mirror of
https://github.com/ROCm/jax.git
synced 2025-04-14 10:56:06 +00:00
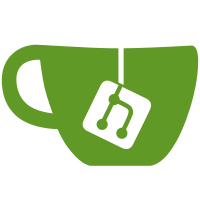
See https://opensource.google/documentation/reference/releasing/contributions#copyright for more details. PiperOrigin-RevId: 476167538
165 lines
5.6 KiB
Python
165 lines
5.6 KiB
Python
# Copyright 2020 The JAX Authors.
|
|
#
|
|
# Licensed under the Apache License, Version 2.0 (the "License");
|
|
# you may not use this file except in compliance with the License.
|
|
# You may obtain a copy of the License at
|
|
#
|
|
# https://www.apache.org/licenses/LICENSE-2.0
|
|
#
|
|
# Unless required by applicable law or agreed to in writing, software
|
|
# distributed under the License is distributed on an "AS IS" BASIS,
|
|
# WITHOUT WARRANTIES OR CONDITIONS OF ANY KIND, either express or implied.
|
|
# See the License for the specific language governing permissions and
|
|
# limitations under the License.
|
|
"""A simple Python microbenchmarking library."""
|
|
|
|
from collections import OrderedDict
|
|
import csv
|
|
import os
|
|
import time
|
|
from typing import Any, Optional, Union, Callable, List, Dict
|
|
|
|
from absl import flags
|
|
import numpy as np
|
|
from tabulate import tabulate
|
|
|
|
from jax._src.util import safe_zip
|
|
|
|
FLAGS = flags.FLAGS
|
|
flags.DEFINE_string(
|
|
"export_dir", None,
|
|
"If set, will save results as CSV files in the specified directory.")
|
|
flags.DEFINE_string(
|
|
"baseline_dir", None,
|
|
"If set, include comparison to baseline in results. Baselines should be "
|
|
"generated with --export_dir and benchmark names are matched to filenames.")
|
|
|
|
def benchmark(f: Callable[[], Any], iters: Optional[int] = None,
|
|
warmup: Optional[int] = None, name: Optional[str] = None,
|
|
target_total_secs: Optional[Union[int, float]] = None):
|
|
"""Benchmarks ``f``. Prints the results and returns the raw times.
|
|
|
|
Args:
|
|
f: The function to be benchmarked. Should take no arguments.
|
|
iters: The number of iterations to run for. If none, runs until
|
|
``target_total_secs`` has elapsed.
|
|
warmup: The number of warmup (untimed) iterations to run for.
|
|
name: The name of the benchmark. Defaults to f.__name__.
|
|
target_total_secs: If ``iters`` isn't specified, the minimum number of
|
|
seconds to run for. Defaults to the env var TARGET_TOTAL_SECS or 10 if
|
|
not set.
|
|
|
|
Returns:
|
|
An ndarray containing the number of seconds each iteration ran for.
|
|
"""
|
|
if target_total_secs is None:
|
|
target_total_secs = int(os.getenv("TARGET_TOTAL_SECS", "10"))
|
|
|
|
if warmup is None:
|
|
if iters is None:
|
|
warmup = 1
|
|
else:
|
|
warmup = np.clip(1, iters // 10, 10)
|
|
for _ in range(warmup):
|
|
f()
|
|
|
|
times: List[float] = []
|
|
count = 0
|
|
while (count < iters if iters is not None
|
|
else sum(times) < target_total_secs):
|
|
start = time.time()
|
|
f()
|
|
end = time.time()
|
|
times.append(end - start)
|
|
count += 1
|
|
|
|
times_arr = np.array(times)
|
|
print("---------Benchmark results for %s---------" % (name or f.__name__))
|
|
print(f"mean={times_arr.mean()} std={times_arr.std()} "
|
|
f"%%std={_pstd(times_arr)} total={times_arr.sum()}")
|
|
print("#iters=%d #warmup=%d" % (count, warmup))
|
|
print()
|
|
return times_arr
|
|
|
|
|
|
def benchmark_suite(prepare: Callable[..., Callable], params_list: List[Dict],
|
|
name: str, target_total_secs: Optional[int] = None):
|
|
"""Benchmarks a function for several combinations of parameters.
|
|
|
|
Prints the summarized results in a table..
|
|
|
|
Args:
|
|
prepare: given kwargs returns a benchmark function specialized to the kwargs.
|
|
params_list: a list of kwargs on which to run the benchmark.
|
|
name: the name of this benchmark suite
|
|
target_total_secs: the ``target_total_secs`` to pass to ``benchmark``.
|
|
"""
|
|
# Sort parameters alphabetically so benchmark results print consistently.
|
|
params_list = [OrderedDict(sorted(p.items())) for p in params_list]
|
|
assert all(p.keys() == params_list[0].keys() for p in params_list)
|
|
|
|
times = []
|
|
for params in params_list:
|
|
f = prepare(**params)
|
|
subname = name + "".join(f"_{n}={_param_str(p)}"
|
|
for n, p in params.items())
|
|
times.append(benchmark(f, name=subname,
|
|
target_total_secs=target_total_secs))
|
|
|
|
param_names = list(params_list[0].keys())
|
|
data_header = param_names + ["mean", "%std", "relative"]
|
|
data = [list(map(_param_str, params.values())) +
|
|
[t.mean(), _pstd(t), t.mean() / times[0].mean()]
|
|
for params, t in safe_zip(params_list, times)]
|
|
|
|
if FLAGS.baseline_dir:
|
|
mean_idx = len(param_names)
|
|
means = _get_baseline_means(FLAGS.baseline_dir, name)
|
|
assert len(means) == len(data), (means, data)
|
|
data_header.append("mean/baseline")
|
|
for idx, mean in enumerate(means):
|
|
data[idx].append(data[idx][mean_idx] / mean)
|
|
|
|
print("---------Benchmark summary for %s---------" % name)
|
|
print(tabulate(data, data_header))
|
|
print()
|
|
|
|
if FLAGS.export_dir:
|
|
filename = _export_results(data_header, data, FLAGS.export_dir, name)
|
|
print(f"Wrote {name} results to {filename}")
|
|
print()
|
|
|
|
|
|
def _get_baseline_means(baseline_dir, name):
|
|
baseline_dir = os.path.expanduser(baseline_dir)
|
|
filename = os.path.join(baseline_dir, name + ".csv")
|
|
if not os.path.exists(filename):
|
|
raise FileNotFoundError("Can't find baseline file: %s" % filename)
|
|
with open(filename, newline="") as csvfile:
|
|
reader = csv.reader(csvfile)
|
|
header = next(reader)
|
|
mean_idx = header.index("mean")
|
|
return [float(row[mean_idx]) for row in reader]
|
|
|
|
|
|
def _export_results(data_header, data, export_dir, name):
|
|
assert "mean" in data_header # For future comparisons via _get_baseline_means
|
|
export_dir = os.path.expanduser(export_dir)
|
|
os.makedirs(export_dir, exist_ok=True)
|
|
filename = os.path.join(export_dir, name + ".csv")
|
|
with open(filename, "w", newline="") as csvfile:
|
|
writer = csv.writer(csvfile)
|
|
writer.writerow(data_header)
|
|
writer.writerows(data)
|
|
return filename
|
|
|
|
|
|
def _param_str(param):
|
|
if callable(param):
|
|
return param.__name__
|
|
return str(param)
|
|
|
|
|
|
def _pstd(x):
|
|
return x.std() / x.mean() * 100
|