mirror of
https://github.com/ROCm/jax.git
synced 2025-04-25 17:56:06 +00:00
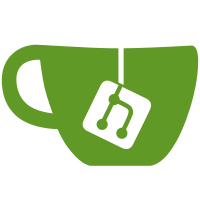
* Make check_dtypes, atol, and rtol keyword-only arguments in jax.test_util APIs. Default to check_dtypes=True. Remove explicit usages of check_dtypes=True from tests. This mostly just removes visual noise from tests. Testing for exact type equality is the sensible default, although there are cases where opting out makes sense. No functional changes intended. * Fix a number of lax reference implementations to preserve types.
428 lines
16 KiB
Python
428 lines
16 KiB
Python
# Copyright 2018 Google LLC
|
|
#
|
|
# Licensed under the Apache License, Version 2.0 (the "License");
|
|
# you may not use this file except in compliance with the License.
|
|
# You may obtain a copy of the License at
|
|
#
|
|
# https://www.apache.org/licenses/LICENSE-2.0
|
|
#
|
|
# Unless required by applicable law or agreed to in writing, software
|
|
# distributed under the License is distributed on an "AS IS" BASIS,
|
|
# WITHOUT WARRANTIES OR CONDITIONS OF ANY KIND, either express or implied.
|
|
# See the License for the specific language governing permissions and
|
|
# limitations under the License.
|
|
|
|
|
|
import builtins
|
|
import collections
|
|
import itertools
|
|
|
|
import numpy as np
|
|
import opt_einsum
|
|
import scipy.special
|
|
|
|
from . import dtypes
|
|
|
|
_slice = builtins.slice
|
|
_max = builtins.max
|
|
_min = builtins.min
|
|
_map = builtins.map
|
|
|
|
neg = np.negative
|
|
sign = np.sign
|
|
floor = np.floor
|
|
ceil = np.ceil
|
|
round = lambda x: np.trunc(x + np.copysign(.5, x)).astype(x.dtype)
|
|
nextafter = np.nextafter
|
|
|
|
is_finite = np.isfinite
|
|
|
|
exp = np.exp
|
|
expm1 = np.expm1
|
|
log = np.log
|
|
log1p = np.log1p
|
|
tanh = np.tanh
|
|
sin = np.sin
|
|
cos = np.cos
|
|
atan2 = np.arctan2
|
|
|
|
sqrt = np.sqrt
|
|
rsqrt = lambda x: np.ones_like(x) / np.sqrt(x)
|
|
square = np.square
|
|
reciprocal = np.reciprocal
|
|
tan = np.tan
|
|
asin = np.arcsin
|
|
acos = np.arccos
|
|
atan = np.arctan
|
|
sinh = np.sinh
|
|
cosh = np.cosh
|
|
asinh = np.arcsinh
|
|
acosh = np.arccosh
|
|
atanh = np.arctanh
|
|
|
|
def betainc(a, b, x): return scipy.special.betainc(a, b, x).astype(x.dtype)
|
|
def lgamma(x): return scipy.special.gammaln(x).astype(x.dtype)
|
|
def digamma(x): return scipy.special.digamma(x).astype(x.dtype)
|
|
igamma = scipy.special.gammainc
|
|
igammac = scipy.special.gammaincc
|
|
def erf(x): return scipy.special.erf(x).astype(x.dtype)
|
|
def erfc(x): return scipy.special.erfc(x).astype(x.dtype)
|
|
def erf_inv(x): return scipy.special.erfinv(x).astype(x.dtype)
|
|
|
|
def bessel_i0e(x): return scipy.special.i0e(x).astype(x.dtype)
|
|
def bessel_i1e(x): return scipy.special.i1e(x).astype(x.dtype)
|
|
|
|
real = np.real
|
|
imag = np.imag
|
|
|
|
def conj(x):
|
|
return np.conj(x) + np.complex64(0)
|
|
|
|
def complex(x, y):
|
|
return x + np.complex64(1j) * y
|
|
|
|
abs = np.absolute
|
|
pow = np.power
|
|
|
|
bitwise_not = np.bitwise_not
|
|
bitwise_and = np.bitwise_and
|
|
bitwise_or = np.bitwise_or
|
|
bitwise_xor = np.bitwise_xor
|
|
|
|
add = np.add
|
|
sub = np.subtract
|
|
mul = np.multiply
|
|
|
|
def div(lhs, rhs):
|
|
if dtypes.issubdtype(dtypes.result_type(lhs), np.integer):
|
|
quotient = np.floor_divide(lhs, rhs)
|
|
select = np.logical_and(np.sign(lhs) != np.sign(rhs),
|
|
np.remainder(lhs, rhs) != 0)
|
|
return np.where(select, quotient + 1, quotient)
|
|
else:
|
|
return np.divide(lhs, rhs)
|
|
|
|
def rem(lhs, rhs):
|
|
return np.sign(lhs) * np.remainder(np.abs(lhs), np.abs(rhs))
|
|
|
|
max = np.maximum
|
|
min = np.minimum
|
|
|
|
shift_left = np.left_shift
|
|
shift_right_arithmetic = np.right_shift
|
|
# TODO shift_right_logical
|
|
|
|
def population_count(x):
|
|
assert x.dtype in (np.uint32, np.uint64)
|
|
m = [
|
|
0x5555555555555555, # binary: 0101...
|
|
0x3333333333333333, # binary: 00110011..
|
|
0x0f0f0f0f0f0f0f0f, # binary: 4 zeros, 4 ones ...
|
|
0x00ff00ff00ff00ff, # binary: 8 zeros, 8 ones ...
|
|
0x0000ffff0000ffff, # binary: 16 zeros, 16 ones ...
|
|
0x00000000ffffffff, # binary: 32 zeros, 32 ones
|
|
]
|
|
|
|
if x.dtype == np.uint32:
|
|
m = list(map(np.uint32, m[:-1]))
|
|
else:
|
|
m = list(map(np.uint64, m))
|
|
|
|
x = (x & m[0]) + ((x >> 1) & m[0]) # put count of each 2 bits into those 2 bits
|
|
x = (x & m[1]) + ((x >> 2) & m[1]) # put count of each 4 bits into those 4 bits
|
|
x = (x & m[2]) + ((x >> 4) & m[2]) # put count of each 8 bits into those 8 bits
|
|
x = (x & m[3]) + ((x >> 8) & m[3]) # put count of each 16 bits into those 16 bits
|
|
x = (x & m[4]) + ((x >> 16) & m[4]) # put count of each 32 bits into those 32 bits
|
|
if x.dtype == np.uint64:
|
|
x = (x & m[5]) + ((x >> 32) & m[5]) # put count of each 64 bits into those 64 bits
|
|
return x
|
|
|
|
eq = np.equal
|
|
ne = np.not_equal
|
|
ge = np.greater_equal
|
|
gt = np.greater
|
|
le = np.less_equal
|
|
lt = np.less
|
|
|
|
def convert_element_type(operand, dtype):
|
|
return np.asarray(operand, dtype=dtype)
|
|
|
|
def bitcast_convert_type(operand, dtype):
|
|
return np.asarray(operand).view(dtype)
|
|
|
|
def clamp(min, operand, max):
|
|
return np.clip(operand, np.clip(min, None, max), max).astype(operand.dtype)
|
|
|
|
def concatenate(operands, dimension):
|
|
return np.concatenate(operands, axis=dimension)
|
|
|
|
def conv(lhs, rhs, window_strides, padding):
|
|
pads = padtype_to_pads(lhs.shape[2:], rhs.shape[2:], window_strides, padding)
|
|
return _conv(lhs, rhs, window_strides, pads)
|
|
|
|
def conv_with_general_padding(
|
|
lhs, rhs, window_strides, padding, lhs_dilation, rhs_dilation):
|
|
return _conv(_dilate(lhs, lhs_dilation), _dilate(rhs, rhs_dilation),
|
|
window_strides, padding)
|
|
|
|
def conv_general_dilated(lhs, rhs, window_strides, padding, lhs_dilation,
|
|
rhs_dilation, dimension_numbers):
|
|
lhs_perm, rhs_perm, out_perm = _conv_general_permutations(dimension_numbers)
|
|
if isinstance(padding, str):
|
|
padding = padtype_to_pads(np.take(lhs.shape, lhs_perm)[2:],
|
|
np.take(rhs.shape, rhs_perm)[2:],
|
|
window_strides, padding)
|
|
trans_lhs = transpose(lhs, lhs_perm)
|
|
trans_rhs = transpose(rhs, rhs_perm)
|
|
out = conv_with_general_padding(trans_lhs, trans_rhs, window_strides, padding,
|
|
lhs_dilation, rhs_dilation)
|
|
return transpose(out, np.argsort(out_perm))
|
|
|
|
dot = np.dot
|
|
|
|
def dot_general(lhs, rhs, dimension_numbers):
|
|
(lhs_contracting, rhs_contracting), (lhs_batch, rhs_batch) = dimension_numbers
|
|
new_id = itertools.count()
|
|
lhs_axis_ids = [next(new_id) for _ in lhs.shape]
|
|
rhs_axis_ids = [next(new_id) for _ in rhs.shape]
|
|
lhs_out_axis_ids = lhs_axis_ids[:]
|
|
rhs_out_axis_ids = rhs_axis_ids[:]
|
|
|
|
for lhs_axis, rhs_axis in zip(lhs_contracting, rhs_contracting):
|
|
shared_id = next(new_id)
|
|
lhs_axis_ids[lhs_axis] = shared_id
|
|
rhs_axis_ids[rhs_axis] = shared_id
|
|
lhs_out_axis_ids[lhs_axis] = None
|
|
rhs_out_axis_ids[rhs_axis] = None
|
|
|
|
batch_ids = []
|
|
for lhs_axis, rhs_axis in zip(lhs_batch, rhs_batch):
|
|
shared_id = next(new_id)
|
|
lhs_axis_ids[lhs_axis] = shared_id
|
|
rhs_axis_ids[rhs_axis] = shared_id
|
|
lhs_out_axis_ids[lhs_axis] = None
|
|
rhs_out_axis_ids[rhs_axis] = None
|
|
batch_ids.append(shared_id)
|
|
|
|
not_none = lambda x: x is not None
|
|
out_axis_ids = filter(not_none,
|
|
batch_ids + lhs_out_axis_ids + rhs_out_axis_ids)
|
|
assert lhs.dtype == rhs.dtype
|
|
dtype = np.float32 if lhs.dtype == dtypes.bfloat16 else None
|
|
out = np.einsum(lhs, lhs_axis_ids, rhs, rhs_axis_ids, out_axis_ids,
|
|
dtype=dtype)
|
|
return out.astype(dtypes.bfloat16) if lhs.dtype == dtypes.bfloat16 else out
|
|
|
|
def broadcast(operand, sizes):
|
|
return np.broadcast_to(operand, sizes + np.shape(operand))
|
|
|
|
def broadcast_in_dim(operand, shape, broadcast_dimensions):
|
|
in_reshape = np.ones(len(shape), dtype=np.int32)
|
|
for i, bd in enumerate(broadcast_dimensions):
|
|
in_reshape[bd] = operand.shape[i]
|
|
return np.broadcast_to(np.reshape(operand, in_reshape), shape)
|
|
|
|
sum = np.sum
|
|
|
|
squeeze = np.squeeze
|
|
|
|
def reshape(operand, new_sizes, dimensions=None):
|
|
if dimensions is None:
|
|
dimensions = range(len(np.shape(operand)))
|
|
return np.reshape(np.transpose(operand, dimensions), new_sizes)
|
|
|
|
def pad(operand, padding_value, padding_config):
|
|
lo, hi, interior = zip(*padding_config)
|
|
outshape = np.add(np.add(np.add(lo, hi), operand.shape),
|
|
np.multiply(interior, np.subtract(operand.shape, 1)))
|
|
out = np.full(outshape, padding_value, operand.dtype)
|
|
lhs_slices = tuple(_slice(l if l > 0 else 0, -h if h > 0 else None, step)
|
|
for l, h, step in zip(lo, hi, np.add(1, interior)))
|
|
rhs_slices = tuple(_slice(l if l < 0 else 0, -h if h < 0 else None)
|
|
for l, h in zip(lo, hi))
|
|
out[lhs_slices] = operand[rhs_slices]
|
|
return out
|
|
|
|
def rev(operand, dimensions):
|
|
dimensions = frozenset(dimensions)
|
|
indexer = (_slice(None, None, -1) if d in dimensions else _slice(None)
|
|
for d in range(np.ndim(operand)))
|
|
return operand[tuple(indexer)]
|
|
|
|
select = np.where
|
|
|
|
def slice(operand, start_indices, limit_indices, strides=None): # pylint: disable=redefined-builtin
|
|
if strides is None:
|
|
strides = np.ones(len(start_indices)).astype(int)
|
|
slices = tuple(_map(_slice, start_indices, limit_indices, strides))
|
|
return operand[slices]
|
|
|
|
def dynamic_slice(operand, start_indices, slice_sizes):
|
|
out = np.zeros(slice_sizes, dtype=operand.dtype)
|
|
idx = tuple(_slice(start, start+size)
|
|
for start, size in zip(start_indices, slice_sizes))
|
|
section = operand[idx]
|
|
out[tuple(_slice(None, stop) for stop in section.shape)] = section
|
|
return out
|
|
|
|
def dynamic_update_slice(operand, update, start_indices):
|
|
slices = tuple(_map(_slice, start_indices, np.add(start_indices, update.shape)))
|
|
updated_operand = np.copy(operand)
|
|
updated_operand[slices] = update
|
|
return updated_operand
|
|
|
|
transpose = np.transpose
|
|
|
|
def reduce(operand, init_value, computation, dimensions): # pylint: disable=redefined-builtin
|
|
reducer = _make_reducer(computation, init_value)
|
|
return reducer(operand, tuple(dimensions)).astype(np.asarray(operand).dtype)
|
|
|
|
def reduce_window(operand, init_value, computation, window_dimensions,
|
|
window_strides, padding):
|
|
op, dims, strides = operand, window_dimensions, window_strides
|
|
pads = padtype_to_pads(op.shape, dims, strides, padding)
|
|
view = _conv_view(op.reshape((1, 1) + op.shape), (1, 1) + dims, strides, pads,
|
|
pad_value=init_value)[0]
|
|
view = view.reshape(view.shape[1:1+len(dims)] + (-1,))
|
|
reducer = _make_reducer(computation, init_value)
|
|
return reducer(view, axis=-1)
|
|
|
|
# TODO(mattjj): select_and_scatter
|
|
|
|
sort = np.sort
|
|
|
|
def sort_key_val(keys, values, dimension=-1):
|
|
idxs = list(np.ix_(*[np.arange(d) for d in keys.shape]))
|
|
idxs[dimension] = np.argsort(keys, axis=dimension)
|
|
return keys[tuple(idxs)], values[tuple(idxs)]
|
|
|
|
### conv util
|
|
|
|
def _conv(lhs, rhs, window_strides, pads):
|
|
view, view_axes, rhs_axes, out_axes = _conv_view(
|
|
lhs, rhs.shape, window_strides, pads, 0.)
|
|
return opt_einsum.contract(
|
|
view, view_axes, rhs, rhs_axes, out_axes, use_blas=True)
|
|
|
|
def padtype_to_pads(in_shape, filter_shape, window_strides, padding):
|
|
if padding.upper() == 'SAME':
|
|
out_shape = np.ceil(np.true_divide(in_shape, window_strides)).astype(int)
|
|
pad_sizes = [_max((out_size - 1) * stride + filter_size - in_size, 0)
|
|
for out_size, stride, filter_size, in_size
|
|
in zip(out_shape, window_strides, filter_shape, in_shape)]
|
|
return [(pad_size // 2, pad_size - pad_size // 2) for pad_size in pad_sizes]
|
|
else:
|
|
return [(0, 0)] * len(in_shape)
|
|
|
|
def _conv_view(lhs, rhs_shape, window_strides, pads, pad_value):
|
|
"""Compute the view (and its axes) of a convolution or window reduction."""
|
|
if (_min(lhs.ndim, len(rhs_shape)) < 2 or lhs.ndim != len(rhs_shape)
|
|
or lhs.shape[1] != rhs_shape[1]):
|
|
raise ValueError('Dimension mismatch')
|
|
if len(window_strides) != len(rhs_shape) - 2:
|
|
raise ValueError('Wrong number of strides for spatial dimensions')
|
|
if len(pads) != len(rhs_shape) - 2:
|
|
raise ValueError('Wrong number of pads for spatial dimensions')
|
|
|
|
lhs = _pad(lhs, [(0, 0)] * 2 + list(pads), pad_value)
|
|
in_shape = lhs.shape[2:]
|
|
filter_shape = rhs_shape[2:]
|
|
dim = len(filter_shape) # number of 'spatial' dimensions in convolution
|
|
|
|
out_strides = np.multiply(window_strides, lhs.strides[2:])
|
|
view_strides = lhs.strides[:1] + tuple(out_strides) + lhs.strides[1:]
|
|
|
|
out_shape = np.floor_divide(
|
|
np.subtract(in_shape, filter_shape), window_strides) + 1
|
|
view_shape = lhs.shape[:1] + tuple(out_shape) + rhs_shape[1:]
|
|
|
|
view = np.lib.stride_tricks.as_strided(lhs, view_shape, view_strides)
|
|
|
|
view_axes = list(range(view.ndim))
|
|
sum_axes = view_axes[-dim-1:]
|
|
rhs_axes = [view.ndim] + sum_axes
|
|
out_axes = [0, view.ndim] + list(range(1, dim+1))
|
|
|
|
return view, view_axes, rhs_axes, out_axes
|
|
|
|
def _pad(arr, pads, pad_value):
|
|
out = np.pad(arr, np.maximum(0, pads), mode='constant',
|
|
constant_values=pad_value).astype(arr.dtype)
|
|
slices = tuple(_slice(abs(lo) if lo < 0 else 0, hi % dim if hi < 0 else None)
|
|
for (lo, hi), dim in zip(pads, np.shape(arr)))
|
|
return out[slices]
|
|
|
|
def _dilate(operand, factors):
|
|
# this logic is like lax.pad, but with two leading dimensions, no edge
|
|
# padding, and factors are at least 1 (interior padding is at least 0)
|
|
outspace = np.add(operand.shape[2:],
|
|
np.multiply(np.subtract(factors, 1),
|
|
np.subtract(operand.shape[2:], 1)))
|
|
out = np.zeros(operand.shape[:2] + tuple(outspace), operand.dtype)
|
|
lhs_slices = tuple(_slice(None, None, step) for step in factors)
|
|
out[(_slice(None),) * 2 + lhs_slices] = operand
|
|
return out
|
|
|
|
def _conv_general_permutations(dimension_numbers):
|
|
lhs_spec, rhs_spec, out_spec = dimension_numbers
|
|
rhs_perm = ((rhs_spec.index('O'), rhs_spec.index('I'))
|
|
+ tuple(i for i, c in enumerate(rhs_spec) if c not in {'O', 'I'}))
|
|
lhs_perm = ((lhs_spec.index('N'), lhs_spec.index('C'))
|
|
+ tuple(sorted((i for i, c in enumerate(lhs_spec)
|
|
if c not in {'N', 'C'}),
|
|
key=lambda i: rhs_spec.index(lhs_spec[i]))))
|
|
out_perm = ((out_spec.index('N'), out_spec.index('C'))
|
|
+ tuple(sorted((i for i, c in enumerate(out_spec)
|
|
if c not in {'N', 'C'}),
|
|
key=lambda i: rhs_spec.index(out_spec[i]))))
|
|
return lhs_perm, rhs_perm, out_perm
|
|
|
|
### reduce util
|
|
|
|
def _make_reducer(py_binop, init_val):
|
|
"""Make a reducer function given a Python binop and an initial value."""
|
|
# It's tempting to use np.ufunc.reduce (even with a ufunc generated by
|
|
# np.frompyfunc(py_binop)), but this may not agree with custom init_val.
|
|
# We make an attempt to uncover an underlying numpy ufunc (which might be
|
|
# wrapped by autograd or lax) and check its identity against init_val.
|
|
monoid_record = _monoids.get(getattr(py_binop, '__name__'))
|
|
if monoid_record:
|
|
reducer, monoid_identity = monoid_record
|
|
if init_val == monoid_identity(dtypes.result_type(init_val)):
|
|
return reducer
|
|
return _reducer_from_pyfunc(py_binop, init_val)
|
|
|
|
def _get_max_identity(dt):
|
|
return -np.inf if dtypes.issubdtype(dt, np.floating) else np.iinfo(dt).min
|
|
|
|
def _get_min_identity(dt):
|
|
return np.inf if dtypes.issubdtype(dt, np.floating) else np.iinfo(dt).max
|
|
|
|
def _identity_getter(op):
|
|
return lambda dtype: np.asarray(op.identity, dtype=dtype)
|
|
|
|
MonoidRecord = collections.namedtuple('MonoidRecord', ['reducer', 'identity'])
|
|
_monoids = {
|
|
'max': MonoidRecord(np.maximum.reduce, _get_max_identity),
|
|
'min': MonoidRecord(np.minimum.reduce, _get_min_identity),
|
|
'add': MonoidRecord(np.add.reduce, _identity_getter(np.add)),
|
|
'mul': MonoidRecord(np.multiply.reduce, _identity_getter(np.multiply)),
|
|
'multiply': MonoidRecord(np.multiply.reduce,
|
|
_identity_getter(np.multiply)),
|
|
'logical_and': MonoidRecord(np.logical_and.reduce,
|
|
_identity_getter(np.logical_and)),
|
|
'logical_or': MonoidRecord(np.logical_or.reduce,
|
|
_identity_getter(np.logical_or)),
|
|
}
|
|
|
|
def _reducer_from_pyfunc(py_binop, init_val):
|
|
def reducer(operand, axis=0):
|
|
axis = range(np.ndim(operand)) if axis is None else axis
|
|
result = np.full(np.delete(np.shape(operand), axis), init_val,
|
|
dtype=np.asarray(operand).dtype)
|
|
for idx, _ in np.ndenumerate(operand):
|
|
out_idx = tuple(np.delete(idx, axis))
|
|
result[out_idx] = py_binop(result[out_idx], operand[idx])
|
|
return result
|
|
return reducer
|