mirror of
https://github.com/ROCm/jax.git
synced 2025-04-17 04:16:07 +00:00
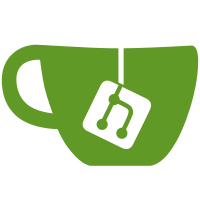
The goal of this change is to catch PRs that introduce new warnings sooner. To help pass the environment variable more easily, rename the jax_test Bazel test macro to jax_multiplatform_test, and introduce a new jax_py_test macro that wraps py_test. Add code to both to set the environment variable. Add code to suppress some new warnings uncovered in CI. PiperOrigin-RevId: 678352286
328 lines
11 KiB
Python
328 lines
11 KiB
Python
# Copyright 2020 The JAX Authors.
|
|
#
|
|
# Licensed under the Apache License, Version 2.0 (the "License");
|
|
# you may not use this file except in compliance with the License.
|
|
# You may obtain a copy of the License at
|
|
#
|
|
# https://www.apache.org/licenses/LICENSE-2.0
|
|
#
|
|
# Unless required by applicable law or agreed to in writing, software
|
|
# distributed under the License is distributed on an "AS IS" BASIS,
|
|
# WITHOUT WARRANTIES OR CONDITIONS OF ANY KIND, either express or implied.
|
|
# See the License for the specific language governing permissions and
|
|
# limitations under the License.
|
|
"""Definitions of two versions of MNIST (model and training code ).
|
|
|
|
One definition uses pure JAX (for those who prefer an example with fewer
|
|
moving parts, at the expense of code size), and another using Flax.
|
|
|
|
See README.md for how these are used.
|
|
"""
|
|
|
|
from __future__ import annotations
|
|
|
|
from collections.abc import Callable, Sequence
|
|
import functools
|
|
import logging
|
|
import re
|
|
import time
|
|
from typing import Any
|
|
import warnings
|
|
from absl import flags
|
|
|
|
import flax
|
|
from flax import linen as nn
|
|
|
|
import jax
|
|
import jax.numpy as jnp
|
|
|
|
from matplotlib import pyplot as plt
|
|
import numpy as np
|
|
import optax
|
|
import tensorflow as tf
|
|
import tensorflow_datasets as tfds # type: ignore
|
|
|
|
_MOCK_DATA = flags.DEFINE_boolean("mock_data", False,
|
|
"Use fake data, for testing.")
|
|
|
|
#### Model parameters
|
|
|
|
# For fun, let's use different batch sizes for training and for evaluation.
|
|
train_batch_size = 128
|
|
test_batch_size = 16
|
|
|
|
# Define common parameters for both the JAX and the Flax models.
|
|
input_shape = (28, 28, 1) # Excluding batch_size
|
|
layer_sizes = [784, 512, 512, 10] # 10 is the number of classes
|
|
param_scale = 0.1
|
|
step_size = 0.001
|
|
|
|
|
|
def load_mnist(split: tfds.Split, batch_size: int):
|
|
"""Loads either training or test MNIST data.
|
|
|
|
Args:
|
|
split: either tfds.Split.TRAIN or tfds.Split.TEST.
|
|
|
|
Returns:
|
|
an iterator with pairs (images, labels). The images have shape
|
|
(B, 28, 28, 1) and the labels have shape (B, 10), where B is the batch_size.
|
|
"""
|
|
if _MOCK_DATA.value:
|
|
with tfds.testing.mock_data(num_examples=batch_size):
|
|
try:
|
|
with warnings.catch_warnings():
|
|
warnings.simplefilter("ignore")
|
|
ds = tfds.load("mnist", split=split)
|
|
except Exception as e:
|
|
m = re.search(r'metadata files were not found in (.+/)mnist/', str(e))
|
|
if m:
|
|
msg = ("TFDS mock_data is missing the mnist metadata files. Run the "
|
|
"`saved_model_main.py` binary and see where TFDS downloads "
|
|
"the mnist data set (typically ~/tensorflow_datasets/mnist). "
|
|
f"Copy the `mnist` directory to {m.group(1)} and re-run the test")
|
|
raise ValueError(msg) from e
|
|
else:
|
|
raise e
|
|
else:
|
|
ds = tfds.load("mnist", split=split)
|
|
|
|
def _prepare_example(x):
|
|
image = tf.cast(x["image"], tf.float32) / 255.0
|
|
label = tf.one_hot(x["label"], 10)
|
|
return (image, label)
|
|
|
|
ds = ds.map(_prepare_example)
|
|
# drop_remainder=True is important for use with Keras
|
|
ds = ds.cache().shuffle(1000).batch(batch_size, drop_remainder=True)
|
|
return ds
|
|
|
|
|
|
class PureJaxMNIST:
|
|
"""An MNIST model written using pure JAX.
|
|
|
|
There is an option for the model to skip the classifier layer, for
|
|
demonstrating reuse of the classifier-less model into a larger model.
|
|
See README.md.
|
|
"""
|
|
|
|
name = "mnist_pure_jax"
|
|
|
|
@staticmethod
|
|
def predict(params: Sequence[tuple[Any, Any]], inputs, with_classifier=True):
|
|
"""The prediction function.
|
|
|
|
Args:
|
|
params: a list with pairs of weights and biases for each layer.
|
|
inputs: the batch of images (B, 28, 28, 1)
|
|
with_classifier: whether to include the classifier layer.
|
|
|
|
Returns:
|
|
either the predictions (B, 10) if with_classifier=True, or the
|
|
final set of logits of shape (B, 512).
|
|
"""
|
|
x = inputs.reshape((inputs.shape[0], -1)) # flatten to f32[B, 784]
|
|
for w, b in params[:-1]:
|
|
x = jnp.dot(x, w) + b
|
|
x = jnp.tanh(x)
|
|
|
|
if not with_classifier:
|
|
return x
|
|
final_w, final_b = params[-1]
|
|
logits = jnp.dot(x, final_w) + final_b
|
|
return logits - jax.scipy.special.logsumexp(
|
|
logits, axis=1, keepdims=True)
|
|
|
|
@staticmethod
|
|
def loss(params, inputs, labels):
|
|
predictions = PureJaxMNIST.predict(params, inputs, with_classifier=True)
|
|
return -jnp.mean(jnp.sum(predictions * labels, axis=1))
|
|
|
|
@staticmethod
|
|
def accuracy(predict: Callable, params, dataset):
|
|
|
|
@jax.jit
|
|
def _per_batch(inputs, labels):
|
|
target_class = jnp.argmax(labels, axis=1)
|
|
predicted_class = jnp.argmax(predict(params, inputs), axis=1)
|
|
return jnp.mean(predicted_class == target_class)
|
|
|
|
batched = [
|
|
_per_batch(inputs, labels) for inputs, labels in tfds.as_numpy(dataset)
|
|
]
|
|
return jnp.mean(jnp.stack(batched))
|
|
|
|
@staticmethod
|
|
def update(params, inputs, labels):
|
|
grads = jax.grad(PureJaxMNIST.loss)(params, inputs, labels)
|
|
return [(w - step_size * dw, b - step_size * db)
|
|
for (w, b), (dw, db) in zip(params, grads)]
|
|
|
|
@staticmethod
|
|
def train(train_ds, test_ds, num_epochs, with_classifier=True):
|
|
"""Trains a pure JAX MNIST predictor.
|
|
|
|
Returns:
|
|
a tuple with two elements:
|
|
- a predictor function with signature "(Params, ImagesBatch) ->
|
|
Predictions".
|
|
If `with_classifier=False` then the output of the predictor function
|
|
is the last layer of logits.
|
|
- the parameters "Params" for the predictor function
|
|
"""
|
|
rng = jax.random.PRNGKey(0)
|
|
params = [(param_scale * jax.random.normal(rng, (m, n)),
|
|
param_scale * jax.random.normal(rng, (n,)))
|
|
for m, n, in zip(layer_sizes[:-1], layer_sizes[1:])]
|
|
|
|
for epoch in range(num_epochs):
|
|
start_time = time.time()
|
|
for inputs, labels in tfds.as_numpy(train_ds):
|
|
params = jax.jit(PureJaxMNIST.update)(params, inputs, labels)
|
|
epoch_time = time.time() - start_time
|
|
train_acc = PureJaxMNIST.accuracy(PureJaxMNIST.predict, params, train_ds)
|
|
test_acc = PureJaxMNIST.accuracy(PureJaxMNIST.predict, params, test_ds)
|
|
logging.info("%s: Epoch %d in %0.2f sec", PureJaxMNIST.name, epoch,
|
|
epoch_time)
|
|
logging.info("%s: Training set accuracy %0.2f%%", PureJaxMNIST.name,
|
|
100. * train_acc)
|
|
logging.info("%s: Test set accuracy %0.2f%%", PureJaxMNIST.name,
|
|
100. * test_acc)
|
|
|
|
return (functools.partial(
|
|
PureJaxMNIST.predict, with_classifier=with_classifier), params)
|
|
|
|
|
|
class FlaxMNIST:
|
|
"""An MNIST model using Flax."""
|
|
|
|
name = "mnist_flax"
|
|
|
|
class Module(nn.Module):
|
|
"""A simple CNN model for MNIST.
|
|
|
|
There is an option for the model to skip the classifier layer, for
|
|
demonstrating reuse of the classifier-less model into a larger model.
|
|
See README.md.
|
|
"""
|
|
|
|
@nn.compact
|
|
def __call__(self, x, with_classifier=True):
|
|
x = nn.Conv(features=32, kernel_size=(3, 3))(x)
|
|
x = nn.relu(x)
|
|
x = nn.avg_pool(x, window_shape=(2, 2), strides=(2, 2))
|
|
x = nn.Conv(features=64, kernel_size=(3, 3))(x)
|
|
x = nn.relu(x)
|
|
x = nn.avg_pool(x, window_shape=(2, 2), strides=(2, 2))
|
|
x = x.reshape((x.shape[0], -1)) # flatten
|
|
x = nn.Dense(features=256)(x)
|
|
x = nn.relu(x)
|
|
if not with_classifier:
|
|
return x
|
|
x = nn.Dense(features=10)(x)
|
|
x = nn.log_softmax(x)
|
|
return x
|
|
|
|
# Create the model and save it
|
|
model = Module()
|
|
|
|
@staticmethod
|
|
def predict(params, inputs, with_classifier=True):
|
|
return FlaxMNIST.model.apply({"params": params},
|
|
inputs,
|
|
with_classifier=with_classifier)
|
|
|
|
@staticmethod
|
|
def loss(params, inputs, labels): # Same as the pure JAX example
|
|
# Must use the classifier layer because the labels are classes
|
|
predictions = FlaxMNIST.predict(params, inputs, with_classifier=True)
|
|
return -jnp.mean(jnp.sum(predictions * labels, axis=1))
|
|
|
|
@staticmethod
|
|
def update(tx, params, opt_state, inputs, labels):
|
|
grad = jax.grad(FlaxMNIST.loss)(params, inputs, labels)
|
|
updates, opt_state = tx.update(grad, opt_state)
|
|
params = optax.apply_updates(params, updates)
|
|
return params, opt_state
|
|
|
|
@staticmethod
|
|
def train(train_ds, test_ds, num_epochs, with_classifier=True):
|
|
"""Trains a pure JAX MNIST predictor.
|
|
|
|
Returns:
|
|
a tuple with two elements:
|
|
- a predictor function with signature "(Params, ImagesBatch) ->
|
|
Predictions".
|
|
If `with_classifier=False` then the output of the predictor function
|
|
is the last layer of logits.
|
|
- the parameters "Params" for the predictor function
|
|
"""
|
|
rng = jax.random.PRNGKey(0)
|
|
momentum_mass = 0.9
|
|
|
|
init_shape = jnp.ones((1,) + input_shape, jnp.float32)
|
|
params = FlaxMNIST.model.init(rng, init_shape)["params"]
|
|
tx = optax.sgd(learning_rate=step_size, momentum=momentum_mass)
|
|
opt_state = tx.init(params)
|
|
|
|
for epoch in range(num_epochs):
|
|
start_time = time.time()
|
|
for inputs, labels in tfds.as_numpy(train_ds):
|
|
params, opt_state = jax.jit(FlaxMNIST.update,
|
|
static_argnums=0)(tx, params, opt_state,
|
|
inputs, labels)
|
|
epoch_time = time.time() - start_time
|
|
# Same accuracy function as for the pure JAX example
|
|
train_acc = PureJaxMNIST.accuracy(FlaxMNIST.predict, params,
|
|
train_ds)
|
|
test_acc = PureJaxMNIST.accuracy(FlaxMNIST.predict, params,
|
|
test_ds)
|
|
logging.info("%s: Epoch %d in %0.2f sec", FlaxMNIST.name, epoch,
|
|
epoch_time)
|
|
logging.info("%s: Training set accuracy %0.2f%%", FlaxMNIST.name,
|
|
100. * train_acc)
|
|
logging.info("%s: Test set accuracy %0.2f%%", FlaxMNIST.name,
|
|
100. * test_acc)
|
|
|
|
# See discussion in README.md for packaging Flax models for conversion
|
|
predict_fn = functools.partial(FlaxMNIST.predict,
|
|
with_classifier=with_classifier)
|
|
return (predict_fn, params)
|
|
|
|
|
|
def plot_images(ds,
|
|
nr_rows: int,
|
|
nr_cols: int,
|
|
title: str,
|
|
inference_fn: Callable | None = None):
|
|
"""Plots a grid of images with their predictions.
|
|
|
|
Params:
|
|
ds: a tensorflow dataset from where to pick the images and labels.
|
|
nr_rows, nr_cols: the size of the grid to plot
|
|
title: the title of the plot
|
|
inference_fn: if None then print the existing label, else use this function
|
|
on the batch of images to produce a batch of inference results, which
|
|
get printed.
|
|
inference_batch_size: the size of the batch of images passed to
|
|
`inference_fn`.
|
|
"""
|
|
count = nr_rows * nr_cols
|
|
fig = plt.figure(figsize=(8., 4.), num=title)
|
|
# Get the first batch
|
|
(images, labels), = list(tfds.as_numpy(ds.take(1)))
|
|
if inference_fn:
|
|
inferred_labels = inference_fn(images)
|
|
for i, image in enumerate(images[:count]):
|
|
digit = fig.add_subplot(nr_rows, nr_cols, i + 1)
|
|
if inference_fn:
|
|
digit_title = f"infer: {np.argmax(inferred_labels[i])}\n"
|
|
else:
|
|
digit_title = ""
|
|
digit_title += f"label: {np.argmax(labels[i])}"
|
|
digit.set_title(digit_title)
|
|
plt.imshow(
|
|
(np.reshape(image, (28, 28)) * 255).astype(np.uint8),
|
|
interpolation="nearest")
|
|
plt.show()
|