mirror of
https://github.com/ROCm/jax.git
synced 2025-04-17 12:26:07 +00:00
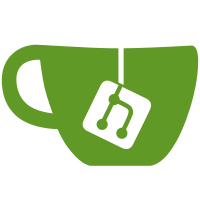
The longer term goal here is to move away from having the config object as part of the public API and migrate towards module-level functions instead. Note that we can preserve the dynamic attribute lookup behavior of the config object via a module-level `__getattr__`
183 lines
6.5 KiB
Python
183 lines
6.5 KiB
Python
# Copyright 2022 The JAX Authors.
|
|
#
|
|
# Licensed under the Apache License, Version 2.0 (the "License");
|
|
# you may not use this file except in compliance with the License.
|
|
# You may obtain a copy of the License at
|
|
#
|
|
# https://www.apache.org/licenses/LICENSE-2.0
|
|
#
|
|
# Unless required by applicable law or agreed to in writing, software
|
|
# distributed under the License is distributed on an "AS IS" BASIS,
|
|
# WITHOUT WARRANTIES OR CONDITIONS OF ANY KIND, either express or implied.
|
|
# See the License for the specific language governing permissions and
|
|
# limitations under the License.
|
|
from functools import partial
|
|
from absl.testing import absltest
|
|
import numpy as np
|
|
import jax
|
|
import jax.numpy as jnp
|
|
from jax._src import config
|
|
from jax._src import test_util as jtu
|
|
from jax.experimental import rnn
|
|
|
|
config.parse_flags_with_absl()
|
|
|
|
|
|
@jtu.with_config(jax_legacy_prng_key='allow')
|
|
class RnnTest(jtu.JaxTestCase):
|
|
|
|
@jtu.sample_product(
|
|
batch_size=[1, 4],
|
|
seq_len=[1, 4],
|
|
input_size=[1, 2],
|
|
hidden_size=[1, 6],
|
|
num_layers=[1, 4],
|
|
bidirectional=[True, False],
|
|
)
|
|
@jtu.run_on_devices("cuda")
|
|
@jax.default_matmul_precision("float32")
|
|
def test_lstm(self, batch_size: int, seq_len: int, input_size: int,
|
|
hidden_size: int, num_layers: int, bidirectional: bool):
|
|
# TODO(phawkins): Partially disable this on cudnn version per b/281071013
|
|
if (batch_size == 1 and seq_len == 4 and input_size == 1 and
|
|
hidden_size == 6 and num_layers == 4 and bidirectional == False):
|
|
self.skipTest("Test requires cudnn >= 8.8")
|
|
|
|
num_directions = 2 if bidirectional else 1
|
|
seq_length_key, root_key = jax.random.split(jax.random.PRNGKey(0))
|
|
|
|
seq_lengths = jax.random.randint(
|
|
seq_length_key, (batch_size,), 1, seq_len, dtype=jnp.int32)
|
|
|
|
k1, k2, k3, k4 = jax.random.split(root_key, 4)
|
|
x = jax.random.normal(
|
|
k1, (batch_size, seq_len, input_size), dtype=jnp.float32)
|
|
h_0 = jax.random.normal(
|
|
k2, (num_directions * num_layers, batch_size, hidden_size),
|
|
dtype=jnp.float32)
|
|
c_0 = jax.random.normal(
|
|
k3, (num_directions * num_layers, batch_size, hidden_size),
|
|
dtype=jnp.float32)
|
|
weights = rnn.init_lstm_weight(k4, input_size, hidden_size, num_layers,
|
|
bidirectional)
|
|
def f(weights, x, h_0, c_0):
|
|
y, h, c = rnn.lstm(
|
|
x,
|
|
h_0,
|
|
c_0,
|
|
weights,
|
|
seq_lengths=seq_lengths,
|
|
input_size=input_size,
|
|
hidden_size=hidden_size,
|
|
num_layers=num_layers,
|
|
dropout=False,
|
|
bidirectional=bidirectional)
|
|
seq_length_mask = jnp.tile(jnp.arange(seq_len, dtype=jnp.int32)[None],
|
|
[batch_size, 1]) < seq_lengths[:, None]
|
|
loss = jnp.sum(jnp.where(seq_length_mask[..., None], y, 0.))
|
|
return loss, (y, h, c)
|
|
|
|
jtu.check_grads(f, (weights, x, h_0, c_0), modes=["rev"], order=1, atol=5E-3, rtol=5E-3)
|
|
|
|
(loss, (y, h_n, c_n)), weights_grad = jax.value_and_grad(f, has_aux=True)(
|
|
weights, x, h_0, c_0)
|
|
|
|
def g(weights, x, h_0, c_0):
|
|
W_ih, W_hh, b_ih, b_hh = rnn.unpack_lstm_weights(weights, input_size,
|
|
hidden_size, num_layers,
|
|
bidirectional)
|
|
y_ref, h_n_ref, c_n_ref = rnn.lstm_ref(
|
|
x,
|
|
h_0,
|
|
c_0,
|
|
W_ih,
|
|
W_hh,
|
|
b_ih,
|
|
b_hh,
|
|
seq_lengths=seq_lengths,
|
|
input_size=input_size,
|
|
hidden_size=hidden_size,
|
|
num_layers=num_layers,
|
|
dropout=False,
|
|
bidirectional=bidirectional)
|
|
seq_length_mask = jnp.tile(jnp.arange(seq_len, dtype=jnp.int32)[None],
|
|
[batch_size, 1]) < seq_lengths[:, None]
|
|
loss = jnp.sum(jnp.where(seq_length_mask[..., None], y_ref, 0.))
|
|
return loss, (y_ref, h_n_ref, c_n_ref)
|
|
|
|
(loss_ref, (y_ref, h_n_ref, c_n_ref)), weights_grad_ref = (
|
|
jax.value_and_grad(g, has_aux=True)(weights, x, h_0, c_0))
|
|
|
|
self.assertAllClose(weights_grad_ref, weights_grad, rtol=1e-5, atol=1e-5)
|
|
np.testing.assert_allclose(loss_ref, loss, rtol=1e-05, atol=1e-5)
|
|
np.testing.assert_allclose(y_ref, y, rtol=1e-05, atol=1e-5)
|
|
np.testing.assert_allclose(h_n_ref, h_n, rtol=1e-05, atol=1e-5)
|
|
np.testing.assert_allclose(c_n_ref, c_n, rtol=1e-05, atol=1e-5)
|
|
|
|
@jtu.sample_product(
|
|
batch_size=[1, 4],
|
|
seq_len=[1, 4],
|
|
input_size=[1, 2],
|
|
hidden_size=[1, 6],
|
|
num_layers=[1, 4],
|
|
bidirectional=[True, False],
|
|
)
|
|
def test_lstm_ref(self, batch_size: int, seq_len: int, input_size: int,
|
|
hidden_size: int, num_layers: int, bidirectional: bool):
|
|
|
|
num_directions = 2 if bidirectional else 1
|
|
|
|
seq_lengths = jax.random.randint(
|
|
jax.random.PRNGKey(0), (batch_size,), 0, seq_len, dtype=jnp.int32)
|
|
|
|
root_key = jax.random.PRNGKey(1)
|
|
k1, k2, k3, k4 = jax.random.split(root_key, 4)
|
|
x = jax.random.normal(
|
|
k1, (batch_size, seq_len, input_size), dtype=jnp.float32)
|
|
h_0 = jax.random.normal(
|
|
k2, (num_directions * num_layers, batch_size, hidden_size),
|
|
dtype=jnp.float32)
|
|
c_0 = jax.random.normal(
|
|
k3, (num_directions * num_layers, batch_size, hidden_size),
|
|
dtype=jnp.float32)
|
|
weights = rnn.init_lstm_weight(k4, input_size, hidden_size, num_layers,
|
|
bidirectional)
|
|
|
|
@partial(jax.value_and_grad, has_aux=True)
|
|
def f(weights, x, h_0, c_0):
|
|
W_ih, W_hh, b_ih, b_hh = rnn.unpack_lstm_weights(weights, input_size,
|
|
hidden_size, num_layers,
|
|
bidirectional)
|
|
y_ref, h_n_ref, c_n_ref = rnn.lstm_ref(
|
|
x,
|
|
h_0,
|
|
c_0,
|
|
W_ih,
|
|
W_hh,
|
|
b_ih,
|
|
b_hh,
|
|
seq_lengths=seq_lengths,
|
|
input_size=input_size,
|
|
hidden_size=hidden_size,
|
|
num_layers=num_layers,
|
|
dropout=False,
|
|
bidirectional=bidirectional)
|
|
|
|
loss = jnp.sum(y_ref)
|
|
|
|
return loss, (y_ref, h_n_ref, c_n_ref)
|
|
|
|
(loss_ref, (y_ref, h_n_ref, c_n_ref)), grad_ref = f(weights, x, h_0, c_0)
|
|
|
|
self.assertFalse(np.isnan(loss_ref))
|
|
self.assertFalse(np.isnan(grad_ref).any())
|
|
|
|
self.assertEqual(y_ref.shape, (batch_size, seq_len, num_directions * hidden_size))
|
|
|
|
for i in range(batch_size):
|
|
y_padded = y_ref[i, seq_lengths[i]:]
|
|
np.testing.assert_allclose(y_padded, jnp.zeros_like(y_padded))
|
|
|
|
if __name__ == '__main__':
|
|
absltest.main(testLoader=jtu.JaxTestLoader())
|