mirror of
https://github.com/ROCm/jax.git
synced 2025-04-14 10:56:06 +00:00
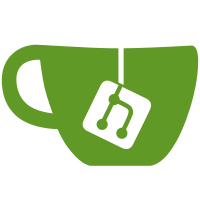
See https://opensource.google/documentation/reference/releasing/contributions#copyright for more details. PiperOrigin-RevId: 476167538
427 lines
17 KiB
Python
427 lines
17 KiB
Python
# Copyright 2021 The JAX Authors.
|
|
#
|
|
# Licensed under the Apache License, Version 2.0 (the "License");
|
|
# you may not use this file except in compliance with the License.
|
|
# You may obtain a copy of the License at
|
|
#
|
|
# https://www.apache.org/licenses/LICENSE-2.0
|
|
#
|
|
# Unless required by applicable law or agreed to in writing, software
|
|
# distributed under the License is distributed on an "AS IS" BASIS,
|
|
# WITHOUT WARRANTIES OR CONDITIONS OF ANY KIND, either express or implied.
|
|
# See the License for the specific language governing permissions and
|
|
# limitations under the License
|
|
|
|
"""Serial algorithm for eigh."""
|
|
|
|
from __future__ import annotations
|
|
|
|
from functools import partial
|
|
from typing import NamedTuple, Tuple
|
|
|
|
import jax
|
|
import jax._src.numpy.lax_numpy as jnp
|
|
import jax._src.numpy.linalg as jnp_linalg
|
|
from jax import lax
|
|
from jax._src.lax import qdwh
|
|
from jax._src.lax import linalg as lax_linalg
|
|
from jax._src.lax.stack import Stack
|
|
|
|
|
|
# QDWH-eigh is a recursive algorithm where the structure of the recursion
|
|
# is determined by the eigenspectrum. Neither JAX nor XLA can handle this kind
|
|
# of recursion, so we instead express the recursion as iteration using an
|
|
# explicit stack.
|
|
|
|
|
|
# TODO(phawkins): consider extracting _mask/_slice/_update_slice into a
|
|
# separate module.
|
|
|
|
def _mask(x, dims, alternative=0):
|
|
"""Masks `x` up to the dynamic shape `dims`.
|
|
|
|
Replaces values outside those dimensions with `alternative`. `alternative` is
|
|
broadcast with `x`.
|
|
"""
|
|
assert jnp.ndim(x) == len(dims)
|
|
mask = None
|
|
for i, d in enumerate(dims):
|
|
if d is not None:
|
|
mask_dim_i = lax.broadcasted_iota(jnp.int32, x.shape, i) < d
|
|
mask = mask_dim_i if mask is None else (mask & mask_dim_i)
|
|
return x if mask is None else jnp.where(mask, x, alternative)
|
|
|
|
def _slice(operand, start_indices, dynamic_slice_sizes, static_slice_sizes,
|
|
fill_value=0):
|
|
"""Similar to lax.dynamic_slice, but handles arrays with dynamic sizes.
|
|
|
|
Returns fill_value instead of clamping start_indices for those elements that
|
|
would overflow the side of the array.
|
|
|
|
Args:
|
|
operand: the array to slice
|
|
start_indices: the offset of the start of the slice
|
|
dynamic_slice_sizes: the true (unpadded) size of the slice
|
|
static_slice_sizes: the padded size of the slice, which must be known at
|
|
compile time. The static size must be larger than the dynamic size.
|
|
fill_value: value with which to replace masked-out elements.
|
|
Returns:
|
|
An array with static shape `static_slice_sizes`, padded from its true
|
|
(dynamic) size `dynamic_slice_sizes`.
|
|
"""
|
|
# We must pad the input array so the dynamic_slice is guaranteed to fall
|
|
# entirely in bounds.
|
|
padded = lax.pad(operand,
|
|
jnp.array(0, operand.dtype),
|
|
[(0, d, 0) for d in static_slice_sizes])
|
|
out = lax.dynamic_slice(padded, tuple(jnp.int32(i) for i in start_indices),
|
|
static_slice_sizes)
|
|
return _mask(out, dynamic_slice_sizes, fill_value)
|
|
|
|
def _update_slice(operand, update, start_indices, update_dims):
|
|
"""
|
|
Similar to lax.dynamic_update_slice, but handles padded updates where padding
|
|
values should not overwrite existing values in the array.
|
|
|
|
Args:
|
|
operand: the array to update
|
|
update: the padded array to write
|
|
start_indices: the offset at which to write `update`.
|
|
update_dims: the true dimensions of the padded update `update`. Only values
|
|
inside the rectangle given by `update_dims` will be overwritten."""
|
|
operand_shape = operand.shape
|
|
operand = lax.pad(operand,
|
|
jnp.array(0, operand.dtype),
|
|
[(0, d, 0) for d in update.shape])
|
|
start_indices = tuple(jnp.int32(i) for i in start_indices)
|
|
t = lax.dynamic_slice(operand, start_indices, update.shape)
|
|
t = _mask(update, update_dims, t)
|
|
operand = lax.dynamic_update_slice(operand, t, start_indices)
|
|
return lax.slice(operand, [0] * operand.ndim, operand_shape)
|
|
|
|
|
|
def _projector_subspace(P, H, n, rank, maxiter=2):
|
|
""" Decomposes the `n x n` rank `rank` Hermitian projector `P` into
|
|
an `n x rank` isometry `V_minus` such that `P = V_minus @ V_minus.conj().T`
|
|
and an `n x (n - rank)` isometry `V_minus` such that
|
|
-(I - P) = V_plus @ V_plus.conj().T`.
|
|
|
|
The subspaces are computed using the naiive QR eigendecomposition
|
|
algorithm, which converges very quickly due to the sharp separation
|
|
between the relevant eigenvalues of the projector.
|
|
|
|
Args:
|
|
P: A rank-`rank` Hermitian projector into the space of `H`'s
|
|
first `rank` eigenpairs. `P` is padded to NxN.
|
|
H: The aforementioned Hermitian matrix, which is used to track
|
|
convergence.
|
|
n: the true (dynamic) shape of `P`.
|
|
rank: Rank of `P`.
|
|
maxiter: Maximum number of iterations.
|
|
Returns:
|
|
V_minus, V_plus: Isometries into the eigenspaces described in the docstring.
|
|
"""
|
|
# Choose an initial guess: the `rank` largest-norm columns of P.
|
|
N, _ = P.shape
|
|
column_norms = jnp_linalg.norm(P, axis=1)
|
|
# `jnp.argsort` ensures NaNs sort last, so set masked-out column norms to NaN.
|
|
column_norms = _mask(column_norms, (n,), jnp.nan)
|
|
sort_idxs = jnp.argsort(column_norms)
|
|
X = P[:, sort_idxs]
|
|
# X = X[:, :rank]
|
|
X = _mask(X, (n, rank))
|
|
|
|
H_norm = jnp_linalg.norm(H)
|
|
thresh = 10.0 * float(jnp.finfo(X.dtype).eps) * H_norm
|
|
|
|
# First iteration skips the matmul.
|
|
def body_f_after_matmul(X):
|
|
Q, _ = jnp_linalg.qr(X, mode="complete")
|
|
# V1 = Q[:, :rank]
|
|
# V2 = Q[:, rank:]
|
|
V1 = _mask(Q, (n, rank))
|
|
V2 = _slice(Q, (0, rank), (n, n - rank), (N, N))
|
|
|
|
# TODO: might be able to get away with lower precision here
|
|
error_matrix = jnp.dot(V2.conj().T, H)
|
|
error_matrix = jnp.dot(error_matrix, V1)
|
|
error = jnp_linalg.norm(error_matrix) / H_norm
|
|
return V1, V2, error
|
|
|
|
def cond_f(args):
|
|
_, _, j, error = args
|
|
still_counting = j < maxiter
|
|
unconverged = error > thresh
|
|
return jnp.logical_and(still_counting, unconverged)[0]
|
|
|
|
def body_f(args):
|
|
V1, _, j, _ = args
|
|
X = jnp.dot(P, V1)
|
|
V1, V2, error = body_f_after_matmul(X)
|
|
return V1, V2, j + 1, error
|
|
|
|
V1, V2, error = body_f_after_matmul(X)
|
|
one = jnp.ones(1, dtype=jnp.int32)
|
|
V1, V2, _, error = lax.while_loop(cond_f, body_f, (V1, V2, one, error))
|
|
return V1, V2
|
|
|
|
|
|
def split_spectrum(H, n, split_point, V0=None):
|
|
""" The Hermitian matrix `H` is split into two matrices `H_minus`
|
|
`H_plus`, respectively sharing its eigenspaces beneath and above
|
|
its `split_point`th eigenvalue.
|
|
|
|
Returns, in addition, `V_minus` and `V_plus`, isometries such that
|
|
`Hi = Vi.conj().T @ H @ Vi`. If `V0` is not None, `V0 @ Vi` are
|
|
returned instead; this allows the overall isometries mapping from
|
|
an initial input matrix to progressively smaller blocks to be formed.
|
|
|
|
Args:
|
|
H: The Hermitian matrix to split.
|
|
split_point: The eigenvalue to split along.
|
|
V0: Matrix of isometries to be updated.
|
|
Returns:
|
|
H_minus: A Hermitian matrix sharing the eigenvalues of `H` beneath
|
|
`split_point`.
|
|
V_minus: An isometry from the input space of `V0` to `H_minus`.
|
|
H_plus: A Hermitian matrix sharing the eigenvalues of `H` above
|
|
`split_point`.
|
|
V_plus: An isometry from the input space of `V0` to `H_plus`.
|
|
rank: The dynamic size of the m subblock.
|
|
"""
|
|
N, _ = H.shape
|
|
H_shift = H - (split_point * jnp.eye(N, dtype=split_point.dtype)).astype(H.dtype)
|
|
U, _, _, _ = qdwh.qdwh(H_shift, is_hermitian=True, dynamic_shape=(n, n))
|
|
P = -0.5 * (U - _mask(jnp.eye(N, dtype=H.dtype), (n, n)))
|
|
rank = jnp.round(jnp.trace(jnp.real(P))).astype(jnp.int32)
|
|
|
|
V_minus, V_plus = _projector_subspace(P, H, n, rank)
|
|
H_minus = (V_minus.conj().T @ H) @ V_minus
|
|
H_plus = (V_plus.conj().T @ H) @ V_plus
|
|
if V0 is not None:
|
|
V_minus = jnp.dot(V0, V_minus)
|
|
V_plus = jnp.dot(V0, V_plus)
|
|
return H_minus, V_minus, H_plus, V_plus, rank
|
|
|
|
|
|
# To help understand the iterative version of the algorithm, the original
|
|
# recursive formulation follows.
|
|
#
|
|
# def _eigh_work(H, V=None, termination_size=128):
|
|
# """ The main work loop performing the symmetric eigendecomposition of H.
|
|
# Each step recursively computes a projector into the space of eigenvalues
|
|
# above jnp.mean(jnp.diag(H)). The result of the projections into and out of
|
|
# that space, along with the isometries accomplishing these, are then computed.
|
|
# This is performed recursively until the projections have size 1, and thus
|
|
# store an eigenvalue of the original input; the corresponding isometry is
|
|
# the related eigenvector. The results are then composed.
|
|
#
|
|
# Args:
|
|
# H: The Hermitian input.
|
|
# V: Stores the isometries projecting H into its subspaces.
|
|
# precision: :class:`~jax.lax.Precision` object specifying the matmul precision.
|
|
#
|
|
# Returns:
|
|
# H, V: The result of the projection.
|
|
# """
|
|
# if H.shape[0] <= termination_size:
|
|
# evals, evecs = jnp_linalg.eigh(H)
|
|
# if V is not None:
|
|
# evecs = jnp.dot(V, evecs)
|
|
# return evals, evecs
|
|
#
|
|
# split_point = jnp.median(jnp.diag(H)) # TODO: Improve this?
|
|
# H_minus, V_minus, H_plus, V_plus = split_spectrum(H, split_point, V0=V)
|
|
# H_minus, V_minus = _eigh_work(H_minus, V=V_minus, termination_size=termination_size)
|
|
# H_plus, V_plus = _eigh_work(H_plus, V=V_plus, termination_size=termination_size)
|
|
#
|
|
# evals = jnp.hstack((H_minus, H_plus))
|
|
# evecs = jnp.hstack((V_minus, V_plus))
|
|
# return evals, evecs
|
|
|
|
class _Subproblem(NamedTuple):
|
|
"""Describes a subproblem of _eigh_work.
|
|
|
|
Each subproblem is a `size` x `size` Hermitian matrix, starting at `offset`
|
|
in the workspace.
|
|
"""
|
|
# The row offset of the block in the matrix of blocks.
|
|
offset: jnp.ndarray
|
|
|
|
# The size of the block.
|
|
size: jnp.ndarray
|
|
|
|
@partial(jax.jit, static_argnames=('termination_size',))
|
|
def _eigh_work(H, n, termination_size=256):
|
|
""" The main work loop performing the symmetric eigendecomposition of H.
|
|
Each step recursively computes a projector into the space of eigenvalues
|
|
above jnp.mean(jnp.diag(H)). The result of the projections into and out of
|
|
that space, along with the isometries accomplishing these, are then computed.
|
|
This is performed recursively until the projections have size 1, and thus
|
|
store an eigenvalue of the original input; the corresponding isometry is
|
|
the related eigenvector. The results are then composed.
|
|
|
|
This function cannot be Jitted because the internal split_spectrum cannot
|
|
be.
|
|
|
|
Args:
|
|
H: The Hermitian input.
|
|
n: The true (dynamic) shape of H.
|
|
|
|
Returns:
|
|
H, V: The result of the projection.
|
|
"""
|
|
# We turn what was originally a recursive algorithm into an iterative
|
|
# algorithm with an explicit stack.
|
|
N, _ = H.shape
|
|
n = jnp.asarray(n, jnp.int32)
|
|
agenda = Stack.create(
|
|
N + 1, _Subproblem(jnp.array(0, jnp.int32), jnp.array(0, jnp.int32)))
|
|
agenda = agenda.push(_Subproblem(offset=jnp.int32(0), size=n))
|
|
|
|
# eigenvectors is the array in which we build the output eigenvectors.
|
|
# We initialize it with the identity matrix so the initial matrix
|
|
# multiplications in_split_spectrum_jittable are the identity.
|
|
eigenvectors = jnp.eye(N, dtype=H.dtype)
|
|
|
|
# blocks is an array representing a stack of Hermitian matrix blocks that we
|
|
# need to recursively decompose. Subproblems are different sizes, so the stack
|
|
# of blocks is ragged. Subproblems are left-aligned (i.e. starting at the 0th
|
|
# column). Here is an ASCII art picture of three blocks A, B, C, embedded
|
|
# in the larger `blocks` workspace (represented with trailing dots).
|
|
#
|
|
# A A A . . .
|
|
# A A A . . .
|
|
# A A A . . .
|
|
# B B . . . .
|
|
# B B . . . .
|
|
# C C C C . .
|
|
# C C C C . .
|
|
# C C C C . .
|
|
# C C C C . .
|
|
#
|
|
# Each step of the algorithm subdivides a block into two subblocks whose
|
|
# sizes sum to the original block size. We overwrite the original block with
|
|
# those two subblocks so we don't need any additional scratch space.
|
|
#
|
|
# At termination, "blocks" will contain 1x1 blocks (i.e., the eigenvalues) in
|
|
# its first column.
|
|
blocks = H
|
|
|
|
def base_case(B, offset, b, agenda, blocks, eigenvectors):
|
|
# Base case: for blocks under a minimum size, we cutoff the recursion
|
|
# and call the TPU Jacobi eigendecomposition implementation. The Jacobi
|
|
# algorithm works well for small matrices but scales poorly, so the two
|
|
# complement each other well.
|
|
H = _slice(blocks, (offset, 0), (b, b), (B, B))
|
|
V = _slice(eigenvectors, (0, offset), (n, b), (N, B))
|
|
|
|
# We replace the masked-out part of the matrix with the identity matrix.
|
|
# We know that the TPU Jacobi eigh implementation will not alter the order
|
|
# of the eigenvalues, so we know the eigendecomposition of the original
|
|
# matrix is in the top-left corner of the eigendecomposition of the padded
|
|
# matrix.
|
|
# It is very important that the underlying eigh implementation does not sort
|
|
# the eigenvalues for this reason! This is currently not true of JAX's CPU
|
|
# and GPU eigendecompositions, and for those platforms this algorithm will
|
|
# only do the right thing if termination_size == 1.
|
|
H = _mask(H, (b, b), jnp.eye(B, dtype=H.dtype))
|
|
eig_vecs, eig_vals = lax.linalg.eigh(H, sort_eigenvalues=False)
|
|
eig_vecs = _mask(eig_vecs, (b, b))
|
|
eig_vals = _mask(eig_vals, (b,))
|
|
eig_vecs = jnp.dot(V, eig_vecs)
|
|
|
|
eig_vals = eig_vals.astype(eig_vecs.dtype)
|
|
blocks = _update_slice(blocks, eig_vals[:, None], (offset, 0), (b, b))
|
|
eigenvectors = _update_slice(eigenvectors, eig_vecs, (0, offset), (n, b))
|
|
return agenda, blocks, eigenvectors
|
|
|
|
def recursive_case(B, offset, b, agenda, blocks, eigenvectors):
|
|
# The recursive case of the algorithm, specialized to a static block size
|
|
# of B.
|
|
H = _slice(blocks, (offset, 0), (b, b), (B, B))
|
|
V = _slice(eigenvectors, (0, offset), (n, b), (N, B))
|
|
|
|
split_point = jnp.nanmedian(_mask(jnp.diag(jnp.real(H)), (b,), jnp.nan)) # TODO: Improve this?
|
|
H_minus, V_minus, H_plus, V_plus, rank = split_spectrum(H, b, split_point, V0=V)
|
|
|
|
blocks = _update_slice(blocks, H_minus, (offset, 0), (rank, rank))
|
|
blocks = _update_slice(blocks, H_plus, (offset + rank, 0), (b - rank, b - rank))
|
|
eigenvectors = _update_slice(eigenvectors, V_minus, (0, offset), (n, rank))
|
|
eigenvectors = _update_slice(eigenvectors, V_plus, (0, offset + rank),
|
|
(n, b - rank))
|
|
|
|
agenda = agenda.push(_Subproblem(offset + rank, (b - rank)))
|
|
agenda = agenda.push(_Subproblem(offset, rank))
|
|
return agenda, blocks, eigenvectors
|
|
|
|
def loop_cond(state):
|
|
agenda, _, _ = state
|
|
return ~agenda.empty()
|
|
|
|
# It would be wasteful to perform all computation padded up to the original
|
|
# matrix size. Instead, we form buckets of padded sizes e.g.,
|
|
# [256, 512, 1024, ..., N], aiming for a balance between compilation time
|
|
# and runtime.
|
|
cutoff = min(N, termination_size)
|
|
buckets = [cutoff]
|
|
branches = [partial(base_case, cutoff)]
|
|
i = cutoff
|
|
while i < N:
|
|
i = min(2 * i, N)
|
|
buckets.append(i)
|
|
branches.append(partial(recursive_case, i))
|
|
buckets = jnp.array(buckets, dtype='int32')
|
|
|
|
def loop_body(state):
|
|
agenda, blocks, eigenvectors = state
|
|
(offset, b), agenda = agenda.pop()
|
|
which = jnp.where(buckets < b, jnp.iinfo(jnp.int32).max, buckets)
|
|
choice = jnp.argmin(which)
|
|
return lax.switch(choice, branches, offset, b, agenda, blocks, eigenvectors)
|
|
|
|
_, blocks, eigenvectors = lax.while_loop(
|
|
loop_cond, loop_body, (agenda, blocks, eigenvectors))
|
|
return blocks[:, 0], eigenvectors
|
|
|
|
|
|
def eigh(H, *, precision="float32", termination_size=256, n=None,
|
|
sort_eigenvalues=True):
|
|
""" Computes the eigendecomposition of the symmetric/Hermitian matrix H.
|
|
|
|
Args:
|
|
H: The `n x n` Hermitian input, padded to `N x N`.
|
|
precision: :class:`~jax.lax.Precision` object specifying the matmul precision.
|
|
termination_size: Recursion ends once the blocks reach this linear size.
|
|
n: the true (dynamic) size of the matrix.
|
|
sort_eigenvalues: If `True`, the eigenvalues will be sorted from lowest to
|
|
highest.
|
|
Returns:
|
|
vals: The `n` eigenvalues of `H`.
|
|
vecs: A unitary matrix such that `vecs[:, i]` is a normalized eigenvector
|
|
of `H` corresponding to `vals[i]`. We have `H @ vecs = vals * vecs` up
|
|
to numerical error.
|
|
"""
|
|
M, N = H.shape
|
|
if M != N:
|
|
raise TypeError(f"Input H of shape {H.shape} must be square.")
|
|
|
|
if N <= termination_size:
|
|
if n is not None:
|
|
H = _mask(H, (n, n), jnp.eye(N, dtype=H.dtype))
|
|
return lax_linalg.eigh_jacobi(
|
|
H, sort_eigenvalues=sort_eigenvalues)
|
|
|
|
# TODO(phawkins): consider rounding N up to a larger size to maximize reuse
|
|
# between matrices.
|
|
|
|
n = N if n is None else n
|
|
with jax.default_matmul_precision(precision):
|
|
eig_vals, eig_vecs = _eigh_work(H, n, termination_size=termination_size)
|
|
eig_vals = _mask(jnp.real(eig_vals), (n,), jnp.nan)
|
|
if sort_eigenvalues:
|
|
sort_idxs = jnp.argsort(eig_vals)
|
|
eig_vals = eig_vals[sort_idxs]
|
|
eig_vecs = eig_vecs[:, sort_idxs]
|
|
return eig_vals, eig_vecs
|