mirror of
https://github.com/ROCm/jax.git
synced 2025-04-17 20:36:05 +00:00
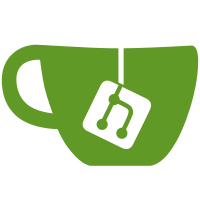
In most cases these are more succinct. This change does not update Pallas/Mosaic. PiperOrigin-RevId: 583448254
697 lines
26 KiB
Python
697 lines
26 KiB
Python
# Copyright 2023 The JAX Authors.
|
|
#
|
|
# Licensed under the Apache License, Version 2.0 (the "License");
|
|
# you may not use this file except in compliance with the License.
|
|
# You may obtain a copy of the License at
|
|
#
|
|
# https://www.apache.org/licenses/LICENSE-2.0
|
|
#
|
|
# Unless required by applicable law or agreed to in writing, software
|
|
# distributed under the License is distributed on an "AS IS" BASIS,
|
|
# WITHOUT WARRANTIES OR CONDITIONS OF ANY KIND, either express or implied.
|
|
# See the License for the specific language governing permissions and
|
|
# limitations under the License
|
|
|
|
""" Special functions
|
|
|
|
LAX decompositions for special functions into their StableHLO counterparts.
|
|
"""
|
|
|
|
from enum import Enum
|
|
import numpy as np
|
|
from functools import partial
|
|
|
|
from jax._src.lax.lax import (add, bitwise_and, bitwise_not, bitwise_or,
|
|
broadcast_in_dim, broadcast_shapes,
|
|
convert_element_type, div, eq, exp, full_like, ge,
|
|
gt, le, log, log1p, lt, mul, ne, neg, reciprocal,
|
|
reduce, select, sign, sqrt, square,
|
|
standard_naryop, standard_unop, sub,
|
|
_const, _dtype,
|
|
_float, _nary_lower_hlo, _ones, _isnan, _reduce)
|
|
from jax._src.lax.control_flow import while_loop
|
|
|
|
from jax._src import dtypes
|
|
from jax._src.interpreters import ad
|
|
from jax._src.interpreters import mlir
|
|
from jax._src.lib.mlir.dialects import chlo
|
|
from jax._src.typing import Array, ArrayLike
|
|
|
|
def betainc(a: ArrayLike, b: ArrayLike, x: ArrayLike) -> Array:
|
|
r"""Elementwise regularized incomplete beta integral."""
|
|
return regularized_incomplete_beta_p.bind(a, b, x)
|
|
|
|
def lgamma(x: ArrayLike) -> Array:
|
|
r"""Elementwise log gamma: :math:`\mathrm{log}(\Gamma(x))`."""
|
|
return lgamma_p.bind(x)
|
|
|
|
def digamma(x: ArrayLike) -> Array:
|
|
r"""Elementwise digamma: :math:`\psi(x)`."""
|
|
return digamma_p.bind(x)
|
|
|
|
def polygamma(m: ArrayLike, x: ArrayLike) -> Array:
|
|
r"""Elementwise polygamma: :math:`\psi^{(m)}(x)`."""
|
|
return polygamma_p.bind(m, x)
|
|
|
|
def igamma(a: ArrayLike, x: ArrayLike) -> Array:
|
|
r"""Elementwise regularized incomplete gamma function."""
|
|
return igamma_p.bind(a, x)
|
|
|
|
def igammac(a: ArrayLike, x: ArrayLike) -> Array:
|
|
r"""Elementwise complementary regularized incomplete gamma function."""
|
|
return igammac_p.bind(a, x)
|
|
|
|
def igamma_grad_a(a: ArrayLike, x: ArrayLike) -> Array:
|
|
r"""Elementwise derivative of the regularized incomplete gamma function."""
|
|
return igamma_grad_a_p.bind(a, x)
|
|
|
|
def random_gamma_grad(a: ArrayLike, x: ArrayLike) -> Array:
|
|
r"""Elementwise derivative of samples from `Gamma(a, 1)`."""
|
|
return random_gamma_grad_p.bind(a, x)
|
|
|
|
def zeta(x: ArrayLike, q: ArrayLike) -> Array:
|
|
r"""Elementwise Hurwitz zeta function: :math:`\zeta(x, q)`"""
|
|
return zeta_p.bind(x, q)
|
|
|
|
def bessel_i0e(x: ArrayLike) -> Array:
|
|
r"""Exponentially scaled modified Bessel function of order 0:
|
|
:math:`\mathrm{i0e}(x) = e^{-|x|} \mathrm{i0}(x)`
|
|
"""
|
|
return bessel_i0e_p.bind(x)
|
|
|
|
def bessel_i1e(x: ArrayLike) -> Array:
|
|
r"""Exponentially scaled modified Bessel function of order 1:
|
|
:math:`\mathrm{i1e}(x) = e^{-|x|} \mathrm{i1}(x)`
|
|
"""
|
|
return bessel_i1e_p.bind(x)
|
|
|
|
def erf(x: ArrayLike) -> Array:
|
|
r"""Elementwise error function: :math:`\mathrm{erf}(x)`."""
|
|
return erf_p.bind(x)
|
|
|
|
def erfc(x: ArrayLike) -> Array:
|
|
r"""Elementwise complementary error function:
|
|
:math:`\mathrm{erfc}(x) = 1 - \mathrm{erf}(x)`."""
|
|
return erfc_p.bind(x)
|
|
|
|
def erf_inv(x: ArrayLike) -> Array:
|
|
r"""Elementwise inverse error function: :math:`\mathrm{erf}^{-1}(x)`."""
|
|
return erf_inv_p.bind(x)
|
|
|
|
def betainc_gradx(g, a, b, x):
|
|
lbeta = lgamma(a) + lgamma(b) - lgamma(a + b)
|
|
partial_x = exp((b - 1) * log1p(-x) +
|
|
(a - 1) * log(x) - lbeta)
|
|
return partial_x * g
|
|
|
|
def betainc_grad_not_implemented(g, a, b, x):
|
|
raise ValueError("Betainc gradient with respect to a and b not supported.")
|
|
|
|
def igamma_gradx(g, a, x):
|
|
return g * exp(-x + (a - _ones(a)) * log(x) - lgamma(a))
|
|
|
|
def igamma_grada(g, a, x):
|
|
return g * igamma_grad_a(a, x)
|
|
|
|
def igammac_gradx(g, a, x):
|
|
return -igamma_gradx(g, a, x)
|
|
|
|
def igammac_grada(g, a, x):
|
|
return -igamma_grada(g, a, x)
|
|
|
|
def polygamma_gradm(g, m, x):
|
|
raise ValueError("polygamma gradient with respect to m is not supported")
|
|
|
|
def polygamma_gradx(g, m, x):
|
|
return g * polygamma(add(m, _const(m, 1)), x)
|
|
|
|
# The below is directly ported from tensorflow/compiler/xla/client/lib/math.cc
|
|
# We try to follow the corresponding functions as closely as possible, so that
|
|
# we can quickly incorporate changes.
|
|
def lentz_thompson_barnett_algorithm(*,num_iterations, small, threshold, nth_partial_numerator, nth_partial_denominator, inputs):
|
|
# Position in the evaluation.
|
|
kIterationIdx = 0
|
|
# Whether or not we have reached the desired tolerance.
|
|
kValuesUnconvergedIdx = 1
|
|
# Ratio between nth canonical numerator and the nth-1 canonical numerator.
|
|
kCIdx = 2
|
|
# Ratio between nth-1 canonical denominator and the nth canonical denominator.
|
|
kDIdx = 3
|
|
# Computed approximant in the evaluation.
|
|
kHIdx = 4
|
|
|
|
def while_cond_fn(values):
|
|
iteration = values[kIterationIdx]
|
|
iterations_remain_cond = lt(iteration, num_iterations)
|
|
values_unconverged_cond = values[kValuesUnconvergedIdx]
|
|
return bitwise_and(iterations_remain_cond, values_unconverged_cond)
|
|
|
|
def while_body_fn(values):
|
|
iteration = values[kIterationIdx]
|
|
partial_numerator = nth_partial_numerator(iteration, *inputs)
|
|
partial_denominator = nth_partial_denominator(iteration, *inputs)
|
|
|
|
c = add(partial_denominator, div(partial_numerator, values[kCIdx]))
|
|
small_constant = full_like(c, small)
|
|
c = select(lt(abs(c), small_constant), small_constant, c)
|
|
d = add(partial_denominator, mul(partial_numerator, values[kDIdx]))
|
|
d = select(lt(abs(d), small_constant), small_constant, d)
|
|
d = reciprocal(d)
|
|
delta = mul(c, d)
|
|
h = mul(values[kHIdx], delta)
|
|
|
|
# Update values
|
|
values[kIterationIdx] = iteration + 1
|
|
values[kCIdx] = c
|
|
values[kDIdx] = d
|
|
values[kHIdx] = h
|
|
# If any values are greater than the tolerance, we have not converged.
|
|
tolerance_comparison = ge(abs(sub(delta, _const(delta, 1.0))), threshold)
|
|
values[kValuesUnconvergedIdx] = _any(tolerance_comparison)
|
|
return values
|
|
|
|
partial_denominator = nth_partial_denominator(0, *inputs)
|
|
h = select(lt(abs(partial_denominator), small),
|
|
broadcast_in_dim(small, partial_denominator.shape, ()),
|
|
partial_denominator)
|
|
values = [1,True,h,full_like(h,0),h]
|
|
values = while_loop(while_cond_fn, while_body_fn, values)
|
|
return values[kHIdx]
|
|
|
|
|
|
def regularized_incomplete_beta_impl(a, b, x, *, dtype):
|
|
shape = a.shape
|
|
|
|
def nth_partial_betainc_numerator(iteration, a, b, x):
|
|
"""
|
|
The partial numerator for the incomplete beta function is given
|
|
here: http://dlmf.nist.gov/8.17.E23 Note that there is a special
|
|
case: the partial numerator for the first iteration is one.
|
|
"""
|
|
iteration_bcast = broadcast_in_dim(iteration, shape, [])
|
|
iteration_is_even = eq(iteration_bcast % full_like(iteration_bcast, 2),
|
|
full_like(iteration_bcast, 0))
|
|
iteration_is_one = eq(iteration_bcast, full_like(iteration_bcast, 1))
|
|
iteration_minus_one = iteration_bcast - full_like(iteration_bcast, 1)
|
|
m = iteration_minus_one // full_like(iteration_minus_one, 2)
|
|
m = convert_element_type(m, dtype)
|
|
one = full_like(a, 1)
|
|
two = full_like(a, 2.0)
|
|
# Partial numerator terms
|
|
even_numerator = -(a + m) * (a + b + m) * x / (
|
|
(a + two * m) * (a + two * m + one))
|
|
odd_numerator = m * (b - m) * x / ((a + two * m - one) * (a + two * m))
|
|
one_numerator = full_like(x, 1.0)
|
|
numerator = select(iteration_is_even, even_numerator, odd_numerator)
|
|
return select(iteration_is_one, one_numerator, numerator)
|
|
|
|
def nth_partial_betainc_denominator(iteration, a, b, x):
|
|
iteration_bcast = broadcast_in_dim(iteration, shape, [])
|
|
return select(eq(iteration_bcast, full_like(iteration_bcast, 0)),
|
|
full_like(x, 0), full_like(x, 1))
|
|
|
|
result_is_nan = bitwise_or(bitwise_or(bitwise_or(
|
|
le(a, full_like(a, 0)), le(b, full_like(b, 0))),
|
|
lt(x, full_like(x, 0))), gt(x, full_like(x, 1)))
|
|
|
|
# The continued fraction will converge rapidly when x < (a+1)/(a+b+2)
|
|
# as per: http://dlmf.nist.gov/8.17.E23
|
|
#
|
|
# Otherwise, we can rewrite using the symmetry relation as per:
|
|
# http://dlmf.nist.gov/8.17.E4
|
|
converges_rapidly = lt(x, (a + full_like(a, 1)) / (a + b + full_like(b, 2.0)))
|
|
a_orig = a
|
|
a = select(converges_rapidly, a, b)
|
|
b = select(converges_rapidly, b, a_orig)
|
|
x = select(converges_rapidly, x, sub(full_like(x, 1), x))
|
|
|
|
continued_fraction = lentz_thompson_barnett_algorithm(
|
|
num_iterations=200 if dtype == np.float32 else 600,
|
|
small=(dtypes.finfo(dtype).eps / 2).astype(dtype),
|
|
threshold=(dtypes.finfo(dtype).eps / 2).astype(dtype),
|
|
nth_partial_numerator=nth_partial_betainc_numerator,
|
|
nth_partial_denominator=nth_partial_betainc_denominator,
|
|
inputs=[a, b, x]
|
|
)
|
|
|
|
lbeta_ab = lgamma(a) + lgamma(b) - lgamma(a + b)
|
|
result = continued_fraction * exp(log(x) * a + log1p(-x) * b - lbeta_ab) / a
|
|
result = select(result_is_nan, full_like(a, float('nan')), result)
|
|
return select(converges_rapidly, result, sub(full_like(result, 1), result))
|
|
|
|
class IgammaMode(Enum):
|
|
VALUE = 1
|
|
DERIVATIVE = 2
|
|
SAMPLE_DERIVATIVE = 3
|
|
|
|
def _any(predicates: Array) -> Array:
|
|
f = _const(predicates, False)
|
|
predicates_shape = predicates.shape
|
|
all_dimensions = tuple(range(len(predicates_shape)))
|
|
return reduce(predicates, f, bitwise_or, all_dimensions)
|
|
|
|
def _igamma_series(ax, x, a, enabled, dtype, mode):
|
|
def cond_fn(vals):
|
|
return _any(vals[0])
|
|
|
|
def body_fn(vals):
|
|
enabled, r, c, ans, x, dc_da, dans_da = vals
|
|
|
|
r = r + _const(r, 1.)
|
|
dc_da = dc_da * (x / r) - (c * x) / (r * r)
|
|
dans_da = dans_da + dc_da
|
|
c = c * (x / r)
|
|
ans = ans + c
|
|
|
|
if mode == IgammaMode.VALUE:
|
|
conditional = bitwise_and(enabled, c / ans > dtypes.finfo(dtype).eps)
|
|
else:
|
|
conditional = bitwise_and(enabled,
|
|
abs(dc_da / dans_da) > dtypes.finfo(dtype).eps)
|
|
|
|
# TODO: Make this a vmap. Might be tricky with the imports.
|
|
return (
|
|
conditional,
|
|
select(enabled, r, vals[1]),
|
|
select(enabled, c, vals[2]),
|
|
select(enabled, ans, vals[3]),
|
|
select(enabled, x, vals[4]),
|
|
select(enabled, dc_da, vals[5]),
|
|
select(enabled, dans_da, vals[6]),
|
|
)
|
|
|
|
init_vals = (
|
|
enabled, a, full_like(a, 1), full_like(a, 1), x, full_like(a, 0),
|
|
full_like(a, 0),
|
|
)
|
|
|
|
vals = while_loop(cond_fn, body_fn, init_vals)
|
|
ans = vals[3]
|
|
dans_da = vals[6]
|
|
|
|
if mode == IgammaMode.VALUE:
|
|
return (ans * ax) / a
|
|
|
|
dlogax_da = log(x) - digamma(a + _const(a, 1))
|
|
|
|
if mode == IgammaMode.DERIVATIVE:
|
|
return ax * (ans * dlogax_da + dans_da) / a
|
|
elif mode == IgammaMode.SAMPLE_DERIVATIVE:
|
|
return -(dans_da + ans * dlogax_da) * x / a
|
|
else:
|
|
raise ValueError("Invalid IgammaMode")
|
|
|
|
def igamma_impl(a, x, *, dtype):
|
|
is_nan = bitwise_or(_isnan(a), _isnan(x))
|
|
x_is_zero = eq(x, _const(x, 0))
|
|
x_is_infinity = eq(x, _const(x, float('inf')))
|
|
domain_error = bitwise_or(lt(x, _const(x, 0)), le(a, _const(a, 0)))
|
|
use_igammac = bitwise_and(gt(x, _const(x, 1)), gt(x, a))
|
|
ax = a * log(x) - x - lgamma(a)
|
|
underflow = lt(ax, -log(dtypes.finfo(dtype).max))
|
|
ax = exp(ax)
|
|
enabled = bitwise_not(
|
|
_reduce(bitwise_or,[x_is_zero, domain_error, underflow, is_nan]))
|
|
|
|
output = select(
|
|
use_igammac,
|
|
_const(a, 1) -
|
|
_igammac_continued_fraction(ax, x, a, bitwise_and(enabled, use_igammac),
|
|
dtype, IgammaMode.VALUE),
|
|
_igamma_series(ax, x, a, bitwise_and(enabled, bitwise_not(use_igammac)),
|
|
dtype, IgammaMode.VALUE)
|
|
)
|
|
output = select(x_is_zero, full_like(a, 0), output)
|
|
output = select(x_is_infinity, full_like(a, 1), output)
|
|
output = select(bitwise_or(domain_error, is_nan),
|
|
full_like(a, float('nan')), output)
|
|
return output
|
|
|
|
def _igammac_continued_fraction(ax, x, a, enabled, dtype, mode):
|
|
eps = dtypes.finfo(dtype).eps
|
|
|
|
def cond_fn(vals):
|
|
enabled, _ans, _t, _y, _x, c, *_ = vals
|
|
return bitwise_and(c < _const(c, 2000), _any(enabled))
|
|
|
|
def body_fn(vals):
|
|
(enabled, ans, t, y, z, c, pkm1, qkm1, pkm2, qkm2,
|
|
dpkm2_da, dqkm2_da, dpkm1_da, dqkm1_da, dans_da) = vals
|
|
|
|
c = c + _const(c, 1)
|
|
y = y + _const(y, 1)
|
|
z = z + _const(z, 2)
|
|
yc = y * c
|
|
pk = pkm1 * z - pkm2 * yc
|
|
qk = qkm1 * z - qkm2 * yc
|
|
qk_is_nonzero = ne(qk, _const(qk, 0))
|
|
r = pk / qk
|
|
|
|
t = select(qk_is_nonzero, abs(div(sub(ans, r), r)), full_like(r, 1))
|
|
ans = select(qk_is_nonzero, r, ans)
|
|
|
|
dpk_da = dpkm1_da * z - pkm1 - dpkm2_da * yc + pkm2 * c
|
|
dqk_da = dqkm1_da * z - qkm1 - dqkm2_da * yc + qkm2 * c
|
|
dans_da_new = select(qk_is_nonzero, div(dpk_da - ans * dqk_da, qk), dans_da)
|
|
grad_conditional = select(qk_is_nonzero,
|
|
abs(dans_da_new - dans_da),
|
|
full_like(dans_da, 1))
|
|
|
|
pkm2 = pkm1
|
|
pkm1 = pk
|
|
qkm2 = qkm1
|
|
qkm1 = qk
|
|
|
|
dpkm2_da = dpkm1_da
|
|
dqkm2_da = dqkm1_da
|
|
dpkm1_da = dpk_da
|
|
dqkm1_da = dqk_da
|
|
|
|
rescale = gt(abs(pk), reciprocal(_const(pk, eps)))
|
|
pkm2 = select(rescale, mul(pkm2, _const(pkm2, eps)), pkm2)
|
|
pkm1 = select(rescale, mul(pkm1, _const(pkm1, eps)), pkm1)
|
|
qkm2 = select(rescale, mul(qkm2, _const(qkm2, eps)), qkm2)
|
|
qkm1 = select(rescale, mul(qkm1, _const(qkm1, eps)), qkm1)
|
|
|
|
dpkm2_da = select(rescale, mul(dpkm2_da, _const(dpkm2_da, eps)), dpkm2_da)
|
|
dqkm2_da = select(rescale, mul(dqkm2_da, _const(dqkm2_da, eps)), dqkm2_da)
|
|
dpkm1_da = select(rescale, mul(dpkm1_da, _const(dpkm1_da, eps)), dpkm1_da)
|
|
dqkm1_da = select(rescale, mul(dqkm1_da, _const(dqkm1_da, eps)), dqkm1_da)
|
|
|
|
if mode == IgammaMode.VALUE:
|
|
conditional = bitwise_and(enabled, t > eps)
|
|
else:
|
|
conditional = bitwise_and(enabled,
|
|
grad_conditional > _const(grad_conditional, eps))
|
|
|
|
return (conditional,
|
|
select(enabled, ans, vals[1]),
|
|
select(enabled, t, vals[2]),
|
|
select(enabled, y, vals[3]),
|
|
select(enabled, z, vals[4]),
|
|
c,
|
|
select(enabled, pkm1, vals[6]),
|
|
select(enabled, qkm1, vals[7]),
|
|
select(enabled, pkm2, vals[8]),
|
|
select(enabled, qkm2, vals[9]),
|
|
select(enabled, dpkm2_da, vals[10]),
|
|
select(enabled, dqkm2_da, vals[11]),
|
|
select(enabled, dpkm1_da, vals[12]),
|
|
select(enabled, dqkm1_da, vals[13]),
|
|
select(enabled, dans_da_new, vals[14]))
|
|
|
|
y = _const(a, 1) - a
|
|
z = x + y + _const(x, 1)
|
|
c = _const(x, 0)
|
|
pkm2 = full_like(x, 1)
|
|
qkm2 = x
|
|
pkm1 = x + _const(x, 1)
|
|
qkm1 = z * x
|
|
ans = pkm1 / qkm1
|
|
t = full_like(x, 1)
|
|
dpkm2_da = full_like(x, 0)
|
|
dqkm2_da = full_like(x, 0)
|
|
dpkm1_da = full_like(x, 0)
|
|
dqkm1_da = -x
|
|
dans_da = (dpkm1_da - ans * dqkm1_da) / qkm1
|
|
init_vals = (enabled, ans, t, y, z,
|
|
c, pkm1, qkm1, pkm2, qkm2,
|
|
dpkm2_da, dqkm2_da, dpkm1_da, dqkm1_da, dans_da)
|
|
|
|
vals = while_loop(cond_fn, body_fn, init_vals)
|
|
ans = vals[1]
|
|
if mode == IgammaMode.VALUE:
|
|
return ans * ax
|
|
dans_da = vals[14]
|
|
dlogax_da = log(x) - digamma(a)
|
|
|
|
if mode == IgammaMode.DERIVATIVE:
|
|
return mul(ax, add(mul(ans, dlogax_da), dans_da))
|
|
elif mode == IgammaMode.SAMPLE_DERIVATIVE:
|
|
return neg(add(dans_da, mul(ans, dlogax_da)) * x)
|
|
else:
|
|
raise ValueError(f"Invalid mode: {mode}")
|
|
|
|
def igammac_impl(a, x, *, dtype):
|
|
out_of_range = bitwise_or(le(x, _const(x, 0)), le(a, _const(a, 0)))
|
|
use_igamma = bitwise_or(lt(x, _const(x, 1)), lt(x, a))
|
|
ax = a * log(x) - x - lgamma(a)
|
|
underflow = lt(ax, -log(dtypes.finfo(dtype).max))
|
|
enabled = bitwise_not(bitwise_or(out_of_range, underflow))
|
|
ax = exp(ax)
|
|
|
|
igamma_call = _igamma_series(ax, x, a, bitwise_and(enabled, use_igamma),
|
|
dtype, IgammaMode.VALUE)
|
|
igammac_cf_call = _igammac_continued_fraction(ax, x, a,
|
|
bitwise_and(enabled, bitwise_not(use_igamma)), dtype, IgammaMode.VALUE)
|
|
|
|
result = select(use_igamma, _const(a, 1) - igamma_call, igammac_cf_call)
|
|
x_is_infinity = eq(x, _const(x, float('inf')))
|
|
result = select(x_is_infinity, full_like(result, 0), result)
|
|
return select(out_of_range, full_like(a, 1), result)
|
|
|
|
def igamma_grad_a_impl(a, x, *, dtype):
|
|
is_nan = bitwise_or(_isnan(a), _isnan(x))
|
|
x_is_zero = eq(x, full_like(x,0))
|
|
domain_error = bitwise_or(lt(x, full_like(x, 0)), le(a, full_like(a, 0)))
|
|
use_igammac = bitwise_and(gt(x, full_like(x,1)), gt(x, a))
|
|
ax = a * log(x) - x - lgamma(a)
|
|
underflow = lt(ax, -log(dtypes.finfo(dtype).max))
|
|
ax = exp(ax)
|
|
enabled = bitwise_not(bitwise_or(bitwise_or(bitwise_or(
|
|
x_is_zero, domain_error), underflow), is_nan))
|
|
output = select(use_igammac,
|
|
-_igammac_continued_fraction(ax, x, a, bitwise_and(enabled, use_igammac),
|
|
dtype, IgammaMode.DERIVATIVE),
|
|
_igamma_series(ax, x, a, bitwise_and(enabled, bitwise_not(use_igammac)),
|
|
dtype, IgammaMode.DERIVATIVE))
|
|
output = select(x_is_zero, full_like(output,0), output)
|
|
output = select(bitwise_or(domain_error, is_nan),
|
|
full_like(a, float('nan')), output)
|
|
return output
|
|
|
|
def random_gamma_grad_impl(a, x, *, dtype):
|
|
is_nan = bitwise_or(_isnan(a), _isnan(x))
|
|
x_is_zero = eq(x, full_like(x,0))
|
|
domain_error = bitwise_or(lt(x, full_like(x,0)), le(a, full_like(a,0)))
|
|
use_igammac = bitwise_and(gt(x, full_like(x,1)), gt(x, a))
|
|
ax = a * log(x) - x - lgamma(a)
|
|
underflow = lt(ax, -log(dtypes.finfo(a.dtype).max))
|
|
ax = exp(ax)
|
|
enabled = bitwise_not(bitwise_or(bitwise_or(bitwise_or
|
|
(x_is_zero, domain_error), underflow), is_nan))
|
|
output = select(use_igammac,
|
|
-_igammac_continued_fraction(ax, x, a, bitwise_and(enabled, use_igammac),
|
|
dtype, IgammaMode.SAMPLE_DERIVATIVE),
|
|
_igamma_series(ax, x, a, bitwise_and(enabled, bitwise_not(use_igammac)),
|
|
dtype, IgammaMode.SAMPLE_DERIVATIVE))
|
|
output = select(x_is_zero, full_like(output,0), output)
|
|
output = select(bitwise_or(domain_error, is_nan),
|
|
full_like(a, float('nan')), output)
|
|
return output
|
|
|
|
def _up_and_broadcast(doit):
|
|
def up_and_broadcast(*args):
|
|
broadcasted_shape = broadcast_shapes(*(a.shape for a in args))
|
|
args = [broadcast_in_dim(a, broadcasted_shape, list(range(a.ndim))) for a in args]
|
|
|
|
a_dtype = args[0].dtype
|
|
needs_upcast = a_dtype == dtypes.bfloat16 or a_dtype == np.float16
|
|
if needs_upcast:
|
|
args = [convert_element_type(a, np.float32) for a in args]
|
|
a_x_type = np.float32
|
|
else:
|
|
a_x_type = a_dtype
|
|
result = doit(*args, dtype=a_x_type)
|
|
if needs_upcast:
|
|
result = convert_element_type(result, a_dtype)
|
|
return result
|
|
return up_and_broadcast
|
|
|
|
|
|
def evaluate_chebyshev_polynomial(x, coefficients):
|
|
b0 = full_like(x,0)
|
|
b1 = full_like(x,0)
|
|
b2 = full_like(x,0)
|
|
for c in coefficients:
|
|
b2 = b1
|
|
b1 = b0
|
|
b0 = x * b1 - b2 + full_like(x, c)
|
|
return 0.5 * (b0 - b2)
|
|
|
|
def _i0e_impl32(x):
|
|
"""
|
|
Computes an approximation to the modified Bessel function of the first kind,
|
|
zeroth order. The following implementation follows Cephes' F32 and F64
|
|
implementation of i0e.
|
|
"""
|
|
i0e_coeffs_a = np.array(
|
|
[-1.30002500998624804212E-8, 6.04699502254191894932E-8,
|
|
-2.67079385394061173391E-7, 1.11738753912010371815E-6,
|
|
-4.41673835845875056359E-6, 1.64484480707288970893E-5,
|
|
-5.75419501008210370398E-5, 1.88502885095841655729E-4,
|
|
-5.76375574538582365885E-4, 1.63947561694133579842E-3,
|
|
-4.32430999505057594430E-3, 1.05464603945949983183E-2,
|
|
-2.37374148058994688156E-2, 4.93052842396707084878E-2,
|
|
-9.49010970480476444210E-2, 1.71620901522208775349E-1,
|
|
-3.04682672343198398683E-1, 6.76795274409476084995E-1]
|
|
)
|
|
i0e_coeffs_b = np.array(
|
|
[3.39623202570838634515E-9, 2.26666899049817806459E-8,
|
|
2.04891858946906374183E-7, 2.89137052083475648297E-6,
|
|
6.88975834691682398426E-5, 3.36911647825569408990E-3,
|
|
8.04490411014108831608E-1]
|
|
)
|
|
|
|
x = abs(x)
|
|
half = full_like(x, 0.5)
|
|
two = full_like(x, 2.0)
|
|
thirty_two = full_like(x, 32.0)
|
|
|
|
result_le_8 = evaluate_chebyshev_polynomial(half * x - two, i0e_coeffs_a)
|
|
result_gt_8 = div(evaluate_chebyshev_polynomial(thirty_two / x - two,
|
|
i0e_coeffs_b), sqrt(x))
|
|
|
|
return select(x <= 8.0, result_le_8, result_gt_8)
|
|
|
|
def _i0e_impl64(x):
|
|
i0e_coeffs_a = np.array(
|
|
[-4.41534164647933937950E-18, 3.33079451882223809783E-17,
|
|
-2.43127984654795469359E-16, 1.71539128555513303061E-15,
|
|
-1.16853328779934516808E-14, 7.67618549860493561688E-14,
|
|
-4.85644678311192946090E-13, 2.95505266312963983461E-12,
|
|
-1.72682629144155570723E-11, 9.67580903537323691224E-11,
|
|
-5.18979560163526290666E-10, 2.65982372468238665035E-9,
|
|
-1.30002500998624804212E-8, 6.04699502254191894932E-8,
|
|
-2.67079385394061173391E-7, 1.11738753912010371815E-6,
|
|
-4.41673835845875056359E-6, 1.64484480707288970893E-5,
|
|
-5.75419501008210370398E-5, 1.88502885095841655729E-4,
|
|
-5.76375574538582365885E-4, 1.63947561694133579842E-3,
|
|
-4.32430999505057594430E-3, 1.05464603945949983183E-2,
|
|
-2.37374148058994688156E-2, 4.93052842396707084878E-2,
|
|
-9.49010970480476444210E-2, 1.71620901522208775349E-1,
|
|
-3.04682672343198398683E-1, 6.76795274409476084995E-1]
|
|
)
|
|
i0e_coeffs_b = np.array(
|
|
[-7.23318048787475395456E-18, -4.83050448594418207126E-18,
|
|
4.46562142029675999901E-17, 3.46122286769746109310E-17,
|
|
-2.82762398051658348494E-16, -3.42548561967721913462E-16,
|
|
1.77256013305652638360E-15, 3.81168066935262242075E-15,
|
|
-9.55484669882830764870E-15, -4.15056934728722208663E-14,
|
|
1.54008621752140982691E-14, 3.85277838274214270114E-13,
|
|
7.18012445138366623367E-13, -1.79417853150680611778E-12,
|
|
-1.32158118404477131188E-11, -3.14991652796324136454E-11,
|
|
1.18891471078464383424E-11, 4.94060238822496958910E-10,
|
|
3.39623202570838634515E-9, 2.26666899049817806459E-8,
|
|
2.04891858946906374183E-7, 2.89137052083475648297E-6,
|
|
6.88975834691682398426E-5, 3.36911647825569408990E-3,
|
|
8.04490411014108831608E-1]
|
|
)
|
|
|
|
x = abs(x)
|
|
half = full_like(x, 0.5)
|
|
two = full_like(x, 2.0)
|
|
thirty_two = full_like(x, 32.0)
|
|
|
|
result_le_8 = evaluate_chebyshev_polynomial(half * x - two, i0e_coeffs_a)
|
|
result_gt_8 = div(evaluate_chebyshev_polynomial(thirty_two / x - two,
|
|
i0e_coeffs_b), sqrt(x))
|
|
|
|
return select(x <= 8.0, result_le_8, result_gt_8)
|
|
|
|
def bessel_i0e_impl(x):
|
|
if x.dtype == np.float64:
|
|
return _i0e_impl64(x)
|
|
elif x.dtype == np.float32:
|
|
return _i0e_impl32(x)
|
|
else:
|
|
# Have to upcast f16 because the magic Cephes coefficients don't have enough
|
|
# precision for it.
|
|
x_dtype = x.dtype
|
|
x = x.astype(np.float32)
|
|
return convert_element_type(_i0e_impl32(x), x_dtype)
|
|
|
|
|
|
regularized_incomplete_beta_p = standard_naryop(
|
|
[_float, _float, _float], 'regularized_incomplete_beta')
|
|
mlir.register_lowering(regularized_incomplete_beta_p,
|
|
mlir.lower_fun(_up_and_broadcast(regularized_incomplete_beta_impl),
|
|
multiple_results=False))
|
|
|
|
ad.defjvp(regularized_incomplete_beta_p,
|
|
betainc_grad_not_implemented,
|
|
betainc_grad_not_implemented,
|
|
betainc_gradx)
|
|
|
|
lgamma_p = standard_unop(_float, 'lgamma')
|
|
ad.defjvp(lgamma_p, lambda g, x: mul(g, digamma(x)))
|
|
mlir.register_lowering(lgamma_p, partial(_nary_lower_hlo, chlo.lgamma))
|
|
|
|
digamma_p = standard_unop(_float, 'digamma')
|
|
mlir.register_lowering(digamma_p, partial(_nary_lower_hlo, chlo.digamma))
|
|
ad.defjvp(digamma_p, lambda g, x: mul(g, polygamma(_const(x, 1), x)))
|
|
|
|
polygamma_p = standard_naryop([_float, _float], 'polygamma')
|
|
mlir.register_lowering(polygamma_p, partial(_nary_lower_hlo, chlo.polygamma))
|
|
ad.defjvp(polygamma_p, polygamma_gradm, polygamma_gradx)
|
|
|
|
igamma_p = standard_naryop([_float, _float], 'igamma')
|
|
mlir.register_lowering(igamma_p, mlir.lower_fun(_up_and_broadcast(igamma_impl),
|
|
multiple_results=False))
|
|
|
|
igamma_grad_a_p = standard_naryop([_float, _float], 'igamma_grad_a')
|
|
mlir.register_lowering(igamma_grad_a_p,
|
|
mlir.lower_fun(_up_and_broadcast(igamma_grad_a_impl),
|
|
multiple_results=False))
|
|
|
|
ad.defjvp(igamma_p, igamma_grada, igamma_gradx)
|
|
|
|
igammac_p = standard_naryop([_float, _float], 'igammac')
|
|
mlir.register_lowering(igammac_p,
|
|
mlir.lower_fun(_up_and_broadcast(igammac_impl),
|
|
multiple_results=False))
|
|
|
|
ad.defjvp(igammac_p, igammac_grada, igammac_gradx)
|
|
|
|
random_gamma_grad_p = standard_naryop([_float, _float], 'random_gamma_grad')
|
|
mlir.register_lowering(random_gamma_grad_p,
|
|
mlir.lower_fun(_up_and_broadcast(random_gamma_grad_impl),
|
|
multiple_results=False))
|
|
|
|
zeta_p = standard_naryop([_float, _float], 'zeta')
|
|
mlir.register_lowering(zeta_p, partial(_nary_lower_hlo, chlo.zeta))
|
|
|
|
bessel_i0e_p = standard_unop(_float, 'bessel_i0e')
|
|
mlir.register_lowering(bessel_i0e_p,
|
|
mlir.lower_fun(bessel_i0e_impl,
|
|
multiple_results=False))
|
|
ad.defjvp2(bessel_i0e_p, lambda g, y, x: g * (bessel_i1e(x) - sign(x) * y))
|
|
|
|
bessel_i1e_p = standard_unop(_float, 'bessel_i1e')
|
|
mlir.register_lowering(bessel_i1e_p,
|
|
partial(_nary_lower_hlo, chlo.bessel_i1e))
|
|
|
|
def _bessel_i1e_jvp(g, y, x):
|
|
eps = dtypes.finfo(_dtype(x)).eps
|
|
x_is_not_tiny = abs(x) > eps
|
|
safe_x = select(x_is_not_tiny, x, full_like(x, eps))
|
|
dy_dx = bessel_i0e(safe_x) - y * (sign(safe_x) + reciprocal(safe_x))
|
|
dy_dx = select(x_is_not_tiny, dy_dx, full_like(x, 0.5))
|
|
return g * dy_dx
|
|
ad.defjvp2(bessel_i1e_p, _bessel_i1e_jvp)
|
|
|
|
erf_p = standard_unop(_float, 'erf')
|
|
ad.defjvp(erf_p, lambda g, x: mul(_const(x, 2. / np.sqrt(np.pi)),
|
|
mul(g, exp(neg(square(x))))))
|
|
mlir.register_lowering(erf_p, partial(_nary_lower_hlo, chlo.erf))
|
|
|
|
erfc_p = standard_unop(_float, 'erfc')
|
|
ad.defjvp(erfc_p, lambda g, x: mul(_const(x, -2. / np.sqrt(np.pi)),
|
|
mul(g, exp(neg(square(x))))))
|
|
mlir.register_lowering(erfc_p, partial(_nary_lower_hlo, chlo.erfc))
|
|
|
|
erf_inv_p = standard_unop(_float, 'erf_inv')
|
|
ad.defjvp2(erf_inv_p, lambda g, ans, x: mul(_const(x, np.sqrt(np.pi) / 2.),
|
|
mul(g, exp(square(ans)))))
|
|
mlir.register_lowering(erf_inv_p, partial(_nary_lower_hlo, chlo.erf_inv))
|