mirror of
https://github.com/ROCm/jax.git
synced 2025-04-16 03:46:06 +00:00
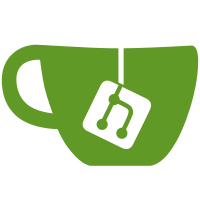
* add more optimizers numerical tests * update examples and readme with new optimziers api * add device_values parameter to xla_call * change optimizers.py to flatten trees and subtrees * remove tree_map2, tree_multimap2, tree_mimomap, tree_prefixmap * add optimizer tests: DeviceTuples and error msgs * make the device_values arg to jit private
600 lines
22 KiB
Python
600 lines
22 KiB
Python
# Copyright 2018 Google LLC
|
|
#
|
|
# Licensed under the Apache License, Version 2.0 (the "License");
|
|
# you may not use this file except in compliance with the License.
|
|
# You may obtain a copy of the License at
|
|
#
|
|
# https://www.apache.org/licenses/LICENSE-2.0
|
|
#
|
|
# Unless required by applicable law or agreed to in writing, software
|
|
# distributed under the License is distributed on an "AS IS" BASIS,
|
|
# WITHOUT WARRANTIES OR CONDITIONS OF ANY KIND, either express or implied.
|
|
# See the License for the specific language governing permissions and
|
|
# limitations under the License.
|
|
|
|
from __future__ import absolute_import
|
|
from __future__ import division
|
|
from __future__ import print_function
|
|
|
|
from collections import namedtuple, defaultdict
|
|
from distutils.util import strtobool
|
|
import itertools as it
|
|
import operator as op
|
|
import os
|
|
|
|
import numpy as onp
|
|
import six
|
|
from six.moves import xrange
|
|
|
|
from ..config import flags
|
|
from .. import core
|
|
from .. import ad_util
|
|
from .. import tree_util
|
|
from .. import linear_util as lu
|
|
from ..abstract_arrays import ConcreteArray, ShapedArray, make_shaped_array, array_types
|
|
from ..core import AbstractTuple, JaxTuple, pack, valid_jaxtype
|
|
from ..util import partial, partialmethod, memoize, unzip2, concatenate, safe_map, prod
|
|
from ..lib import xla_bridge as xb
|
|
from . import partial_eval as pe
|
|
from . import ad
|
|
|
|
FLAGS = flags.FLAGS
|
|
flags.DEFINE_bool('jax_device_values',
|
|
strtobool(os.getenv('JAX_DEVICE_VALUES', "True")),
|
|
'Enable device-persistent values.')
|
|
flags.DEFINE_bool('jax_debug_nans',
|
|
strtobool(os.getenv('JAX_DEBUG_NANS', "False")),
|
|
'Add nan checks to every operation.')
|
|
|
|
def apply_primitive(prim, *args, **kwargs):
|
|
abstract_args = map(abstractify, args)
|
|
compiled_fun = xla_primitive_callable(prim, *abstract_args, **kwargs)
|
|
return compiled_fun(*args)
|
|
|
|
@memoize
|
|
def xla_primitive_callable(prim, *abstract_args, **kwargs):
|
|
shapes = tuple(map(xla_shape, abstract_args))
|
|
built_c = primitive_computation(prim, *shapes, **kwargs)
|
|
result_shape = xla_shape_to_result_shape(built_c.GetReturnValueShape())
|
|
handle_result = result_handler(result_shape)
|
|
compiled = built_c.Compile(shapes, xb.get_compile_options(),
|
|
backend=xb.get_backend())
|
|
return partial(execute_compiled_primitive, prim.name, compiled, handle_result)
|
|
|
|
@memoize
|
|
def primitive_computation(prim, *shapes, **kwargs):
|
|
c = xb.make_computation_builder("primitive_computation")
|
|
xla_args = map(c.ParameterWithShape, shapes)
|
|
xla_result = translation_rule(prim)(c, *xla_args, **kwargs)
|
|
try:
|
|
return c.Build()
|
|
except RuntimeError as e:
|
|
# try for a better error message by using the abstract_eval checks
|
|
prim.abstract_eval(*map(aval_from_xla_shape, shapes), **kwargs)
|
|
raise e
|
|
|
|
def aval_from_xla_shape(shape):
|
|
if shape.is_tuple():
|
|
return AbstractTuple(map(aval_from_xla_shape, shape.tuple_shapes()))
|
|
else:
|
|
return ShapedArray(shape.dimensions(), shape.element_type())
|
|
|
|
def execute_compiled_primitive(name, compiled, result_handler, *args):
|
|
input_bufs = [device_put(x) for x in args]
|
|
out_buf = compiled.Execute(input_bufs, not core.skip_checks)
|
|
check_nans(name, out_buf)
|
|
return result_handler(out_buf)
|
|
|
|
def check_nans(name, buf):
|
|
FLAGS.jax_debug_nans and _check_nans(name, buf.shape(), buf)
|
|
|
|
def _check_nans(name, xla_shape, buf):
|
|
if xla_shape.is_tuple():
|
|
_map(partial(_check_nans, name), xla_shape.tuple_shapes(), buf.destructure())
|
|
else:
|
|
if onp.issubdtype(xla_shape.element_type(), onp.floating):
|
|
pyval = buf.to_py()
|
|
if onp.any(onp.isnan(pyval)):
|
|
msg = "invalid value (nan) encountered in {}"
|
|
raise FloatingPointError(msg.format(name))
|
|
|
|
def device_put(x, device_num=0):
|
|
"""Place a Python value `x` on device number `device_num`.
|
|
|
|
This is a wrapper around jax.lib.xla_bridge.device_put to handle
|
|
additional Python types, namely
|
|
1. the array-like types DeviceArray (which is already backed by device
|
|
memory, though may be on the wrong device) and its subclass DeviceConstant
|
|
(which represents a lazy value to be instantiated), and
|
|
2. the tuple-like types DeviceTuple (which is already backed by device
|
|
memory, though may be on the wrong device) and JaxTuple (which may have some
|
|
elements that are backed by device memory on the correct device).
|
|
In particular, this function avoids transferring data already placed on the
|
|
correct device, and handles instantiating DeviceConstants.
|
|
|
|
Args:
|
|
x: a tuplelike-tree with arraylike leaves representing the value to be
|
|
transferred to the device, where tuplelike means a JaxTuple or
|
|
DeviceTuple, and arraylike includes DeviceArray, DeviceConstant, and
|
|
anything that has an '__array__' attr.
|
|
device_num: an int representing the target physical device number.
|
|
|
|
Returns:
|
|
A buffer representing the input `x` placed on the appropriate device.
|
|
"""
|
|
x = canonicalize_pyval_dtype(x)
|
|
t = type(x)
|
|
if t is DeviceArray or t is DeviceTuple:
|
|
if x.device_buffer.device() == device_num:
|
|
return x.device_buffer
|
|
else:
|
|
# TODO(phawkins): perform a direct device-to-device copy rather than
|
|
# bouncing via the host.
|
|
return device_put(x.device_buffer.to_py(), device_num)
|
|
elif isinstance(x, DeviceConstant):
|
|
return instantiate_device_constant(x, device_num=device_num)
|
|
elif isinstance(x, (DeviceArray, onp.ndarray)):
|
|
return xb.device_put(x, device_num) # handle arraylikes
|
|
elif isinstance(x, JaxTuple):
|
|
element_bufs = tuple(map(partial(device_put, device_num=device_num), x))
|
|
return xb.make_tuple(element_bufs, device_num)
|
|
else:
|
|
raise TypeError(t)
|
|
|
|
def device_put_many(xs_and_devices):
|
|
"""Place multiple Python values on multiple devices in parallel.
|
|
|
|
This is a wrapper around jax.lib.xla_bridge.device_put_many to handle
|
|
additional Python types. See the docstring for jax.interpreters.xla.device_put
|
|
for more information.
|
|
|
|
Args:
|
|
xs_and_devices: a sequence of (pyval, device_num) pairs in which device_num
|
|
is an int representing the target physical device number and pyval is a
|
|
tuple-like tree with arraylike leaves (see the device_put docstring).
|
|
|
|
Returns:
|
|
A sequence of buffers representing the inputs placed on the corresponding
|
|
device numbers.
|
|
"""
|
|
transfer_indices = []
|
|
transfers = []
|
|
outputs = [None] * len(xs_and_devices)
|
|
for i, (x, device_num) in enumerate(xs_and_devices):
|
|
x = canonicalize_pyval_dtype(x)
|
|
t = type(x)
|
|
if t is DeviceArray or t is DeviceTuple:
|
|
if x.device_buffer.device() == device_num:
|
|
outputs[i] = x.device_buffer
|
|
else:
|
|
transfer_indices.append(i)
|
|
# TODO(phawkins): perform a direct device-to-device copy rather than
|
|
# bouncing via the host.
|
|
transfers.append((x.device_buffer.to_py(), device_num))
|
|
elif isinstance(x, DeviceConstant):
|
|
outputs[i] = instantiate_device_constant(x, device_num=device_num)
|
|
elif hasattr(t, '__array__'):
|
|
transfer_indices.append(i)
|
|
transfers.append((x, device_num)) # handle arraylikes
|
|
elif t is JaxTuple:
|
|
# TODO(mattjj,phawkins): improve this to avoid device_put call
|
|
element_bufs = tuple(map(partial(device_put, device_num=device_num), x))
|
|
outputs[i] = xb.make_tuple(element_bufs, device_num)
|
|
else:
|
|
raise TypeError(t)
|
|
|
|
transfer_results = xb.device_put_many(transfers)
|
|
for i, result in zip(transfer_indices, transfer_results):
|
|
outputs[i] = result
|
|
return outputs
|
|
|
|
|
|
# When we execute an XLA computation, we get a raw device buffer back and need
|
|
# to package it into a suitable Python object to return to the user. To avoid
|
|
# unnecessary device-to-host transfers, we typically return a DeviceValue that
|
|
# acts just like a familiar Python type (e.g. an ndarray or JaxTuple) but is
|
|
# lazy in that it only copies data back to the host as required. Since the same
|
|
# DeviceValue type is formed on every execution of a compiled computation, at
|
|
# compile time we set up result handler functions and thus avoid redoing some of
|
|
# the Python bookkeeping work on every execution. Since XLA shapes are slower to
|
|
# manipulate than simple Python builtins, we store the metadata required for
|
|
# forming the DeviceValue result in special ResultArray / ResultTuple classes.
|
|
|
|
# Every JaxType needs to map to an XLA type. However this function's design is
|
|
# based on the assumption that XLA types can be mapped uniquely back to a
|
|
# JaxType, i.e. that the mapping is bijective. That assumption could be relaxed,
|
|
# but it would mean we need to do a bit more bookkeping on the Python side to
|
|
# track abstract values of outputs.
|
|
def xla_shape_to_result_shape(xla_shape):
|
|
if xla_shape.is_tuple():
|
|
aval = aval_from_xla_shape(xla_shape)
|
|
result_shapes = tuple(map(xla_shape_to_result_shape, xla_shape.tuple_shapes()))
|
|
return ResultTuple((aval, result_shapes))
|
|
else:
|
|
shape, dtype = xla_shape.dimensions(), xla_shape.element_type()
|
|
ndim, size = len(shape), prod(shape)
|
|
return ResultArray((shape, dtype, ndim, size))
|
|
class ResultTuple(tuple): pass
|
|
class ResultArray(tuple): pass
|
|
|
|
def result_handler(result_shape):
|
|
if FLAGS.jax_device_values:
|
|
return device_persistent_result_handler(result_shape)
|
|
else:
|
|
return pyval_result_handler(result_shape)
|
|
|
|
def device_persistent_result_handler(result_shape):
|
|
t = type(result_shape)
|
|
if t is ResultArray:
|
|
return partial(DeviceArray, result_shape)
|
|
elif t is ResultTuple:
|
|
return partial(DeviceTuple, result_shape)
|
|
else:
|
|
raise TypeError(t)
|
|
|
|
def pyval_result_handler(result_shape):
|
|
t = type(result_shape)
|
|
if t is ResultArray:
|
|
return lambda buf: buf.to_py()
|
|
elif t is ResultTuple:
|
|
_, result_shapes = result_shape
|
|
handlers = list(map(pyval_result_handler, result_shapes))
|
|
return lambda buf: JaxTuple(h(b) for h, b in zip(handlers, buf.destructure()))
|
|
else:
|
|
raise TypeError(t)
|
|
|
|
|
|
def compile_jaxpr(jaxpr, const_vals, *abstract_args):
|
|
arg_shapes = list(map(xla_shape, abstract_args))
|
|
built_c = jaxpr_computation(jaxpr, const_vals, (), *arg_shapes)
|
|
result_shape = xla_shape_to_result_shape(built_c.GetReturnValueShape())
|
|
return built_c.Compile(arg_shapes, xb.get_compile_options(),
|
|
backend=xb.get_backend()), result_shape
|
|
|
|
def build_jaxpr(jaxpr, const_vals, *abstract_args):
|
|
arg_shapes = list(map(xla_shape, abstract_args))
|
|
built_c = jaxpr_computation(jaxpr, const_vals, (), *arg_shapes)
|
|
return built_c
|
|
|
|
def jaxpr_computation(jaxpr, const_vals, freevar_shapes, *arg_shapes):
|
|
c = xb.make_computation_builder("jaxpr_computation")
|
|
|
|
def read(v):
|
|
return env[v]
|
|
|
|
def write(v, node):
|
|
assert node is not None
|
|
env[v] = node
|
|
|
|
env = {}
|
|
consts_env = dict(zip(jaxpr.constvars, const_vals))
|
|
write(core.unitvar, c.Tuple())
|
|
if const_vals:
|
|
_map(write, jaxpr.constvars, map(c.Constant, const_vals))
|
|
_map(write, jaxpr.freevars, map(c.ParameterWithShape, freevar_shapes))
|
|
else:
|
|
all_freevars = it.chain(jaxpr.constvars, jaxpr.freevars)
|
|
_map(write, all_freevars, map(c.ParameterWithShape, freevar_shapes))
|
|
_map(write, jaxpr.invars, map(c.ParameterWithShape, arg_shapes))
|
|
for eqn in jaxpr.eqns:
|
|
in_nodes = list(map(read, eqn.invars))
|
|
subcs = [
|
|
jaxpr_computation(
|
|
subjaxpr, (),
|
|
tuple(map(c.GetShape, map(read, const_bindings + freevar_bindings))),
|
|
*map(c.GetShape, in_nodes))
|
|
for subjaxpr, const_bindings, freevar_bindings in eqn.bound_subjaxprs]
|
|
subfuns = [(subc, tuple(map(read, const_bindings + freevar_bindings)))
|
|
for subc, (_, const_bindings, freevar_bindings)
|
|
in zip(subcs, eqn.bound_subjaxprs)]
|
|
ans = translation_rule(eqn.primitive)(c, *(subfuns + in_nodes), **eqn.params)
|
|
out_nodes = xla_destructure(c, ans) if eqn.destructure else [ans]
|
|
_map(write, eqn.outvars, out_nodes)
|
|
return c.Build(read(jaxpr.outvar))
|
|
|
|
def _map(f, *xs):
|
|
return tuple(map(f, *xs))
|
|
|
|
def xla_destructure(c, ans):
|
|
num_elements = len(c.GetShape(ans).tuple_shapes())
|
|
return [c.GetTupleElement(ans, i) for i in range(num_elements)]
|
|
|
|
def tuple_constant(c, val, canonicalize_types=True):
|
|
return c.Tuple(*map(c.Constant, val))
|
|
xb.register_constant_handler(JaxTuple, tuple_constant)
|
|
|
|
def translation_rule(p):
|
|
backend = xb.get_backend()
|
|
backend_specific_rule = backend_specific_translations[backend.platform].get(p)
|
|
try:
|
|
return backend_specific_rule or translations[p]
|
|
except KeyError:
|
|
raise NotImplementedError(
|
|
"XLA translation rule for '{}' not implemented".format(p))
|
|
|
|
|
|
def lower_fun(fun, c, *xla_args, **params):
|
|
xla_shapes = tuple(map(c.GetShape, xla_args))
|
|
avals = map(aval_from_xla_shape, xla_shapes)
|
|
pvals = [pe.PartialVal((a, core.unit)) for a in avals]
|
|
jaxpr, _, consts = pe.trace_unwrapped_to_jaxpr(fun, pvals, **params)
|
|
built_c = jaxpr_computation(jaxpr, consts, (), *xla_shapes)
|
|
return c.Call(built_c, xla_args)
|
|
|
|
|
|
translations = {}
|
|
backend_specific_translations = defaultdict(dict)
|
|
|
|
translations[core.pack_p] = lambda c, *xs: c.Tuple(*xs)
|
|
translations[core.call_p] = lambda c, subc_a1, *a2: c.Call(subc_a1[0],
|
|
subc_a1[1] + a2)
|
|
translations[core.identity_p] = lambda c, x: x
|
|
|
|
def zeros_like_translation_rule(c, x):
|
|
def _zeros_like(shape):
|
|
if shape.is_tuple():
|
|
return c.Tuple(*(_zeros_like(x) for x in shape.tuple_shapes()))
|
|
else:
|
|
return c.Broadcast(c.Constant(onp.array(0, shape.element_type())),
|
|
shape.dimensions())
|
|
return _zeros_like(c.GetShape(x))
|
|
translations[ad_util.zeros_like_p] = zeros_like_translation_rule
|
|
|
|
# TODO(mattjj): add_jaxvals should handle any jaxval
|
|
translations[ad_util.add_jaxvals_p] = lambda c, x, y: c.Add(x, y)
|
|
|
|
|
|
def canonicalize_pyval_dtype(x):
|
|
try:
|
|
return canonicalize_dtype_handlers[type(x)](x)
|
|
except KeyError:
|
|
msg = "No canonicalize handler registered for type: {}"
|
|
raise TypeError(msg.format(type(x)))
|
|
|
|
canonicalize_dtype_handlers = {}
|
|
|
|
def canonicalize_tuple_dtype(tup):
|
|
return JaxTuple(map(canonicalize_pyval_dtype, tup))
|
|
canonicalize_dtype_handlers[JaxTuple] = canonicalize_tuple_dtype
|
|
|
|
def canonicalize_ndarray_dtype(x):
|
|
return onp.asarray(x, xb.canonicalize_dtype(onp.result_type(x)))
|
|
|
|
for t in array_types:
|
|
canonicalize_dtype_handlers[t] = canonicalize_ndarray_dtype
|
|
|
|
def identity(x): return x
|
|
|
|
|
|
def abstractify(x):
|
|
try:
|
|
return pytype_aval_mappings[type(x)](x)
|
|
except KeyError:
|
|
raise TypeError("No abstraction handler for type: {}".format(type(x)))
|
|
|
|
pytype_aval_mappings = {}
|
|
|
|
def abstractify_tuple(tup):
|
|
return AbstractTuple(map(abstractify, tup))
|
|
pytype_aval_mappings[JaxTuple] = abstractify_tuple
|
|
pytype_aval_mappings[AbstractTuple] = abstractify_tuple
|
|
|
|
for t in array_types:
|
|
pytype_aval_mappings[t] = make_shaped_array
|
|
|
|
|
|
class DeviceValue(object):
|
|
"""A DeviceValue represents a value backed by device memory."""
|
|
__slots__ = ["device_buffer"]
|
|
def __init__(self, device_buffer):
|
|
self.device_buffer = device_buffer
|
|
|
|
class DeviceTuple(DeviceValue):
|
|
"""A DeviceTuple is a JaxTuple backed by a single device memory buffer."""
|
|
__slots__ = ["aval", "result_shapes"]
|
|
|
|
def __init__(self, result_shape, device_buffer):
|
|
self.device_buffer = device_buffer
|
|
self.aval, self.result_shapes = result_shape
|
|
|
|
def __iter__(self):
|
|
bufs = self.device_buffer.destructure()
|
|
handlers = map(device_persistent_result_handler, self.result_shapes)
|
|
elts = [handler(buf) for handler, buf in zip(handlers, bufs)]
|
|
return iter(elts)
|
|
|
|
def __len__(self):
|
|
return len(self.aval)
|
|
|
|
def __repr__(self):
|
|
return 'DeviceTuple(len={length})'.format(length=len(self))
|
|
|
|
|
|
# DeviceValues don't need to be dtype-canonicalized because we assume values on
|
|
# the device have already been canonicalized.
|
|
core.pytype_aval_mappings[DeviceTuple] = core.pytype_aval_mappings[JaxTuple]
|
|
pytype_aval_mappings[DeviceTuple] = op.attrgetter('aval')
|
|
canonicalize_dtype_handlers[DeviceTuple] = identity
|
|
|
|
|
|
def forward_method(attrname, self, fun, *args):
|
|
return fun(getattr(self, attrname), *args)
|
|
forward_to_value = partial(forward_method, "_value")
|
|
|
|
class DeviceArray(DeviceValue):
|
|
"""A DeviceArray is an ndarray backed by a single device memory buffer."""
|
|
# We don't subclass ndarray because that would open up a host of issues,
|
|
# but lax_numpy.py overrides isinstance behavior and attaches ndarray methods.
|
|
__slots__ = ["shape", "dtype", "ndim", "size", "_npy_value"]
|
|
__array_priority__ = 100.
|
|
|
|
def __init__(self, result_shape, device_buffer):
|
|
self.device_buffer = device_buffer
|
|
self.shape, self.dtype, self.ndim, self.size = result_shape
|
|
self._npy_value = None
|
|
|
|
# TODO make device_buffer a property, make the _npy_value writeable, invalidate
|
|
@property
|
|
def _value(self):
|
|
if self._npy_value is None:
|
|
self._npy_value = self.device_buffer.to_py()
|
|
self._npy_value.flags.writeable = False
|
|
return self._npy_value
|
|
|
|
def copy(self):
|
|
"""Returns an ndarray (backed by host memory, not device memory)."""
|
|
return onp.asarray(self)
|
|
|
|
def __repr__(self):
|
|
return onp.array_repr(self)
|
|
|
|
def item(self):
|
|
if onp.issubdtype(self.dtype, onp.complexfloating):
|
|
return complex(self)
|
|
elif onp.issubdtype(self.dtype, onp.floating):
|
|
return float(self)
|
|
elif onp.issubdtype(self.dtype, onp.integer):
|
|
return int(self)
|
|
elif onp.issubdtype(self.dtype, onp.bool_):
|
|
return bool(self)
|
|
else:
|
|
raise TypeError(self.dtype)
|
|
|
|
def __len__(self):
|
|
try:
|
|
return self.shape[0]
|
|
except IndexError:
|
|
raise TypeError("len() of unsized object") # same as numpy error
|
|
|
|
def __iter__(self):
|
|
if self.ndim == 0:
|
|
raise TypeError("iteration over a 0-d array") # same as numpy error
|
|
else:
|
|
return (self[i] for i in xrange(self.shape[0]))
|
|
|
|
def __reversed__(self):
|
|
if self.ndim == 0:
|
|
raise TypeError("iteration over a 0-d array")
|
|
else:
|
|
return (self[i] for i in xrange(self.shape[0] - 1, -1, -1))
|
|
|
|
def __format__(self, format_spec):
|
|
# Simulates behavior of https://github.com/numpy/numpy/pull/9883
|
|
if self.ndim == 0:
|
|
return format(self._value[()], format_spec)
|
|
else:
|
|
return format(self._value, format_spec)
|
|
|
|
__array__ = partialmethod(forward_to_value, onp.asarray)
|
|
__str__ = partialmethod(forward_to_value, str)
|
|
__bool__ = __nonzero__ = partialmethod(forward_to_value, bool)
|
|
__float__ = partialmethod(forward_to_value, float)
|
|
__int__ = partialmethod(forward_to_value, int)
|
|
if six.PY2:
|
|
__long__ = partialmethod(forward_to_value, long) # noqa: F821
|
|
__complex__ = partialmethod(forward_to_value, complex)
|
|
__hex__ = partialmethod(forward_to_value, hex)
|
|
__oct__ = partialmethod(forward_to_value, oct)
|
|
|
|
# pickle saves and loads just like an ndarray
|
|
__reduce__ = partialmethod(forward_to_value, op.methodcaller("__reduce__"))
|
|
|
|
# clobbered when jax.numpy is imported, but useful in tests
|
|
def __eq__(self, other): return self._value == other
|
|
|
|
def __hash__(self):
|
|
# TODO(mattjj): this is not semantically correct because it is possible
|
|
# __eq__ is true for values with unequal __hash__ values. However, the
|
|
# main use case at the moment is memoization for which false negatives are
|
|
# fine.
|
|
return id(self)
|
|
|
|
|
|
# DeviceValues don't need to be canonicalized because we assume values on the
|
|
# device have already been canonicalized.
|
|
core.pytype_aval_mappings[DeviceArray] = ConcreteArray
|
|
pytype_aval_mappings[DeviceArray] = make_shaped_array
|
|
canonicalize_dtype_handlers[DeviceArray] = identity
|
|
|
|
def _device_array_constant_handler(c, val, canonicalize_types=True):
|
|
return c.Constant(onp.asarray(val), canonicalize_types=canonicalize_types)
|
|
xb.register_constant_handler(DeviceArray, _device_array_constant_handler)
|
|
|
|
pytype_aval_mappings[ConcreteArray] = make_shaped_array
|
|
pytype_aval_mappings[ShapedArray] = identity
|
|
|
|
|
|
class DeviceConstant(DeviceArray):
|
|
@staticmethod
|
|
def constant_handler(c, constant_instance, canonicalize_types=True):
|
|
assert False
|
|
|
|
def instantiate_device_constant(const, cutoff=1e6, device_num=0):
|
|
# dispatch an XLA Computation to build the constant on the device if it's
|
|
# large, or alternatively build it on the host and transfer it if it's small
|
|
# TODO(mattjj): need a way to instantiate on a specific device
|
|
assert isinstance(const, DeviceConstant)
|
|
if const.size > cutoff and device_num == 0:
|
|
c = xb.make_computation_builder("constant_instantiating_computation")
|
|
xla_const = const.constant_handler(c, const)
|
|
compiled = c.Build(xla_const).Compile((), xb.get_compile_options(),
|
|
backend=xb.get_backend())
|
|
return compiled.Execute(())
|
|
else:
|
|
return xb.device_put(onp.asarray(const), device_num)
|
|
|
|
|
|
def xla_shape(x):
|
|
try:
|
|
return xb.Shape.array_shape(x.dtype, x.shape)
|
|
except AttributeError:
|
|
if type(x) in (core.AbstractTuple, core.JaxTuple):
|
|
return xb.Shape.tuple_shape(tuple(map(xla_shape, x)))
|
|
else:
|
|
raise TypeError(type(x))
|
|
|
|
|
|
def xla_call_impl(fun, *args, **params):
|
|
device_values = FLAGS.jax_device_values and params.pop('device_values')
|
|
compiled_fun = xla_callable(fun, device_values, *map(abstractify, args))
|
|
try:
|
|
return compiled_fun(*args)
|
|
except FloatingPointError:
|
|
print("Invalid value encountered in the output of a jit function. "
|
|
"Calling the de-optimized version.")
|
|
return fun.call_wrapped(*args) # probably won't return
|
|
|
|
|
|
@lu.memoize
|
|
def xla_callable(fun, device_values, *abstract_args):
|
|
pvals = [pe.PartialVal((aval, core.unit)) for aval in abstract_args]
|
|
with core.new_master(pe.JaxprTrace, True) as master:
|
|
jaxpr, (pval, consts, env) = pe.trace_to_subjaxpr(fun, master, False).call_wrapped(pvals)
|
|
assert not env # no subtraces here (though cond might eventually need them)
|
|
compiled, result_shape = compile_jaxpr(jaxpr, consts, *abstract_args)
|
|
del master, consts, jaxpr, env
|
|
if device_values:
|
|
handle_result = device_persistent_result_handler(result_shape)
|
|
else:
|
|
handle_result = pyval_result_handler(result_shape)
|
|
return partial(execute_compiled, compiled, pval, handle_result)
|
|
|
|
def execute_compiled(compiled, pval, handle_result, *args):
|
|
input_bufs = [device_put(x) for x in args]
|
|
out_buf = compiled.Execute(input_bufs, not core.skip_checks)
|
|
check_nans("jit-compiled computation", out_buf)
|
|
return pe.merge_pvals(handle_result(out_buf), pval)
|
|
|
|
|
|
def xla_call_translation_rule(c, subc_a1, *a2, **params):
|
|
subc, a1 = subc_a1
|
|
return c.Call(subc, a1 + a2)
|
|
|
|
xla_call_p = core.Primitive('xla_call')
|
|
xla_call = partial(core.call_bind, xla_call_p)
|
|
xla_call_p.def_custom_bind(xla_call)
|
|
xla_call_p.def_impl(xla_call_impl)
|
|
|
|
translations[xla_call_p] = xla_call_translation_rule
|
|
ad.primitive_transposes[xla_call_p] = partial(ad.call_transpose, xla_call_p)
|