mirror of
https://github.com/ROCm/jax.git
synced 2025-04-15 19:36:06 +00:00
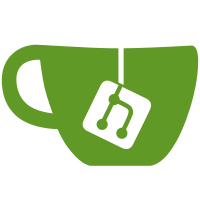
These docstrings do not make the tests any more clear and typically just duplicate the test module name. PiperOrigin-RevId: 737611977
1880 lines
65 KiB
Python
1880 lines
65 KiB
Python
# Copyright 2020 The JAX Authors.
|
|
#
|
|
# Licensed under the Apache License, Version 2.0 (the "License");
|
|
# you may not use this file except in compliance with the License.
|
|
# You may obtain a copy of the License at
|
|
#
|
|
# https://www.apache.org/licenses/LICENSE-2.0
|
|
#
|
|
# Unless required by applicable law or agreed to in writing, software
|
|
# distributed under the License is distributed on an "AS IS" BASIS,
|
|
# WITHOUT WARRANTIES OR CONDITIONS OF ANY KIND, either express or implied.
|
|
# See the License for the specific language governing permissions and
|
|
# limitations under the License.
|
|
|
|
from collections.abc import Callable
|
|
import contextlib
|
|
from functools import partial
|
|
import os
|
|
import unittest
|
|
|
|
from absl import logging
|
|
from absl.testing import absltest
|
|
from absl.testing import parameterized
|
|
import jax
|
|
from jax import dlpack
|
|
from jax import dtypes
|
|
from jax import export
|
|
from jax import lax
|
|
from jax import numpy as jnp
|
|
from jax._src import config
|
|
from jax._src import test_util as jtu
|
|
from jax._src.lib.mlir import ir
|
|
from jax._src.lib.mlir.dialects import hlo
|
|
from jax.experimental import jax2tf
|
|
from jax.experimental.jax2tf.tests import tf_test_util
|
|
import numpy as np
|
|
|
|
try:
|
|
import tensorflow as tf
|
|
except ImportError:
|
|
tf = None
|
|
|
|
jax.config.parse_flags_with_absl()
|
|
|
|
|
|
def _maybe_jit(with_jit: bool, func: Callable) -> Callable:
|
|
if with_jit:
|
|
return jax.jit(func)
|
|
else:
|
|
return func
|
|
|
|
def _maybe_tf_jit(with_jit: bool, func: Callable) -> Callable:
|
|
if with_jit:
|
|
return tf.function(func, autograph=False, jit_compile=True)
|
|
else:
|
|
return func
|
|
|
|
def _named_test(**kwargs):
|
|
return dict(kwargs,
|
|
testcase_name = "_".join([f"{k}={kwargs[k]}" for k in sorted(kwargs.keys())]))
|
|
|
|
_parameterized_jit = parameterized.named_parameters(
|
|
_named_test(with_jit=with_jit)
|
|
for with_jit in [True, False])
|
|
|
|
_call_tf_non_compilable_error = "Error compiling TensorFlow function"
|
|
_call_tf_dynamic_shape_error = "call_tf cannot call functions whose output has dynamic shape"
|
|
|
|
class CallTfTest(tf_test_util.JaxToTfTestCase):
|
|
|
|
def setUp(self):
|
|
if tf is None:
|
|
raise unittest.SkipTest("Test requires tensorflow")
|
|
# TODO(b/171320191): this line works around a missing context initialization
|
|
# bug in TensorFlow.
|
|
_ = tf.add(1, 1)
|
|
super().setUp()
|
|
# One TF device of each device_type
|
|
self.tf_devices = []
|
|
for tf_device in tf.config.list_logical_devices():
|
|
if tf_device.device_type == "TPU_SYSTEM":
|
|
continue # A virtual device
|
|
if all(tf_device.device_type != d.device_type for d in self.tf_devices):
|
|
self.tf_devices.append(tf_device)
|
|
self.warning_ctx = jtu.ignore_warning(
|
|
message=(
|
|
"(jax2tf.convert with native_serialization=False has been deprecated"
|
|
"|Calling from_dlpack with a DLPack tensor is deprecated)"
|
|
)
|
|
)
|
|
self.warning_ctx.__enter__()
|
|
|
|
def tearDown(self):
|
|
self.warning_ctx.__exit__(None, None, None)
|
|
super().tearDown()
|
|
|
|
@_parameterized_jit
|
|
def test_eval_scalar_arg(self, with_jit=True):
|
|
def f_tf(x):
|
|
return tf.math.sin(x)
|
|
x = 3.
|
|
res = _maybe_jit(with_jit, jax2tf.call_tf(f_tf))(x)
|
|
self.assertAllClose(jnp.sin(x), res)
|
|
|
|
@_parameterized_jit
|
|
def test_eval_scalar_res(self, with_jit=True):
|
|
x = 3.
|
|
res = _maybe_jit(with_jit, jax2tf.call_tf(lambda x: 4.))(x)
|
|
self.assertAllClose(4., res, check_dtypes=False)
|
|
|
|
@_parameterized_jit
|
|
def test_eval_numpy_arg(self, with_jit=True):
|
|
x = np.ones((2, 3), dtype=np.float32)
|
|
res = _maybe_jit(with_jit, jax2tf.call_tf(tf.math.sin))(x)
|
|
self.assertAllClose(jnp.sin(x), res)
|
|
|
|
@_parameterized_jit
|
|
def test_eval_numpy_res(self, with_jit=False):
|
|
x = np.ones((2, 3))
|
|
res = _maybe_jit(with_jit, jax2tf.call_tf(lambda _: x))(x)
|
|
self.assertAllClose(x, res)
|
|
|
|
@_parameterized_jit
|
|
def test_eval_devicearray_arg(self, with_jit=False):
|
|
x = jnp.ones((2, 3), dtype=np.float32)
|
|
res = _maybe_jit(with_jit, jax2tf.call_tf(tf.math.sin))(x)
|
|
self.assertAllClose(jnp.sin(x), res)
|
|
|
|
x = jnp.array(3.0, dtype=jnp.bfloat16)
|
|
res = jax2tf.call_tf(lambda x: x)(x)
|
|
self.assertAllClose(x, res)
|
|
# bfloat16 scalar will create a copy.
|
|
with self.assertRaises(AssertionError):
|
|
self.assertTrue(np.shares_memory(x, res))
|
|
|
|
@_parameterized_jit
|
|
def test_eval_pytree(self, with_jit=True):
|
|
|
|
def fun_tf(x: dict, y: tuple) -> tuple:
|
|
return (x["first"] * x["second"], y[0] + y[1])
|
|
|
|
x = dict(first=np.float32(3.), second=np.float32(4.))
|
|
y = (np.float64(5.), np.float64(6.))
|
|
fun_jax = _maybe_jit(with_jit, jax2tf.call_tf(fun_tf))
|
|
res = fun_jax(x, y)
|
|
self.assertAllClose((np.float32(12.), np.float64(11.)), res)
|
|
|
|
def test_result_tuple(self):
|
|
x1 = np.ones(3, dtype=np.int32)
|
|
x2 = np.ones(5, dtype=np.float32)
|
|
def fun_tf():
|
|
return tf.tuple([x1, x2])
|
|
|
|
fun_jax = jax.jit(jax2tf.call_tf(fun_tf))
|
|
res = fun_jax()
|
|
self.assertAllClose(res, (x1, x2))
|
|
|
|
def test_error_non_compilable_strings(self):
|
|
# Check that in op-by-op we call a function in eager mode.
|
|
def f_tf_non_compilable(x):
|
|
return tf.strings.length(tf.strings.format("Hello {}!", [x]))
|
|
|
|
f_jax = jax2tf.call_tf(f_tf_non_compilable)
|
|
x = np.float32(0.7)
|
|
self.assertAllClose(f_tf_non_compilable(x).numpy(), f_jax(x))
|
|
with self.assertRaisesRegex(ValueError,
|
|
_call_tf_non_compilable_error):
|
|
jax.jit(f_jax)(x)
|
|
|
|
with self.assertRaisesRegex(ValueError,
|
|
_call_tf_non_compilable_error):
|
|
lax.cond(True, lambda x: f_jax(x), lambda x: f_jax(x), x)
|
|
|
|
def test_error_non_compilable_dynamic_shape(self):
|
|
# Check that in op-by-op we call a function in eager mode.
|
|
def f_tf_non_compilable(x):
|
|
return tf.cond(x[0], lambda: x[1:], lambda: x)
|
|
|
|
f_jax = jax2tf.call_tf(f_tf_non_compilable)
|
|
x = np.array([True, False], dtype=np.bool_)
|
|
self.assertAllClose(f_tf_non_compilable(x), f_jax(x)) # Works in eager mode
|
|
|
|
with self.assertRaisesRegex(ValueError, _call_tf_dynamic_shape_error):
|
|
jax.jit(f_jax)(x)
|
|
|
|
def test_error_bad_result_tensorarray(self):
|
|
# Call a function that returns a tf.TensorArray. This should be detected
|
|
# early on. If we don't the function is actually compilable but returns
|
|
# a tuple instead of a single result.
|
|
def fun_tf():
|
|
ta = tf.TensorArray(tf.int32, size=0, dynamic_size=True)
|
|
ta = ta.unstack([0, 1, 2, 3, 4])
|
|
return ta
|
|
|
|
with self.assertRaisesRegex(ValueError,
|
|
"The called TF function returns a result that is not convertible to JAX"):
|
|
fun_jax = jax.jit(jax2tf.call_tf(fun_tf))
|
|
fun_jax()
|
|
|
|
def test_error_bad_result_string(self):
|
|
def fun_tf():
|
|
return tf.constant("foo")
|
|
|
|
# Now under jit, should fail because the function is not compilable
|
|
with self.assertRaisesRegex(ValueError,
|
|
"The called TF function returns a result that is not convertible to JAX"):
|
|
fun_jax = jax.jit(jax2tf.call_tf(fun_tf))
|
|
fun_jax()
|
|
|
|
@_parameterized_jit
|
|
def test_control_flow(self, with_jit=True):
|
|
|
|
def times_5_tf(x):
|
|
# Multiply x * 5 using a loop
|
|
c = lambda i, acc: tf.less(i, 5)
|
|
b = lambda i, acc: (tf.add(i, 1), tf.add(acc, x))
|
|
_, acc = tf.while_loop(c, b, [tf.constant(0), tf.constant(0.)])
|
|
return acc
|
|
|
|
def fun_jax(x):
|
|
# Calls times_5_tf 3 times in a loop
|
|
def body(_, acc):
|
|
return jax2tf.call_tf(times_5_tf)(acc)
|
|
|
|
return lax.fori_loop(0, 3, body, x)
|
|
|
|
x = np.float32(3.)
|
|
res = _maybe_jit(with_jit, fun_jax)(x)
|
|
self.assertAllClose(np.float32(x * 5 * 5 * 5), res)
|
|
|
|
@parameterized.named_parameters(
|
|
dict(
|
|
testcase_name=f"_{dtype.__name__}{'_jit' if with_jit else ''}",
|
|
dtype=dtype,
|
|
with_jit=with_jit)
|
|
for dtype in set(jtu.dtypes.all) - {np.bool_}
|
|
for with_jit in [True, False])
|
|
def test_dtypes(self, dtype=np.int32, with_jit=True):
|
|
|
|
def fun_tf(x):
|
|
# AddV2 supports more types
|
|
return tf.raw_ops.AddV2(x=x, y=tf.constant(3, dtype=dtype))
|
|
|
|
def fun_jax(x):
|
|
return jax2tf.call_tf(fun_tf)(x) + x
|
|
|
|
x = np.ones((3,), dtype=dtype)
|
|
res = _maybe_jit(with_jit, fun_jax)(x)
|
|
self.assertAllClose(dtype(2 * x + 3), res)
|
|
|
|
@_parameterized_jit
|
|
def test_bool(self, with_jit=False):
|
|
|
|
def fun_tf(x, y):
|
|
return tf.math.logical_and(x, y)
|
|
|
|
x = np.array([True, False, True, False], dtype=np.bool_)
|
|
y = np.array([True, True, False, False], dtype=np.bool_)
|
|
res = _maybe_jit(with_jit, jax2tf.call_tf(fun_tf))(x, y)
|
|
self.assertAllClose(
|
|
np.array([True, False, False, False], dtype=np.bool_), res)
|
|
|
|
@_parameterized_jit
|
|
def test_x64_input(self, with_jit=True):
|
|
def f_tf(x):
|
|
return tf.math.sin(x)
|
|
|
|
x = 5. # TF interprets this as f64
|
|
res_call_tf = _maybe_jit(with_jit, jax2tf.call_tf(f_tf))(x)
|
|
res_jax = jnp.sin(x)
|
|
self.assertAllClose(res_call_tf, res_jax)
|
|
|
|
@_parameterized_jit
|
|
def test_x64_output(self, with_jit=True):
|
|
def f_tf(x):
|
|
return (tf.constant(3., tf.float64), x)
|
|
|
|
x = np.float32(5.)
|
|
res_call_tf = _maybe_jit(with_jit, jax2tf.call_tf(f_tf))(x)
|
|
res_jax = (3., x)
|
|
self.assertAllClose(res_call_tf, res_jax)
|
|
|
|
res_call_tf_jit = jax.jit(jax2tf.call_tf(f_tf))(x)
|
|
self.assertAllClose(res_call_tf_jit, res_jax)
|
|
|
|
@_parameterized_jit
|
|
def test_with_var_read(self, with_jit=True):
|
|
# The variable is placed on the default TF device.
|
|
outer_var_array = np.array([3., 4.], dtype=np.float32)
|
|
outer_var = tf.Variable(outer_var_array)
|
|
|
|
def fun_tf(x):
|
|
return x * outer_var + 1.
|
|
|
|
x = np.array([2., 5.,], dtype=np.float32)
|
|
res = _maybe_jit(with_jit, jax2tf.call_tf(fun_tf))(x)
|
|
self.assertAllClose(x * outer_var_array + 1., res, check_dtypes=False)
|
|
|
|
@_parameterized_jit
|
|
def test_with_var_read_x64(self, with_jit=True):
|
|
outer_var_array = np.array([3., 4.], dtype=np.float64)
|
|
outer_var = tf.Variable(outer_var_array)
|
|
|
|
def fun_tf(x):
|
|
return x * tf.cast(outer_var, x.dtype) + 1.
|
|
|
|
x = np.array([2., 5.,], dtype=np.float32)
|
|
res = _maybe_jit(with_jit, jax2tf.call_tf(fun_tf))(x)
|
|
self.assertAllClose(x * outer_var_array + 1., res, check_dtypes=False)
|
|
|
|
def test_with_var_different_shape(self):
|
|
# See https://github.com/jax-ml/jax/issues/6050
|
|
v = tf.Variable((4., 2.), dtype=tf.float32)
|
|
|
|
def tf_func(x):
|
|
return v + x
|
|
x = np.float32(123.)
|
|
tf_out = tf_func(x)
|
|
|
|
jax_func = jax.jit(jax2tf.call_tf(tf_func))
|
|
jax_out = jax_func(x)
|
|
|
|
self.assertAllClose(tf_out, jax_out, check_dtypes=False)
|
|
|
|
@_parameterized_jit
|
|
def test_with_var_write_error(self, with_jit=True):
|
|
if with_jit:
|
|
raise unittest.SkipTest("variable writes not yet working")
|
|
outer_var = tf.Variable(3., dtype=np.float32)
|
|
|
|
def fun_tf(x):
|
|
outer_var.assign(tf.constant(4.))
|
|
return x * outer_var + 1.
|
|
|
|
x = np.float32(2.)
|
|
res = _maybe_jit(with_jit, jax2tf.call_tf(fun_tf))(x)
|
|
self.assertAllClose(x * 4. + 1, res, check_dtypes=False)
|
|
|
|
@_parameterized_jit
|
|
def test_with_tensor_capture(self, with_jit=True):
|
|
outer_tensor = tf.constant(3., dtype=np.float32)
|
|
|
|
def fun_tf(x):
|
|
return x * outer_tensor + 1.
|
|
|
|
x = np.float32(2.)
|
|
res = _maybe_jit(with_jit, jax2tf.call_tf(fun_tf))(x)
|
|
self.assertAllClose(x * 3. + 1., res, check_dtypes=False)
|
|
|
|
@_parameterized_jit
|
|
def test_with_tensor_capture_x64(self, with_jit=True):
|
|
outer_tensor = tf.constant(3., dtype=np.float64)
|
|
|
|
def fun_tf(x):
|
|
return x * tf.cast(outer_tensor * 3.14, tf.float32) + 1.
|
|
|
|
x = np.float32(2.)
|
|
res = _maybe_jit(with_jit, jax2tf.call_tf(fun_tf))(x)
|
|
self.assertAllClose(x * 3. * 3.14 + 1., res, check_dtypes=False)
|
|
|
|
@_parameterized_jit
|
|
def test_with_value_capture(self, with_jit=True):
|
|
outer_val = np.array(3., dtype=np.float32)
|
|
|
|
def fun_tf(x):
|
|
return x * outer_val + 1.
|
|
|
|
x = np.float32(2.)
|
|
res = _maybe_jit(with_jit, jax2tf.call_tf(fun_tf))(x)
|
|
self.assertAllClose(x * 3. + 1., res, check_dtypes=False)
|
|
|
|
@_parameterized_jit
|
|
def test_with_multiple_capture(self, with_jit=True):
|
|
if jtu.test_device_matches(["gpu"]):
|
|
raise unittest.SkipTest("Test fails on GPU")
|
|
v2 = tf.Variable(2., dtype=np.float32)
|
|
v3 = tf.Variable(3., dtype=np.float32)
|
|
t4 = tf.constant(4., dtype=np.float32)
|
|
t5 = tf.constant(5., dtype=np.float32)
|
|
|
|
def fun_tf(x):
|
|
return (x * v3 + t4 + v2) * v3 + t5
|
|
|
|
x = np.float32(2.)
|
|
res = _maybe_jit(with_jit, jax2tf.call_tf(fun_tf))(x)
|
|
self.assertAllClose((x * 3. + 4. + 2.) * 3. + 5., res, check_dtypes=False)
|
|
|
|
def test_with_capture_then_convert_again(self):
|
|
captured_by_tf = tf.Variable(np.arange(1024, dtype=np.float32))
|
|
def tf_fn(x):
|
|
return tf.math.add(x, captured_by_tf)
|
|
|
|
x = np.arange(1024, dtype=np.float32)
|
|
res = jax2tf.convert(jax2tf.call_tf(tf_fn))(x)
|
|
self.assertAllClose(res, 2 * x)
|
|
|
|
# The bug appears only when we use non-eager mode on the converted func
|
|
res = tf.function(jax2tf.convert(jax2tf.call_tf(tf_fn)),
|
|
autograph=False)(x)
|
|
self.assertAllClose(res, 2 * x)
|
|
|
|
@_parameterized_jit
|
|
def test_grad(self, with_jit=False):
|
|
x = np.float32(3.)
|
|
res = _maybe_jit(with_jit, jax.grad(jax2tf.call_tf(tf.math.sin)))(x)
|
|
self.assertAllClose(np.cos(x), res)
|
|
|
|
@_parameterized_jit
|
|
def test_grad_pytree(self, with_jit=False):
|
|
|
|
def fun_tf(x: dict, y: tuple) -> tuple:
|
|
return x["first"] * x["second"] + 3. * y[0] + 4. * y[1]
|
|
|
|
x = dict(first=np.float32(3.), second=np.float32(4.))
|
|
y = (np.float32(5.), np.float32(6.))
|
|
grad_x = _maybe_jit(with_jit, jax.grad(jax2tf.call_tf(fun_tf)))(x, y)
|
|
self.assertAllClose(
|
|
dict(first=np.float32(4.), second=np.float32(3.)), grad_x)
|
|
|
|
def test_grad_nested(self):
|
|
# We embed the call_tf function in a larger function whose gradient we take
|
|
# It is relevant here that the cotangents flowing through the call_tf
|
|
# function are not scalars.
|
|
|
|
b = np.array([[11., 12., 13.], [21., 22., 23.]], dtype=np.float32) # [2, 3]
|
|
c = np.array([[31., 32.], [41., 42.], [51., 52.], [61., 62.]], dtype=np.float32) # [4, 2]
|
|
x_dict = dict(b=b, c=c) # b:[2, 3], c=[4, 2]
|
|
# res: dict(r:[4, 3], s:[4, 2])
|
|
def f_tf(x_dict):
|
|
return dict(r=tf.matmul(x_dict["c"], x_dict["b"]), s=7. * x_dict["c"])
|
|
|
|
@jax.jit # To recognize it in jaxpr
|
|
def f_jax(x_dict):
|
|
return dict(r=jnp.matmul(x_dict["c"], x_dict["b"]), s=7. * x_dict["c"])
|
|
|
|
def loss(functional, x_dict):
|
|
prediction = functional(x_dict) # r:[4, 3], s:[4, 2]
|
|
weights = np.array([1., 2., 3., 4.], dtype=np.float32) # [4]
|
|
weighted_pred = jnp.matmul(weights, prediction["r"]) # [3]
|
|
return jnp.sum(weighted_pred) + 4. * jnp.sum(prediction["s"])
|
|
|
|
g_fun_with_tf = jax.grad(partial(loss, jax2tf.call_tf(f_tf)))
|
|
g_fun_with_jax = jax.grad(partial(loss, f_jax))
|
|
|
|
g_tf = g_fun_with_tf(x_dict)
|
|
g_jax = g_fun_with_jax(x_dict)
|
|
self.assertAllClose(g_jax, g_tf)
|
|
|
|
def test_grad_int_argument(self):
|
|
# Similar to https://github.com/jax-ml/jax/issues/6975
|
|
# state is a pytree that contains an integer and a boolean.
|
|
# The function returns an integer and a boolean.
|
|
def f(param, state, x):
|
|
return param * x, state
|
|
|
|
param = np.array([0.7, 0.9], dtype=np.float32)
|
|
state = dict(array=np.float32(1.), counter=7, truth=True)
|
|
x = np.float32(3.)
|
|
|
|
# tf.function is important, without it the bug does not appear
|
|
f_call_tf = jax2tf.call_tf(f)
|
|
g_call_tf = jax.grad(lambda *args: jnp.sum(f_call_tf(*args)[0]))(param, state, x)
|
|
g = jax.grad(lambda *args: jnp.sum(f(*args)[0]))(param, state, x)
|
|
self.assertAllClose(g_call_tf, g)
|
|
|
|
def test_grad_int_argument_unused(self):
|
|
batch_size = 5
|
|
inputs = np.ones((batch_size, 3), dtype=np.float32)
|
|
rng = np.array([1, 2], dtype=np.uint32)
|
|
params = np.float32(.5)
|
|
|
|
# rng is integer, unused
|
|
def jax_model(params, rng, inputs):
|
|
return jnp.ones([batch_size, 2], dtype=jnp.float32)
|
|
|
|
tf_model = jax2tf.convert(jax_model, with_gradient=True)
|
|
|
|
def _loss_fn(inference_fn, params, rng, inputs):
|
|
prediction = inference_fn(params, rng, inputs)
|
|
return jnp.mean(prediction)
|
|
|
|
jax_loss_fn = partial(_loss_fn, jax_model)
|
|
jax_grad = jax.grad(jax_loss_fn)(params, rng, inputs)
|
|
|
|
paramsv = tf.Variable(params)
|
|
with tf.GradientTape() as tape:
|
|
tf_prediction = tf_model(paramsv, rng, inputs)
|
|
tf_loss = tf.reduce_mean(tf_prediction)
|
|
|
|
tf_grad = tape.gradient(tf_loss, paramsv)
|
|
self.assertAllClose(jax_grad, tf_grad.numpy())
|
|
|
|
call_tf_loss_fn = partial(_loss_fn, jax2tf.call_tf(tf_model))
|
|
call_tf_grad = jax.grad(call_tf_loss_fn)(params, rng, inputs)
|
|
self.assertAllClose(jax_grad, call_tf_grad)
|
|
|
|
def test_grad_with_float0_result(self):
|
|
# Gradient over integer-argument functions, with float0 result
|
|
def f_jax(x, y): # x is an int, y is a float; res is a (int, float)
|
|
return (2 * x, 2 * x + y * y)
|
|
def f_tf(x, y):
|
|
# TF needs explicit casts
|
|
return (2 * x, tf.cast(2 * x, dtype=y.dtype) + y * y)
|
|
|
|
def wrapper(functional, x, y): # x: i32
|
|
return jnp.sum(2. * functional(3 * x, 4. * y)[1])
|
|
|
|
grad_g = jax.grad(partial(wrapper, f_jax),
|
|
allow_int=True, argnums=(0, 1))
|
|
grad_g_call_tf = jax.grad(partial(wrapper, jax2tf.call_tf(f_tf)),
|
|
allow_int=True, argnums=(0, 1))
|
|
|
|
x = np.int32(2)
|
|
y = np.float32(3.)
|
|
g_jax = grad_g(x, y)
|
|
g_call_tf = grad_g_call_tf(x, y)
|
|
self.assertEqual(g_jax[0].dtype, dtypes.float0)
|
|
self.assertEqual(g_call_tf[0].dtype, dtypes.float0)
|
|
self.assertAllClose(g_jax[1], g_call_tf[1])
|
|
|
|
@_parameterized_jit
|
|
def test_grad_custom(self, with_jit=False):
|
|
|
|
@tf.custom_gradient
|
|
def func_square_tf(x):
|
|
# Like x ** 2, but with custom grad 3. * x
|
|
def grad(dy, variables=None):
|
|
# dy, = dys
|
|
return 3. * x * dy,
|
|
|
|
return x * x, grad
|
|
|
|
x = np.float32(4.)
|
|
grad_x = _maybe_jit(with_jit, jax.grad(jax2tf.call_tf(func_square_tf)))(x)
|
|
self.assertAllClose(np.float32(3.) * x, grad_x)
|
|
|
|
@parameterized.named_parameters(
|
|
dict(
|
|
testcase_name=f"_{degree=}{'_jit' if with_jit else ''}",
|
|
degree=degree,
|
|
with_jit=with_jit)
|
|
for degree in [1, 2, 3, 4]
|
|
for with_jit in [True, False])
|
|
def test_higher_order_grad(self, degree=2, with_jit=False):
|
|
|
|
def fun_tf(x):
|
|
return 2. * x * x * x
|
|
|
|
def fun_jax(x):
|
|
return 3. * _maybe_jit(with_jit, jax2tf.call_tf(fun_tf))(x)
|
|
|
|
def fun_jax_pure(x):
|
|
return 3. * fun_tf(x)
|
|
|
|
grad_jax = fun_jax
|
|
grad_jax_pure = fun_jax_pure
|
|
for _ in range(degree):
|
|
grad_jax = jax.grad(grad_jax)
|
|
grad_jax_pure = jax.grad(grad_jax_pure)
|
|
|
|
res_jax = grad_jax(np.float32(5.))
|
|
logging.info("Grad of %s degree is %s", degree, res_jax)
|
|
self.assertAllClose(res_jax, grad_jax_pure(np.float32(5.)))
|
|
|
|
def test_pmap(self):
|
|
logging.info("Running test_pmap on %s devices", jax.local_device_count())
|
|
|
|
def plus_2_tf(x):
|
|
return tf.math.add(2., x)
|
|
|
|
def fun_jax(x):
|
|
return np.float32(3.) * jax2tf.call_tf(plus_2_tf)(x)
|
|
|
|
x = np.arange(jax.local_device_count(), dtype=np.float32)
|
|
res = jax.pmap(fun_jax)(x)
|
|
self.assertAllClose(np.float32(3. * (x + 2)), res)
|
|
|
|
def test_function_compile_time_constant_inputs(self):
|
|
# Call a function for which shape inference does not give an output
|
|
# shape.
|
|
x = np.array([1, 2, 3], dtype=np.int32)
|
|
def fun_tf(x): # x:i32[3]
|
|
# Indexing with a dynamic slice makes the TF shape inference return
|
|
# a partially known shape.
|
|
end_idx = x[1]
|
|
res = x[0:end_idx]
|
|
return res
|
|
|
|
# Call in eager mode. Should work!
|
|
res1 = jax2tf.call_tf(fun_tf)(x)
|
|
self.assertAllClose(x[0:x[1]], res1)
|
|
|
|
# Now under jit, should fail because the function is not compilable
|
|
with self.assertRaisesRegex(ValueError, _call_tf_dynamic_shape_error):
|
|
fun_jax = jax.jit(jax2tf.call_tf(fun_tf))
|
|
fun_jax(x)
|
|
|
|
def test_experimental_get_compiler_ir_design_doc(self):
|
|
# Not a test of call_tf, but more of how experimental_get_compiler_ir works.
|
|
# Examples are from the design doc.
|
|
|
|
# Constant slice. This is the common case.
|
|
x = np.zeros((10,), dtype=np.int32)
|
|
|
|
def fun_tf(x):
|
|
begin = 0
|
|
return x[begin:5]
|
|
|
|
hlo = tf.function(fun_tf, jit_compile=True, autograph=False).experimental_get_compiler_ir(x)()
|
|
self.assertIn("(arg0.1: s32[10]) -> s32[5]", hlo)
|
|
|
|
# Non-constant slice, but compile-time constant depending only on values.
|
|
x = np.zeros((10,), dtype=np.int32)
|
|
|
|
# Non-constant slice, but compile-time constant depending only on shapes.
|
|
x = np.zeros((10,), dtype=np.int32)
|
|
|
|
def fun_tf(x):
|
|
begin = tf.shape(x)[0] - 2 # begin is a compile-time constant, even if x is not
|
|
return x[begin:]
|
|
|
|
hlo = tf.function(fun_tf, jit_compile=True, autograph=False).experimental_get_compiler_ir(x)()
|
|
self.assertIn("(arg0.1: s32[10]) -> s32[2]", hlo)
|
|
|
|
# Capture a variable
|
|
outer_var = tf.Variable(np.array([3.], dtype=np.float32))
|
|
x = np.array([2., 3., 4.], dtype=np.float32)
|
|
|
|
def fun_tf(x):
|
|
return x * tf.broadcast_to(outer_var, x.shape) + 1.
|
|
|
|
hlo = tf.function(fun_tf, jit_compile=True, autograph=False).experimental_get_compiler_ir(x)()
|
|
self.assertIn("(arg0.1: f32[3], arg1.2: f32[1]) -> f32[3]", hlo)
|
|
|
|
# Capture a constant
|
|
outer_ct = np.array([3.], dtype=np.float32)
|
|
x = np.array([2., 3., 4.], dtype=np.float32)
|
|
|
|
def fun_tf(x):
|
|
return x * tf.broadcast_to(outer_ct, x.shape) + 1.
|
|
|
|
hlo = tf.function(fun_tf, jit_compile=True, autograph=False).experimental_get_compiler_ir(x)()
|
|
self.assertIn("(arg0.1: f32[3]) -> f32[3]", hlo)
|
|
|
|
# Call get_compiler_ir in a function context
|
|
x = np.array([2., 3., 4.], dtype=np.float32)
|
|
|
|
def fun_tf_outer(x):
|
|
x_const = tf.constant(0, shape=x.shape, dtype=x.dtype)
|
|
_ = tf.function(tf.math.sin, jit_compile=True, autograph=False).experimental_get_compiler_ir(x_const)()
|
|
|
|
# TODO(b/193754660)
|
|
# with self.assertRaisesRegex(
|
|
# TypeError, "An op outside of the function building code is being passed"):
|
|
# tf.function(fun_tf_outer)(x)
|
|
#
|
|
# with self.assertRaisesRegex(
|
|
# TypeError, "An op outside of the function building code is being passed"):
|
|
# tf.function(fun_tf_outer, jit_compile=True)(x)
|
|
|
|
# Call get_concrete_function in a graph context
|
|
def fun_tf_outer_2(x):
|
|
_ = tf.function(tf.math.sin, jit_compile=True).get_concrete_function(tf.TensorSpec(x.shape, x.dtype))
|
|
return x
|
|
|
|
# Outside of a function context, this works.
|
|
_ = tf.function(fun_tf_outer_2)(x)
|
|
_ = tf.function(fun_tf_outer_2, jit_compile=True)(x)
|
|
|
|
def test_repro_193754660(self):
|
|
# Try to reproduce b/193754660. I can't.
|
|
# We have to have tf.function(jax2tf.convert(jax2tf.call_tf(f_tf))).
|
|
# The get_compiler_ir will indeed fail for f_tf. Then we try to use
|
|
# shape inference for f_tf.
|
|
# I thought to use a f_tf that uses an op without shape inference, e.g.,
|
|
# tfxla.gather. If we wash it through a saved_model I expect that shape
|
|
# inference would not work on it. Instead, shape inference works!!!
|
|
x = np.array([0, 1, 2, 3, 4, 5], dtype=np.int32)
|
|
def f_jax(x):
|
|
return x[1]
|
|
f_tf = jax2tf.convert(f_jax)
|
|
f_tf_rt, _ = tf_test_util.SaveAndLoadFunction(f_tf, input_args=[x])
|
|
f_jax2 = jax2tf.call_tf(f_tf_rt)
|
|
f_tf2 = jax2tf.convert(f_jax2)
|
|
res = tf.function(f_tf2, autograph=False)(x)
|
|
self.assertAllClose(res.numpy(), f_jax(x))
|
|
|
|
def test_effectful(self):
|
|
x = np.ones((3,), dtype=np.float32)
|
|
lower_effect = jax.jit(jax2tf.call_tf(tf.math.sin, has_side_effects=True)).lower(x)
|
|
self.assertNotEmpty(lower_effect._lowering.compile_args["unordered_effects"])
|
|
|
|
lower_no_effect = jax.jit(jax2tf.call_tf(tf.math.sin, has_side_effects=False)).lower(x)
|
|
self.assertEmpty(lower_no_effect._lowering.compile_args["unordered_effects"])
|
|
|
|
def test_module_documentation(self):
|
|
def cos_tf(x):
|
|
return tf.math.cos(x)
|
|
|
|
# Compute cos with TF and sin with JAX
|
|
def cos_tf_sin_jax(x):
|
|
return jax.numpy.sin(jax2tf.call_tf(cos_tf)(x))
|
|
|
|
# Calls `cos_tf` in TF eager mode
|
|
x = np.float32(1.)
|
|
cos_tf_sin_jax(x)
|
|
|
|
# Compiles `cos_tf` using TF and embeds the XLA computation into the JAX
|
|
# XLA computation (containing `sin`). The XLA compiler may even be able to
|
|
# fuse through JAX-TF computations.
|
|
jax.jit(cos_tf_sin_jax)(x)
|
|
|
|
# Uses TF gradient for `cos_tf` and JAX gradient for `sin`
|
|
jax.grad(cos_tf_sin_jax)(x)
|
|
|
|
logging.info(jax.make_jaxpr(cos_tf_sin_jax)(x))
|
|
|
|
def test_tf_gather(self):
|
|
"""tf_gather gradient output is tf.IndexSlices."""
|
|
operand = jnp.array(np.random.uniform(size=(100, 128)))
|
|
indices = jnp.array(np.random.randint(low=0, high=100, size=(4000,)))
|
|
|
|
@tf.function(jit_compile=True, autograph=False)
|
|
def fun_tf(operand, indices):
|
|
return tf.experimental.numpy.std(tf.gather(operand, indices))
|
|
|
|
fun_jax = jax2tf.call_tf(fun_tf)
|
|
grad_fun_jax = jax.grad(fun_jax)
|
|
grad_res = grad_fun_jax(operand, indices)
|
|
self.assertEqual(grad_res.shape, (100, 128))
|
|
|
|
def test_output_shape_dtype_none(self):
|
|
x = jnp.zeros((10), dtype=jnp.float32)
|
|
|
|
@tf.function(jit_compile=True, autograph=False)
|
|
def fun_tf(x): # pylint: disable=unused-argument
|
|
return
|
|
|
|
fun_jax_1 = jax2tf.call_tf(fun_tf, output_shape_dtype=None)
|
|
fun_jax_2 = jax2tf.call_tf(fun_tf)
|
|
self.assertIsNone(fun_jax_1(x))
|
|
self.assertIsNone(fun_jax_2(x))
|
|
fun_jax_3 = jax2tf.call_tf(
|
|
fun_tf, output_shape_dtype=jax.ShapeDtypeStruct((10,), jnp.float32)
|
|
)
|
|
with self.assertRaisesRegex(
|
|
ValueError,
|
|
"The pytree of the TensorFlow function results does not match the"
|
|
" pytree of the declared output_shape_dtype",
|
|
):
|
|
_ = fun_jax_3(x)
|
|
|
|
def test_output_shape_dtype_not_none(self):
|
|
x = jnp.zeros((10), dtype=jnp.float32)
|
|
|
|
@tf.function(jit_compile=True, autograph=False)
|
|
def fun_tf(x):
|
|
return x
|
|
|
|
fun_jax_1 = jax2tf.call_tf(
|
|
fun_tf, output_shape_dtype=jax.ShapeDtypeStruct((10,), jnp.float32)
|
|
)
|
|
fun_jax_2 = jax2tf.call_tf(fun_tf)
|
|
self.assertAllClose(fun_jax_1(x), fun_jax_2(x))
|
|
|
|
fun_jax_3 = jax2tf.call_tf(fun_tf, output_shape_dtype=None)
|
|
with self.assertRaisesRegex(
|
|
ValueError,
|
|
"The pytree of the TensorFlow function results does not match the"
|
|
" pytree of the declared output_shape_dtype",
|
|
):
|
|
_ = fun_jax_3(x)
|
|
|
|
def test_multi_platform(self):
|
|
def tf_fun(x):
|
|
return tf.math.sin(x)
|
|
|
|
def f_jax(x):
|
|
return jnp.cos(jax2tf.call_tf(tf_fun)(jnp.cos(x)))
|
|
x = np.arange(12, dtype=np.float32).reshape((3, 4))
|
|
|
|
# Find platforms that are available for both JAX and TF
|
|
# Pick one device from each available platform
|
|
jax_platforms = []
|
|
for backend in ["cpu", "gpu", "tpu"]:
|
|
try:
|
|
devices = jax.devices(backend)
|
|
except RuntimeError:
|
|
devices = []
|
|
if devices:
|
|
jax_platforms.append(devices[0].platform)
|
|
|
|
jax_and_tf_platforms = (
|
|
set(jax_platforms) & {d.device_type.lower()
|
|
for d in self.tf_devices})
|
|
|
|
lowering_platforms = ("tpu", "cpu", "cuda")
|
|
|
|
exp = export.export(jax.jit(f_jax),
|
|
platforms=lowering_platforms)(x)
|
|
for jax_platform in jax_and_tf_platforms:
|
|
with self.subTest(jax_platform):
|
|
jax_device = jax.devices(jax_platform)[0]
|
|
x_device = jax.device_put(x, jax_device)
|
|
logging.info("Running harness natively on %s", jax_device)
|
|
native_res = f_jax(x_device)
|
|
logging.info("Running exported harness on %s", jax_device)
|
|
exported_res = exp.call(x_device)
|
|
self.assertAllClose(native_res, exported_res)
|
|
|
|
def test_multi_platform_call_tf_graph(self):
|
|
def tf_fun(x):
|
|
return tf.math.sin(x)
|
|
|
|
def f_jax(x):
|
|
return jnp.cos(jax2tf.call_tf(tf_fun,
|
|
call_tf_graph=True,
|
|
ordered=True)(jnp.cos(x)))
|
|
x = np.arange(12, dtype=np.float32).reshape((3, 4))
|
|
# When we use call_tf_graph we can serialize for multiple platforms
|
|
lowering_platforms = ("tpu", "cpu", "cuda")
|
|
# We must use jax2tf.convert to run a call_tf(call_tf_graph)
|
|
# TODO(necula): if we remove the tf.function and we have multiple platforms
|
|
# then we attempt to lower call_tf multiple times and only the first
|
|
# lowering will have the proper side effects for the function_list.
|
|
f_tf = tf.function(jax2tf.convert(
|
|
f_jax,
|
|
native_serialization=True,
|
|
native_serialization_platforms=lowering_platforms))
|
|
for tf_device in self.tf_devices:
|
|
with self.subTest(tf_device.device_type):
|
|
logging.info(
|
|
f"Running on tf_device = {tf_device} of device_type = {tf_device.device_type}")
|
|
with tf.device(tf_device):
|
|
res = f_tf(x)
|
|
self.assertAllClose(res, f_jax(x))
|
|
|
|
@parameterized.named_parameters(
|
|
{"testcase_name": f"_type={type_.__name__}", "type_": type_}
|
|
for type_ in dlpack.SUPPORTED_DTYPES
|
|
)
|
|
def test_avoid_copy_between_gpu_and_cpu(self, type_):
|
|
try:
|
|
gpu_devices = jax.devices("gpu")
|
|
except RuntimeError:
|
|
gpu_devices = []
|
|
if not gpu_devices:
|
|
raise unittest.SkipTest("Test requires a GPU device.")
|
|
|
|
def tf_fun(x):
|
|
if type_ == jnp.bool_:
|
|
return tf.math.logical_or(x, True)
|
|
else:
|
|
return x + 1
|
|
|
|
jax_array_on_gpu = jnp.zeros([1], type_, device=gpu_devices[0])
|
|
|
|
# Since the input array is already on a GPU device, we expect that no memory
|
|
# copy occurs between GPU and CPU. Thus, we expect no errors raised by the
|
|
# transfer guard.
|
|
# There are two exceptions:
|
|
# First, when dtype is "int32". This is because almost all TensorFlow
|
|
# kernels for GPU devices keep int32 tensors in host memory.
|
|
# (https://github.com/tensorflow/tensorflow/blob/4eb3e36d1b0cd511e1677e740bd093f42365cf9f/tensorflow/python/eager/pywrap_tensor.cc#L352-L354)
|
|
# Hence, for "int32", we do expect a "host-to-device" copy.
|
|
# Second, when using PJRT C API runtime. This is because it currently skips dlpack
|
|
# to workaround "PJRT C API does not support GetDefaultLayout" runtime error.
|
|
# https://github.com/openxla/xla/blob/762bde36adf22792e91c38fe87cabe5af05bfadc/xla/pjrt/pjrt_c_api_client.h#L285-L289
|
|
@contextlib.contextmanager
|
|
def _transfer_guard(guard_level):
|
|
with contextlib.ExitStack() as stack:
|
|
stack.enter_context(jax.transfer_guard_device_to_device(guard_level))
|
|
stack.enter_context(jax.transfer_guard_device_to_host(guard_level))
|
|
if type_ != jnp.int32:
|
|
stack.enter_context(jax.transfer_guard_host_to_device(guard_level))
|
|
yield
|
|
|
|
with _transfer_guard("disallow_explicit"):
|
|
jax2tf.call_tf(tf_fun)(jax_array_on_gpu)
|
|
|
|
|
|
class RoundTripToJaxTest(tf_test_util.JaxToTfTestCase):
|
|
"Reloading output of jax2tf into JAX with call_tf"
|
|
|
|
def setUp(self):
|
|
if tf is None:
|
|
raise unittest.SkipTest("Test requires tensorflow")
|
|
# TODO(b/171320191): this line works around a missing context initialization
|
|
# bug in TensorFlow.
|
|
_ = tf.add(1, 1)
|
|
super().setUp()
|
|
self.warning_ctx = jtu.ignore_warning(
|
|
message=(
|
|
"(jax2tf.convert with native_serialization=False has been deprecated"
|
|
"|Calling from_dlpack with a DLPack tensor is deprecated)"
|
|
)
|
|
)
|
|
self.warning_ctx.__enter__()
|
|
|
|
def tearDown(self):
|
|
self.warning_ctx.__exit__(None, None, None)
|
|
super().tearDown()
|
|
|
|
def test_simple(self):
|
|
f_jax = jnp.sin
|
|
f_jax_rt = jax2tf.call_tf(jax2tf.convert(f_jax))
|
|
x = np.float32(0.7)
|
|
self.assertAllClose(f_jax(x), f_jax_rt(x))
|
|
|
|
def test_pytree(self):
|
|
def f_jax(x): # x: dict(a=f32, b=f32)
|
|
return dict(a=x["a"]+1., b=x)
|
|
x = dict(a=0.7, b=0.8)
|
|
f_jax_rt = jax2tf.call_tf(jax2tf.convert(f_jax))
|
|
self.assertAllClose(f_jax(x), f_jax_rt(x))
|
|
|
|
def test_custom_grad(self):
|
|
@jax.custom_vjp
|
|
def f(x):
|
|
return x * x
|
|
|
|
# f_fwd: a -> (b, residual)
|
|
def f_fwd(x):
|
|
return f(x), np.float32(3.) * x
|
|
# f_bwd: (residual, CT b) -> [CT a]
|
|
def f_bwd(residual, ct_b):
|
|
return residual * ct_b,
|
|
|
|
f.defvjp(f_fwd, f_bwd)
|
|
|
|
f_rt = jax2tf.call_tf(jax2tf.convert(f, with_gradient=True))
|
|
x = np.float32(0.7)
|
|
self.assertAllClose(f(x), f_rt(x))
|
|
self.assertAllClose(jax.grad(f)(x), jax.grad(f_rt)(x))
|
|
|
|
def test_shape_poly(self):
|
|
f_jax = jnp.sin
|
|
f_jax_rt = jax2tf.call_tf(jax2tf.convert(f_jax,
|
|
polymorphic_shapes=["(b, ...)"]))
|
|
x = np.array([0.7, 0.8], dtype=np.float32)
|
|
self.assertAllClose(f_jax(x), f_jax_rt(x))
|
|
|
|
def test_saved_model_simple(self):
|
|
x = np.array([0.7, 0.8], dtype=np.float32)
|
|
def f_jax(x):
|
|
return jnp.sin(x)
|
|
|
|
f_tf = jax2tf.convert(f_jax)
|
|
restored_tf, _ = tf_test_util.SaveAndLoadFunction(f_tf, input_args=[x])
|
|
restored_jax = jax2tf.call_tf(restored_tf)
|
|
self.assertAllClose(f_jax(x), restored_jax(x))
|
|
|
|
def test_saved_model_variables(self):
|
|
param = np.array([1., 2.], dtype=np.float32)
|
|
x = np.array([0.7, 0.8], dtype=np.float32)
|
|
def f_jax(param, x):
|
|
return jnp.sin(x) + jnp.cos(param)
|
|
|
|
param_v = tf.Variable(param)
|
|
f_tf = jax2tf.convert(f_jax)
|
|
_, restored_model = tf_test_util.SaveAndLoadFunction(
|
|
lambda x: f_tf(param_v, x),
|
|
input_args=[x],
|
|
variables=[param_v])
|
|
restored_jax = jax2tf.call_tf(restored_model.f)
|
|
self.assertAllClose(f_jax(param, x), restored_jax(x))
|
|
self.assertAllClose(f_jax(param, x), jax.jit(restored_jax)(x))
|
|
self.assertAllClose(f_jax(param, x), jax2tf.convert(restored_jax)(x))
|
|
self.assertAllClose(f_jax(param, x),
|
|
tf.function(jax2tf.convert(restored_jax),
|
|
autograph=False)(x))
|
|
self.assertAllClose(f_jax(param, x),
|
|
tf.function(jax2tf.convert(restored_jax),
|
|
autograph=True)(x))
|
|
|
|
def test_saved_model_shape_poly(self):
|
|
tracing_count = 0
|
|
x = np.array([0.7, 0.8], dtype=np.float32)
|
|
def f_jax(x):
|
|
nonlocal tracing_count
|
|
tracing_count += 1
|
|
return jnp.sin(x)
|
|
|
|
f_tf = jax2tf.convert(f_jax, polymorphic_shapes=["(b, ...)"])
|
|
res_jax = f_jax(x)
|
|
self.assertEqual(1, tracing_count)
|
|
# Will trace twice, it seems. Once to get the result signature, and once again
|
|
# for the actual saving.
|
|
restored_f, _ = tf_test_util.SaveAndLoadFunction(
|
|
f_tf, input_signature=[tf.TensorSpec([None], x.dtype)])
|
|
self.assertGreaterEqual(tracing_count, 2)
|
|
tracing_count = 0
|
|
f_jax_rt = jax2tf.call_tf(restored_f)
|
|
self.assertAllClose(res_jax, f_jax_rt(x))
|
|
# Ensure that restored_f works at other batch size as well
|
|
y = np.concatenate([x, x])
|
|
self.assertEqual(0, tracing_count)
|
|
res_jax_y = f_jax(y)
|
|
self.assertEqual(1, tracing_count)
|
|
# No more tracing for f_jax_rt
|
|
self.assertAllClose(res_jax_y, f_jax_rt(y))
|
|
self.assertEqual(1, tracing_count)
|
|
|
|
def test_custom_grad_saved_model(self):
|
|
|
|
@jax.custom_vjp
|
|
def f(x):
|
|
return x * x
|
|
|
|
# f_fwd: a -> (b, residual)
|
|
def f_fwd(x):
|
|
return f(x), np.float32(3.) * x
|
|
# f_bwd: (residual, CT b) -> [CT a]
|
|
def f_bwd(residual, ct_b):
|
|
return residual * ct_b,
|
|
|
|
f.defvjp(f_fwd, f_bwd)
|
|
def g(x):
|
|
return jnp.sum(f(x))
|
|
|
|
g_tf, _ = tf_test_util.SaveAndLoadFunction(
|
|
jax2tf.convert(g, with_gradient=True),
|
|
input_signature=[tf.TensorSpec(shape=(1,), dtype=tf.float32)],
|
|
)
|
|
g_rt = jax2tf.call_tf(g_tf)
|
|
x = np.array([0.7], dtype=np.float32)
|
|
self.assertAllClose(g(x), g_rt(x))
|
|
self.assertAllClose(jax.grad(g)(x), jax.grad(g_rt)(x))
|
|
|
|
def test_without_gradient_saved_model(self):
|
|
# Explicitly with_gradient=False
|
|
f_jax = jnp.sum
|
|
|
|
x = np.array([0.7, 0.8], dtype=np.float32)
|
|
f_tf, _ = tf_test_util.SaveAndLoadFunction(
|
|
jax2tf.convert(f_jax, with_gradient=False),
|
|
input_args=[x])
|
|
f_rt = jax2tf.call_tf(f_tf)
|
|
|
|
self.assertAllClose(f_jax(x), f_rt(x))
|
|
with self.assertRaisesRegex(Exception,
|
|
"Gradient explicitly disabled.*jax2tf-converted function does not support gradients. Use `with_gradient` parameter to enable gradients"):
|
|
jax.grad(f_rt)(x)
|
|
|
|
def test_saved_model_no_gradients(self):
|
|
# Save without gradients
|
|
f_jax = jnp.sum
|
|
|
|
x = np.array([0.7, 0.8], dtype=np.float32)
|
|
f_tf, _ = tf_test_util.SaveAndLoadFunction(
|
|
jax2tf.convert(f_jax, with_gradient=True), input_args=[x],
|
|
save_gradients=False)
|
|
f_rt = jax2tf.call_tf(f_tf)
|
|
|
|
self.assertAllClose(f_jax(x), f_rt(x))
|
|
# TODO: clean this up b/191117111: it should fail with a clear error
|
|
# The following results in a confusing error:
|
|
# TypeError: tf.Graph captured an external symbolic tensor.
|
|
with self.assertRaises(TypeError):
|
|
_ = jax.grad(f_rt)(x)
|
|
|
|
def test_call_tf_under_function_context(self):
|
|
def fun_jax(x, y):
|
|
z = jax2tf.call_tf(tf.math.sin)(x) + jnp.cos(y)
|
|
return z
|
|
|
|
x = np.array([-1.0, 0.0, 1.0], dtype=np.float32)
|
|
y = np.array([-0.5, 0.0, 0.5], dtype=np.float32)
|
|
|
|
converted_fun = tf.function(
|
|
jax2tf.convert(fun_jax, native_serialization=True)
|
|
)
|
|
expected = np.sin(x) + np.cos(y)
|
|
res = tf.function(converted_fun, jit_compile=True, autograph=False)(x, y)
|
|
self.assertAllClose(expected, res.numpy(), atol=1e-5, rtol=1e-5)
|
|
|
|
@parameterized.named_parameters(
|
|
dict(
|
|
testcase_name=f"_{dtype.__name__}",
|
|
dtype=dtype,
|
|
)
|
|
for dtype in set(jtu.dtypes.all_floating)
|
|
)
|
|
def test_all_floating_input_gradient(self, dtype):
|
|
def tf_f(x):
|
|
res = tf.math.sin(x)
|
|
return tf.reduce_sum(res)
|
|
|
|
jax_f = jax2tf.call_tf(tf_f)
|
|
tf_f_rt = jax2tf.convert(jax_f)
|
|
x = jnp.array([5.0, 6.0, 7.0]).astype(dtype)
|
|
|
|
def assert_all_close_support_bfloat16(baseline, candidate):
|
|
def conversion(x):
|
|
# convert scalar to array and bfloat16 to float32
|
|
# to support self.assertAllClose numpy array comparison.
|
|
if x.shape == tf.TensorShape([]):
|
|
x = tf.convert_to_tensor([x])
|
|
if dtype == jnp.float16:
|
|
x = tf.cast(x, tf.float32)
|
|
return x
|
|
|
|
baseline = jax.tree_util.tree_map(conversion, baseline)
|
|
candidate = jax.tree_util.tree_map(conversion, candidate)
|
|
tol = (
|
|
1e-2
|
|
if jtu.test_device_matches(["tpu"]) and dtype == np.float16
|
|
else None
|
|
)
|
|
self.assertAllClose(baseline, candidate, atol=tol, rtol=tol)
|
|
|
|
# Eager mode
|
|
assert_all_close_support_bfloat16(tf_f(x), tf_f_rt(x))
|
|
|
|
# Compiled function mode
|
|
assert_all_close_support_bfloat16(
|
|
tf.function(tf_f)(x), tf.function(tf_f_rt)(x)
|
|
)
|
|
|
|
# Compiled function mode with jit_compiled=True
|
|
assert_all_close_support_bfloat16(
|
|
tf.function(tf_f, jit_compile=True)(x),
|
|
tf.function(tf_f_rt, jit_compile=True)(x),
|
|
)
|
|
|
|
# RoundTrip test for the gradient
|
|
grad_fun_jax = jax.grad(jax2tf.call_tf(tf_f))
|
|
grad_fun_jax_rt = jax2tf.call_tf(jax2tf.convert(grad_fun_jax))
|
|
|
|
# Eager mode
|
|
assert_all_close_support_bfloat16(grad_fun_jax(x), grad_fun_jax_rt(x))
|
|
|
|
# Jit mode
|
|
assert_all_close_support_bfloat16(
|
|
jax.jit(grad_fun_jax)(x), jax.jit(grad_fun_jax_rt)(x)
|
|
)
|
|
|
|
@parameterized.named_parameters(
|
|
dict(
|
|
testcase_name=f"_{dtype.__name__}",
|
|
dtype=dtype,
|
|
)
|
|
for dtype in set(jtu.dtypes.complex)
|
|
)
|
|
def test_complex_input_gradient(self, dtype):
|
|
def tf_f(x):
|
|
res = tf.math.sin(x)
|
|
return tf.reduce_sum(res)
|
|
|
|
x = jnp.array([(5.0 + 4.0j), (6.0 + 3.0j), (7.0 + 8.0j)]).astype(dtype)
|
|
|
|
jax_f = jax2tf.call_tf(tf_f)
|
|
tf_f_rt = jax2tf.convert(jax_f)
|
|
|
|
# Eager mode
|
|
self.assertAllClose(tf_f(x), tf_f_rt(x))
|
|
|
|
# tf.function context
|
|
self.assertAllClose(tf.function(tf_f)(x), tf.function(tf_f_rt)(x))
|
|
|
|
# tf.function context with jit_compiled=True
|
|
self.assertAllClose(
|
|
tf.function(tf_f, jit_compile=True)(x),
|
|
tf.function(tf_f_rt, jit_compile=True)(x),
|
|
)
|
|
|
|
# RoundTrip test for the gradient
|
|
grad_fun_jax = jax.grad(jax2tf.call_tf(tf_f), holomorphic=True)
|
|
grad_fun_jax_rt = jax2tf.call_tf(jax2tf.convert(grad_fun_jax))
|
|
|
|
# Eager mode
|
|
self.assertAllClose(grad_fun_jax(x), grad_fun_jax_rt(x))
|
|
|
|
# Jit mode
|
|
self.assertAllClose(jax.jit(grad_fun_jax)(x), jax.jit(grad_fun_jax_rt)(x))
|
|
|
|
def test_grad_pytree_arg_with_none_leaf(self):
|
|
def tf_f(x, params):
|
|
return x * params["y"]
|
|
|
|
x = jnp.array(1.0)
|
|
y = jnp.array(2.0)
|
|
actual = jax.grad(
|
|
jax2tf.call_tf(tf_f), argnums=(1,))(x, {"y": y, "other": None})
|
|
self.assertDictEqual(actual[0], {"y": x, "other": None})
|
|
|
|
|
|
class RoundTripToTfTest(tf_test_util.JaxToTfTestCase):
|
|
"Reloading output of call_tf into TF with jax2tf."
|
|
|
|
def setUp(self):
|
|
if tf is None:
|
|
raise unittest.SkipTest("Test requires tensorflow")
|
|
# TODO(b/171320191): this line works around a missing context initialization
|
|
# bug in TensorFlow.
|
|
_ = tf.add(1, 1)
|
|
super().setUp()
|
|
self.warning_ctx = jtu.ignore_warning(
|
|
message=(
|
|
"(jax2tf.convert with native_serialization=False has been deprecated"
|
|
"|Calling from_dlpack with a DLPack tensor is deprecated)"
|
|
)
|
|
)
|
|
self.warning_ctx.__enter__()
|
|
|
|
def tearDown(self):
|
|
self.warning_ctx.__exit__(None, None, None)
|
|
super().tearDown()
|
|
|
|
def test_alternate(self):
|
|
# Alternate sin/cos with sin in TF and cos in JAX
|
|
f_tf_inner = tf.math.sin
|
|
def f_jax(x_jax):
|
|
y_jax = jnp.cos(x_jax)
|
|
z_jax = jax2tf.call_tf(f_tf_inner)(y_jax)
|
|
return jnp.cos(z_jax)
|
|
def f_tf_outer(x_tf):
|
|
y_tf = tf.math.sin(x_tf)
|
|
z_tf = jax2tf.convert(f_jax)(y_tf)
|
|
return tf.math.sin(z_tf)
|
|
|
|
x = np.float32(0.7)
|
|
|
|
self.assertAllClose(np.sin(np.cos(np.sin(np.cos(np.sin(x))))),
|
|
f_tf_outer(x).numpy())
|
|
xv = tf.Variable(x)
|
|
with tf.GradientTape() as tape:
|
|
res = f_tf_outer(xv)
|
|
g_tf = tape.gradient(res, xv)
|
|
_, gf = tf_test_util.ComputeTfValueAndGrad(f_tf_outer, (x,))
|
|
# Eager
|
|
expected_res = np.sin(np.cos(np.sin(np.cos(np.sin(x)))))
|
|
self.assertAllClose(expected_res, f_tf_outer(x).numpy())
|
|
|
|
# Gradient
|
|
expected_grad = (np.cos(np.cos(np.sin(np.cos(np.sin(x))))) *
|
|
np.sin(np.sin(np.cos(np.sin(x)))) *
|
|
np.cos(np.cos(np.sin(x))) *
|
|
np.sin(np.sin(x)) *
|
|
np.cos(x))
|
|
self.assertAllClose(expected_grad, g_tf.numpy())
|
|
|
|
# Graph
|
|
self.assertAllClose(expected_res,
|
|
tf.function(f_tf_outer, autograph=False)(x).numpy())
|
|
|
|
# Compiled
|
|
self.assertAllClose(expected_res,
|
|
tf.function(f_tf_outer, autograph=False,
|
|
jit_compile=True)(x).numpy())
|
|
|
|
def test_saved_model(self):
|
|
x = np.array([.7, .8], dtype=np.float32)
|
|
def fun_tf(x):
|
|
return tf.math.sin(x)
|
|
def fun_jax(x):
|
|
return jax2tf.call_tf(fun_tf)(x)
|
|
|
|
# Now convert and save to SavedModel
|
|
fun_tf_rt = jax2tf.convert(fun_jax)
|
|
res = fun_tf_rt(x)
|
|
self.assertAllClose(np.sin(x), res.numpy())
|
|
|
|
res = tf.function(fun_tf_rt, autograph=False)(x)
|
|
self.assertAllClose(np.sin(x), res.numpy())
|
|
|
|
res = tf.function(fun_tf_rt, jit_compile=True, autograph=False)(x)
|
|
self.assertAllClose(np.sin(x), res.numpy())
|
|
|
|
reloaded_f, _ = tf_test_util.SaveAndLoadFunction(
|
|
fun_tf_rt, input_args=[x])
|
|
res = reloaded_f(x)
|
|
self.assertAllClose(np.sin(x), res.numpy())
|
|
|
|
def test_saved_model_polymorphic_input_static_output(self):
|
|
x = np.array([.7, .8], dtype=np.float32)
|
|
def fun_tf(x):
|
|
return tf.math.reduce_sum(tf.math.sin(x))
|
|
def fun_jax(x):
|
|
return jax2tf.call_tf(fun_tf)(x)
|
|
|
|
# Now convert and save to SavedModel
|
|
fun_tf_rt = jax2tf.convert(fun_jax)
|
|
res = fun_tf_rt(x)
|
|
self.assertAllClose(fun_tf(x), res.numpy())
|
|
|
|
res = tf.function(fun_tf_rt, autograph=False)(x)
|
|
self.assertAllClose(fun_tf(x), res.numpy())
|
|
|
|
res = tf.function(fun_tf_rt, jit_compile=True, autograph=False)(x)
|
|
self.assertAllClose(fun_tf(x), res.numpy())
|
|
|
|
reloaded_f, _ = tf_test_util.SaveAndLoadFunction(
|
|
fun_tf_rt, input_args=[x])
|
|
res = reloaded_f(x)
|
|
self.assertAllClose(fun_tf(x), res.numpy())
|
|
|
|
def test_function_dynamic_shape(self):
|
|
# Call a function for which shape inference does not give an output
|
|
# shape.
|
|
x = np.array([-1, 0, 1], dtype=np.int32)
|
|
def fun_tf(x): # x:i32[3]
|
|
# The shape depends on the value of x
|
|
return tf.cond(x[0] >= 0, lambda: x, lambda: x[1:])
|
|
|
|
# Call in eager mode. Should work!
|
|
res1 = jax2tf.call_tf(fun_tf)(x)
|
|
expected = x[1:]
|
|
self.assertAllClose(expected, res1, check_dtypes=False)
|
|
|
|
# Now under jit, should fail because the function is not compilable
|
|
with self.assertRaisesRegex(ValueError, _call_tf_dynamic_shape_error):
|
|
fun_jax = jax.jit(jax2tf.call_tf(fun_tf))
|
|
fun_jax(x)
|
|
|
|
# TODO(necula): this should work in op-by-op mode, but it fails because
|
|
# jax2tf.convert does abstract evaluation.
|
|
with self.assertRaisesRegex(ValueError, _call_tf_dynamic_shape_error):
|
|
fun_tf_rt = jax2tf.convert(jax2tf.call_tf(fun_tf))
|
|
fun_tf_rt(x)
|
|
|
|
@_parameterized_jit
|
|
def test_shape_poly_static_output_shape(self, with_jit=True):
|
|
if jax.config.jax2tf_default_native_serialization:
|
|
raise unittest.SkipTest("TODO(b/268386622): call_tf with shape polymorphism and native serialization.")
|
|
x = np.array([0.7, 0.8], dtype=np.float32)
|
|
|
|
def fun_tf(x):
|
|
return tf.math.reduce_sum(tf.math.sin(x))
|
|
|
|
fun_jax = jax2tf.call_tf(fun_tf)
|
|
fun_tf_rt = _maybe_tf_jit(with_jit,
|
|
jax2tf.convert(fun_jax, polymorphic_shapes=["b, ..."]))
|
|
self.assertAllClose(fun_tf(x), fun_tf_rt(x))
|
|
|
|
@_parameterized_jit
|
|
def test_shape_poly(self, with_jit=False):
|
|
if jax.config.jax2tf_default_native_serialization:
|
|
raise unittest.SkipTest("TODO(b/268386622): call_tf with shape polymorphism and native serialization.")
|
|
x = np.array([7, 8, 9, 10], dtype=np.float32)
|
|
def fun_jax(x):
|
|
y = jax2tf.call_tf(tf.math.sin,
|
|
output_shape_dtype=jax.ShapeDtypeStruct(x.shape, x.dtype))(x)
|
|
z = jnp.cos(y)
|
|
w = jax2tf.call_tf(lambda z: tf.concat([z, z], axis=0),
|
|
output_shape_dtype=jax.ShapeDtypeStruct((2 * z.shape[0],), z.dtype))(z)
|
|
assert w.shape[0] == 2 * x.shape[0]
|
|
return w
|
|
|
|
fun_tf_rt = _maybe_tf_jit(with_jit,
|
|
jax2tf.convert(fun_jax, polymorphic_shapes=["b, ..."]))
|
|
res_tf = fun_tf_rt(x)
|
|
self.assertAllClose(fun_jax(x), res_tf)
|
|
|
|
@_parameterized_jit
|
|
def test_shape_poly_pytree_result(self, with_jit=True):
|
|
if jax.config.jax2tf_default_native_serialization:
|
|
raise unittest.SkipTest("TODO(b/268386622): call_tf with shape polymorphism and native serialization.")
|
|
x = np.array([7, 8, 9, 10], dtype=np.float32)
|
|
def fun_jax(x):
|
|
# Returns a tuple
|
|
y = jax2tf.call_tf(lambda x: (x, tf.concat([x, x], axis=0)),
|
|
output_shape_dtype=(jax.ShapeDtypeStruct(x.shape, x.dtype),
|
|
jax.ShapeDtypeStruct((2 * x.shape[0],), x.dtype)))(x)
|
|
assert y[0].shape[0] == x.shape[0]
|
|
assert y[1].shape[0] == 2 * x.shape[0]
|
|
return y
|
|
|
|
fun_tf_rt = _maybe_tf_jit(with_jit,
|
|
jax2tf.convert(fun_jax, polymorphic_shapes=["b, ..."]))
|
|
res_tf = fun_tf_rt(x)
|
|
self.assertAllClose(fun_jax(x), res_tf)
|
|
|
|
@_parameterized_jit
|
|
def test_shape_poly_error_no_output_shape_dtype(self, with_jit=True):
|
|
x = np.array([7, 8, 9, 10], dtype=np.float32)
|
|
def fun_jax(x):
|
|
return jax2tf.call_tf(tf.math.sin)(x)
|
|
|
|
fun_tf_rt = _maybe_tf_jit(with_jit,
|
|
jax2tf.convert(fun_jax, polymorphic_shapes=["b, ..."]))
|
|
with self.assertRaisesRegex(ValueError, _call_tf_dynamic_shape_error):
|
|
fun_tf_rt(x)
|
|
|
|
@_parameterized_jit
|
|
def test_shape_poly_error_mismatch_output_shape_dtype_tree(self, with_jit=False):
|
|
x = np.array([7, 8, 9, 10], dtype=np.float32)
|
|
def fun_jax(x):
|
|
return jax2tf.call_tf(tf.math.sin,
|
|
output_shape_dtype=(jax.ShapeDtypeStruct(x.shape, x.dtype),
|
|
jax.ShapeDtypeStruct(x.shape, x.dtype)))(x)
|
|
|
|
fun_tf_rt = _maybe_tf_jit(with_jit,
|
|
jax2tf.convert(fun_jax, polymorphic_shapes=["b, ..."]))
|
|
|
|
with self.assertRaisesRegex(
|
|
ValueError,
|
|
"The pytree of the TensorFlow function results does not match the pytree of the declared output_shape_dtype"):
|
|
fun_tf_rt(x)
|
|
|
|
@parameterized.named_parameters(
|
|
_named_test(with_jit=with_jit, kind=kind)
|
|
for with_jit in [True, False]
|
|
for kind in ["bad_rank", "bad_dim", "bad_dtype", "bad_dtype_x64"])
|
|
def test_shape_poly_error_mismatch_output_shape_dtype(self, with_jit=False, kind="bad_rank"):
|
|
x = np.array([7, 8, 9, 10], dtype=np.float32)
|
|
|
|
if kind == "bad_rank":
|
|
def fun_jax(x):
|
|
return jax2tf.call_tf(lambda x: x,
|
|
# Wrong shape rank
|
|
output_shape_dtype=jax.ShapeDtypeStruct((), x.dtype))(x)
|
|
elif kind == "bad_dim":
|
|
def fun_jax(x):
|
|
bad_shape = (5 + x.shape[0],)
|
|
y = jax2tf.call_tf(lambda x: x,
|
|
# Wrong dimension
|
|
output_shape_dtype=jax.ShapeDtypeStruct(bad_shape, x.dtype))(x)
|
|
# JAX will believe that the following is Ok, leading to downstream error in TF
|
|
return y + jnp.ones(bad_shape, dtype=x.dtype)
|
|
elif kind == "bad_dtype":
|
|
def fun_jax(x):
|
|
return jax2tf.call_tf(lambda x: x,
|
|
output_shape_dtype=jax.ShapeDtypeStruct(x.shape, np.int32))(x)
|
|
elif kind == "bad_dtype_x64":
|
|
def fun_jax(x):
|
|
return jax2tf.call_tf(lambda x: x * np.float64(3.),
|
|
output_shape_dtype=jax.ShapeDtypeStruct(x.shape, np.float64))(x)
|
|
else:
|
|
assert False
|
|
expect_ex = ValueError
|
|
expect_error = r"The shapes or dtypes returned by the TensorFlow function do not match the declared output_shape_dtype"
|
|
|
|
# Call without shape polymorphism
|
|
fun_tf_rt = _maybe_tf_jit(with_jit, jax2tf.convert(fun_jax))
|
|
with self.assertRaisesRegex(expect_ex, expect_error):
|
|
fun_tf_rt(x)
|
|
|
|
# Now with shape polymorphism
|
|
if kind == "bad_dim" and with_jit:
|
|
# TODO: in jit more the error pops up later, at AddV2
|
|
expect_error = "Dimensions must be equal, but are 4 and 9 for .* AddV2"
|
|
if kind == "bad_dim" and jax.config.jax2tf_default_native_serialization:
|
|
# TODO(b/268386622): call_tf with shape polymorphism and native serialization.
|
|
expect_error = "Error compiling TensorFlow function"
|
|
fun_tf_rt = _maybe_tf_jit(with_jit,
|
|
jax2tf.convert(fun_jax, polymorphic_shapes=["b, ..."]))
|
|
with self.assertRaisesRegex(expect_ex, expect_error):
|
|
fun_tf_rt(x)
|
|
|
|
def test_inner_native_serialization(self):
|
|
# Two nested jax2tf, the inner one being with native serialization
|
|
x = np.ones((3,), dtype=np.float32)
|
|
def f_inner_jax(x):
|
|
return jnp.sin(x)
|
|
def f_outer_jax(x):
|
|
f_inner_tf = jax2tf.convert(f_inner_jax, native_serialization=True)
|
|
return jnp.cos(jax2tf.call_tf(f_inner_tf)(x))
|
|
|
|
f_outer_tf = tf.function(
|
|
jax2tf.convert(f_outer_jax, native_serialization=False),
|
|
autograph=False)
|
|
f_outer_graph = str(f_outer_tf.get_concrete_function(tf.convert_to_tensor(x)).graph.as_graph_def())
|
|
# Quick way to check that there is an XlaCallModule op, and a Cos op, but no Sin op
|
|
self.assertIn('op: "Cos"', f_outer_graph)
|
|
self.assertIn('op: "XlaCallModule"', f_outer_graph)
|
|
self.assertNotIn('op: "Sin"', f_outer_graph)
|
|
|
|
@parameterized.named_parameters(
|
|
_named_test(f2_function=f2_function, f2_saved_model=f2_saved_model,
|
|
f4_function=f4_function, f4_saved_model=f4_saved_model)
|
|
for f2_function in [True, False]
|
|
for f2_saved_model in [True, False]
|
|
for f4_function in [True, False]
|
|
for f4_saved_model in [True, False])
|
|
def test_several_round_trips(self,
|
|
f2_function=False, f2_saved_model=False,
|
|
f4_function=False, f4_saved_model=False):
|
|
if (f2_saved_model and
|
|
f4_saved_model and
|
|
not jax.config.jax2tf_default_native_serialization):
|
|
# TODO: Getting error Found invalid capture Tensor("jax2tf_vjp/jax2tf_arg_0:0", shape=(), dtype=float32) when saving custom gradients
|
|
# when saving f4, but only with non-native serialization.
|
|
raise unittest.SkipTest("TODO: error invalid capture when saving custom gradients")
|
|
x = np.array(.7, dtype=np.float32)
|
|
# f(n)(x) = 2. * x^n
|
|
def f(n):
|
|
def fn(x):
|
|
acc = np.array(2., dtype=x.dtype)
|
|
for i in range(n):
|
|
acc *= x
|
|
return acc
|
|
return fn
|
|
|
|
f2_tf = lambda x: x * jax2tf.convert(f(1))(x)
|
|
if f2_function:
|
|
f2_tf = tf.function(f2_tf, autograph=False)
|
|
if f2_saved_model:
|
|
f2_tf, _ = tf_test_util.SaveAndLoadFunction(f2_tf, input_args=[x])
|
|
|
|
self.assertAllClose(f(2)(x), f2_tf(x).numpy())
|
|
_, (g_f2_ft,) = tf_test_util.ComputeTfValueAndGrad(f2_tf, [x])
|
|
self.assertAllClose(jax.grad(f(2))(x), g_f2_ft.numpy())
|
|
|
|
f3_jax = lambda x: x * jax2tf.call_tf(f2_tf)(x)
|
|
self.assertAllClose(f(3)(x), f3_jax(x))
|
|
self.assertAllClose(f(3)(x), jax.jit(f3_jax)(x))
|
|
self.assertAllClose(jax.grad(f(3))(x), jax.grad(f3_jax)(x))
|
|
|
|
f4_tf = lambda x: x * jax2tf.convert(f3_jax)(x)
|
|
self.assertAllClose(f(4)(x), f4_tf(x).numpy())
|
|
_, (g_f4_ft,) = tf_test_util.ComputeTfValueAndGrad(f4_tf, [x])
|
|
self.assertAllClose(jax.grad(f(4))(x), g_f4_ft.numpy())
|
|
|
|
if f4_function:
|
|
f4_tf = tf.function(f4_tf, autograph=False)
|
|
if f4_saved_model:
|
|
f4_tf, _ = tf_test_util.SaveAndLoadFunction(f4_tf, input_args=[x])
|
|
self.assertAllClose(f(4)(x), f4_tf(x).numpy())
|
|
_, (g_f4_ft,) = tf_test_util.ComputeTfValueAndGrad(f4_tf, [x])
|
|
self.assertAllClose(jax.grad(f(4))(x), g_f4_ft.numpy())
|
|
|
|
@classmethod
|
|
def _walk_stablehlo_operations(cls, op, cb):
|
|
"""walk the stablehlo operation recursive with callback function."""
|
|
cb(op)
|
|
for region in op.operation.regions:
|
|
for block in region:
|
|
for op in block:
|
|
cls._walk_stablehlo_operations(op, cb)
|
|
|
|
def test_call_tf_graph(self):
|
|
const = tf.Variable(0.0, dtype=tf.float32)
|
|
|
|
@tf.function(jit_compile=True)
|
|
def tf_func_1(x):
|
|
return x * x + const
|
|
|
|
@tf.function
|
|
def tf_func_2(x, y):
|
|
return tf_func_1(x) + y
|
|
|
|
@tf.function
|
|
def tf_func_3(x, y, z):
|
|
return tf_func_2(x, y) + z, z
|
|
|
|
x = jnp.array(3.0, dtype=jnp.float32)
|
|
y = jnp.array(3.0, dtype=jnp.float32)
|
|
z = jnp.array(5.0, dtype=jnp.float32)
|
|
f_jax = jax.jit(jax2tf.call_tf(tf_func_3, call_tf_graph=False))
|
|
stablehlo_module = f_jax.lower(x, y, z).compiler_ir("stablehlo")
|
|
self.assertNotIn("stablehlo.custom_call", str(stablehlo_module))
|
|
|
|
f_jax = jax.jit(
|
|
jax2tf.call_tf(
|
|
tf_func_3,
|
|
call_tf_graph=True,
|
|
)
|
|
)
|
|
with self.assertRaisesRegex(
|
|
ValueError,
|
|
"call_tf_graph=True only support exporting by jax2tf.convert currently",
|
|
):
|
|
stablehlo_module = f_jax.lower(x, y, z).compiler_ir("stablehlo")
|
|
self.assertIn("stablehlo.custom_call", str(stablehlo_module))
|
|
|
|
called_index_list = []
|
|
|
|
def _extract_info(op):
|
|
if op.operation.name != "stablehlo.custom_call":
|
|
return
|
|
tf_backend_config = ir.DictAttr(op.attributes["tf.backend_config"])
|
|
called_index = ir.IntegerAttr(tf_backend_config["called_index"]).value
|
|
called_index_list.append(called_index)
|
|
|
|
self._walk_stablehlo_operations(stablehlo_module, _extract_info)
|
|
self.assertLen(called_index_list, 1)
|
|
|
|
@parameterized.named_parameters(
|
|
dict(
|
|
testcase_name="multiple_outputs",
|
|
tf_f=lambda x: tf.py_function(np.sin, [x], tf.float32),
|
|
output_shape_dtype=jax.ShapeDtypeStruct((10,), jnp.float32),
|
|
),
|
|
dict(
|
|
testcase_name="zero_outputs",
|
|
tf_f=lambda x: print(tf.strings.length(tf.constant("hello, world"))),
|
|
output_shape_dtype=None,
|
|
),
|
|
)
|
|
def test_call_tf_graph_non_compilable(self, tf_f, output_shape_dtype):
|
|
inputs = jnp.ones([10], dtype=jnp.float32)
|
|
called_index_list = []
|
|
xla_call_module_list = []
|
|
|
|
def _extract_info(op):
|
|
if op.operation.name != "stablehlo.custom_call":
|
|
return
|
|
tf_backend_config = ir.DictAttr(op.attributes["tf.backend_config"])
|
|
called_index = ir.IntegerAttr(tf_backend_config["called_index"]).value
|
|
called_index_list.append(called_index)
|
|
|
|
jax_f = jax2tf.call_tf(
|
|
tf_f,
|
|
call_tf_graph=True,
|
|
output_shape_dtype=output_shape_dtype,
|
|
)
|
|
|
|
# Eager mode
|
|
self.assertAllClose(tf_f(inputs), jax_f(inputs))
|
|
|
|
# Jit mode
|
|
stablehlo_module = None
|
|
with self.assertRaisesRegex(
|
|
ValueError,
|
|
"call_tf_graph=True only support exporting by jax2tf.convert currently",
|
|
):
|
|
stablehlo_module = jax.jit(jax_f).lower(inputs).compiler_ir("stablehlo")
|
|
if stablehlo_module:
|
|
self.assertIn(
|
|
"stablehlo.custom_call @tf.call_tf_function",
|
|
str(stablehlo_module),
|
|
)
|
|
self.assertIn("tf.backend_config", str(stablehlo_module))
|
|
self._walk_stablehlo_operations(stablehlo_module, _extract_info)
|
|
self.assertLen(called_index_list, 1)
|
|
|
|
# Test model exporting and reloading.
|
|
# There is no runtime support yet so it can not run.
|
|
tf_f_rt = jax2tf.convert(
|
|
jax_f,
|
|
native_serialization=True,
|
|
with_gradient=False,
|
|
)
|
|
_, restored_model = tf_test_util.SaveAndLoadFunction(
|
|
tf_f_rt, input_args=[inputs]
|
|
)
|
|
func_def = restored_model.f.concrete_functions[0]
|
|
|
|
for node_def in func_def.graph.as_graph_def().node:
|
|
if node_def.op == "XlaCallModule":
|
|
xla_call_module_list.append(node_def)
|
|
# There is only one xla_call_module in the saved model.
|
|
self.assertLen(xla_call_module_list, 1)
|
|
|
|
# Check the xla_call_module version and function_list attributes.
|
|
xla_call_module = xla_call_module_list[0]
|
|
self.assertGreaterEqual(xla_call_module.attr["version"].i, 5)
|
|
self.assertIn("function_list", str(xla_call_module.attr))
|
|
xla_call_module_list.clear()
|
|
called_index_list.clear()
|
|
|
|
# If JAX calls same tensorflow function by `jax2tf.call_tf` twice,
|
|
# it should return two different tf concrete functions.
|
|
def jax_f_2(x):
|
|
res1 = jax2tf.call_tf(
|
|
tf_f,
|
|
call_tf_graph=True,
|
|
output_shape_dtype=output_shape_dtype,
|
|
)(x)
|
|
res2 = jax2tf.call_tf(
|
|
tf_f,
|
|
call_tf_graph=True,
|
|
output_shape_dtype=output_shape_dtype,
|
|
)(x)
|
|
return res1, res2
|
|
stablehlo_module = None
|
|
with self.assertRaisesRegex(ValueError, "call_tf_graph=True only support exporting by jax2tf.convert currently"):
|
|
stablehlo_module = jax.jit(jax_f_2).lower(inputs).compiler_ir("stablehlo")
|
|
if stablehlo_module:
|
|
self._walk_stablehlo_operations(stablehlo_module, _extract_info)
|
|
xla_call_module_list.clear()
|
|
|
|
def test_b279454591(self):
|
|
"""Test case when tensorflow function returns `StatefulPartitionedCall` op."""
|
|
inputs = jnp.ones([10], dtype=jnp.float32)
|
|
|
|
# With one or more outputs, it is okay.
|
|
def tf_f(x):
|
|
y = tf.math.sin(3.0)
|
|
tf.print(y)
|
|
return x
|
|
|
|
jax_f = jax2tf.call_tf(
|
|
tf.function(tf_f),
|
|
call_tf_graph=True,
|
|
)
|
|
tf_f_rt = jax2tf.convert(
|
|
jax_f,
|
|
native_serialization=True,
|
|
with_gradient=False,
|
|
)
|
|
_, _ = tf_test_util.SaveAndLoadFunction(tf_f_rt, input_args=[inputs])
|
|
|
|
# With zero output, it return `StatefulPartitionedCall` op instead.
|
|
def tf_f_2():
|
|
y = tf.math.sin(3.0)
|
|
tf.print(y)
|
|
return
|
|
|
|
jax_f_2 = jax2tf.call_tf(tf.function(tf_f_2), call_tf_graph=True)
|
|
tf_f_rt_2 = jax2tf.convert(
|
|
jax_f_2,
|
|
native_serialization=True,
|
|
with_gradient=False,
|
|
)
|
|
_, _ = tf_test_util.SaveAndLoadFunction(tf_f_rt_2, input_args=[])
|
|
|
|
@jtu.parameterized_filterable(
|
|
kwargs=[dict(version=version) for version in [9]]
|
|
)
|
|
def test_call_tf_graph_ordered(self, *, version: int):
|
|
with config.jax_export_calling_convention_version(version):
|
|
logging.info(
|
|
"Using JAX serialization version %s",
|
|
jax.config.jax_export_calling_convention_version)
|
|
|
|
@tf.function
|
|
def tf_print(x):
|
|
tf.print(x)
|
|
|
|
call_tf_print = jax2tf.call_tf(
|
|
tf_print,
|
|
call_tf_graph=True,
|
|
ordered=True,
|
|
)
|
|
|
|
x = jnp.array(1.0, dtype=jnp.float32)
|
|
|
|
def body(i, x):
|
|
call_tf_print(x)
|
|
return x + 1
|
|
|
|
@jax.jit
|
|
def f_jax(x):
|
|
return jax.lax.fori_loop(0, 4, body, x)
|
|
|
|
num_custom_calls = 0
|
|
|
|
def _check_mlir_ops(op):
|
|
nonlocal num_custom_calls
|
|
|
|
if (
|
|
op.operation.name == "stablehlo.custom_call"
|
|
and ir.StringAttr(op.attributes["call_target_name"]).value
|
|
== "tf.call_tf_function"
|
|
):
|
|
num_custom_calls += 1
|
|
|
|
# The custom call op must have `has_token_input_output` attribute.
|
|
tf_backend_config = ir.DictAttr(op.attributes["tf.backend_config"])
|
|
self.assertTrue(
|
|
ir.BoolAttr(tf_backend_config["has_token_input_output"]).value
|
|
)
|
|
|
|
# Verify that the first argument/result of the custom call op is a token
|
|
# type. This is a calling convention defined by `has_token_input_output`.
|
|
self.assertTrue(hlo.TokenType.isinstance(op.operands[0].type))
|
|
self.assertTrue(hlo.TokenType.isinstance(op.results[0].type))
|
|
|
|
stablehlo_module = None
|
|
with self.assertRaisesRegex(
|
|
ValueError,
|
|
"call_tf_graph=True only support exporting by jax2tf.convert currently",
|
|
):
|
|
lower = f_jax.lower(x)
|
|
self.assertNotEmpty(lower._lowering.compile_args["ordered_effects"])
|
|
stablehlo_module = lower.compiler_ir("stablehlo")
|
|
if stablehlo_module:
|
|
self._walk_stablehlo_operations(stablehlo_module, _check_mlir_ops)
|
|
self.assertEqual(num_custom_calls, 1)
|
|
|
|
f_tf = jax2tf.convert(
|
|
f_jax,
|
|
native_serialization=True,
|
|
with_gradient=False,
|
|
)
|
|
_, restored_model = tf_test_util.SaveAndLoadFunction(f_tf, input_args=[x])
|
|
|
|
@jtu.parameterized_filterable(
|
|
kwargs=[dict(poly=poly, version=version)
|
|
for poly in [True, False]
|
|
for version in [9]]
|
|
)
|
|
def test_call_tf_ordered_dead_inputs(self, *, poly: bool, version: int):
|
|
with config.jax_export_calling_convention_version(version):
|
|
logging.info(
|
|
"Using JAX serialization version %s",
|
|
jax.config.jax_export_calling_convention_version)
|
|
def f_jax(x1, x_dead, x3):
|
|
return (x1, jax2tf.call_tf(lambda x: tf.math.sin(x), ordered=True,
|
|
call_tf_graph=True)(x3))
|
|
if poly:
|
|
polymorphic_shapes = ["b", None, None]
|
|
else:
|
|
polymorphic_shapes = None
|
|
f_tf = jax2tf.convert(f_jax, polymorphic_shapes=polymorphic_shapes)
|
|
x1 = np.arange(3, dtype=np.float32)
|
|
x_dead = np.arange(4, dtype=np.float32)
|
|
x3 = np.arange(5, dtype=np.float32)
|
|
self.assertAllClose(f_jax(x1, x_dead, x3),
|
|
f_tf(x1, x_dead, x3))
|
|
|
|
@jtu.parameterized_filterable(
|
|
kwargs=[dict(ordered=ordered, version=version)
|
|
for ordered in [True, False]
|
|
for version in [9]
|
|
]
|
|
)
|
|
def test_call_tf_graph_polymorphic(self, ordered: bool, version: int):
|
|
with config.jax_export_calling_convention_version(version):
|
|
logging.info(
|
|
"Using JAX serialization version %s",
|
|
jax.config.jax_export_calling_convention_version)
|
|
|
|
@tf.function(jit_compile=True, autograph=False)
|
|
@partial(jax2tf.convert,
|
|
with_gradient=False,
|
|
native_serialization=True,
|
|
polymorphic_shapes=["(b)"])
|
|
@jax.jit
|
|
def tf_f_2(x):
|
|
tf_f = lambda x: print(tf.strings.length(tf.constant("hello, world")))
|
|
jax2tf.call_tf(tf_f,
|
|
call_tf_graph=True,
|
|
ordered=ordered)(x)
|
|
return x
|
|
|
|
x = np.arange(3, dtype=np.int32)
|
|
_ = tf.function(tf_f_2, autograph=False).get_concrete_function(x)
|
|
|
|
# TODO(b/293927250): call_tf_graph=True only accept concrete_function. The
|
|
# workaround here is to set `module.call=concrete_fn.`.
|
|
@unittest.skip(
|
|
"The root cause here is because the XLACallModule.function_list attribute"
|
|
" depends on JAX call_tf lowering. The 2nd time tf.SavedModel TF tracing"
|
|
" will not trigger call_tf tracing since it was already cached. The"
|
|
" solution is to create the `CallTFContext` to make TF tracing and JAX"
|
|
" tracing work together correctly."
|
|
)
|
|
def test_call_tf_graph_save_and_load(self):
|
|
def jax_func(x):
|
|
def func_tf(x):
|
|
return tf.math.sin(x)
|
|
|
|
return jnp.cos(
|
|
jax2tf.call_tf(func_tf, output_shape_dtype=x, call_tf_graph=True)(x)
|
|
)
|
|
data_inputs = (np.array([0.5, 0.7], dtype=np.float32),)
|
|
|
|
def tf_func(the_input):
|
|
res = jax2tf.convert(jax_func, native_serialization=True)(the_input)
|
|
return tf.identity(res, name="the_result")
|
|
|
|
jit_tf_func = tf.function(
|
|
tf_func,
|
|
autograph=False,
|
|
jit_compile=True,
|
|
)
|
|
# The next line is necessary to reproduce this issue. It trigger TF
|
|
# ConcreteFunction tracing. Otherwise, you will fail with another error
|
|
# `Found zero restored functions for caller function`.
|
|
_ = jit_tf_func.get_concrete_function(*data_inputs)
|
|
module = tf.Module()
|
|
module.call = jit_tf_func # Switching to concrete_function works.
|
|
root_dir = self.create_tempdir()
|
|
saved_model_dir = os.path.join(root_dir, "saved_model")
|
|
tf.saved_model.save(
|
|
module,
|
|
saved_model_dir,
|
|
options=tf.saved_model.SaveOptions(experimental_custom_gradients=False),
|
|
)
|
|
loaded_model = tf.saved_model.load(saved_model_dir)
|
|
res = loaded_model.call(*data_inputs)
|
|
self.assertAllClose(jax_func(*data_inputs), res)
|
|
|
|
|
|
if __name__ == "__main__":
|
|
absltest.main(testLoader=jtu.JaxTestLoader())
|