mirror of
https://github.com/ROCm/jax.git
synced 2025-04-18 12:56:07 +00:00
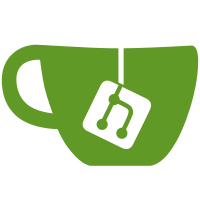
An upcoming change to XLA:CPU will disable reassociation on floating point operators by default which is an unsound fast math optimization. This change is being made to fix numerical errors in softmax computations caused by reassocation. After that change, we will enable reassociation only in reduction operators where it is very important for performance and the XLA operator contract allows that. Since this change alters the order of operations, it may cause small numerical changes leading to test failures. This change relaxes test tolerances to make tests pass. PiperOrigin-RevId: 431453240
256 lines
7.9 KiB
Python
256 lines
7.9 KiB
Python
# Copyright 2020 Google LLC
|
|
#
|
|
# Licensed under the Apache License, Version 2.0 (the "License");
|
|
# you may not use this file except in compliance with the License.
|
|
# You may obtain a copy of the License at
|
|
#
|
|
# https://www.apache.org/licenses/LICENSE-2.0
|
|
#
|
|
# Unless required by applicable law or agreed to in writing, software
|
|
# distributed under the License is distributed on an "AS IS" BASIS,
|
|
# WITHOUT WARRANTIES OR CONDITIONS OF ANY KIND, either express or implied.
|
|
# See the License for the specific language governing permissions and
|
|
# limitations under the License.
|
|
|
|
from functools import partial
|
|
|
|
from absl.testing import absltest
|
|
import numpy as np
|
|
|
|
import jax
|
|
from jax._src import test_util as jtu
|
|
import jax.numpy as jnp
|
|
from jax.experimental.ode import odeint
|
|
from jax.tree_util import tree_map
|
|
|
|
import scipy.integrate as osp_integrate
|
|
|
|
from jax.config import config
|
|
config.parse_flags_with_absl()
|
|
|
|
|
|
class ODETest(jtu.JaxTestCase):
|
|
|
|
def check_against_scipy(self, fun, y0, tspace, *args, tol=1e-1):
|
|
y0, tspace = np.array(y0), np.array(tspace)
|
|
np_fun = partial(fun, np)
|
|
scipy_result = jnp.asarray(osp_integrate.odeint(np_fun, y0, tspace, args))
|
|
|
|
y0, tspace = jnp.array(y0), jnp.array(tspace)
|
|
jax_fun = partial(fun, jnp)
|
|
jax_result = odeint(jax_fun, y0, tspace, *args)
|
|
|
|
self.assertAllClose(jax_result, scipy_result, check_dtypes=False, atol=tol, rtol=tol)
|
|
|
|
@jtu.skip_on_devices("tpu")
|
|
def test_pend_grads(self):
|
|
def pend(_np, y, _, m, g):
|
|
theta, omega = y
|
|
return [omega, -m * omega - g * _np.sin(theta)]
|
|
|
|
y0 = [np.pi - 0.1, 0.0]
|
|
ts = np.linspace(0., 1., 11)
|
|
args = (0.25, 9.8)
|
|
tol = 1e-1 if jtu.num_float_bits(np.float64) == 32 else 1e-3
|
|
|
|
self.check_against_scipy(pend, y0, ts, *args, tol=tol)
|
|
|
|
integrate = partial(odeint, partial(pend, jnp))
|
|
jtu.check_grads(integrate, (y0, ts, *args), modes=["rev"], order=2,
|
|
atol=tol, rtol=tol)
|
|
|
|
@jtu.skip_on_devices("tpu", "gpu")
|
|
def test_pytree_state(self):
|
|
"""Test calling odeint with y(t) values that are pytrees."""
|
|
def dynamics(y, _t):
|
|
return tree_map(jnp.negative, y)
|
|
|
|
y0 = (np.array(-0.1), np.array([[[0.1]]]))
|
|
ts = np.linspace(0., 1., 11)
|
|
tol = 1e-1 if jtu.num_float_bits(np.float64) == 32 else 1e-3
|
|
|
|
integrate = partial(odeint, dynamics)
|
|
jtu.check_grads(integrate, (y0, ts), modes=["rev"], order=2,
|
|
atol=tol, rtol=tol)
|
|
|
|
@jtu.skip_on_devices("tpu")
|
|
def test_weird_time_pendulum_grads(self):
|
|
"""Test that gradients are correct when the dynamics depend on t."""
|
|
def dynamics(_np, y, t):
|
|
return _np.array([y[1] * -t, -1 * y[1] - 9.8 * _np.sin(y[0])])
|
|
|
|
y0 = [np.pi - 0.1, 0.0]
|
|
ts = np.linspace(0., 1., 11)
|
|
tol = 1e-1 if jtu.num_float_bits(np.float64) == 32 else 1e-3
|
|
|
|
self.check_against_scipy(dynamics, y0, ts, tol=tol)
|
|
|
|
integrate = partial(odeint, partial(dynamics, jnp))
|
|
jtu.check_grads(integrate, (y0, ts), modes=["rev"], order=2,
|
|
rtol=tol, atol=tol)
|
|
|
|
@jtu.skip_on_devices("tpu", "gpu")
|
|
def test_decay(self):
|
|
def decay(_np, y, t, arg1, arg2):
|
|
return -_np.sqrt(t) - y + arg1 - _np.mean((y + arg2)**2)
|
|
|
|
|
|
rng = self.rng()
|
|
args = (rng.randn(3), rng.randn(3))
|
|
y0 = rng.randn(3)
|
|
ts = np.linspace(0.1, 0.2, 4)
|
|
tol = 1e-1 if jtu.num_float_bits(np.float64) == 32 else 1e-3
|
|
|
|
self.check_against_scipy(decay, y0, ts, *args, tol=tol)
|
|
|
|
integrate = partial(odeint, partial(decay, jnp))
|
|
jtu.check_grads(integrate, (y0, ts, *args), modes=["rev"], order=2,
|
|
rtol=tol, atol=tol)
|
|
|
|
@jtu.skip_on_devices("tpu", "gpu")
|
|
def test_swoop(self):
|
|
def swoop(_np, y, t, arg1, arg2):
|
|
return _np.array(y - _np.sin(t) - _np.cos(t) * arg1 + arg2)
|
|
|
|
ts = np.array([0.1, 0.2])
|
|
tol = 1e-1 if jtu.num_float_bits(np.float64) == 32 else 1e-3
|
|
y0 = np.linspace(0.1, 0.9, 10)
|
|
args = (0.1, 0.2)
|
|
|
|
self.check_against_scipy(swoop, y0, ts, *args, tol=tol)
|
|
|
|
integrate = partial(odeint, partial(swoop, jnp))
|
|
jtu.check_grads(integrate, (y0, ts, *args), modes=["rev"], order=2,
|
|
rtol=tol, atol=tol)
|
|
|
|
@jtu.skip_on_devices("tpu", "gpu")
|
|
def test_swoop_bigger(self):
|
|
def swoop(_np, y, t, arg1, arg2):
|
|
return _np.array(y - _np.sin(t) - _np.cos(t) * arg1 + arg2)
|
|
|
|
ts = np.array([0.1, 0.2])
|
|
tol = 1e-1 if jtu.num_float_bits(np.float64) == 32 else 1e-3
|
|
big_y0 = np.linspace(1.1, 10.9, 10)
|
|
args = (0.1, 0.3)
|
|
|
|
self.check_against_scipy(swoop, big_y0, ts, *args, tol=tol)
|
|
|
|
integrate = partial(odeint, partial(swoop, jnp))
|
|
jtu.check_grads(integrate, (big_y0, ts, *args), modes=["rev"], order=2,
|
|
rtol=tol, atol=tol)
|
|
|
|
@jtu.skip_on_devices("tpu", "gpu")
|
|
def test_odeint_vmap_grad(self):
|
|
# https://github.com/google/jax/issues/2531
|
|
|
|
def dx_dt(x, *args):
|
|
return 0.1 * x
|
|
|
|
def f(x, y):
|
|
y0 = jnp.array([x, y])
|
|
t = jnp.array([0., 5.])
|
|
y = odeint(dx_dt, y0, t)
|
|
return y[-1].sum()
|
|
|
|
def g(x):
|
|
# Two initial values for the ODE
|
|
y0_arr = jnp.array([[x, 0.1],
|
|
[x, 0.2]])
|
|
|
|
# Run ODE twice
|
|
t = jnp.array([0., 5.])
|
|
y = jax.vmap(lambda y0: odeint(dx_dt, y0, t))(y0_arr)
|
|
return y[:,-1].sum()
|
|
|
|
ans = jax.grad(g)(2.) # don't crash
|
|
expected = jax.grad(f, 0)(2., 0.1) + jax.grad(f, 0)(2., 0.2)
|
|
|
|
atol = {jnp.float32: 1e-5, jnp.float64: 5e-15}
|
|
rtol = {jnp.float32: 1e-5, jnp.float64: 2e-15}
|
|
self.assertAllClose(ans, expected, check_dtypes=False, atol=atol, rtol=rtol)
|
|
|
|
@jtu.skip_on_devices("tpu", "gpu")
|
|
def test_disable_jit_odeint_with_vmap(self):
|
|
# https://github.com/google/jax/issues/2598
|
|
with jax.disable_jit():
|
|
t = jnp.array([0.0, 1.0])
|
|
x0_eval = jnp.zeros((5, 2))
|
|
f = lambda x0: odeint(lambda x, _t: x, x0, t)
|
|
jax.vmap(f)(x0_eval) # doesn't crash
|
|
|
|
@jtu.skip_on_devices("tpu", "gpu")
|
|
def test_grad_closure(self):
|
|
# simplification of https://github.com/google/jax/issues/2718
|
|
def experiment(x):
|
|
def model(y, t):
|
|
return -x * y
|
|
history = odeint(model, 1., np.arange(0, 10, 0.1))
|
|
return history[-1]
|
|
jtu.check_grads(experiment, (0.01,), modes=["rev"], order=1)
|
|
|
|
@jtu.skip_on_devices("tpu", "gpu")
|
|
def test_grad_closure_with_vmap(self):
|
|
# https://github.com/google/jax/issues/2718
|
|
@jax.jit
|
|
def experiment(x):
|
|
def model(y, t):
|
|
return -x * y
|
|
history = odeint(model, 1., np.arange(0, 10, 0.1))
|
|
return history[-1]
|
|
|
|
gradfun = jax.value_and_grad(experiment)
|
|
t = np.arange(0., 1., 0.01)
|
|
h, g = jax.vmap(gradfun)(t) # doesn't crash
|
|
ans = h[11], g[11]
|
|
|
|
expected_h = experiment(t[11])
|
|
expected_g = (experiment(t[11] + 1e-5) - expected_h) / 1e-5
|
|
expected = expected_h, expected_g
|
|
|
|
self.assertAllClose(ans, expected, check_dtypes=False, atol=1e-2, rtol=1e-2)
|
|
|
|
@jtu.skip_on_devices("tpu", "gpu")
|
|
def test_forward_mode_error(self):
|
|
# https://github.com/google/jax/issues/3558
|
|
|
|
def f(k):
|
|
return odeint(lambda x, t: k*x, 1., jnp.linspace(0, 1., 50)).sum()
|
|
|
|
with self.assertRaisesRegex(TypeError, "can't apply forward-mode.*"):
|
|
jax.jacfwd(f)(3.)
|
|
|
|
@jtu.skip_on_devices("tpu", "gpu")
|
|
def test_closure_nondiff(self):
|
|
# https://github.com/google/jax/issues/3584
|
|
|
|
def dz_dt(z, t):
|
|
return jnp.stack([z[0], z[1]])
|
|
|
|
def f(z):
|
|
y = odeint(dz_dt, z, jnp.arange(10.))
|
|
return jnp.sum(y)
|
|
|
|
jax.grad(f)(jnp.ones(2)) # doesn't crash
|
|
|
|
@jtu.skip_on_devices("tpu", "gpu")
|
|
def test_complex_odeint(self):
|
|
# https://github.com/google/jax/issues/3986
|
|
# https://github.com/google/jax/issues/8757
|
|
|
|
def dy_dt(y, t, alpha):
|
|
return alpha * y * jnp.exp(-t)
|
|
|
|
def f(y0, ts, alpha):
|
|
return odeint(dy_dt, y0, ts, alpha).real
|
|
|
|
alpha = 3 + 4j
|
|
y0 = 1 + 2j
|
|
ts = jnp.linspace(0., 1., 11)
|
|
tol = 1e-1 if jtu.num_float_bits(np.float64) == 32 else 1e-3
|
|
|
|
jtu.check_grads(f, (y0, ts, alpha), modes=["rev"], order=2, atol=tol, rtol=tol)
|
|
|
|
|
|
if __name__ == '__main__':
|
|
absltest.main(testLoader=jtu.JaxTestLoader())
|