mirror of
https://github.com/ROCm/jax.git
synced 2025-04-19 05:16:06 +00:00
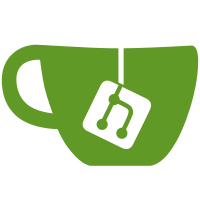
Convert shardings returned by XLA (when propagation is on for input and output) for extended dtypes to user shardings which allows to remove `are_out_shardings_from_xla`. PiperOrigin-RevId: 611246986
112 lines
3.8 KiB
Python
112 lines
3.8 KiB
Python
# Copyright 2023 The JAX Authors.
|
|
#
|
|
# Licensed under the Apache License, Version 2.0 (the "License");
|
|
# you may not use this file except in compliance with the License.
|
|
# You may obtain a copy of the License at
|
|
#
|
|
# https://www.apache.org/licenses/LICENSE-2.0
|
|
#
|
|
# Unless required by applicable law or agreed to in writing, software
|
|
# distributed under the License is distributed on an "AS IS" BASIS,
|
|
# WITHOUT WARRANTIES OR CONDITIONS OF ANY KIND, either express or implied.
|
|
# See the License for the specific language governing permissions and
|
|
# limitations under the License.
|
|
"""Sharding utilities"""
|
|
|
|
from __future__ import annotations
|
|
|
|
from collections.abc import Sequence
|
|
import itertools
|
|
from typing import Union
|
|
|
|
import numpy as np
|
|
|
|
from jax._src.lib import xla_client as xc
|
|
|
|
|
|
def get_num_ways_dim_sharded(
|
|
hlo_sharding: xc.HloSharding) -> tuple[list[int], int]:
|
|
if hlo_sharding.is_replicated(): # type: ignore
|
|
return [], 1
|
|
partitions = hlo_sharding.tile_assignment_dimensions()
|
|
subgroup_types = hlo_sharding.subgroup_types()
|
|
|
|
if subgroup_types == [xc.OpSharding.Type.REPLICATED]:
|
|
replicate_on_last_tile_dim = True
|
|
else:
|
|
replicate_on_last_tile_dim = hlo_sharding.replicate_on_last_tile_dim()
|
|
if subgroup_types:
|
|
raise NotImplementedError(
|
|
"Unhandled OpSharding type. Please open a bug report!")
|
|
num_replicas = 1
|
|
if replicate_on_last_tile_dim:
|
|
num_replicas = partitions[-1]
|
|
partitions = partitions[:-1]
|
|
return list(partitions), num_replicas
|
|
|
|
|
|
def is_op_sharding_replicated(op: xc.OpSharding | xc.HloSharding) -> bool:
|
|
if isinstance(op, xc.OpSharding):
|
|
op = xc.HloSharding.from_proto(op)
|
|
if op.num_devices() == 1:
|
|
return True
|
|
return op.is_replicated() # type: ignore
|
|
|
|
|
|
def are_op_shardings_equal(op1: xc.OpSharding | xc.HloSharding,
|
|
op2: xc.OpSharding | xc.HloSharding) -> bool:
|
|
if id(op1) == id(op2):
|
|
return True
|
|
if is_op_sharding_replicated(op1) and is_op_sharding_replicated(op2):
|
|
return True
|
|
hc1 = xc.HloSharding.from_proto(op1) if isinstance(op1, xc.OpSharding) else op1
|
|
hc2 = xc.HloSharding.from_proto(op2) if isinstance(op2, xc.OpSharding) else op2
|
|
return hc1 == hc2
|
|
|
|
|
|
_Index = Union[int, slice, tuple[Union[int, slice], ...]]
|
|
|
|
|
|
def op_sharding_to_numpy_indices(
|
|
hlo_sharding: xc.HloSharding, shape: Sequence[int],
|
|
num_devices: int) -> np.ndarray:
|
|
indices = np.empty(num_devices, dtype=np.object_)
|
|
|
|
# num_devices is required as an argument when hlo_sharding is
|
|
# REPLICATED. `jax.device_count()` cannot be used because you can create
|
|
# an opsharding with less number of devices than `jax.device_count()`.
|
|
if is_op_sharding_replicated(hlo_sharding):
|
|
indices.fill((slice(None),) * len(shape))
|
|
return indices
|
|
|
|
assert num_devices == hlo_sharding.num_devices()
|
|
|
|
partitions, num_replicas = get_num_ways_dim_sharded(hlo_sharding)
|
|
assert len(partitions) == len(shape), (len(partitions), len(shape))
|
|
|
|
axis_indices: list[Sequence[_Index]] = []
|
|
for dim, n_shards in zip(shape, partitions):
|
|
if n_shards == 1:
|
|
axis_indices.append([slice(None)])
|
|
elif n_shards > 1:
|
|
shard_size, ragged = divmod(dim, n_shards)
|
|
assert not ragged, (dim, n_shards)
|
|
axis_indices.append([slice(i * shard_size, (i + 1) * shard_size)
|
|
for i in range(n_shards)])
|
|
else:
|
|
raise AssertionError('Unrecognized number of shards. Please file a bug!')
|
|
|
|
device_it = iter(hlo_sharding.tile_assignment_devices())
|
|
|
|
for i, idxs in enumerate(itertools.product(*axis_indices)):
|
|
for _ in range(num_replicas):
|
|
indices[next(device_it)] = idxs
|
|
return indices
|
|
|
|
|
|
def op_sharding_to_indices(
|
|
op_sharding: xc.HloSharding, shape: Sequence[int],
|
|
num_devices: int) -> tuple[tuple[slice, ...], ...]:
|
|
indices = op_sharding_to_numpy_indices(op_sharding, shape, num_devices)
|
|
return tuple(indices.flat)
|