs.
|
||
if (not copy and isinstance(object, np.ndarray) and
|
||
(dtype is None or dtype == object.dtype) and (ndmin <= object.ndim) and
|
||
device is None):
|
||
# Keep the output uncommitted.
|
||
return jax.device_put(object)
|
||
|
||
# String arrays need separate handling because XLA does not support string
|
||
# as a data type.
|
||
if dtypes.is_string_dtype(dtype) or (
|
||
hasattr(object, "dtype") and dtypes.is_string_dtype(object.dtype)
|
||
):
|
||
return _make_string_array(
|
||
object=object, dtype=dtype, ndmin=ndmin, device=device
|
||
)
|
||
|
||
# For Python scalar literals, call coerce_to_array to catch any overflow
|
||
# errors. We don't use dtypes.is_python_scalar because we don't want this
|
||
# triggering for traced values. We do this here because it matters whether or
|
||
# not dtype is None. We don't assign the result because we want the raw object
|
||
# to be used for type inference below.
|
||
if isinstance(object, (bool, int, float, complex)):
|
||
_ = dtypes.coerce_to_array(object, dtype)
|
||
elif not isinstance(object, Array):
|
||
# Check if object supports any of the data exchange protocols
|
||
# (except dlpack, see data-apis/array-api#301). If it does,
|
||
# consume the object as jax array and continue (but not return) so
|
||
# that other array() arguments get processed against the input
|
||
# object.
|
||
#
|
||
# Notice that data exchange protocols define dtype in the
|
||
# corresponding data structures and it may not be available as
|
||
# object.dtype. So, we'll resolve the protocols here before
|
||
# evaluating object.dtype.
|
||
if hasattr(object, '__jax_array__'):
|
||
object = object.__jax_array__()
|
||
elif hasattr(object, '__cuda_array_interface__'):
|
||
cai = object.__cuda_array_interface__
|
||
backend = xla_bridge.get_backend("cuda")
|
||
if cuda_plugin_extension is None:
|
||
device_id = None
|
||
else:
|
||
device_id = cuda_plugin_extension.get_device_ordinal(cai["data"][0])
|
||
object = xc._xla.cuda_array_interface_to_buffer(
|
||
cai=cai, gpu_backend=backend, device_id=device_id)
|
||
|
||
object = tree_map(lambda leaf: leaf.__jax_array__()
|
||
if hasattr(leaf, "__jax_array__") else leaf, object)
|
||
leaves = tree_leaves(object, is_leaf=lambda x: x is None)
|
||
if any(leaf is None for leaf in leaves):
|
||
# Added Nov 16 2023
|
||
if deprecations.is_accelerated("jax-numpy-array-none"):
|
||
raise TypeError("None is not a valid value for jnp.array")
|
||
warnings.warn(
|
||
"None encountered in jnp.array(); this is currently treated as NaN. "
|
||
"In the future this will result in an error.",
|
||
FutureWarning, stacklevel=2)
|
||
leaves = tree_leaves(object)
|
||
if dtype is None:
|
||
# Use lattice_result_type rather than result_type to avoid canonicalization.
|
||
# Otherwise, weakly-typed inputs would have their dtypes canonicalized.
|
||
try:
|
||
dtype = dtypes._lattice_result_type(*leaves)[0] if leaves else dtypes.float_
|
||
except TypeError:
|
||
# This happens if, e.g. one of the entries is a memoryview object.
|
||
# This is rare, so we only handle it if the normal path fails.
|
||
leaves = [_convert_to_array_if_dtype_fails(leaf) for leaf in leaves]
|
||
dtype = dtypes._lattice_result_type(*leaves)[0]
|
||
|
||
if not weak_type:
|
||
dtype = dtypes.canonicalize_dtype(dtype, allow_extended_dtype=True) # type: ignore[assignment]
|
||
|
||
out: ArrayLike
|
||
|
||
if all(not isinstance(leaf, Array) for leaf in leaves):
|
||
# TODO(jakevdp): falling back to numpy here fails to overflow for lists
|
||
# containing large integers; see discussion in
|
||
# https://github.com/jax-ml/jax/pull/6047. More correct would be to call
|
||
# coerce_to_array on each leaf, but this may have performance implications.
|
||
out = np.asarray(object, dtype=dtype)
|
||
elif isinstance(object, Array):
|
||
assert object.aval is not None
|
||
out = _array_copy(object) if copy else object
|
||
elif isinstance(object, (list, tuple)):
|
||
if object:
|
||
out = stack([asarray(elt, dtype=dtype) for elt in object])
|
||
else:
|
||
out = np.array([], dtype=dtype)
|
||
elif _supports_buffer_protocol(object):
|
||
object = memoryview(object)
|
||
# TODO(jakevdp): update this once we support NumPy 2.0 semantics for the copy arg.
|
||
out = np.array(object) if copy else np.asarray(object)
|
||
else:
|
||
raise TypeError(f"Unexpected input type for array: {type(object)}")
|
||
out_array: Array = lax_internal._convert_element_type(
|
||
out, dtype, weak_type=weak_type, sharding=sharding)
|
||
if ndmin > np.ndim(out_array):
|
||
out_array = lax.expand_dims(out_array, range(ndmin - np.ndim(out_array)))
|
||
return out_array
|
||
|
||
|
||
def canonicalize_device_to_sharding(device: xc.Device | Sharding | None
|
||
) -> Sharding | None:
|
||
if isinstance(device, xc.Device):
|
||
return SingleDeviceSharding(device)
|
||
return device
|
||
|
||
|
||
def _convert_to_array_if_dtype_fails(x: ArrayLike) -> ArrayLike:
|
||
try:
|
||
dtypes.dtype(x)
|
||
except TypeError:
|
||
return np.asarray(x)
|
||
else:
|
||
return x
|
||
|
||
|
||
@export
|
||
def astype(x: ArrayLike, dtype: DTypeLike | None,
|
||
/, *, copy: bool = False,
|
||
device: xc.Device | Sharding | None = None) -> Array:
|
||
"""Convert an array to a specified dtype.
|
||
|
||
JAX imlementation of :func:`numpy.astype`.
|
||
|
||
This is implemented via :func:`jax.lax.convert_element_type`, which may
|
||
have slightly different behavior than :func:`numpy.astype` in some cases.
|
||
In particular, the details of float-to-int and int-to-float casts are
|
||
implementation dependent.
|
||
|
||
Args:
|
||
x: input array to convert
|
||
dtype: output dtype
|
||
copy: if True, then always return a copy. If False (default) then only
|
||
return a copy if necessary.
|
||
device: optionally specify the device to which the output will be committed.
|
||
|
||
Returns:
|
||
An array with the same shape as ``x``, containing values of the specified
|
||
dtype.
|
||
|
||
See Also:
|
||
- :func:`jax.lax.convert_element_type`: lower-level function for XLA-style
|
||
dtype conversions.
|
||
|
||
Examples:
|
||
>>> x = jnp.array([0, 1, 2, 3])
|
||
>>> x
|
||
Array([0, 1, 2, 3], dtype=int32)
|
||
>>> x.astype('float32')
|
||
Array([0.0, 1.0, 2.0, 3.0], dtype=float32)
|
||
|
||
>>> y = jnp.array([0.0, 0.5, 1.0])
|
||
>>> y.astype(int) # truncates fractional values
|
||
Array([0, 0, 1], dtype=int32)
|
||
"""
|
||
x_arr = util.ensure_arraylike("astype", x)
|
||
|
||
if dtype is None:
|
||
dtype = dtypes.canonicalize_dtype(dtypes.float_)
|
||
dtypes.check_user_dtype_supported(dtype, "astype")
|
||
if issubdtype(x_arr.dtype, np.complexfloating):
|
||
if dtypes.isdtype(dtype, ("integral", "real floating")):
|
||
deprecations.warn(
|
||
"jax-numpy-astype-complex-to-real",
|
||
"Casting from complex to real dtypes will soon raise a ValueError. "
|
||
"Please first use jnp.real or jnp.imag to take the real/imaginary "
|
||
"component of your input.",
|
||
stacklevel=2)
|
||
elif np.dtype(dtype) == bool:
|
||
# convert_element_type(complex, bool) has the wrong semantics.
|
||
x_arr = (x_arr != _lax_const(x_arr, 0))
|
||
|
||
# We offer a more specific warning than the usual ComplexWarning so we prefer
|
||
# to issue our warning.
|
||
result = lax_internal._convert_element_type(
|
||
x_arr, dtype, sharding=util.normalize_device_to_sharding(device),
|
||
warn_on_complex_to_real_cast=False)
|
||
return _array_copy(result) if copy else result
|
||
|
||
|
||
@export
|
||
def asarray(a: Any, dtype: DTypeLike | None = None, order: str | None = None,
|
||
*, copy: bool | None = None,
|
||
device: xc.Device | Sharding | None = None) -> Array:
|
||
"""Convert an object to a JAX array.
|
||
|
||
JAX implementation of :func:`numpy.asarray`.
|
||
|
||
Args:
|
||
a: an object that is convertible to an array. This includes JAX
|
||
arrays, NumPy arrays, Python scalars, Python collections like lists
|
||
and tuples, objects with an ``__array__`` method, and objects
|
||
supporting the Python buffer protocol.
|
||
dtype: optionally specify the dtype of the output array. If not
|
||
specified it will be inferred from the input.
|
||
order: not implemented in JAX
|
||
copy: optional boolean specifying the copy mode. If True, then always
|
||
return a copy. If False, then error if a copy is necessary. Default is
|
||
None, which will only copy when necessary.
|
||
device: optional :class:`~jax.Device` or :class:`~jax.sharding.Sharding`
|
||
to which the created array will be committed.
|
||
|
||
Returns:
|
||
A JAX array constructed from the input.
|
||
|
||
See also:
|
||
- :func:`jax.numpy.array`: like `asarray`, but defaults to `copy=True`.
|
||
- :func:`jax.numpy.from_dlpack`: construct a JAX array from an object
|
||
that implements the dlpack interface.
|
||
- :func:`jax.numpy.frombuffer`: construct a JAX array from an object
|
||
that implements the buffer interface.
|
||
|
||
Examples:
|
||
Constructing JAX arrays from Python scalars:
|
||
|
||
>>> jnp.asarray(True)
|
||
Array(True, dtype=bool)
|
||
>>> jnp.asarray(42)
|
||
Array(42, dtype=int32, weak_type=True)
|
||
>>> jnp.asarray(3.5)
|
||
Array(3.5, dtype=float32, weak_type=True)
|
||
>>> jnp.asarray(1 + 1j)
|
||
Array(1.+1.j, dtype=complex64, weak_type=True)
|
||
|
||
Constructing JAX arrays from Python collections:
|
||
|
||
>>> jnp.asarray([1, 2, 3]) # list of ints -> 1D array
|
||
Array([1, 2, 3], dtype=int32)
|
||
>>> jnp.asarray([(1, 2, 3), (4, 5, 6)]) # list of tuples of ints -> 2D array
|
||
Array([[1, 2, 3],
|
||
[4, 5, 6]], dtype=int32)
|
||
>>> jnp.asarray(range(5))
|
||
Array([0, 1, 2, 3, 4], dtype=int32)
|
||
|
||
Constructing JAX arrays from NumPy arrays:
|
||
|
||
>>> jnp.asarray(np.linspace(0, 2, 5))
|
||
Array([0. , 0.5, 1. , 1.5, 2. ], dtype=float32)
|
||
|
||
Constructing a JAX array via the Python buffer interface, using Python's
|
||
built-in :mod:`array` module.
|
||
|
||
>>> from array import array
|
||
>>> pybuffer = array('i', [2, 3, 5, 7])
|
||
>>> jnp.asarray(pybuffer)
|
||
Array([2, 3, 5, 7], dtype=int32)
|
||
"""
|
||
# For copy=False, the array API specifies that we raise a ValueError if the input supports
|
||
# the buffer protocol but a copy is required. Since array() supports the buffer protocol
|
||
# via numpy, this is only the case when the default device is not 'cpu'
|
||
if (copy is False and not isinstance(a, Array)
|
||
and jax.default_backend() != 'cpu'
|
||
and _supports_buffer_protocol(a)):
|
||
raise ValueError(f"jnp.asarray: cannot convert object of type {type(a)} to JAX Array "
|
||
f"on backend={jax.default_backend()!r} with copy=False. "
|
||
"Consider using copy=None or copy=True instead.")
|
||
dtypes.check_user_dtype_supported(dtype, "asarray")
|
||
if dtype is not None:
|
||
dtype = dtypes.canonicalize_dtype(dtype, allow_extended_dtype=True) # type: ignore[assignment]
|
||
return array(a, dtype=dtype, copy=bool(copy), order=order, device=device)
|
||
|
||
|
||
@export
|
||
def copy(a: ArrayLike, order: str | None = None) -> Array:
|
||
"""Return a copy of the array.
|
||
|
||
JAX implementation of :func:`numpy.copy`.
|
||
|
||
Args:
|
||
a: arraylike object to copy
|
||
order: not implemented in JAX
|
||
|
||
Returns:
|
||
a copy of the input array ``a``.
|
||
|
||
See Also:
|
||
- :func:`jax.numpy.array`: create an array with or without a copy.
|
||
- :meth:`jax.Array.copy`: same function accessed as an array method.
|
||
|
||
Examples:
|
||
Since JAX arrays are immutable, in most cases explicit array copies
|
||
are not necessary. One exception is when using a function with donated
|
||
arguments (see the ``donate_argnums`` argument to :func:`jax.jit`).
|
||
|
||
>>> f = jax.jit(lambda x: 2 * x, donate_argnums=0)
|
||
>>> x = jnp.arange(4)
|
||
>>> y = f(x)
|
||
>>> print(y)
|
||
[0 2 4 6]
|
||
|
||
Because we marked ``x`` as being donated, the original array is no longer
|
||
available:
|
||
|
||
>>> print(x) # doctest: +IGNORE_EXCEPTION_DETAIL
|
||
Traceback (most recent call last):
|
||
RuntimeError: Array has been deleted with shape=int32[4].
|
||
|
||
In situations like this, an explicit copy will let you keep access to the
|
||
original buffer:
|
||
|
||
>>> x = jnp.arange(4)
|
||
>>> y = f(x.copy())
|
||
>>> print(y)
|
||
[0 2 4 6]
|
||
>>> print(x)
|
||
[0 1 2 3]
|
||
"""
|
||
util.check_arraylike("copy", a)
|
||
return array(a, copy=True, order=order)
|
||
|
||
|
||
@export
|
||
def array_equal(a1: ArrayLike, a2: ArrayLike, equal_nan: bool = False) -> Array:
|
||
"""Check if two arrays are element-wise equal.
|
||
|
||
JAX implementation of :func:`numpy.array_equal`.
|
||
|
||
Args:
|
||
a1: first input array to compare.
|
||
a2: second input array to compare.
|
||
equal_nan: Boolean. If ``True``, NaNs in ``a1`` will be considered
|
||
equal to NaNs in ``a2``. Default is ``False``.
|
||
|
||
Returns:
|
||
Boolean scalar array indicating whether the input arrays are element-wise equal.
|
||
|
||
See Also:
|
||
- :func:`jax.numpy.allclose`
|
||
- :func:`jax.numpy.array_equiv`
|
||
|
||
Examples:
|
||
>>> jnp.array_equal(jnp.array([1, 2, 3]), jnp.array([1, 2, 3]))
|
||
Array(True, dtype=bool)
|
||
>>> jnp.array_equal(jnp.array([1, 2, 3]), jnp.array([1, 2]))
|
||
Array(False, dtype=bool)
|
||
>>> jnp.array_equal(jnp.array([1, 2, 3]), jnp.array([1, 2, 4]))
|
||
Array(False, dtype=bool)
|
||
>>> jnp.array_equal(jnp.array([1, 2, float('nan')]),
|
||
... jnp.array([1, 2, float('nan')]))
|
||
Array(False, dtype=bool)
|
||
>>> jnp.array_equal(jnp.array([1, 2, float('nan')]),
|
||
... jnp.array([1, 2, float('nan')]), equal_nan=True)
|
||
Array(True, dtype=bool)
|
||
"""
|
||
a1, a2 = asarray(a1), asarray(a2)
|
||
if np.shape(a1) != np.shape(a2):
|
||
return array(False, dtype=bool)
|
||
eq = asarray(a1 == a2)
|
||
if equal_nan:
|
||
eq = ufuncs.logical_or(eq, ufuncs.logical_and(ufuncs.isnan(a1), ufuncs.isnan(a2)))
|
||
return reductions.all(eq)
|
||
|
||
|
||
@export
|
||
def array_equiv(a1: ArrayLike, a2: ArrayLike) -> Array:
|
||
"""Check if two arrays are element-wise equal.
|
||
|
||
JAX implementation of :func:`numpy.array_equiv`.
|
||
|
||
This function will return ``False`` if the input arrays cannot be broadcasted
|
||
to the same shape.
|
||
|
||
Args:
|
||
a1: first input array to compare.
|
||
a2: second input array to compare.
|
||
|
||
Returns:
|
||
Boolean scalar array indicating whether the input arrays are
|
||
element-wise equal after broadcasting.
|
||
|
||
See Also:
|
||
- :func:`jax.numpy.allclose`
|
||
- :func:`jax.numpy.array_equal`
|
||
|
||
Examples:
|
||
>>> jnp.array_equiv(jnp.array([1, 2, 3]), jnp.array([1, 2, 3]))
|
||
Array(True, dtype=bool)
|
||
>>> jnp.array_equiv(jnp.array([1, 2, 3]), jnp.array([1, 2, 4]))
|
||
Array(False, dtype=bool)
|
||
>>> jnp.array_equiv(jnp.array([[1, 2, 3], [1, 2, 3]]),
|
||
... jnp.array([1, 2, 3]))
|
||
Array(True, dtype=bool)
|
||
"""
|
||
a1, a2 = asarray(a1), asarray(a2)
|
||
try:
|
||
eq = ufuncs.equal(a1, a2)
|
||
except ValueError:
|
||
# shapes are not broadcastable
|
||
return array(False)
|
||
return reductions.all(eq)
|
||
|
||
|
||
# General np.from* style functions mostly delegate to numpy.
|
||
|
||
@export
|
||
def frombuffer(buffer: bytes | Any, dtype: DTypeLike = float,
|
||
count: int = -1, offset: int = 0) -> Array:
|
||
r"""Convert a buffer into a 1-D JAX array.
|
||
|
||
JAX implementation of :func:`numpy.frombuffer`.
|
||
|
||
Args:
|
||
buffer: an object containing the data. It must be either a bytes object with
|
||
a length that is an integer multiple of the dtype element size, or
|
||
it must be an object exporting the `Python buffer interface`_.
|
||
dtype: optional. Desired data type for the array. Default is ``float64``.
|
||
This specifies the dtype used to parse the buffer, but note that after parsing,
|
||
64-bit values will be cast to 32-bit JAX arrays if the ``jax_enable_x64``
|
||
flag is set to ``False``.
|
||
count: optional integer specifying the number of items to read from the buffer.
|
||
If -1 (default), all items from the buffer are read.
|
||
offset: optional integer specifying the number of bytes to skip at the beginning
|
||
of the buffer. Default is 0.
|
||
|
||
Returns:
|
||
A 1-D JAX array representing the interpreted data from the buffer.
|
||
|
||
See also:
|
||
- :func:`jax.numpy.fromstring`: convert a string of text into 1-D JAX array.
|
||
|
||
Examples:
|
||
Using a bytes buffer:
|
||
|
||
>>> buf = b"\x00\x01\x02\x03\x04"
|
||
>>> jnp.frombuffer(buf, dtype=jnp.uint8)
|
||
Array([0, 1, 2, 3, 4], dtype=uint8)
|
||
>>> jnp.frombuffer(buf, dtype=jnp.uint8, offset=1)
|
||
Array([1, 2, 3, 4], dtype=uint8)
|
||
|
||
Constructing a JAX array via the Python buffer interface, using Python's
|
||
built-in :mod:`array` module.
|
||
|
||
>>> from array import array
|
||
>>> pybuffer = array('i', [0, 1, 2, 3, 4])
|
||
>>> jnp.frombuffer(pybuffer, dtype=jnp.int32)
|
||
Array([0, 1, 2, 3, 4], dtype=int32)
|
||
|
||
.. _Python buffer interface: https://docs.python.org/3/c-api/buffer.html
|
||
"""
|
||
return asarray(np.frombuffer(buffer=buffer, dtype=dtype, count=count, offset=offset))
|
||
|
||
|
||
@export
|
||
def fromfile(*args, **kwargs):
|
||
"""Unimplemented JAX wrapper for jnp.fromfile.
|
||
|
||
This function is left deliberately unimplemented because it may be non-pure and thus
|
||
unsafe for use with JIT and other JAX transformations. Consider using
|
||
``jnp.asarray(np.fromfile(...))`` instead, although care should be taken if ``np.fromfile``
|
||
is used within jax transformations because of its potential side-effect of consuming the
|
||
file object; for more information see `Common Gotchas: Pure Functions
|
||
<https://jax.readthedocs.io/en/latest/notebooks/Common_Gotchas_in_JAX.html#pure-functions>`_.
|
||
"""
|
||
raise NotImplementedError(
|
||
"jnp.fromfile() is not implemented because it may be non-pure and thus unsafe for use "
|
||
"with JIT and other JAX transformations. Consider using jnp.asarray(np.fromfile(...)) "
|
||
"instead, although care should be taken if np.fromfile is used within a jax transformations "
|
||
"because of its potential side-effect of consuming the file object; for more information see "
|
||
"https://jax.readthedocs.io/en/latest/notebooks/Common_Gotchas_in_JAX.html#pure-functions")
|
||
|
||
|
||
@export
|
||
def fromiter(*args, **kwargs):
|
||
"""Unimplemented JAX wrapper for jnp.fromiter.
|
||
|
||
This function is left deliberately unimplemented because it may be non-pure and thus
|
||
unsafe for use with JIT and other JAX transformations. Consider using
|
||
``jnp.asarray(np.fromiter(...))`` instead, although care should be taken if ``np.fromiter``
|
||
is used within jax transformations because of its potential side-effect of consuming the
|
||
iterable object; for more information see `Common Gotchas: Pure Functions
|
||
<https://jax.readthedocs.io/en/latest/notebooks/Common_Gotchas_in_JAX.html#pure-functions>`_.
|
||
"""
|
||
raise NotImplementedError(
|
||
"jnp.fromiter() is not implemented because it may be non-pure and thus unsafe for use "
|
||
"with JIT and other JAX transformations. Consider using jnp.asarray(np.fromiter(...)) "
|
||
"instead, although care should be taken if np.fromiter is used within a jax transformations "
|
||
"because of its potential side-effect of consuming the iterable object; for more information see "
|
||
"https://jax.readthedocs.io/en/latest/notebooks/Common_Gotchas_in_JAX.html#pure-functions")
|
||
|
||
|
||
@export
|
||
def from_dlpack(x: Any, /, *, device: xc.Device | Sharding | None = None,
|
||
copy: bool | None = None) -> Array:
|
||
"""Construct a JAX array via DLPack.
|
||
|
||
JAX implementation of :func:`numpy.from_dlpack`.
|
||
|
||
Args:
|
||
x: An object that implements the DLPack_ protocol via the ``__dlpack__``
|
||
and ``__dlpack_device__`` methods, or a legacy DLPack tensor on either
|
||
CPU or GPU.
|
||
device: An optional :class:`~jax.Device` or :class:`~jax.sharding.Sharding`,
|
||
representing the single device onto which the returned array should be placed.
|
||
If given, then the result is committed to the device. If unspecified,
|
||
the resulting array will be unpacked onto the same device it originated from.
|
||
Setting ``device`` to a device different from the source of ``external_array``
|
||
will require a copy, meaning ``copy`` must be set to either ``True`` or ``None``.
|
||
copy: An optional boolean, controlling whether or not a copy is performed.
|
||
If ``copy=True`` then a copy is always performed, even if unpacked onto the
|
||
same device. If ``copy=False`` then the copy is never performed and will raise
|
||
an error if necessary. When ``copy=None`` (default) then a copy may be performed
|
||
if needed for a device transfer.
|
||
|
||
Returns:
|
||
A JAX array of the imput buffer.
|
||
|
||
Note:
|
||
While JAX arrays are always immutable, dlpack buffers cannot be marked as
|
||
immutable, and it is possible for processes external to JAX to mutate them
|
||
in-place. If a JAX Array is constructed from a dlpack buffer without copying
|
||
and the source buffer is later modified in-place, it may lead to undefined
|
||
behavior when using the associated JAX array.
|
||
|
||
Examples:
|
||
Passing data between NumPy and JAX via DLPack_:
|
||
|
||
>>> import numpy as np
|
||
>>> rng = np.random.default_rng(42)
|
||
>>> x_numpy = rng.random(4, dtype='float32')
|
||
>>> print(x_numpy)
|
||
[0.08925092 0.773956 0.6545715 0.43887842]
|
||
>>> hasattr(x_numpy, "__dlpack__") # NumPy supports the DLPack interface
|
||
True
|
||
|
||
>>> import jax.numpy as jnp
|
||
>>> x_jax = jnp.from_dlpack(x_numpy)
|
||
>>> print(x_jax)
|
||
[0.08925092 0.773956 0.6545715 0.43887842]
|
||
>>> hasattr(x_jax, "__dlpack__") # JAX supports the DLPack interface
|
||
True
|
||
|
||
>>> x_numpy_round_trip = np.from_dlpack(x_jax)
|
||
>>> print(x_numpy_round_trip)
|
||
[0.08925092 0.773956 0.6545715 0.43887842]
|
||
|
||
.. _DLPack: https://dmlc.github.io/dlpack
|
||
"""
|
||
from jax.dlpack import from_dlpack # pylint: disable=g-import-not-at-top
|
||
return from_dlpack(x, device=device, copy=copy)
|
||
|
||
|
||
@export
|
||
def fromfunction(function: Callable[..., Array], shape: Any,
|
||
*, dtype: DTypeLike = float, **kwargs) -> Array:
|
||
"""Create an array from a function applied over indices.
|
||
|
||
JAX implementation of :func:`numpy.fromfunction`. The JAX implementation
|
||
differs in that it dispatches via :func:`jax.vmap`, and so unlike in NumPy
|
||
the function logically operates on scalar inputs, and need not explicitly
|
||
handle broadcasted inputs (See *Examples* below).
|
||
|
||
Args:
|
||
function: a function that takes *N* dynamic scalars and outputs a scalar.
|
||
shape: a length-*N* tuple of integers specifying the output shape.
|
||
dtype: optionally specify the dtype of the inputs. Defaults to floating-point.
|
||
kwargs: additional keyword arguments are passed statically to ``function``.
|
||
|
||
Returns:
|
||
An array of shape ``shape`` if ``function`` returns a scalar, or in general
|
||
a pytree of arrays with leading dimensions ``shape``, as determined by the
|
||
output of ``function``.
|
||
|
||
See also:
|
||
- :func:`jax.vmap`: the core transformation that the :func:`fromfunction`
|
||
API is built on.
|
||
|
||
Examples:
|
||
Generate a multiplication table of a given shape:
|
||
|
||
>>> jnp.fromfunction(jnp.multiply, shape=(3, 6), dtype=int)
|
||
Array([[ 0, 0, 0, 0, 0, 0],
|
||
[ 0, 1, 2, 3, 4, 5],
|
||
[ 0, 2, 4, 6, 8, 10]], dtype=int32)
|
||
|
||
When ``function`` returns a non-scalar the output will have leading
|
||
dimension of ``shape``:
|
||
|
||
>>> def f(x):
|
||
... return (x + 1) * jnp.arange(3)
|
||
>>> jnp.fromfunction(f, shape=(2,))
|
||
Array([[0., 1., 2.],
|
||
[0., 2., 4.]], dtype=float32)
|
||
|
||
``function`` may return multiple results, in which case each is mapped
|
||
independently:
|
||
|
||
>>> def f(x, y):
|
||
... return x + y, x * y
|
||
>>> x_plus_y, x_times_y = jnp.fromfunction(f, shape=(3, 5))
|
||
>>> print(x_plus_y)
|
||
[[0. 1. 2. 3. 4.]
|
||
[1. 2. 3. 4. 5.]
|
||
[2. 3. 4. 5. 6.]]
|
||
>>> print(x_times_y)
|
||
[[0. 0. 0. 0. 0.]
|
||
[0. 1. 2. 3. 4.]
|
||
[0. 2. 4. 6. 8.]]
|
||
|
||
The JAX implementation differs slightly from NumPy's implementation. In
|
||
:func:`numpy.fromfunction`, the function is expected to explicitly operate
|
||
element-wise on the full grid of input values:
|
||
|
||
>>> def f(x, y):
|
||
... print(f"{x.shape = }\\n{y.shape = }")
|
||
... return x + y
|
||
...
|
||
>>> np.fromfunction(f, (2, 3))
|
||
x.shape = (2, 3)
|
||
y.shape = (2, 3)
|
||
array([[0., 1., 2.],
|
||
[1., 2., 3.]])
|
||
|
||
In :func:`jax.numpy.fromfunction`, the function is vectorized via
|
||
:func:`jax.vmap`, and so is expected to operate on scalar values:
|
||
|
||
>>> jnp.fromfunction(f, (2, 3))
|
||
x.shape = ()
|
||
y.shape = ()
|
||
Array([[0., 1., 2.],
|
||
[1., 2., 3.]], dtype=float32)
|
||
"""
|
||
shape = core.canonicalize_shape(shape, context="shape argument of jnp.fromfunction()")
|
||
for i in range(len(shape)):
|
||
in_axes = [0 if i == j else None for j in range(len(shape))]
|
||
function = jax.vmap(function, in_axes=tuple(in_axes[::-1]))
|
||
return function(*(arange(s, dtype=dtype) for s in shape), **kwargs)
|
||
|
||
|
||
@export
|
||
def fromstring(string: str, dtype: DTypeLike = float, count: int = -1, *, sep: str) -> Array:
|
||
"""Convert a string of text into 1-D JAX array.
|
||
|
||
JAX implementation of :func:`numpy.fromstring`.
|
||
|
||
Args:
|
||
string: input string containing the data.
|
||
dtype: optional. Desired data type for the array. Default is ``float``.
|
||
count: optional integer specifying the number of items to read from the string.
|
||
If -1 (default), all items are read.
|
||
sep: the string used to separate values in the input string.
|
||
|
||
Returns:
|
||
A 1-D JAX array containing the parsed data from the input string.
|
||
|
||
See also:
|
||
- :func:`jax.numpy.frombuffer`: construct a JAX array from an object
|
||
that implements the buffer interface.
|
||
|
||
Examples:
|
||
>>> jnp.fromstring("1 2 3", dtype=int, sep=" ")
|
||
Array([1, 2, 3], dtype=int32)
|
||
>>> jnp.fromstring("0.1, 0.2, 0.3", dtype=float, count=2, sep=",")
|
||
Array([0.1, 0.2], dtype=float32)
|
||
"""
|
||
return asarray(np.fromstring(string=string, dtype=dtype, count=count, sep=sep))
|
||
|
||
|
||
@export
|
||
def eye(N: DimSize, M: DimSize | None = None,
|
||
k: int | ArrayLike = 0,
|
||
dtype: DTypeLike | None = None,
|
||
*, device: xc.Device | Sharding | None = None) -> Array:
|
||
"""Create a square or rectangular identity matrix
|
||
|
||
JAX implementation of :func:`numpy.eye`.
|
||
|
||
Args:
|
||
N: integer specifying the first dimension of the array.
|
||
M: optional integer specifying the second dimension of the array;
|
||
defaults to the same value as ``N``.
|
||
k: optional integer specifying the offset of the diagonal. Use positive
|
||
values for upper diagonals, and negative values for lower diagonals.
|
||
Default is zero.
|
||
dtype: optional dtype; defaults to floating point.
|
||
device: optional :class:`~jax.Device` or :class:`~jax.sharding.Sharding`
|
||
to which the created array will be committed.
|
||
|
||
Returns:
|
||
Identity array of shape ``(N, M)``, or ``(N, N)`` if ``M`` is not specified.
|
||
|
||
See also:
|
||
:func:`jax.numpy.identity`: Simpler API for generating square identity matrices.
|
||
|
||
Examples:
|
||
A simple 3x3 identity matrix:
|
||
|
||
>>> jnp.eye(3)
|
||
Array([[1., 0., 0.],
|
||
[0., 1., 0.],
|
||
[0., 0., 1.]], dtype=float32)
|
||
|
||
Integer identity matrices with offset diagonals:
|
||
|
||
>>> jnp.eye(3, k=1, dtype=int)
|
||
Array([[0, 1, 0],
|
||
[0, 0, 1],
|
||
[0, 0, 0]], dtype=int32)
|
||
>>> jnp.eye(3, k=-1, dtype=int)
|
||
Array([[0, 0, 0],
|
||
[1, 0, 0],
|
||
[0, 1, 0]], dtype=int32)
|
||
|
||
Non-square identity matrix:
|
||
|
||
>>> jnp.eye(3, 5, k=1)
|
||
Array([[0., 1., 0., 0., 0.],
|
||
[0., 0., 1., 0., 0.],
|
||
[0., 0., 0., 1., 0.]], dtype=float32)
|
||
"""
|
||
# TODO(vfdev-5): optimize putting the array directly on the device specified
|
||
# instead of putting it on default device and then on the specific device
|
||
output = _eye(N, M=M, k=k, dtype=dtype)
|
||
if device is not None:
|
||
return jax.device_put(output, device=device)
|
||
return output
|
||
|
||
|
||
def _eye(N: DimSize, M: DimSize | None = None,
|
||
k: int | ArrayLike = 0,
|
||
dtype: DTypeLike | None = None) -> Array:
|
||
dtypes.check_user_dtype_supported(dtype, "eye")
|
||
if isinstance(k, int):
|
||
k = lax_internal._clip_int_to_valid_range(k, np.int32,
|
||
"`argument `k` of jax.numpy.eye")
|
||
offset = util.ensure_arraylike("eye", k)
|
||
if not (offset.shape == () and dtypes.issubdtype(offset.dtype, np.integer)):
|
||
raise ValueError(f"k must be a scalar integer; got {k}")
|
||
N_int = core.canonicalize_dim(N, "argument of 'N' jnp.eye()")
|
||
M_int = N_int if M is None else core.canonicalize_dim(M, "argument 'M' of jnp.eye()")
|
||
if N_int < 0 or M_int < 0:
|
||
raise ValueError(f"negative dimensions are not allowed, got {N} and {M}")
|
||
i = lax.broadcasted_iota(offset.dtype, (N_int, M_int), 0)
|
||
j = lax.broadcasted_iota(offset.dtype, (N_int, M_int), 1)
|
||
return (i + offset == j).astype(dtype)
|
||
|
||
|
||
@export
|
||
def identity(n: DimSize, dtype: DTypeLike | None = None) -> Array:
|
||
"""Create a square identity matrix
|
||
|
||
JAX implementation of :func:`numpy.identity`.
|
||
|
||
Args:
|
||
n: integer specifying the size of each array dimension.
|
||
dtype: optional dtype; defaults to floating point.
|
||
|
||
Returns:
|
||
Identity array of shape ``(n, n)``.
|
||
|
||
See also:
|
||
:func:`jax.numpy.eye`: non-square and/or offset identity matrices.
|
||
|
||
Examples:
|
||
A simple 3x3 identity matrix:
|
||
|
||
>>> jnp.identity(3)
|
||
Array([[1., 0., 0.],
|
||
[0., 1., 0.],
|
||
[0., 0., 1.]], dtype=float32)
|
||
|
||
A 2x2 integer identity matrix:
|
||
|
||
>>> jnp.identity(2, dtype=int)
|
||
Array([[1, 0],
|
||
[0, 1]], dtype=int32)
|
||
"""
|
||
dtypes.check_user_dtype_supported(dtype, "identity")
|
||
return eye(n, dtype=dtype)
|
||
|
||
|
||
@export
|
||
def arange(start: ArrayLike | DimSize, stop: ArrayLike | DimSize | None = None,
|
||
step: ArrayLike | None = None, dtype: DTypeLike | None = None,
|
||
*, device: xc.Device | Sharding | None = None) -> Array:
|
||
"""Create an array of evenly-spaced values.
|
||
|
||
JAX implementation of :func:`numpy.arange`, implemented in terms of
|
||
:func:`jax.lax.iota`.
|
||
|
||
Similar to Python's :func:`range` function, this can be called with a few
|
||
different positional signatures:
|
||
|
||
- ``jnp.arange(stop)``: generate values from 0 to ``stop``, stepping by 1.
|
||
- ``jnp.arange(start, stop)``: generate values from ``start`` to ``stop``,
|
||
stepping by 1.
|
||
- ``jnp.arange(start, stop, step)``: generate values from ``start`` to ``stop``,
|
||
stepping by ``step``.
|
||
|
||
Like with Python's :func:`range` function, the starting value is inclusive,
|
||
and the stop value is exclusive.
|
||
|
||
Args:
|
||
start: start of the interval, inclusive.
|
||
stop: optional end of the interval, exclusive. If not specified, then
|
||
``(start, stop) = (0, start)``
|
||
step: optional step size for the interval. Default = 1.
|
||
dtype: optional dtype for the returned array; if not specified it will
|
||
be determined via type promotion of `start`, `stop`, and `step`.
|
||
device: (optional) :class:`~jax.Device` or :class:`~jax.sharding.Sharding`
|
||
to which the created array will be committed.
|
||
|
||
Returns:
|
||
Array of evenly-spaced values from ``start`` to ``stop``, separated by ``step``.
|
||
|
||
Note:
|
||
Using ``arange`` with a floating-point ``step`` argument can lead to unexpected
|
||
results due to accumulation of floating-point errors, especially with
|
||
lower-precision data types like ``float8_*`` and ``bfloat16``.
|
||
To avoid precision errors, consider generating a range of integers, and scaling
|
||
it to the desired range. For example, instead of this::
|
||
|
||
jnp.arange(-1, 1, 0.01, dtype='bfloat16')
|
||
|
||
it can be more accurate to generate a sequence of integers, and scale them::
|
||
|
||
(jnp.arange(-100, 100) * 0.01).astype('bfloat16')
|
||
|
||
Examples:
|
||
Single-argument version specifies only the ``stop`` value:
|
||
|
||
>>> jnp.arange(4)
|
||
Array([0, 1, 2, 3], dtype=int32)
|
||
|
||
Passing a floating-point ``stop`` value leads to a floating-point result:
|
||
|
||
>>> jnp.arange(4.0)
|
||
Array([0., 1., 2., 3.], dtype=float32)
|
||
|
||
Two-argument version specifies ``start`` and ``stop``, with ``step=1``:
|
||
|
||
>>> jnp.arange(1, 6)
|
||
Array([1, 2, 3, 4, 5], dtype=int32)
|
||
|
||
Three-argument version specifies ``start``, ``stop``, and ``step``:
|
||
|
||
>>> jnp.arange(0, 2, 0.5)
|
||
Array([0. , 0.5, 1. , 1.5], dtype=float32)
|
||
|
||
See Also:
|
||
- :func:`jax.numpy.linspace`: generate a fixed number of evenly-spaced values.
|
||
- :func:`jax.lax.iota`: directly generate integer sequences in XLA.
|
||
"""
|
||
# TODO(vfdev-5): optimize putting the array directly on the device specified
|
||
# instead of putting it on default device and then on the specific device
|
||
output = _arange(start, stop=stop, step=step, dtype=dtype)
|
||
if device is not None:
|
||
return jax.device_put(output, device=device)
|
||
return output
|
||
|
||
|
||
def _arange(start: ArrayLike | DimSize, stop: ArrayLike | DimSize | None = None,
|
||
step: ArrayLike | None = None, dtype: DTypeLike | None = None) -> Array:
|
||
dtypes.check_user_dtype_supported(dtype, "arange")
|
||
if not config.dynamic_shapes.value:
|
||
util.check_arraylike("arange", start)
|
||
if stop is None and step is None:
|
||
start = core.concrete_or_error(None, start, "It arose in the jnp.arange argument 'stop'")
|
||
else:
|
||
start = core.concrete_or_error(None, start, "It arose in the jnp.arange argument 'start'")
|
||
util.check_arraylike_or_none("arange", None, stop, step)
|
||
stop = core.concrete_or_error(None, stop, "It arose in the jnp.arange argument 'stop'")
|
||
step = core.concrete_or_error(None, step, "It arose in the jnp.arange argument 'step'")
|
||
start_name = "stop" if stop is None and step is None else "start"
|
||
for name, val in [(start_name, start), ("stop", stop), ("step", step)]:
|
||
if val is not None and np.ndim(val) != 0:
|
||
raise ValueError(f"jax.numpy.arange: arguments must be scalars; got {name}={val}")
|
||
if any(core.is_symbolic_dim(v) for v in (start, stop, step)):
|
||
# Some dynamic shapes
|
||
if stop is None and step is None:
|
||
stop = start
|
||
start = 0
|
||
step = 1
|
||
elif stop is not None and step is None:
|
||
step = 1
|
||
return _arange_dynamic(start, stop, step, dtype or dtypes.canonicalize_dtype(np.int64))
|
||
if dtype is None:
|
||
dtype = result_type(start, *(x for x in [stop, step] if x is not None))
|
||
dtype = dtypes.jax_dtype(dtype)
|
||
if stop is None and step is None:
|
||
start_dtype = _dtype(start)
|
||
if (not dtypes.issubdtype(start_dtype, np.integer) and
|
||
not dtypes.issubdtype(start_dtype, dtypes.extended)):
|
||
ceil_ = ufuncs.ceil if isinstance(start, core.Tracer) else np.ceil
|
||
start = ceil_(start).astype(int)
|
||
return lax.iota(dtype, start) # type: ignore[arg-type]
|
||
else:
|
||
if step is None and start == 0 and stop is not None:
|
||
return lax.iota(dtype, np.ceil(stop).astype(int))
|
||
return array(np.arange(start, stop=stop, step=step, dtype=dtype))
|
||
|
||
|
||
def _arange_dynamic(
|
||
start: DimSize, stop: DimSize, step: DimSize, dtype: DTypeLike) -> Array:
|
||
# Here if at least one of start, stop, step are dynamic.
|
||
if any(not core.is_dim(v) for v in (start, stop, step)):
|
||
raise ValueError(
|
||
"In arange with non-constant arguments all of start, stop, and step "
|
||
f"must be either dimension expressions or integers: start={start}, "
|
||
f"stop={stop}, step={step}")
|
||
# Must resolve statically if step is {<0, ==0, >0}
|
||
try:
|
||
if step == 0:
|
||
raise ValueError("arange has step == 0")
|
||
step_gt_0 = (step > 0)
|
||
except core.InconclusiveDimensionOperation as e:
|
||
raise core.InconclusiveDimensionOperation(
|
||
f"In arange with non-constant arguments the step ({step}) must " +
|
||
f"be resolved statically if it is > 0 or < 0.\nDetails: {e}")
|
||
gap = step if step_gt_0 else - step
|
||
distance = (stop - start) if step_gt_0 else (start - stop)
|
||
size = core.max_dim(0, distance + gap - 1) // gap
|
||
return (array(start, dtype=dtype) +
|
||
array(step, dtype=dtype) * lax.iota(dtype, size))
|
||
|
||
@overload
|
||
def linspace(start: ArrayLike, stop: ArrayLike, num: int = 50,
|
||
endpoint: bool = True, retstep: Literal[False] = False,
|
||
dtype: DTypeLike | None = None,
|
||
axis: int = 0,
|
||
*, device: xc.Device | Sharding | None = None) -> Array: ...
|
||
@overload
|
||
def linspace(start: ArrayLike, stop: ArrayLike, num: int,
|
||
endpoint: bool, retstep: Literal[True],
|
||
dtype: DTypeLike | None = None,
|
||
axis: int = 0,
|
||
*, device: xc.Device | Sharding | None = None) -> tuple[Array, Array]: ...
|
||
@overload
|
||
def linspace(start: ArrayLike, stop: ArrayLike, num: int = 50,
|
||
endpoint: bool = True, *, retstep: Literal[True],
|
||
dtype: DTypeLike | None = None,
|
||
axis: int = 0,
|
||
device: xc.Device | Sharding | None = None) -> tuple[Array, Array]: ...
|
||
@overload
|
||
def linspace(start: ArrayLike, stop: ArrayLike, num: int = 50,
|
||
endpoint: bool = True, retstep: bool = False,
|
||
dtype: DTypeLike | None = None,
|
||
axis: int = 0,
|
||
*, device: xc.Device | Sharding | None = None) -> Array | tuple[Array, Array]: ...
|
||
@export
|
||
def linspace(start: ArrayLike, stop: ArrayLike, num: int = 50,
|
||
endpoint: bool = True, retstep: bool = False,
|
||
dtype: DTypeLike | None = None,
|
||
axis: int = 0,
|
||
*, device: xc.Device | Sharding | None = None) -> Array | tuple[Array, Array]:
|
||
"""Return evenly-spaced numbers within an interval.
|
||
|
||
JAX implementation of :func:`numpy.linspace`.
|
||
|
||
Args:
|
||
start: scalar or array of starting values.
|
||
stop: scalar or array of stop values.
|
||
num: number of values to generate. Default: 50.
|
||
endpoint: if True (default) then include the ``stop`` value in the result.
|
||
If False, then exclude the ``stop`` value.
|
||
retstep: If True, then return a ``(result, step)`` tuple, where ``step`` is the
|
||
interval between adjacent values in ``result``.
|
||
axis: integer axis along which to generate the linspace. Defaults to zero.
|
||
device: optional :class:`~jax.Device` or :class:`~jax.sharding.Sharding`
|
||
to which the created array will be committed.
|
||
|
||
Returns:
|
||
An array ``values``, or a tuple ``(values, step)`` if ``retstep`` is True, where:
|
||
|
||
- ``values`` is an array of evenly-spaced values from ``start`` to ``stop``
|
||
- ``step`` is the interval between adjacent values.
|
||
|
||
See also:
|
||
- :func:`jax.numpy.arange`: Generate ``N`` evenly-spaced values given a starting
|
||
point and a step
|
||
- :func:`jax.numpy.logspace`: Generate logarithmically-spaced values.
|
||
- :func:`jax.numpy.geomspace`: Generate geometrically-spaced values.
|
||
|
||
Examples:
|
||
List of 5 values between 0 and 10:
|
||
|
||
>>> jnp.linspace(0, 10, 5)
|
||
Array([ 0. , 2.5, 5. , 7.5, 10. ], dtype=float32)
|
||
|
||
List of 8 values between 0 and 10, excluding the endpoint:
|
||
|
||
>>> jnp.linspace(0, 10, 8, endpoint=False)
|
||
Array([0. , 1.25, 2.5 , 3.75, 5. , 6.25, 7.5 , 8.75], dtype=float32)
|
||
|
||
List of values and the step size between them
|
||
|
||
>>> vals, step = jnp.linspace(0, 10, 9, retstep=True)
|
||
>>> vals
|
||
Array([ 0. , 1.25, 2.5 , 3.75, 5. , 6.25, 7.5 , 8.75, 10. ], dtype=float32)
|
||
>>> step
|
||
Array(1.25, dtype=float32)
|
||
|
||
Multi-dimensional linspace:
|
||
|
||
>>> start = jnp.array([0, 5])
|
||
>>> stop = jnp.array([5, 10])
|
||
>>> jnp.linspace(start, stop, 5)
|
||
Array([[ 0. , 5. ],
|
||
[ 1.25, 6.25],
|
||
[ 2.5 , 7.5 ],
|
||
[ 3.75, 8.75],
|
||
[ 5. , 10. ]], dtype=float32)
|
||
"""
|
||
num = core.concrete_dim_or_error(num, "'num' argument of jnp.linspace")
|
||
axis = core.concrete_or_error(operator.index, axis, "'axis' argument of jnp.linspace")
|
||
return _linspace(start, stop, num, endpoint, retstep, dtype, axis, device=device)
|
||
|
||
@partial(jit, static_argnames=('num', 'endpoint', 'retstep', 'dtype', 'axis', 'device'))
|
||
def _linspace(start: ArrayLike, stop: ArrayLike, num: int = 50,
|
||
endpoint: bool = True, retstep: bool = False,
|
||
dtype: DTypeLike | None = None,
|
||
axis: int = 0,
|
||
*, device: xc.Device | Sharding | None = None) -> Array | tuple[Array, Array]:
|
||
"""Implementation of linspace differentiable in start and stop args."""
|
||
dtypes.check_user_dtype_supported(dtype, "linspace")
|
||
if num < 0:
|
||
raise ValueError(f"Number of samples, {num}, must be non-negative.")
|
||
start, stop = util.ensure_arraylike("linspace", start, stop)
|
||
|
||
if dtype is None:
|
||
dtype = dtypes.to_inexact_dtype(result_type(start, stop))
|
||
dtype = dtypes.jax_dtype(dtype)
|
||
computation_dtype = dtypes.to_inexact_dtype(dtype)
|
||
start = start.astype(computation_dtype)
|
||
stop = stop.astype(computation_dtype)
|
||
|
||
bounds_shape = list(lax.broadcast_shapes(np.shape(start), np.shape(stop)))
|
||
broadcast_start = broadcast_to(start, bounds_shape)
|
||
broadcast_stop = broadcast_to(stop, bounds_shape)
|
||
axis = len(bounds_shape) + axis + 1 if axis < 0 else axis
|
||
bounds_shape.insert(axis, 1)
|
||
div = (num - 1) if endpoint else num
|
||
if num > 1:
|
||
delta: Array = lax.convert_element_type(stop - start, computation_dtype) / array(div, dtype=computation_dtype)
|
||
iota_shape = [1,] * len(bounds_shape)
|
||
iota_shape[axis] = div
|
||
# This approach recovers the endpoints with float32 arithmetic,
|
||
# but can lead to rounding errors for integer outputs.
|
||
real_dtype = finfo(computation_dtype).dtype
|
||
step = reshape(lax.iota(real_dtype, div), iota_shape) / array(div, real_dtype)
|
||
step = step.astype(computation_dtype)
|
||
out = (reshape(broadcast_start, bounds_shape) * (1 - step) +
|
||
reshape(broadcast_stop, bounds_shape) * step)
|
||
|
||
if endpoint:
|
||
out = lax.concatenate([out, lax.expand_dims(broadcast_stop, (axis,))],
|
||
_canonicalize_axis(axis, out.ndim))
|
||
|
||
elif num == 1:
|
||
delta = asarray(np.nan if endpoint else stop - start, dtype=computation_dtype)
|
||
out = reshape(broadcast_start, bounds_shape)
|
||
else: # num == 0 degenerate case, match numpy behavior
|
||
empty_shape = list(lax.broadcast_shapes(np.shape(start), np.shape(stop)))
|
||
empty_shape.insert(axis, 0)
|
||
delta = asarray(np.nan, dtype=computation_dtype)
|
||
out = reshape(array([], dtype=dtype), empty_shape)
|
||
|
||
if issubdtype(dtype, np.integer) and not issubdtype(out.dtype, np.integer):
|
||
out = lax.floor(out)
|
||
|
||
sharding = canonicalize_device_to_sharding(device)
|
||
result = lax_internal._convert_element_type(out, dtype, sharding=sharding)
|
||
return (result, delta) if retstep else result
|
||
|
||
|
||
@export
|
||
def logspace(start: ArrayLike, stop: ArrayLike, num: int = 50,
|
||
endpoint: bool = True, base: ArrayLike = 10.0,
|
||
dtype: DTypeLike | None = None, axis: int = 0) -> Array:
|
||
"""Generate logarithmically-spaced values.
|
||
|
||
JAX implementation of :func:`numpy.logspace`.
|
||
|
||
Args:
|
||
start: scalar or array. Used to specify the start value. The start value is
|
||
``base ** start``.
|
||
stop: scalar or array. Used to specify the stop value. The end value is
|
||
``base ** stop``.
|
||
num: int, optional, default=50. Number of values to generate.
|
||
endpoint: bool, optional, default=True. If True, then include the ``stop`` value
|
||
in the result. If False, then exclude the ``stop`` value.
|
||
base: scalar or array, optional, default=10. Specifies the base of the logarithm.
|
||
dtype: optional. Specifies the dtype of the output.
|
||
axis: int, optional, default=0. Axis along which to generate the logspace.
|
||
|
||
Returns:
|
||
An array of logarithm.
|
||
|
||
See also:
|
||
- :func:`jax.numpy.arange`: Generate ``N`` evenly-spaced values given a starting
|
||
point and a step value.
|
||
- :func:`jax.numpy.linspace`: Generate evenly-spaced values.
|
||
- :func:`jax.numpy.geomspace`: Generate geometrically-spaced values.
|
||
|
||
Examples:
|
||
List 5 logarithmically spaced values between 1 (``10 ** 0``) and 100
|
||
(``10 ** 2``):
|
||
|
||
>>> with jnp.printoptions(precision=3, suppress=True):
|
||
... jnp.logspace(0, 2, 5)
|
||
Array([ 1. , 3.162, 10. , 31.623, 100. ], dtype=float32)
|
||
|
||
List 5 logarithmically-spaced values between 1(``10 ** 0``) and 100
|
||
(``10 ** 2``), excluding endpoint:
|
||
|
||
>>> with jnp.printoptions(precision=3, suppress=True):
|
||
... jnp.logspace(0, 2, 5, endpoint=False)
|
||
Array([ 1. , 2.512, 6.31 , 15.849, 39.811], dtype=float32)
|
||
|
||
List 7 logarithmically-spaced values between 1 (``2 ** 0``) and 4 (``2 ** 2``)
|
||
with base 2:
|
||
|
||
>>> with jnp.printoptions(precision=3, suppress=True):
|
||
... jnp.logspace(0, 2, 7, base=2)
|
||
Array([1. , 1.26 , 1.587, 2. , 2.52 , 3.175, 4. ], dtype=float32)
|
||
|
||
Multi-dimensional logspace:
|
||
|
||
>>> start = jnp.array([0, 5])
|
||
>>> stop = jnp.array([5, 0])
|
||
>>> base = jnp.array([2, 3])
|
||
>>> with jnp.printoptions(precision=3, suppress=True):
|
||
... jnp.logspace(start, stop, 5, base=base)
|
||
Array([[ 1. , 243. ],
|
||
[ 2.378, 61.547],
|
||
[ 5.657, 15.588],
|
||
[ 13.454, 3.948],
|
||
[ 32. , 1. ]], dtype=float32)
|
||
"""
|
||
num = core.concrete_or_error(operator.index, num, "'num' argument of jnp.logspace")
|
||
axis = core.concrete_or_error(operator.index, axis, "'axis' argument of jnp.logspace")
|
||
return _logspace(start, stop, num, endpoint, base, dtype, axis)
|
||
|
||
@partial(jit, static_argnames=('num', 'endpoint', 'dtype', 'axis'))
|
||
def _logspace(start: ArrayLike, stop: ArrayLike, num: int = 50,
|
||
endpoint: bool = True, base: ArrayLike = 10.0,
|
||
dtype: DTypeLike | None = None, axis: int = 0) -> Array:
|
||
"""Implementation of logspace differentiable in start and stop args."""
|
||
dtypes.check_user_dtype_supported(dtype, "logspace")
|
||
if dtype is None:
|
||
dtype = dtypes.to_inexact_dtype(result_type(start, stop))
|
||
dtype = dtypes.jax_dtype(dtype)
|
||
computation_dtype = dtypes.to_inexact_dtype(dtype)
|
||
start, stop = util.ensure_arraylike("logspace", start, stop)
|
||
start = start.astype(computation_dtype)
|
||
stop = stop.astype(computation_dtype)
|
||
lin = linspace(start, stop, num,
|
||
endpoint=endpoint, retstep=False, dtype=None, axis=axis)
|
||
return lax.convert_element_type(ufuncs.power(base, lin), dtype)
|
||
|
||
|
||
@export
|
||
def geomspace(start: ArrayLike, stop: ArrayLike, num: int = 50, endpoint: bool = True,
|
||
dtype: DTypeLike | None = None, axis: int = 0) -> Array:
|
||
"""Generate geometrically-spaced values.
|
||
|
||
JAX implementation of :func:`numpy.geomspace`.
|
||
|
||
Args:
|
||
start: scalar or array. Specifies the starting values.
|
||
stop: scalar or array. Specifies the stop values.
|
||
num: int, optional, default=50. Number of values to generate.
|
||
endpoint: bool, optional, default=True. If True, then include the ``stop`` value
|
||
in the result. If False, then exclude the ``stop`` value.
|
||
dtype: optional. Specifies the dtype of the output.
|
||
axis: int, optional, default=0. Axis along which to generate the geomspace.
|
||
|
||
Returns:
|
||
An array containing the geometrically-spaced values.
|
||
|
||
See also:
|
||
- :func:`jax.numpy.arange`: Generate ``N`` evenly-spaced values given a starting
|
||
point and a step value.
|
||
- :func:`jax.numpy.linspace`: Generate evenly-spaced values.
|
||
- :func:`jax.numpy.logspace`: Generate logarithmically-spaced values.
|
||
|
||
Examples:
|
||
List 5 geometrically-spaced values between 1 and 16:
|
||
|
||
>>> with jnp.printoptions(precision=3, suppress=True):
|
||
... jnp.geomspace(1, 16, 5)
|
||
Array([ 1., 2., 4., 8., 16.], dtype=float32)
|
||
|
||
List 4 geomtrically-spaced values between 1 and 16, with ``endpoint=False``:
|
||
|
||
>>> with jnp.printoptions(precision=3, suppress=True):
|
||
... jnp.geomspace(1, 16, 4, endpoint=False)
|
||
Array([1., 2., 4., 8.], dtype=float32)
|
||
|
||
Multi-dimensional geomspace:
|
||
|
||
>>> start = jnp.array([1, 1000])
|
||
>>> stop = jnp.array([27, 1])
|
||
>>> with jnp.printoptions(precision=3, suppress=True):
|
||
... jnp.geomspace(start, stop, 4)
|
||
Array([[ 1., 1000.],
|
||
[ 3., 100.],
|
||
[ 9., 10.],
|
||
[ 27., 1.]], dtype=float32)
|
||
"""
|
||
num = core.concrete_or_error(operator.index, num, "'num' argument of jnp.geomspace")
|
||
axis = core.concrete_or_error(operator.index, axis, "'axis' argument of jnp.geomspace")
|
||
return _geomspace(start, stop, num, endpoint, dtype, axis)
|
||
|
||
@partial(jit, static_argnames=('num', 'endpoint', 'dtype', 'axis'))
|
||
def _geomspace(start: ArrayLike, stop: ArrayLike, num: int = 50, endpoint: bool = True,
|
||
dtype: DTypeLike | None = None, axis: int = 0) -> Array:
|
||
"""Implementation of geomspace differentiable in start and stop args."""
|
||
dtypes.check_user_dtype_supported(dtype, "geomspace")
|
||
if dtype is None:
|
||
dtype = dtypes.to_inexact_dtype(result_type(start, stop))
|
||
dtype = dtypes.jax_dtype(dtype)
|
||
computation_dtype = dtypes.to_inexact_dtype(dtype)
|
||
start, stop = util.ensure_arraylike("geomspace", start, stop)
|
||
start = start.astype(computation_dtype)
|
||
stop = stop.astype(computation_dtype)
|
||
|
||
sign = ufuncs.sign(start)
|
||
res = sign * logspace(ufuncs.log10(start / sign), ufuncs.log10(stop / sign),
|
||
num, endpoint=endpoint, base=10.0,
|
||
dtype=computation_dtype, axis=0)
|
||
if axis != 0:
|
||
res = moveaxis(res, 0, axis)
|
||
return lax.convert_element_type(res, dtype)
|
||
|
||
|
||
@export
|
||
def meshgrid(*xi: ArrayLike, copy: bool = True, sparse: bool = False,
|
||
indexing: str = 'xy') -> list[Array]:
|
||
"""Construct N-dimensional grid arrays from N 1-dimensional vectors.
|
||
|
||
JAX implementation of :func:`numpy.meshgrid`.
|
||
|
||
Args:
|
||
xi: N arrays to convert to a grid.
|
||
copy: whether to copy the input arrays. JAX supports only ``copy=True``,
|
||
though under JIT compilation the compiler may opt to avoid copies.
|
||
sparse: if False (default), then each returned arrays will be of shape
|
||
``[len(x1), len(x2), ..., len(xN)]``. If False, then returned arrays
|
||
will be of shape ``[1, 1, ..., len(xi), ..., 1, 1]``.
|
||
indexing: options are ``'xy'`` for cartesian indexing (default) or ``'ij'``
|
||
for matrix indexing.
|
||
|
||
Returns:
|
||
A length-N list of grid arrays.
|
||
|
||
See also:
|
||
- :func:`jax.numpy.indices`: generate a grid of indices.
|
||
- :obj:`jax.numpy.mgrid`: create a meshgrid using indexing syntax.
|
||
- :obj:`jax.numpy.ogrid`: create an open meshgrid using indexing syntax.
|
||
|
||
Examples:
|
||
For the following examples, we'll use these 1D arrays as inputs:
|
||
|
||
>>> x = jnp.array([1, 2])
|
||
>>> y = jnp.array([10, 20, 30])
|
||
|
||
2D cartesian mesh grid:
|
||
|
||
>>> x_grid, y_grid = jnp.meshgrid(x, y)
|
||
>>> print(x_grid)
|
||
[[1 2]
|
||
[1 2]
|
||
[1 2]]
|
||
>>> print(y_grid)
|
||
[[10 10]
|
||
[20 20]
|
||
[30 30]]
|
||
|
||
2D sparse cartesian mesh grid:
|
||
|
||
>>> x_grid, y_grid = jnp.meshgrid(x, y, sparse=True)
|
||
>>> print(x_grid)
|
||
[[1 2]]
|
||
>>> print(y_grid)
|
||
[[10]
|
||
[20]
|
||
[30]]
|
||
|
||
2D matrix-index mesh grid:
|
||
|
||
>>> x_grid, y_grid = jnp.meshgrid(x, y, indexing='ij')
|
||
>>> print(x_grid)
|
||
[[1 1 1]
|
||
[2 2 2]]
|
||
>>> print(y_grid)
|
||
[[10 20 30]
|
||
[10 20 30]]
|
||
"""
|
||
args = list(util.ensure_arraylike_tuple("meshgrid", tuple(xi)))
|
||
if not copy:
|
||
raise ValueError("jax.numpy.meshgrid only supports copy=True")
|
||
if indexing not in ["xy", "ij"]:
|
||
raise ValueError(f"Valid values for indexing are 'xy' and 'ij', got {indexing}")
|
||
if any(a.ndim != 1 for a in args):
|
||
raise ValueError("Arguments to jax.numpy.meshgrid must be 1D, got shapes "
|
||
f"{[a.shape for a in args]}")
|
||
if indexing == "xy" and len(args) >= 2:
|
||
args[0], args[1] = args[1], args[0]
|
||
shape = [1 if sparse else a.shape[0] for a in args]
|
||
_a_shape = lambda i, a: [*shape[:i], a.shape[0], *shape[i + 1:]] if sparse else shape
|
||
output = [lax.broadcast_in_dim(a, _a_shape(i, a), (i,)) for i, a, in enumerate(args)]
|
||
if indexing == "xy" and len(args) >= 2:
|
||
output[0], output[1] = output[1], output[0]
|
||
return output
|
||
|
||
|
||
@export
|
||
@jit
|
||
def i0(x: ArrayLike) -> Array:
|
||
r"""Calculate modified Bessel function of first kind, zeroth order.
|
||
|
||
JAX implementation of :func:`numpy.i0`.
|
||
|
||
Modified Bessel function of first kind, zeroth order is defined by:
|
||
|
||
.. math::
|
||
|
||
\mathrm{i0}(x) = I_0(x) = \sum_{k=0}^{\infty} \frac{(x^2/4)^k}{(k!)^2}
|
||
|
||
Args:
|
||
x: scalar or array. Specifies the argument of Bessel function. Complex inputs
|
||
are not supported.
|
||
|
||
Returns:
|
||
An array containing the corresponding values of the modified Bessel function
|
||
of ``x``.
|
||
|
||
See also:
|
||
- :func:`jax.scipy.special.i0`: Calculates the modified Bessel function of
|
||
zeroth order.
|
||
- :func:`jax.scipy.special.i1`: Calculates the modified Bessel function of
|
||
first order.
|
||
- :func:`jax.scipy.special.i0e`: Calculates the exponentially scaled modified
|
||
Bessel function of zeroth order.
|
||
|
||
Examples:
|
||
>>> x = jnp.array([-2, -1, 0, 1, 2])
|
||
>>> jnp.i0(x)
|
||
Array([2.2795851, 1.266066 , 1.0000001, 1.266066 , 2.2795851], dtype=float32)
|
||
"""
|
||
x_arr, = util.promote_args_inexact("i0", x)
|
||
if not issubdtype(x_arr.dtype, np.floating):
|
||
raise ValueError(f"Unsupported input type to jax.numpy.i0: {_dtype(x)}")
|
||
return _i0(x_arr)
|
||
|
||
|
||
@custom_jvp
|
||
def _i0(x):
|
||
abs_x = lax.abs(x)
|
||
return lax.mul(lax.exp(abs_x), lax.bessel_i0e(abs_x))
|
||
|
||
@_i0.defjvp
|
||
def _i0_jvp(primals, tangents):
|
||
primal_out, tangent_out = jax.jvp(_i0.fun, primals, tangents)
|
||
return primal_out, where(primals[0] == 0, 0.0, tangent_out)
|
||
|
||
@export
|
||
def ix_(*args: ArrayLike) -> tuple[Array, ...]:
|
||
"""Return a multi-dimensional grid (open mesh) from N one-dimensional sequences.
|
||
|
||
JAX implementation of :func:`numpy.ix_`.
|
||
|
||
Args:
|
||
*args: N one-dimensional arrays
|
||
|
||
Returns:
|
||
Tuple of Jax arrays forming an open mesh, each with N dimensions.
|
||
|
||
See Also:
|
||
- :obj:`jax.numpy.ogrid`
|
||
- :obj:`jax.numpy.mgrid`
|
||
- :func:`jax.numpy.meshgrid`
|
||
|
||
Examples:
|
||
>>> rows = jnp.array([0, 2])
|
||
>>> cols = jnp.array([1, 3])
|
||
>>> open_mesh = jnp.ix_(rows, cols)
|
||
>>> open_mesh
|
||
(Array([[0],
|
||
[2]], dtype=int32), Array([[1, 3]], dtype=int32))
|
||
>>> [grid.shape for grid in open_mesh]
|
||
[(2, 1), (1, 2)]
|
||
>>> x = jnp.array([[10, 20, 30, 40],
|
||
... [50, 60, 70, 80],
|
||
... [90, 100, 110, 120],
|
||
... [130, 140, 150, 160]])
|
||
>>> x[open_mesh]
|
||
Array([[ 20, 40],
|
||
[100, 120]], dtype=int32)
|
||
"""
|
||
args = util.ensure_arraylike_tuple("ix", args)
|
||
n = len(args)
|
||
output = []
|
||
for i, a in enumerate(args):
|
||
if len(a.shape) != 1:
|
||
msg = "Arguments to jax.numpy.ix_ must be 1-dimensional, got shape {}"
|
||
raise ValueError(msg.format(a.shape))
|
||
if _dtype(a) == bool:
|
||
raise NotImplementedError(
|
||
"Boolean arguments to jax.numpy.ix_ are not implemented")
|
||
shape = [1] * n
|
||
shape[i] = a.shape[0]
|
||
if a.size == 0:
|
||
# Numpy uses an integer index type for empty arrays.
|
||
output.append(lax.full(shape, np.zeros((), np.intp)))
|
||
else:
|
||
output.append(lax.broadcast_in_dim(a, shape, (i,)))
|
||
return tuple(output)
|
||
|
||
|
||
@overload
|
||
def indices(dimensions: Sequence[int], dtype: DTypeLike | None = None,
|
||
sparse: Literal[False] = False) -> Array: ...
|
||
@overload
|
||
def indices(dimensions: Sequence[int], dtype: DTypeLike | None = None,
|
||
*, sparse: Literal[True]) -> tuple[Array, ...]: ...
|
||
@overload
|
||
def indices(dimensions: Sequence[int], dtype: DTypeLike | None = None,
|
||
sparse: bool = False) -> Array | tuple[Array, ...]: ...
|
||
@export
|
||
def indices(dimensions: Sequence[int], dtype: DTypeLike | None = None,
|
||
sparse: bool = False) -> Array | tuple[Array, ...]:
|
||
"""Generate arrays of grid indices.
|
||
|
||
JAX implementation of :func:`numpy.indices`.
|
||
|
||
Args:
|
||
dimensions: the shape of the grid.
|
||
dtype: the dtype of the indices (defaults to integer).
|
||
sparse: if True, then return sparse indices. Default is False, which
|
||
returns dense indices.
|
||
|
||
Returns:
|
||
An array of shape ``(len(dimensions), *dimensions)`` If ``sparse`` is False,
|
||
or a sequence of arrays of the same length as ``dimensions`` if ``sparse`` is True.
|
||
|
||
See also:
|
||
- :func:`jax.numpy.meshgrid`: generate a grid from arbitrary input arrays.
|
||
- :obj:`jax.numpy.mgrid`: generate dense indices using a slicing syntax.
|
||
- :obj:`jax.numpy.ogrid`: generate sparse indices using a slicing syntax.
|
||
|
||
Examples:
|
||
>>> jnp.indices((2, 3))
|
||
Array([[[0, 0, 0],
|
||
[1, 1, 1]],
|
||
<BLANKLINE>
|
||
[[0, 1, 2],
|
||
[0, 1, 2]]], dtype=int32)
|
||
>>> jnp.indices((2, 3), sparse=True)
|
||
(Array([[0],
|
||
[1]], dtype=int32), Array([[0, 1, 2]], dtype=int32))
|
||
"""
|
||
dtypes.check_user_dtype_supported(dtype, "indices")
|
||
dtype = dtype or dtypes.canonicalize_dtype(dtypes.int_)
|
||
dimensions = tuple(
|
||
core.concrete_or_error(operator.index, d, "dimensions argument of jnp.indices")
|
||
for d in dimensions)
|
||
N = len(dimensions)
|
||
output = []
|
||
s = dimensions
|
||
for i, dim in enumerate(dimensions):
|
||
idx = lax.iota(dtype, dim)
|
||
if sparse:
|
||
s = (1,)*i + (dim,) + (1,)*(N - i - 1)
|
||
output.append(lax.broadcast_in_dim(idx, s, (i,)))
|
||
if sparse:
|
||
return tuple(output)
|
||
return stack(output, 0) if output else array([], dtype=dtype)
|
||
|
||
|
||
@export
|
||
def repeat(a: ArrayLike, repeats: ArrayLike, axis: int | None = None, *,
|
||
total_repeat_length: int | None = None) -> Array:
|
||
"""Construct an array from repeated elements.
|
||
|
||
JAX implementation of :func:`numpy.repeat`.
|
||
|
||
Args:
|
||
a: N-dimensional array
|
||
repeats: 1D integer array specifying the number of repeats. Must match the
|
||
length of the repeated axis.
|
||
axis: integer specifying the axis of ``a`` along which to construct the
|
||
repeated array. If None (default) then ``a`` is first flattened.
|
||
total_repeat_length: this must be specified statically for ``jnp.repeat``
|
||
to be compatible with :func:`~jax.jit` and other JAX transformations.
|
||
If ``sum(repeats)`` is larger than the specified ``total_repeat_length``,
|
||
the remaining values will be discarded. If ``sum(repeats)`` is smaller
|
||
than ``total_repeat_length``, the final value will be repeated.
|
||
|
||
Returns:
|
||
an array constructed from repeated values of ``a``.
|
||
|
||
See Also:
|
||
- :func:`jax.numpy.tile`: repeat a full array rather than individual values.
|
||
|
||
Examples:
|
||
Repeat each value twice along the last axis:
|
||
|
||
>>> a = jnp.array([[1, 2],
|
||
... [3, 4]])
|
||
>>> jnp.repeat(a, 2, axis=-1)
|
||
Array([[1, 1, 2, 2],
|
||
[3, 3, 4, 4]], dtype=int32)
|
||
|
||
If ``axis`` is not specified, the input array will be flattened:
|
||
|
||
>>> jnp.repeat(a, 2)
|
||
Array([1, 1, 2, 2, 3, 3, 4, 4], dtype=int32)
|
||
|
||
Pass an array to ``repeats`` to repeat each value a different number of times:
|
||
|
||
>>> repeats = jnp.array([2, 3])
|
||
>>> jnp.repeat(a, repeats, axis=1)
|
||
Array([[1, 1, 2, 2, 2],
|
||
[3, 3, 4, 4, 4]], dtype=int32)
|
||
|
||
In order to use ``repeat`` within ``jit`` and other JAX transformations, the
|
||
size of the output must be specified statically using ``total_repeat_length``:
|
||
|
||
>>> jit_repeat = jax.jit(jnp.repeat, static_argnames=['axis', 'total_repeat_length'])
|
||
>>> jit_repeat(a, repeats, axis=1, total_repeat_length=5)
|
||
Array([[1, 1, 2, 2, 2],
|
||
[3, 3, 4, 4, 4]], dtype=int32)
|
||
|
||
If `total_repeat_length` is smaller than ``sum(repeats)``, the result will be truncated:
|
||
|
||
>>> jit_repeat(a, repeats, axis=1, total_repeat_length=4)
|
||
Array([[1, 1, 2, 2],
|
||
[3, 3, 4, 4]], dtype=int32)
|
||
|
||
If it is larger, then the additional entries will be filled with the final value:
|
||
|
||
>>> jit_repeat(a, repeats, axis=1, total_repeat_length=7)
|
||
Array([[1, 1, 2, 2, 2, 2, 2],
|
||
[3, 3, 4, 4, 4, 4, 4]], dtype=int32)
|
||
"""
|
||
arr = util.ensure_arraylike("repeat", a)
|
||
core.is_dim(repeats) or util.check_arraylike("repeat", repeats)
|
||
|
||
if axis is None:
|
||
arr = arr.ravel()
|
||
axis = 0
|
||
|
||
axis = core.concrete_or_error(operator.index, axis, "'axis' argument of jnp.repeat()")
|
||
assert isinstance(axis, int) # to appease mypy
|
||
|
||
if core.is_symbolic_dim(repeats):
|
||
if total_repeat_length is not None:
|
||
raise ValueError("jnp.repeat with a non-constant `repeats` is supported only "
|
||
f"when `total_repeat_length` is None. ({repeats=} {total_repeat_length=})")
|
||
|
||
# If total_repeat_length is not given, use a default.
|
||
if total_repeat_length is None:
|
||
repeats = core.concrete_or_error(None, repeats,
|
||
"When jit-compiling jnp.repeat, the total number of repeats must be static. "
|
||
"To fix this, either specify a static value for `repeats`, or pass a static "
|
||
"value to `total_repeat_length`.")
|
||
|
||
# Fast path for when repeats is a scalar.
|
||
if np.ndim(repeats) == 0 and np.ndim(arr) != 0:
|
||
input_shape = arr.shape
|
||
axis = _canonicalize_axis(axis, len(input_shape))
|
||
aux_axis = axis + 1
|
||
aux_shape: list[DimSize] = list(input_shape)
|
||
aux_shape.insert(aux_axis, operator.index(repeats) if core.is_constant_dim(repeats) else repeats) # type: ignore
|
||
arr = lax.broadcast_in_dim(
|
||
arr, aux_shape, [i for i in range(len(aux_shape)) if i != aux_axis])
|
||
result_shape: list[DimSize] = list(input_shape)
|
||
result_shape[axis] *= repeats
|
||
return arr.reshape(result_shape)
|
||
|
||
repeats = np.ravel(repeats)
|
||
if arr.ndim != 0:
|
||
repeats = np.broadcast_to(repeats, [arr.shape[axis]])
|
||
total_repeat_length = np.sum(repeats)
|
||
else:
|
||
repeats = ravel(repeats)
|
||
if arr.ndim != 0:
|
||
repeats = broadcast_to(repeats, [arr.shape[axis]])
|
||
|
||
# Special case when a is a scalar.
|
||
if arr.ndim == 0:
|
||
if np.shape(repeats) == (1,):
|
||
return full([total_repeat_length], arr)
|
||
else:
|
||
raise ValueError('`repeat` with a scalar parameter `a` is only '
|
||
'implemented for scalar values of the parameter `repeats`.')
|
||
|
||
# Special case if total_repeat_length is zero.
|
||
if total_repeat_length == 0:
|
||
result_shape = list(arr.shape)
|
||
result_shape[axis] = 0
|
||
return reshape(array([], dtype=arr.dtype), result_shape)
|
||
|
||
# If repeats is on a zero sized axis, then return the array.
|
||
if arr.shape[axis] == 0:
|
||
return arr
|
||
|
||
# This implementation of repeat avoid having to instantiate a large.
|
||
# intermediate tensor.
|
||
|
||
# Modify repeats from e.g. [1,2,0,5] -> [0,1,2,0] for exclusive repeat.
|
||
exclusive_repeats = roll(repeats, shift=1).at[0].set(0)
|
||
# Cumsum to get indices of new number in repeated tensor, e.g. [0, 1, 3, 3]
|
||
scatter_indices = reductions.cumsum(exclusive_repeats)
|
||
# Scatter these onto a zero buffer, e.g. [1,1,0,2,0,0,0,0]
|
||
block_split_indicators = zeros([total_repeat_length], dtype='int32')
|
||
block_split_indicators = block_split_indicators.at[scatter_indices].add(1)
|
||
# Cumsum again to get scatter indices for repeat, e.g. [0,1,1,3,3,3,3,3]
|
||
gather_indices = reductions.cumsum(block_split_indicators) - 1
|
||
return indexing.take(arr, gather_indices, axis=axis)
|
||
|
||
|
||
@export
|
||
@partial(jit, static_argnames=('axis',))
|
||
def trapezoid(y: ArrayLike, x: ArrayLike | None = None, dx: ArrayLike = 1.0,
|
||
axis: int = -1) -> Array:
|
||
r"""
|
||
Integrate along the given axis using the composite trapezoidal rule.
|
||
|
||
JAX implementation of :func:`numpy.trapezoid`
|
||
|
||
The trapezoidal rule approximates the integral under a curve by summing the
|
||
areas of trapezoids formed between adjacent data points.
|
||
|
||
Args:
|
||
y: array of data to integrate.
|
||
x: optional array of sample points corresponding to the ``y`` values. If not
|
||
provided, ``x`` defaults to equally spaced with spacing given by ``dx``.
|
||
dx: The spacing between sample points when `x` is None (default: 1.0).
|
||
axis: The axis along which to integrate (default: -1)
|
||
|
||
Returns:
|
||
The definite integral approximated by the trapezoidal rule.
|
||
|
||
Examples:
|
||
Integrate over a regular grid, with spacing 1.0:
|
||
|
||
>>> y = jnp.array([1, 2, 3, 2, 3, 2, 1])
|
||
>>> jnp.trapezoid(y, dx=1.0)
|
||
Array(13., dtype=float32)
|
||
|
||
Integrate over an irregular grid:
|
||
|
||
>>> x = jnp.array([0, 2, 5, 7, 10, 15, 20])
|
||
>>> jnp.trapezoid(y, x)
|
||
Array(43., dtype=float32)
|
||
|
||
Approximate :math:`\int_0^{2\pi} \sin^2(x)dx`, which equals :math:`\pi`:
|
||
|
||
>>> x = jnp.linspace(0, 2 * jnp.pi, 1000)
|
||
>>> y = jnp.sin(x) ** 2
|
||
>>> result = jnp.trapezoid(y, x)
|
||
>>> jnp.allclose(result, jnp.pi)
|
||
Array(True, dtype=bool)
|
||
"""
|
||
# TODO(phawkins): remove this annotation after fixing jnp types.
|
||
dx_array: Array
|
||
if x is None:
|
||
util.check_arraylike('trapezoid', y)
|
||
y_arr, = util.promote_dtypes_inexact(y)
|
||
dx_array = asarray(dx)
|
||
else:
|
||
util.check_arraylike('trapezoid', y, x)
|
||
y_arr, x_arr = util.promote_dtypes_inexact(y, x)
|
||
if x_arr.ndim == 1:
|
||
dx_array = diff(x_arr)
|
||
else:
|
||
dx_array = moveaxis(diff(x_arr, axis=axis), axis, -1)
|
||
y_arr = moveaxis(y_arr, axis, -1)
|
||
return 0.5 * (dx_array * (y_arr[..., 1:] + y_arr[..., :-1])).sum(-1)
|
||
|
||
|
||
@export
|
||
def tri(N: int, M: int | None = None, k: int = 0, dtype: DTypeLike | None = None) -> Array:
|
||
r"""Return an array with ones on and below the diagonal and zeros elsewhere.
|
||
|
||
JAX implementation of :func:`numpy.tri`
|
||
|
||
Args:
|
||
N: int. Dimension of the rows of the returned array.
|
||
M: optional, int. Dimension of the columns of the returned array. If not
|
||
specified, then ``M = N``.
|
||
k: optional, int, default=0. Specifies the sub-diagonal on and below which
|
||
the array is filled with ones. ``k=0`` refers to main diagonal, ``k<0``
|
||
refers to sub-diagonal below the main diagonal and ``k>0`` refers to
|
||
sub-diagonal above the main diagonal.
|
||
dtype: optional, data type of the returned array. The default type is float.
|
||
|
||
Returns:
|
||
An array of shape ``(N, M)`` containing the lower triangle with elements
|
||
below the sub-diagonal specified by ``k`` are set to one and zero elsewhere.
|
||
|
||
See also:
|
||
- :func:`jax.numpy.tril`: Returns a lower triangle of an array.
|
||
- :func:`jax.numpy.triu`: Returns an upper triangle of an array.
|
||
|
||
Examples:
|
||
>>> jnp.tri(3)
|
||
Array([[1., 0., 0.],
|
||
[1., 1., 0.],
|
||
[1., 1., 1.]], dtype=float32)
|
||
|
||
When ``M`` is not equal to ``N``:
|
||
|
||
>>> jnp.tri(3, 4)
|
||
Array([[1., 0., 0., 0.],
|
||
[1., 1., 0., 0.],
|
||
[1., 1., 1., 0.]], dtype=float32)
|
||
|
||
when ``k>0``:
|
||
|
||
>>> jnp.tri(3, k=1)
|
||
Array([[1., 1., 0.],
|
||
[1., 1., 1.],
|
||
[1., 1., 1.]], dtype=float32)
|
||
|
||
When ``k<0``:
|
||
|
||
>>> jnp.tri(3, 4, k=-1)
|
||
Array([[0., 0., 0., 0.],
|
||
[1., 0., 0., 0.],
|
||
[1., 1., 0., 0.]], dtype=float32)
|
||
"""
|
||
dtypes.check_user_dtype_supported(dtype, "tri")
|
||
M = M if M is not None else N
|
||
dtype = dtype or np.dtype('float32')
|
||
return lax_internal._tri(dtype, (N, M), k)
|
||
|
||
|
||
@export
|
||
@partial(jit, static_argnames=('k',))
|
||
def tril(m: ArrayLike, k: int = 0) -> Array:
|
||
r"""Return lower triangle of an array.
|
||
|
||
JAX implementation of :func:`numpy.tril`
|
||
|
||
Args:
|
||
m: input array. Must have ``m.ndim >= 2``.
|
||
k: k: optional, int, default=0. Specifies the sub-diagonal above which the
|
||
elements of the array are set to zero. ``k=0`` refers to main diagonal,
|
||
``k<0`` refers to sub-diagonal below the main diagonal and ``k>0`` refers
|
||
to sub-diagonal above the main diagonal.
|
||
|
||
Returns:
|
||
An array with same shape as input containing the lower triangle of the given
|
||
array with elements above the sub-diagonal specified by ``k`` are set to
|
||
zero.
|
||
|
||
See also:
|
||
- :func:`jax.numpy.triu`: Returns an upper triangle of an array.
|
||
- :func:`jax.numpy.tri`: Returns an array with ones on and below the
|
||
diagonal and zeros elsewhere.
|
||
|
||
Examples:
|
||
>>> x = jnp.array([[1, 2, 3, 4],
|
||
... [5, 6, 7, 8],
|
||
... [9, 10, 11, 12]])
|
||
>>> jnp.tril(x)
|
||
Array([[ 1, 0, 0, 0],
|
||
[ 5, 6, 0, 0],
|
||
[ 9, 10, 11, 0]], dtype=int32)
|
||
>>> jnp.tril(x, k=1)
|
||
Array([[ 1, 2, 0, 0],
|
||
[ 5, 6, 7, 0],
|
||
[ 9, 10, 11, 12]], dtype=int32)
|
||
>>> jnp.tril(x, k=-1)
|
||
Array([[ 0, 0, 0, 0],
|
||
[ 5, 0, 0, 0],
|
||
[ 9, 10, 0, 0]], dtype=int32)
|
||
|
||
When ``m.ndim > 2``, ``jnp.tril`` operates batch-wise on the trailing axes.
|
||
|
||
>>> x1 = jnp.array([[[1, 2],
|
||
... [3, 4]],
|
||
... [[5, 6],
|
||
... [7, 8]]])
|
||
>>> jnp.tril(x1)
|
||
Array([[[1, 0],
|
||
[3, 4]],
|
||
<BLANKLINE>
|
||
[[5, 0],
|
||
[7, 8]]], dtype=int32)
|
||
"""
|
||
util.check_arraylike("tril", m)
|
||
m_shape = np.shape(m)
|
||
if len(m_shape) < 2:
|
||
raise ValueError("Argument to jax.numpy.tril must be at least 2D")
|
||
N, M = m_shape[-2:]
|
||
mask = tri(N, M, k=k, dtype=bool)
|
||
return lax.select(lax.broadcast(mask, m_shape[:-2]), m, zeros_like(m))
|
||
|
||
|
||
@export
|
||
@partial(jit, static_argnames=('k',))
|
||
def triu(m: ArrayLike, k: int = 0) -> Array:
|
||
r"""Return upper triangle of an array.
|
||
|
||
JAX implementation of :func:`numpy.triu`
|
||
|
||
Args:
|
||
m: input array. Must have ``m.ndim >= 2``.
|
||
k: optional, int, default=0. Specifies the sub-diagonal below which the
|
||
elements of the array are set to zero. ``k=0`` refers to main diagonal,
|
||
``k<0`` refers to sub-diagonal below the main diagonal and ``k>0`` refers
|
||
to sub-diagonal above the main diagonal.
|
||
|
||
Returns:
|
||
An array with same shape as input containing the upper triangle of the given
|
||
array with elements below the sub-diagonal specified by ``k`` are set to
|
||
zero.
|
||
|
||
See also:
|
||
- :func:`jax.numpy.tril`: Returns a lower triangle of an array.
|
||
- :func:`jax.numpy.tri`: Returns an array with ones on and below the
|
||
diagonal and zeros elsewhere.
|
||
|
||
Examples:
|
||
>>> x = jnp.array([[1, 2, 3],
|
||
... [4, 5, 6],
|
||
... [7, 8, 9],
|
||
... [10, 11, 12]])
|
||
>>> jnp.triu(x)
|
||
Array([[1, 2, 3],
|
||
[0, 5, 6],
|
||
[0, 0, 9],
|
||
[0, 0, 0]], dtype=int32)
|
||
>>> jnp.triu(x, k=1)
|
||
Array([[0, 2, 3],
|
||
[0, 0, 6],
|
||
[0, 0, 0],
|
||
[0, 0, 0]], dtype=int32)
|
||
>>> jnp.triu(x, k=-1)
|
||
Array([[ 1, 2, 3],
|
||
[ 4, 5, 6],
|
||
[ 0, 8, 9],
|
||
[ 0, 0, 12]], dtype=int32)
|
||
|
||
When ``m.ndim > 2``, ``jnp.triu`` operates batch-wise on the trailing axes.
|
||
|
||
>>> x1 = jnp.array([[[1, 2],
|
||
... [3, 4]],
|
||
... [[5, 6],
|
||
... [7, 8]]])
|
||
>>> jnp.triu(x1)
|
||
Array([[[1, 2],
|
||
[0, 4]],
|
||
<BLANKLINE>
|
||
[[5, 6],
|
||
[0, 8]]], dtype=int32)
|
||
"""
|
||
util.check_arraylike("triu", m)
|
||
m_shape = np.shape(m)
|
||
if len(m_shape) < 2:
|
||
raise ValueError("Argument to jax.numpy.triu must be at least 2D")
|
||
N, M = m_shape[-2:]
|
||
mask = tri(N, M, k=k - 1, dtype=bool)
|
||
return lax.select(lax.broadcast(mask, m_shape[:-2]), zeros_like(m), m)
|
||
|
||
|
||
@export
|
||
@partial(jit, static_argnames=('axis1', 'axis2', 'dtype'))
|
||
def trace(a: ArrayLike, offset: int | ArrayLike = 0, axis1: int = 0, axis2: int = 1,
|
||
dtype: DTypeLike | None = None, out: None = None) -> Array:
|
||
"""Calculate sum of the diagonal of input along the given axes.
|
||
|
||
JAX implementation of :func:`numpy.trace`.
|
||
|
||
Args:
|
||
a: input array. Must have ``a.ndim >= 2``.
|
||
offset: optional, int, default=0. Diagonal offset from the main diagonal.
|
||
Can be positive or negative.
|
||
axis1: optional, default=0. The first axis along which to take the sum of
|
||
diagonal. Must be a static integer value.
|
||
axis2: optional, default=1. The second axis along which to take the sum of
|
||
diagonal. Must be a static integer value.
|
||
dtype: optional. The dtype of the output array. Should be provided as static
|
||
argument in JIT compilation.
|
||
out: Not used by JAX.
|
||
|
||
Returns:
|
||
An array of dimension x.ndim-2 containing the sum of the diagonal elements
|
||
along axes (axis1, axis2)
|
||
|
||
See also:
|
||
- :func:`jax.numpy.diag`: Returns the specified diagonal or constructs a diagonal
|
||
array
|
||
- :func:`jax.numpy.diagonal`: Returns the specified diagonal of an array.
|
||
- :func:`jax.numpy.diagflat`: Returns a 2-D array with the flattened input array
|
||
laid out on the diagonal.
|
||
|
||
Examples:
|
||
>>> x = jnp.arange(1, 9).reshape(2, 2, 2)
|
||
>>> x
|
||
Array([[[1, 2],
|
||
[3, 4]],
|
||
<BLANKLINE>
|
||
[[5, 6],
|
||
[7, 8]]], dtype=int32)
|
||
>>> jnp.trace(x)
|
||
Array([ 8, 10], dtype=int32)
|
||
>>> jnp.trace(x, offset=1)
|
||
Array([3, 4], dtype=int32)
|
||
>>> jnp.trace(x, axis1=1, axis2=2)
|
||
Array([ 5, 13], dtype=int32)
|
||
>>> jnp.trace(x, offset=1, axis1=1, axis2=2)
|
||
Array([2, 6], dtype=int32)
|
||
"""
|
||
util.check_arraylike("trace", a)
|
||
if out is not None:
|
||
raise NotImplementedError("The 'out' argument to jnp.trace is not supported.")
|
||
|
||
if _canonicalize_axis(axis1, np.ndim(a)) == _canonicalize_axis(axis2, np.ndim(a)):
|
||
raise ValueError(f"axis1 and axis2 can not be same. axis1={axis1} and axis2={axis2}")
|
||
|
||
dtypes.check_user_dtype_supported(dtype, "trace")
|
||
|
||
a_shape = np.shape(a)
|
||
a = moveaxis(a, (axis1, axis2), (-2, -1))
|
||
|
||
# Mask out the diagonal and reduce.
|
||
a = where(eye(a_shape[axis1], a_shape[axis2], k=offset, dtype=bool),
|
||
a, zeros_like(a))
|
||
return reductions.sum(a, axis=(-2, -1), dtype=dtype)
|
||
|
||
|
||
@export
|
||
def mask_indices(n: int,
|
||
mask_func: Callable[[ArrayLike, int], Array],
|
||
k: int = 0, *, size: int | None = None) -> tuple[Array, Array]:
|
||
"""Return indices of a mask of an (n, n) array.
|
||
|
||
Args:
|
||
n: static integer array dimension.
|
||
mask_func: a function that takes a shape ``(n, n)`` array and
|
||
an optional offset ``k``, and returns a shape ``(n, n)`` mask.
|
||
Examples of functions with this signature are
|
||
:func:`~jax.numpy.triu` and :func:`~jax.numpy.tril`.
|
||
k: a scalar value passed to ``mask_func``.
|
||
size: optional argument specifying the static size of the output arrays.
|
||
This is passed to :func:`~jax.numpy.nonzero` when generating the indices
|
||
from the mask.
|
||
|
||
Returns:
|
||
a tuple of indices where ``mask_func`` is nonzero.
|
||
|
||
See also:
|
||
- :func:`jax.numpy.triu_indices`: compute ``mask_indices`` for :func:`~jax.numpy.triu`.
|
||
- :func:`jax.numpy.tril_indices`: compute ``mask_indices`` for :func:`~jax.numpy.tril`.
|
||
|
||
Examples:
|
||
Calling ``mask_indices`` on built-in masking functions:
|
||
|
||
>>> jnp.mask_indices(3, jnp.triu)
|
||
(Array([0, 0, 0, 1, 1, 2], dtype=int32), Array([0, 1, 2, 1, 2, 2], dtype=int32))
|
||
|
||
>>> jnp.mask_indices(3, jnp.tril)
|
||
(Array([0, 1, 1, 2, 2, 2], dtype=int32), Array([0, 0, 1, 0, 1, 2], dtype=int32))
|
||
|
||
Calling ``mask_indices`` on a custom masking function:
|
||
|
||
>>> def mask_func(x, k=0):
|
||
... i = jnp.arange(x.shape[0])[:, None]
|
||
... j = jnp.arange(x.shape[1])
|
||
... return (i + 1) % (j + 1 + k) == 0
|
||
>>> mask_func(jnp.ones((3, 3)))
|
||
Array([[ True, False, False],
|
||
[ True, True, False],
|
||
[ True, False, True]], dtype=bool)
|
||
>>> jnp.mask_indices(3, mask_func)
|
||
(Array([0, 1, 1, 2, 2], dtype=int32), Array([0, 0, 1, 0, 2], dtype=int32))
|
||
"""
|
||
i, j = nonzero(mask_func(ones((n, n)), k), size=size)
|
||
return (i, j)
|
||
|
||
|
||
def _triu_size(n, m, k):
|
||
if k < 0:
|
||
return n * m - _triu_size(m, n, (1 - k))
|
||
elif k >= m:
|
||
return 0
|
||
else:
|
||
mk = min(n, m - k)
|
||
return mk * (mk + 1) // 2 + mk * (m - k - mk)
|
||
|
||
|
||
@export
|
||
def triu_indices(n: int, k: int = 0, m: int | None = None) -> tuple[Array, Array]:
|
||
"""Return the indices of upper triangle of an array of size ``(n, m)``.
|
||
|
||
JAX implementation of :func:`numpy.triu_indices`.
|
||
|
||
Args:
|
||
n: int. Number of rows of the array for which the indices are returned.
|
||
k: optional, int, default=0. Specifies the sub-diagonal on and above which
|
||
the indices of upper triangle are returned. ``k=0`` refers to main diagonal,
|
||
``k<0`` refers to sub-diagonal below the main diagonal and ``k>0`` refers
|
||
to sub-diagonal above the main diagonal.
|
||
m: optional, int. Number of columns of the array for which the indices are
|
||
returned. If not specified, then ``m = n``.
|
||
|
||
Returns:
|
||
A tuple of two arrays containing the indices of the upper triangle, one along
|
||
each axis.
|
||
|
||
See also:
|
||
- :func:`jax.numpy.tril_indices`: Returns the indices of lower triangle of an
|
||
array of size ``(n, m)``.
|
||
- :func:`jax.numpy.triu_indices_from`: Returns the indices of upper triangle
|
||
of a given array.
|
||
- :func:`jax.numpy.tril_indices_from`: Returns the indices of lower triangle
|
||
of a given array.
|
||
|
||
Examples:
|
||
If only ``n`` is provided in input, the indices of upper triangle of an array
|
||
of size ``(n, n)`` array are returned.
|
||
|
||
>>> jnp.triu_indices(3)
|
||
(Array([0, 0, 0, 1, 1, 2], dtype=int32), Array([0, 1, 2, 1, 2, 2], dtype=int32))
|
||
|
||
If both ``n`` and ``m`` are provided in input, the indices of upper triangle
|
||
of an ``(n, m)`` array are returned.
|
||
|
||
>>> jnp.triu_indices(3, m=2)
|
||
(Array([0, 0, 1], dtype=int32), Array([0, 1, 1], dtype=int32))
|
||
|
||
If ``k = 1``, the indices on and above the first sub-diagonal above the main
|
||
diagonal are returned.
|
||
|
||
>>> jnp.triu_indices(3, k=1)
|
||
(Array([0, 0, 1], dtype=int32), Array([1, 2, 2], dtype=int32))
|
||
|
||
If ``k = -1``, the indices on and above the first sub-diagonal below the main
|
||
diagonal are returned.
|
||
|
||
>>> jnp.triu_indices(3, k=-1)
|
||
(Array([0, 0, 0, 1, 1, 1, 2, 2], dtype=int32), Array([0, 1, 2, 0, 1, 2, 1, 2], dtype=int32))
|
||
"""
|
||
n = core.concrete_or_error(operator.index, n, "n argument of jnp.triu_indices")
|
||
k = core.concrete_or_error(operator.index, k, "k argument of jnp.triu_indices")
|
||
m = n if m is None else core.concrete_or_error(operator.index, m, "m argument of jnp.triu_indices")
|
||
i, j = nonzero(triu(ones((n, m)), k=k), size=_triu_size(n, m, k))
|
||
return i, j
|
||
|
||
|
||
@export
|
||
def tril_indices(n: int, k: int = 0, m: int | None = None) -> tuple[Array, Array]:
|
||
"""Return the indices of lower triangle of an array of size ``(n, m)``.
|
||
|
||
JAX implementation of :func:`numpy.tril_indices`.
|
||
|
||
Args:
|
||
n: int. Number of rows of the array for which the indices are returned.
|
||
k: optional, int, default=0. Specifies the sub-diagonal on and below which
|
||
the indices of lower triangle are returned. ``k=0`` refers to main diagonal,
|
||
``k<0`` refers to sub-diagonal below the main diagonal and ``k>0`` refers
|
||
to sub-diagonal above the main diagonal.
|
||
m: optional, int. Number of columns of the array for which the indices are
|
||
returned. If not specified, then ``m = n``.
|
||
|
||
Returns:
|
||
A tuple of two arrays containing the indices of the lower triangle, one along
|
||
each axis.
|
||
|
||
See also:
|
||
- :func:`jax.numpy.triu_indices`: Returns the indices of upper triangle of an
|
||
array of size ``(n, m)``.
|
||
- :func:`jax.numpy.triu_indices_from`: Returns the indices of upper triangle
|
||
of a given array.
|
||
- :func:`jax.numpy.tril_indices_from`: Returns the indices of lower triangle
|
||
of a given array.
|
||
|
||
Examples:
|
||
If only ``n`` is provided in input, the indices of lower triangle of an array
|
||
of size ``(n, n)`` array are returned.
|
||
|
||
>>> jnp.tril_indices(3)
|
||
(Array([0, 1, 1, 2, 2, 2], dtype=int32), Array([0, 0, 1, 0, 1, 2], dtype=int32))
|
||
|
||
If both ``n`` and ``m`` are provided in input, the indices of lower triangle
|
||
of an ``(n, m)`` array are returned.
|
||
|
||
>>> jnp.tril_indices(3, m=2)
|
||
(Array([0, 1, 1, 2, 2], dtype=int32), Array([0, 0, 1, 0, 1], dtype=int32))
|
||
|
||
If ``k = 1``, the indices on and below the first sub-diagonal above the main
|
||
diagonal are returned.
|
||
|
||
>>> jnp.tril_indices(3, k=1)
|
||
(Array([0, 0, 1, 1, 1, 2, 2, 2], dtype=int32), Array([0, 1, 0, 1, 2, 0, 1, 2], dtype=int32))
|
||
|
||
If ``k = -1``, the indices on and below the first sub-diagonal below the main
|
||
diagonal are returned.
|
||
|
||
>>> jnp.tril_indices(3, k=-1)
|
||
(Array([1, 2, 2], dtype=int32), Array([0, 0, 1], dtype=int32))
|
||
"""
|
||
n = core.concrete_or_error(operator.index, n, "n argument of jnp.triu_indices")
|
||
k = core.concrete_or_error(operator.index, k, "k argument of jnp.triu_indices")
|
||
m = n if m is None else core.concrete_or_error(operator.index, m, "m argument of jnp.triu_indices")
|
||
i, j = nonzero(tril(ones((n, m)), k=k), size=_triu_size(m, n, -k))
|
||
return i, j
|
||
|
||
|
||
@export
|
||
def triu_indices_from(arr: ArrayLike, k: int = 0) -> tuple[Array, Array]:
|
||
"""Return the indices of upper triangle of a given array.
|
||
|
||
JAX implementation of :func:`numpy.triu_indices_from`.
|
||
|
||
Args:
|
||
arr: input array. Must have ``arr.ndim == 2``.
|
||
k: optional, int, default=0. Specifies the sub-diagonal on and above which
|
||
the indices of upper triangle are returned. ``k=0`` refers to main diagonal,
|
||
``k<0`` refers to sub-diagonal below the main diagonal and ``k>0`` refers
|
||
to sub-diagonal above the main diagonal.
|
||
|
||
Returns:
|
||
A tuple of two arrays containing the indices of the upper triangle, one along
|
||
each axis.
|
||
|
||
See also:
|
||
- :func:`jax.numpy.tril_indices_from`: Returns the indices of lower triangle
|
||
of a given array.
|
||
- :func:`jax.numpy.triu_indices`: Returns the indices of upper triangle of an
|
||
array of size ``(n, m)``.
|
||
- :func:`jax.numpy.triu`: Return an upper triangle of an array.
|
||
|
||
Examples:
|
||
>>> arr = jnp.array([[1, 2, 3],
|
||
... [4, 5, 6],
|
||
... [7, 8, 9]])
|
||
>>> jnp.triu_indices_from(arr)
|
||
(Array([0, 0, 0, 1, 1, 2], dtype=int32), Array([0, 1, 2, 1, 2, 2], dtype=int32))
|
||
|
||
Elements indexed by ``jnp.triu_indices_from`` correspond to those in the
|
||
output of ``jnp.triu``.
|
||
|
||
>>> ind = jnp.triu_indices_from(arr)
|
||
>>> arr[ind]
|
||
Array([1, 2, 3, 5, 6, 9], dtype=int32)
|
||
>>> jnp.triu(arr)
|
||
Array([[1, 2, 3],
|
||
[0, 5, 6],
|
||
[0, 0, 9]], dtype=int32)
|
||
|
||
When ``k > 0``:
|
||
|
||
>>> jnp.triu_indices_from(arr, k=1)
|
||
(Array([0, 0, 1], dtype=int32), Array([1, 2, 2], dtype=int32))
|
||
|
||
When ``k < 0``:
|
||
|
||
>>> jnp.triu_indices_from(arr, k=-1)
|
||
(Array([0, 0, 0, 1, 1, 1, 2, 2], dtype=int32), Array([0, 1, 2, 0, 1, 2, 1, 2], dtype=int32))
|
||
"""
|
||
arr_shape = np.shape(arr)
|
||
if len(arr_shape) != 2:
|
||
raise ValueError("Only 2-D inputs are accepted")
|
||
return triu_indices(arr_shape[0], k=k, m=arr_shape[1])
|
||
|
||
|
||
@export
|
||
def tril_indices_from(arr: ArrayLike, k: int = 0) -> tuple[Array, Array]:
|
||
"""Return the indices of lower triangle of a given array.
|
||
|
||
JAX implementation of :func:`numpy.tril_indices_from`.
|
||
|
||
Args:
|
||
arr: input array. Must have ``arr.ndim == 2``.
|
||
k: optional, int, default=0. Specifies the sub-diagonal on and below which
|
||
the indices of upper triangle are returned. ``k=0`` refers to main diagonal,
|
||
``k<0`` refers to sub-diagonal below the main diagonal and ``k>0`` refers
|
||
to sub-diagonal above the main diagonal.
|
||
|
||
Returns:
|
||
A tuple of two arrays containing the indices of the lower triangle, one along
|
||
each axis.
|
||
|
||
See also:
|
||
- :func:`jax.numpy.triu_indices_from`: Returns the indices of upper triangle
|
||
of a given array.
|
||
- :func:`jax.numpy.tril_indices`: Returns the indices of lower triangle of an
|
||
array of size ``(n, m)``.
|
||
- :func:`jax.numpy.tril`: Returns a lower triangle of an array
|
||
|
||
Examples:
|
||
>>> arr = jnp.array([[1, 2, 3],
|
||
... [4, 5, 6],
|
||
... [7, 8, 9]])
|
||
>>> jnp.tril_indices_from(arr)
|
||
(Array([0, 1, 1, 2, 2, 2], dtype=int32), Array([0, 0, 1, 0, 1, 2], dtype=int32))
|
||
|
||
Elements indexed by ``jnp.tril_indices_from`` correspond to those in the
|
||
output of ``jnp.tril``.
|
||
|
||
>>> ind = jnp.tril_indices_from(arr)
|
||
>>> arr[ind]
|
||
Array([1, 4, 5, 7, 8, 9], dtype=int32)
|
||
>>> jnp.tril(arr)
|
||
Array([[1, 0, 0],
|
||
[4, 5, 0],
|
||
[7, 8, 9]], dtype=int32)
|
||
|
||
When ``k > 0``:
|
||
|
||
>>> jnp.tril_indices_from(arr, k=1)
|
||
(Array([0, 0, 1, 1, 1, 2, 2, 2], dtype=int32), Array([0, 1, 0, 1, 2, 0, 1, 2], dtype=int32))
|
||
|
||
When ``k < 0``:
|
||
|
||
>>> jnp.tril_indices_from(arr, k=-1)
|
||
(Array([1, 2, 2], dtype=int32), Array([0, 0, 1], dtype=int32))
|
||
"""
|
||
arr_shape = np.shape(arr)
|
||
if len(arr_shape) != 2:
|
||
raise ValueError("Only 2-D inputs are accepted")
|
||
return tril_indices(arr_shape[0], k=k, m=arr_shape[1])
|
||
|
||
|
||
@export
|
||
def fill_diagonal(a: ArrayLike, val: ArrayLike, wrap: bool = False, *,
|
||
inplace: bool = True) -> Array:
|
||
"""Return a copy of the array with the diagonal overwritten.
|
||
|
||
JAX implementation of :func:`numpy.fill_diagonal`.
|
||
|
||
The semantics of :func:`numpy.fill_diagonal` are to modify arrays in-place, which
|
||
is not possible for JAX's immutable arrays. The JAX version returns a modified
|
||
copy of the input, and adds the ``inplace`` parameter which must be set to
|
||
`False`` by the user as a reminder of this API difference.
|
||
|
||
Args:
|
||
a: input array. Must have ``a.ndim >= 2``. If ``a.ndim >= 3``, then all
|
||
dimensions must be the same size.
|
||
val: scalar or array with which to fill the diagonal. If an array, it will
|
||
be flattened and repeated to fill the diagonal entries.
|
||
inplace: must be set to False to indicate that the input is not modified
|
||
in-place, but rather a modified copy is returned.
|
||
|
||
Returns:
|
||
A copy of ``a`` with the diagonal set to ``val``.
|
||
|
||
Examples:
|
||
>>> x = jnp.zeros((3, 3), dtype=int)
|
||
>>> jnp.fill_diagonal(x, jnp.array([1, 2, 3]), inplace=False)
|
||
Array([[1, 0, 0],
|
||
[0, 2, 0],
|
||
[0, 0, 3]], dtype=int32)
|
||
|
||
Unlike :func:`numpy.fill_diagonal`, the input ``x`` is not modified.
|
||
|
||
If the diagonal value has too many entries, it will be truncated
|
||
|
||
>>> jnp.fill_diagonal(x, jnp.arange(100, 200), inplace=False)
|
||
Array([[100, 0, 0],
|
||
[ 0, 101, 0],
|
||
[ 0, 0, 102]], dtype=int32)
|
||
|
||
If the diagonal has too few entries, it will be repeated:
|
||
|
||
>>> x = jnp.zeros((4, 4), dtype=int)
|
||
>>> jnp.fill_diagonal(x, jnp.array([3, 4]), inplace=False)
|
||
Array([[3, 0, 0, 0],
|
||
[0, 4, 0, 0],
|
||
[0, 0, 3, 0],
|
||
[0, 0, 0, 4]], dtype=int32)
|
||
|
||
For non-square arrays, the diagonal of the leading square slice is filled:
|
||
|
||
>>> x = jnp.zeros((3, 5), dtype=int)
|
||
>>> jnp.fill_diagonal(x, 1, inplace=False)
|
||
Array([[1, 0, 0, 0, 0],
|
||
[0, 1, 0, 0, 0],
|
||
[0, 0, 1, 0, 0]], dtype=int32)
|
||
|
||
And for square N-dimensional arrays, the N-dimensional diagonal is filled:
|
||
|
||
>>> y = jnp.zeros((2, 2, 2))
|
||
>>> jnp.fill_diagonal(y, 1, inplace=False)
|
||
Array([[[1., 0.],
|
||
[0., 0.]],
|
||
<BLANKLINE>
|
||
[[0., 0.],
|
||
[0., 1.]]], dtype=float32)
|
||
"""
|
||
if inplace:
|
||
raise NotImplementedError("JAX arrays are immutable, must use inplace=False")
|
||
if wrap:
|
||
raise NotImplementedError("wrap=True is not implemented, must use wrap=False")
|
||
a, val = util.ensure_arraylike("fill_diagonal", a, val)
|
||
if a.ndim < 2:
|
||
raise ValueError("array must be at least 2-d")
|
||
if a.ndim > 2 and not all(n == a.shape[0] for n in a.shape[1:]):
|
||
raise ValueError("All dimensions of input must be of equal length")
|
||
n = min(a.shape)
|
||
idx = diag_indices(n, a.ndim)
|
||
return a.at[idx].set(val if val.ndim == 0 else _tile_to_size(val.ravel(), n))
|
||
|
||
|
||
@export
|
||
def diag_indices(n: int, ndim: int = 2) -> tuple[Array, ...]:
|
||
"""Return indices for accessing the main diagonal of a multidimensional array.
|
||
|
||
JAX implementation of :func:`numpy.diag_indices`.
|
||
|
||
Args:
|
||
n: int. The size of each dimension of the square array.
|
||
ndim: optional, int, default=2. The number of dimensions of the array.
|
||
|
||
Returns:
|
||
A tuple of arrays, each of length `n`, containing the indices to access
|
||
the main diagonal.
|
||
|
||
See also:
|
||
- :func:`jax.numpy.diag_indices_from`
|
||
- :func:`jax.numpy.diagonal`
|
||
|
||
Examples:
|
||
>>> jnp.diag_indices(3)
|
||
(Array([0, 1, 2], dtype=int32), Array([0, 1, 2], dtype=int32))
|
||
>>> jnp.diag_indices(4, ndim=3)
|
||
(Array([0, 1, 2, 3], dtype=int32),
|
||
Array([0, 1, 2, 3], dtype=int32),
|
||
Array([0, 1, 2, 3], dtype=int32))
|
||
"""
|
||
n = core.concrete_or_error(operator.index, n, "'n' argument of jnp.diag_indices()")
|
||
ndim = core.concrete_or_error(operator.index, ndim, "'ndim' argument of jnp.diag_indices()")
|
||
if n < 0:
|
||
raise ValueError("n argument to diag_indices must be nonnegative, got {}"
|
||
.format(n))
|
||
if ndim < 0:
|
||
raise ValueError("ndim argument to diag_indices must be nonnegative, got {}"
|
||
.format(ndim))
|
||
return (lax.iota(dtypes.int_, n),) * ndim
|
||
|
||
|
||
@export
|
||
def diag_indices_from(arr: ArrayLike) -> tuple[Array, ...]:
|
||
"""Return indices for accessing the main diagonal of a given array.
|
||
|
||
JAX implementation of :func:`numpy.diag_indices_from`.
|
||
|
||
Args:
|
||
arr: Input array. Must be at least 2-dimensional and have equal length along
|
||
all dimensions.
|
||
|
||
Returns:
|
||
A tuple of arrays containing the indices to access the main diagonal of
|
||
the input array.
|
||
|
||
See also:
|
||
- :func:`jax.numpy.diag_indices`
|
||
- :func:`jax.numpy.diagonal`
|
||
|
||
Examples:
|
||
>>> arr = jnp.array([[1, 2, 3],
|
||
... [4, 5, 6],
|
||
... [7, 8, 9]])
|
||
>>> jnp.diag_indices_from(arr)
|
||
(Array([0, 1, 2], dtype=int32), Array([0, 1, 2], dtype=int32))
|
||
>>> arr = jnp.array([[[1, 2], [3, 4]],
|
||
... [[5, 6], [7, 8]]])
|
||
>>> jnp.diag_indices_from(arr)
|
||
(Array([0, 1], dtype=int32),
|
||
Array([0, 1], dtype=int32),
|
||
Array([0, 1], dtype=int32))
|
||
"""
|
||
util.check_arraylike("diag_indices_from", arr)
|
||
nd = np.ndim(arr)
|
||
if not np.ndim(arr) >= 2:
|
||
raise ValueError("input array must be at least 2-d")
|
||
|
||
s = np.shape(arr)
|
||
if len(set(np.shape(arr))) != 1:
|
||
raise ValueError("All dimensions of input must be of equal length")
|
||
|
||
return diag_indices(s[0], ndim=nd)
|
||
|
||
|
||
@export
|
||
@partial(jit, static_argnames=('offset', 'axis1', 'axis2'))
|
||
def diagonal(a: ArrayLike, offset: int = 0, axis1: int = 0,
|
||
axis2: int = 1) -> Array:
|
||
"""Returns the specified diagonal of an array.
|
||
|
||
JAX implementation of :func:`numpy.diagonal`.
|
||
|
||
The JAX version always returns a copy of the input, although if this is used
|
||
within a JIT compilation, the compiler may avoid the copy.
|
||
|
||
Args:
|
||
a: Input array. Must be at least 2-dimensional.
|
||
offset: optional, default=0. Diagonal offset from the main diagonal.
|
||
Must be a static integer value. Can be positive or negative.
|
||
axis1: optional, default=0. The first axis along which to take the diagonal.
|
||
axis2: optional, default=1. The second axis along which to take the diagonal.
|
||
|
||
Returns:
|
||
A 1D array for 2D input, and in general a N-1 dimensional array
|
||
for N-dimensional input.
|
||
|
||
See also:
|
||
- :func:`jax.numpy.diag`
|
||
- :func:`jax.numpy.diagflat`
|
||
|
||
Examples:
|
||
>>> x = jnp.array([[1, 2, 3],
|
||
... [4, 5, 6],
|
||
... [7, 8, 9]])
|
||
>>> jnp.diagonal(x)
|
||
Array([1, 5, 9], dtype=int32)
|
||
>>> jnp.diagonal(x, offset=1)
|
||
Array([2, 6], dtype=int32)
|
||
>>> jnp.diagonal(x, offset=-1)
|
||
Array([4, 8], dtype=int32)
|
||
"""
|
||
util.check_arraylike("diagonal", a)
|
||
|
||
if np.ndim(a) < 2:
|
||
raise ValueError("diagonal requires an array of at least two dimensions.")
|
||
offset = core.concrete_or_error(operator.index, offset, "'offset' argument of jnp.diagonal()")
|
||
|
||
def _default_diag(a):
|
||
a_shape = np.shape(a)
|
||
|
||
a = moveaxis(a, (axis1, axis2), (-2, -1))
|
||
|
||
diag_size = max(
|
||
0, min(a_shape[axis1] + min(offset, 0), a_shape[axis2] - max(offset, 0))
|
||
)
|
||
i = arange(diag_size)
|
||
j = arange(abs(offset), abs(offset) + diag_size)
|
||
return a[..., i, j] if offset >= 0 else a[..., j, i]
|
||
|
||
|
||
# The mosaic lowering rule for diag is only defined for square arrays.
|
||
# TODO(mvoz): Add support for offsets.
|
||
if np.shape(a)[0] != np.shape(a)[1] or np.ndim(a) != 2 or offset != 0 or _dtype(a) == bool:
|
||
return _default_diag(a)
|
||
else:
|
||
a_shape_eye = eye(np.shape(a)[0], dtype=_dtype(a))
|
||
|
||
def _mosaic_diag(a):
|
||
def _sum(x, axis):
|
||
return lax.reduce(
|
||
x,
|
||
np.array(0, _dtype(x)),
|
||
lax.add if _dtype(x) != bool else lax.bitwise_or,
|
||
(axis,),
|
||
)
|
||
return _sum(lax.mul(a_shape_eye, a), axis=0)
|
||
return lax.platform_dependent(a, default=_default_diag, mosaic=_mosaic_diag)
|
||
|
||
|
||
@export
|
||
def diag(v: ArrayLike, k: int = 0) -> Array:
|
||
"""Returns the specified diagonal or constructs a diagonal array.
|
||
|
||
JAX implementation of :func:`numpy.diag`.
|
||
|
||
The JAX version always returns a copy of the input, although if this is used
|
||
within a JIT compilation, the compiler may avoid the copy.
|
||
|
||
Args:
|
||
v: Input array. Can be a 1-D array to create a diagonal matrix or a
|
||
2-D array to extract a diagonal.
|
||
k: optional, default=0. Diagonal offset. Positive values place the diagonal
|
||
above the main diagonal, negative values place it below the main diagonal.
|
||
|
||
Returns:
|
||
If `v` is a 2-D array, a 1-D array containing the diagonal elements.
|
||
If `v` is a 1-D array, a 2-D array with the input elements placed along the
|
||
specified diagonal.
|
||
|
||
See also:
|
||
- :func:`jax.numpy.diagflat`
|
||
- :func:`jax.numpy.diagonal`
|
||
|
||
Examples:
|
||
Creating a diagonal matrix from a 1-D array:
|
||
|
||
>>> jnp.diag(jnp.array([1, 2, 3]))
|
||
Array([[1, 0, 0],
|
||
[0, 2, 0],
|
||
[0, 0, 3]], dtype=int32)
|
||
|
||
Specifying a diagonal offset:
|
||
|
||
>>> jnp.diag(jnp.array([1, 2, 3]), k=1)
|
||
Array([[0, 1, 0, 0],
|
||
[0, 0, 2, 0],
|
||
[0, 0, 0, 3],
|
||
[0, 0, 0, 0]], dtype=int32)
|
||
|
||
Extracting a diagonal from a 2-D array:
|
||
|
||
>>> x = jnp.array([[1, 2, 3],
|
||
... [4, 5, 6],
|
||
... [7, 8, 9]])
|
||
>>> jnp.diag(x)
|
||
Array([1, 5, 9], dtype=int32)
|
||
"""
|
||
return _diag(v, operator.index(k))
|
||
|
||
@partial(jit, static_argnames=('k',))
|
||
def _diag(v, k):
|
||
util.check_arraylike("diag", v)
|
||
v_shape = np.shape(v)
|
||
if len(v_shape) == 1:
|
||
zero = lambda x: lax.full_like(x, shape=(), fill_value=0)
|
||
n = v_shape[0] + abs(k)
|
||
v = lax.pad(v, zero(v), ((max(0, k), max(0, -k), 0),))
|
||
return where(eye(n, k=k, dtype=bool), v, zeros_like(v))
|
||
elif len(v_shape) == 2:
|
||
return diagonal(v, offset=k)
|
||
else:
|
||
raise ValueError("diag input must be 1d or 2d")
|
||
|
||
|
||
@export
|
||
def diagflat(v: ArrayLike, k: int = 0) -> Array:
|
||
"""Return a 2-D array with the flattened input array laid out on the diagonal.
|
||
|
||
JAX implementation of :func:`numpy.diagflat`.
|
||
|
||
This differs from `np.diagflat` for some scalar values of `v`. JAX always returns
|
||
a two-dimensional array, whereas NumPy may return a scalar depending on the type
|
||
of `v`.
|
||
|
||
Args:
|
||
v: Input array. Can be N-dimensional but is flattened to 1D.
|
||
k: optional, default=0. Diagonal offset. Positive values place the diagonal
|
||
above the main diagonal, negative values place it below the main diagonal.
|
||
|
||
Returns:
|
||
A 2D array with the input elements placed along the diagonal with the
|
||
specified offset (k). The remaining entries are filled with zeros.
|
||
|
||
See also:
|
||
- :func:`jax.numpy.diag`
|
||
- :func:`jax.numpy.diagonal`
|
||
|
||
Examples:
|
||
>>> jnp.diagflat(jnp.array([1, 2, 3]))
|
||
Array([[1, 0, 0],
|
||
[0, 2, 0],
|
||
[0, 0, 3]], dtype=int32)
|
||
>>> jnp.diagflat(jnp.array([1, 2, 3]), k=1)
|
||
Array([[0, 1, 0, 0],
|
||
[0, 0, 2, 0],
|
||
[0, 0, 0, 3],
|
||
[0, 0, 0, 0]], dtype=int32)
|
||
>>> a = jnp.array([[1, 2],
|
||
... [3, 4]])
|
||
>>> jnp.diagflat(a)
|
||
Array([[1, 0, 0, 0],
|
||
[0, 2, 0, 0],
|
||
[0, 0, 3, 0],
|
||
[0, 0, 0, 4]], dtype=int32)
|
||
"""
|
||
util.check_arraylike("diagflat", v)
|
||
v_ravel = ravel(v)
|
||
v_length = len(v_ravel)
|
||
adj_length = v_length + abs(k)
|
||
res = zeros(adj_length*adj_length, dtype=v_ravel.dtype)
|
||
i = arange(0, adj_length-abs(k))
|
||
if (k >= 0):
|
||
fi = i+k+i*adj_length
|
||
else:
|
||
fi = i+(i-k)*adj_length
|
||
res = res.at[fi].set(v_ravel)
|
||
res = res.reshape(adj_length, adj_length)
|
||
return res
|
||
|
||
|
||
# TODO(jakevdp): add support for N-dimensional inputs as in NumPy v2.2
|
||
@export
|
||
def trim_zeros(filt: ArrayLike, trim: str ='fb') -> Array:
|
||
"""Trim leading and/or trailing zeros of the input array.
|
||
|
||
JAX implementation of :func:`numpy.trim_zeros`.
|
||
|
||
Args:
|
||
filt: input array. Must have ``filt.ndim == 1``.
|
||
trim: string, optional, default = ``fb``. Specifies from which end the input
|
||
is trimmed.
|
||
|
||
- ``f`` - trims only the leading zeros.
|
||
- ``b`` - trims only the trailing zeros.
|
||
- ``fb`` - trims both leading and trailing zeros.
|
||
|
||
Returns:
|
||
An array containing the trimmed input with same dtype as ``filt``.
|
||
|
||
Examples:
|
||
>>> x = jnp.array([0, 0, 2, 0, 1, 4, 3, 0, 0, 0])
|
||
>>> jnp.trim_zeros(x)
|
||
Array([2, 0, 1, 4, 3], dtype=int32)
|
||
"""
|
||
# Non-array inputs are deprecated 2024-09-11
|
||
util.check_arraylike("trim_zeros", filt, emit_warning=True)
|
||
core.concrete_or_error(None, filt,
|
||
"Error arose in the `filt` argument of trim_zeros()")
|
||
filt_arr = jax.numpy.asarray(filt)
|
||
del filt
|
||
if filt_arr.ndim != 1:
|
||
# Added on 2024-09-11
|
||
if deprecations.is_accelerated("jax-numpy-trimzeros-not-1d-array"):
|
||
raise TypeError(f"'filt' must be 1-D array, but received {filt_arr.ndim}-D array.")
|
||
warnings.warn(
|
||
"Passing arrays with ndim != 1 to jnp.trim_zeros() is deprecated. Currently, it "
|
||
"works with Arrays having ndim != 1. In the future this will result in an error.",
|
||
DeprecationWarning, stacklevel=2)
|
||
nz = (filt_arr == 0)
|
||
if reductions.all(nz):
|
||
return empty(0, filt_arr.dtype)
|
||
start: Array | int = argmin(nz) if 'f' in trim.lower() else 0
|
||
end: Array | int = argmin(nz[::-1]) if 'b' in trim.lower() else 0
|
||
return filt_arr[start:len(filt_arr) - end]
|
||
|
||
|
||
def trim_zeros_tol(filt, tol, trim='fb'):
|
||
filt = core.concrete_or_error(asarray, filt,
|
||
"Error arose in the `filt` argument of trim_zeros_tol()")
|
||
nz = (ufuncs.abs(filt) < tol)
|
||
if reductions.all(nz):
|
||
return empty(0, _dtype(filt))
|
||
start = argmin(nz) if 'f' in trim.lower() else 0
|
||
end = argmin(nz[::-1]) if 'b' in trim.lower() else 0
|
||
return filt[start:len(filt) - end]
|
||
|
||
|
||
@export
|
||
@partial(jit, static_argnames=('axis',))
|
||
def append(
|
||
arr: ArrayLike, values: ArrayLike, axis: int | None = None
|
||
) -> Array:
|
||
"""Return a new array with values appended to the end of the original array.
|
||
|
||
JAX implementation of :func:`numpy.append`.
|
||
|
||
Args:
|
||
arr: original array.
|
||
values: values to be appended to the array. The ``values`` must have
|
||
the same number of dimensions as ``arr``, and all dimensions must
|
||
match except in the specified axis.
|
||
axis: axis along which to append values. If None (default), both ``arr``
|
||
and ``values`` will be flattened before appending.
|
||
|
||
Returns:
|
||
A new array with values appended to ``arr``.
|
||
|
||
See also:
|
||
- :func:`jax.numpy.insert`
|
||
- :func:`jax.numpy.delete`
|
||
|
||
Examples:
|
||
>>> a = jnp.array([1, 2, 3])
|
||
>>> b = jnp.array([4, 5, 6])
|
||
>>> jnp.append(a, b)
|
||
Array([1, 2, 3, 4, 5, 6], dtype=int32)
|
||
|
||
Appending along a specific axis:
|
||
|
||
>>> a = jnp.array([[1, 2],
|
||
... [3, 4]])
|
||
>>> b = jnp.array([[5, 6]])
|
||
>>> jnp.append(a, b, axis=0)
|
||
Array([[1, 2],
|
||
[3, 4],
|
||
[5, 6]], dtype=int32)
|
||
|
||
Appending along a trailing axis:
|
||
|
||
>>> a = jnp.array([[1, 2, 3],
|
||
... [4, 5, 6]])
|
||
>>> b = jnp.array([[7], [8]])
|
||
>>> jnp.append(a, b, axis=1)
|
||
Array([[1, 2, 3, 7],
|
||
[4, 5, 6, 8]], dtype=int32)
|
||
"""
|
||
if axis is None:
|
||
return concatenate([ravel(arr), ravel(values)], 0)
|
||
else:
|
||
return concatenate([arr, values], axis=axis)
|
||
|
||
|
||
@export
|
||
def delete(
|
||
arr: ArrayLike,
|
||
obj: ArrayLike | slice,
|
||
axis: int | None = None,
|
||
*,
|
||
assume_unique_indices: bool = False,
|
||
) -> Array:
|
||
"""Delete entry or entries from an array.
|
||
|
||
JAX implementation of :func:`numpy.delete`.
|
||
|
||
Args:
|
||
arr: array from which entries will be deleted.
|
||
obj: index, indices, or slice to be deleted.
|
||
axis: axis along which entries will be deleted.
|
||
assume_unique_indices: In case of array-like integer (not boolean) indices,
|
||
assume the indices are unique, and perform the deletion in a way that is
|
||
compatible with JIT and other JAX transformations.
|
||
|
||
Returns:
|
||
Copy of ``arr`` with specified indices deleted.
|
||
|
||
Note:
|
||
``delete()`` usually requires the index specification to be static. If the
|
||
index is an integer array that is guaranteed to contain unique entries, you
|
||
may specify ``assume_unique_indices=True`` to perform the operation in a
|
||
manner that does not require static indices.
|
||
|
||
See also:
|
||
- :func:`jax.numpy.insert`: insert entries into an array.
|
||
|
||
Examples:
|
||
Delete entries from a 1D array:
|
||
|
||
>>> a = jnp.array([4, 5, 6, 7, 8, 9])
|
||
>>> jnp.delete(a, 2)
|
||
Array([4, 5, 7, 8, 9], dtype=int32)
|
||
>>> jnp.delete(a, slice(1, 4)) # delete a[1:4]
|
||
Array([4, 8, 9], dtype=int32)
|
||
>>> jnp.delete(a, slice(None, None, 2)) # delete a[::2]
|
||
Array([5, 7, 9], dtype=int32)
|
||
|
||
Delete entries from a 2D array along a specified axis:
|
||
|
||
>>> a2 = jnp.array([[4, 5, 6],
|
||
... [7, 8, 9]])
|
||
>>> jnp.delete(a2, 1, axis=1)
|
||
Array([[4, 6],
|
||
[7, 9]], dtype=int32)
|
||
|
||
Delete multiple entries via a sequence of indices:
|
||
|
||
>>> indices = jnp.array([0, 1, 3])
|
||
>>> jnp.delete(a, indices)
|
||
Array([6, 8, 9], dtype=int32)
|
||
|
||
This will fail under :func:`~jax.jit` and other transformations, because
|
||
the output shape cannot be known with the possibility of duplicate indices:
|
||
|
||
>>> jax.jit(jnp.delete)(a, indices) # doctest: +IGNORE_EXCEPTION_DETAIL
|
||
Traceback (most recent call last):
|
||
...
|
||
ConcretizationTypeError: Abstract tracer value encountered where concrete value is expected: traced array with shape int32[3].
|
||
|
||
If you can ensure that the indices are unique, pass ``assume_unique_indices``
|
||
to allow this to be executed under JIT:
|
||
|
||
>>> jit_delete = jax.jit(jnp.delete, static_argnames=['assume_unique_indices'])
|
||
>>> jit_delete(a, indices, assume_unique_indices=True)
|
||
Array([6, 8, 9], dtype=int32)
|
||
"""
|
||
a = util.ensure_arraylike("delete", arr)
|
||
if axis is None:
|
||
a = a.ravel()
|
||
axis = 0
|
||
axis = _canonicalize_axis(axis, a.ndim)
|
||
|
||
# Case 1: obj is a static integer.
|
||
try:
|
||
obj = operator.index(obj) # type: ignore[arg-type]
|
||
obj = _canonicalize_axis(obj, a.shape[axis])
|
||
except TypeError:
|
||
pass
|
||
else:
|
||
idx = tuple(slice(None) for i in range(axis))
|
||
return concatenate([a[idx + (slice(0, obj),)], a[idx + (slice(obj + 1, None),)]], axis=axis)
|
||
|
||
# Case 2: obj is a static slice.
|
||
if isinstance(obj, slice):
|
||
obj = arange(a.shape[axis])[obj]
|
||
assume_unique_indices = True
|
||
|
||
# Case 3: obj is an array
|
||
# NB: pass both arrays to check for appropriate error message.
|
||
util.check_arraylike("delete", a, obj)
|
||
|
||
# Case 3a: unique integer indices; delete in a JIT-compatible way
|
||
if issubdtype(_dtype(obj), np.integer) and assume_unique_indices:
|
||
obj = asarray(obj).ravel()
|
||
obj = clip(where(obj < 0, obj + a.shape[axis], obj), 0, a.shape[axis])
|
||
obj = sort(obj)
|
||
obj -= arange(len(obj)) # type: ignore[arg-type,operator]
|
||
i = arange(a.shape[axis] - obj.size)
|
||
i += (i[None, :] >= obj[:, None]).sum(0)
|
||
return a[(slice(None),) * axis + (i,)]
|
||
|
||
# Case 3b: non-unique indices: must be static.
|
||
obj_array = core.concrete_or_error(np.asarray, obj, "'obj' array argument of jnp.delete()")
|
||
if issubdtype(obj_array.dtype, np.integer):
|
||
# TODO(jakevdp): in theory this could be done dynamically if obj has no duplicates,
|
||
# but this would require the complement of lax.gather.
|
||
mask = np.ones(a.shape[axis], dtype=bool)
|
||
mask[obj_array] = False
|
||
elif obj_array.dtype == bool:
|
||
if obj_array.shape != (a.shape[axis],):
|
||
raise ValueError("np.delete(arr, obj): for boolean indices, obj must be one-dimensional "
|
||
"with length matching specified axis.")
|
||
mask = ~obj_array
|
||
else:
|
||
raise ValueError(f"np.delete(arr, obj): got obj.dtype={obj_array.dtype}; must be integer or bool.")
|
||
return a[tuple(slice(None) for i in range(axis)) + (mask,)]
|
||
|
||
|
||
@export
|
||
def insert(arr: ArrayLike, obj: ArrayLike | slice, values: ArrayLike,
|
||
axis: int | None = None) -> Array:
|
||
"""Insert entries into an array at specified indices.
|
||
|
||
JAX implementation of :func:`numpy.insert`.
|
||
|
||
Args:
|
||
arr: array object into which values will be inserted.
|
||
obj: slice or array of indices specifying insertion locations.
|
||
values: array of values to be inserted.
|
||
axis: specify the insertion axis in the case of multi-dimensional
|
||
arrays. If unspecified, ``arr`` will be flattened.
|
||
|
||
Returns:
|
||
A copy of ``arr`` with values inserted at the specified locations.
|
||
|
||
See also:
|
||
- :func:`jax.numpy.delete`: delete entries from an array.
|
||
|
||
Examples:
|
||
Inserting a single value:
|
||
|
||
>>> x = jnp.arange(5)
|
||
>>> jnp.insert(x, 2, 99)
|
||
Array([ 0, 1, 99, 2, 3, 4], dtype=int32)
|
||
|
||
Inserting multiple identical values using a slice:
|
||
|
||
>>> jnp.insert(x, slice(None, None, 2), -1)
|
||
Array([-1, 0, 1, -1, 2, 3, -1, 4], dtype=int32)
|
||
|
||
Inserting multiple values using an index:
|
||
|
||
>>> indices = jnp.array([4, 2, 5])
|
||
>>> values = jnp.array([10, 11, 12])
|
||
>>> jnp.insert(x, indices, values)
|
||
Array([ 0, 1, 11, 2, 3, 10, 4, 12], dtype=int32)
|
||
|
||
Inserting columns into a 2D array:
|
||
|
||
>>> x = jnp.array([[1, 2, 3],
|
||
... [4, 5, 6]])
|
||
>>> indices = jnp.array([1, 3])
|
||
>>> values = jnp.array([[10, 11],
|
||
... [12, 13]])
|
||
>>> jnp.insert(x, indices, values, axis=1)
|
||
Array([[ 1, 10, 2, 3, 11],
|
||
[ 4, 12, 5, 6, 13]], dtype=int32)
|
||
"""
|
||
a, _, values_arr = util.ensure_arraylike("insert", arr, 0 if isinstance(obj, slice) else obj, values)
|
||
|
||
if axis is None:
|
||
a = ravel(a)
|
||
axis = 0
|
||
axis = core.concrete_or_error(None, axis, "axis argument of jnp.insert()")
|
||
axis = _canonicalize_axis(axis, a.ndim)
|
||
if isinstance(obj, slice):
|
||
indices = arange(*obj.indices(a.shape[axis]))
|
||
else:
|
||
indices = asarray(obj)
|
||
|
||
if indices.ndim > 1:
|
||
raise ValueError("jnp.insert(): obj must be a slice, a one-dimensional "
|
||
f"array, or a scalar; got {obj}")
|
||
if not np.issubdtype(indices.dtype, np.integer):
|
||
if indices.size == 0 and not isinstance(obj, Array):
|
||
indices = indices.astype(int)
|
||
else:
|
||
# Note: np.insert allows boolean inputs but the behavior is deprecated.
|
||
raise ValueError("jnp.insert(): index array must be "
|
||
f"integer typed; got {obj}")
|
||
values_arr = array(values_arr, ndmin=a.ndim, dtype=a.dtype, copy=False)
|
||
|
||
if indices.size == 1:
|
||
index = ravel(indices)[0]
|
||
if indices.ndim == 0:
|
||
values_arr = moveaxis(values_arr, 0, axis)
|
||
indices = full(values_arr.shape[axis], index)
|
||
n_input = a.shape[axis]
|
||
n_insert = broadcast_shapes(indices.shape, (values_arr.shape[axis],))[0]
|
||
out_shape = list(a.shape)
|
||
out_shape[axis] += n_insert
|
||
out = zeros_like(a, shape=tuple(out_shape))
|
||
|
||
indices = where(indices < 0, indices + n_input, indices)
|
||
indices = clip(indices, 0, n_input)
|
||
|
||
values_ind = indices.at[argsort(indices)].add(arange(n_insert, dtype=indices.dtype))
|
||
arr_mask = ones(n_input + n_insert, dtype=bool).at[values_ind].set(False)
|
||
arr_ind = where(arr_mask, size=n_input)[0]
|
||
|
||
out = out.at[(slice(None),) * axis + (values_ind,)].set(values_arr)
|
||
out = out.at[(slice(None),) * axis + (arr_ind,)].set(a)
|
||
|
||
return out
|
||
|
||
|
||
@export
|
||
def apply_along_axis(
|
||
func1d: Callable, axis: int, arr: ArrayLike, *args, **kwargs
|
||
) -> Array:
|
||
"""Apply a function to 1D array slices along an axis.
|
||
|
||
JAX implementation of :func:`numpy.apply_along_axis`. While NumPy implements
|
||
this iteratively, JAX implements this via :func:`jax.vmap`, and so ``func1d``
|
||
must be compatible with ``vmap``.
|
||
|
||
Args:
|
||
func1d: a callable function with signature ``func1d(arr, /, *args, **kwargs)``
|
||
where ``*args`` and ``**kwargs`` are the additional positional and keyword
|
||
arguments passed to :func:`apply_along_axis`.
|
||
axis: integer axis along which to apply the function.
|
||
arr: the array over which to apply the function.
|
||
args, kwargs: additional positional and keyword arguments are passed through
|
||
to ``func1d``.
|
||
|
||
Returns:
|
||
The result of ``func1d`` applied along the specified axis.
|
||
|
||
See also:
|
||
- :func:`jax.vmap`: a more direct way to create a vectorized version of a function.
|
||
- :func:`jax.numpy.apply_over_axes`: repeatedly apply a function over multiple axes.
|
||
- :func:`jax.numpy.vectorize`: create a vectorized version of a function.
|
||
|
||
Examples:
|
||
A simple example in two dimensions, where the function is applied either row-wise
|
||
or column-wise:
|
||
|
||
>>> x = jnp.array([[1, 2, 3],
|
||
... [4, 5, 6]])
|
||
>>> def func1d(x):
|
||
... return jnp.sum(x ** 2)
|
||
>>> jnp.apply_along_axis(func1d, 0, x)
|
||
Array([17, 29, 45], dtype=int32)
|
||
>>> jnp.apply_along_axis(func1d, 1, x)
|
||
Array([14, 77], dtype=int32)
|
||
|
||
For 2D inputs, this can be equivalently expressed using :func:`jax.vmap`,
|
||
though note that `vmap` specifies the mapped axis rather than the applied axis:
|
||
|
||
>>> jax.vmap(func1d, in_axes=1)(x) # same as applying along axis 0
|
||
Array([17, 29, 45], dtype=int32)
|
||
>>> jax.vmap(func1d, in_axes=0)(x) # same as applying along axis 1
|
||
Array([14, 77], dtype=int32)
|
||
|
||
For 3D inputs, :func:`apply_along_axis` is equivalent to mapping over two
|
||
dimensions:
|
||
|
||
>>> x_3d = jnp.arange(24).reshape(2, 3, 4)
|
||
>>> jnp.apply_along_axis(func1d, 2, x_3d)
|
||
Array([[ 14, 126, 366],
|
||
[ 734, 1230, 1854]], dtype=int32)
|
||
>>> jax.vmap(jax.vmap(func1d))(x_3d)
|
||
Array([[ 14, 126, 366],
|
||
[ 734, 1230, 1854]], dtype=int32)
|
||
|
||
The applied function may also take arbitrary positional or keyword arguments,
|
||
which should be passed directly as additional arguments to :func:`apply_along_axis`:
|
||
|
||
>>> def func1d(x, exponent):
|
||
... return jnp.sum(x ** exponent)
|
||
>>> jnp.apply_along_axis(func1d, 0, x, exponent=3)
|
||
Array([ 65, 133, 243], dtype=int32)
|
||
"""
|
||
util.check_arraylike("apply_along_axis", arr)
|
||
num_dims = np.ndim(arr)
|
||
axis = _canonicalize_axis(axis, num_dims)
|
||
func = lambda arr: func1d(arr, *args, **kwargs)
|
||
for i in range(1, num_dims - axis):
|
||
func = jax.vmap(func, in_axes=i, out_axes=-1)
|
||
for i in range(axis):
|
||
func = jax.vmap(func, in_axes=0, out_axes=0)
|
||
return func(arr)
|
||
|
||
|
||
@export
|
||
def apply_over_axes(func: Callable[[ArrayLike, int], Array], a: ArrayLike,
|
||
axes: Sequence[int]) -> Array:
|
||
"""Apply a function repeatedly over specified axes.
|
||
|
||
JAX implementation of :func:`numpy.apply_over_axes`.
|
||
|
||
Args:
|
||
func: the function to apply, with signature ``func(Array, int) -> Array``, and
|
||
where ``y = func(x, axis)`` must satisfy ``y.ndim in [x.ndim, x.ndim - 1]``.
|
||
a: N-dimensional array over which to apply the function.
|
||
axes: the sequence of axes over which to apply the function.
|
||
|
||
Returns:
|
||
An N-dimensional array containing the result of the repeated function application.
|
||
|
||
See also:
|
||
- :func:`jax.numpy.apply_along_axis`: apply a 1D function along a single axis.
|
||
|
||
Examples:
|
||
This function is designed to have similar semantics to typical associative
|
||
:mod:`jax.numpy` reductions over one or more axes with ``keepdims=True``.
|
||
For example:
|
||
|
||
>>> x = jnp.array([[1, 2, 3],
|
||
... [4, 5, 6]])
|
||
|
||
>>> jnp.apply_over_axes(jnp.sum, x, [0])
|
||
Array([[5, 7, 9]], dtype=int32)
|
||
>>> jnp.sum(x, [0], keepdims=True)
|
||
Array([[5, 7, 9]], dtype=int32)
|
||
|
||
>>> jnp.apply_over_axes(jnp.min, x, [1])
|
||
Array([[1],
|
||
[4]], dtype=int32)
|
||
>>> jnp.min(x, [1], keepdims=True)
|
||
Array([[1],
|
||
[4]], dtype=int32)
|
||
|
||
>>> jnp.apply_over_axes(jnp.prod, x, [0, 1])
|
||
Array([[720]], dtype=int32)
|
||
>>> jnp.prod(x, [0, 1], keepdims=True)
|
||
Array([[720]], dtype=int32)
|
||
"""
|
||
a_arr = util.ensure_arraylike("apply_over_axes", a)
|
||
for axis in axes:
|
||
b = func(a_arr, axis)
|
||
if b.ndim == a_arr.ndim:
|
||
a_arr = b
|
||
elif b.ndim == a_arr.ndim - 1:
|
||
a_arr = expand_dims(b, axis)
|
||
else:
|
||
raise ValueError("function is not returning an array of the correct shape")
|
||
return a_arr
|
||
|
||
|
||
@export
|
||
@partial(jit, static_argnames=('axisa', 'axisb', 'axisc', 'axis'))
|
||
def cross(a, b, axisa: int = -1, axisb: int = -1, axisc: int = -1,
|
||
axis: int | None = None):
|
||
r"""Compute the (batched) cross product of two arrays.
|
||
|
||
JAX implementation of :func:`numpy.cross`.
|
||
|
||
This computes the 2-dimensional or 3-dimensional cross product,
|
||
|
||
.. math::
|
||
|
||
c = a \times b
|
||
|
||
In 3 dimensions, ``c`` is a length-3 array. In 2 dimensions, ``c`` is
|
||
a scalar.
|
||
|
||
Args:
|
||
a: N-dimensional array. ``a.shape[axisa]`` indicates the dimension of
|
||
the cross product, and must be 2 or 3.
|
||
b: N-dimensional array. Must have ``b.shape[axisb] == a.shape[axisb]``,
|
||
and other dimensions of ``a`` and ``b`` must be broadcast compatible.
|
||
axisa: specicy the axis of ``a`` along which to compute the cross product.
|
||
axisb: specicy the axis of ``b`` along which to compute the cross product.
|
||
axisc: specicy the axis of ``c`` along which the cross product result
|
||
will be stored.
|
||
axis: if specified, this overrides ``axisa``, ``axisb``, and ``axisc``
|
||
with a single value.
|
||
|
||
Returns:
|
||
The array ``c`` containing the (batched) cross product of ``a`` and ``b``
|
||
along the specified axes.
|
||
|
||
See also:
|
||
- :func:`jax.numpy.linalg.cross`: an array API compatible function for
|
||
computing cross products over 3-vectors.
|
||
|
||
Examples:
|
||
A 2-dimensional cross product returns a scalar:
|
||
|
||
>>> a = jnp.array([1, 2])
|
||
>>> b = jnp.array([3, 4])
|
||
>>> jnp.cross(a, b)
|
||
Array(-2, dtype=int32)
|
||
|
||
A 3-dimensional cross product returns a length-3 vector:
|
||
|
||
>>> a = jnp.array([1, 2, 3])
|
||
>>> b = jnp.array([4, 5, 6])
|
||
>>> jnp.cross(a, b)
|
||
Array([-3, 6, -3], dtype=int32)
|
||
|
||
With multi-dimensional inputs, the cross-product is computed along
|
||
the last axis by default. Here's a batched 3-dimensional cross
|
||
product, operating on the rows of the inputs:
|
||
|
||
>>> a = jnp.array([[1, 2, 3],
|
||
... [3, 4, 3]])
|
||
>>> b = jnp.array([[2, 3, 2],
|
||
... [4, 5, 6]])
|
||
>>> jnp.cross(a, b)
|
||
Array([[-5, 4, -1],
|
||
[ 9, -6, -1]], dtype=int32)
|
||
|
||
Specifying axis=0 makes this a batched 2-dimensional cross product,
|
||
operating on the columns of the inputs:
|
||
|
||
>>> jnp.cross(a, b, axis=0)
|
||
Array([-2, -2, 12], dtype=int32)
|
||
|
||
Equivalently, we can independently specify the axis of the inputs ``a``
|
||
and ``b`` and the output ``c``:
|
||
|
||
>>> jnp.cross(a, b, axisa=0, axisb=0, axisc=0)
|
||
Array([-2, -2, 12], dtype=int32)
|
||
"""
|
||
# TODO(jakevdp): NumPy 2.0 deprecates 2D inputs. Follow suit here.
|
||
util.check_arraylike("cross", a, b)
|
||
if axis is not None:
|
||
axisa = axis
|
||
axisb = axis
|
||
axisc = axis
|
||
a = moveaxis(a, axisa, -1)
|
||
b = moveaxis(b, axisb, -1)
|
||
|
||
if a.shape[-1] not in (2, 3) or b.shape[-1] not in (2, 3):
|
||
raise ValueError("Dimension must be either 2 or 3 for cross product")
|
||
|
||
if a.shape[-1] == 2 and b.shape[-1] == 2:
|
||
return a[..., 0] * b[..., 1] - a[..., 1] * b[..., 0]
|
||
|
||
a0 = a[..., 0]
|
||
a1 = a[..., 1]
|
||
a2 = a[..., 2] if a.shape[-1] == 3 else zeros_like(a0)
|
||
b0 = b[..., 0]
|
||
b1 = b[..., 1]
|
||
b2 = b[..., 2] if b.shape[-1] == 3 else zeros_like(b0)
|
||
c = array([a1 * b2 - a2 * b1, a2 * b0 - a0 * b2, a0 * b1 - a1 * b0])
|
||
return moveaxis(c, 0, axisc)
|
||
|
||
|
||
@export
|
||
@jit
|
||
def kron(a: ArrayLike, b: ArrayLike) -> Array:
|
||
"""Compute the Kronecker product of two input arrays.
|
||
|
||
JAX implementation of :func:`numpy.kron`.
|
||
|
||
The Kronecker product is an operation on two matrices of arbitrary size that
|
||
produces a block matrix. Each element of the first matrix ``a`` is multiplied by
|
||
the entire second matrix ``b``. If ``a`` has shape (m, n) and ``b``
|
||
has shape (p, q), the resulting matrix will have shape (m * p, n * q).
|
||
|
||
Args:
|
||
a: first input array with any shape.
|
||
b: second input array with any shape.
|
||
|
||
Returns:
|
||
A new array representing the Kronecker product of the inputs ``a`` and ``b``.
|
||
The shape of the output is the element-wise product of the input shapes.
|
||
|
||
See also:
|
||
- :func:`jax.numpy.outer`: compute the outer product of two arrays.
|
||
|
||
Examples:
|
||
>>> a = jnp.array([[1, 2],
|
||
... [3, 4]])
|
||
>>> b = jnp.array([[5, 6],
|
||
... [7, 8]])
|
||
>>> jnp.kron(a, b)
|
||
Array([[ 5, 6, 10, 12],
|
||
[ 7, 8, 14, 16],
|
||
[15, 18, 20, 24],
|
||
[21, 24, 28, 32]], dtype=int32)
|
||
"""
|
||
util.check_arraylike("kron", a, b)
|
||
a, b = util.promote_dtypes(a, b)
|
||
if np.ndim(a) < np.ndim(b):
|
||
a = expand_dims(a, range(np.ndim(b) - np.ndim(a)))
|
||
elif np.ndim(b) < np.ndim(a):
|
||
b = expand_dims(b, range(np.ndim(a) - np.ndim(b)))
|
||
a_reshaped = expand_dims(a, range(1, 2 * np.ndim(a), 2))
|
||
b_reshaped = expand_dims(b, range(0, 2 * np.ndim(b), 2))
|
||
out_shape = tuple(np.multiply(np.shape(a), np.shape(b)))
|
||
return reshape(lax.mul(a_reshaped, b_reshaped), out_shape)
|
||
|
||
|
||
@export
|
||
@partial(jit, static_argnames=('N', 'increasing'))
|
||
def vander(
|
||
x: ArrayLike, N: int | None = None, increasing: bool = False
|
||
) -> Array:
|
||
"""Generate a Vandermonde matrix.
|
||
|
||
JAX implementation of :func:`numpy.vander`.
|
||
|
||
Args:
|
||
x: input array. Must have ``x.ndim == 1``.
|
||
N: int, optional, default=None. Specifies the number of the columns the
|
||
output matrix. If not specified, ``N = len(x)``.
|
||
increasing: bool, optional, default=False. Specifies the order of the powers
|
||
of the columns. If ``True``, the powers increase from left to right,
|
||
:math:`[x^0, x^1, ..., x^{(N-1)}]`. By default, the powers decrease from left to
|
||
right :math:`[x^{(N-1)}, ..., x^1, x^0]`.
|
||
|
||
Returns:
|
||
An array of shape ``[len(x), N]`` containing the generated Vandermonde matrix.
|
||
|
||
Examples:
|
||
>>> x = jnp.array([1, 2, 3, 4])
|
||
>>> jnp.vander(x)
|
||
Array([[ 1, 1, 1, 1],
|
||
[ 8, 4, 2, 1],
|
||
[27, 9, 3, 1],
|
||
[64, 16, 4, 1]], dtype=int32)
|
||
|
||
If ``N = 2``, generates a Vandermonde matrix with ``2`` columns.
|
||
|
||
>>> jnp.vander(x, N=2)
|
||
Array([[1, 1],
|
||
[2, 1],
|
||
[3, 1],
|
||
[4, 1]], dtype=int32)
|
||
|
||
Generates the Vandermonde matrix in increaing order of powers, when
|
||
``increasing=True``.
|
||
|
||
>>> jnp.vander(x, increasing=True)
|
||
Array([[ 1, 1, 1, 1],
|
||
[ 1, 2, 4, 8],
|
||
[ 1, 3, 9, 27],
|
||
[ 1, 4, 16, 64]], dtype=int32)
|
||
"""
|
||
x = util.ensure_arraylike("vander", x)
|
||
if x.ndim != 1:
|
||
raise ValueError("x must be a one-dimensional array")
|
||
N = x.shape[0] if N is None else core.concrete_or_error(
|
||
operator.index, N, "'N' argument of jnp.vander()")
|
||
if N < 0:
|
||
raise ValueError("N must be nonnegative")
|
||
|
||
iota = lax.iota(x.dtype, N)
|
||
if not increasing:
|
||
iota = lax.sub(_lax_const(iota, N - 1), iota)
|
||
|
||
return ufuncs.power(x[..., None], expand_dims(iota, tuple(range(x.ndim))))
|
||
|
||
|
||
### Misc
|
||
|
||
@export
|
||
def argwhere(
|
||
a: ArrayLike,
|
||
*,
|
||
size: int | None = None,
|
||
fill_value: ArrayLike | None = None,
|
||
) -> Array:
|
||
"""Find the indices of nonzero array elements
|
||
|
||
JAX implementation of :func:`numpy.argwhere`.
|
||
|
||
``jnp.argwhere(x)`` is essentially equivalent to ``jnp.column_stack(jnp.nonzero(x))``
|
||
with special handling for zero-dimensional (i.e. scalar) inputs.
|
||
|
||
Because the size of the output of ``argwhere`` is data-dependent, the function is not
|
||
typically compatible with JIT. The JAX version adds the optional ``size`` argument, which
|
||
specifies the size of the leading dimension of the output - it must be specified statically
|
||
for ``jnp.argwhere`` to be compiled with non-static operands. See :func:`jax.numpy.nonzero`
|
||
for a full discussion of ``size`` and its semantics.
|
||
|
||
Args:
|
||
a: array for which to find nonzero elements
|
||
size: optional integer specifying statically the number of expected nonzero elements.
|
||
This must be specified in order to use ``argwhere`` within JAX transformations like
|
||
:func:`jax.jit`. See :func:`jax.numpy.nonzero` for more information.
|
||
fill_value: optional array specifying the fill value when ``size`` is specified.
|
||
See :func:`jax.numpy.nonzero` for more information.
|
||
|
||
Returns:
|
||
a two-dimensional array of shape ``[size, x.ndim]``. If ``size`` is not specified as
|
||
an argument, it is equal to the number of nonzero elements in ``x``.
|
||
|
||
See Also:
|
||
- :func:`jax.numpy.where`
|
||
- :func:`jax.numpy.nonzero`
|
||
|
||
Examples:
|
||
Two-dimensional array:
|
||
|
||
>>> x = jnp.array([[1, 0, 2],
|
||
... [0, 3, 0]])
|
||
>>> jnp.argwhere(x)
|
||
Array([[0, 0],
|
||
[0, 2],
|
||
[1, 1]], dtype=int32)
|
||
|
||
Equivalent computation using :func:`jax.numpy.column_stack` and :func:`jax.numpy.nonzero`:
|
||
|
||
>>> jnp.column_stack(jnp.nonzero(x))
|
||
Array([[0, 0],
|
||
[0, 2],
|
||
[1, 1]], dtype=int32)
|
||
|
||
Special case for zero-dimensional (i.e. scalar) inputs:
|
||
|
||
>>> jnp.argwhere(1)
|
||
Array([], shape=(1, 0), dtype=int32)
|
||
>>> jnp.argwhere(0)
|
||
Array([], shape=(0, 0), dtype=int32)
|
||
"""
|
||
result = transpose(vstack(nonzero(atleast_1d(a), size=size, fill_value=fill_value)))
|
||
if np.ndim(a) == 0:
|
||
return result[:0].reshape(result.shape[0], 0)
|
||
return result.reshape(result.shape[0], np.ndim(a))
|
||
|
||
|
||
@export
|
||
def argmax(a: ArrayLike, axis: int | None = None, out: None = None,
|
||
keepdims: bool | None = None) -> Array:
|
||
"""Return the index of the maximum value of an array.
|
||
|
||
JAX implementation of :func:`numpy.argmax`.
|
||
|
||
Args:
|
||
a: input array
|
||
axis: optional integer specifying the axis along which to find the maximum
|
||
value. If ``axis`` is not specified, ``a`` will be flattened.
|
||
out: unused by JAX
|
||
keepdims: if True, then return an array with the same number of dimensions
|
||
as ``a``.
|
||
|
||
Returns:
|
||
an array containing the index of the maximum value along the specified axis.
|
||
|
||
See also:
|
||
- :func:`jax.numpy.argmin`: return the index of the minimum value.
|
||
- :func:`jax.numpy.nanargmax`: compute ``argmax`` while ignoring NaN values.
|
||
|
||
Examples:
|
||
>>> x = jnp.array([1, 3, 5, 4, 2])
|
||
>>> jnp.argmax(x)
|
||
Array(2, dtype=int32)
|
||
|
||
>>> x = jnp.array([[1, 3, 2],
|
||
... [5, 4, 1]])
|
||
>>> jnp.argmax(x, axis=1)
|
||
Array([1, 0], dtype=int32)
|
||
|
||
>>> jnp.argmax(x, axis=1, keepdims=True)
|
||
Array([[1],
|
||
[0]], dtype=int32)
|
||
"""
|
||
arr = util.ensure_arraylike("argmax", a)
|
||
if out is not None:
|
||
raise NotImplementedError("The 'out' argument to jnp.argmax is not supported.")
|
||
return _argmax(arr, None if axis is None else operator.index(axis),
|
||
keepdims=bool(keepdims))
|
||
|
||
@partial(jit, static_argnames=('axis', 'keepdims'), inline=True)
|
||
def _argmax(a: Array, axis: int | None = None, keepdims: bool = False) -> Array:
|
||
if axis is None:
|
||
dims = list(range(np.ndim(a)))
|
||
a = ravel(a)
|
||
axis = 0
|
||
else:
|
||
dims = [axis]
|
||
if a.shape[axis] == 0:
|
||
raise ValueError("attempt to get argmax of an empty sequence")
|
||
result = lax.argmax(a, _canonicalize_axis(axis, a.ndim), dtypes.canonicalize_dtype(dtypes.int_))
|
||
return expand_dims(result, dims) if keepdims else result
|
||
|
||
|
||
@export
|
||
def argmin(a: ArrayLike, axis: int | None = None, out: None = None,
|
||
keepdims: bool | None = None) -> Array:
|
||
"""Return the index of the minimum value of an array.
|
||
|
||
JAX implementation of :func:`numpy.argmin`.
|
||
|
||
Args:
|
||
a: input array
|
||
axis: optional integer specifying the axis along which to find the minimum
|
||
value. If ``axis`` is not specified, ``a`` will be flattened.
|
||
out: unused by JAX
|
||
keepdims: if True, then return an array with the same number of dimensions
|
||
as ``a``.
|
||
|
||
Returns:
|
||
an array containing the index of the minimum value along the specified axis.
|
||
|
||
See also:
|
||
- :func:`jax.numpy.argmax`: return the index of the maximum value.
|
||
- :func:`jax.numpy.nanargmin`: compute ``argmin`` while ignoring NaN values.
|
||
|
||
Examples:
|
||
>>> x = jnp.array([1, 3, 5, 4, 2])
|
||
>>> jnp.argmin(x)
|
||
Array(0, dtype=int32)
|
||
|
||
>>> x = jnp.array([[1, 3, 2],
|
||
... [5, 4, 1]])
|
||
>>> jnp.argmin(x, axis=1)
|
||
Array([0, 2], dtype=int32)
|
||
|
||
>>> jnp.argmin(x, axis=1, keepdims=True)
|
||
Array([[0],
|
||
[2]], dtype=int32)
|
||
"""
|
||
arr = util.ensure_arraylike("argmin", a)
|
||
if out is not None:
|
||
raise NotImplementedError("The 'out' argument to jnp.argmin is not supported.")
|
||
return _argmin(arr, None if axis is None else operator.index(axis),
|
||
keepdims=bool(keepdims))
|
||
|
||
@partial(jit, static_argnames=('axis', 'keepdims'), inline=True)
|
||
def _argmin(a: Array, axis: int | None = None, keepdims: bool = False) -> Array:
|
||
if axis is None:
|
||
dims = list(range(np.ndim(a)))
|
||
a = ravel(a)
|
||
axis = 0
|
||
else:
|
||
dims = [axis]
|
||
if a.shape[axis] == 0:
|
||
raise ValueError("attempt to get argmin of an empty sequence")
|
||
result = lax.argmin(a, _canonicalize_axis(axis, a.ndim), dtypes.canonicalize_dtype(dtypes.int_))
|
||
return expand_dims(result, dims) if keepdims else result
|
||
|
||
|
||
@export
|
||
def nanargmax(
|
||
a: ArrayLike,
|
||
axis: int | None = None,
|
||
out: None = None,
|
||
keepdims: bool | None = None,
|
||
) -> Array:
|
||
"""Return the index of the maximum value of an array, ignoring NaNs.
|
||
|
||
JAX implementation of :func:`numpy.nanargmax`.
|
||
|
||
Args:
|
||
a: input array
|
||
axis: optional integer specifying the axis along which to find the maximum
|
||
value. If ``axis`` is not specified, ``a`` will be flattened.
|
||
out: unused by JAX
|
||
keepdims: if True, then return an array with the same number of dimensions
|
||
as ``a``.
|
||
|
||
Returns:
|
||
an array containing the index of the maximum value along the specified axis.
|
||
|
||
Note:
|
||
In the case of an axis with all-NaN values, the returned index will be -1.
|
||
This differs from the behavior of :func:`numpy.nanargmax`, which raises an error.
|
||
|
||
See also:
|
||
- :func:`jax.numpy.argmax`: return the index of the maximum value.
|
||
- :func:`jax.numpy.nanargmin`: compute ``argmin`` while ignoring NaN values.
|
||
|
||
Examples:
|
||
>>> x = jnp.array([1, 3, 5, 4, jnp.nan])
|
||
|
||
Using a standard :func:`~jax.numpy.argmax` leads to potentially unexpected results:
|
||
|
||
>>> jnp.argmax(x)
|
||
Array(4, dtype=int32)
|
||
|
||
Using ``nanargmax`` returns the index of the maximum non-NaN value.
|
||
|
||
>>> jnp.nanargmax(x)
|
||
Array(2, dtype=int32)
|
||
|
||
>>> x = jnp.array([[1, 3, jnp.nan],
|
||
... [5, 4, jnp.nan]])
|
||
>>> jnp.nanargmax(x, axis=1)
|
||
Array([1, 0], dtype=int32)
|
||
|
||
>>> jnp.nanargmax(x, axis=1, keepdims=True)
|
||
Array([[1],
|
||
[0]], dtype=int32)
|
||
"""
|
||
if out is not None:
|
||
raise NotImplementedError("The 'out' argument to jnp.nanargmax is not supported.")
|
||
return _nanargmax(a, None if axis is None else operator.index(axis), keepdims=bool(keepdims))
|
||
|
||
|
||
@partial(jit, static_argnames=('axis', 'keepdims'))
|
||
def _nanargmax(a, axis: int | None = None, keepdims: bool = False):
|
||
util.check_arraylike("nanargmax", a)
|
||
if not issubdtype(_dtype(a), np.inexact):
|
||
return argmax(a, axis=axis, keepdims=keepdims)
|
||
nan_mask = ufuncs.isnan(a)
|
||
a = where(nan_mask, -np.inf, a)
|
||
res = argmax(a, axis=axis, keepdims=keepdims)
|
||
return where(reductions.all(nan_mask, axis=axis, keepdims=keepdims), -1, res)
|
||
|
||
|
||
@export
|
||
def nanargmin(
|
||
a: ArrayLike,
|
||
axis: int | None = None,
|
||
out: None = None,
|
||
keepdims: bool | None = None,
|
||
) -> Array:
|
||
|
||
"""Return the index of the minimum value of an array, ignoring NaNs.
|
||
|
||
JAX implementation of :func:`numpy.nanargmin`.
|
||
|
||
Args:
|
||
a: input array
|
||
axis: optional integer specifying the axis along which to find the maximum
|
||
value. If ``axis`` is not specified, ``a`` will be flattened.
|
||
out: unused by JAX
|
||
keepdims: if True, then return an array with the same number of dimensions
|
||
as ``a``.
|
||
|
||
Returns:
|
||
an array containing the index of the minimum value along the specified axis.
|
||
|
||
Note:
|
||
In the case of an axis with all-NaN values, the returned index will be -1.
|
||
This differs from the behavior of :func:`numpy.nanargmin`, which raises an error.
|
||
|
||
See also:
|
||
- :func:`jax.numpy.argmin`: return the index of the minimum value.
|
||
- :func:`jax.numpy.nanargmax`: compute ``argmax`` while ignoring NaN values.
|
||
|
||
Examples:
|
||
>>> x = jnp.array([jnp.nan, 3, 5, 4, 2])
|
||
>>> jnp.nanargmin(x)
|
||
Array(4, dtype=int32)
|
||
|
||
>>> x = jnp.array([[1, 3, jnp.nan],
|
||
... [5, 4, jnp.nan]])
|
||
>>> jnp.nanargmin(x, axis=1)
|
||
Array([0, 1], dtype=int32)
|
||
|
||
>>> jnp.nanargmin(x, axis=1, keepdims=True)
|
||
Array([[0],
|
||
[1]], dtype=int32)
|
||
"""
|
||
if out is not None:
|
||
raise NotImplementedError("The 'out' argument to jnp.nanargmin is not supported.")
|
||
return _nanargmin(a, None if axis is None else operator.index(axis), keepdims=bool(keepdims))
|
||
|
||
|
||
@partial(jit, static_argnames=('axis', 'keepdims'))
|
||
def _nanargmin(a, axis: int | None = None, keepdims : bool = False):
|
||
util.check_arraylike("nanargmin", a)
|
||
if not issubdtype(_dtype(a), np.inexact):
|
||
return argmin(a, axis=axis, keepdims=keepdims)
|
||
nan_mask = ufuncs.isnan(a)
|
||
a = where(nan_mask, np.inf, a)
|
||
res = argmin(a, axis=axis, keepdims=keepdims)
|
||
return where(reductions.all(nan_mask, axis=axis, keepdims=keepdims), -1, res)
|
||
|
||
|
||
@partial(jit, static_argnums=(2,))
|
||
def _roll_dynamic(a: Array, shift: Array, axis: Sequence[int]) -> Array:
|
||
b_shape = lax.broadcast_shapes(shift.shape, np.shape(axis))
|
||
if len(b_shape) != 1:
|
||
msg = "'shift' and 'axis' arguments to roll must be scalars or 1D arrays"
|
||
raise ValueError(msg)
|
||
|
||
for x, i in zip(broadcast_to(shift, b_shape),
|
||
np.broadcast_to(axis, b_shape)):
|
||
a_shape_i = array(a.shape[i], dtype=np.int32)
|
||
x = ufuncs.remainder(lax.convert_element_type(x, np.int32),
|
||
lax.max(a_shape_i, np.int32(1)))
|
||
a_concat = lax.concatenate((a, a), i)
|
||
a = lax.dynamic_slice_in_dim(a_concat, a_shape_i - x, a.shape[i], axis=i)
|
||
return a
|
||
|
||
@partial(jit, static_argnums=(1, 2))
|
||
def _roll_static(a: Array, shift: Sequence[int], axis: Sequence[int]) -> Array:
|
||
for ax, s in zip(*np.broadcast_arrays(axis, shift)):
|
||
if a.shape[ax] == 0:
|
||
continue
|
||
i = (-s) % a.shape[ax]
|
||
a = lax.concatenate([lax.slice_in_dim(a, i, a.shape[ax], axis=ax),
|
||
lax.slice_in_dim(a, 0, i, axis=ax)],
|
||
dimension=ax)
|
||
return a
|
||
|
||
|
||
@export
|
||
def roll(a: ArrayLike, shift: ArrayLike | Sequence[int],
|
||
axis: int | Sequence[int] | None = None) -> Array:
|
||
"""Roll the elements of an array along a specified axis.
|
||
|
||
JAX implementation of :func:`numpy.roll`.
|
||
|
||
Args:
|
||
a: input array.
|
||
shift: the number of positions to shift the specified axis. If an integer,
|
||
all axes are shifted by the same amount. If a tuple, the shift for each
|
||
axis is specified individually.
|
||
axis: the axis or axes to roll. If ``None``, the array is flattened, shifted,
|
||
and then reshaped to its original shape.
|
||
|
||
Returns:
|
||
A copy of ``a`` with elements rolled along the specified axis or axes.
|
||
|
||
See also:
|
||
- :func:`jax.numpy.rollaxis`: roll the specified axis to a given position.
|
||
|
||
Examples:
|
||
>>> a = jnp.array([0, 1, 2, 3, 4, 5])
|
||
>>> jnp.roll(a, 2)
|
||
Array([4, 5, 0, 1, 2, 3], dtype=int32)
|
||
|
||
Roll elements along a specific axis:
|
||
|
||
>>> a = jnp.array([[ 0, 1, 2, 3],
|
||
... [ 4, 5, 6, 7],
|
||
... [ 8, 9, 10, 11]])
|
||
>>> jnp.roll(a, 1, axis=0)
|
||
Array([[ 8, 9, 10, 11],
|
||
[ 0, 1, 2, 3],
|
||
[ 4, 5, 6, 7]], dtype=int32)
|
||
>>> jnp.roll(a, [2, 3], axis=[0, 1])
|
||
Array([[ 5, 6, 7, 4],
|
||
[ 9, 10, 11, 8],
|
||
[ 1, 2, 3, 0]], dtype=int32)
|
||
"""
|
||
arr = util.ensure_arraylike("roll", a)
|
||
if axis is None:
|
||
return roll(arr.ravel(), shift, 0).reshape(arr.shape)
|
||
axis = _ensure_index_tuple(axis)
|
||
axis = tuple(_canonicalize_axis(ax, arr.ndim) for ax in axis)
|
||
try:
|
||
shift = _ensure_index_tuple(shift)
|
||
except TypeError:
|
||
return _roll_dynamic(arr, asarray(shift), axis)
|
||
else:
|
||
return _roll_static(arr, shift, axis)
|
||
|
||
|
||
@export
|
||
@partial(jit, static_argnames=('axis', 'start'))
|
||
def rollaxis(a: ArrayLike, axis: int, start: int = 0) -> Array:
|
||
"""Roll the specified axis to a given position.
|
||
|
||
JAX implementation of :func:`numpy.rollaxis`.
|
||
|
||
This function exists for compatibility with NumPy, but in most cases the newer
|
||
:func:`jax.numpy.moveaxis` instead, because the meaning of its arguments is
|
||
more intuitive.
|
||
|
||
Args:
|
||
a: input array.
|
||
axis: index of the axis to roll forward.
|
||
start: index toward which the axis will be rolled (default = 0). After
|
||
normalizing negative axes, if ``start <= axis``, the axis is rolled to
|
||
the ``start`` index; if ``start > axis``, the axis is rolled until the
|
||
position before ``start``.
|
||
|
||
Returns:
|
||
Copy of ``a`` with rolled axis.
|
||
|
||
Notes:
|
||
Unlike :func:`numpy.rollaxis`, :func:`jax.numpy.rollaxis` will return a copy rather
|
||
than a view of the input array. However, under JIT, the compiler will optimize away
|
||
such copies when possible, so this doesn't have performance impacts in practice.
|
||
|
||
See also:
|
||
- :func:`jax.numpy.moveaxis`: newer API with clearer semantics than ``rollaxis``;
|
||
this should be preferred to ``rollaxis`` in most cases.
|
||
- :func:`jax.numpy.swapaxes`: swap two axes.
|
||
- :func:`jax.numpy.transpose`: general permutation of axes.
|
||
|
||
Examples:
|
||
>>> a = jnp.ones((2, 3, 4, 5))
|
||
|
||
Roll axis 2 to the start of the array:
|
||
|
||
>>> jnp.rollaxis(a, 2).shape
|
||
(4, 2, 3, 5)
|
||
|
||
Roll axis 1 to the end of the array:
|
||
|
||
>>> jnp.rollaxis(a, 1, a.ndim).shape
|
||
(2, 4, 5, 3)
|
||
|
||
Equivalent of these two with :func:`~jax.numpy.moveaxis`
|
||
|
||
>>> jnp.moveaxis(a, 2, 0).shape
|
||
(4, 2, 3, 5)
|
||
>>> jnp.moveaxis(a, 1, -1).shape
|
||
(2, 4, 5, 3)
|
||
"""
|
||
util.check_arraylike("rollaxis", a)
|
||
start = core.concrete_or_error(operator.index, start, "'start' argument of jnp.rollaxis()")
|
||
a_ndim = np.ndim(a)
|
||
axis = _canonicalize_axis(axis, a_ndim)
|
||
if not (-a_ndim <= start <= a_ndim):
|
||
raise ValueError(f"{start=} must satisfy {-a_ndim}<=start<={a_ndim}")
|
||
if start < 0:
|
||
start += a_ndim
|
||
if start > axis:
|
||
start -= 1
|
||
return moveaxis(a, axis, start)
|
||
|
||
|
||
@export
|
||
@partial(jit, static_argnames=('axis', 'bitorder'))
|
||
def packbits(a: ArrayLike, axis: int | None = None, bitorder: str = "big") -> Array:
|
||
"""Pack array of bits into a uint8 array.
|
||
|
||
JAX implementation of :func:`numpy.packbits`
|
||
|
||
Args:
|
||
a: N-dimensional array of bits to pack.
|
||
axis: optional axis along which to pack bits. If not specified, ``a`` will
|
||
be flattened.
|
||
bitorder: ``"big"`` (default) or ``"little"``: specify whether the bit order
|
||
is big-endian or little-endian.
|
||
|
||
Returns:
|
||
A uint8 array of packed values.
|
||
|
||
See also:
|
||
- :func:`jax.numpy.unpackbits`: inverse of ``packbits``.
|
||
|
||
Examples:
|
||
Packing bits in one dimension:
|
||
|
||
>>> bits = jnp.array([0, 0, 0, 0, 0, 1, 1, 1])
|
||
>>> jnp.packbits(bits)
|
||
Array([7], dtype=uint8)
|
||
>>> 0b00000111 # equivalent bit-wise representation:
|
||
7
|
||
|
||
Optionally specifying little-endian convention:
|
||
|
||
>>> jnp.packbits(bits, bitorder="little")
|
||
Array([224], dtype=uint8)
|
||
>>> 0b11100000 # equivalent bit-wise representation
|
||
224
|
||
|
||
If the number of bits is not a multiple of 8, it will be right-padded
|
||
with zeros:
|
||
|
||
>>> jnp.packbits(jnp.array([1, 0, 1]))
|
||
Array([160], dtype=uint8)
|
||
>>> jnp.packbits(jnp.array([1, 0, 1, 0, 0, 0, 0, 0]))
|
||
Array([160], dtype=uint8)
|
||
|
||
For a multi-dimensional input, bits may be packed along a specified axis:
|
||
|
||
>>> a = jnp.array([[1, 1, 0, 1, 0, 1, 0, 0, 1, 0, 0, 1, 0, 1, 1, 0],
|
||
... [0, 1, 0, 0, 0, 1, 0, 1, 1, 1, 0, 0, 1, 1, 1, 1]])
|
||
>>> vals = jnp.packbits(a, axis=1)
|
||
>>> vals
|
||
Array([[212, 150],
|
||
[ 69, 207]], dtype=uint8)
|
||
|
||
The inverse of ``packbits`` is provided by :func:`~jax.numpy.unpackbits`:
|
||
|
||
>>> jnp.unpackbits(vals, axis=1)
|
||
Array([[1, 1, 0, 1, 0, 1, 0, 0, 1, 0, 0, 1, 0, 1, 1, 0],
|
||
[0, 1, 0, 0, 0, 1, 0, 1, 1, 1, 0, 0, 1, 1, 1, 1]], dtype=uint8)
|
||
"""
|
||
arr = util.ensure_arraylike("packbits", a)
|
||
if not (issubdtype(arr.dtype, np.integer) or issubdtype(arr.dtype, np.bool_)):
|
||
raise TypeError('Expected an input array of integer or boolean data type')
|
||
if bitorder not in ['little', 'big']:
|
||
raise ValueError("'order' must be either 'little' or 'big'")
|
||
arr = lax.gt(arr, _lax_const(a, 0)).astype('uint8')
|
||
bits = arange(8, dtype='uint8')
|
||
if bitorder == 'big':
|
||
bits = bits[::-1]
|
||
if axis is None:
|
||
arr = ravel(arr)
|
||
axis = 0
|
||
arr = swapaxes(arr, axis, -1)
|
||
|
||
remainder = arr.shape[-1] % 8
|
||
if remainder:
|
||
arr = lax.pad(arr, np.uint8(0),
|
||
(arr.ndim - 1) * [(0, 0, 0)] + [(0, 8 - remainder, 0)])
|
||
|
||
arr = arr.reshape(arr.shape[:-1] + (arr.shape[-1] // 8, 8))
|
||
bits = expand_dims(bits, tuple(range(arr.ndim - 1)))
|
||
packed = (arr << bits).sum(-1).astype('uint8')
|
||
return swapaxes(packed, axis, -1)
|
||
|
||
|
||
@export
|
||
@partial(jit, static_argnames=('axis', 'count', 'bitorder'))
|
||
def unpackbits(
|
||
a: ArrayLike,
|
||
axis: int | None = None,
|
||
count: int | None = None,
|
||
bitorder: str = "big",
|
||
) -> Array:
|
||
"""Unpack the bits in a uint8 array.
|
||
|
||
JAX implementation of :func:`numpy.unpackbits`.
|
||
|
||
Args:
|
||
a: N-dimensional array of type ``uint8``.
|
||
axis: optional axis along which to unpack. If not specified, ``a`` will
|
||
be flattened
|
||
count: specify the number of bits to unpack (if positive) or the number
|
||
of bits to trim from the end (if negative).
|
||
bitorder: ``"big"`` (default) or ``"little"``: specify whether the bit order
|
||
is big-endian or little-endian.
|
||
|
||
Returns:
|
||
a uint8 array of unpacked bits.
|
||
|
||
See also:
|
||
- :func:`jax.numpy.packbits`: this inverse of ``unpackbits``.
|
||
|
||
Examples:
|
||
Unpacking bits from a scalar:
|
||
|
||
>>> jnp.unpackbits(jnp.uint8(27)) # big-endian by default
|
||
Array([0, 0, 0, 1, 1, 0, 1, 1], dtype=uint8)
|
||
>>> jnp.unpackbits(jnp.uint8(27), bitorder="little")
|
||
Array([1, 1, 0, 1, 1, 0, 0, 0], dtype=uint8)
|
||
|
||
Compare this to the Python binary representation:
|
||
|
||
>>> 0b00011011
|
||
27
|
||
|
||
Unpacking bits along an axis:
|
||
|
||
>>> vals = jnp.array([[154],
|
||
... [ 49]], dtype='uint8')
|
||
>>> bits = jnp.unpackbits(vals, axis=1)
|
||
>>> bits
|
||
Array([[1, 0, 0, 1, 1, 0, 1, 0],
|
||
[0, 0, 1, 1, 0, 0, 0, 1]], dtype=uint8)
|
||
|
||
Using :func:`~jax.numpy.packbits` to invert this:
|
||
|
||
>>> jnp.packbits(bits, axis=1)
|
||
Array([[154],
|
||
[ 49]], dtype=uint8)
|
||
|
||
The ``count`` keyword lets ``unpackbits`` serve as an inverse of ``packbits``
|
||
in cases where not all bits are present:
|
||
|
||
>>> bits = jnp.array([1, 1, 0, 1, 1, 0, 1, 1, 0, 1, 1]) # 11 bits
|
||
>>> vals = jnp.packbits(bits)
|
||
>>> vals
|
||
Array([219, 96], dtype=uint8)
|
||
>>> jnp.unpackbits(vals) # 16 zero-padded bits
|
||
Array([1, 1, 0, 1, 1, 0, 1, 1, 0, 1, 1, 0, 0, 0, 0, 0], dtype=uint8)
|
||
>>> jnp.unpackbits(vals, count=11) # specify 11 output bits
|
||
Array([1, 1, 0, 1, 1, 0, 1, 1, 0, 1, 1], dtype=uint8)
|
||
>>> jnp.unpackbits(vals, count=-5) # specify 5 bits to be trimmed
|
||
Array([1, 1, 0, 1, 1, 0, 1, 1, 0, 1, 1], dtype=uint8)
|
||
"""
|
||
arr = util.ensure_arraylike("unpackbits", a)
|
||
if arr.dtype != np.uint8:
|
||
raise TypeError("Expected an input array of unsigned byte data type")
|
||
if bitorder not in ['little', 'big']:
|
||
raise ValueError("'order' must be either 'little' or 'big'")
|
||
bits = asarray(1) << arange(8, dtype='uint8')
|
||
if bitorder == 'big':
|
||
bits = bits[::-1]
|
||
if axis is None:
|
||
arr = ravel(arr)
|
||
axis = 0
|
||
arr = swapaxes(arr, axis, -1)
|
||
unpacked = ((arr[..., None] & expand_dims(bits, tuple(range(arr.ndim)))) > 0).astype('uint8')
|
||
unpacked = unpacked.reshape(unpacked.shape[:-2] + (-1,))
|
||
if count is not None:
|
||
if count > unpacked.shape[-1]:
|
||
unpacked = pad(unpacked, [(0, 0)] * (unpacked.ndim - 1) + [(0, count - unpacked.shape[-1])])
|
||
else:
|
||
unpacked = unpacked[..., :count]
|
||
return swapaxes(unpacked, axis, -1)
|
||
|
||
|
||
def _gcd_cond_fn(xs: tuple[Array, Array]) -> Array:
|
||
x1, x2 = xs
|
||
return reductions.any(x2 != 0)
|
||
|
||
def _gcd_body_fn(xs: tuple[Array, Array]) -> tuple[Array, Array]:
|
||
x1, x2 = xs
|
||
x1, x2 = (where(x2 != 0, x2, x1),
|
||
where(x2 != 0, lax.rem(x1, x2), _lax_const(x2, 0)))
|
||
return (where(x1 < x2, x2, x1), where(x1 < x2, x1, x2))
|
||
|
||
|
||
@export
|
||
@jit
|
||
def gcd(x1: ArrayLike, x2: ArrayLike) -> Array:
|
||
"""Compute the greatest common divisor of two arrays.
|
||
|
||
JAX implementation of :func:`numpy.gcd`.
|
||
|
||
Args:
|
||
x1: First input array. The elements must have integer dtype.
|
||
x2: Second input array. The elements must have integer dtype.
|
||
|
||
Returns:
|
||
An array containing the greatest common divisors of the corresponding
|
||
elements from the absolute values of `x1` and `x2`.
|
||
|
||
See also:
|
||
- :func:`jax.numpy.lcm`: compute the least common multiple of two arrays.
|
||
|
||
Examples:
|
||
Scalar inputs:
|
||
|
||
>>> jnp.gcd(12, 18)
|
||
Array(6, dtype=int32, weak_type=True)
|
||
|
||
Array inputs:
|
||
|
||
>>> x1 = jnp.array([12, 18, 24])
|
||
>>> x2 = jnp.array([5, 10, 15])
|
||
>>> jnp.gcd(x1, x2)
|
||
Array([1, 2, 3], dtype=int32)
|
||
|
||
Broadcasting:
|
||
|
||
>>> x1 = jnp.array([12])
|
||
>>> x2 = jnp.array([6, 9, 12])
|
||
>>> jnp.gcd(x1, x2)
|
||
Array([ 6, 3, 12], dtype=int32)
|
||
"""
|
||
util.check_arraylike("gcd", x1, x2)
|
||
x1, x2 = util.promote_dtypes(x1, x2)
|
||
if not issubdtype(_dtype(x1), np.integer):
|
||
raise ValueError("Arguments to jax.numpy.gcd must be integers.")
|
||
x1, x2 = broadcast_arrays(x1, x2)
|
||
gcd, _ = lax.while_loop(_gcd_cond_fn, _gcd_body_fn, (ufuncs.abs(x1), ufuncs.abs(x2)))
|
||
return gcd
|
||
|
||
|
||
@export
|
||
@jit
|
||
def lcm(x1: ArrayLike, x2: ArrayLike) -> Array:
|
||
"""Compute the least common multiple of two arrays.
|
||
|
||
JAX implementation of :func:`numpy.lcm`.
|
||
|
||
Args:
|
||
x1: First input array. The elements must have integer dtype.
|
||
x2: Second input array. The elements must have integer dtype.
|
||
|
||
Returns:
|
||
An array containing the least common multiple of the corresponding
|
||
elements from the absolute values of `x1` and `x2`.
|
||
|
||
See also:
|
||
- :func:`jax.numpy.gcd`: compute the greatest common divisor of two arrays.
|
||
|
||
Examples:
|
||
Scalar inputs:
|
||
|
||
>>> jnp.lcm(12, 18)
|
||
Array(36, dtype=int32, weak_type=True)
|
||
|
||
Array inputs:
|
||
|
||
>>> x1 = jnp.array([12, 18, 24])
|
||
>>> x2 = jnp.array([5, 10, 15])
|
||
>>> jnp.lcm(x1, x2)
|
||
Array([ 60, 90, 120], dtype=int32)
|
||
|
||
Broadcasting:
|
||
|
||
>>> x1 = jnp.array([12])
|
||
>>> x2 = jnp.array([6, 9, 12])
|
||
>>> jnp.lcm(x1, x2)
|
||
Array([12, 36, 12], dtype=int32)
|
||
"""
|
||
util.check_arraylike("lcm", x1, x2)
|
||
x1, x2 = util.promote_dtypes(x1, x2)
|
||
x1, x2 = ufuncs.abs(x1), ufuncs.abs(x2)
|
||
if not issubdtype(_dtype(x1), np.integer):
|
||
raise ValueError("Arguments to jax.numpy.lcm must be integers.")
|
||
d = gcd(x1, x2)
|
||
return where(d == 0, _lax_const(d, 0),
|
||
ufuncs.multiply(x1, ufuncs.floor_divide(x2, d)))
|
||
|
||
|
||
@export
|
||
def extract(condition: ArrayLike, arr: ArrayLike,
|
||
*, size: int | None = None, fill_value: ArrayLike = 0) -> Array:
|
||
"""Return the elements of an array that satisfy a condition.
|
||
|
||
JAX implementation of :func:`numpy.extract`.
|
||
|
||
Args:
|
||
condition: array of conditions. Will be converted to boolean and flattened to 1D.
|
||
arr: array of values to extract. Will be flattened to 1D.
|
||
size: optional static size for output. Must be specified in order for ``extract``
|
||
to be compatible with JAX transformations like :func:`~jax.jit` or :func:`~jax.vmap`.
|
||
fill_value: if ``size`` is specified, fill padded entries with this value (default: 0).
|
||
|
||
Returns:
|
||
1D array of extracted entries . If ``size`` is specified, the result will have shape
|
||
``(size,)`` and be right-padded with ``fill_value``. If ``size`` is not specified,
|
||
the output shape will depend on the number of True entries in ``condition``.
|
||
|
||
Notes:
|
||
This function does not require strict shape agreement between ``condition`` and ``arr``.
|
||
If ``condition.size > arr.size``, then ``condition`` will be truncated, and if
|
||
``arr.size > condition.size``, then ``arr`` will be truncated.
|
||
|
||
See also:
|
||
:func:`jax.numpy.compress`: multi-dimensional version of ``extract``.
|
||
|
||
Examples:
|
||
Extract values from a 1D array:
|
||
|
||
>>> x = jnp.array([1, 2, 3, 4, 5, 6])
|
||
>>> mask = (x % 2 == 0)
|
||
>>> jnp.extract(mask, x)
|
||
Array([2, 4, 6], dtype=int32)
|
||
|
||
In the simplest case, this is equivalent to boolean indexing:
|
||
|
||
>>> x[mask]
|
||
Array([2, 4, 6], dtype=int32)
|
||
|
||
For use with JAX transformations, you can pass the ``size`` argument to
|
||
specify a static shape for the output, along with an optional ``fill_value``
|
||
that defaults to zero:
|
||
|
||
>>> jnp.extract(mask, x, size=len(x), fill_value=0)
|
||
Array([2, 4, 6, 0, 0, 0], dtype=int32)
|
||
|
||
Notice that unlike with boolean indexing, ``extract`` does not require strict
|
||
agreement between the sizes of the array and condition, and will effectively
|
||
truncate both to the minimum size:
|
||
|
||
>>> short_mask = jnp.array([False, True])
|
||
>>> jnp.extract(short_mask, x)
|
||
Array([2], dtype=int32)
|
||
>>> long_mask = jnp.array([True, False, True, False, False, False, False, False])
|
||
>>> jnp.extract(long_mask, x)
|
||
Array([1, 3], dtype=int32)
|
||
"""
|
||
util.check_arraylike("extreact", condition, arr, fill_value)
|
||
return compress(ravel(condition), ravel(arr), size=size, fill_value=fill_value)
|
||
|
||
|
||
@export
|
||
def compress(condition: ArrayLike, a: ArrayLike, axis: int | None = None,
|
||
*, size: int | None = None, fill_value: ArrayLike = 0, out: None = None) -> Array:
|
||
"""Compress an array along a given axis using a boolean condition.
|
||
|
||
JAX implementation of :func:`numpy.compress`.
|
||
|
||
Args:
|
||
condition: 1-dimensional array of conditions. Will be converted to boolean.
|
||
a: N-dimensional array of values.
|
||
axis: axis along which to compress. If None (default) then ``a`` will be
|
||
flattened, and axis will be set to 0.
|
||
size: optional static size for output. Must be specified in order for ``compress``
|
||
to be compatible with JAX transformations like :func:`~jax.jit` or :func:`~jax.vmap`.
|
||
fill_value: if ``size`` is specified, fill padded entries with this value (default: 0).
|
||
out: not implemented by JAX.
|
||
|
||
Returns:
|
||
An array of dimension ``a.ndim``, compressed along the specified axis.
|
||
|
||
See also:
|
||
- :func:`jax.numpy.extract`: 1D version of ``compress``.
|
||
- :meth:`jax.Array.compress`: equivalent functionality as an array method.
|
||
|
||
Notes:
|
||
This function does not require strict shape agreement between ``condition`` and ``a``.
|
||
If ``condition.size > a.shape[axis]``, then ``condition`` will be truncated, and if
|
||
``a.shape[axis] > condition.size``, then ``a`` will be truncated.
|
||
|
||
Examples:
|
||
Compressing along the rows of a 2D array:
|
||
|
||
>>> a = jnp.array([[1, 2, 3, 4],
|
||
... [5, 6, 7, 8],
|
||
... [9, 10, 11, 12]])
|
||
>>> condition = jnp.array([True, False, True])
|
||
>>> jnp.compress(condition, a, axis=0)
|
||
Array([[ 1, 2, 3, 4],
|
||
[ 9, 10, 11, 12]], dtype=int32)
|
||
|
||
For convenience, you can equivalently use the :meth:`~jax.Array.compress`
|
||
method of JAX arrays:
|
||
|
||
>>> a.compress(condition, axis=0)
|
||
Array([[ 1, 2, 3, 4],
|
||
[ 9, 10, 11, 12]], dtype=int32)
|
||
|
||
Note that the condition need not match the shape of the specified axis;
|
||
here we compress the columns with the length-3 condition. Values beyond
|
||
the size of the condition are ignored:
|
||
|
||
>>> jnp.compress(condition, a, axis=1)
|
||
Array([[ 1, 3],
|
||
[ 5, 7],
|
||
[ 9, 11]], dtype=int32)
|
||
|
||
The optional ``size`` argument lets you specify a static output size so
|
||
that the output is statically-shaped, and so this function can be used
|
||
with transformations like :func:`~jax.jit` and :func:`~jax.vmap`:
|
||
|
||
>>> f = lambda c, a: jnp.extract(c, a, size=len(a), fill_value=0)
|
||
>>> mask = (a % 3 == 0)
|
||
>>> jax.vmap(f)(mask, a)
|
||
Array([[ 3, 0, 0, 0],
|
||
[ 6, 0, 0, 0],
|
||
[ 9, 12, 0, 0]], dtype=int32)
|
||
"""
|
||
condition_arr, arr, fill_value = util.ensure_arraylike("compress", condition, a, fill_value)
|
||
condition_arr = condition_arr.astype(bool)
|
||
if out is not None:
|
||
raise NotImplementedError("The 'out' argument to jnp.compress is not supported.")
|
||
if condition_arr.ndim != 1:
|
||
raise ValueError("condition must be a 1D array")
|
||
if axis is None:
|
||
axis = 0
|
||
arr = ravel(arr)
|
||
else:
|
||
arr = moveaxis(arr, axis, 0)
|
||
condition_arr, extra = condition_arr[:arr.shape[0]], condition_arr[arr.shape[0]:]
|
||
arr = arr[:condition_arr.shape[0]]
|
||
|
||
if size is None:
|
||
if reductions.any(extra):
|
||
raise ValueError("condition contains entries that are out of bounds")
|
||
result = arr[condition_arr]
|
||
elif not 0 <= size <= arr.shape[0]:
|
||
raise ValueError("size must be positive and not greater than the size of the array axis;"
|
||
f" got {size=} for a.shape[axis]={arr.shape[0]}")
|
||
else:
|
||
mask = expand_dims(condition_arr, range(1, arr.ndim))
|
||
arr = where(mask, arr, array(fill_value, dtype=arr.dtype))
|
||
result = arr[argsort(condition_arr, stable=True, descending=True)][:size]
|
||
return moveaxis(result, 0, axis)
|
||
|
||
|
||
@export
|
||
@partial(jit, static_argnames=('rowvar', 'bias', 'ddof'))
|
||
def cov(m: ArrayLike, y: ArrayLike | None = None, rowvar: bool = True,
|
||
bias: bool = False, ddof: int | None = None,
|
||
fweights: ArrayLike | None = None,
|
||
aweights: ArrayLike | None = None) -> Array:
|
||
r"""Estimate the weighted sample covariance.
|
||
|
||
JAX implementation of :func:`numpy.cov`.
|
||
|
||
The covariance :math:`C_{ij}` between variable *i* and variable *j* is defined
|
||
as
|
||
|
||
.. math::
|
||
|
||
cov[X_i, X_j] = E[(X_i - E[X_i])(X_j - E[X_j])]
|
||
|
||
Given an array of *N* observations of the variables :math:`X_i` and :math:`X_j`,
|
||
this can be estimated via the sample covariance:
|
||
|
||
.. math::
|
||
|
||
C_{ij} = \frac{1}{N - 1} \sum_{n=1}^N (X_{in} - \overline{X_i})(X_{jn} - \overline{X_j})
|
||
|
||
Where :math:`\overline{X_i} = \frac{1}{N} \sum_{k=1}^N X_{ik}` is the mean of the
|
||
observations.
|
||
|
||
Args:
|
||
m: array of shape ``(M, N)`` (if ``rowvar`` is True), or ``(N, M)``
|
||
(if ``rowvar`` is False) representing ``N`` observations of ``M`` variables.
|
||
``m`` may also be one-dimensional, representing ``N`` observations of a
|
||
single variable.
|
||
y: optional set of additional observations, with the same form as ``m``. If
|
||
specified, then ``y`` is combined with ``m``, i.e. for the default
|
||
``rowvar = True`` case, ``m`` becomes ``jnp.vstack([m, y])``.
|
||
rowvar: if True (default) then each row of ``m`` represents a variable. If
|
||
False, then each column represents a variable.
|
||
bias: if False (default) then normalize the covariance by ``N - 1``. If True,
|
||
then normalize the covariance by ``N``
|
||
ddof: specify the degrees of freedom. Defaults to ``1`` if ``bias`` is False,
|
||
or to ``0`` if ``bias`` is True.
|
||
fweights: optional array of integer frequency weights of shape ``(N,)``. This
|
||
is an absolute weight specifying the number of times each observation is
|
||
included in the computation.
|
||
aweights: optional array of observation weights of shape ``(N,)``. This is
|
||
a relative weight specifying the "importance" of each observation. In the
|
||
``ddof=0`` case, it is equivalent to assigning probabilities to each
|
||
observation.
|
||
|
||
Returns:
|
||
A covariance matrix of shape ``(M, M)``, or a scalar with shape ``()`` if ``M = 1``.
|
||
|
||
See also:
|
||
- :func:`jax.numpy.corrcoef`: compute the correlation coefficient, a normalized
|
||
version of the covariance matrix.
|
||
|
||
Examples:
|
||
Consider these observations of two variables that correlate perfectly.
|
||
The covariance matrix in this case is a 2x2 matrix of ones:
|
||
|
||
>>> x = jnp.array([[0, 1, 2],
|
||
... [0, 1, 2]])
|
||
>>> jnp.cov(x)
|
||
Array([[1., 1.],
|
||
[1., 1.]], dtype=float32)
|
||
|
||
Now consider these observations of two variables that are perfectly
|
||
anti-correlated. The covariance matrix in this case has ``-1`` in the
|
||
off-diagonal:
|
||
|
||
>>> x = jnp.array([[-1, 0, 1],
|
||
... [ 1, 0, -1]])
|
||
>>> jnp.cov(x)
|
||
Array([[ 1., -1.],
|
||
[-1., 1.]], dtype=float32)
|
||
|
||
Equivalently, these sequences can be specified as separate arguments,
|
||
in which case they are stacked before continuing the computation.
|
||
|
||
>>> x = jnp.array([-1, 0, 1])
|
||
>>> y = jnp.array([1, 0, -1])
|
||
>>> jnp.cov(x, y)
|
||
Array([[ 1., -1.],
|
||
[-1., 1.]], dtype=float32)
|
||
|
||
In general, the entries of the covariance matrix may be any positive
|
||
or negative real value. For example, here is the covariance of 100
|
||
points drawn from a 3-dimensional standard normal distribution:
|
||
|
||
>>> key = jax.random.key(0)
|
||
>>> x = jax.random.normal(key, shape=(3, 100))
|
||
>>> with jnp.printoptions(precision=2):
|
||
... print(jnp.cov(x))
|
||
[[0.9 0.03 0.1 ]
|
||
[0.03 1. 0.01]
|
||
[0.1 0.01 0.85]]
|
||
"""
|
||
if y is not None:
|
||
m, y = util.promote_args_inexact("cov", m, y)
|
||
if y.ndim > 2:
|
||
raise ValueError("y has more than 2 dimensions")
|
||
else:
|
||
m, = util.promote_args_inexact("cov", m)
|
||
|
||
if m.ndim > 2:
|
||
raise ValueError("m has more than 2 dimensions") # same as numpy error
|
||
|
||
X = atleast_2d(m)
|
||
if not rowvar and X.shape[0] != 1:
|
||
X = X.T
|
||
if X.shape[0] == 0:
|
||
return array([]).reshape(0, 0)
|
||
|
||
if y is not None:
|
||
y_arr = atleast_2d(y)
|
||
if not rowvar and y_arr.shape[0] != 1:
|
||
y_arr = y_arr.T
|
||
X = concatenate((X, y_arr), axis=0)
|
||
if ddof is None:
|
||
ddof = 1 if bias == 0 else 0
|
||
|
||
w: Array | None = None
|
||
if fweights is not None:
|
||
fweights = util.ensure_arraylike("cov", fweights)
|
||
if np.ndim(fweights) > 1:
|
||
raise RuntimeError("cannot handle multidimensional fweights")
|
||
if np.shape(fweights)[0] != X.shape[1]:
|
||
raise RuntimeError("incompatible numbers of samples and fweights")
|
||
if not issubdtype(_dtype(fweights), np.integer):
|
||
raise TypeError("fweights must be integer.")
|
||
# Ensure positive fweights; note that numpy raises an error on negative fweights.
|
||
w = abs(fweights)
|
||
if aweights is not None:
|
||
aweights = util.ensure_arraylike("cov", aweights)
|
||
if np.ndim(aweights) > 1:
|
||
raise RuntimeError("cannot handle multidimensional aweights")
|
||
if np.shape(aweights)[0] != X.shape[1]:
|
||
raise RuntimeError("incompatible numbers of samples and aweights")
|
||
# Ensure positive aweights: note that numpy raises an error for negative aweights.
|
||
aweights = abs(aweights)
|
||
w = aweights if w is None else w * aweights
|
||
|
||
avg, w_sum = reductions.average(X, axis=1, weights=w, returned=True)
|
||
w_sum = w_sum[0]
|
||
|
||
if w is None:
|
||
f = X.shape[1] - ddof
|
||
elif ddof == 0:
|
||
f = w_sum
|
||
elif aweights is None:
|
||
f = w_sum - ddof
|
||
else:
|
||
f = w_sum - ddof * reductions.sum(w * aweights) / w_sum
|
||
|
||
X = X - avg[:, None]
|
||
X_T = X.T if w is None else (X * lax.broadcast_to_rank(w, X.ndim)).T
|
||
return ufuncs.true_divide(tensor_contractions.dot(X, X_T.conj()), f).squeeze()
|
||
|
||
|
||
@export
|
||
@partial(jit, static_argnames=('rowvar',))
|
||
def corrcoef(x: ArrayLike, y: ArrayLike | None = None, rowvar: bool = True) -> Array:
|
||
r"""Compute the Pearson correlation coefficients.
|
||
|
||
JAX implementation of :func:`numpy.corrcoef`.
|
||
|
||
This is a normalized version of the sample covariance computed by :func:`jax.numpy.cov`.
|
||
For a sample covariance :math:`C_{ij}`, the correlation coefficients are
|
||
|
||
.. math::
|
||
|
||
R_{ij} = \frac{C_{ij}}{\sqrt{C_{ii}C_{jj}}}
|
||
|
||
they are constructed such that the values satisfy :math:`-1 \le R_{ij} \le 1`.
|
||
|
||
Args:
|
||
x: array of shape ``(M, N)`` (if ``rowvar`` is True), or ``(N, M)``
|
||
(if ``rowvar`` is False) representing ``N`` observations of ``M`` variables.
|
||
``x`` may also be one-dimensional, representing ``N`` observations of a
|
||
single variable.
|
||
y: optional set of additional observations, with the same form as ``m``. If
|
||
specified, then ``y`` is combined with ``m``, i.e. for the default
|
||
``rowvar = True`` case, ``m`` becomes ``jnp.vstack([m, y])``.
|
||
rowvar: if True (default) then each row of ``m`` represents a variable. If
|
||
False, then each column represents a variable.
|
||
|
||
Returns:
|
||
A covariance matrix of shape ``(M, M)``.
|
||
|
||
See also:
|
||
- :func:`jax.numpy.cov`: compute the covariance matrix.
|
||
|
||
Examples:
|
||
Consider these observations of two variables that correlate perfectly.
|
||
The correlation matrix in this case is a 2x2 matrix of ones:
|
||
|
||
>>> x = jnp.array([[0, 1, 2],
|
||
... [0, 1, 2]])
|
||
>>> jnp.corrcoef(x)
|
||
Array([[1., 1.],
|
||
[1., 1.]], dtype=float32)
|
||
|
||
Now consider these observations of two variables that are perfectly
|
||
anti-correlated. The correlation matrix in this case has ``-1`` in the
|
||
off-diagonal:
|
||
|
||
>>> x = jnp.array([[-1, 0, 1],
|
||
... [ 1, 0, -1]])
|
||
>>> jnp.corrcoef(x)
|
||
Array([[ 1., -1.],
|
||
[-1., 1.]], dtype=float32)
|
||
|
||
Equivalently, these sequences can be specified as separate arguments,
|
||
in which case they are stacked before continuing the computation.
|
||
|
||
>>> x = jnp.array([-1, 0, 1])
|
||
>>> y = jnp.array([1, 0, -1])
|
||
>>> jnp.corrcoef(x, y)
|
||
Array([[ 1., -1.],
|
||
[-1., 1.]], dtype=float32)
|
||
|
||
The entries of the correlation matrix are normalized such that they
|
||
lie within the range -1 to +1, where +1 indicates perfect correlation
|
||
and -1 indicates perfect anti-correlation. For example, here is the
|
||
correlation of 100 points drawn from a 3-dimensional standard normal
|
||
distribution:
|
||
|
||
>>> key = jax.random.key(0)
|
||
>>> x = jax.random.normal(key, shape=(3, 100))
|
||
>>> with jnp.printoptions(precision=2):
|
||
... print(jnp.corrcoef(x))
|
||
[[1. 0.03 0.12]
|
||
[0.03 1. 0.01]
|
||
[0.12 0.01 1. ]]
|
||
"""
|
||
util.check_arraylike("corrcoef", x)
|
||
c = cov(x, y, rowvar)
|
||
if len(np.shape(c)) == 0:
|
||
# scalar - this should yield nan for values (nan/nan, inf/inf, 0/0), 1 otherwise
|
||
return ufuncs.divide(c, c)
|
||
d = diag(c)
|
||
stddev = ufuncs.sqrt(ufuncs.real(d)).astype(c.dtype)
|
||
c = c / stddev[:, None] / stddev[None, :]
|
||
|
||
real_part = clip(ufuncs.real(c), -1, 1)
|
||
if iscomplexobj(c):
|
||
complex_part = clip(ufuncs.imag(c), -1, 1)
|
||
c = lax.complex(real_part, complex_part)
|
||
else:
|
||
c = real_part
|
||
return c
|
||
|
||
|
||
@partial(vectorize, excluded={0, 1, 3, 4})
|
||
def _searchsorted_via_scan(unrolled: bool, sorted_arr: Array, query: Array, side: str, dtype: type) -> Array:
|
||
op = _sort_le_comparator if side == 'left' else _sort_lt_comparator
|
||
unsigned_dtype = np.uint32 if dtype == np.int32 else np.uint64
|
||
def body_fun(state, _):
|
||
low, high = state
|
||
mid = low.astype(unsigned_dtype) + high.astype(unsigned_dtype)
|
||
mid = lax.div(mid, unsigned_dtype(2)).astype(dtype)
|
||
go_left = op(query, sorted_arr[mid])
|
||
return (where(go_left, low, mid), where(go_left, mid, high)), ()
|
||
n_levels = int(np.ceil(np.log2(len(sorted_arr) + 1)))
|
||
init = (array(0, dtype=dtype), array(len(sorted_arr), dtype=dtype))
|
||
carry, _ = lax.scan(body_fun, init, (), length=n_levels,
|
||
unroll=n_levels if unrolled else 1)
|
||
return carry[1]
|
||
|
||
|
||
def _searchsorted_via_sort(sorted_arr: Array, query: Array, side: str, dtype: type) -> Array:
|
||
working_dtype = np.dtype('int32') if sorted_arr.size + query.size < np.iinfo(np.int32).max else np.dtype('int64')
|
||
def _rank(x):
|
||
idx = lax.iota(working_dtype, x.shape[0])
|
||
return zeros_like(idx).at[argsort(x)].set(idx)
|
||
query_flat = query.ravel()
|
||
if side == 'left':
|
||
index = _rank(lax.concatenate([query_flat, sorted_arr], 0))[:query.size]
|
||
else:
|
||
index = _rank(lax.concatenate([sorted_arr, query_flat], 0))[sorted_arr.size:]
|
||
return lax.reshape(lax.sub(index, _rank(query_flat)), np.shape(query)).astype(dtype)
|
||
|
||
|
||
def _searchsorted_via_compare_all(sorted_arr: Array, query: Array, side: str, dtype: type) -> Array:
|
||
op = _sort_lt_comparator if side == 'left' else _sort_le_comparator
|
||
comparisons = jax.vmap(op, in_axes=(0, None))(sorted_arr, query)
|
||
return comparisons.sum(dtype=dtype, axis=0)
|
||
|
||
|
||
@export
|
||
@partial(jit, static_argnames=('side', 'method'))
|
||
def searchsorted(a: ArrayLike, v: ArrayLike, side: str = 'left',
|
||
sorter: ArrayLike | None = None, *, method: str = 'scan') -> Array:
|
||
"""Perform a binary search within a sorted array.
|
||
|
||
JAX implementation of :func:`numpy.searchsorted`.
|
||
|
||
This will return the indices within a sorted array ``a`` where values in ``v``
|
||
can be inserted to maintain its sort order.
|
||
|
||
Args:
|
||
a: one-dimensional array, assumed to be in sorted order unless ``sorter`` is specified.
|
||
v: N-dimensional array of query values
|
||
side: ``'left'`` (default) or ``'right'``; specifies whether insertion indices will be
|
||
to the left or the right in case of ties.
|
||
sorter: optional array of indices specifying the sort order of ``a``. If specified,
|
||
then the algorithm assumes that ``a[sorter]`` is in sorted order.
|
||
method: one of ``'scan'`` (default), ``'scan_unrolled'``, ``'sort'`` or ``'compare_all'``.
|
||
See *Note* below.
|
||
|
||
Returns:
|
||
Array of insertion indices of shape ``v.shape``.
|
||
|
||
Note:
|
||
The ``method`` argument controls the algorithm used to compute the insertion indices.
|
||
|
||
- ``'scan'`` (the default) tends to be more performant on CPU, particularly when ``a`` is
|
||
very large.
|
||
- ``'scan_unrolled'`` is more performant on GPU at the expense of additional compile time.
|
||
- ``'sort'`` is often more performant on accelerator backends like GPU and TPU, particularly
|
||
when ``v`` is very large.
|
||
- ``'compare_all'`` tends to be the most performant when ``a`` is very small.
|
||
|
||
Examples:
|
||
Searching for a single value:
|
||
|
||
>>> a = jnp.array([1, 2, 2, 3, 4, 5, 5])
|
||
>>> jnp.searchsorted(a, 2)
|
||
Array(1, dtype=int32)
|
||
>>> jnp.searchsorted(a, 2, side='right')
|
||
Array(3, dtype=int32)
|
||
|
||
Searching for a batch of values:
|
||
|
||
>>> vals = jnp.array([0, 3, 8, 1.5, 2])
|
||
>>> jnp.searchsorted(a, vals)
|
||
Array([0, 3, 7, 1, 1], dtype=int32)
|
||
|
||
Optionally, the ``sorter`` argument can be used to find insertion indices into
|
||
an array sorted via :func:`jax.numpy.argsort`:
|
||
|
||
>>> a = jnp.array([4, 3, 5, 1, 2])
|
||
>>> sorter = jnp.argsort(a)
|
||
>>> jnp.searchsorted(a, vals, sorter=sorter)
|
||
Array([0, 2, 5, 1, 1], dtype=int32)
|
||
|
||
The result is equivalent to passing the sorted array:
|
||
|
||
>>> jnp.searchsorted(jnp.sort(a), vals)
|
||
Array([0, 2, 5, 1, 1], dtype=int32)
|
||
"""
|
||
if sorter is None:
|
||
util.check_arraylike("searchsorted", a, v)
|
||
else:
|
||
util.check_arraylike("searchsorted", a, v, sorter)
|
||
if side not in ['left', 'right']:
|
||
raise ValueError(f"{side!r} is an invalid value for keyword 'side'. "
|
||
"Expected one of ['left', 'right'].")
|
||
if method not in ['scan', 'scan_unrolled', 'sort', 'compare_all']:
|
||
raise ValueError(
|
||
f"{method!r} is an invalid value for keyword 'method'. "
|
||
"Expected one of ['sort', 'scan', 'scan_unrolled', 'compare_all'].")
|
||
if np.ndim(a) != 1:
|
||
raise ValueError("a should be 1-dimensional")
|
||
a, v = util.promote_dtypes(a, v)
|
||
if sorter is not None:
|
||
a = a[sorter]
|
||
dtype = np.dtype('int32') if a.shape[0] <= np.iinfo(np.int32).max else np.dtype('int64')
|
||
if a.shape[0] == 0:
|
||
return zeros_like(v, dtype=dtype)
|
||
impl = {
|
||
'scan': partial(_searchsorted_via_scan, False),
|
||
'scan_unrolled': partial(_searchsorted_via_scan, True),
|
||
'sort': _searchsorted_via_sort,
|
||
'compare_all': _searchsorted_via_compare_all,
|
||
}[method]
|
||
return impl(a, v, side, dtype) # type: ignore
|
||
|
||
|
||
@export
|
||
@partial(jit, static_argnames=('right', 'method'))
|
||
def digitize(x: ArrayLike, bins: ArrayLike, right: bool = False,
|
||
*, method: str | None = None) -> Array:
|
||
"""Convert an array to bin indices.
|
||
|
||
JAX implementation of :func:`numpy.digitize`.
|
||
|
||
Args:
|
||
x: array of values to digitize.
|
||
bins: 1D array of bin edges. Must be monotonically increasing or decreasing.
|
||
right: if true, the intervals include the right bin edges. If false (default)
|
||
the intervals include the left bin edges.
|
||
method: optional method argument to be passed to :func:`~jax.numpy.searchsorted`.
|
||
See that function for available options.
|
||
|
||
Returns:
|
||
An integer array of the same shape as ``x`` indicating the bin number that
|
||
the values are in.
|
||
|
||
See also:
|
||
- :func:`jax.numpy.searchsorted`: find insertion indices for values in a
|
||
sorted array.
|
||
- :func:`jax.numpy.histogram`: compute frequency of array values within
|
||
specified bins.
|
||
|
||
Examples:
|
||
>>> x = jnp.array([1.0, 2.0, 2.5, 1.5, 3.0, 3.5])
|
||
>>> bins = jnp.array([1, 2, 3])
|
||
>>> jnp.digitize(x, bins)
|
||
Array([1, 2, 2, 1, 3, 3], dtype=int32)
|
||
>>> jnp.digitize(x, bins, right=True)
|
||
Array([0, 1, 2, 1, 2, 3], dtype=int32)
|
||
|
||
``digitize`` supports reverse-ordered bins as well:
|
||
|
||
>>> bins = jnp.array([3, 2, 1])
|
||
>>> jnp.digitize(x, bins)
|
||
Array([2, 1, 1, 2, 0, 0], dtype=int32)
|
||
"""
|
||
x, bins_arr = util.ensure_arraylike("digitize", x, bins)
|
||
right = core.concrete_or_error(bool, right, "right argument of jnp.digitize()")
|
||
if bins_arr.ndim != 1:
|
||
raise ValueError(f"digitize: bins must be a 1-dimensional array; got {bins=}")
|
||
if bins_arr.shape[0] == 0:
|
||
return zeros_like(x, dtype=np.int32)
|
||
side = 'right' if not right else 'left'
|
||
kwds: dict[str, str] = {} if method is None else {'method': method}
|
||
return where(
|
||
bins_arr[-1] >= bins_arr[0],
|
||
searchsorted(bins_arr, x, side=side, **kwds),
|
||
bins_arr.shape[0] - searchsorted(bins_arr[::-1], x, side=side, **kwds)
|
||
)
|
||
|
||
|
||
@export
|
||
def piecewise(x: ArrayLike, condlist: Array | Sequence[ArrayLike],
|
||
funclist: list[ArrayLike | Callable[..., Array]],
|
||
*args, **kw) -> Array:
|
||
"""Evaluate a function defined piecewise across the domain.
|
||
|
||
JAX implementation of :func:`numpy.piecewise`, in terms of :func:`jax.lax.switch`.
|
||
|
||
Note:
|
||
Unlike :func:`numpy.piecewise`, :func:`jax.numpy.piecewise` requires functions
|
||
in ``funclist`` to be traceable by JAX, as it is implemented via
|
||
:func:`jax.lax.switch`.
|
||
|
||
Args:
|
||
x: array of input values.
|
||
condlist: boolean array or sequence of boolean arrays corresponding to the
|
||
functions in ``funclist``. If a sequence of arrays, the length of each
|
||
array must match the length of ``x``
|
||
funclist: list of arrays or functions; must either be the same length as
|
||
``condlist``, or have length ``len(condlist) + 1``, in which case the
|
||
last entry is the default applied when none of the conditions are True.
|
||
Alternatively, entries of ``funclist`` may be numerical values, in which
|
||
case they indicate a constant function.
|
||
args, kwargs: additional arguments are passed to each function in
|
||
``funclist``.
|
||
|
||
Returns:
|
||
An array which is the result of evaluating the functions on ``x`` at
|
||
the specified conditions.
|
||
|
||
See also:
|
||
- :func:`jax.lax.switch`: choose between *N* functions based on an index.
|
||
- :func:`jax.lax.cond`: choose between two functions based on a boolean condition.
|
||
- :func:`jax.numpy.where`: choose between two results based on a boolean mask.
|
||
- :func:`jax.lax.select`: choose between two results based on a boolean mask.
|
||
- :func:`jax.lax.select_n`: choose between *N* results based on a boolean mask.
|
||
|
||
Examples:
|
||
Here's an example of a function which is zero for negative values, and linear
|
||
for positive values:
|
||
|
||
>>> x = jnp.array([-4, -3, -2, -1, 0, 1, 2, 3, 4])
|
||
|
||
>>> condlist = [x < 0, x >= 0]
|
||
>>> funclist = [lambda x: 0 * x, lambda x: x]
|
||
>>> jnp.piecewise(x, condlist, funclist)
|
||
Array([0, 0, 0, 0, 0, 1, 2, 3, 4], dtype=int32)
|
||
|
||
``funclist`` can also contain a simple scalar value for constant functions:
|
||
|
||
>>> condlist = [x < 0, x >= 0]
|
||
>>> funclist = [0, lambda x: x]
|
||
>>> jnp.piecewise(x, condlist, funclist)
|
||
Array([0, 0, 0, 0, 0, 1, 2, 3, 4], dtype=int32)
|
||
|
||
You can specify a default value by appending an extra condition to ``funclist``:
|
||
|
||
>>> condlist = [x < -1, x > 1]
|
||
>>> funclist = [lambda x: 1 + x, lambda x: x - 1, 0]
|
||
>>> jnp.piecewise(x, condlist, funclist)
|
||
Array([-3, -2, -1, 0, 0, 0, 1, 2, 3], dtype=int32)
|
||
|
||
``condlist`` may also be a simple array of scalar conditions, in which case
|
||
the associated function applies to the whole range
|
||
|
||
>>> condlist = jnp.array([False, True, False])
|
||
>>> funclist = [lambda x: x * 0, lambda x: x * 10, lambda x: x * 100]
|
||
>>> jnp.piecewise(x, condlist, funclist)
|
||
Array([-40, -30, -20, -10, 0, 10, 20, 30, 40], dtype=int32)
|
||
"""
|
||
x_arr = util.ensure_arraylike("piecewise", x)
|
||
nc, nf = len(condlist), len(funclist)
|
||
if nf == nc + 1:
|
||
funclist = funclist[-1:] + funclist[:-1]
|
||
elif nf == nc:
|
||
funclist = [0] + list(funclist)
|
||
else:
|
||
raise ValueError(f"with {nc} condition(s), either {nc} or {nc+1} functions are expected; got {nf}")
|
||
consts = {i: c for i, c in enumerate(funclist) if not callable(c)}
|
||
funcs = {i: f for i, f in enumerate(funclist) if callable(f)}
|
||
return _piecewise(x_arr, asarray(condlist, dtype=bool), consts,
|
||
frozenset(funcs.items()), # dict is not hashable.
|
||
*args, **kw)
|
||
|
||
@partial(jit, static_argnames=['funcs'])
|
||
def _piecewise(x: Array, condlist: Array, consts: dict[int, ArrayLike],
|
||
funcs: frozenset[tuple[int, Callable[..., Array]]],
|
||
*args, **kw) -> Array:
|
||
funcdict = dict(funcs)
|
||
funclist = [consts.get(i, funcdict.get(i)) for i in range(len(condlist) + 1)]
|
||
indices = argmax(reductions.cumsum(concatenate([zeros_like(condlist[:1]), condlist], 0), 0), 0)
|
||
dtype = _dtype(x)
|
||
def _call(f):
|
||
return lambda x: f(x, *args, **kw).astype(dtype)
|
||
def _const(v):
|
||
return lambda x: array(v, dtype=dtype)
|
||
funclist = [_call(f) if callable(f) else _const(f) for f in funclist]
|
||
return vectorize(lax.switch, excluded=(1,))(indices, funclist, x)
|
||
|
||
|
||
def _tile_to_size(arr: Array, size: int) -> Array:
|
||
assert arr.ndim == 1
|
||
if arr.size < size:
|
||
arr = tile(arr, int(np.ceil(size / arr.size)))
|
||
assert arr.size >= size
|
||
return arr[:size] if arr.size > size else arr
|