mirror of
https://github.com/ROCm/jax.git
synced 2025-04-15 19:36:06 +00:00
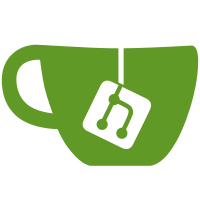
The goal of this change is to catch PRs that introduce new warnings sooner. To help pass the environment variable more easily, rename the jax_test Bazel test macro to jax_multiplatform_test, and introduce a new jax_py_test macro that wraps py_test. Add code to both to set the environment variable. Add code to suppress some new warnings uncovered in CI. PiperOrigin-RevId: 678352286
1958 lines
77 KiB
Python
1958 lines
77 KiB
Python
# Copyright 2021 The JAX Authors.
|
|
#
|
|
# Licensed under the Apache License, Version 2.0 (the "License");
|
|
# you may not use this file except in compliance with the License.
|
|
# You may obtain a copy of the License at
|
|
#
|
|
# https://www.apache.org/licenses/LICENSE-2.0
|
|
#
|
|
# Unless required by applicable law or agreed to in writing, software
|
|
# distributed under the License is distributed on an "AS IS" BASIS,
|
|
# WITHOUT WARRANTIES OR CONDITIONS OF ANY KIND, either express or implied.
|
|
# See the License for the specific language governing permissions and
|
|
# limitations under the License.
|
|
|
|
import contextlib
|
|
from functools import partial
|
|
import itertools
|
|
import math
|
|
import operator
|
|
import random
|
|
import unittest
|
|
|
|
from absl.testing import absltest
|
|
import jax
|
|
from jax import jit
|
|
from jax import lax
|
|
from jax import vmap
|
|
from jax._src import test_util as jtu
|
|
from jax._src import xla_bridge
|
|
from jax._src.lax.lax import remaining
|
|
from jax._src.util import unzip2
|
|
from jax.experimental import sparse
|
|
from jax.experimental.sparse import bcoo as sparse_bcoo
|
|
from jax.experimental.sparse import bcsr as sparse_bcsr
|
|
from jax.experimental.sparse import test_util as sptu
|
|
from jax.experimental.sparse import util as sparse_util
|
|
import jax.numpy as jnp
|
|
import jax.random
|
|
from jax.util import split_list
|
|
import numpy as np
|
|
|
|
jax.config.parse_flags_with_absl()
|
|
|
|
COMPATIBLE_SHAPE_PAIRS = [
|
|
[(), ()],
|
|
[(), (1,)],
|
|
[(3,), (1, 3)],
|
|
[(3, 1), (3,)],
|
|
[(6,), (2, 3)],
|
|
[(3, 2), (6,)],
|
|
[(2, 3), (1, 6)],
|
|
[(2, 4), (4, 1, 2)],
|
|
[(3, 4, 5), (2, 6, 5)],
|
|
[(2,), (2,)],
|
|
]
|
|
|
|
|
|
def _generate_batched_dot_general_properties(
|
|
shapes=((5,), (2, 3), (2, 3, 4), (2, 3, 4, 4)), sparse_format="bcoo"
|
|
) -> sptu.BatchedDotGeneralProperties:
|
|
"""Generator of properties for bcoo_dot_general tests."""
|
|
rng = random.Random(0)
|
|
|
|
if sparse_format not in ['bcoo', 'bcsr']:
|
|
raise ValueError(f"Sparse format {sparse_format} not supported.")
|
|
|
|
for shape in shapes:
|
|
for layout in sptu.iter_sparse_layouts(shape):
|
|
if sparse_format == "bcsr" and layout.n_sparse != 2:
|
|
continue
|
|
subsets = split_list(range(len(shape)), [layout.n_batch, layout.n_sparse])
|
|
for batch_dims in sptu.iter_subsets(range(layout.n_batch)):
|
|
for contracting_dims in sptu.iter_subsets(
|
|
remaining(range(layout.n_batch + layout.n_sparse), batch_dims)
|
|
):
|
|
# We want coverage of permutations without generating hundreds of thousands of test cases;
|
|
# we do this by deterministic pseudo-random sampling instead of iterating.
|
|
rhs_permute = rng.sample(range(len(shape)), len(shape))
|
|
lhs_permute = list(
|
|
itertools.chain.from_iterable(
|
|
rng.sample(subset, len(subset)) for subset in subsets
|
|
)
|
|
)
|
|
yield sptu.BatchedDotGeneralProperties(
|
|
lhs_shape=tuple(shape[p] for p in lhs_permute),
|
|
rhs_shape=tuple(shape[p] for p in rhs_permute),
|
|
n_batch=layout.n_batch,
|
|
n_dense=layout.n_dense,
|
|
dimension_numbers=(
|
|
(
|
|
[lhs_permute.index(d) for d in contracting_dims],
|
|
[rhs_permute.index(d) for d in contracting_dims],
|
|
),
|
|
(
|
|
[lhs_permute.index(d) for d in batch_dims],
|
|
[rhs_permute.index(d) for d in batch_dims],
|
|
),
|
|
),
|
|
)
|
|
|
|
|
|
def _generate_bcoo_dot_general_sampled_properties(
|
|
shapes=((5,), (2, 3), (2, 3, 4), (2, 3, 4, 4))
|
|
) -> sptu.BatchedDotGeneralProperties:
|
|
"""Generator of properties for bcoo_dot_general_sampled tests."""
|
|
rng = random.Random(0)
|
|
|
|
for shape in shapes:
|
|
for batch_dims in sptu.iter_subsets(range(len(shape))):
|
|
for contracting_dims in sptu.iter_subsets(
|
|
remaining(range(len(shape)), batch_dims)
|
|
):
|
|
# We want coverage of permutations without generating hundreds of thousands of test cases;
|
|
# we do this by deterministic pseudo-random sampling instead of iterating.
|
|
lhs_permute = rng.sample(range(len(shape)), len(shape))
|
|
rhs_permute = rng.sample(range(len(shape)), len(shape))
|
|
lhs_shape = tuple(shape[p] for p in lhs_permute)
|
|
rhs_shape = tuple(shape[p] for p in rhs_permute)
|
|
dimension_numbers = (
|
|
(
|
|
[lhs_permute.index(d) for d in contracting_dims],
|
|
[rhs_permute.index(d) for d in contracting_dims],
|
|
),
|
|
(
|
|
[lhs_permute.index(d) for d in batch_dims],
|
|
[rhs_permute.index(d) for d in batch_dims],
|
|
),
|
|
)
|
|
out = jax.eval_shape(partial(lax.dot_general, dimension_numbers=dimension_numbers),
|
|
jax.ShapeDtypeStruct(lhs_shape, 'float32'), jax.ShapeDtypeStruct(rhs_shape, 'float32'))
|
|
for layout in sptu.iter_sparse_layouts(out.shape):
|
|
yield sptu.BatchedDotGeneralProperties(
|
|
lhs_shape=lhs_shape,
|
|
rhs_shape=rhs_shape,
|
|
n_batch=layout.n_batch,
|
|
n_dense=layout.n_dense,
|
|
dimension_numbers=dimension_numbers,
|
|
)
|
|
|
|
|
|
all_dtypes = jtu.dtypes.integer + jtu.dtypes.floating + jtu.dtypes.complex
|
|
|
|
def _is_required_cuda_version_satisfied(cuda_version):
|
|
version = xla_bridge.get_backend().platform_version
|
|
if version == "<unknown>" or "rocm" in version.split():
|
|
return False
|
|
else:
|
|
return int(version.split()[-1]) >= cuda_version
|
|
|
|
class BCOOTest(sptu.SparseTestCase):
|
|
|
|
def gpu_matmul_warning_context(self, msg):
|
|
if jax.config.jax_bcoo_cusparse_lowering:
|
|
return self.assertWarnsRegex(sparse.CuSparseEfficiencyWarning, msg)
|
|
return contextlib.nullcontext()
|
|
|
|
def test_repr(self):
|
|
x = sparse.BCOO.fromdense(jnp.arange(5, dtype='float32'))
|
|
self.assertEqual(repr(x), "BCOO(float32[5], nse=4)")
|
|
|
|
y = sparse.BCOO.fromdense(jnp.arange(6, dtype='float32').reshape(2, 3), n_batch=1)
|
|
self.assertEqual(repr(y), "BCOO(float32[2, 3], nse=3, n_batch=1)")
|
|
|
|
y = sparse.BCOO.fromdense(jnp.arange(6, dtype='float32').reshape(2, 3), n_batch=1, n_dense=1)
|
|
self.assertEqual(repr(y), "BCOO(float32[2, 3], nse=1, n_batch=1, n_dense=1)")
|
|
|
|
M_invalid = sparse.BCOO.fromdense(jnp.arange(6, dtype='float32').reshape(2, 3))
|
|
M_invalid.indices = jnp.array([])
|
|
self.assertEqual(repr(M_invalid), "BCOO(<invalid>)")
|
|
|
|
@jit
|
|
def f(x):
|
|
self.assertEqual(repr(x), "DynamicJaxprTracer[BCOO(float32[5], nse=4)]")
|
|
f(x)
|
|
|
|
@jtu.sample_product(
|
|
[
|
|
dict(shape=shape, n_batch=layout.n_batch, n_dense=layout.n_dense)
|
|
for shape in [(5,), (5, 8), (8, 5), (3, 4, 5), (3, 4, 3, 2)]
|
|
for layout in sptu.iter_sparse_layouts(shape)
|
|
],
|
|
dtype=all_dtypes,
|
|
)
|
|
def test_empty(self, shape, dtype, n_batch, n_dense):
|
|
M = sparse.empty(shape, dtype=dtype, n_batch=n_batch, n_dense=n_dense)
|
|
self.assertIsInstance(M, sparse.BCOO)
|
|
self.assertEqual(M.nse, 0)
|
|
self.assertEqual(M.n_batch, n_batch)
|
|
self.assertEqual(M.n_dense, n_dense)
|
|
self.assertEqual(M.dtype, dtype)
|
|
self.assertArraysEqual(M.todense(), jnp.empty(shape, dtype))
|
|
|
|
@jtu.sample_product(
|
|
[
|
|
dict(n_batch=layout.n_batch, n_dense=layout.n_dense)
|
|
for layout in sptu.iter_sparse_layouts((3, 3))
|
|
],
|
|
N=[3, 5],
|
|
M=[None, 4],
|
|
k=[-3, -1, 0, 2, 4],
|
|
dtype=all_dtypes,
|
|
)
|
|
def test_eye(self, N, M, k, dtype, n_batch, n_dense):
|
|
mat = sparse.eye(N, M, k, dtype=dtype, n_batch=n_batch, n_dense=n_dense)
|
|
expected = jnp.eye(N, M, k, dtype=dtype)
|
|
expected_nse = sparse.BCOO.fromdense(expected, n_batch=n_batch, n_dense=n_dense).nse
|
|
|
|
self.assertIsInstance(mat, sparse.BCOO)
|
|
self.assertEqual(mat.n_batch, n_batch)
|
|
self.assertEqual(mat.n_dense, n_dense)
|
|
self.assertEqual(mat.dtype, dtype)
|
|
self.assertEqual(mat.nse, expected_nse)
|
|
self.assertArraysEqual(mat.todense(), expected)
|
|
|
|
@jtu.sample_product(
|
|
[
|
|
dict(shape=shape, n_batch=layout.n_batch, n_dense=layout.n_dense)
|
|
for shape in [(5,), (5, 8), (8, 5), (3, 4, 5), (3, 4, 3, 2)]
|
|
for layout in sptu.iter_sparse_layouts(shape)
|
|
],
|
|
dtype=all_dtypes,
|
|
)
|
|
def test_bcoo_dense_round_trip(self, shape, dtype, n_batch, n_dense):
|
|
n_sparse = len(shape) - n_batch - n_dense
|
|
rng = sptu.rand_sparse(self.rng())
|
|
M = rng(shape, dtype)
|
|
nse = sparse.util._count_stored_elements(M, n_batch=n_batch, n_dense=n_dense)
|
|
def round_trip(M):
|
|
return sparse.BCOO.fromdense(M, nse=nse, n_batch=n_batch, n_dense=n_dense).todense()
|
|
args_maker = lambda: [M]
|
|
ident = lambda x: x
|
|
|
|
self._CheckAgainstNumpy(ident, round_trip, args_maker)
|
|
self._CompileAndCheck(round_trip, args_maker)
|
|
self._CheckBatchingSparse(ident, round_trip, args_maker, bdims=self._random_bdims(n_batch))
|
|
if jnp.issubdtype(dtype, jnp.floating):
|
|
# For n_sparse != 0, we can't use an identity because output zeros must not
|
|
# be dependent on input zeros. This mimics the code in count_stored_elements().
|
|
def expected(M):
|
|
if n_sparse == 0: return M
|
|
mask = (M != 0).any(range(M.ndim - n_dense, M.ndim), keepdims=True)
|
|
return jnp.where(mask, M, 0)
|
|
self._CheckGradsSparse(expected, round_trip, args_maker)
|
|
|
|
def test_bcoo_fromdense_sorted_and_unique_indices(self):
|
|
rng = self.rng()
|
|
rng_sparse = sptu.rand_sparse(rng)
|
|
mat = sparse.BCOO.fromdense(rng_sparse((5, 6), np.float32))
|
|
perm = rng.permutation(mat.nse)
|
|
mat_unsorted = sparse.BCOO((mat.data[perm], mat.indices[perm]),
|
|
shape=mat.shape,
|
|
unique_indices=mat.unique_indices)
|
|
mat_resorted = mat_unsorted.sort_indices()
|
|
with self.subTest('sorted indices'):
|
|
self.assertArraysEqual(mat.indices, mat_resorted.indices)
|
|
self.assertArraysEqual(mat.data, mat_resorted.data)
|
|
|
|
with self.subTest('unique indices'):
|
|
self.assertTrue(mat.unique_indices)
|
|
self.assertTrue(mat_unsorted.unique_indices)
|
|
self.assertTrue(mat_resorted.unique_indices)
|
|
|
|
@jtu.sample_product(
|
|
[
|
|
dict(shape=shape, n_batch=layout.n_batch, n_dense=layout.n_dense)
|
|
for shape in [(5,), (5, 8), (8, 5), (3, 4, 5), (3, 4, 3, 2)]
|
|
for layout in sptu.iter_sparse_layouts(shape)
|
|
],
|
|
dtype=jtu.dtypes.floating + jtu.dtypes.complex,
|
|
assume_unique=[True, False, None],
|
|
)
|
|
def test_bcoo_extract(self, shape, dtype, n_batch, n_dense, assume_unique):
|
|
rng = sptu.rand_sparse(self.rng())
|
|
|
|
def args_maker():
|
|
x = rng(shape, dtype)
|
|
x_bcoo = sparse.bcoo_fromdense(x, n_batch=n_batch, n_dense=n_dense)
|
|
# Unique indices are required for this test when assume_unique == True.
|
|
self.assertTrue(x_bcoo.unique_indices)
|
|
return x_bcoo, x
|
|
|
|
dense_op = lambda _, x: x
|
|
sparse_op = partial(sparse.bcoo_extract, assume_unique=assume_unique)
|
|
|
|
self._CheckAgainstDense(dense_op, sparse_op, args_maker)
|
|
self._CheckBatchingSparse(dense_op, sparse_op, args_maker, bdims=2 * self._random_bdims(n_batch))
|
|
|
|
def test_bcoo_extract_duplicate_indices(self):
|
|
data = jnp.array([1, 3, 9, 27, 81, 243])
|
|
indices = jnp.array([[0], [5], [0], [3], [2], [3]])
|
|
shape = (6,)
|
|
mat = sparse.BCOO((data, indices), shape=shape).todense()
|
|
|
|
data1 = sparse_bcoo._bcoo_extract(indices, mat, assume_unique=True)
|
|
self.assertArraysEqual(data1, jnp.array([10, 3, 10, 270, 81, 270]))
|
|
|
|
data2 = sparse_bcoo._bcoo_extract(indices, mat, assume_unique=False)
|
|
self.assertArraysEqual(data2, jnp.array([10, 3, 0, 270, 81, 0]))
|
|
|
|
def test_bcoo_extract_duplicate_indices_n_sparse_0(self):
|
|
data = jnp.arange(6).reshape(3, 2)
|
|
indices = jnp.empty((3, 2, 0), dtype=int)
|
|
shape = (3,)
|
|
mat = sparse.BCOO((data, indices), shape=shape).todense()
|
|
|
|
data1 = sparse_bcoo._bcoo_extract(indices, mat, assume_unique=True)
|
|
self.assertArraysEqual(data1, jnp.array([[1, 1], [5, 5], [9, 9]]))
|
|
|
|
data2 = sparse_bcoo._bcoo_extract(indices, mat, assume_unique=False)
|
|
self.assertArraysEqual(data2, jnp.array([[1, 0], [5, 0], [9, 0]]))
|
|
|
|
def test_bcoo_extract_batching(self):
|
|
# https://github.com/jax-ml/jax/issues/9431
|
|
indices = jnp.zeros((4, 1, 1), dtype=int)
|
|
mat = jnp.arange(4.).reshape((4, 1))
|
|
|
|
# in_axes = (0, None)
|
|
expected = jnp.vstack([sparse_bcoo._bcoo_extract(i, mat[0]) for i in indices])
|
|
actual = vmap(sparse_bcoo._bcoo_extract, in_axes=(0, None))(indices, mat[0])
|
|
self.assertArraysEqual(expected, actual)
|
|
|
|
# in_axes = (None, 0)
|
|
expected = jnp.vstack([sparse_bcoo._bcoo_extract(indices[0], m) for m in mat])
|
|
actual = vmap(sparse_bcoo._bcoo_extract, in_axes=(None, 0))(indices[0], mat)
|
|
self.assertArraysEqual(expected, actual)
|
|
|
|
# in_axes = (0, 0)
|
|
expected = jnp.vstack([sparse_bcoo._bcoo_extract(i, m) for i, m in zip(indices, mat)])
|
|
actual = vmap(sparse_bcoo._bcoo_extract, in_axes=0)(indices, mat)
|
|
self.assertArraysEqual(expected, actual)
|
|
|
|
@jtu.sample_product(
|
|
[
|
|
dict(shape=shape, n_batch=layout.n_batch, n_dense=layout.n_dense)
|
|
for shape in [(5,), (5, 8), (8, 5), (3, 4, 5), (3, 4, 3, 2)]
|
|
for layout in sptu.iter_sparse_layouts(shape)
|
|
],
|
|
dtype=jtu.dtypes.floating,
|
|
)
|
|
def test_bcoo_extract_ad(self, shape, dtype, n_batch, n_dense):
|
|
rng = sptu.rand_sparse(self.rng())
|
|
M = rng(shape, dtype)
|
|
nse = sparse.util._count_stored_elements(M, n_batch=n_batch,
|
|
n_dense=n_dense)
|
|
data, indices = sparse_bcoo._bcoo_fromdense(M, nse=nse, n_batch=n_batch, n_dense=n_dense)
|
|
|
|
extract = partial(sparse_bcoo._bcoo_extract, indices)
|
|
j1 = jax.jacfwd(extract)(M)
|
|
j2 = jax.jacrev(extract)(M)
|
|
hess = jax.hessian(extract)(M)
|
|
self.assertArraysAllClose(j1, j2)
|
|
self.assertEqual(j1.shape, data.shape + M.shape)
|
|
self.assertEqual(hess.shape, data.shape + 2 * M.shape)
|
|
|
|
def test_bcoo_extract_zero_nse(self):
|
|
# Regression test for https://github.com/jax-ml/jax/issues/13653
|
|
|
|
# (n_batch, n_sparse, n_dense) = (1, 0, 0), nse = 2
|
|
args_maker = lambda: (jnp.zeros((3, 2, 0), dtype='int32'), jnp.arange(3))
|
|
self._CompileAndCheck(sparse_bcoo._bcoo_extract, args_maker)
|
|
|
|
# (n_batch, n_sparse, n_dense) = (0, 0, 1), nse = 2
|
|
args_maker = lambda: (jnp.zeros((2, 0), dtype='int32'), jnp.arange(3))
|
|
self._CompileAndCheck(sparse_bcoo._bcoo_extract, args_maker)
|
|
|
|
@jtu.sample_product(
|
|
[
|
|
dict(shape=shape, n_batch=layout.n_batch, n_dense=layout.n_dense)
|
|
for shape in [(5,), (5, 8), (8, 5), (3, 4, 5), (3, 4, 3, 2)]
|
|
for layout in sptu.iter_sparse_layouts(shape)
|
|
],
|
|
dtype=jtu.dtypes.numeric,
|
|
)
|
|
def test_bcoo_transpose(self, shape, dtype, n_batch, n_dense):
|
|
n_sparse = len(shape) - n_batch - n_dense
|
|
rng = self.rng()
|
|
sprng = sptu.rand_bcoo(rng, n_batch=n_batch, n_dense=n_dense)
|
|
|
|
permutation = np.concatenate([
|
|
rng.permutation(range(n_batch)),
|
|
rng.permutation(range(n_batch, n_batch + n_sparse)),
|
|
rng.permutation(range(n_batch + n_sparse, len(shape))),
|
|
]).astype(int)
|
|
|
|
args_maker = lambda: [sprng(shape, dtype)]
|
|
dense_func = partial(lax.transpose, permutation=permutation)
|
|
sparse_func = partial(sparse.bcoo_transpose, permutation=permutation)
|
|
|
|
self._CheckAgainstDense(dense_func, sparse_func, args_maker)
|
|
if jnp.issubdtype(dtype, jnp.floating):
|
|
self._CheckGradsSparse(dense_func, sparse_func, args_maker)
|
|
self._CheckBatchingSparse(dense_func, sparse_func, args_maker, bdims=self._random_bdims(n_batch))
|
|
|
|
def test_bcoo_transpose_indices_sorted(self):
|
|
rng = self.rng()
|
|
rng_sparse = sptu.rand_sparse(rng)
|
|
n_batch, n_dense = 2, 2
|
|
shape = (2, 3, 4, 5, 6, 7, 8)
|
|
mat = sparse.BCOO.fromdense(rng_sparse(shape, np.float32),
|
|
n_dense=n_dense, n_batch=n_batch)
|
|
|
|
permutations = (1, 0, 2, 3, 4, 6, 5)
|
|
mat_T_indices_sorted = mat.transpose(axes=permutations)
|
|
self.assertTrue(mat_T_indices_sorted.indices_sorted)
|
|
|
|
permutations = (0, 1, 3, 2, 4, 5, 6)
|
|
mat_T_indices_unsorted = mat.transpose(axes=permutations)
|
|
self.assertFalse(mat_T_indices_unsorted.indices_sorted)
|
|
|
|
@jtu.sample_product(
|
|
[
|
|
dict(shape=shape, n_batch=layout.n_batch, n_dense=layout.n_dense)
|
|
for shape in [(5, 8), (8, 5), (3, 4, 5), (3, 4, 3, 2)]
|
|
for layout in sptu.iter_sparse_layouts(shape, min_n_batch=1)
|
|
],
|
|
dtype=jtu.dtypes.floating + jtu.dtypes.complex,
|
|
)
|
|
def test_bcoo_todense_partial_batch(self, shape, dtype, n_batch, n_dense):
|
|
rng = sptu.rand_sparse(self.rng())
|
|
M = rng(shape, dtype)
|
|
nse = sparse.util._count_stored_elements(M, n_batch=n_batch,
|
|
n_dense=n_dense)
|
|
data, indices = sparse_bcoo._bcoo_fromdense(M, nse=nse, n_batch=n_batch, n_dense=n_dense)
|
|
|
|
M1 = sparse_bcoo._bcoo_todense(data, indices[:1], spinfo=sparse_util.SparseInfo(M.shape))
|
|
M2 = sparse_bcoo._bcoo_todense(data, jnp.stack(shape[0] * [indices[0]]), spinfo=sparse_util.SparseInfo(M.shape))
|
|
self.assertAllClose(M1, M2)
|
|
|
|
M3 = sparse_bcoo._bcoo_todense(data[:1], indices, spinfo=sparse_util.SparseInfo(M.shape))
|
|
M4 = sparse_bcoo._bcoo_todense(jnp.stack(shape[0] * [data[0]]), indices, spinfo=sparse_util.SparseInfo(M.shape))
|
|
self.assertAllClose(M3, M4)
|
|
|
|
@jtu.sample_product(
|
|
props=_generate_batched_dot_general_properties(),
|
|
dtype=jtu.dtypes.floating + jtu.dtypes.complex,
|
|
)
|
|
@jax.default_matmul_precision("float32")
|
|
def test_bcoo_dot_general(
|
|
self, dtype: np.dtype, props: sptu.BatchedDotGeneralProperties
|
|
):
|
|
rng = jtu.rand_default(self.rng())
|
|
sprng = sptu.rand_bcoo(self.rng(), n_batch=props.n_batch, n_dense=props.n_dense)
|
|
args_maker = lambda: [sprng(props.lhs_shape, dtype),
|
|
rng(props.rhs_shape, dtype)]
|
|
dense_fun = partial(lax.dot_general, dimension_numbers=props.dimension_numbers)
|
|
sparse_fun = partial(sparse.bcoo_dot_general, dimension_numbers=props.dimension_numbers)
|
|
|
|
tol = {np.float64: 1E-12, np.complex128: 1E-12,
|
|
np.float32: 1E-5, np.complex64: 1E-5}
|
|
self._CheckAgainstDense(dense_fun, sparse_fun, args_maker, tol=tol)
|
|
if jnp.issubdtype(dtype, jnp.floating) and props.n_dense == 0:
|
|
# Dense dimensions not yet fully supported in reverse mode.
|
|
modes = ['fwd'] if props.n_dense != 0 else ['fwd', 'rev']
|
|
self._CheckGradsSparse(dense_fun, sparse_fun, args_maker, modes=modes, atol=tol, rtol=tol)
|
|
self._CheckBatchingSparse(dense_fun, sparse_fun, args_maker, atol=tol, rtol=tol,
|
|
bdims=self._random_bdims(props.n_batch, len(props.rhs_shape)))
|
|
|
|
@jtu.sample_product(
|
|
[
|
|
dict(
|
|
lhs_shape=lhs_shape,
|
|
rhs_shape=rhs_shape,
|
|
lhs_contracting=lhs_contracting,
|
|
rhs_contracting=rhs_contracting,
|
|
)
|
|
for lhs_shape, rhs_shape, lhs_contracting, rhs_contracting in [
|
|
[(5,), (5,), [0], [0]],
|
|
[(5,), (5, 7), [0], [0]],
|
|
[(5,), (7, 5), [0], [1]],
|
|
[(5, 7), (5,), [0], [0]],
|
|
[(7, 5), (5,), [1], [0]],
|
|
[(3, 5), (2, 5), [1], [1]],
|
|
[(3, 5), (5, 2), [1], [0]],
|
|
[(5, 3), (2, 5), [0], [1]],
|
|
[(5, 3), (5, 2), [0], [0]],
|
|
]
|
|
],
|
|
dtype=jtu.dtypes.floating + jtu.dtypes.complex,
|
|
)
|
|
@jax.default_matmul_precision("float32")
|
|
@jtu.run_on_devices("gpu")
|
|
def test_bcoo_dot_general_cusparse(
|
|
self, lhs_shape, rhs_shape, dtype, lhs_contracting, rhs_contracting
|
|
):
|
|
rng = jtu.rand_small(self.rng())
|
|
rng_sparse = sptu.rand_sparse(self.rng())
|
|
def args_maker():
|
|
lhs = rng_sparse(lhs_shape, dtype)
|
|
rhs = rng(rhs_shape, dtype)
|
|
nse = sparse.util._count_stored_elements(lhs, n_batch=0, n_dense=0)
|
|
lhs_bcoo = sparse_bcoo.bcoo_fromdense(lhs, nse=nse, index_dtype=jnp.int32)
|
|
return lhs_bcoo, lhs, rhs
|
|
|
|
dimension_numbers = ((lhs_contracting, rhs_contracting), ([], []))
|
|
|
|
def f_dense(lhs_bcoo, lhs, rhs):
|
|
return lax.dot_general(lhs, rhs, dimension_numbers=dimension_numbers)
|
|
|
|
def f_sparse(lhs_bcoo, lhs, rhs):
|
|
return sparse_bcoo.bcoo_dot_general(lhs_bcoo, rhs,
|
|
dimension_numbers=dimension_numbers)
|
|
|
|
self._CompileAndCheck(f_sparse, args_maker)
|
|
self._CheckAgainstNumpy(f_dense, f_sparse, args_maker)
|
|
|
|
@jtu.sample_product(
|
|
[
|
|
dict(
|
|
n_batch=n_batch,
|
|
lhs_shape=lhs_shape,
|
|
rhs_shape=rhs_shape,
|
|
lhs_contracting=lhs_contracting,
|
|
rhs_contracting=rhs_contracting,
|
|
)
|
|
for n_batch, lhs_shape, rhs_shape, lhs_contracting, rhs_contracting in [
|
|
[1, (1, 2, 3), (3, 2), [2], [0]],
|
|
[1, (1, 3, 2), (3, 2), [1], [0]],
|
|
[1, (1, 3, 2), (4, 3), [1], [1]],
|
|
[1, (4, 2, 3), (3, 5), [2], [0]],
|
|
[1, (4, 2, 3), (2, 5), [1], [0]],
|
|
[1, (4, 2, 3), (5, 3), [2], [1]],
|
|
]
|
|
],
|
|
dtype=jtu.dtypes.floating + jtu.dtypes.complex,
|
|
)
|
|
@jax.default_matmul_precision("float32")
|
|
@jtu.run_on_devices("gpu")
|
|
def test_bcoo_batched_matmat_cusparse(
|
|
self,
|
|
n_batch,
|
|
lhs_shape,
|
|
rhs_shape,
|
|
dtype,
|
|
lhs_contracting,
|
|
rhs_contracting,
|
|
):
|
|
rng = jtu.rand_small(self.rng())
|
|
rng_sparse = sptu.rand_sparse(self.rng())
|
|
def args_maker():
|
|
lhs = rng_sparse(lhs_shape, dtype)
|
|
rhs = rng(rhs_shape, dtype)
|
|
nse = sparse.util._count_stored_elements(lhs, n_batch=n_batch,
|
|
n_dense=0)
|
|
lhs_bcoo = sparse_bcoo.bcoo_fromdense(lhs, n_batch=n_batch, nse=nse,
|
|
index_dtype=jnp.int32)
|
|
return lhs_bcoo, lhs, rhs
|
|
|
|
dimension_numbers = ((lhs_contracting, rhs_contracting), ([], []))
|
|
|
|
def f_dense(lhs_bcoo, lhs, rhs):
|
|
return lax.dot_general(lhs, rhs, dimension_numbers=dimension_numbers)
|
|
|
|
def f_sparse(lhs_bcoo, lhs, rhs):
|
|
return sparse_bcoo.bcoo_dot_general(lhs_bcoo, rhs,
|
|
dimension_numbers=dimension_numbers)
|
|
|
|
# TODO(tianjianlu): In some cases, this fails python_should_be_executing.
|
|
# self._CompileAndCheck(f_sparse, args_maker)
|
|
self._CheckAgainstNumpy(f_dense, f_sparse, args_maker)
|
|
|
|
@jtu.sample_product(
|
|
[
|
|
dict(
|
|
n_batch=n_batch,
|
|
lhs_shape=lhs_shape,
|
|
rhs_shape=rhs_shape,
|
|
lhs_contracting=lhs_contracting,
|
|
rhs_contracting=rhs_contracting,
|
|
)
|
|
for n_batch, lhs_shape, rhs_shape, lhs_contracting, rhs_contracting in [
|
|
[1, (1, 2, 3), (3), [2], [0]],
|
|
[1, (1, 2), (3, 2), [1], [1]],
|
|
]
|
|
],
|
|
dtype=jtu.dtypes.floating + jtu.dtypes.complex,
|
|
)
|
|
@jtu.run_on_devices("gpu")
|
|
def test_bcoo_batched_matmat_default_lowering(
|
|
self,
|
|
n_batch,
|
|
lhs_shape,
|
|
rhs_shape,
|
|
dtype,
|
|
lhs_contracting,
|
|
rhs_contracting,
|
|
):
|
|
rng = jtu.rand_small(self.rng())
|
|
rng_sparse = sptu.rand_sparse(self.rng())
|
|
lhs = rng_sparse(lhs_shape, dtype)
|
|
rhs = rng(rhs_shape, dtype)
|
|
nse = sparse.util._count_stored_elements(lhs, n_batch=n_batch,
|
|
n_dense=0)
|
|
lhs_bcoo = sparse_bcoo.bcoo_fromdense(
|
|
lhs, n_batch=n_batch, nse=nse, index_dtype=jnp.int32
|
|
)
|
|
dimension_numbers = ((lhs_contracting, rhs_contracting), ([], []))
|
|
matmat_expected = lax.dot_general(lhs, rhs,
|
|
dimension_numbers=dimension_numbers)
|
|
sp_matmat = jit(partial(sparse_bcoo.bcoo_dot_general,
|
|
dimension_numbers=dimension_numbers))
|
|
|
|
# TODO(jakevdp): uncomment once batching is supported again.
|
|
# with self.gpu_matmul_warning_context(
|
|
# "bcoo_dot_general GPU lowering currently does not support this batch-mode computation.*"):
|
|
matmat_default_lowering_fallback = sp_matmat(lhs_bcoo, rhs)
|
|
self.assertArraysEqual(matmat_expected, matmat_default_lowering_fallback)
|
|
|
|
@jtu.run_on_devices("gpu")
|
|
def test_bcoo_dot_general_oob_and_unsorted_indices_cusparse(self):
|
|
"""Tests bcoo dot general with out-of-bound and unsorted indices."""
|
|
|
|
rhs = jnp.ones((5, 3), dtype=jnp.float32)
|
|
|
|
# It creates out-of-bound indices when nse > nnz.
|
|
lhs_mat_dense = jnp.array([[1, 0, 2, 3, 0], [0, 0, 0, 4, 0]],
|
|
dtype=jnp.float32)
|
|
lhs_mat_bcoo = sparse.BCOO.fromdense(lhs_mat_dense, nse=7)
|
|
rng = self.rng()
|
|
perm = rng.permutation(lhs_mat_bcoo.nse)
|
|
lhs_mat_bcoo_unsorted = sparse.BCOO(
|
|
(lhs_mat_bcoo.data[perm], lhs_mat_bcoo.indices[perm]),
|
|
shape=lhs_mat_dense.shape)
|
|
|
|
dimension_numbers_2d = (([1], [0]), ([], []))
|
|
sp_matmat = jit(partial(sparse_bcoo.bcoo_dot_general,
|
|
dimension_numbers=dimension_numbers_2d))
|
|
|
|
matmat_expected = lax.dot_general(lhs_mat_dense, rhs,
|
|
dimension_numbers=dimension_numbers_2d)
|
|
with self.subTest(msg="2D"):
|
|
with self.gpu_matmul_warning_context(
|
|
"bcoo_dot_general GPU lowering requires matrices with sorted indices*"):
|
|
matmat_unsorted_fallback = sp_matmat(lhs_mat_bcoo_unsorted, rhs)
|
|
self.assertArraysEqual(matmat_expected, matmat_unsorted_fallback)
|
|
|
|
lhs_vec_dense = jnp.array([0, 1, 0, 2, 0], dtype=jnp.float32)
|
|
lhs_vec_bcoo = sparse.BCOO.fromdense(lhs_vec_dense, nse=5)
|
|
rng = self.rng()
|
|
perm = rng.permutation(lhs_vec_bcoo.nse)
|
|
lhs_vec_bcoo_unsorted = sparse.BCOO(
|
|
(lhs_vec_bcoo.data[perm], lhs_vec_bcoo.indices[perm]),
|
|
shape=lhs_vec_dense.shape, indices_sorted=False)
|
|
|
|
dimension_numbers_1d = (([0], [0]), ([], []))
|
|
sp_vecmat = jit(partial(sparse_bcoo.bcoo_dot_general,
|
|
dimension_numbers=dimension_numbers_1d))
|
|
|
|
vecmat_expected = lax.dot_general(lhs_vec_dense, rhs,
|
|
dimension_numbers=dimension_numbers_1d)
|
|
|
|
with self.subTest(msg="1D"):
|
|
with self.gpu_matmul_warning_context(
|
|
"bcoo_dot_general GPU lowering requires matrices with sorted indices*"):
|
|
vecmat_unsorted_fallback = sp_vecmat(lhs_vec_bcoo_unsorted, rhs)
|
|
self.assertArraysEqual(vecmat_expected, vecmat_unsorted_fallback)
|
|
|
|
@jtu.sample_product(
|
|
props=_generate_batched_dot_general_properties(),
|
|
dtype=jtu.dtypes.floating + jtu.dtypes.complex,
|
|
)
|
|
@jax.default_matmul_precision("float32")
|
|
def test_bcoo_rdot_general(
|
|
self, dtype: np.dtype, props: sptu.BatchedDotGeneralProperties
|
|
):
|
|
rng = jtu.rand_default(self.rng())
|
|
sprng = sptu.rand_bcoo(self.rng(), n_batch=props.n_batch, n_dense=props.n_dense)
|
|
args_maker = lambda: [rng(props.rhs_shape, dtype),
|
|
sprng(props.lhs_shape, dtype)]
|
|
dimension_numbers = tuple(d[::-1] for d in props.dimension_numbers)
|
|
sparse_fun = partial(sparse.bcoo_dot_general, dimension_numbers=dimension_numbers)
|
|
dense_fun = partial(lax.dot_general, dimension_numbers=dimension_numbers)
|
|
|
|
tol = {np.float64: 1E-12, np.complex128: 1E-12,
|
|
np.float32: 1E-5, np.complex64: 1E-5}
|
|
self._CheckAgainstDense(dense_fun, sparse_fun, args_maker, tol=tol)
|
|
if jnp.issubdtype(dtype, jnp.floating):
|
|
# Dense dimensions not yet fully supported in reverse mode.
|
|
modes = ['fwd'] if props.n_dense != 0 else ['fwd', 'rev']
|
|
self._CheckGradsSparse(dense_fun, sparse_fun, args_maker, modes=modes, atol=tol, rtol=tol)
|
|
|
|
@jtu.sample_product(
|
|
[
|
|
dict(
|
|
n_batch=n_batch,
|
|
n_dense=n_dense,
|
|
lhs_shape=lhs_shape,
|
|
rhs_shape=rhs_shape,
|
|
dimension_numbers=dimension_numbers,
|
|
)
|
|
for lhs_shape, rhs_shape, dimension_numbers, n_batch, n_dense in [
|
|
((3, 3, 2), (3, 2, 4), (([2], [1]), ([0], [0])), 1, 0),
|
|
((3, 3, 2), (3, 2, 4), (([2], [1]), ([0], [0])), 2, 0),
|
|
((3, 3, 2), (2, 3, 4), (([2], [0]), ([0], [1])), 1, 0),
|
|
((3, 3, 2), (2, 3, 4), (([2], [0]), ([0], [1])), 2, 0),
|
|
(
|
|
(3, 4, 2, 4),
|
|
(3, 4, 3, 2),
|
|
(([2], [3]), ([0, 1], [0, 1])),
|
|
2,
|
|
0,
|
|
),
|
|
(
|
|
(3, 4, 2, 4),
|
|
(3, 4, 3, 2),
|
|
(([2], [3]), ([0, 1], [0, 1])),
|
|
2,
|
|
1,
|
|
),
|
|
]
|
|
],
|
|
dtype=jtu.dtypes.floating + jtu.dtypes.complex,
|
|
)
|
|
@jax.default_matmul_precision("float32")
|
|
def test_bcoo_dot_general_partial_batch(
|
|
self, lhs_shape, rhs_shape, dtype, dimension_numbers, n_batch, n_dense
|
|
):
|
|
rng = jtu.rand_small(self.rng())
|
|
rng_sparse = sptu.rand_sparse(self.rng())
|
|
|
|
X = rng_sparse(lhs_shape, dtype)
|
|
nse = sparse.util._count_stored_elements(X, n_batch=n_batch,
|
|
n_dense=n_dense)
|
|
data, indices = sparse_bcoo._bcoo_fromdense(X, nse=nse, n_batch=n_batch, n_dense=n_dense)
|
|
Y = rng(rhs_shape, dtype)
|
|
|
|
def f_dense(X, Y):
|
|
return lax.dot_general(X, Y, dimension_numbers=dimension_numbers)
|
|
|
|
def f_sparse(data, indices, Y):
|
|
return sparse_bcoo._bcoo_dot_general(data, indices, Y, lhs_spinfo=sparse_util.SparseInfo(X.shape),
|
|
dimension_numbers=dimension_numbers, preferred_element_type=None)
|
|
|
|
for data, indices in itertools.product([data, data[:1]], [indices, indices[:1]]):
|
|
X = sparse_bcoo._bcoo_todense(data, indices, spinfo=sparse_util.SparseInfo(X.shape))
|
|
self.assertAllClose(f_dense(X, Y), f_sparse(data, indices, Y))
|
|
|
|
@jtu.sample_product(
|
|
props=_generate_bcoo_dot_general_sampled_properties(),
|
|
dtype=jtu.dtypes.floating + jtu.dtypes.complex,
|
|
)
|
|
@jax.default_matmul_precision("float32")
|
|
@jtu.skip_on_flag("jax_skip_slow_tests", True)
|
|
def test_bcoo_dot_general_sampled(self, props, dtype):
|
|
rng = jtu.rand_default(self.rng())
|
|
sprng = sptu.rand_bcoo(self.rng(), n_batch=props.n_batch, n_dense=props.n_dense)
|
|
out = jax.eval_shape(partial(lax.dot_general, dimension_numbers=props.dimension_numbers),
|
|
jax.ShapeDtypeStruct(props.lhs_shape, dtype),
|
|
jax.ShapeDtypeStruct(props.rhs_shape, dtype))
|
|
args_maker = lambda: [rng(props.lhs_shape, dtype), rng(props.rhs_shape, dtype),
|
|
sprng(out.shape, dtype).indices]
|
|
|
|
def dense_fun(lhs, rhs, indices):
|
|
AB = lax.dot_general(lhs, rhs, dimension_numbers=props.dimension_numbers)
|
|
return sparse_bcoo._bcoo_extract(indices, AB)
|
|
def sparse_fun(lhs, rhs, indices):
|
|
return sparse.bcoo_dot_general_sampled(
|
|
lhs, rhs, indices, dimension_numbers=props.dimension_numbers)
|
|
|
|
self._CheckAgainstDense(dense_fun, sparse_fun, args_maker)
|
|
if jnp.issubdtype(dtype, jnp.floating):
|
|
# Note: forward mode fails for some sparse layouts.
|
|
# TODO(jakevdp) fix forward-mode autodiff & enable tests here.
|
|
self._CheckGradsSparse(dense_fun, sparse_fun, args_maker, modes=['rev'], argnums=[0, 1])
|
|
|
|
@jtu.sample_product(
|
|
[
|
|
{
|
|
"xshape": xshape,
|
|
"yshape": yshape,
|
|
"lhs_contract": lhs_contract,
|
|
"rhs_contract": rhs_contract,
|
|
}
|
|
for (xshape, yshape, lhs_contract, rhs_contract) in [
|
|
[(4, 3), (4, 5), (0,), (0,)],
|
|
[(3, 4), (4, 5), (1,), (0,)],
|
|
[(4, 3), (5, 4), (0,), (1,)],
|
|
[(3, 4), (5, 4), (1,), (1,)],
|
|
[(3,), (3,), (), ()],
|
|
[(3,), (5,), (), ()],
|
|
[(5,), (3,), (), ()],
|
|
[(5,), (5,), (), ()],
|
|
]
|
|
],
|
|
dtype=jtu.dtypes.floating + jtu.dtypes.complex,
|
|
n_batch=[0, 1, 2],
|
|
)
|
|
@jax.default_matmul_precision("float32")
|
|
def test_bcoo_dot_general_sampled_fast_cases(
|
|
self, xshape, yshape, lhs_contract, rhs_contract, n_batch, dtype):
|
|
rng = jtu.rand_default(self.rng())
|
|
sprng = sptu.rand_bcoo(self.rng(), n_batch=n_batch)
|
|
dimension_numbers = ((lhs_contract, rhs_contract), ([], []))
|
|
|
|
out_shape = jax.eval_shape(partial(lax.dot_general, dimension_numbers=dimension_numbers),
|
|
jax.ShapeDtypeStruct(xshape, dtype), jax.ShapeDtypeStruct(yshape, dtype))
|
|
|
|
args_maker = lambda: [rng(xshape, dtype), rng(yshape, dtype),
|
|
sprng(out_shape.shape, out_shape.dtype).indices]
|
|
|
|
def f1(x, y, indices):
|
|
mat_full = lax.dot_general(x, y, dimension_numbers=dimension_numbers)
|
|
return sparse_bcoo._bcoo_extract(indices, mat_full)
|
|
|
|
def f2(x, y, indices):
|
|
return sparse.bcoo_dot_general_sampled(x, y, indices, dimension_numbers=dimension_numbers)
|
|
|
|
self._CheckAgainstNumpy(f1, f2, args_maker, tol=sptu.MATMUL_TOL)
|
|
self._CompileAndCheck(f2, args_maker, tol=sptu.MATMUL_TOL)
|
|
|
|
@jtu.sample_product(
|
|
[
|
|
dict(
|
|
n_batch=n_batch,
|
|
n_dense=n_dense,
|
|
lhs_shape=lhs_shape,
|
|
rhs_shape=rhs_shape,
|
|
dimension_numbers=dimension_numbers,
|
|
)
|
|
for lhs_shape, rhs_shape, dimension_numbers, n_batch, n_dense in [
|
|
((3, 3, 2), (3, 2, 4), (([2], [1]), ([0], [0])), 1, 0),
|
|
((3, 3, 2), (3, 2, 4), (([2], [1]), ([0], [0])), 1, 1),
|
|
((3, 3, 2), (3, 2, 4), (([2], [1]), ([0], [0])), 2, 0),
|
|
((3, 3, 2), (2, 3, 4), (([2], [0]), ([0], [1])), 1, 0),
|
|
((3, 3, 2), (2, 3, 4), (([2], [0]), ([0], [1])), 1, 1),
|
|
((3, 3, 2), (2, 3, 4), (([2], [0]), ([0], [1])), 2, 0),
|
|
(
|
|
(3, 4, 2, 4),
|
|
(3, 4, 3, 2),
|
|
(([2], [3]), ([0, 1], [0, 1])),
|
|
2,
|
|
0,
|
|
),
|
|
(
|
|
(3, 4, 2, 4),
|
|
(3, 4, 3, 2),
|
|
(([2], [3]), ([0, 1], [0, 1])),
|
|
2,
|
|
1,
|
|
),
|
|
]
|
|
],
|
|
dtype=jtu.dtypes.floating,
|
|
)
|
|
@jax.default_matmul_precision("float32")
|
|
def test_bcoo_dot_general_sampled_ad(self, lhs_shape, rhs_shape, dtype, dimension_numbers, n_batch, n_dense):
|
|
rng = jtu.rand_default(self.rng())
|
|
sprng = sptu.rand_sparse(self.rng())
|
|
out_shape = lax.dot_general(
|
|
jnp.zeros(lhs_shape),
|
|
jnp.zeros(rhs_shape),
|
|
dimension_numbers=dimension_numbers,
|
|
).shape
|
|
|
|
lhs = rng(lhs_shape, dtype)
|
|
rhs = rng(rhs_shape, dtype)
|
|
indices = sparse.BCOO.fromdense(sprng(out_shape, dtype),
|
|
n_batch=n_batch, n_dense=n_dense).indices
|
|
|
|
def dense_fun(lhs, rhs, indices):
|
|
AB = lax.dot_general(lhs, rhs, dimension_numbers=dimension_numbers)
|
|
return sparse_bcoo._bcoo_extract(indices, AB)
|
|
def sparse_fun(lhs, rhs, indices):
|
|
return sparse.bcoo_dot_general_sampled(
|
|
lhs, rhs, indices, dimension_numbers=dimension_numbers
|
|
)
|
|
|
|
jf_dense = jax.jacfwd(dense_fun)(lhs, rhs, indices)
|
|
jf_sparse = jax.jacfwd(sparse_fun)(lhs, rhs, indices)
|
|
jr_dense = jax.jacrev(dense_fun)(lhs, rhs, indices)
|
|
jr_sparse = jax.jacrev(sparse_fun)(lhs, rhs, indices)
|
|
|
|
self.assertAllClose(jf_sparse, jf_dense)
|
|
self.assertAllClose(jr_sparse, jr_dense)
|
|
self.assertAllClose(jf_sparse, jr_sparse)
|
|
|
|
@jtu.sample_product(
|
|
[
|
|
dict(
|
|
lhs_n_batch=lhs_n_batch,
|
|
rhs_n_batch=rhs_n_batch,
|
|
lhs_shape=lhs_shape,
|
|
rhs_shape=rhs_shape,
|
|
dimension_numbers=dimension_numbers,
|
|
)
|
|
for lhs_shape, lhs_n_batch, rhs_shape, rhs_n_batch, dimension_numbers in [
|
|
# (batched) outer products (no contraction)
|
|
((5,), 0, (6,), 0, (([], []), ([], []))),
|
|
((3, 5), 0, (2, 4), 0, (([], []), ([], []))),
|
|
((3, 5), 1, (3, 4), 1, (([], []), ([0], [0]))),
|
|
# (batched) vector-vector products
|
|
((5,), 0, (5,), 0, (([0], [0]), ([], []))),
|
|
((7,), 0, (7,), 0, (([0], [0]), ([], []))),
|
|
((5, 7), 1, (7,), 0, (([1], [0]), ([], []))),
|
|
((2, 3, 4), 2, (2, 4), 1, (([2], [1]), ([0], [0]))),
|
|
((2, 3, 4), 2, (2, 4), 1, (([2], [1]), ([], []))),
|
|
((2, 3, 4), 2, (3, 4), 1, (([2], [1]), ([1], [0]))),
|
|
((2, 3, 4), 2, (3, 4), 1, (([2], [1]), ([], []))),
|
|
# (batched) matrix-vector products
|
|
((5, 7), 0, (7,), 0, (([1], [0]), ([], []))),
|
|
((2, 3, 4), 1, (4,), 0, (([2], [0]), ([], []))),
|
|
((2, 3, 4), 1, (2, 4), 1, (([2], [1]), ([0], [0]))),
|
|
((3, 2, 4), 1, (3, 4), 1, (([2], [1]), ([0], [0]))),
|
|
((2, 3, 4), 0, (2,), 0, (([0], [0]), ([], []))),
|
|
# (batched) matrix-matrix products
|
|
((5, 7), 0, (7, 3), 0, (([1], [0]), ([], []))),
|
|
((2, 3, 4), 1, (4, 3), 0, (([2], [0]), ([], []))),
|
|
((2, 3, 4), 1, (2, 4, 3), 1, (([2], [1]), ([0], [0]))),
|
|
# more general operations
|
|
(
|
|
(2, 3, 4, 3),
|
|
1,
|
|
(2, 4, 3, 4),
|
|
1,
|
|
(([2, 3], [1, 2]), ([0], [0])),
|
|
),
|
|
(
|
|
(2, 3, 4, 3, 1),
|
|
2,
|
|
(3, 2, 3, 4),
|
|
2,
|
|
(([2, 3], [3, 2]), ([0, 1], [1, 0])),
|
|
),
|
|
]
|
|
],
|
|
swap=[True, False],
|
|
dtype=jtu.dtypes.floating + jtu.dtypes.complex,
|
|
)
|
|
@jax.default_matmul_precision("float32")
|
|
@jtu.skip_on_flag("jax_skip_slow_tests", True)
|
|
def test_bcoo_spdot_general(self, lhs_shape, lhs_n_batch, rhs_shape, rhs_n_batch, dtype, swap, dimension_numbers):
|
|
if swap:
|
|
dimension_numbers = tuple(d[::-1] for d in dimension_numbers)
|
|
lhs_shape, rhs_shape = rhs_shape, lhs_shape
|
|
lhs_n_batch, rhs_n_batch = rhs_n_batch, lhs_n_batch
|
|
|
|
lhs_n_sparse = len(lhs_shape) - lhs_n_batch
|
|
rhs_batch = dimension_numbers[1][1]
|
|
lhs_contracting = dimension_numbers[0][0]
|
|
should_error = (rhs_n_batch > len(rhs_batch) and lhs_n_sparse > len(lhs_contracting))
|
|
|
|
sprng = sptu.rand_bcoo(self.rng())
|
|
args_maker = lambda: [sprng(lhs_shape, dtype, n_batch=lhs_n_batch),
|
|
sprng(rhs_shape, dtype, n_batch=rhs_n_batch)]
|
|
|
|
def f_dense(x, y):
|
|
return lax.dot_general(x, y, dimension_numbers=dimension_numbers)
|
|
|
|
def f_sparse(xsp, ysp):
|
|
return sparse.bcoo_dot_general(xsp, ysp, dimension_numbers=dimension_numbers)
|
|
|
|
if should_error:
|
|
with self.assertRaisesRegex(ValueError, ".*cannot have unused batch dims on rhs with unused sparse dims on lhs."):
|
|
f_sparse(*args_maker())
|
|
else:
|
|
tol = {"float32": 1E-5, "complex64": 1E-5, "float64": 1E-14, "complex128": 1E-14}
|
|
self._CheckAgainstDense(f_dense, f_sparse, args_maker, tol=tol)
|
|
self._CheckBatchingSparse(f_dense, f_sparse, args_maker, tol=tol)
|
|
if jnp.issubdtype(dtype, jnp.floating):
|
|
self._CheckGradsSparse(f_dense, f_sparse, args_maker, modes=['fwd'])
|
|
|
|
@jtu.sample_product(lhs_shape=[(5,), (4, 5)], rhs_shape=[(5,), (5, 4)])
|
|
@jax.default_matmul_precision("float32")
|
|
def test_bcoo_spdot_general_nse(self, lhs_shape, rhs_shape):
|
|
rng = sptu.rand_bcoo(self.rng())
|
|
dtype = jnp.float32
|
|
lhs = rng(lhs_shape, dtype)
|
|
rhs = rng(rhs_shape, dtype)
|
|
out = lhs @ rhs
|
|
|
|
expected_out = lhs.todense() @ rhs.todense()
|
|
expected_nse = min(lhs.nse * rhs.nse, out.size)
|
|
|
|
self.assertArraysAllClose(out.todense(), expected_out)
|
|
self.assertEqual(out.nse, expected_nse)
|
|
|
|
@jtu.ignore_warning(message="bcoo_dot_general cusparse/hipsparse lowering not available")
|
|
def test_bcoo_spdot_general_ad_bug(self):
|
|
# Regression test for https://github.com/jax-ml/jax/issues/10163
|
|
A_indices = jnp.array([[0, 1], [0, 2], [1, 1], [1, 2], [1, 0]])
|
|
A_values = jnp.array([-2.0, 1.0, -1.0, 0.5, 2.0])
|
|
A_shape = (2, 3)
|
|
|
|
B_indices = jnp.array([[0, 2], [2, 1], [0, 3], [1, 3], [1, 0], [0, 0]])
|
|
B_values = jnp.array([10.0, 100.0, 1000.0, -5.0, -50.0, -500.0])
|
|
B_shape = (3, 4)
|
|
|
|
def sp_sp_product(v1, v2):
|
|
A = sparse.BCOO((v1, A_indices), shape=A_shape)
|
|
B = sparse.BCOO((v2, B_indices), shape=B_shape)
|
|
return (A @ B).todense()
|
|
|
|
def sp_de_product(v1, v2):
|
|
A = sparse.BCOO((v1, A_indices), shape=A_shape)
|
|
B = sparse.BCOO((v2, B_indices), shape=B_shape).todense()
|
|
return A @ B
|
|
|
|
def de_de_product(v1, v2):
|
|
sparse1 = sparse.BCOO((v1, A_indices), shape=A_shape).todense()
|
|
dense2 = sparse.BCOO((v2, B_indices), shape=B_shape).todense()
|
|
return sparse1 @ dense2
|
|
|
|
sp_sp_jac = jax.jacfwd(sp_sp_product, argnums=1)(A_values, B_values)
|
|
sp_de_jac = jax.jacfwd(sp_de_product, argnums=1)(A_values, B_values)
|
|
de_de_jac = jax.jacfwd(de_de_product, argnums=1)(A_values, B_values)
|
|
|
|
self.assertAllClose(sp_sp_jac, de_de_jac)
|
|
self.assertAllClose(sp_de_jac, de_de_jac)
|
|
|
|
@jtu.sample_product(
|
|
[
|
|
dict(shape=shape, n_batch=layout.n_batch, n_dense=layout.n_dense)
|
|
for shape in [(), (5,), (5, 8), (3, 4, 5), (3, 4, 3, 2)]
|
|
for layout in sptu.iter_sparse_layouts(shape)
|
|
],
|
|
dtype=jtu.dtypes.numeric,
|
|
)
|
|
def test_bcoo_slice(self, shape, dtype, n_batch, n_dense):
|
|
rng = self.rng()
|
|
sprng = sptu.rand_bcoo(rng, n_batch=n_batch, n_dense=n_dense)
|
|
args_maker = lambda: [sprng(shape, dtype)]
|
|
|
|
slices = rng.randint(0, np.array(shape) + 1, (2, len(shape))).T
|
|
slices.sort(1)
|
|
start_indices, limit_indices = unzip2(slices)
|
|
strides = list(rng.randint(1, 4, len(shape)))
|
|
kwds = dict(start_indices=start_indices, limit_indices=limit_indices, strides=strides)
|
|
|
|
dense_func = partial(lax.slice, **kwds)
|
|
sparse_func = partial(sparse.bcoo_slice, **kwds)
|
|
|
|
self._CheckAgainstDense(dense_func, sparse_func, args_maker)
|
|
if jnp.issubdtype(dtype, jnp.floating):
|
|
self._CheckGradsSparse(dense_func, sparse_func, args_maker)
|
|
|
|
mat, = args_maker()
|
|
out = sparse_func(mat)
|
|
|
|
# Array layout is the same
|
|
self.assertEqual(mat.n_batch, out.n_batch)
|
|
self.assertEqual(mat.n_sparse, out.n_sparse)
|
|
self.assertEqual(mat.n_dense, out.n_dense)
|
|
|
|
# Unnecessary padding eliminated
|
|
max_nse = math.prod(out.shape[out.n_batch: out.n_batch + out.n_sparse])
|
|
self.assertLessEqual(out.nse, max_nse)
|
|
|
|
@jtu.sample_product(
|
|
[
|
|
dict(shape=shape, n_batch=layout.n_batch, n_dense=layout.n_dense)
|
|
for shape in [(), (5,), (5, 8), (3, 4, 5), (3, 4, 3, 2)]
|
|
for layout in sptu.iter_sparse_layouts(shape)
|
|
],
|
|
dtype=jtu.dtypes.numeric,
|
|
)
|
|
def test_bcoo_dynamic_slice(self, shape, dtype, n_batch, n_dense):
|
|
rng = self.rng()
|
|
sprng = sptu.rand_bcoo(rng, n_batch=n_batch, n_dense=n_dense)
|
|
args_maker = lambda: [sprng(shape, dtype)]
|
|
|
|
rng = self.rng()
|
|
# Note: test out-of-range start indices
|
|
start_indices = rng.randint(-max(shape, default=0), max(shape, default=0), len(shape))
|
|
slice_sizes = rng.randint(0, shape, len(shape))
|
|
kwds = dict(start_indices=start_indices, slice_sizes=slice_sizes)
|
|
dense_func = partial(lax.dynamic_slice, **kwds)
|
|
sparse_func = partial(sparse.bcoo_dynamic_slice, **kwds)
|
|
|
|
self._CheckAgainstDense(dense_func, sparse_func, args_maker)
|
|
if jnp.issubdtype(dtype, jnp.floating):
|
|
self._CheckGradsSparse(dense_func, sparse_func, args_maker)
|
|
|
|
mat, = args_maker()
|
|
out = sparse_func(mat)
|
|
|
|
# Array layout is the same
|
|
self.assertEqual(mat.n_batch, out.n_batch)
|
|
self.assertEqual(mat.n_sparse, out.n_sparse)
|
|
self.assertEqual(mat.n_dense, out.n_dense)
|
|
|
|
# Unnecessary padding eliminated
|
|
max_nse = math.prod(out.shape[out.n_batch: out.n_batch + out.n_sparse])
|
|
self.assertLessEqual(out.nse, max_nse)
|
|
|
|
@jtu.sample_product(
|
|
[
|
|
dict(shape=shape, n_batch=n_batch, n_dense=n_dense, idx=idx)
|
|
for shape, idx in [
|
|
[(5,), np.index_exp[:]],
|
|
[(5,), np.index_exp[4]],
|
|
[(5,), np.index_exp[::2]],
|
|
[(5,), np.index_exp[1::2]],
|
|
[(5,), 1],
|
|
[(3, 4), np.index_exp[1]],
|
|
[(3, 4), np.index_exp[1, 2]],
|
|
[(3, 4), np.index_exp[np.array([1, 2])]],
|
|
[(3, 4), np.index_exp[np.array([[1], [2]]), 0]],
|
|
[(3, 4), np.index_exp[np.array([[1], [2]]), 1:]],
|
|
[(3, 4), np.index_exp[np.array([True, False, True])]],
|
|
[(3, 4), np.index_exp[:2, np.array([True, False, True, False])]],
|
|
[(3, 4), np.index_exp[None, 0, np.array([[2]])]],
|
|
[(3, 4, 5), np.index_exp[2]],
|
|
[(3, 4, 5), np.index_exp[:, 2]],
|
|
]
|
|
for n_batch in range(len(shape) + 1)
|
|
for n_dense in [0] # TODO(jakevdp): add tests with n_dense
|
|
],
|
|
dtype=jtu.dtypes.numeric,
|
|
)
|
|
def test_bcoo_getitem(self, shape, dtype, n_batch, n_dense, idx):
|
|
sprng = sptu.rand_bcoo(self.rng(), n_batch=n_batch, n_dense=n_dense)
|
|
args_maker = lambda: [sprng(shape, dtype)]
|
|
|
|
fun = lambda x: x[idx]
|
|
|
|
self._CheckAgainstDense(fun, fun, args_maker)
|
|
if jnp.issubdtype(dtype, jnp.floating):
|
|
self._CheckGradsSparse(fun, fun, args_maker)
|
|
|
|
@jtu.sample_product(
|
|
[
|
|
dict(shape=shape, n_batch=n_batch, n_dense=n_dense)
|
|
for shape in [(2,), (3, 4), (5, 6, 2)]
|
|
for n_batch in range(len(shape) + 1)
|
|
for n_dense in [0] # TODO(jakevdp): add tests with n_dense
|
|
],
|
|
dtype=jtu.dtypes.numeric,
|
|
)
|
|
def test_bcoo_iter(self, shape, dtype, n_batch, n_dense):
|
|
sprng = sptu.rand_sparse(self.rng())
|
|
args_maker = lambda: [sprng(shape, dtype)]
|
|
|
|
self._CheckAgainstDense(list, list, args_maker)
|
|
|
|
@jtu.sample_product(
|
|
[
|
|
dict(
|
|
shape=shape,
|
|
n_batch=layout.n_batch,
|
|
n_dense=layout.n_dense,
|
|
nse=nse,
|
|
)
|
|
for shape in [(5,), (5, 8), (8, 5), (3, 4, 5), (3, 4, 3, 2)]
|
|
for layout in sptu.iter_sparse_layouts(shape)
|
|
for nse in [None, math.prod(shape) - 1]
|
|
],
|
|
dtype=jtu.dtypes.floating + jtu.dtypes.complex,
|
|
remove_zeros=[True, False],
|
|
)
|
|
@jtu.skip_on_flag("jax_skip_slow_tests", True)
|
|
def test_bcoo_sum_duplicates(self, shape, dtype, n_batch, n_dense, nse, remove_zeros):
|
|
sprng = sptu.rand_bcoo(self.rng(), n_batch=n_batch, n_dense=n_dense)
|
|
|
|
def args_maker():
|
|
# Create a matrix with duplicate indices
|
|
M = sprng(shape, dtype)
|
|
new_indices = jnp.concatenate([M.indices, M.indices], axis=n_batch)
|
|
new_data = jnp.concatenate([M.data, M.data], axis=n_batch)
|
|
return [sparse.BCOO((new_data, new_indices), shape=M.shape)]
|
|
|
|
dense_fun = lambda x: x
|
|
def sparse_fun(x):
|
|
out = x.sum_duplicates(nse=nse, remove_zeros=remove_zeros)
|
|
self.assertTrue(out.unique_indices)
|
|
if nse:
|
|
self.assertEqual(out.nse, nse)
|
|
return out
|
|
self._CheckAgainstDense(dense_fun, sparse_fun, args_maker, check_jit=(nse is not None))
|
|
if jnp.issubdtype(dtype, jnp.floating):
|
|
self._CheckGradsSparse(dense_fun, sparse_fun, args_maker)
|
|
if nse is not None:
|
|
self._CheckBatchingSparse(dense_fun, sparse_fun, args_maker)
|
|
|
|
@jtu.sample_product(
|
|
[
|
|
dict(shape=shape, n_batch=layout.n_batch, n_dense=layout.n_dense)
|
|
for shape in [(5,), (5, 8), (8, 5), (3, 4, 5), (3, 4, 3, 2)]
|
|
for layout in sptu.iter_sparse_layouts(shape)
|
|
],
|
|
dtype=jtu.dtypes.floating + jtu.dtypes.complex,
|
|
)
|
|
def test_bcoo_sort_indices(self, shape, dtype, n_batch, n_dense):
|
|
rng_sparse = sptu.rand_sparse(self.rng(), rand_method=jtu.rand_some_zero)
|
|
M = sparse.BCOO.fromdense(rng_sparse(shape, dtype), n_batch=n_batch, n_dense=n_dense)
|
|
M.indices = M.indices[..., ::-1, :]
|
|
M.indices_sorted = False
|
|
|
|
M_sorted = M.sort_indices()
|
|
self.assertArraysEqual(M.todense(), M_sorted.todense())
|
|
self.assertEqual(M.unique_indices, M_sorted.unique_indices)
|
|
self.assertEqual(True, M_sorted.indices_sorted)
|
|
|
|
indices = M_sorted.indices
|
|
if indices.size > 0:
|
|
flatind = indices.reshape(-1, *indices.shape[-2:]).transpose(0, 2, 1)
|
|
sorted = jax.vmap(jnp.lexsort)(flatind[:, ::-1])
|
|
self.assertArraysEqual(sorted, lax.broadcasted_iota(sorted.dtype, sorted.shape, sorted.ndim - 1))
|
|
|
|
@jtu.sample_product(
|
|
[
|
|
dict(shape=shape, n_batch=layout.n_batch, n_dense=layout.n_dense)
|
|
for shape in [(5,), (5, 8), (8, 5), (3, 4, 5), (3, 4, 3, 2)]
|
|
for layout in sptu.iter_sparse_layouts(shape, min_n_batch=1)
|
|
],
|
|
dtype=jtu.dtypes.floating + jtu.dtypes.complex,
|
|
)
|
|
def test_bcoo_sort_indices_batching(self, shape, dtype, n_batch, n_dense):
|
|
rng_sparse = sptu.rand_sparse(self.rng(), rand_method=jtu.rand_some_zero)
|
|
M = sparse.BCOO.fromdense(rng_sparse(shape, dtype), n_batch=n_batch, n_dense=n_dense)
|
|
M.indices = M.indices[..., ::-1, :]
|
|
M.indices_sorted = False
|
|
|
|
identity = lambda M: M
|
|
sort_ind = lambda M: M.sort_indices()
|
|
for b in range(n_batch):
|
|
identity = jax.vmap(identity, in_axes=b)
|
|
sort_ind = jax.vmap(sort_ind, in_axes=b)
|
|
M_sorted = sort_ind(M)
|
|
M_expected = identity(M)
|
|
self.assertArraysEqual(M_expected.todense(), M_sorted.todense())
|
|
self.assertEqual(M.unique_indices, M_sorted.unique_indices)
|
|
self.assertEqual(True, M_sorted.indices_sorted)
|
|
|
|
@jtu.sample_product(
|
|
[
|
|
dict(shape=shape, n_batch=layout.n_batch, n_dense=layout.n_dense)
|
|
for shape in [(5,), (5, 8), (8, 5), (3, 4, 5), (3, 4, 3, 2)]
|
|
for layout in sptu.iter_sparse_layouts(shape)
|
|
],
|
|
dtype=jtu.dtypes.floating,
|
|
)
|
|
def test_bcoo_sort_indices_ad(self, shape, dtype, n_batch, n_dense):
|
|
rng_sparse = sptu.rand_sparse(self.rng(), rand_method=jtu.rand_some_zero)
|
|
M = sparse.BCOO.fromdense(rng_sparse(shape, dtype), n_batch=n_batch, n_dense=n_dense)
|
|
M.indices = M.indices[..., ::-1, :]
|
|
|
|
def sort_indices(data):
|
|
return sparse.BCOO((data, M.indices), shape=M.shape).sort_indices().data
|
|
|
|
data_dot_fwd = jax.jacfwd(sort_indices)(M.data)
|
|
data_dot_rev = jax.jacrev(sort_indices)(M.data)
|
|
|
|
self.assertAllClose(data_dot_fwd, data_dot_rev)
|
|
|
|
def test_bcoo_sort_indices_broadcasted(self):
|
|
rng_index = jtu.rand_int(self.rng(), low=0, high=10)
|
|
rng_data = jtu.rand_default(self.rng())
|
|
|
|
# Construct matrix with three broadcasted batch dimensions.
|
|
indices = rng_index((1, 3, 1, 10, 2), dtype='int32')
|
|
data = rng_data((1, 1, 4, 10, 3), dtype='int32')
|
|
shape = (2, 3, 4, 5, 4, 3)
|
|
mat = sparse.BCOO((data, indices), shape=shape)
|
|
|
|
indices_shape_out = indices.shape
|
|
data_shape_out = (*map(max, indices.shape[:3], data.shape[:3]), *data.shape[3:])
|
|
|
|
mat_sorted = sparse.bcoo_sort_indices(mat)
|
|
assert mat_sorted.indices.shape == indices_shape_out
|
|
assert mat_sorted.data.shape == data_shape_out
|
|
self.assertArraysEqual(mat.todense(), mat_sorted.todense())
|
|
|
|
mat_sorted_jit = jit(sparse.bcoo_sort_indices)(mat)
|
|
assert mat_sorted_jit.indices.shape == indices_shape_out
|
|
assert mat_sorted_jit.data.shape == data_shape_out
|
|
self.assertArraysEqual(mat.todense(), mat_sorted_jit.todense())
|
|
|
|
def test_bcoo_sum_duplicates_inferred_nse(self):
|
|
x = sparse.BCOO.fromdense(jnp.diag(jnp.arange(4)))
|
|
self.assertEqual(x.nse, 3)
|
|
y = x + x.T
|
|
self.assertEqual(y.nse, 6)
|
|
y2 = y.sum_duplicates()
|
|
self.assertEqual(y2.nse, 3)
|
|
self.assertArraysEqual(y.todense(), y2.todense())
|
|
|
|
def test_bcoo_sum_duplicates_remove_zeros(self):
|
|
data = jnp.array([0, 1, 0, 0])
|
|
indices = jnp.array([[0], [1], [2], [3]])
|
|
x = sparse.BCOO((data, indices), shape=(4,))
|
|
self.assertEqual(x.nse, 4)
|
|
|
|
y1 = x.sum_duplicates(remove_zeros=True)
|
|
self.assertArraysEqual(x.todense(), y1.todense())
|
|
self.assertEqual(y1.nse, 1)
|
|
|
|
y2 = x.sum_duplicates(remove_zeros=False)
|
|
self.assertArraysEqual(x.todense(), y2.todense())
|
|
self.assertEqual(y2.nse, x.nse)
|
|
|
|
def test_bcoo_sum_duplicates_padding(self):
|
|
# Regression test for https://github.com/jax-ml/jax/issues/8163
|
|
size = 3
|
|
data = jnp.array([1, 0, 0])
|
|
indices = jnp.array([1, size, size])[:, None]
|
|
x = sparse.BCOO((data, indices), shape=(3,))
|
|
y = x.sum_duplicates(nse=x.nse)
|
|
self.assertArraysEqual(x.todense(), y.todense())
|
|
self.assertArraysEqual(x.indices, y.indices)
|
|
self.assertArraysEqual(x.data, y.data)
|
|
|
|
@jtu.sample_product(
|
|
[
|
|
dict(
|
|
shape=shape,
|
|
n_batch=layout.n_batch,
|
|
n_dense=layout.n_dense,
|
|
axes=axes,
|
|
)
|
|
for shape in [(5,), (5, 8), (8, 5), (3, 4, 5), (3, 4, 3, 2)]
|
|
for layout in sptu.iter_sparse_layouts(shape)
|
|
for naxes in range(len(shape))
|
|
for axes in itertools.combinations(range(len(shape)), naxes)
|
|
],
|
|
dtype=jtu.dtypes.floating + jtu.dtypes.complex,
|
|
)
|
|
def test_bcoo_reduce_sum(self, shape, dtype, n_batch, n_dense, axes):
|
|
sprng = sptu.rand_bcoo(self.rng(), n_batch=n_batch, n_dense=n_dense)
|
|
args_maker = lambda: [sprng(shape, dtype)]
|
|
sparse_fun = partial(sparse.bcoo_reduce_sum, axes=axes)
|
|
dense_fun = partial(lambda x: x.sum(axes))
|
|
|
|
tol = {np.float64: 1E-14}
|
|
self._CheckAgainstDense(dense_fun, sparse_fun, args_maker, tol=tol)
|
|
if jnp.issubdtype(dtype, jnp.floating):
|
|
self._CheckGradsSparse(dense_fun, sparse_fun, args_maker)
|
|
|
|
@jtu.sample_product(
|
|
[
|
|
dict(
|
|
shape=shape,
|
|
dimensions=dimensions,
|
|
n_batch=layout.n_batch,
|
|
n_dense=layout.n_dense,
|
|
)
|
|
for shape, dimensions in [
|
|
[(1,), (0,)],
|
|
[(1,), (-1,)],
|
|
[(2, 1, 4), (1,)],
|
|
[(2, 1, 3, 1), (1,)],
|
|
[(2, 1, 3, 1), (1, 3)],
|
|
[(2, 1, 3, 1), (3,)],
|
|
]
|
|
for layout in sptu.iter_sparse_layouts(shape)
|
|
],
|
|
dtype=jtu.dtypes.numeric,
|
|
)
|
|
def test_bcoo_squeeze(self, shape, dtype, dimensions, n_batch, n_dense):
|
|
sprng = sptu.rand_bcoo(self.rng(), n_batch=n_batch, n_dense=n_dense)
|
|
args_maker = lambda: [sprng(shape, dtype)]
|
|
dense_func = partial(lax.squeeze, dimensions=dimensions)
|
|
sparse_func = partial(sparse.bcoo_squeeze, dimensions=dimensions)
|
|
|
|
self._CheckAgainstDense(dense_func, sparse_func, args_maker)
|
|
if jnp.issubdtype(dtype, jnp.floating):
|
|
self._CheckGradsSparse(dense_func, sparse_func, args_maker)
|
|
|
|
@jtu.sample_product(
|
|
[
|
|
dict(batch_shapes=shapes, batch_perm=perm)
|
|
for shapes in COMPATIBLE_SHAPE_PAIRS
|
|
for perm in itertools.permutations(range(len(shapes[0])))
|
|
],
|
|
[
|
|
dict(sparse_shapes=shapes, sparse_perm=perm)
|
|
for shapes in COMPATIBLE_SHAPE_PAIRS
|
|
for perm in itertools.permutations(range(len(shapes[0])))
|
|
],
|
|
[
|
|
dict(dense_shapes=shapes, dense_perm=perm)
|
|
for shapes in [[(), ()]] # TODO(jakevdp) add support for dense shapes
|
|
for perm in itertools.permutations(range(len(shapes[0])))
|
|
],
|
|
dtype=jtu.dtypes.numeric,
|
|
)
|
|
def test_bcoo_reshape(
|
|
self,
|
|
batch_shapes,
|
|
sparse_shapes,
|
|
dense_shapes,
|
|
batch_perm,
|
|
sparse_perm,
|
|
dense_perm,
|
|
dtype,
|
|
):
|
|
# Sparse reshapes cannot mix between sparse, dense, and batch dimensions.
|
|
shape = (*batch_shapes[0], *sparse_shapes[0], *dense_shapes[0])
|
|
new_sizes = (*batch_shapes[1], *sparse_shapes[1], *dense_shapes[1])
|
|
n_batch = len(batch_shapes[0])
|
|
n_sparse = len(sparse_shapes[0])
|
|
n_dense = len(dense_shapes[0])
|
|
dimensions = (
|
|
*batch_perm,
|
|
*(dim + n_batch for dim in sparse_perm),
|
|
*(dim + n_batch + n_sparse for dim in dense_perm),
|
|
)
|
|
|
|
rng = sptu.rand_bcoo(self.rng(), n_batch=n_batch, n_dense=n_dense)
|
|
args_maker = lambda: [rng(shape, dtype)]
|
|
|
|
sparse_func = partial(sparse.bcoo_reshape, new_sizes=new_sizes, dimensions=dimensions)
|
|
dense_func = partial(lax.reshape, new_sizes=new_sizes, dimensions=dimensions)
|
|
|
|
self._CheckAgainstDense(dense_func, sparse_func, args_maker)
|
|
if jnp.issubdtype(dtype, jnp.floating):
|
|
self._CheckGradsSparse(dense_func, sparse_func, args_maker)
|
|
|
|
def test_bcoo_reshape_error(self):
|
|
x = sparse.BCOO.fromdense(jnp.ones((2, 2, 3)), n_batch=1)
|
|
with self.assertRaisesRegex(ValueError, ".*cannot mix batch and sparse dimensions.*"):
|
|
x.reshape(3, 2, 2)
|
|
y = sparse.BCOO((x.data[:1], x.indices), shape=x.shape)
|
|
with self.assertRaisesRegex(NotImplementedError, "reshape of arrays with broadcasted batch dimensions."):
|
|
y.reshape(2, 3, 2)
|
|
|
|
@jtu.sample_product(
|
|
[
|
|
dict(
|
|
shape=shape,
|
|
dimensions=dimensions,
|
|
n_batch=layout.n_batch,
|
|
n_dense=layout.n_dense,
|
|
)
|
|
for shape in [(3,), (3, 4), (3, 4, 5)]
|
|
for dimensions in sptu.iter_subsets(range(len(shape)))
|
|
for layout in sptu.iter_sparse_layouts(shape)
|
|
],
|
|
dtype=jtu.dtypes.numeric,
|
|
)
|
|
def test_bcoo_rev(self, shape, dtype, n_batch, n_dense, dimensions):
|
|
sprng = sptu.rand_bcoo(self.rng(), n_batch=n_batch, n_dense=n_dense)
|
|
args_maker = lambda: [sprng(shape, dtype)]
|
|
dense_func = partial(lax.rev, dimensions=dimensions)
|
|
sparse_func = partial(sparse.bcoo_rev, dimensions=dimensions)
|
|
|
|
self._CheckAgainstDense(dense_func, sparse_func, args_maker)
|
|
if jnp.issubdtype(dtype, jnp.floating):
|
|
self._CheckGradsSparse(dense_func, sparse_func, args_maker)
|
|
|
|
def test_bcsr_matmul_with_out_of_bounds_data(self):
|
|
# Simple regression test of a failure mode for cuSparse.
|
|
data = jnp.array([1, 2, 3, 4], dtype='float32')
|
|
indices = jnp.array([0, 1, 2, 3])
|
|
indptr = jnp.array([0, 1, 3, 3])
|
|
M = sparse.BCSR((data, indices, indptr), shape=(3, 4))
|
|
x = jnp.array([1, 2, 3, 4], dtype='float32')
|
|
|
|
sparse_result = jax.jit(operator.matmul)(M, x)
|
|
dense_result = jax.jit(operator.matmul)(M.todense(), x)
|
|
self.assertAllClose(sparse_result, dense_result)
|
|
|
|
@jtu.sample_product(
|
|
[
|
|
dict(lhs_shape=lhs_shape, rhs_shape=rhs_shape)
|
|
for lhs_shape, rhs_shape in [
|
|
[(3, 4), (4,)],
|
|
[(3, 4), (4, 5)],
|
|
[(3, 4), (2, 4, 5)],
|
|
]
|
|
],
|
|
lhs_dtype=all_dtypes,
|
|
rhs_dtype=all_dtypes,
|
|
)
|
|
@jax.default_matmul_precision("float32")
|
|
@jtu.ignore_warning(category=sparse.CuSparseEfficiencyWarning)
|
|
def test_bcsr_matmul(self, lhs_shape, lhs_dtype, rhs_shape, rhs_dtype):
|
|
# Note: currently, batch dimensions in matmul must correspond to batch
|
|
# dimensions in the sparse representation.
|
|
n_batch_lhs = max(0, len(lhs_shape) - 2)
|
|
|
|
rng = jtu.rand_default(self.rng())
|
|
sprng = sptu.rand_bcsr(self.rng())
|
|
args_maker = lambda: [sprng(lhs_shape, lhs_dtype, n_batch=n_batch_lhs),
|
|
jnp.array(rng(rhs_shape, rhs_dtype))]
|
|
|
|
tol = {np.float64: 1E-7, np.complex128: 1E-6,
|
|
np.float32: 2E-6, np.complex64: 2E-6}
|
|
|
|
with jtu.strict_promotion_if_dtypes_match([lhs_dtype, rhs_dtype]):
|
|
self._CheckAgainstDense(operator.matmul, operator.matmul, args_maker,
|
|
tol=tol)
|
|
|
|
@jtu.sample_product(
|
|
[
|
|
dict(lhs_shape=lhs_shape, rhs_shape=rhs_shape)
|
|
for lhs_shape, rhs_shape in [
|
|
[(3,), (3,)],
|
|
[(3, 4), (4,)],
|
|
[(4,), (4, 5)],
|
|
[(3, 4), (4, 5)],
|
|
[(3, 4), (2, 4, 5)],
|
|
[(2, 3, 4), (4, 5)],
|
|
[(2, 3, 4), (2, 4, 5)],
|
|
]
|
|
],
|
|
lhs_dtype=all_dtypes,
|
|
rhs_dtype=all_dtypes,
|
|
)
|
|
@jax.default_matmul_precision("float32")
|
|
@jtu.ignore_warning(category=sparse.CuSparseEfficiencyWarning)
|
|
def test_bcoo_matmul(self, lhs_shape, lhs_dtype, rhs_shape, rhs_dtype):
|
|
if (jtu.test_device_matches(["cuda"]) and
|
|
_is_required_cuda_version_satisfied(12000)):
|
|
raise unittest.SkipTest("Triggers a bug in cuda-12 b/287344632")
|
|
|
|
# Note: currently, batch dimensions in matmul must correspond to batch
|
|
# dimensions in the sparse representation.
|
|
n_batch_lhs = max(0, len(lhs_shape) - 2)
|
|
n_batch_rhs = max(0, len(rhs_shape) - 2)
|
|
|
|
rng = jtu.rand_default(self.rng())
|
|
sprng = sptu.rand_bcoo(self.rng())
|
|
args_maker_de_sp = lambda: [jnp.array(rng(lhs_shape, lhs_dtype)),
|
|
sprng(rhs_shape, rhs_dtype, n_batch=n_batch_rhs)]
|
|
args_maker_sp_de = lambda: [sprng(lhs_shape, lhs_dtype, n_batch=n_batch_lhs),
|
|
jnp.array(rng(rhs_shape, rhs_dtype))]
|
|
|
|
tol = {np.float64: 1E-7, np.complex128: 1E-7,
|
|
np.float32: 1E-6, np.complex64: 1E-6}
|
|
|
|
with jtu.strict_promotion_if_dtypes_match([lhs_dtype, rhs_dtype]):
|
|
self._CheckAgainstDense(operator.matmul, operator.matmul, args_maker_de_sp, tol=tol)
|
|
self._CheckAgainstDense(operator.matmul, operator.matmul, args_maker_sp_de, tol=tol)
|
|
|
|
@jtu.sample_product(
|
|
[
|
|
dict(
|
|
lhs_shape=lhs_shape,
|
|
rhs_shape=rhs_shape,
|
|
n_batch=layout.n_batch,
|
|
n_dense=layout.n_dense,
|
|
)
|
|
for lhs_shape, rhs_shape in [
|
|
[(3,), ()],
|
|
[(3,), (1,)],
|
|
[(3,), (3,)],
|
|
[(3, 4), ()],
|
|
[(3, 4), (4,)],
|
|
[(3, 4), (3, 1)],
|
|
[(3, 4), (3, 4)],
|
|
[(3, 4, 5), (4, 5)],
|
|
[(3, 4, 5), (3, 1, 1)],
|
|
[(3, 4, 5), (1, 4, 1)],
|
|
]
|
|
for layout in sptu.iter_sparse_layouts(lhs_shape)
|
|
],
|
|
lhs_dtype=all_dtypes,
|
|
rhs_dtype=all_dtypes,
|
|
)
|
|
@jax.numpy_rank_promotion(
|
|
"allow"
|
|
) # This test explicitly exercises implicit rank promotion.
|
|
def test_bcoo_mul_dense(self, lhs_shape, lhs_dtype, rhs_shape, rhs_dtype, n_batch, n_dense):
|
|
rng = jtu.rand_default(self.rng())
|
|
sprng = sptu.rand_bcoo(self.rng(), n_batch=n_batch, n_dense=n_dense)
|
|
|
|
args_maker_sp_de = lambda: [sprng(lhs_shape, lhs_dtype), jnp.array(rng(rhs_shape, rhs_dtype))]
|
|
args_maker_de_sp = lambda: [jnp.array(rng(rhs_shape, rhs_dtype)), sprng(lhs_shape, lhs_dtype)]
|
|
|
|
tol = {np.float64: 1E-13, np.complex128: 1E-13,
|
|
np.float32: 1E-6, np.complex64: 1E-6}
|
|
|
|
with jtu.strict_promotion_if_dtypes_match([lhs_dtype, rhs_dtype]):
|
|
self._CheckAgainstDense(operator.mul, operator.mul, args_maker_de_sp, tol=tol)
|
|
self._CheckAgainstDense(operator.mul, operator.mul, args_maker_sp_de, tol=tol)
|
|
|
|
@jtu.sample_product(
|
|
[
|
|
dict(
|
|
lhs_shape=lhs_shape,
|
|
rhs_shape=rhs_shape,
|
|
lhs_n_batch=lhs_n_batch,
|
|
rhs_n_batch=rhs_n_batch,
|
|
n_dense=n_dense,
|
|
)
|
|
# TODO(jakevdp): add broadcasted shapes (from bcoo_mul_dense) once sparse-sparse mul
|
|
# supports inputs of differing rank.
|
|
for lhs_shape, rhs_shape in [
|
|
[(3,), (1,)],
|
|
[(3,), (3,)],
|
|
[(3, 4), (1, 1)],
|
|
[(3, 4), (1, 4)],
|
|
[(3, 4), (3, 1)],
|
|
[(3, 4), (3, 4)],
|
|
[(3, 4, 5), (1, 4, 5)],
|
|
[(3, 4, 5), (3, 1, 1)],
|
|
[(3, 4, 5), (1, 4, 1)],
|
|
]
|
|
# TODO(jakevdp): add tests for batch & dense dimensions.
|
|
for lhs_n_batch in range(len(lhs_shape) + 1)
|
|
for rhs_n_batch in range(len(lhs_shape) + 1)
|
|
for n_dense in range(
|
|
len(lhs_shape) + 1 - max(lhs_n_batch, rhs_n_batch)
|
|
)
|
|
],
|
|
lhs_dtype=all_dtypes,
|
|
rhs_dtype=all_dtypes,
|
|
)
|
|
def test_bcoo_mul_sparse(self, lhs_shape, lhs_dtype, rhs_shape, rhs_dtype, lhs_n_batch, rhs_n_batch, n_dense):
|
|
sprng = sptu.rand_bcoo(self.rng(), n_dense=n_dense)
|
|
args_maker = lambda: [sprng(lhs_shape, lhs_dtype, n_batch=lhs_n_batch),
|
|
sprng(rhs_shape, rhs_dtype, n_batch=rhs_n_batch)]
|
|
|
|
tol = {np.float64: 1E-13, np.complex128: 1E-13,
|
|
np.float32: 1E-5, np.complex64: 1E-5}
|
|
|
|
with jtu.strict_promotion_if_dtypes_match([lhs_dtype, rhs_dtype]):
|
|
self._CheckAgainstDense(operator.mul, operator.mul, args_maker, tol=tol)
|
|
|
|
def test_bcoo_mul_sparse_with_duplicates(self):
|
|
# Regression test for https://github.com/jax-ml/jax/issues/8888
|
|
indices = jnp.array([[0, 1, 0, 0, 1, 1],
|
|
[1, 0, 1, 2, 0, 2]]).T
|
|
data = jnp.array([1, 2, 3, 4, 5, 6])
|
|
mat = sparse.BCOO((data, indices), shape=(3, 3))
|
|
self.assertArraysEqual((mat * mat).todense(), mat.todense() * mat.todense())
|
|
|
|
@jtu.sample_product(
|
|
[
|
|
dict(shape=shape, n_batch=layout.n_batch, n_dense=layout.n_dense)
|
|
for shape in [(), (3,), (3, 5), (3, 5, 4)]
|
|
for layout in sptu.iter_sparse_layouts(shape)
|
|
],
|
|
dtype=all_dtypes,
|
|
)
|
|
def test_bcoo_broadcast_in_dim(self, shape, dtype, n_batch, n_dense):
|
|
rng = sptu.rand_sparse(self.rng())
|
|
x = jnp.array(rng(shape, dtype))
|
|
xsp = sparse.BCOO.fromdense(x, n_batch=n_batch, n_dense=n_dense)
|
|
|
|
self.assertEqual(xsp[None].n_batch, xsp.n_batch + 1)
|
|
self.assertArraysEqual(xsp[None].todense(), x[None])
|
|
|
|
if len(shape) >= 1:
|
|
self.assertEqual(xsp[:, None].n_batch, xsp.n_batch if xsp.n_batch < 1 else xsp.n_batch + 1)
|
|
self.assertArraysEqual(xsp[:, None].todense(), x[:, None])
|
|
self.assertArraysEqual(xsp[:, None, None].todense(), x[:, None, None])
|
|
if len(shape) >= 2:
|
|
self.assertEqual(xsp[:, :, None].n_batch, xsp.n_batch if xsp.n_batch < 2 else xsp.n_batch + 1)
|
|
self.assertArraysEqual(xsp[:, :, None].todense(), x[:, :, None])
|
|
self.assertArraysEqual(xsp[:, None, :, None].todense(), x[:, None, :, None])
|
|
|
|
@jtu.sample_product(
|
|
[
|
|
dict(
|
|
shape=shape,
|
|
n_batch=layout.n_batch,
|
|
n_dense=layout.n_dense,
|
|
dimension=dimension,
|
|
)
|
|
for shape in [(3,), (3, 5), (3, 5, 4)]
|
|
for layout in sptu.iter_sparse_layouts(shape)
|
|
for dimension in range(
|
|
len(shape) - layout.n_dense
|
|
) # Concatenation of dense dimensions not implemented.
|
|
],
|
|
dtype=all_dtypes,
|
|
)
|
|
def test_bcoo_concatenate(self, shape, dtype, n_batch, n_dense, dimension):
|
|
sprng = sptu.rand_bcoo(self.rng(), n_batch=n_batch, n_dense=n_dense)
|
|
args_maker = lambda: [[sprng(shape, dtype) for i in range(3)]]
|
|
dense_func = partial(lax.concatenate, dimension=dimension)
|
|
sparse_func = partial(sparse.bcoo_concatenate, dimension=dimension)
|
|
|
|
self._CheckAgainstDense(dense_func, sparse_func, args_maker)
|
|
if jnp.issubdtype(dtype, jnp.floating):
|
|
self._CheckGradsSparse(dense_func, sparse_func, args_maker)
|
|
|
|
@jtu.sample_product(
|
|
lhs_shape=[(1, 1, 5), (1, 1, 10)],
|
|
rhs_shape=[(1, 1, 5), (1, 1, 10)],
|
|
padding=["SAME", "VALID", [(3, 3)]],
|
|
dtype=jtu.dtypes.inexact,
|
|
format=["sp-de", "de-sp", "sp-sp"],
|
|
)
|
|
@jax.default_matmul_precision("float32")
|
|
@jtu.skip_on_flag("jax_skip_slow_tests", True)
|
|
def test_bcoo_conv_general_dilated(self, lhs_shape, rhs_shape, dtype, padding, format):
|
|
kwds = dict(window_strides=(1,), padding=padding)
|
|
sparse_fun = partial(sparse.bcoo_conv_general_dilated, **kwds)
|
|
dense_fun = partial(lax.conv_general_dilated, **kwds)
|
|
sprng = sptu.rand_bcoo(self.rng(), n_batch=2, n_dense=0)
|
|
rng = jtu.rand_default(self.rng())
|
|
|
|
def args_maker():
|
|
lhs = (sprng if format.startswith('sp') else rng)(lhs_shape, dtype)
|
|
rhs = (sprng if format.endswith('sp') else rng)(rhs_shape, dtype)
|
|
return lhs, rhs
|
|
|
|
tol = {np.float32: 1E-5, np.complex64: 1E-5, np.float64: 1E-14, np.complex128: 1E-14}
|
|
self._CheckAgainstDense(dense_fun, sparse_fun, args_maker, tol=tol)
|
|
|
|
def test_bcoo_vmap_shape(self, shape=(2, 3, 4, 5), dtype=np.float32):
|
|
# This test checks that BCOO shape metadata interacts correctly with vmap.
|
|
rng = sptu.rand_sparse(self.rng())
|
|
M = rng(shape, dtype)
|
|
|
|
def make_bcoo(M):
|
|
return sparse_bcoo._bcoo_fromdense(M, nse=math.prod(M.shape[:-1]), n_dense=1)
|
|
|
|
todense = partial(sparse_bcoo._bcoo_todense, spinfo=sparse_util.SparseInfo(shape))
|
|
|
|
for _ in range(3):
|
|
make_bcoo = jax.vmap(make_bcoo)
|
|
Msp_data, Msp_indices = make_bcoo(M)
|
|
Msp_dense = todense(Msp_data, Msp_indices)
|
|
self.assertEqual(Msp_dense.shape, M.shape)
|
|
self.assertArraysEqual(Msp_dense, M)
|
|
|
|
@jtu.sample_product(
|
|
[
|
|
dict(
|
|
shape=shape,
|
|
n_batch=layout.n_batch,
|
|
n_dense=layout.n_dense,
|
|
n_batch_out=layout_out.n_batch,
|
|
n_dense_out=layout_out.n_dense,
|
|
)
|
|
for shape in [(5,), (5, 8), (8, 5), (3, 4, 5), (3, 4, 3, 2)]
|
|
for layout in sptu.iter_sparse_layouts(shape)
|
|
for layout_out in sptu.iter_sparse_layouts(shape)
|
|
],
|
|
dtype=jtu.dtypes.integer,
|
|
)
|
|
def test_bcoo_update_layout(self, shape, dtype, n_batch, n_batch_out, n_dense, n_dense_out):
|
|
rng = sptu.rand_sparse(self.rng())
|
|
mat = sparse.BCOO.fromdense(rng(shape, dtype), n_batch=n_batch, n_dense=n_dense)
|
|
kwds = dict(n_batch=n_batch_out, n_dense=n_dense_out)
|
|
# TODO(jakevdp): in case of length-0 or length-1 shapes errors/warnings will not be raised.
|
|
if n_dense_out > n_dense or n_batch_out > n_batch:
|
|
with self.assertRaises(sparse.SparseEfficiencyError):
|
|
sparse.bcoo_update_layout(mat, **kwds)
|
|
with self.assertRaises(sparse.SparseEfficiencyError):
|
|
sparse.bcoo_update_layout(mat, **kwds, on_inefficient='error')
|
|
with self.assertWarns(sparse.SparseEfficiencyWarning):
|
|
sparse.bcoo_update_layout(mat, **kwds, on_inefficient='warn')
|
|
kwds['on_inefficient'] = None
|
|
mat_new = sparse.bcoo_update_layout(mat, **kwds)
|
|
self.assertEqual(mat_new.n_batch, n_batch_out)
|
|
self.assertEqual(mat_new.n_dense, n_dense_out)
|
|
self.assertArraysEqual(mat.todense(), mat_new.todense())
|
|
|
|
def test_bcoo_update_layout_method(self, shape=(2, 3, 4)):
|
|
# simple test to make sure update_layout method properly forwards.
|
|
rng = sptu.rand_sparse(self.rng())
|
|
mat = sparse.BCOO.fromdense(rng((2, 3, 4), 'float32'), n_batch=1, n_dense=1)
|
|
mat_new = mat.update_layout(n_batch=0, n_dense=0)
|
|
self.assertEqual(mat_new.n_batch, 0)
|
|
self.assertEqual(mat_new.n_dense, 0)
|
|
self.assertArraysEqual(mat.todense(), mat_new.todense())
|
|
|
|
def test_bcoo_bad_fillvals(self):
|
|
# Extra values have 100 rather than zero. This lets us check that logic is
|
|
# properly ignoring these indices.
|
|
data = jnp.array([1, 2, 3, 100, 100])
|
|
indices = jnp.array([1, 2, 3, 5, 5])[:, None]
|
|
x_sp = sparse.BCOO((data, indices), shape=(5,))
|
|
x_de = x_sp.todense()
|
|
|
|
data = jnp.array([3, 2, 100, 100])
|
|
indices = jnp.array([2, 3, 5, 5])[:, None]
|
|
y_sp = sparse.BCOO((data, indices), shape=(5,))
|
|
y_de = y_sp.todense()
|
|
|
|
self.assertArraysEqual(x_de, jnp.array([0, 1, 2, 3, 0]))
|
|
self.assertArraysEqual(y_de, jnp.array([0, 0, 3, 2, 0]))
|
|
|
|
self.assertArraysEqual(x_sp.sum_duplicates().todense(), x_de)
|
|
self.assertArraysEqual(y_sp.sum_duplicates().todense(), y_de)
|
|
|
|
# reduce_sum:
|
|
self.assertArraysEqual(x_sp.sum(), x_de.sum())
|
|
|
|
# bcoo_dot_general
|
|
self.assertArraysEqual(x_sp @ y_de, x_de @ y_de)
|
|
|
|
# bcoo_rdot_general
|
|
self.assertArraysEqual(x_de @ y_sp, x_de @ y_de)
|
|
|
|
# bcoo_spdot_general
|
|
self.assertArraysEqual((x_sp @ y_sp).todense(), x_de @ y_de)
|
|
self.assertArraysEqual((y_sp @ x_sp).todense(), y_de @ x_de)
|
|
|
|
|
|
# TODO(tianjianlu): Unify the testing for BCOOTest and BCSRTest.
|
|
class BCSRTest(sptu.SparseTestCase):
|
|
|
|
@jtu.sample_product(
|
|
[
|
|
dict(shape=shape, n_batch=layout.n_batch, n_dense=layout.n_dense)
|
|
for shape in [(5, 8), (8, 5), (3, 4, 5), (3, 4, 3, 2)]
|
|
for layout in sptu.iter_bcsr_layouts(shape)
|
|
],
|
|
dtype=all_dtypes,
|
|
)
|
|
def test_bcsr_dense_round_trip(self, shape, dtype, n_batch, n_dense):
|
|
n_sparse = len(shape) - n_batch - n_dense
|
|
rng = sptu.rand_sparse(self.rng())
|
|
M = rng(shape, dtype)
|
|
nse = sparse.util._count_stored_elements(M, n_batch=n_batch, n_dense=n_dense)
|
|
def round_trip(M):
|
|
return sparse.BCSR.fromdense(M, nse=nse, n_batch=n_batch, n_dense=n_dense).todense()
|
|
args_maker = lambda: [M]
|
|
ident = lambda x: x
|
|
|
|
self._CheckAgainstNumpy(ident, round_trip, args_maker)
|
|
self._CompileAndCheck(round_trip, args_maker)
|
|
self._CheckBatchingSparse(ident, round_trip, args_maker, bdims=self._random_bdims(n_batch))
|
|
if jnp.issubdtype(dtype, jnp.floating):
|
|
# For n_sparse != 0, we can't use an identity because output zeros must not
|
|
# be dependent on input zeros. This mimics the code in count_stored_elements().
|
|
def expected(M):
|
|
if n_sparse == 0: return M
|
|
mask = (M != 0).any(range(M.ndim - n_dense, M.ndim), keepdims=True)
|
|
return jnp.where(mask, M, 0)
|
|
self._CheckGradsSparse(expected, round_trip, args_maker)
|
|
|
|
@jtu.sample_product(
|
|
[
|
|
dict(shape=shape, n_batch=n_batch)
|
|
for shape in [(5, 8), (8, 5), (3, 4, 5), (3, 4, 3, 2)]
|
|
for n_batch in range(len(shape) - 1)
|
|
],
|
|
dtype=jtu.dtypes.floating + jtu.dtypes.complex,
|
|
)
|
|
def test_bcsr_bcoo_round_trip(self, shape, n_batch, dtype):
|
|
n_sparse = 2
|
|
n_dense = len(shape) - n_sparse - n_batch
|
|
rng = self.rng()
|
|
sprng = sptu.rand_bcsr(rng, n_batch=n_batch, n_dense=n_dense)
|
|
|
|
M_bcsr = sprng(shape, dtype)
|
|
self.assertIsInstance(M_bcsr, sparse.BCSR)
|
|
|
|
M_dense = M_bcsr.todense()
|
|
M_bcoo = M_bcsr.to_bcoo()
|
|
self.assertIsInstance(M_bcoo, sparse.BCOO)
|
|
self.assertAllClose(M_dense, M_bcoo.todense())
|
|
|
|
M_bcsr2 = sparse.BCSR.from_bcoo(M_bcoo)
|
|
self.assertAllClose(M_dense, M_bcsr2.todense())
|
|
self.assertArraysEqual(M_bcsr.indptr, M_bcsr2.indptr)
|
|
|
|
# TODO(jakevdp): This will only be true in general when M_bcsr.indices is sorted.
|
|
# self.assertSparseArraysEquivalent(M_bcsr, M_bcsr2)
|
|
|
|
@jtu.sample_product(
|
|
[
|
|
dict(shape=shape, n_batch=n_batch)
|
|
for shape in [(5, 8), (8, 5), (3, 4, 5), (3, 4, 3, 2)]
|
|
for n_batch in range(len(shape) - 1)
|
|
],
|
|
dtype=jtu.dtypes.floating + jtu.dtypes.complex,
|
|
)
|
|
def test_bcsr_extract(self, shape, dtype, n_batch):
|
|
n_dense = len(shape) - n_batch - 2
|
|
rng = sptu.rand_sparse(self.rng())
|
|
M = rng(shape, dtype)
|
|
nse = sparse.util._count_stored_elements(M, n_batch=n_batch,
|
|
n_dense=n_dense)
|
|
data, indices, indptr = sparse_bcsr._bcsr_fromdense(
|
|
M, nse=nse, n_batch=n_batch, n_dense=n_dense)
|
|
data2 = sparse.bcsr_extract(indices, indptr, M)
|
|
self.assertArraysEqual(data, data2)
|
|
args_maker_bcsr_extract = lambda: [indices, indptr, M]
|
|
self._CompileAndCheck(sparse.bcsr_extract, args_maker_bcsr_extract)
|
|
|
|
@jtu.sample_product(
|
|
props=_generate_batched_dot_general_properties(
|
|
shapes=((2, 3), (2, 3, 4), (2, 3, 4, 4)), sparse_format="bcsr"
|
|
),
|
|
dtype=jtu.dtypes.floating + jtu.dtypes.complex,
|
|
)
|
|
@jax.default_matmul_precision("float32")
|
|
def test_bcsr_dot_general(
|
|
self, dtype: np.dtype, props: sptu.BatchedDotGeneralProperties
|
|
):
|
|
rng = jtu.rand_default(self.rng())
|
|
sprng = sptu.rand_bcsr(self.rng(), n_batch=props.n_batch, n_dense=props.n_dense)
|
|
args_maker = lambda: [sprng(props.lhs_shape, dtype),
|
|
rng(props.rhs_shape, dtype)]
|
|
dense_fun = partial(lax.dot_general,
|
|
dimension_numbers=props.dimension_numbers)
|
|
sparse_fun = partial(sparse.bcsr_dot_general,
|
|
dimension_numbers=props.dimension_numbers)
|
|
|
|
tol = {np.float64: 1E-12, np.complex128: 1E-12,
|
|
np.float32: 1E-5, np.complex64: 1E-5}
|
|
|
|
self._CheckAgainstDense(dense_fun, sparse_fun, args_maker, tol=tol)
|
|
if jnp.issubdtype(dtype, jnp.floating) and props.n_dense == 0:
|
|
# Dense dimensions not yet fully supported in reverse mode.
|
|
modes = ['fwd'] if props.n_dense != 0 else ['fwd', 'rev']
|
|
self._CheckGradsSparse(dense_fun, sparse_fun, args_maker, modes=modes, atol=tol, rtol=tol)
|
|
self._CheckBatchingSparse(dense_fun, sparse_fun, args_maker, atol=tol, rtol=tol,
|
|
bdims=self._random_bdims(props.n_batch, len(props.rhs_shape)))
|
|
|
|
@jtu.sample_product(
|
|
[
|
|
dict(shape=shape, n_batch=layout.n_batch, n_dense=layout.n_dense)
|
|
for shape in [(3, 5), (3, 5, 4)]
|
|
for layout in sptu.iter_bcsr_layouts(shape)
|
|
],
|
|
dtype=all_dtypes,
|
|
)
|
|
def test_bcsr_broadcast_in_dim(self, shape, dtype, n_batch, n_dense):
|
|
rng = sptu.rand_sparse(self.rng())
|
|
x = jnp.array(rng(shape, dtype))
|
|
xsp = sparse.BCSR.fromdense(x, n_batch=n_batch, n_dense=n_dense)
|
|
|
|
self.assertEqual(xsp[None].n_batch, xsp.n_batch + 1)
|
|
self.assertArraysEqual(xsp[None].todense(), x[None])
|
|
|
|
if n_batch == 1:
|
|
self.assertEqual(xsp[:, None].n_batch, xsp.n_batch + 1)
|
|
self.assertArraysEqual(xsp[:, None].todense(), x[:, None])
|
|
|
|
@jtu.sample_product(
|
|
[
|
|
dict(
|
|
shape=shape,
|
|
n_batch=layout.n_batch,
|
|
n_dense=layout.n_dense,
|
|
dimension=dimension,
|
|
)
|
|
for shape in [(3, 5), (3, 5, 4)]
|
|
for layout in sptu.iter_sparse_layouts(shape)
|
|
for dimension in range(
|
|
len(shape) - layout.n_dense
|
|
) # Concatenation of dense dimensions not implemented.
|
|
],
|
|
dtype=all_dtypes,
|
|
)
|
|
def test_bcsr_concatenate(self, shape, dtype, n_batch, n_dense, dimension):
|
|
sprng = sptu.rand_bcoo(self.rng(), n_batch=n_batch, n_dense=n_dense)
|
|
args_maker = lambda: [[sprng(shape, dtype) for i in range(3)]]
|
|
dense_func = partial(lax.concatenate, dimension=dimension)
|
|
sparse_func = partial(sparse.bcoo_concatenate, dimension=dimension)
|
|
|
|
self._CheckAgainstDense(dense_func, sparse_func, args_maker)
|
|
if jnp.issubdtype(dtype, jnp.floating):
|
|
self._CheckGradsSparse(dense_func, sparse_func, args_maker)
|
|
|
|
def test_bcoo_spdot_abstract_eval_bug(self):
|
|
# Regression test for https://github.com/jax-ml/jax/issues/21921
|
|
lhs = sparse.BCOO(
|
|
(jnp.float32([[1]]), lax.broadcasted_iota(jnp.int32, (10, 1, 1), 0)),
|
|
shape=(10, 10))
|
|
rhs = sparse.BCOO(
|
|
(jnp.float32([1]), jnp.int32([[3]])),
|
|
shape=(10,))
|
|
args_maker = lambda: [lhs, rhs]
|
|
def func(lhs, rhs):
|
|
return (lhs @ rhs).todense()
|
|
self._CompileAndCheck(func, args_maker)
|
|
|
|
if __name__ == "__main__":
|
|
absltest.main(testLoader=jtu.JaxTestLoader())
|