mirror of
https://github.com/ROCm/jax.git
synced 2025-04-14 10:56:06 +00:00
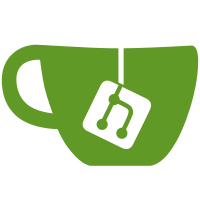
See https://opensource.google/documentation/reference/releasing/contributions#copyright for more details. PiperOrigin-RevId: 476167538
469 lines
18 KiB
Python
469 lines
18 KiB
Python
# Copyright 2022 The JAX Authors.
|
|
#
|
|
# Licensed under the Apache License, Version 2.0 (the "License");
|
|
# you may not use this file except in compliance with the License.
|
|
# You may obtain a copy of the License at
|
|
#
|
|
# https://www.apache.org/licenses/LICENSE-2.0
|
|
#
|
|
# Unless required by applicable law or agreed to in writing, software
|
|
# distributed under the License is distributed on an "AS IS" BASIS,
|
|
# WITHOUT WARRANTIES OR CONDITIONS OF ANY KIND, either express or implied.
|
|
# See the License for the specific language governing permissions and
|
|
# limitations under the License.
|
|
"""Module for the custom linear solve and utilities."""
|
|
import collections
|
|
from functools import partial
|
|
import operator
|
|
|
|
import jax
|
|
from jax import core
|
|
from jax import lax
|
|
from jax import linear_util as lu
|
|
from jax.core import raise_to_shaped
|
|
from jax.interpreters import ad
|
|
from jax.interpreters import batching
|
|
from jax.interpreters import mlir
|
|
from jax.interpreters import partial_eval as pe
|
|
from jax.interpreters import xla
|
|
from jax.tree_util import (tree_flatten, treedef_children, tree_leaves,
|
|
tree_unflatten, treedef_tuple)
|
|
from jax._src import ad_util
|
|
from jax._src.traceback_util import api_boundary
|
|
from jax._src.util import split_list, safe_map
|
|
import numpy as np
|
|
|
|
from jax._src.lax.control_flow.common import (
|
|
_abstractify,
|
|
_check_tree,
|
|
_initial_style_jaxpr,
|
|
)
|
|
|
|
_map = safe_map
|
|
|
|
_RootTuple = collections.namedtuple('_RootTuple', 'f, solve, l_and_s')
|
|
|
|
|
|
def _split_root_args(args, const_lengths):
|
|
params_list = split_list(args, list(const_lengths))
|
|
return _RootTuple(*params_list[:-1]), params_list[-1]
|
|
|
|
|
|
@api_boundary
|
|
def custom_root(f, initial_guess, solve, tangent_solve, has_aux=False):
|
|
"""Differentiably solve for a roots of a function.
|
|
|
|
This is a low-level routine, mostly intended for internal use in JAX.
|
|
Gradients of custom_root() are defined with respect to closed-over variables
|
|
from the provided function ``f`` via the implicit function theorem:
|
|
https://en.wikipedia.org/wiki/Implicit_function_theorem
|
|
|
|
Args:
|
|
f: function for which to find a root. Should accept a single argument,
|
|
return a tree of arrays with the same structure as its input.
|
|
initial_guess: initial guess for a zero of f.
|
|
solve: function to solve for the roots of f. Should take two positional
|
|
arguments, f and initial_guess, and return a solution with the same
|
|
structure as initial_guess such that func(solution) = 0. In other words,
|
|
the following is assumed to be true (but not checked)::
|
|
|
|
solution = solve(f, initial_guess)
|
|
error = f(solution)
|
|
assert all(error == 0)
|
|
|
|
tangent_solve: function to solve the tangent system. Should take two
|
|
positional arguments, a linear function ``g`` (the function ``f``
|
|
linearized at its root) and a tree of array(s) ``y`` with the same
|
|
structure as initial_guess, and return a solution ``x`` such that
|
|
``g(x)=y``:
|
|
|
|
- For scalar ``y``, use ``lambda g, y: y / g(1.0)``.
|
|
- For vector ``y``, you could use a linear solve with the Jacobian, if
|
|
dimensionality of ``y`` is not too large:
|
|
``lambda g, y: np.linalg.solve(jacobian(g)(y), y)``.
|
|
has_aux: bool indicating whether the ``solve`` function returns
|
|
auxiliary data like solver diagnostics as a second argument.
|
|
|
|
Returns:
|
|
The result of calling solve(f, initial_guess) with gradients defined via
|
|
implicit differentiation assuming ``f(solve(f, initial_guess)) == 0``.
|
|
"""
|
|
guess_flat, in_args_tree = tree_flatten((initial_guess,))
|
|
guess_avals = tuple(_map(_abstractify, guess_flat))
|
|
f_jaxpr, f_consts, out_tree = _initial_style_jaxpr(
|
|
f, in_args_tree, guess_avals)
|
|
|
|
in_tree, = treedef_children(in_args_tree)
|
|
_check_tree("f", "initial_guess", out_tree, in_tree, False)
|
|
|
|
solve_jaxpr, solve_consts, solution_tree = _initial_style_jaxpr(
|
|
partial(solve, f), in_args_tree, guess_avals)
|
|
_check_tree("solve", "initial_guess", solution_tree, in_tree, has_aux)
|
|
|
|
def linearize_and_solve(x, b):
|
|
unchecked_zeros, f_jvp = jax.linearize(f, x)
|
|
return tangent_solve(f_jvp, b)
|
|
|
|
l_and_s_jaxpr, l_and_s_consts, out_tree = _initial_style_jaxpr(
|
|
linearize_and_solve, treedef_tuple((in_tree,) * 2), guess_avals * 2)
|
|
_check_tree("tangent_solve", "x", out_tree, in_tree, False)
|
|
|
|
all_consts = [f_consts, solve_consts, l_and_s_consts]
|
|
const_lengths = _RootTuple(*_map(len, all_consts))
|
|
jaxprs = _RootTuple(f_jaxpr, solve_jaxpr, l_and_s_jaxpr)
|
|
|
|
solution_flat = _custom_root(
|
|
const_lengths, jaxprs, *(_flatten(all_consts) + guess_flat))
|
|
return tree_unflatten(solution_tree, solution_flat)
|
|
|
|
|
|
@partial(jax.custom_jvp, nondiff_argnums=(0, 1))
|
|
def _custom_root(const_lengths, jaxprs, *args):
|
|
params, initial_guess = _split_root_args(args, const_lengths)
|
|
solution = core.jaxpr_as_fun(jaxprs.solve)(*(params.solve + initial_guess))
|
|
return solution
|
|
|
|
|
|
@_custom_root.defjvp
|
|
def _root_jvp(const_lengths, jaxprs, primals, tangents):
|
|
params, _ = _split_root_args(primals, const_lengths)
|
|
sol = _custom_root(const_lengths, jaxprs, *primals)
|
|
|
|
f_out_vals = len(jaxprs.f.out_avals)
|
|
solution, aux = split_list(sol, [f_out_vals])
|
|
|
|
params_dot, _ = _split_root_args(tangents, const_lengths)
|
|
|
|
# F(m, u) = 0 # system of equations in u, parameterized by m
|
|
# # solution is u*(m) defined in a neighborhood
|
|
# F(m, u*(m)) = 0 # satisfied in a neighborhood
|
|
#
|
|
# ∂_0 F(m, u*(m)) + ∂_1 F(m, u*(m)) ∂ u*(m) = 0 # implied by line above
|
|
# ∂ u*(m) = - (∂_1 F(m, u*(m)))^{-1} ∂_0 F(m, u*(m)) # rearrange
|
|
#
|
|
# ∂ u*(m)[v] = - (∂_1 F(m, u*(m)))^{-1} [∂_0 F(m, u*(m))[v]] # jvp
|
|
|
|
f = core.jaxpr_as_fun(jaxprs.f)
|
|
linearize_and_solve = partial(
|
|
core.jaxpr_as_fun(jaxprs.l_and_s), *params.l_and_s)
|
|
f_at_solution = lambda *params: f(*params, *solution)
|
|
_, rhs = ad.jvp(lu.wrap_init(f_at_solution)).call_wrapped(
|
|
params.f, params_dot.f)
|
|
solution_dot = _map(
|
|
operator.neg, linearize_and_solve(*solution, *rhs))
|
|
# append aux, create symbolic zero tangents for the aux values
|
|
solution += aux
|
|
solution_dot += _map(lax.zeros_like_array, aux)
|
|
|
|
return solution, solution_dot
|
|
|
|
|
|
class _LinearSolveTuple(collections.namedtuple(
|
|
'_LinearSolveTuple', 'matvec, vecmat, solve, transpose_solve')):
|
|
|
|
def transpose(self):
|
|
return type(self)(self.vecmat, self.matvec, self.transpose_solve, self.solve)
|
|
|
|
|
|
def _split_linear_solve_args(args, const_lengths):
|
|
params_list = split_list(args, list(const_lengths))
|
|
return _LinearSolveTuple(*params_list[:-1]), params_list[-1]
|
|
|
|
|
|
def _transpose_one_output(linear_fun, primals):
|
|
transpose_fun = jax.linear_transpose(linear_fun, primals)
|
|
def transposed_fun(x):
|
|
(y,) = transpose_fun(x)
|
|
return y
|
|
return transposed_fun
|
|
|
|
|
|
def _flatten(args):
|
|
return [x for arg in args for x in arg]
|
|
|
|
|
|
def _check_shapes(func_name, expected_name, actual, expected):
|
|
actual_shapes = _map(np.shape, tree_leaves(actual))
|
|
expected_shapes = _map(np.shape, tree_leaves(expected))
|
|
if actual_shapes != expected_shapes:
|
|
raise ValueError(
|
|
f"{func_name}() output shapes must match {expected_name}, "
|
|
f"got {actual_shapes} and {expected_shapes}")
|
|
|
|
|
|
@api_boundary
|
|
def custom_linear_solve(
|
|
matvec, b, solve, transpose_solve=None, symmetric=False, has_aux=False):
|
|
"""Perform a matrix-free linear solve with implicitly defined gradients.
|
|
|
|
This function allows for overriding or defining gradients for a linear
|
|
solve directly via implicit differentiation at the solution, rather than by
|
|
differentiating *through* the solve operation. This can sometimes be much faster
|
|
or more numerically stable, or differentiating through the solve operation
|
|
may not even be implemented (e.g., if ``solve`` uses ``lax.while_loop``).
|
|
|
|
Required invariant::
|
|
|
|
x = solve(matvec, b) # solve the linear equation
|
|
assert matvec(x) == b # not checked
|
|
|
|
Args:
|
|
matvec: linear function to invert. Must be differentiable.
|
|
b: constant right handle side of the equation. May be any nested structure
|
|
of arrays.
|
|
solve: higher level function that solves for solution to the linear
|
|
equation, i.e., ``solve(matvec, x) == x`` for all ``x`` of the same form
|
|
as ``b``. This function need not be differentiable.
|
|
transpose_solve: higher level function for solving the transpose linear
|
|
equation, i.e., ``transpose_solve(vecmat, x) == x``, where ``vecmat`` is
|
|
the transpose of the linear map ``matvec`` (computed automatically with
|
|
autodiff). Required for backwards mode automatic differentiation, unless
|
|
``symmetric=True``, in which case ``solve`` provides the default value.
|
|
symmetric: bool indicating if it is safe to assume the linear map
|
|
corresponds to a symmetric matrix, i.e., ``matvec == vecmat``.
|
|
has_aux: bool indicating whether the ``solve`` and ``transpose_solve`` functions
|
|
return auxiliary data like solver diagnostics as a second argument.
|
|
|
|
Returns:
|
|
Result of ``solve(matvec, b)``, with gradients defined assuming that the
|
|
solution ``x`` satisfies the linear equation ``matvec(x) == b``.
|
|
"""
|
|
if transpose_solve is None and symmetric:
|
|
transpose_solve = solve
|
|
|
|
b_flat, in_args_tree = tree_flatten((b,))
|
|
b_avals = tuple(_map(_abstractify, b_flat))
|
|
|
|
tree, = treedef_children(in_args_tree)
|
|
|
|
def _shape_checked(fun, name, has_aux):
|
|
def f(x):
|
|
y = fun(x)
|
|
_check_shapes(name, "b", y, b_flat)
|
|
return y
|
|
|
|
def f_aux(x):
|
|
y, aux = fun(x)
|
|
_check_shapes(name, "b", y, b_flat)
|
|
return y, aux
|
|
|
|
return f_aux if has_aux else f
|
|
|
|
# no auxiliary data assumed for matvec
|
|
matvec_jaxpr, matvec_consts, out_tree = _initial_style_jaxpr(
|
|
_shape_checked(matvec, "matvec", False), in_args_tree, b_avals,
|
|
'custom_linear_solve')
|
|
_check_tree("matvec", "b", out_tree, tree, False)
|
|
|
|
solve_jaxpr, solve_consts, out_tree = _initial_style_jaxpr(
|
|
_shape_checked(partial(solve, matvec), "solve", has_aux), in_args_tree, b_avals,
|
|
'custom_linear_solve')
|
|
_check_tree("solve", "b", out_tree, tree, has_aux)
|
|
|
|
if transpose_solve is None:
|
|
vecmat_jaxpr = tr_solve_jaxpr = None
|
|
vecmat_consts = tr_solve_consts = []
|
|
else:
|
|
if symmetric:
|
|
vecmat = matvec
|
|
vecmat_jaxpr = matvec_jaxpr
|
|
vecmat_consts = matvec_consts
|
|
else:
|
|
vecmat = _transpose_one_output(matvec, b)
|
|
vecmat_jaxpr, vecmat_consts, out_tree = _initial_style_jaxpr(
|
|
vecmat, in_args_tree, b_avals, 'custom_linear_solve')
|
|
assert out_tree == tree
|
|
|
|
tr_solve_jaxpr, tr_solve_consts, out_tree = _initial_style_jaxpr(
|
|
_shape_checked(partial(transpose_solve, vecmat), "transpose_solve", has_aux),
|
|
in_args_tree, b_avals, 'custom_linear_solve')
|
|
_check_tree("transpose_solve", "b", out_tree, tree, has_aux)
|
|
|
|
all_consts = [matvec_consts, vecmat_consts, solve_consts, tr_solve_consts]
|
|
const_lengths = _LinearSolveTuple(*_map(len, all_consts))
|
|
jaxprs = _LinearSolveTuple(
|
|
matvec_jaxpr, vecmat_jaxpr, solve_jaxpr, tr_solve_jaxpr)
|
|
|
|
out_flat = linear_solve_p.bind(
|
|
*(_flatten(all_consts) + b_flat),
|
|
const_lengths=const_lengths, jaxprs=jaxprs)
|
|
|
|
return tree_unflatten(out_tree, out_flat)
|
|
|
|
|
|
def _linear_solve_abstract_eval(*args, const_lengths, jaxprs):
|
|
args_to_raise = args[sum(const_lengths):]
|
|
|
|
# raise aux_args to shaped arrays as well if present
|
|
# number of aux args is the difference in out_avals
|
|
# of solve and matvec (since they map to the same vector space)
|
|
|
|
num_aux = len(jaxprs.solve.out_avals) - len(jaxprs.matvec.out_avals)
|
|
if num_aux > 0:
|
|
args_to_raise += tuple(jaxprs.solve.out_avals[-num_aux:])
|
|
return _map(raise_to_shaped, args_to_raise)
|
|
|
|
|
|
def _custom_linear_solve_impl(*args, const_lengths, jaxprs):
|
|
params, b = _split_linear_solve_args(args, const_lengths)
|
|
x = core.jaxpr_as_fun(jaxprs.solve)(*(params.solve + b))
|
|
return x
|
|
|
|
|
|
def _tangent_linear_map(func, params, params_dot, *x):
|
|
"""Compute the tangent of a linear map.
|
|
|
|
Assuming ``func(*params, *x)`` is linear in ``x`` and computes ``A @ x``,
|
|
this function computes ``∂A @ x``.
|
|
"""
|
|
assert any(type(p) is not ad_util.Zero for p in params_dot)
|
|
zeros = _map(ad_util.Zero.from_value, x)
|
|
_, out_tangent = ad.jvp(lu.wrap_init(func)).call_wrapped(
|
|
params + list(x), params_dot + zeros)
|
|
return out_tangent
|
|
|
|
|
|
def _custom_linear_solve_jvp(primals, tangents, const_lengths, jaxprs):
|
|
# A x - b = 0
|
|
# ∂A x + A ∂x - ∂b = 0
|
|
# ∂x = A^{-1} (∂b - ∂A x)
|
|
|
|
kwargs = dict(const_lengths=const_lengths, jaxprs=jaxprs)
|
|
x = linear_solve_p.bind(*primals, **kwargs)
|
|
|
|
params, _ = _split_linear_solve_args(primals, const_lengths)
|
|
params_dot, b_dot = _split_linear_solve_args(tangents, const_lengths)
|
|
|
|
num_x_leaves = len(b_dot)
|
|
# x is a flat tree with possible aux values appended
|
|
# since x_tree == b_tree == b_dot_tree, we can cut off
|
|
# aux values with len info provided by b_dot tree here
|
|
x_leaves, _ = split_list(x, [num_x_leaves])
|
|
|
|
if all(type(p) is ad_util.Zero for p in params_dot.matvec):
|
|
# no need to evaluate matvec_tangents
|
|
rhs = b_dot
|
|
else:
|
|
matvec_tangents = _tangent_linear_map(
|
|
core.jaxpr_as_fun(jaxprs.matvec), params.matvec, params_dot.matvec, *x_leaves)
|
|
rhs = _map(ad.add_tangents, b_dot, _map(operator.neg, matvec_tangents))
|
|
|
|
x_dot = linear_solve_p.bind(*(_flatten(params) + rhs), **kwargs)
|
|
|
|
# split into x tangents and aux tangents (these become zero)
|
|
dx_leaves, daux_leaves = split_list(x_dot, [num_x_leaves])
|
|
|
|
daux_leaves = _map(ad_util.Zero.from_value, daux_leaves)
|
|
|
|
x_dot = dx_leaves + daux_leaves
|
|
|
|
return x, x_dot
|
|
|
|
|
|
def _linear_solve_transpose_rule(cotangent, *primals, const_lengths, jaxprs):
|
|
if jaxprs.transpose_solve is None:
|
|
raise TypeError('transpose_solve required for backwards mode automatic '
|
|
'differentiation of custom_linear_solve')
|
|
|
|
params, b = _split_linear_solve_args(primals, const_lengths)
|
|
# split off symbolic zeros in the cotangent if present
|
|
x_cotangent, _ = split_list(cotangent, [len(b)])
|
|
assert all(ad.is_undefined_primal(x) for x in b)
|
|
cotangent_b_full = linear_solve_p.bind(
|
|
*(_flatten(params.transpose()) + x_cotangent),
|
|
const_lengths=const_lengths.transpose(), jaxprs=jaxprs.transpose())
|
|
# drop aux values in cotangent computation
|
|
cotangent_b, _ = split_list(cotangent_b_full, [len(b)])
|
|
return [None] * sum(const_lengths) + cotangent_b
|
|
|
|
|
|
def _linear_solve_batching_rule(axis_size, axis_name, main_type, args, dims,
|
|
const_lengths, jaxprs):
|
|
orig_bat = [d is not batching.not_mapped for d in dims]
|
|
|
|
params, b = _split_linear_solve_args(args, const_lengths)
|
|
params_dims, b_dims = _split_linear_solve_args(dims, const_lengths)
|
|
params_bat, orig_b_bat = _split_linear_solve_args(orig_bat, const_lengths)
|
|
|
|
(matvec, vecmat, solve, solve_t) = jaxprs
|
|
(matvec_bat, vecmat_bat, solve_bat, solve_t_bat) = params_bat
|
|
|
|
num_aux = len(solve.out_avals) - len(matvec.out_avals)
|
|
# Fixpoint computation of which parts of x and b are batched; we need to
|
|
# ensure this is consistent between all four jaxprs
|
|
b_bat = orig_b_bat
|
|
x_bat = [False] * len(solve.out_avals)
|
|
for i in range(1 + len(orig_b_bat) + len(solve.out_avals)):
|
|
# Apply vecmat and solve -> new batched parts of x
|
|
solve_jaxpr_batched, solve_x_bat = batching.batch_jaxpr(
|
|
solve, axis_size, solve_bat + b_bat, instantiate=x_bat,
|
|
axis_name=axis_name, main_type=main_type)
|
|
if vecmat is None:
|
|
vecmat_jaxpr_batched = None
|
|
x_bat_out = solve_x_bat
|
|
else:
|
|
vecmat_jaxpr_batched, vecmat_x_bat = batching.batch_jaxpr(
|
|
vecmat, axis_size, vecmat_bat + b_bat, instantiate=x_bat,
|
|
axis_name=axis_name, main_type=main_type)
|
|
# batch all aux data by default
|
|
x_bat_out = _map(operator.or_, vecmat_x_bat + [True] * num_aux, solve_x_bat)
|
|
|
|
# Apply matvec and solve_t -> new batched parts of b
|
|
matvec_jaxpr_batched, matvec_b_bat = batching.batch_jaxpr(
|
|
matvec, axis_size, matvec_bat + x_bat_out, instantiate=b_bat,
|
|
axis_name=axis_name, main_type=main_type)
|
|
if solve_t is None:
|
|
solve_t_jaxpr_batched = None
|
|
b_bat_out = _map(operator.or_, matvec_b_bat, orig_b_bat)
|
|
else:
|
|
solve_t_jaxpr_batched, solve_t_b_aux_bat = batching.batch_jaxpr(
|
|
solve_t, axis_size, solve_t_bat + x_bat_out, instantiate=b_bat,
|
|
axis_name=axis_name, main_type=main_type)
|
|
assert len(solve_t_b_aux_bat) == len(orig_b_bat) + num_aux
|
|
solve_t_b_bat, _ = split_list(solve_t_b_aux_bat, [len(orig_b_bat)])
|
|
b_bat_out = _map(lambda m, s, o: m or s or o, matvec_b_bat, solve_t_b_bat,
|
|
orig_b_bat)
|
|
if x_bat_out == x_bat and b_bat_out == b_bat:
|
|
break
|
|
else:
|
|
x_bat = x_bat_out
|
|
b_bat = b_bat_out
|
|
else:
|
|
assert False, "Fixedpoint not reached"
|
|
|
|
batched_jaxprs = _LinearSolveTuple(matvec_jaxpr_batched, vecmat_jaxpr_batched,
|
|
solve_jaxpr_batched, solve_t_jaxpr_batched)
|
|
|
|
# Move batched axes to the front
|
|
new_params = [
|
|
batching.moveaxis(x, d, 0)
|
|
if d is not batching.not_mapped and d != 0 else x
|
|
for x, d in zip(_flatten(params), _flatten(params_dims))
|
|
]
|
|
# Broadcast out b if necessary
|
|
new_b = [
|
|
batching.broadcast(x, axis_size, 0) if now_bat and not was_bat else
|
|
batching.moveaxis(x, d, 0) if now_bat and d != 0 else x
|
|
for x, d, was_bat, now_bat in zip(b, b_dims, orig_b_bat, b_bat)
|
|
]
|
|
|
|
outs = linear_solve_p.bind(
|
|
*(new_params + new_b),
|
|
const_lengths=const_lengths,
|
|
jaxprs=batched_jaxprs)
|
|
out_dims = [0 if batched else batching.not_mapped for batched in solve_x_bat]
|
|
return outs, out_dims
|
|
|
|
|
|
linear_solve_p = core.AxisPrimitive('custom_linear_solve')
|
|
linear_solve_p.multiple_results = True
|
|
linear_solve_p.def_impl(_custom_linear_solve_impl)
|
|
linear_solve_p.def_abstract_eval(_linear_solve_abstract_eval)
|
|
ad.primitive_jvps[linear_solve_p] = _custom_linear_solve_jvp
|
|
xla.register_initial_style_primitive(linear_solve_p)
|
|
mlir.register_lowering(
|
|
linear_solve_p, mlir.lower_fun(_custom_linear_solve_impl,
|
|
multiple_results=True))
|
|
ad.primitive_transposes[linear_solve_p] = _linear_solve_transpose_rule
|
|
batching.axis_primitive_batchers[linear_solve_p] = _linear_solve_batching_rule
|