mirror of
https://github.com/ROCm/jax.git
synced 2025-04-14 10:56:06 +00:00
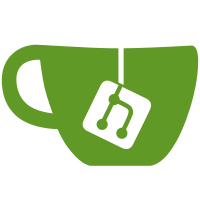
See https://opensource.google/documentation/reference/releasing/contributions#copyright for more details. PiperOrigin-RevId: 476167538
1176 lines
47 KiB
Python
1176 lines
47 KiB
Python
# Copyright 2020 The JAX Authors.
|
|
#
|
|
# Licensed under the Apache License, Version 2.0 (the "License");
|
|
# you may not use this file except in compliance with the License.
|
|
# You may obtain a copy of the License at
|
|
#
|
|
# https://www.apache.org/licenses/LICENSE-2.0
|
|
#
|
|
# Unless required by applicable law or agreed to in writing, software
|
|
# distributed under the License is distributed on an "AS IS" BASIS,
|
|
# WITHOUT WARRANTIES OR CONDITIONS OF ANY KIND, either express or implied.
|
|
# See the License for the specific language governing permissions and
|
|
# limitations under the License.
|
|
|
|
from functools import update_wrapper, reduce, partial
|
|
import inspect
|
|
import operator as op
|
|
from typing import (Callable, Generic, Optional, Sequence, Tuple, TypeVar, Set,
|
|
Any)
|
|
|
|
from jax import core
|
|
from jax import linear_util as lu
|
|
from jax.custom_transpose import custom_transpose
|
|
from jax.tree_util import (tree_flatten, tree_unflatten, tree_map,
|
|
treedef_is_leaf, treedef_tuple,
|
|
register_pytree_node_class, tree_leaves)
|
|
from jax._src import custom_api_util
|
|
from jax._src import dtypes
|
|
from jax._src.lax import lax
|
|
from jax._src.util import cache, safe_zip, safe_map, split_list, Unhashable
|
|
from jax._src.api_util import flatten_fun_nokwargs, argnums_partial
|
|
from jax.core import raise_to_shaped
|
|
from jax.errors import UnexpectedTracerError
|
|
from jax._src.ad_util import Zero, zeros_like_aval, stop_gradient_p
|
|
from jax.interpreters import partial_eval as pe
|
|
from jax.interpreters import ad
|
|
from jax.interpreters import batching
|
|
from jax.interpreters import mlir
|
|
from jax.interpreters import xla
|
|
from jax.interpreters.batching import not_mapped
|
|
from jax.config import config
|
|
|
|
from jax._src import traceback_util
|
|
traceback_util.register_exclusion(__file__)
|
|
|
|
map = safe_map
|
|
zip = safe_zip
|
|
|
|
|
|
### util
|
|
|
|
def _resolve_kwargs(fun, args, kwargs):
|
|
ba = inspect.signature(fun).bind(*args, **kwargs)
|
|
ba.apply_defaults()
|
|
if ba.kwargs:
|
|
raise TypeError("keyword arguments could not be resolved to positions")
|
|
else:
|
|
return ba.args
|
|
|
|
def _initial_style_jaxpr(fun, in_avals):
|
|
jaxpr, _, consts = pe.trace_to_jaxpr_dynamic(fun, in_avals)
|
|
return jaxpr, consts
|
|
|
|
def _close_jaxpr(jaxpr):
|
|
return core.ClosedJaxpr(pe.convert_constvars_jaxpr(jaxpr), ())
|
|
|
|
def _initial_style_staging() -> bool:
|
|
return core.thread_local_state.trace_state.initial_style
|
|
|
|
def _sum_tangents(_, x, *xs):
|
|
return reduce(ad.add_tangents, xs, x)
|
|
|
|
def _zeros_like_pytree(x):
|
|
return tree_map(Zero.from_value, x)
|
|
|
|
@partial(partial, tree_map)
|
|
def _stop_gradient(x):
|
|
if isinstance(x, core.Tracer):
|
|
return stop_gradient_p.bind(x)
|
|
else:
|
|
return x
|
|
|
|
|
|
### JVPs
|
|
ReturnValue = TypeVar('ReturnValue')
|
|
|
|
@custom_api_util.register_custom_decorator_type
|
|
class custom_jvp(Generic[ReturnValue]):
|
|
"""Set up a JAX-transformable function for a custom JVP rule definition.
|
|
|
|
This class is meant to be used as a function decorator. Instances are
|
|
callables that behave similarly to the underlying function to which the
|
|
decorator was applied, except when a differentiation transformation (like
|
|
:py:func:`jax.jvp` or :py:func:`jax.grad`) is applied, in which case a custom
|
|
user-supplied JVP rule function is used instead of tracing into and
|
|
performing automatic differentiation of the underlying function's
|
|
implementation.
|
|
|
|
There are two instance methods available for defining the custom JVP rule:
|
|
:py:func:`~jax.custom_jvp.defjvp` for defining a *single* custom JVP rule for
|
|
all the function's inputs, and for convenience
|
|
:py:func:`~jax.custom_jvp.defjvps`, which wraps
|
|
:py:func:`~jax.custom_jvp.defjvp`, and allows you to provide separate
|
|
definitions for the partial derivatives of the function w.r.t. each of its
|
|
arguments.
|
|
|
|
For example::
|
|
|
|
@jax.custom_jvp
|
|
def f(x, y):
|
|
return jnp.sin(x) * y
|
|
|
|
@f.defjvp
|
|
def f_jvp(primals, tangents):
|
|
x, y = primals
|
|
x_dot, y_dot = tangents
|
|
primal_out = f(x, y)
|
|
tangent_out = jnp.cos(x) * x_dot * y + jnp.sin(x) * y_dot
|
|
return primal_out, tangent_out
|
|
|
|
For a more detailed introduction, see the tutorial_.
|
|
|
|
.. _tutorial: https://jax.readthedocs.io/en/latest/notebooks/Custom_derivative_rules_for_Python_code.html
|
|
"""
|
|
|
|
def __init__(self,
|
|
fun: Callable[..., ReturnValue],
|
|
nondiff_argnums: Tuple[int, ...] = ()):
|
|
update_wrapper(self, fun)
|
|
self.fun = fun
|
|
self.nondiff_argnums = nondiff_argnums
|
|
self.jvp: Optional[Callable[..., Tuple[ReturnValue, ReturnValue]]] = None
|
|
|
|
__getattr__ = custom_api_util.forward_attr
|
|
|
|
def defjvp(self, jvp: Callable[..., Tuple[ReturnValue, ReturnValue]]
|
|
) -> Callable[..., Tuple[ReturnValue, ReturnValue]]:
|
|
"""Define a custom JVP rule for the function represented by this instance.
|
|
|
|
Args:
|
|
jvp: a Python callable representing the custom JVP rule. When there are no
|
|
``nondiff_argnums``, the ``jvp`` function should accept two arguments,
|
|
where the first is a tuple of primal inputs and the second is a tuple of
|
|
tangent inputs. The lengths of both tuples are equal to the number of
|
|
parameters of the ``custom_jvp`` function. The ``jvp`` function should
|
|
produce as output a pair where the first element is the primal output
|
|
and the second element is the tangent output. Elements of the input and
|
|
output tuples may be arrays or any nested tuples/lists/dicts thereof.
|
|
|
|
Returns:
|
|
None.
|
|
|
|
Example::
|
|
|
|
@jax.custom_jvp
|
|
def f(x, y):
|
|
return jnp.sin(x) * y
|
|
|
|
@f.defjvp
|
|
def f_jvp(primals, tangents):
|
|
x, y = primals
|
|
x_dot, y_dot = tangents
|
|
primal_out = f(x, y)
|
|
tangent_out = jnp.cos(x) * x_dot * y + jnp.sin(x) * y_dot
|
|
return primal_out, tangent_out
|
|
"""
|
|
self.jvp = jvp
|
|
return jvp
|
|
|
|
def defjvps(self, *jvps: Optional[Callable[..., ReturnValue]]):
|
|
"""Convenience wrapper for defining JVPs for each argument separately.
|
|
|
|
This convenience wrapper cannot be used together with ``nondiff_argnums``.
|
|
|
|
Args:
|
|
*jvps: a sequence of functions, one for each positional argument of the
|
|
``custom_jvp`` function. Each function takes as arguments the tangent
|
|
value for the corresponding primal input, the primal output, and the
|
|
primal inputs. See the example below.
|
|
|
|
Returns:
|
|
None.
|
|
|
|
Example::
|
|
|
|
@jax.custom_jvp
|
|
def f(x, y):
|
|
return jnp.sin(x) * y
|
|
|
|
f.defjvps(lambda x_dot, primal_out, x, y: jnp.cos(x) * x_dot * y,
|
|
lambda y_dot, primal_out, x, y: jnp.sin(x) * y_dot)
|
|
"""
|
|
if self.nondiff_argnums:
|
|
raise TypeError("Can't use ``defjvps`` with ``nondiff_argnums``.")
|
|
|
|
def jvp(primals, tangents):
|
|
primal_out = self(*primals)
|
|
zeros = _zeros_like_pytree(primal_out)
|
|
all_tangents_out = [jvp(t, primal_out, *primals) if jvp else zeros
|
|
for t, jvp in zip(tangents, jvps)]
|
|
tangent_out = tree_map(_sum_tangents, primal_out, *all_tangents_out)
|
|
return primal_out, tangent_out
|
|
|
|
self.defjvp(jvp)
|
|
|
|
@traceback_util.api_boundary
|
|
def __call__(self, *args: Any, **kwargs: Any) -> ReturnValue: # pytype: disable=invalid-annotation
|
|
if not self.jvp:
|
|
msg = "No JVP defined for custom_jvp function {} using defjvp."
|
|
raise AttributeError(msg.format(self.__name__))
|
|
args = _resolve_kwargs(self.fun, args, kwargs)
|
|
if self.nondiff_argnums:
|
|
nondiff_argnums = set(self.nondiff_argnums)
|
|
args = tuple(_stop_gradient(x) if i in nondiff_argnums else x
|
|
for i, x in enumerate(args))
|
|
diff_argnums = [i for i in range(len(args)) if i not in nondiff_argnums]
|
|
f_, dyn_args = argnums_partial(lu.wrap_init(self.fun), diff_argnums, args,
|
|
require_static_args_hashable=False)
|
|
static_args = [args[i] for i in self.nondiff_argnums]
|
|
jvp = _add_args(lu.wrap_init(self.jvp), static_args)
|
|
else:
|
|
f_, dyn_args = lu.wrap_init(self.fun), args
|
|
jvp = lu.wrap_init(self.jvp)
|
|
args_flat, in_tree = tree_flatten(dyn_args)
|
|
flat_fun, out_tree1 = flatten_fun_nokwargs(f_, in_tree)
|
|
flat_jvp, out_tree2 = _flatten_jvp(jvp, in_tree)
|
|
out_flat = custom_jvp_call_p.bind(flat_fun, flat_jvp, *args_flat)
|
|
_, out_tree = lu.merge_linear_aux(out_tree1, out_tree2)
|
|
return tree_unflatten(out_tree, out_flat)
|
|
|
|
def _add_args(f, extra_args):
|
|
return _add_args_(f, tuple(Unhashable(arg) for arg in extra_args))
|
|
|
|
@lu.transformation
|
|
def _add_args_(extra_args, *args, **kwargs):
|
|
extra_args = tuple(arg.val for arg in extra_args)
|
|
all_args = (extra_args + args)
|
|
yield (yield all_args, kwargs)
|
|
|
|
@lu.transformation_with_aux
|
|
def _flatten_jvp(in_tree, *args):
|
|
primals_in, tangents_in = split_list(args, [len(args) // 2])
|
|
py_primals = tree_unflatten(in_tree, primals_in)
|
|
py_tangents = tree_unflatten(in_tree, tangents_in)
|
|
pair_out = yield (py_primals, py_tangents), {}
|
|
if not isinstance(pair_out, (list, tuple)) or len(pair_out) != 2:
|
|
msg = ("Custom JVP rule must produce a pair (list or tuple of length two) "
|
|
"representing primal and tangent outputs, got {}.")
|
|
raise TypeError(msg.format(pair_out))
|
|
py_primals_out, py_tangents_out = pair_out
|
|
primals_out, out_tree = tree_flatten(py_primals_out)
|
|
tangents_out, out_tree2 = tree_flatten(py_tangents_out)
|
|
if out_tree != out_tree2:
|
|
msg = ("Custom JVP rule must produce primal and tangent outputs with equal "
|
|
"container (pytree) structures, but got {} and {} respectively.")
|
|
raise TypeError(msg.format(out_tree, out_tree2))
|
|
# TODO(mattjj): compare primals' tangent types to tangent objects' types
|
|
primal_avals_out = [
|
|
raise_to_shaped(core.get_aval(x), weak_type=False).strip_named_shape()
|
|
for x in primals_out]
|
|
tangent_avals_out = [
|
|
raise_to_shaped(core.get_aval(t), weak_type=False).strip_named_shape()
|
|
for t in tangents_out]
|
|
if primal_avals_out != tangent_avals_out:
|
|
if len(primal_avals_out) == 1:
|
|
(av1,), (av2,) = primal_avals_out, tangent_avals_out
|
|
msg = ("Custom JVP rule must produce primal and tangent outputs with "
|
|
"equal shapes and dtypes, but got {} and {} respectively.")
|
|
raise TypeError(msg.format(av1.str_short(), av2.str_short()))
|
|
else:
|
|
msg = ("Custom JVP rule must produce primal and tangent outputs with "
|
|
"equal shapes and dtypes, but got:\n{}")
|
|
disagreements = (
|
|
f" primal {av1.str_short()} for tangent {av2.str_short()}"
|
|
for av1, av2 in zip(primal_avals_out, tangent_avals_out) if av1 != av2)
|
|
raise TypeError(msg.format('\n'.join(disagreements)))
|
|
yield primals_out + tangents_out, out_tree
|
|
|
|
class CustomJVPCallPrimitive(core.Primitive):
|
|
multiple_results = True
|
|
|
|
def bind(self, fun, jvp, *args):
|
|
args = map(core.full_lower, args)
|
|
top_trace = core.find_top_trace(args)
|
|
fun, env_trace_todo1 = process_env_traces(
|
|
fun, self, top_trace and top_trace.level, False)
|
|
jvp, env_trace_todo2 = process_env_traces(
|
|
jvp, self, top_trace and top_trace.level, True)
|
|
tracers = map(top_trace.full_raise, args) # type: ignore
|
|
outs = top_trace.process_custom_jvp_call(self, fun, jvp, tracers) # type: ignore
|
|
_, env_trace_todo = lu.merge_linear_aux(env_trace_todo1, env_trace_todo2)
|
|
return _apply_todos(env_trace_todo, map(core.full_lower, outs))
|
|
|
|
def impl(self, fun, _, *args):
|
|
with core.new_sublevel():
|
|
return fun.call_wrapped(*args)
|
|
|
|
def post_process(self, trace, out_tracers, jvp_was_run: bool):
|
|
return trace.post_process_custom_jvp_call(out_tracers, jvp_was_run)
|
|
|
|
def get_bind_params(self, params):
|
|
new_params = dict(params)
|
|
call_jaxpr = new_params.pop('call_jaxpr')
|
|
num_consts = new_params.pop('num_consts')
|
|
jvp_jaxpr_thunk = new_params.pop('jvp_jaxpr_thunk')
|
|
fun = lu.wrap_init(core.jaxpr_as_fun(call_jaxpr))
|
|
|
|
@lu.wrap_init
|
|
def jvp(*xs):
|
|
jvp_jaxpr, jvp_consts = jvp_jaxpr_thunk()
|
|
n, ragged = divmod(len(xs), 2)
|
|
assert not ragged
|
|
primals, tangents = xs[num_consts:n], xs[n+num_consts:]
|
|
return core.eval_jaxpr(jvp_jaxpr, jvp_consts, *primals, *tangents)
|
|
|
|
return [fun, jvp], new_params
|
|
|
|
@lu.transformation_with_aux
|
|
def process_env_traces(primitive, level: int, jvp_was_run: bool, *args):
|
|
outs = yield args, {}
|
|
todo = []
|
|
while True:
|
|
tracers = [x for x in outs if isinstance(x, core.Tracer)
|
|
and (level is None or x._trace.level > level)]
|
|
if tracers:
|
|
ans = max(tracers, key=lambda x: x._trace.level)
|
|
else:
|
|
break
|
|
trace = ans._trace.main.with_cur_sublevel()
|
|
outs = map(trace.full_raise, outs)
|
|
outs, cur_todo = primitive.post_process(trace, outs, jvp_was_run)
|
|
todo.append(cur_todo)
|
|
yield outs, tuple(todo) # Ensure the aux output is immutable
|
|
|
|
def _apply_todos(todos, outs):
|
|
todos_list = list(todos)
|
|
while todos_list:
|
|
outs = map(core.full_lower, todos_list.pop()(outs))
|
|
return outs
|
|
|
|
|
|
allowed_effects: Set[core.Effect] = set()
|
|
allowed_effects.add(lax.InOutFeedEffect.Infeed)
|
|
allowed_effects.add(lax.InOutFeedEffect.Outfeed)
|
|
|
|
|
|
custom_jvp_call_p = CustomJVPCallPrimitive('custom_jvp_call')
|
|
|
|
def _custom_jvp_call_typecheck(*in_avals, call_jaxpr, jvp_jaxpr_thunk, num_consts):
|
|
# TODO(mattjj): could do more checking here...
|
|
del in_avals, jvp_jaxpr_thunk, num_consts
|
|
disallowed_effects = {eff for eff in call_jaxpr.effects if eff not in
|
|
allowed_effects}
|
|
if disallowed_effects:
|
|
raise NotImplementedError(
|
|
f'Effects not supported in `custom_jvp`: {disallowed_effects}')
|
|
return call_jaxpr.out_avals, call_jaxpr.effects
|
|
core.custom_typechecks[custom_jvp_call_p] = _custom_jvp_call_typecheck
|
|
|
|
def _custom_jvp_call_mlir_translation(ctx, *args, call_jaxpr, jvp_jaxpr_thunk,
|
|
num_consts):
|
|
del jvp_jaxpr_thunk, num_consts
|
|
args_ = map(mlir.wrap_singleton_ir_values, args)
|
|
consts = mlir._ir_consts(call_jaxpr.consts)
|
|
out, tokens = mlir.jaxpr_subcomp(ctx.module_context, call_jaxpr.jaxpr,
|
|
ctx.tokens_in, consts, *args_)
|
|
ctx.set_tokens_out(tokens)
|
|
return out
|
|
mlir.register_lowering(custom_jvp_call_p, _custom_jvp_call_mlir_translation)
|
|
|
|
# If a (multi)linear function is defined with a custom jvp, then
|
|
# custom_jvp_call_ can appear in jaxprs to be transposed. Since it's already
|
|
# been linearized, we can drop the jvp rule.
|
|
def _custom_jvp_call_transpose(params, jaxpr, args, ct, _, reduce_axes):
|
|
del params
|
|
return ad.backward_pass(jaxpr.jaxpr, reduce_axes, None, jaxpr.consts, args, ct)
|
|
ad.primitive_transposes[custom_jvp_call_p] = _custom_jvp_call_transpose
|
|
|
|
|
|
### VJPs
|
|
|
|
@custom_api_util.register_custom_decorator_type
|
|
class custom_vjp(Generic[ReturnValue]):
|
|
"""Set up a JAX-transformable function for a custom VJP rule definition.
|
|
|
|
This class is meant to be used as a function decorator. Instances are
|
|
callables that behave similarly to the underlying function to which the
|
|
decorator was applied, except when a reverse-mode differentiation
|
|
transformation (like :py:func:`jax.grad`) is applied, in which case a custom
|
|
user-supplied VJP rule function is used instead of tracing into and performing
|
|
automatic differentiation of the underlying function's implementation. There
|
|
is a single instance method, :py:func:`~jax.custom_vjp.defvjp`, which may be
|
|
used to define the custom VJP rule.
|
|
|
|
This decorator precludes the use of forward-mode automatic differentiation.
|
|
|
|
For example::
|
|
|
|
@jax.custom_vjp
|
|
def f(x, y):
|
|
return jnp.sin(x) * y
|
|
|
|
def f_fwd(x, y):
|
|
return f(x, y), (jnp.cos(x), jnp.sin(x), y)
|
|
|
|
def f_bwd(res, g):
|
|
cos_x, sin_x, y = res
|
|
return (cos_x * g * y, sin_x * g)
|
|
|
|
f.defvjp(f_fwd, f_bwd)
|
|
|
|
For a more detailed introduction, see the tutorial_.
|
|
|
|
.. _tutorial: https://jax.readthedocs.io/en/latest/notebooks/Custom_derivative_rules_for_Python_code.html
|
|
"""
|
|
|
|
def __init__(self,
|
|
fun: Callable[..., ReturnValue],
|
|
nondiff_argnums: Tuple[int, ...] = ()):
|
|
update_wrapper(self, fun)
|
|
self.fun = fun
|
|
self.nondiff_argnums = nondiff_argnums
|
|
self.fwd: Optional[Callable[..., Tuple[ReturnValue, Any]]] = None
|
|
self.bwd: Optional[Callable[..., Tuple[Any, ...]]] = None
|
|
|
|
__getattr__ = custom_api_util.forward_attr
|
|
|
|
def defvjp(self,
|
|
fwd: Callable[..., Tuple[ReturnValue, Any]],
|
|
bwd: Callable[..., Tuple[Any, ...]]) -> None:
|
|
"""Define a custom VJP rule for the function represented by this instance.
|
|
|
|
Args:
|
|
fwd: a Python callable representing the forward pass of the custom VJP
|
|
rule. When there are no ``nondiff_argnums``, the ``fwd`` function has
|
|
the same input signature as the underlying primal function. It should
|
|
return as output a pair, where the first element represents the primal
|
|
output and the second element represents any "residual" values to store
|
|
from the forward pass for use on the backward pass by the function
|
|
``bwd``. Input arguments and elements of the output pair may be arrays
|
|
or nested tuples/lists/dicts thereof.
|
|
bwd: a Python callable representing the backward pass of the custom VJP
|
|
rule. When there are no ``nondiff_argnums``, the ``bwd`` function takes
|
|
two arguments, where the first is the "residual" values produced on the
|
|
forward pass by ``fwd``, and the second is the output cotangent with the
|
|
same structure as the primal function output. The output of ``bwd`` must
|
|
be a tuple of length equal to the number of arguments of the primal
|
|
function, and the tuple elements may be arrays or nested
|
|
tuples/lists/dicts thereof so as to match the structure of the primal
|
|
input arguments.
|
|
|
|
Returns:
|
|
None.
|
|
|
|
Example::
|
|
|
|
@jax.custom_vjp
|
|
def f(x, y):
|
|
return jnp.sin(x) * y
|
|
|
|
def f_fwd(x, y):
|
|
return f(x, y), (jnp.cos(x), jnp.sin(x), y)
|
|
|
|
def f_bwd(res, g):
|
|
cos_x, sin_x, y = res
|
|
return (cos_x * g * y, sin_x * g)
|
|
|
|
f.defvjp(f_fwd, f_bwd)
|
|
"""
|
|
self.fwd = fwd
|
|
self.bwd = bwd
|
|
|
|
@traceback_util.api_boundary
|
|
def __call__(self, *args: Any, **kwargs: Any) -> ReturnValue: # pytype: disable=invalid-annotation
|
|
if not self.fwd or not self.bwd:
|
|
msg = "No VJP defined for custom_vjp function {} using defvjp."
|
|
raise AttributeError(msg.format(self.__name__))
|
|
args = _resolve_kwargs(self.fun, args, kwargs)
|
|
if config.jax_enable_custom_vjp_by_custom_transpose:
|
|
if self.nondiff_argnums:
|
|
raise NotImplementedError(
|
|
'nondiff_argnums not implemented for new custom_vjp')
|
|
return custom_vjp_by_custom_transpose(self.fun, self.fwd, self.bwd)(*args)
|
|
else:
|
|
if self.nondiff_argnums:
|
|
for i in self.nondiff_argnums: _check_for_tracers(args[i])
|
|
nondiff_argnums = set(self.nondiff_argnums)
|
|
dyn_argnums = [i for i in range(len(args)) if i not in nondiff_argnums]
|
|
f_, dyn_args = argnums_partial(lu.wrap_init(self.fun), dyn_argnums,
|
|
args, require_static_args_hashable=False)
|
|
static_args = [args[i] for i in self.nondiff_argnums]
|
|
fwd, _ = argnums_partial(lu.wrap_init(self.fwd), dyn_argnums, args,
|
|
require_static_args_hashable=False)
|
|
bwd = _add_args(lu.wrap_init(self.bwd), static_args)
|
|
else:
|
|
f_, dyn_args = lu.wrap_init(self.fun), args
|
|
fwd, bwd = lu.wrap_init(self.fwd), lu.wrap_init(self.bwd)
|
|
args_flat, in_tree = tree_flatten(dyn_args)
|
|
in_avals = [core.raise_to_shaped(core.get_aval(x)) for x in args_flat]
|
|
flat_fun, out_tree = flatten_fun_nokwargs(f_, in_tree)
|
|
flat_fwd, out_trees = _flatten_fwd(fwd, in_tree)
|
|
flat_bwd = _flatten_bwd(bwd, in_tree, in_avals, out_trees)
|
|
out_flat = custom_vjp_call_p.bind(flat_fun, flat_fwd, flat_bwd,
|
|
*args_flat, out_trees=out_trees)
|
|
fst, aux = lu.merge_linear_aux(out_tree, out_trees)
|
|
out_tree = aux if fst else aux[0]
|
|
return tree_unflatten(out_tree, out_flat)
|
|
|
|
def _check_for_tracers(x):
|
|
for leaf in tree_leaves(x):
|
|
if isinstance(x, core.Tracer):
|
|
msg = ("Found a JAX Tracer object passed as an argument to a custom_vjp "
|
|
"function in a position indicated by nondiff_argnums as "
|
|
"non-differentiable. Tracers cannot be passed as non-differentiable "
|
|
"arguments to custom_vjp functions; instead, nondiff_argnums should "
|
|
"only be used for arguments that can't be or contain JAX tracers, "
|
|
"e.g. function-valued arguments. In particular, array-valued "
|
|
"arguments should typically not be indicated as nondiff_argnums.")
|
|
raise UnexpectedTracerError(msg)
|
|
|
|
@lu.transformation_with_aux
|
|
def _flatten_fwd(in_tree, *args):
|
|
py_args = tree_unflatten(in_tree, args)
|
|
pair_out = yield py_args, {}
|
|
if not isinstance(pair_out, (list, tuple)) or len(pair_out) != 2:
|
|
msg = ("Custom VJP fwd function must produce a pair (list or tuple of "
|
|
"length two) representing primal outputs and residuals (values "
|
|
"stored from the forward pass for use on the backward pass), "
|
|
"got {}.")
|
|
raise TypeError(msg.format(pair_out))
|
|
py_outs, res = pair_out
|
|
out, out_tree = tree_flatten(py_outs)
|
|
res, res_tree = tree_flatten(res)
|
|
yield res + out, (out_tree, res_tree)
|
|
|
|
@lu.transformation
|
|
def _flatten_bwd(in_tree, in_avals, out_trees, *args):
|
|
out_tree, res_tree = out_trees()
|
|
assert len(args) == res_tree.num_leaves + out_tree.num_leaves
|
|
res, cts_out = split_list(args, [res_tree.num_leaves])
|
|
py_res = tree_unflatten(res_tree, res)
|
|
py_cts_out = tree_unflatten(out_tree, cts_out)
|
|
py_cts_in = yield (py_res, py_cts_out), {}
|
|
# For each None in py_cts_in, indicating an argument for which the rule
|
|
# produces no cotangent, we replace it with a pytree with the structure of the
|
|
# corresponding subtree of in_tree and with leaves of a non-pytree sentinel
|
|
# object, to be replaced with Nones in the final returned result.
|
|
zero = object() # non-pytree sentinel to replace Nones in py_cts_in
|
|
dummy = tree_unflatten(in_tree, [object()] * in_tree.num_leaves)
|
|
cts_in_flat = []
|
|
append = lambda x, d: cts_in_flat.extend([x] * len(tree_flatten(d)[0])) or x
|
|
try:
|
|
if not isinstance(py_cts_in, tuple):
|
|
raise ValueError
|
|
tree_map(append,
|
|
tuple(zero if ct is None else ct for ct in py_cts_in), dummy)
|
|
except ValueError:
|
|
_, in_tree2 = tree_flatten(py_cts_in)
|
|
msg = ("Custom VJP rule must produce an output with the same container "
|
|
"(pytree) structure as the args tuple of the primal function, "
|
|
"and in particular must produce a tuple of length equal to the "
|
|
"number of arguments to the primal function, but got VJP output "
|
|
"structure {} for primal input structure {}.")
|
|
raise TypeError(msg.format(in_tree2, in_tree)) from None
|
|
# Ignore any None cotangents, and any corresponding to inputs for which the
|
|
# type doesn't equal the tangent type (i.e. float0s)
|
|
# TODO(mattjj): change this to check if tangent type represents 0dim vspace
|
|
yield [Zero(a.at_least_vspace()) if ct is zero or a != a.at_least_vspace()
|
|
else ct for a, ct in zip(in_avals, cts_in_flat)]
|
|
|
|
|
|
class CustomVJPCallPrimitive(core.CallPrimitive):
|
|
initial_style: core.Primitive
|
|
|
|
def bind(self, fun, fwd, bwd, *args, out_trees):
|
|
args = map(core.full_lower, args)
|
|
top_trace = core.find_top_trace(args)
|
|
fun, env_trace_todo1 = process_env_traces(
|
|
fun, self, top_trace and top_trace.level, False)
|
|
fwd, env_trace_todo2 = process_env_traces_fwd(
|
|
fwd, top_trace and top_trace.level, out_trees)
|
|
tracers = map(top_trace.full_raise, args) # type: ignore
|
|
bwd_ = lu.wrap_init(lambda *args: bwd.call_wrapped(*args))
|
|
outs = top_trace.process_custom_vjp_call(self, fun, fwd, bwd_, tracers,
|
|
out_trees=out_trees)
|
|
fst, env_trace_todo = lu.merge_linear_aux(env_trace_todo1, env_trace_todo2)
|
|
if fst:
|
|
return _apply_todos(env_trace_todo, map(core.full_lower, outs))
|
|
else:
|
|
env_trace_todo, bwd_transform = env_trace_todo
|
|
bwd = _apply_bwd_transform(bwd_transform, bwd)
|
|
return _apply_todos(env_trace_todo, map(core.full_lower, outs))
|
|
|
|
def impl(self, fun, fwd, bwd, *args, out_trees):
|
|
del fwd, bwd, out_trees
|
|
with core.new_sublevel():
|
|
return fun.call_wrapped(*args)
|
|
|
|
def post_process(self, trace, out_tracers, params):
|
|
return trace.post_process_custom_vjp_call(out_tracers, params)
|
|
custom_vjp_call_p = CustomVJPCallPrimitive('custom_vjp_call')
|
|
|
|
@lu.transformation_with_aux
|
|
def process_env_traces_fwd(level: int, out_trees, *args):
|
|
outs = yield args, {}
|
|
todo = []
|
|
bwd_transforms = []
|
|
while True:
|
|
tracers = [x for x in outs if isinstance(x, core.Tracer)
|
|
and (level is None or x._trace.level > level)]
|
|
if tracers:
|
|
ans = max(tracers, key=lambda x: x._trace.level)
|
|
else:
|
|
break
|
|
trace = ans._trace.main.with_cur_sublevel()
|
|
outs = map(trace.full_raise, outs)
|
|
outs, cur_todo, bwd_xform = trace.post_process_custom_vjp_call_fwd(outs, out_trees)
|
|
todo.append(cur_todo)
|
|
bwd_transforms.append(bwd_xform)
|
|
yield outs, (tuple(todo), tuple(bwd_transforms))
|
|
|
|
|
|
def _apply_bwd_transform(todos, bwd):
|
|
todos_list = list(todos)
|
|
while todos_list:
|
|
bwd = todos_list.pop()(bwd)
|
|
return bwd
|
|
|
|
def _custom_vjp_call_jaxpr_impl(*args, fun_jaxpr, **_):
|
|
return core.jaxpr_as_fun(fun_jaxpr)(*args)
|
|
|
|
def _custom_vjp_call_jaxpr_abstract_eval(*_, fun_jaxpr, **__):
|
|
disallowed_effects = {eff for eff in fun_jaxpr.effects if eff not in
|
|
allowed_effects}
|
|
if disallowed_effects:
|
|
raise NotImplementedError(
|
|
f'Effects not supported in `custom_vjp`: {disallowed_effects}')
|
|
return fun_jaxpr.out_avals, fun_jaxpr.effects
|
|
|
|
custom_vjp_call_jaxpr_p = core.AxisPrimitive('custom_vjp_call_jaxpr')
|
|
custom_vjp_call_jaxpr_p.multiple_results = True
|
|
custom_vjp_call_jaxpr_p.def_impl(_custom_vjp_call_jaxpr_impl)
|
|
custom_vjp_call_jaxpr_p.def_effectful_abstract_eval(_custom_vjp_call_jaxpr_abstract_eval)
|
|
CustomVJPCallPrimitive.initial_style = custom_vjp_call_jaxpr_p
|
|
|
|
mlir.register_lowering(custom_vjp_call_jaxpr_p, mlir.lower_fun(
|
|
_custom_vjp_call_jaxpr_impl, multiple_results=True))
|
|
|
|
|
|
def _custom_vjp_call_jaxpr_jvp(
|
|
primals, tangents, *, fun_jaxpr: core.ClosedJaxpr,
|
|
fwd_jaxpr_thunk: Callable[[], Tuple[core.Jaxpr, Sequence[Any]]],
|
|
bwd: lu.WrappedFun, out_trees: Callable, num_consts: int):
|
|
_, args = split_list(primals, [num_consts])
|
|
consts_dot, args_dot = split_list(tangents, [num_consts])
|
|
if any(type(t) is not Zero for t in consts_dot):
|
|
raise ad.CustomVJPException()
|
|
fwd_jaxpr, fwd_consts = fwd_jaxpr_thunk() # consts can be tracers!
|
|
out_tree, res_tree = out_trees()
|
|
args_dot = map(ad.instantiate_zeros, args_dot)
|
|
# Cast float0 to zeros with the primal dtype because custom vjp rules don't
|
|
# currently handle float0s
|
|
args_dot = map(ad.replace_float0s, args, args_dot)
|
|
res_and_primals_out = core.eval_jaxpr(fwd_jaxpr, fwd_consts, *args)
|
|
res, primals_out = split_list(res_and_primals_out, [res_tree.num_leaves])
|
|
avals_out = [raise_to_shaped(core.get_aval(x)) for x in primals_out]
|
|
tangents_out = ad.custom_lin_p.bind(
|
|
*res, *args_dot, num_res=res_tree.num_leaves, bwd=bwd, out_avals=avals_out)
|
|
tangents_out = map(ad.recast_to_float0, primals_out, tangents_out)
|
|
return primals_out, tangents_out
|
|
ad.primitive_jvps[custom_vjp_call_jaxpr_p] = _custom_vjp_call_jaxpr_jvp
|
|
|
|
def _custom_vjp_call_jaxpr_vmap(spmd_axis_name,
|
|
axis_size, axis_name, main_type, args, in_dims, *, fun_jaxpr: core.ClosedJaxpr,
|
|
fwd_jaxpr_thunk: Callable[[], Tuple[core.Jaxpr, Sequence[Any]]],
|
|
bwd: lu.WrappedFun, out_trees: Callable, num_consts: int):
|
|
args = [batching.moveaxis(x, d, 0) if d is not not_mapped and d != 0
|
|
else x for x, d in zip(args, in_dims)]
|
|
|
|
in_batched = [d is not not_mapped for d in in_dims]
|
|
_, args_batched = split_list(in_batched, [num_consts])
|
|
batched_fun_jaxpr, out_batched = batching.batch_jaxpr(
|
|
fun_jaxpr, axis_size, in_batched, False, axis_name, main_type)
|
|
out_dims1 = [0 if b else not_mapped for b in out_batched]
|
|
out_dims2 = []
|
|
|
|
@pe._memoize
|
|
def batched_fwd_jaxpr_thunk():
|
|
fwd_jaxpr = core.ClosedJaxpr(*fwd_jaxpr_thunk()) # consts can be tracers
|
|
batched_fwd_jaxpr, out_batched = batching.batch_jaxpr(
|
|
fwd_jaxpr, axis_size, args_batched, False, axis_name, main_type)
|
|
out_dims2.append([0 if b else not_mapped for b in out_batched])
|
|
return batched_fwd_jaxpr.jaxpr, batched_fwd_jaxpr.consts
|
|
|
|
fwd_args_batched = [0 if b else not_mapped for b in args_batched]
|
|
fwd_out_dims = lambda: out_dims2[0]
|
|
batched_bwd = batching.batch_custom_vjp_bwd(bwd, axis_name, axis_size, fwd_out_dims,
|
|
fwd_args_batched, main_type, spmd_axis_name)
|
|
|
|
batched_outs = custom_vjp_call_jaxpr_p.bind(
|
|
*args, fun_jaxpr=batched_fun_jaxpr,
|
|
fwd_jaxpr_thunk=batched_fwd_jaxpr_thunk, bwd=batched_bwd,
|
|
out_trees=out_trees, num_consts=num_consts)
|
|
out_dims = out_dims2[0] if out_dims2 else out_dims1
|
|
return batched_outs, out_dims
|
|
batching.spmd_axis_primitive_batchers[custom_vjp_call_jaxpr_p] = _custom_vjp_call_jaxpr_vmap
|
|
batching.axis_primitive_batchers[custom_vjp_call_jaxpr_p] = partial(_custom_vjp_call_jaxpr_vmap, None)
|
|
|
|
xla.register_initial_style_primitive(custom_vjp_call_jaxpr_p)
|
|
|
|
batching.primitive_batchers[ad.custom_lin_p] = ad._raise_custom_vjp_error_on_jvp
|
|
mlir.register_lowering(ad.custom_lin_p, ad._raise_custom_vjp_error_on_jvp)
|
|
|
|
|
|
def custom_gradient(fun):
|
|
"""Convenience function for defining custom VJP rules (aka custom gradients).
|
|
|
|
While the canonical way to define custom VJP rules is via ``jax.custom_vjp``,
|
|
the ``custom_gradient`` convenience wrapper follows TensorFlow's
|
|
``tf.custom_gradient`` API. The difference here is that ``custom_gradient``
|
|
can be used as a decorator on one function that returns both the primal value
|
|
(representing the output of the mathematical function to be differentiated)
|
|
and the VJP (gradient) function. See
|
|
https://www.tensorflow.org/api_docs/python/tf/custom_gradient.
|
|
|
|
If the mathematical function to be differentiated has Haskell-like signature
|
|
``a -> b``, then the Python callable ``fun`` should have the signature
|
|
``a -> (b, CT b --o CT a)`` where we use ``CT x`` to denote a cotangent type
|
|
for ``x`` and the ``--o`` arrow to denote a linear function. See the example
|
|
below. That is, ``fun`` should return a pair where the first element
|
|
represents the value of the mathematical function to be differentiated and the
|
|
second element is a function to be called on the backward pass of reverse-mode
|
|
automatic differentiation (i.e. the "custom gradient" function).
|
|
|
|
The function returned as the second element of the output of ``fun`` can close
|
|
over intermediate values computed when evaluating the function to be
|
|
differentiated. That is, use lexical closure to share work between the forward
|
|
pass and the backward pass of reverse-mode automatic differentiation. However,
|
|
it cannot perform Python control flow which depends on the values of the
|
|
closed-over intermediate values or its cotangent arguments; if the function
|
|
includes such control flow, an error is raised.
|
|
|
|
Args:
|
|
fun: a Python callable specifying both the mathematical function to be
|
|
differentiated and its reverse-mode differentiation rule. It should return
|
|
a pair consisting of an output value and a Python callable that represents
|
|
the custom gradient function.
|
|
|
|
Returns:
|
|
A Python callable that accepts the same arguments as ``fun`` and returns the
|
|
output value specified by the first element of ``fun``'s output pair.
|
|
|
|
For example:
|
|
|
|
>>> @jax.custom_gradient
|
|
... def f(x):
|
|
... return x ** 2, lambda g: (g * x,)
|
|
...
|
|
>>> print(f(3.))
|
|
9.0
|
|
>>> print(jax.grad(f)(3.))
|
|
3.0
|
|
|
|
An example with a function on two arguments, so that the VJP function must
|
|
return a tuple of length two:
|
|
|
|
>>> @jax.custom_gradient
|
|
... def f(x, y):
|
|
... return x * y, lambda g: (y, x)
|
|
...
|
|
>>> print(f(3., 4.))
|
|
12.0
|
|
>>> print(jax.grad(f, argnums=(0, 1))(3., 4.))
|
|
(4.0, 3.0)
|
|
"""
|
|
@custom_vjp
|
|
def wrapped_fun(*args, **kwargs):
|
|
ans, _ = fun(*args, **kwargs)
|
|
return ans
|
|
|
|
def fwd(*args, **kwargs):
|
|
ans, rule = fun(*args, **kwargs)
|
|
ans_flat, out_tree = tree_flatten((ans,))
|
|
rule, in_tree = flatten_fun_nokwargs(lu.wrap_init(rule), out_tree)
|
|
ans_avals = [core.get_aval(x).at_least_vspace() for x in ans_flat]
|
|
jaxpr, _, consts = pe.trace_to_jaxpr_dynamic(rule, ans_avals)
|
|
return ans, Residuals(jaxpr, in_tree(), out_tree, consts)
|
|
|
|
def bwd(res, cts):
|
|
jaxpr, in_tree, out_tree, consts = res
|
|
cts_flat, out_tree_ = tree_flatten((cts,))
|
|
if out_tree != out_tree_: raise TypeError(f'{out_tree}\n!=\n{out_tree_}')
|
|
cts_out = core.eval_jaxpr(jaxpr, consts, *cts_flat)
|
|
cts_out = tree_unflatten(in_tree, cts_out)
|
|
if treedef_is_leaf(in_tree):
|
|
cts_out = (cts_out,)
|
|
return cts_out
|
|
|
|
wrapped_fun.defvjp(fwd, bwd)
|
|
return wrapped_fun
|
|
|
|
@register_pytree_node_class
|
|
class Residuals:
|
|
def __init__(self, jaxpr, in_tree, out_tree, consts):
|
|
self.jaxpr = jaxpr
|
|
self.in_tree = in_tree
|
|
self.out_tree = out_tree
|
|
self.consts = consts
|
|
def __iter__(self):
|
|
return iter((self.jaxpr, self.in_tree, self.out_tree, self.consts))
|
|
def tree_flatten(self):
|
|
return self.consts, (self.jaxpr, self.in_tree, self.out_tree)
|
|
@classmethod
|
|
def tree_unflatten(cls, aux, consts):
|
|
jaxpr, in_tree, out_tree = aux
|
|
return cls(jaxpr, in_tree, out_tree, consts)
|
|
|
|
|
|
def closure_convert(fun, *example_args):
|
|
"""Closure conversion utility, for use with higher-order custom derivatives.
|
|
|
|
To define custom derivatives such as with ``jax.custom_vjp(f)``, the target
|
|
function ``f`` must take, as formal arguments, all values involved in
|
|
differentiation. If ``f`` is a higher-order function, in that it accepts as an
|
|
argument a Python function ``g``, then values stored away in ``g``'s closure
|
|
will not be visible to the custom derivative rules, and attempts at AD
|
|
involving these values will fail. One way around this is to convert the
|
|
closure by extracting these values, and to pass them as explicit formal
|
|
arguments across the custom derivative boundary. This utility carries out that
|
|
conversion. More precisely, it closure-converts the function ``fun``
|
|
specialized to the types of the arguments given in ``example_args``.
|
|
|
|
When we refer here to "values in the closure" of ``fun``, we do not mean the
|
|
values that are captured by Python directly when ``fun`` is defined (e.g. the
|
|
Python objects in ``fun.__closure__``, if the attribute exists). Rather, we
|
|
mean values encountered during the execution of ``fun`` on ``example_args``
|
|
that determine its output. This may include, for instance, arrays captured
|
|
transitively in Python closures, i.e. in the Python closure of functions
|
|
called by ``fun``, the closures of the functions that they call, and so forth.
|
|
|
|
The function ``fun`` must be a pure function.
|
|
|
|
Example usage::
|
|
|
|
def minimize(objective_fn, x0):
|
|
converted_fn, aux_args = closure_convert(objective_fn, x0)
|
|
return _minimize(converted_fn, x0, *aux_args)
|
|
|
|
@partial(custom_vjp, nondiff_argnums=(0,))
|
|
def _minimize(objective_fn, x0, *args):
|
|
z = objective_fn(x0, *args)
|
|
# ... find minimizer x_opt ...
|
|
return x_opt
|
|
|
|
def fwd(objective_fn, x0, *args):
|
|
y = _minimize(objective_fn, x0, *args)
|
|
return y, (y, args)
|
|
|
|
def rev(objective_fn, res, g):
|
|
y, args = res
|
|
y_bar = g
|
|
# ... custom reverse-mode AD ...
|
|
return x0_bar, *args_bars
|
|
|
|
_minimize.defvjp(fwd, rev)
|
|
|
|
Args:
|
|
fun: Python callable to be converted. Must be a pure function.
|
|
example_args: Arrays, scalars, or (nested) standard Python
|
|
containers (tuples, lists, dicts, namedtuples, i.e., pytrees)
|
|
thereof, used to determine the types of the formal arguments to
|
|
``fun``. This type-specialized form of ``fun`` is the function
|
|
that will be closure converted.
|
|
|
|
Returns:
|
|
A pair comprising (i) a Python callable, accepting the same
|
|
arguments as ``fun`` followed by arguments corresponding to the
|
|
values hoisted from its closure, and (ii) a list of values hoisted
|
|
from the closure.
|
|
"""
|
|
flat_args, in_tree = tree_flatten(example_args)
|
|
in_avals = tuple(map(abstractify, flat_args))
|
|
if config.jax_check_tracer_leaks:
|
|
return _closure_convert_for_avals.__wrapped__(fun, in_tree, in_avals)
|
|
else:
|
|
return _closure_convert_for_avals(fun, in_tree, in_avals)
|
|
|
|
def _maybe_perturbed(x: Any) -> bool:
|
|
# False if x can't represent an AD-perturbed value (i.e. a value
|
|
# with a nontrivial tangent attached), up to heuristics, and True otherwise.
|
|
# See https://github.com/google/jax/issues/6415 for motivation.
|
|
x = core.full_lower(x)
|
|
if not isinstance(x, core.Tracer):
|
|
# If x is not a Tracer, it can't be perturbed.
|
|
return False
|
|
elif isinstance(x, pe.DynamicJaxprTracer):
|
|
# If x is a DynamicJaxprTracer then we're staging out; differentiation could
|
|
# happen later, but some types always have trivial tangents.
|
|
vspace = x.aval.at_least_vspace()
|
|
return not (vspace is core.abstract_token or
|
|
getattr(vspace, 'dtype', None) == dtypes.float0)
|
|
elif not isinstance(x, ad.JVPTracer):
|
|
# If x is not a JVPTracer, recursively check its contents.
|
|
return any(_maybe_perturbed(attr) for name, attr in x._contents())
|
|
else:
|
|
return True # We can't be sure!
|
|
|
|
@cache()
|
|
def _closure_convert_for_avals(fun, in_tree, in_avals):
|
|
wrapped_fun, out_tree = flatten_fun_nokwargs(lu.wrap_init(fun), in_tree)
|
|
jaxpr, out_pvals, consts = pe.trace_to_jaxpr_dynamic(wrapped_fun, in_avals)
|
|
out_tree = out_tree()
|
|
|
|
(closure_consts, hoisted_consts), merge = partition_list(_maybe_perturbed, consts)
|
|
num_consts = len(hoisted_consts)
|
|
|
|
def converted_fun(*args_hconsts):
|
|
num_args = len(args_hconsts) - num_consts
|
|
args, hoisted_consts = split_list(args_hconsts, [num_args])
|
|
consts = merge(closure_consts, hoisted_consts)
|
|
all_args, in_tree2 = tree_flatten(tuple(args))
|
|
assert in_tree == in_tree2
|
|
out_flat = core.eval_jaxpr(jaxpr, consts, *all_args)
|
|
return tree_unflatten(out_tree, out_flat)
|
|
|
|
return converted_fun, hoisted_consts
|
|
|
|
def partition_list(choice, lst):
|
|
out = [], []
|
|
which = [out[choice(elt)].append(elt) or choice(elt) for elt in lst]
|
|
def merge(l1, l2):
|
|
i1, i2 = iter(l1), iter(l2)
|
|
return [next(i2 if snd else i1) for snd in which]
|
|
return out, merge
|
|
|
|
def abstractify(x):
|
|
return core.raise_to_shaped(core.get_aval(x))
|
|
|
|
|
|
### Custom transposition
|
|
|
|
def linear_call(fun: Callable, fun_transpose: Callable, residual_args,
|
|
linear_args):
|
|
"""Call a linear function, with a custom implementation for its transpose.
|
|
|
|
The `Haskell-like type signatures`_ of ``fun`` and ``fun_transpose`` are:
|
|
|
|
.. code-block:: haskell
|
|
|
|
fun :: r -> a -o b
|
|
fun_transpose :: r -> b -o a
|
|
|
|
where the ``-o`` arrow indicates a linear function, ``r`` is the
|
|
residual input type and ``a`` is the linear input type.
|
|
|
|
The functions ``fun`` and ``fun_transpose`` are coupled as
|
|
transposes of one another. Specifically, the transpose of a
|
|
``linear_call`` primitive is another ``linear_call`` to
|
|
``fun_transpose``, with ``fun`` as its custom transposition.
|
|
|
|
For example:
|
|
|
|
>>> def f(r, x):
|
|
... return x / r
|
|
|
|
>>> def t(r, t):
|
|
... return t / r
|
|
|
|
>>> def div_add(x, denom):
|
|
... return x + linear_call(f, t, denom, x)
|
|
|
|
>>> def transpose(f, x_example):
|
|
... def transposed(y):
|
|
... x, = jax.linear_transpose(f, x_example)(y)
|
|
... return x
|
|
... return transposed
|
|
|
|
>>> div_add(9., 3.)
|
|
DeviceArray(12., dtype=float32, weak_type=True)
|
|
|
|
>>> transpose(partial(div_add, denom=3.), 1.)(18.) # custom
|
|
DeviceArray(24., dtype=float32, weak_type=True)
|
|
|
|
>>> transpose(lambda x: x + x / 3., 1.)(18.) # reference
|
|
DeviceArray(24., dtype=float32, weak_type=True)
|
|
|
|
The above definition of ``f`` illustrates the purpose of a residual
|
|
argument: division is linear in one of its inputs (the dividend
|
|
``x``) but not the other (the divisor ``r``).
|
|
|
|
As another example:
|
|
|
|
>>> def custom_id(x):
|
|
... def f(_, x): return x
|
|
... def t(_, t): return 7.
|
|
... return linear_call(f, t, (), x)
|
|
>>> custom_id(1.)
|
|
1.0
|
|
>>> transpose(custom_id, 1.)(1.)
|
|
7.0
|
|
>>> transpose(transpose(custom_id, 1.), 1.)(1.)
|
|
1.0
|
|
>>> transpose(transpose(transpose(custom_id, 1.), 1.), 1.)(1.)
|
|
7.0
|
|
|
|
Args:
|
|
fun: a Python callable specifying a linear function. It should
|
|
take two arguments: one of "residual" inputs (type ``r``),
|
|
i.e. inputs in which the function is not necessarly linear, and
|
|
one of "linear" inputs (type ``a``). It should return output
|
|
whose components are linear in the linear input (type ``b``).
|
|
fun_transpose: a Python callable specifying a structurally linear
|
|
function that is the transpose of ``fun`` with respect to its
|
|
linear inputs. Its first argument is the same residual inputs
|
|
(``r``) as ``fun``. Its second argument is of type
|
|
``b``. Finally, its output is of type ``a`` and each of its
|
|
component are linear in its second argument (the ``b`` inputs).
|
|
residual_args: Argument in which ``fun`` and ``fun_transpose`` are
|
|
not necessarily linear. Not involved in transposition.
|
|
linear_args: Argument in which ``fun`` and ``fun_transpose`` are
|
|
linear and with respect to which the two are transposes.
|
|
|
|
Returns:
|
|
The call result, i.e. ``fun(residual_args, linear_args)``.
|
|
|
|
.. _Haskell-like type signatures: https://wiki.haskell.org/Type_signature
|
|
"""
|
|
operands_res, res_tree = tree_flatten(residual_args)
|
|
operands_lin, lin_tree = tree_flatten(linear_args)
|
|
|
|
f_in_tree = treedef_tuple((res_tree, lin_tree))
|
|
f, out_tree = flatten_fun_nokwargs(lu.wrap_init(fun), f_in_tree)
|
|
|
|
res_avals = map(abstractify, operands_res)
|
|
lin_avals = map(abstractify, operands_lin)
|
|
f_jaxpr, f_consts = _initial_style_jaxpr(f, (*res_avals, *lin_avals))
|
|
f_jaxpr = _close_jaxpr(f_jaxpr)
|
|
out_avals = map(core.raise_to_shaped, f_jaxpr.out_avals)
|
|
|
|
t_in_tree = treedef_tuple((res_tree, out_tree()))
|
|
t, t_out_tree = flatten_fun_nokwargs(lu.wrap_init(fun_transpose), t_in_tree)
|
|
|
|
t_jaxpr, t_consts = _initial_style_jaxpr(t, (*res_avals, *out_avals))
|
|
t_jaxpr = _close_jaxpr(t_jaxpr)
|
|
|
|
if t_out_tree() != lin_tree:
|
|
raise TypeError(
|
|
'transpose output pytree structure must match that of linear inputs, '
|
|
f'got output structure {t_out_tree()} '
|
|
f'and input structure {lin_tree}.')
|
|
|
|
out = linear_call_p.bind(*f_consts, *t_consts, *operands_res, *operands_lin,
|
|
callee=f_jaxpr,
|
|
transpose=t_jaxpr,
|
|
num_callee_consts=len(f_consts),
|
|
num_transpose_consts=len(t_consts),
|
|
num_res=len(operands_res))
|
|
|
|
return tree_unflatten(out_tree(), out)
|
|
|
|
def _linear_call_impl(*args, callee, transpose, num_callee_consts,
|
|
num_transpose_consts, num_res):
|
|
del transpose
|
|
consts, _, operands_res, operands_lin = split_list(
|
|
args, [num_callee_consts, num_transpose_consts, num_res])
|
|
return core.eval_jaxpr(callee.jaxpr, (), *consts, *operands_res, *operands_lin)
|
|
|
|
def _linear_call_transpose_rule(cts, *args, callee, transpose,
|
|
num_callee_consts,
|
|
num_transpose_consts, num_res):
|
|
f_consts, t_consts, operands_res, operands_lin = split_list(
|
|
args, [num_callee_consts, num_transpose_consts, num_res])
|
|
_, _, cts_avals = split_list(
|
|
transpose.in_avals, [num_transpose_consts, num_res])
|
|
|
|
assert all(ad.is_undefined_primal(x) for x in operands_lin)
|
|
assert all(not ad.is_undefined_primal(x) for x in operands_res)
|
|
|
|
cts = [zeros_like_aval(a) if type(ct) is Zero else ct
|
|
for ct, a in zip(cts, cts_avals)]
|
|
|
|
cts_out = linear_call_p.bind(*t_consts, *f_consts, *operands_res, *cts,
|
|
callee=transpose,
|
|
transpose=callee,
|
|
num_callee_consts=len(t_consts),
|
|
num_transpose_consts=len(f_consts),
|
|
num_res=len(operands_res))
|
|
|
|
return [None] * (num_callee_consts + num_transpose_consts + num_res) + cts_out
|
|
|
|
def _linear_call_abstract_eval(*args, **kwargs):
|
|
return map(core.raise_to_shaped, kwargs['callee'].out_avals)
|
|
|
|
linear_call_p = core.Primitive('linear_call')
|
|
linear_call_p.multiple_results = True
|
|
linear_call_p.def_impl(_linear_call_impl)
|
|
linear_call_p.def_abstract_eval(_linear_call_abstract_eval)
|
|
ad.primitive_transposes[linear_call_p] = _linear_call_transpose_rule
|
|
xla.register_initial_style_primitive(linear_call_p)
|
|
mlir.register_lowering(linear_call_p, mlir.lower_fun(
|
|
_linear_call_impl, multiple_results=True))
|
|
|
|
|
|
# A stageable primitive that fails when evaluated
|
|
unreachable_p: core.Primitive = core.Primitive('unreachable')
|
|
unreachable_p.multiple_results = True
|
|
|
|
def unreachable_impl(*_, out_avals, exc_type, message):
|
|
del out_avals
|
|
raise exc_type(message)
|
|
|
|
# Evaluation raises an exception
|
|
unreachable_p.def_impl(unreachable_impl)
|
|
|
|
# Translation raises an exception
|
|
# TODO(frostig,mattjj): We have no good way to translate a function
|
|
# that errs. Since MHLO lowering over-approximates concrete evaluation,
|
|
# we err on MHLO lowering for the time being.
|
|
mlir.register_lowering(unreachable_p, unreachable_impl)
|
|
|
|
# Abstract evaluation proceeds without issue, to allow for staging
|
|
unreachable_p.def_abstract_eval(lambda *_, out_avals, **__: out_avals)
|
|
|
|
def unreachable(*args, out_avals=None, exc_type=TypeError,
|
|
message='unreachable'):
|
|
"""Fail when evaluated concretely (but allow for staging).
|
|
|
|
This function allows one to assert an impossibility of
|
|
evaluation. It can be used to guarantee that evaluation does not
|
|
"reach" a certain point in the sense that it does not execute, but
|
|
it can nonetheless be staged out by JAX without error.
|
|
|
|
Args:
|
|
*args: The arbitrary pytree of arguments to the function.
|
|
out_avals: Optional specification of the output types of this
|
|
function invocation from the point of view of staging. If
|
|
``None``, these are chosen as equal to types of input arguments.
|
|
exc_type: Optional constructor for the Python exception raised if
|
|
evaluated.
|
|
message: Optional string message for the Python exception raised
|
|
if evaluated.
|
|
|
|
"""
|
|
if out_avals is None:
|
|
out_avals = tree_map(core.get_aval, args)
|
|
|
|
args_flat, in_tree = tree_flatten(args)
|
|
out_avals_flat, out_tree = tree_flatten(out_avals)
|
|
out = unreachable_p.bind(*args_flat, out_avals=out_avals_flat,
|
|
exc_type=exc_type, message=message)
|
|
return tree_unflatten(out_tree, out)
|
|
|
|
|
|
disallow_jvp = partial(
|
|
unreachable,
|
|
exc_type=TypeError,
|
|
message="can't apply forward-mode autodiff (jvp) to a custom_vjp function.")
|
|
|
|
|
|
def custom_vjp_by_custom_transpose(fun, fwd, bwd):
|
|
fun = custom_jvp(fun)
|
|
|
|
@fun.defjvp
|
|
def jvp(primals, tangents):
|
|
outs, residuals = fwd(*primals)
|
|
tan_out_types = tree_map(lambda o: core.get_aval(o).at_least_vspace(), outs)
|
|
tan_fn = custom_transpose(partial(disallow_jvp, out_avals=tan_out_types))
|
|
tan_fn.def_transpose(bwd)
|
|
return outs, tan_fn(tan_out_types, residuals, tangents)
|
|
|
|
return fun
|
|
|
|
|
|
# TODO(mattjj): remove these stubs, which exist to avoid breaking internal users
|
|
custom_jvp_call_jaxpr_p = core.Primitive("custom_jvp_call_jaxpr")
|