mirror of
https://github.com/ROCm/jax.git
synced 2025-04-16 03:46:06 +00:00
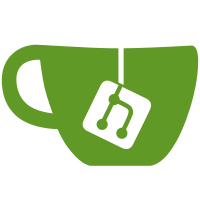
This one is particularly annoying, because we have to break up the MMA into two collective N=256 MMAs. However, TensorCore only updates a contiguous chunk of columns in TMEM and so after executing two of those we end up with a TMEM layout that looks like this: ``` Contributing CTA | 0 | 1 | 0 | 1 | N local | 0:128 | 0:128 | 128:256 | 128:256 | N | 0:128 | 256:384 | 128:256 | 384:512 | ``` You can see that the TMEM columns no longer monotonically go over all columns until N=512, but they include a number of jumps! We could fix this on the load side, by ensuring that each CTA in the group does a strided load along the tiled dimension, but that just seems more trouble than it's worth (and is not that well supported by TMA unless we increase the number of striding levels). Instead, we encode this weirdness in the TMEM layout we use and make sure to rearrange the data properly while loading the tiles into registers. PiperOrigin-RevId: 735791426
270 lines
9.2 KiB
Python
270 lines
9.2 KiB
Python
# Copyright 2025 The JAX Authors. All Rights Reserved.
|
|
#
|
|
# Licensed under the Apache License, Version 2.0 (the "License");
|
|
# you may not use this file except in compliance with the License.
|
|
# You may obtain a copy of the License at
|
|
#
|
|
# http://www.apache.org/licenses/LICENSE-2.0
|
|
#
|
|
# Unless required by applicable law or agreed to in writing, software
|
|
# distributed under the License is distributed on an "AS IS" BASIS,
|
|
# WITHOUT WARRANTIES OR CONDITIONS OF ANY KIND, either express or implied.
|
|
# See the License for the specific language governing permissions and
|
|
# limitations under the License.
|
|
# ==============================================================================
|
|
"""Matmul kernel for Blackwell."""
|
|
|
|
import itertools
|
|
|
|
import jax
|
|
from jax._src.interpreters import mlir
|
|
from jax._src.lib.mlir import ir
|
|
from jax._src.lib.mlir.dialects import arith
|
|
from jax._src.lib.mlir.dialects import gpu
|
|
from jax._src.lib.mlir.dialects import nvvm
|
|
from jax.experimental.mosaic import gpu as mgpu
|
|
from jax.experimental.mosaic.gpu import c, ds
|
|
from jax.experimental.mosaic.gpu import tcgen05
|
|
from jax.experimental.mosaic.gpu import profiler
|
|
import jax.numpy as jnp
|
|
import jax.random as jr
|
|
import numpy as np
|
|
|
|
|
|
BLACKWELL_MMA_FP16_K = 16
|
|
TMA_WARP = 1
|
|
MMA_WARP = 0
|
|
|
|
|
|
def bytecount(shape, dtype):
|
|
return int(np.prod(shape) * dtype.dtype.itemsize)
|
|
|
|
|
|
def build_kernel(
|
|
m, n, k,
|
|
tile_m: int = 128,
|
|
tile_n: int = 128,
|
|
grid_tile_m: int = 1,
|
|
max_concurrent_steps: int = 2,
|
|
collective: bool = False,
|
|
):
|
|
i1 = ir.IntegerType.get_signless(1)
|
|
i32 = ir.IntegerType.get_signless(32)
|
|
index = ir.IndexType.get()
|
|
|
|
swizzle = 128
|
|
swizzle_elems = tile_k = swizzle // 2
|
|
tiling = (8, swizzle_elems)
|
|
|
|
in_dtype = jnp.float16
|
|
k_loop_iter = k // tile_k
|
|
max_concurrent_steps = min(max_concurrent_steps, k_loop_iter)
|
|
|
|
block_tile_m = tile_m
|
|
block_tile_n = tile_n
|
|
if collective:
|
|
tile_m *= 2
|
|
tile_n *= 2
|
|
if grid_tile_m == 1:
|
|
grid_tile_m = 2
|
|
|
|
if m % tile_m != 0:
|
|
raise ValueError(f"{m=} must be divisible by {tile_m=}")
|
|
if n % tile_n != 0:
|
|
raise ValueError(f"{n=} must be divisible by {tile_n=}")
|
|
if k % tile_k != 0:
|
|
raise ValueError(f"{k=} must be divisible by {tile_k=}")
|
|
if (m // tile_m) % grid_tile_m:
|
|
raise ValueError(f"{m=} // {tile_m=} must be divisible by {grid_tile_m=}")
|
|
|
|
def kernel(ctx, a, b, d, smem):
|
|
((a_smem, b_smem), d_smem), barriers, mma_done_barrier, acc = smem
|
|
(ab_full_barriers, ab_empty_barriers) = barriers
|
|
|
|
warp_idx = mgpu.warp_idx(sync=True)
|
|
is_warp_leader = nvvm.elect_sync(i1)
|
|
is_leader_of = lambda i: arith.andi(arith.cmpi(arith.CmpIPredicate.eq, warp_idx, c(i, i32)), is_warp_leader)
|
|
is_leader_block = arith.cmpi(arith.CmpIPredicate.eq, ctx.cluster_idx(gpu.Dimension.x), c(0, index))
|
|
|
|
m_idx = arith.addi(
|
|
gpu.block_id(gpu.Dimension.x),
|
|
arith.muli(gpu.block_id(gpu.Dimension.z), c(grid_tile_m, index)),
|
|
)
|
|
n_idx = gpu.block_id(gpu.Dimension.y)
|
|
block_m_start = arith.muli(m_idx, c(block_tile_m, index))
|
|
# All blocks in the cluster share the same m_start -- align it!
|
|
m_start = arith.muli(arith.divui(block_m_start, c(tile_m, index)), c(tile_m, index))
|
|
n_start = arith.muli(n_idx, c(tile_n,index))
|
|
|
|
|
|
with mgpu.when(is_leader_of(TMA_WARP)):
|
|
@mgpu.fori(c(k_loop_iter, index), None)
|
|
def _tma_body(ki, _):
|
|
slot = arith.remui(ki, c(max_concurrent_steps, index))
|
|
# TODO(apaszke): Use a predicate instead of a conditional.
|
|
with mgpu.when(arith.cmpi(arith.CmpIPredicate.uge, ki, c(max_concurrent_steps, index))):
|
|
ab_empty_barriers[slot].wait()
|
|
full_barrier = ab_full_barriers[slot]
|
|
with mgpu.when(is_leader_block):
|
|
full_barrier.arrive_expect_tx(
|
|
bytecount((tile_m, tile_k), in_dtype) + bytecount((tile_n, tile_k), in_dtype)
|
|
)
|
|
k_start = arith.muli(ki, c(tile_k, index))
|
|
common_args = dict(
|
|
swizzle=swizzle,
|
|
barrier=full_barrier,
|
|
arrive=False,
|
|
uniform=False,
|
|
collective=gpu.Dimension.x,
|
|
partitioned=0, # Non-contracting dim is always 0.
|
|
)
|
|
ctx.async_copy(
|
|
src_ref=a,
|
|
dst_ref=mgpu.memref_slice(a_smem, slot),
|
|
gmem_slice=(ds(m_start, tile_m), ds(k_start, tile_k)),
|
|
gmem_transform=mgpu.TileTransform(tiling),
|
|
**common_args,
|
|
)
|
|
ctx.async_copy(
|
|
src_ref=b,
|
|
dst_ref=mgpu.memref_slice(b_smem, slot),
|
|
gmem_slice=(ds(n_start, tile_n), ds(k_start, tile_k)),
|
|
gmem_transform=mgpu.TileTransform(tiling),
|
|
**common_args,
|
|
)
|
|
|
|
with mgpu.when(arith.andi(is_leader_of(MMA_WARP), is_leader_block)):
|
|
@mgpu.fori(c(k_loop_iter, index), arith.constant(i1, 0))
|
|
def _mma_body(ki, accumulate):
|
|
slot = arith.remui(ki, c(max_concurrent_steps, index))
|
|
ab_full_barriers[slot].wait()
|
|
tcgen05.mma(
|
|
acc,
|
|
mgpu.memref_slice(a_smem, slot),
|
|
mgpu.memref_transpose(mgpu.memref_slice(b_smem, slot), (1, 0, 3, 2)),
|
|
a_swizzle=swizzle,
|
|
b_swizzle=swizzle,
|
|
accumulate=accumulate,
|
|
collective=collective,
|
|
)
|
|
accumulate = arith.constant(i1, 1)
|
|
is_last_iter = arith.cmpi(
|
|
arith.CmpIPredicate.eq, ki, c(k_loop_iter - 1, index)
|
|
)
|
|
barrier_ptr = arith.select(
|
|
is_last_iter,
|
|
mma_done_barrier.get_ptr(),
|
|
ab_empty_barriers[slot].get_ptr(),
|
|
)
|
|
tcgen05.commit_arrive(barrier_ptr, collective=collective, ctx=ctx)
|
|
return accumulate
|
|
|
|
gpu.barrier()
|
|
mma_done_barrier.wait(for_tensor_core=True)
|
|
|
|
acc[:].astype(ir.F16Type.get()).store_tiled(d_smem, swizzle=128)
|
|
mgpu.commit_shared()
|
|
ctx.async_copy(
|
|
src_ref=d_smem,
|
|
dst_ref=d,
|
|
gmem_slice=(ds(block_m_start, block_tile_m), ds(n_start, tile_n)),
|
|
gmem_transform=mgpu.TileTransform((128, swizzle_elems)),
|
|
swizzle=swizzle,
|
|
)
|
|
ctx.await_async_copy(0)
|
|
|
|
compute_buffers = (
|
|
jax.ShapeDtypeStruct(
|
|
mgpu.tile_shape((max_concurrent_steps, block_tile_m, tile_k), tiling),
|
|
jnp.float16),
|
|
jax.ShapeDtypeStruct(
|
|
mgpu.tile_shape((max_concurrent_steps, block_tile_n, tile_k), tiling),
|
|
jnp.float16),
|
|
)
|
|
epilogue_buffer = jax.ShapeDtypeStruct(
|
|
mgpu.tile_shape((block_tile_m, tile_n), (128, swizzle_elems)),
|
|
jnp.float16)
|
|
smem_buffers = mgpu.Union([compute_buffers, epilogue_buffer])
|
|
smem = (
|
|
smem_buffers,
|
|
[mgpu.Barrier(arrival_count=1, num_barriers=max_concurrent_steps)] * 2,
|
|
mgpu.Barrier(arrival_count=1),
|
|
mgpu.TMEM((128, tile_n), jnp.float32, collective=collective),
|
|
)
|
|
return mgpu.as_gpu_kernel(
|
|
kernel,
|
|
(grid_tile_m, n // tile_n, m // (block_tile_m * grid_tile_m)),
|
|
(128, 1, 1),
|
|
(
|
|
jax.ShapeDtypeStruct((m, k), jnp.float16),
|
|
jax.ShapeDtypeStruct((n, k), jnp.float16),
|
|
),
|
|
jax.ShapeDtypeStruct((m, n), jnp.float16),
|
|
smem,
|
|
cluster=(2 if collective else 1, 1, 1),
|
|
)
|
|
|
|
|
|
def main(unused_argv):
|
|
m, k, n = 8192, 4096, 8192
|
|
|
|
ka, kb = jr.split(jr.key(0), 2)
|
|
a = jr.normal(key=ka, shape=(m, k), dtype=jnp.float16)
|
|
b = jr.normal(key=kb, shape=(n, k), dtype=jnp.float16)
|
|
|
|
tile_m = (128,)
|
|
tile_n = (128, 256, 512)
|
|
max_concurrent_steps = (2, 4, 5, 6)
|
|
grid_tile_m = (1, 2, 4, 8, 16)
|
|
collective = (False, True)
|
|
configs = itertools.product(collective, tile_m, tile_n, grid_tile_m, max_concurrent_steps)
|
|
names = ("collective", "tile_m", "tile_n", "grid_tile_m", "max_concurrent_steps")
|
|
best_runtime = float("inf")
|
|
best_kwargs = {}
|
|
for config in configs:
|
|
kwargs = dict(zip(names, config))
|
|
tile_m = kwargs["tile_m"]
|
|
tile_n = kwargs["tile_n"]
|
|
if kwargs["collective"]:
|
|
tile_m *= 2
|
|
tile_n *= 2
|
|
if m < tile_m or n < tile_n:
|
|
continue
|
|
if tile_n > 512:
|
|
continue
|
|
if (m // tile_m) % kwargs["grid_tile_m"]:
|
|
continue
|
|
try:
|
|
with mlir.make_ir_context(), ir.Location.unknown():
|
|
f = build_kernel(m, n, k, **kwargs)
|
|
_, runtime = profiler.measure(f)(a, b)
|
|
except ValueError as e:
|
|
if "Mosaic GPU kernel exceeds available shared memory" not in str(e):
|
|
raise
|
|
runtime = float("inf")
|
|
else:
|
|
print(" ".join(f"{k}={v}" for k, v in kwargs.items()), int(runtime * 1000))
|
|
if runtime < best_runtime:
|
|
best_runtime = runtime
|
|
best_kwargs = kwargs
|
|
if not best_kwargs:
|
|
raise ValueError("No valid configuration found")
|
|
|
|
with mlir.make_ir_context(), ir.Location.unknown():
|
|
d, runtime = profiler.measure(build_kernel(m, n, k, **best_kwargs))(a, b)
|
|
d_ref, ref_runtime = profiler.measure(jax.jit(lambda a, b: a @ b.T))(a, b)
|
|
|
|
tflops = float(2 * k * m * n) / (runtime / 1e3) / 1e12
|
|
ref_tflops = float(2 * k * m * n) / (ref_runtime / 1e3) / 1e12
|
|
print("Best parameters: ", " ".join(f"{k}={v}" for k, v in best_kwargs.items()))
|
|
print(f"Kernel: {runtime * 1000:.1f} us = {tflops:.1f} TFLOPS")
|
|
print(f"Reference: {ref_runtime * 1000:.1f} us = {ref_tflops:.1f} TFLOPS")
|
|
np.testing.assert_allclose(d, d_ref, atol=1e-3, rtol=1e-3)
|
|
|
|
|
|
if __name__ == "__main__":
|
|
from absl import app
|
|
import jax
|
|
jax.config.config_with_absl()
|
|
app.run(main)
|