mirror of
https://github.com/ROCm/jax.git
synced 2025-04-15 19:36:06 +00:00
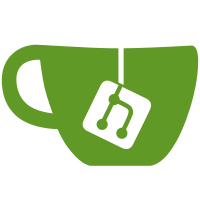
These docstrings do not make the tests any more clear and typically just duplicate the test module name. PiperOrigin-RevId: 737611977
787 lines
26 KiB
Python
787 lines
26 KiB
Python
# Copyright 2023 The JAX Authors.
|
|
#
|
|
# Licensed under the Apache License, Version 2.0 (the "License");
|
|
# you may not use this file except in compliance with the License.
|
|
# You may obtain a copy of the License at
|
|
#
|
|
# https://www.apache.org/licenses/LICENSE-2.0
|
|
#
|
|
# Unless required by applicable law or agreed to in writing, software
|
|
# distributed under the License is distributed on an "AS IS" BASIS,
|
|
# WITHOUT WARRANTIES OR CONDITIONS OF ANY KIND, either express or implied.
|
|
# See the License for the specific language governing permissions and
|
|
# limitations under the License.
|
|
|
|
from __future__ import annotations
|
|
|
|
from collections.abc import Callable
|
|
import dataclasses
|
|
import functools
|
|
from typing import Any, TypeVar
|
|
import unittest
|
|
|
|
from absl.testing import absltest
|
|
from absl.testing import parameterized
|
|
import jax
|
|
from jax import random
|
|
from jax._src import test_util as jtu
|
|
from jax.experimental.pallas.ops.tpu.splash_attention import splash_attention_kernel as splash
|
|
from jax.experimental.pallas.ops.tpu.splash_attention import splash_attention_mask as mask_lib
|
|
from jax.experimental.pallas.ops.tpu.splash_attention.splash_attention_mask_info import process_mask
|
|
import jax.numpy as jnp
|
|
import numpy as np
|
|
|
|
|
|
try:
|
|
import hypothesis as hp
|
|
import hypothesis.strategies as hps
|
|
except (ModuleNotFoundError, ImportError):
|
|
raise unittest.SkipTest("these tests require hypothesis")
|
|
|
|
jax.config.parse_flags_with_absl()
|
|
jtu.setup_hypothesis(max_examples=5)
|
|
|
|
partial = functools.partial
|
|
Draw = TypeVar("Draw", bound=Callable[[hps.SearchStrategy[Any]], Any])
|
|
|
|
|
|
@hps.composite
|
|
def segment_ids_strategy(draw, seq_len: int) -> splash.SegmentIds:
|
|
boundaries = hps.sets(hps.integers(1, seq_len - 1), min_size=1, max_size=4)
|
|
bounds = sorted(draw(boundaries))
|
|
ids_array = np.empty((seq_len,), dtype=np.int32)
|
|
for i, (start, end) in enumerate(zip((0, *bounds), (*bounds, seq_len))):
|
|
# Not sure why, but short segments can trip things up
|
|
if end - start < 2:
|
|
end = start + 2
|
|
ids_array[start:end] = i
|
|
return splash.SegmentIds(ids_array, ids_array)
|
|
|
|
|
|
def seed_strategy() -> hps.SearchStrategy[int]:
|
|
return hps.integers(min_value=0, max_value=4)
|
|
|
|
|
|
class Mask:
|
|
|
|
def get_mask(self) -> mask_lib.Mask:
|
|
raise NotImplementedError()
|
|
|
|
|
|
def full_mask_strategy(
|
|
q_seq_len: int, kv_seq_len: int
|
|
) -> hps.SearchStrategy[Mask]:
|
|
return hps.just(FullMask(q_seq_len, kv_seq_len))
|
|
|
|
|
|
@dataclasses.dataclass
|
|
class FullMask(Mask):
|
|
q_seq_len: int
|
|
kv_seq_len: int
|
|
|
|
def get_mask(self) -> mask_lib.Mask:
|
|
return mask_lib.FullMask((self.q_seq_len, self.kv_seq_len))
|
|
|
|
|
|
def causal_mask_strategy(
|
|
q_seq_len: int, kv_seq_len: int
|
|
) -> hps.SearchStrategy[Mask]:
|
|
return hps.just(CausalMask(q_seq_len, kv_seq_len))
|
|
|
|
|
|
@dataclasses.dataclass
|
|
class CausalMask(Mask):
|
|
q_seq_len: int
|
|
kv_seq_len: int
|
|
|
|
def get_mask(self) -> mask_lib.Mask:
|
|
return mask_lib.CausalMask((self.q_seq_len, self.kv_seq_len))
|
|
|
|
|
|
@dataclasses.dataclass
|
|
class LocalAttentionMask(Mask):
|
|
seq_len: int
|
|
left: int | None
|
|
right: int | None
|
|
offset: int
|
|
|
|
def get_mask(self) -> mask_lib.Mask:
|
|
mask = mask_lib.LocalMask(
|
|
(self.seq_len, self.seq_len),
|
|
(self.left, self.right),
|
|
offset=self.offset,
|
|
)
|
|
# Make sure that no row is full of zeros as this is leads to undefined
|
|
# softmax.
|
|
diagonal = mask_lib.NumpyMask(np.identity(self.seq_len, dtype=np.bool_))
|
|
return mask | diagonal
|
|
|
|
|
|
@hps.composite
|
|
def local_attention_mask_strategy(draw: Draw, seq_len: int) -> Mask:
|
|
left_window = draw(
|
|
hps.one_of(hps.none(), hps.integers(min_value=0, max_value=seq_len))
|
|
)
|
|
right_window = draw(
|
|
hps.one_of(hps.none(), hps.integers(min_value=0, max_value=seq_len))
|
|
)
|
|
offset = draw(hps.integers(min_value=-seq_len, max_value=seq_len - 1))
|
|
return LocalAttentionMask(seq_len, left_window, right_window, offset=offset)
|
|
|
|
|
|
@dataclasses.dataclass
|
|
class RandomMask(Mask):
|
|
q_seq_len: int
|
|
kv_seq_len: int
|
|
sparsity: float
|
|
seed: int
|
|
|
|
def get_mask(self) -> mask_lib.Mask:
|
|
mask = mask_lib.make_random_mask(
|
|
(self.q_seq_len, self.kv_seq_len), self.sparsity, self.seed
|
|
)
|
|
# Make sure that no row is full of zeros as this is leads to undefined
|
|
# softmax.
|
|
mask[:, 0] = True
|
|
|
|
return mask_lib.NumpyMask(mask)
|
|
|
|
|
|
@hps.composite
|
|
def random_mask_strategy(draw: Draw, q_seq_len: int, kv_seq_len: int) -> Mask:
|
|
seed = draw(hps.integers(min_value=0, max_value=2**32 - 1))
|
|
sparsity = draw(hps.floats(min_value=0.0, max_value=0.5))
|
|
return RandomMask(q_seq_len, kv_seq_len, sparsity, seed)
|
|
|
|
|
|
@dataclasses.dataclass
|
|
class ComposeMask(Mask):
|
|
left: Mask
|
|
right: Mask
|
|
op: Callable[[mask_lib.Mask, mask_lib.Mask], mask_lib.Mask]
|
|
|
|
def get_mask(self) -> mask_lib.Mask:
|
|
return self.op(self.left.get_mask(), self.right.get_mask())
|
|
|
|
|
|
@hps.composite
|
|
def compose_mask_strategy(draw: Draw, q_seq_len: int, kv_seq_len: int) -> Mask:
|
|
mask1 = draw(mask_strategy(q_seq_len, kv_seq_len))
|
|
mask2 = draw(mask_strategy(q_seq_len, kv_seq_len))
|
|
op = draw(
|
|
hps.one_of(hps.just(mask_lib.LogicalOr), hps.just(mask_lib.LogicalAnd))
|
|
)
|
|
return ComposeMask(mask1, mask2, op)
|
|
|
|
|
|
@hps.composite
|
|
def mask_strategy(draw: Draw, q_seq_len: int, kv_seq_len: int) -> Mask:
|
|
oneof = [
|
|
causal_mask_strategy(q_seq_len, kv_seq_len),
|
|
full_mask_strategy(q_seq_len, kv_seq_len),
|
|
random_mask_strategy(q_seq_len, kv_seq_len),
|
|
# TODO(amagni) Composing masks creates masks that produce minor numerical
|
|
# differences. We should investigate this in the future.
|
|
# compose_mask_strategy(q_seq_len, kv_seq_len),
|
|
]
|
|
|
|
if q_seq_len == kv_seq_len:
|
|
oneof.append(local_attention_mask_strategy(q_seq_len))
|
|
|
|
return draw(hps.one_of(oneof))
|
|
|
|
|
|
@hps.composite
|
|
def mha_mask_strategy(
|
|
draw: Draw, q_seq_len: int, kv_seq_len: int, num_heads: int
|
|
) -> np.ndarray:
|
|
return np.stack(
|
|
[draw(mask_strategy(q_seq_len, kv_seq_len)) for _ in range(num_heads)]
|
|
)
|
|
|
|
|
|
@hps.composite
|
|
def sequence_length_strategy(draw: Draw) -> tuple[int, int]:
|
|
q_seq_len = draw(hps.sampled_from([1024, 2048, 4096]))
|
|
kv_seq_len = draw(hps.sampled_from([1024, 2048, 4096]))
|
|
return q_seq_len, kv_seq_len
|
|
|
|
|
|
@hps.composite
|
|
def attention_strategy(draw: Draw) -> tuple[int, int, int, np.dtype]:
|
|
q_seq_len, kv_seq_len = draw(sequence_length_strategy())
|
|
head_dim_qk, head_dim_v = draw(
|
|
hps.sampled_from([(128, 128), (256, 256), (192, 128)])
|
|
)
|
|
if q_seq_len >= 4096 and kv_seq_len >= 4096:
|
|
# Do not draw bfloat16 on longer sequence lengths, as this increases
|
|
# the risk of numerical precision errors causing false positives in
|
|
# tests.
|
|
dtype = np.dtype("float32")
|
|
else:
|
|
dtype = draw(hps.sampled_from([np.dtype("float32"), np.dtype(jnp.bfloat16)]))
|
|
return q_seq_len, kv_seq_len, head_dim_qk, head_dim_v, dtype
|
|
|
|
|
|
@hps.composite
|
|
def mha_strategy(draw: Draw) -> tuple[int, int, int, int, int, np.dtype]:
|
|
q_seq_len, kv_seq_len, head_dim_qk, head_dim_v, dtype = draw(
|
|
attention_strategy()
|
|
)
|
|
num_q_heads, num_kv_heads = draw(
|
|
hps.sampled_from([(1, 1), (2, 2), (4, 1), (8, 4), (6, 2)])
|
|
)
|
|
return (
|
|
q_seq_len,
|
|
kv_seq_len,
|
|
num_q_heads,
|
|
num_kv_heads,
|
|
head_dim_qk,
|
|
head_dim_v,
|
|
dtype,
|
|
)
|
|
|
|
|
|
@hps.composite
|
|
def block_sizes_strategy(
|
|
draw: Draw,
|
|
q_seq_len: int,
|
|
kv_seq_len: int,
|
|
include_bwd_blocks: bool = False,
|
|
use_fused_bwd_kernel: bool = False,
|
|
) -> splash.BlockSizes:
|
|
all_block_shapes = [128, 256, 512]
|
|
q_layout = draw(hps.sampled_from(splash.QKVLayout))
|
|
k_layout = draw(hps.sampled_from(splash.QKVLayout))
|
|
v_layout = draw(hps.sampled_from(splash.QKVLayout))
|
|
layouts = dict(q_layout=q_layout, k_layout=k_layout, v_layout=v_layout)
|
|
q_valid_block_shapes = [bs for bs in all_block_shapes if bs <= q_seq_len]
|
|
kv_valid_block_shapes = [bs for bs in all_block_shapes if bs <= kv_seq_len]
|
|
bq, bkv = (
|
|
draw(hps.sampled_from(q_valid_block_shapes)),
|
|
draw(hps.sampled_from(kv_valid_block_shapes)),
|
|
)
|
|
bkv_compute = draw(
|
|
hps.sampled_from([None, *[b for b in kv_valid_block_shapes if b <= bkv]])
|
|
)
|
|
if not include_bwd_blocks:
|
|
return splash.BlockSizes(
|
|
block_q=bq, block_kv=bkv, block_kv_compute=bkv_compute, **layouts
|
|
)
|
|
all_block_shapes = [128, 256]
|
|
q_valid_block_shapes = [bs for bs in all_block_shapes if bs <= q_seq_len]
|
|
kv_valid_block_shapes = [bs for bs in all_block_shapes if bs <= kv_seq_len]
|
|
bq_dkv, bkv_dkv = (
|
|
draw(hps.sampled_from(q_valid_block_shapes)),
|
|
draw(hps.sampled_from(kv_valid_block_shapes)),
|
|
)
|
|
if use_fused_bwd_kernel:
|
|
bq_dq, bkv_dq = None, None
|
|
else:
|
|
bq_dq = draw(hps.sampled_from(q_valid_block_shapes))
|
|
bkv_dq = draw(hps.sampled_from(kv_valid_block_shapes))
|
|
block_kv_dkv_compute = draw(
|
|
hps.sampled_from(
|
|
[None, *[b for b in kv_valid_block_shapes if b <= bkv_dkv]]
|
|
)
|
|
)
|
|
return splash.BlockSizes(
|
|
block_q=bq,
|
|
block_kv=bkv,
|
|
block_kv_compute=bkv_compute,
|
|
block_q_dkv=bq_dkv,
|
|
block_kv_dkv=bkv_dkv,
|
|
block_kv_dkv_compute=block_kv_dkv_compute,
|
|
block_q_dq=bq_dq,
|
|
block_kv_dq=bkv_dq,
|
|
use_fused_bwd_kernel=use_fused_bwd_kernel,
|
|
**layouts,
|
|
)
|
|
|
|
|
|
def attn_logits_soft_cap_strategy() -> hps.SearchStrategy[float | None]:
|
|
return hps.one_of(hps.just(None), hps.floats(min_value=1.0, max_value=50.0))
|
|
|
|
|
|
def to_dynamic_mask(mask: mask_lib.MultiHeadMask) -> jax.Array:
|
|
q_seq_len, kv_seq_len = mask.masks[0].shape
|
|
full_mask_slice = (slice(0, q_seq_len), slice(0, kv_seq_len))
|
|
dynamic_mask = jnp.stack([m[full_mask_slice] for m in mask.masks], axis=0)
|
|
|
|
return dynamic_mask
|
|
|
|
|
|
@jtu.with_config(jax_traceback_filtering="off")
|
|
class PallasBaseTest(jtu.JaxTestCase):
|
|
INTERPRET = False
|
|
|
|
def setUp(self):
|
|
if not self.INTERPRET:
|
|
if not jtu.test_device_matches(["tpu"]):
|
|
self.skipTest("Only interpret mode supported on non-TPU")
|
|
# TODO(b/327487669): selectively re-enable tests that works on TPU v3.
|
|
if not jtu.is_device_tpu_at_least(4):
|
|
self.skipTest("Not supported on TPU generations <= 3")
|
|
if jtu.test_device_matches(["cpu"]) and jax.config.x64_enabled:
|
|
self.skipTest("On CPU the test works only in 32-bit")
|
|
|
|
super().setUp()
|
|
|
|
def _assert_allclose(self, x, y, **kwargs):
|
|
if x.dtype == np.dtype(jnp.bfloat16):
|
|
x = x.astype(np.float32)
|
|
if y.dtype == np.dtype(jnp.bfloat16):
|
|
y = y.astype(np.float32)
|
|
self.assertEqual(x.dtype, y.dtype)
|
|
self.assertTupleEqual(x.shape, y.shape)
|
|
np.testing.assert_allclose(x, y, **kwargs)
|
|
|
|
|
|
class SplashAttentionTest(PallasBaseTest):
|
|
@parameterized.product(
|
|
is_mqa=(False, True),
|
|
is_segmented=(False, True),
|
|
is_dynamic_mask=(False, True),
|
|
)
|
|
@hp.given(hps.data())
|
|
def test_splash_attention(self, is_mqa, is_segmented, is_dynamic_mask, data):
|
|
seed = data.draw(seed_strategy())
|
|
key = random.key(seed)
|
|
k1, k2, k3 = random.split(key, 3)
|
|
|
|
(
|
|
q_seq_len,
|
|
kv_seq_len,
|
|
num_q_heads,
|
|
num_kv_heads,
|
|
head_dim_qk,
|
|
head_dim_v,
|
|
dtype,
|
|
) = data.draw(mha_strategy())
|
|
|
|
# Avoid segment ids for rectangular matrices, as its hard to enforce
|
|
# valid masks (non-0 rows).
|
|
hp.assume(q_seq_len == kv_seq_len or not is_segmented)
|
|
|
|
q = random.uniform(k1, (num_q_heads, q_seq_len, head_dim_qk), dtype=dtype)
|
|
if is_mqa:
|
|
k = random.uniform(k2, (kv_seq_len, head_dim_qk), dtype=dtype)
|
|
v = random.uniform(k3, (kv_seq_len, head_dim_v), dtype=dtype)
|
|
else:
|
|
k = random.uniform(
|
|
k2, (num_kv_heads, kv_seq_len, head_dim_qk), dtype=dtype
|
|
)
|
|
v = random.uniform(
|
|
k3, (num_kv_heads, kv_seq_len, head_dim_v), dtype=dtype
|
|
)
|
|
|
|
segment_ids = None
|
|
if is_segmented:
|
|
assert q_seq_len == kv_seq_len
|
|
segment_ids = data.draw(segment_ids_strategy(q_seq_len))
|
|
|
|
attn_logits_soft_cap = data.draw(attn_logits_soft_cap_strategy())
|
|
masks = data.draw(mha_mask_strategy(q_seq_len, kv_seq_len, num_q_heads))
|
|
mask = mask_lib.MultiHeadMask(tuple(m.get_mask() for m in masks))
|
|
if is_dynamic_mask:
|
|
mask = to_dynamic_mask(mask)
|
|
block_sizes = data.draw(block_sizes_strategy(q_seq_len, kv_seq_len))
|
|
|
|
if is_mqa:
|
|
attn_ref = splash.make_masked_mqa_reference(mask)
|
|
attn = splash.make_splash_mqa_single_device(
|
|
mask,
|
|
block_sizes=block_sizes,
|
|
attn_logits_soft_cap=attn_logits_soft_cap,
|
|
interpret=self.INTERPRET,
|
|
)
|
|
else:
|
|
attn_ref = splash.make_masked_mha_reference(mask)
|
|
attn = splash.make_splash_mha_single_device(
|
|
mask,
|
|
block_sizes=block_sizes,
|
|
attn_logits_soft_cap=attn_logits_soft_cap,
|
|
interpret=self.INTERPRET,
|
|
)
|
|
o = attn(q, k, v, segment_ids)
|
|
o_ref = attn_ref(
|
|
q.astype(np.float32),
|
|
k.astype(np.float32),
|
|
v.astype(np.float32),
|
|
segment_ids,
|
|
attn_logits_soft_cap=attn_logits_soft_cap,
|
|
)
|
|
self._assert_allclose(o, o_ref, atol=3e-3, rtol=3e-3)
|
|
|
|
@parameterized.product(
|
|
is_mqa=(False, True),
|
|
is_segmented=(False, True),
|
|
is_dynamic_mask=(False, True),
|
|
)
|
|
@hp.given(hps.data())
|
|
def test_splash_attention_fwd(
|
|
self, is_mqa, is_segmented, is_dynamic_mask, data
|
|
):
|
|
seed = data.draw(seed_strategy())
|
|
key = random.key(seed)
|
|
k1, k2, k3 = random.split(key, 3)
|
|
|
|
(
|
|
q_seq_len,
|
|
kv_seq_len,
|
|
num_q_heads,
|
|
num_kv_heads,
|
|
head_dim_qk,
|
|
head_dim_v,
|
|
dtype,
|
|
) = data.draw(mha_strategy())
|
|
|
|
# Avoid segment ids for rectangular matrices, as its hard to enforce
|
|
# valid masks (non-0 rows).
|
|
hp.assume(q_seq_len == kv_seq_len or not is_segmented)
|
|
|
|
q = random.uniform(k1, (num_q_heads, q_seq_len, head_dim_qk), dtype=dtype)
|
|
if is_mqa:
|
|
k = random.uniform(k2, (kv_seq_len, head_dim_qk), dtype=dtype)
|
|
v = random.uniform(k3, (kv_seq_len, head_dim_v), dtype=dtype)
|
|
else:
|
|
k = random.uniform(
|
|
k2, (num_kv_heads, kv_seq_len, head_dim_qk), dtype=dtype
|
|
)
|
|
v = random.uniform(
|
|
k3, (num_kv_heads, kv_seq_len, head_dim_v), dtype=dtype
|
|
)
|
|
|
|
segment_ids = None
|
|
if is_segmented:
|
|
assert q_seq_len == kv_seq_len
|
|
segment_ids = data.draw(segment_ids_strategy(q_seq_len))
|
|
attn_logits_soft_cap = data.draw(attn_logits_soft_cap_strategy())
|
|
masks = data.draw(mha_mask_strategy(q_seq_len, kv_seq_len, num_q_heads))
|
|
mask = mask_lib.MultiHeadMask(tuple(m.get_mask() for m in masks))
|
|
if is_dynamic_mask:
|
|
mask = to_dynamic_mask(mask)
|
|
block_sizes = data.draw(block_sizes_strategy(q_seq_len, kv_seq_len))
|
|
if is_mqa:
|
|
attn_ref = splash.make_masked_mqa_reference(mask)
|
|
attn = splash.make_splash_mqa_single_device(
|
|
mask,
|
|
block_sizes=block_sizes,
|
|
save_residuals=True,
|
|
attn_logits_soft_cap=attn_logits_soft_cap,
|
|
interpret=self.INTERPRET,
|
|
)
|
|
else:
|
|
attn_ref = splash.make_masked_mha_reference(mask)
|
|
attn = splash.make_splash_mha_single_device(
|
|
mask,
|
|
block_sizes=block_sizes,
|
|
save_residuals=True,
|
|
attn_logits_soft_cap=attn_logits_soft_cap,
|
|
interpret=self.INTERPRET,
|
|
)
|
|
attn_ref = partial(
|
|
attn_ref,
|
|
save_residuals=True,
|
|
attn_logits_soft_cap=attn_logits_soft_cap,
|
|
)
|
|
o, (logsumexp,) = attn(q, k, v, segment_ids)
|
|
o_ref, (logsumexp_ref,) = attn_ref(
|
|
q.astype(jnp.float32),
|
|
k.astype(jnp.float32),
|
|
v.astype(jnp.float32),
|
|
segment_ids,
|
|
)
|
|
self._assert_allclose(o, o_ref, atol=3e-3, rtol=3e-3)
|
|
self._assert_allclose(logsumexp, logsumexp_ref, atol=1e-3, rtol=1e-3)
|
|
|
|
@parameterized.product(
|
|
is_segmented=(False, True),
|
|
)
|
|
@hp.given(hps.data())
|
|
def test_splash_attention_custom_bwd(self, is_segmented, data):
|
|
seed = data.draw(seed_strategy(), label="seed")
|
|
key = random.key(1 + seed)
|
|
k1, k2, k3, k4 = random.split(key, 4)
|
|
|
|
q_seq_len, kv_seq_len, head_dim_qk, head_dim_v, dtype = data.draw(
|
|
attention_strategy()
|
|
)
|
|
|
|
# Avoid segment ids for rectangular matrices, as it's hard to enforce
|
|
# valid masks (non-0 rows).
|
|
hp.assume(q_seq_len == kv_seq_len or not is_segmented)
|
|
|
|
q = random.uniform(k1, (q_seq_len, head_dim_qk), dtype=dtype)
|
|
k = random.uniform(k2, (kv_seq_len, head_dim_qk), dtype=dtype)
|
|
v = random.uniform(k3, (kv_seq_len, head_dim_v), dtype=dtype)
|
|
segment_ids = None
|
|
if is_segmented:
|
|
assert q_seq_len == kv_seq_len
|
|
segment_ids = data.draw(segment_ids_strategy(q_seq_len))
|
|
masks = data.draw(mha_mask_strategy(q_seq_len, kv_seq_len, 1))
|
|
mask = jnp.array(masks[0].get_mask()[:, :])
|
|
attn_logits_soft_cap = data.draw(attn_logits_soft_cap_strategy(),
|
|
label="logit_cap")
|
|
attn_ref = partial(splash.attention_reference, mask,
|
|
attn_logits_soft_cap=attn_logits_soft_cap)
|
|
attn_custom = partial(splash.attention_reference_custom, mask,
|
|
attn_logits_soft_cap=attn_logits_soft_cap)
|
|
attn_custom_vanilla = partial(splash.attention_reference_custom, mask,
|
|
custom_type="vanilla",
|
|
attn_logits_soft_cap=attn_logits_soft_cap)
|
|
o_ref, attn_vjp_ref = jax.vjp(attn_ref, q, k, v, segment_ids)
|
|
q32, k32, v32 = jax.tree.map(lambda x: x.astype(jnp.float32),
|
|
(q, k, v))
|
|
o_custom = attn_custom(q32, k32, v32, segment_ids)
|
|
_, attn_vjp = jax.vjp(attn_custom, q32, k32, v32, segment_ids)
|
|
_, attn_vanilla_vjp = jax.vjp(attn_custom_vanilla, q32, k32, v32,
|
|
segment_ids)
|
|
do = random.uniform(k4, o_custom.shape, dtype=o_custom.dtype) / 10.
|
|
# These should be identical
|
|
self._assert_allclose(o_custom, o_ref, atol=1e-5, rtol=1e-5)
|
|
dq, dk, dv, _ = attn_vjp(do)
|
|
dq_vanilla, dk_vanilla, dv_vanilla, _ = attn_vanilla_vjp(do)
|
|
dq_ref, dk_ref, dv_ref, _ = attn_vjp_ref(do)
|
|
# These will be different because of reassociation
|
|
if dtype == jnp.bfloat16:
|
|
atols = {"dv": 4e-3, "dq": 0.05, "dk": 0.05}
|
|
atols_v = {"dv": 8e-3, "dq": 2e-2, "dk": 2e-2}
|
|
rtols = {"dv": 4e-3, "dq": 0.05, "dk": 0.05}
|
|
rtols_v = {"dv": 8e-3, "dq": 2e-2, "dk": 2e-2}
|
|
if jtu.is_device_tpu(version=5):
|
|
atols["dk"] = 0.065
|
|
elif dtype == jnp.float32:
|
|
atols = {"dv": 3e-3, "dq": 0.05, "dk": 0.05}
|
|
atols_v = {"dv": 4e-4, "dq": 2e-3, "dk": 3e-3}
|
|
rtols = {"dv": 3e-3, "dq": 0.05, "dk": 0.05}
|
|
rtols_v = {"dv": 8e-3, "dq": 5e-4, "dk": 5e-4}
|
|
if jtu.is_device_tpu(version=4):
|
|
atols["dk"] = 0.09
|
|
else:
|
|
raise NotImplementedError
|
|
self._assert_allclose(
|
|
dv_vanilla, dv_ref, atol=atols_v["dv"], rtol=rtols_v["dv"]
|
|
)
|
|
self._assert_allclose(dv, dv_ref, atol=atols["dv"], rtol=rtols["dv"])
|
|
self._assert_allclose(
|
|
dq_vanilla, dq_ref, atol=atols_v["dq"], rtol=rtols_v["dq"]
|
|
)
|
|
self._assert_allclose(dq, dq_ref, atol=atols["dq"], rtol=rtols["dq"])
|
|
self._assert_allclose(
|
|
dk_vanilla, dk_ref, atol=atols_v["dk"], rtol=rtols_v["dk"]
|
|
)
|
|
self._assert_allclose(dk, dk_ref, atol=atols["dk"], rtol=rtols["dk"])
|
|
|
|
@parameterized.product(
|
|
is_mqa=(False, True),
|
|
is_segmented=(False, True),
|
|
downcast_smem_data=(False, True),
|
|
use_fused_bwd_kernel=(False, True),
|
|
use_dynamic_mask=(False, True),
|
|
)
|
|
@hp.given(hps.data())
|
|
def test_splash_attention_bwd(
|
|
self,
|
|
is_mqa,
|
|
is_segmented,
|
|
downcast_smem_data,
|
|
use_fused_bwd_kernel,
|
|
use_dynamic_mask,
|
|
data,
|
|
):
|
|
seed = data.draw(seed_strategy())
|
|
key = random.key(seed)
|
|
k1, k2, k3, k4 = random.split(key, 4)
|
|
|
|
(
|
|
q_seq_len,
|
|
kv_seq_len,
|
|
num_q_heads,
|
|
num_kv_heads,
|
|
head_dim_qk,
|
|
head_dim_v,
|
|
dtype,
|
|
) = data.draw(mha_strategy())
|
|
|
|
# Avoid segment ids for rectangular matrices, as it's hard to enforce
|
|
# valid masks (non-0 rows).
|
|
hp.assume(q_seq_len == kv_seq_len or not is_segmented)
|
|
|
|
q = random.uniform(k1, (num_q_heads, q_seq_len, head_dim_qk), dtype=dtype)
|
|
if is_mqa:
|
|
k = random.uniform(k2, (kv_seq_len, head_dim_qk), dtype=dtype)
|
|
v = random.uniform(k3, (kv_seq_len, head_dim_v), dtype=dtype)
|
|
else:
|
|
k = random.uniform(
|
|
k2, (num_kv_heads, kv_seq_len, head_dim_qk), dtype=dtype
|
|
)
|
|
v = random.uniform(
|
|
k3, (num_kv_heads, kv_seq_len, head_dim_v), dtype=dtype
|
|
)
|
|
|
|
segment_ids = None
|
|
if is_segmented:
|
|
assert q_seq_len == kv_seq_len
|
|
segment_ids = data.draw(segment_ids_strategy(q_seq_len))
|
|
attn_logits_soft_cap = data.draw(attn_logits_soft_cap_strategy())
|
|
masks = data.draw(mha_mask_strategy(q_seq_len, kv_seq_len, num_q_heads))
|
|
mask = mask_lib.MultiHeadMask(tuple(m.get_mask() for m in masks))
|
|
if use_dynamic_mask:
|
|
mask = to_dynamic_mask(mask)
|
|
block_sizes = data.draw(
|
|
block_sizes_strategy(q_seq_len, kv_seq_len, include_bwd_blocks=True,
|
|
use_fused_bwd_kernel=use_fused_bwd_kernel)
|
|
)
|
|
if is_mqa:
|
|
attn_ref = splash.make_masked_mqa_reference(mask, backward_impl="custom")
|
|
attn = splash.make_splash_mqa_single_device(
|
|
mask,
|
|
block_sizes=block_sizes,
|
|
downcast_smem_data=downcast_smem_data,
|
|
attn_logits_soft_cap=attn_logits_soft_cap,
|
|
interpret=self.INTERPRET,
|
|
)
|
|
else:
|
|
attn_ref = splash.make_masked_mha_reference(mask, backward_impl="custom")
|
|
attn = splash.make_splash_mha_single_device(
|
|
mask,
|
|
block_sizes=block_sizes,
|
|
downcast_smem_data=downcast_smem_data,
|
|
attn_logits_soft_cap=attn_logits_soft_cap,
|
|
interpret=self.INTERPRET,
|
|
)
|
|
o, attn_vjp = jax.vjp(attn, q, k, v, segment_ids)
|
|
q32, k32, v32 = jax.tree.map(
|
|
lambda x: x.astype(jnp.float32), (q, k, v)
|
|
)
|
|
o_ref, (logsumexp,) = attn_ref(
|
|
q32,
|
|
k32,
|
|
v32,
|
|
segment_ids,
|
|
save_residuals=True,
|
|
attn_logits_soft_cap=attn_logits_soft_cap,
|
|
)
|
|
self._assert_allclose(o, o_ref, atol=3e-3, rtol=3e-3)
|
|
|
|
do = random.uniform(k4, o.shape, dtype=o.dtype)
|
|
dq, dk, dv, _ = attn_vjp(do)
|
|
def bwd(
|
|
mask, q, k, v, segment_ids, o, logsumexp, do
|
|
) -> tuple[jax.Array, jax.Array, jax.Array]:
|
|
_, dq, dk, dv, _ = splash._attention_reference_custom_bwd(
|
|
splash.DEFAULT_MASK_VALUE,
|
|
False,
|
|
"flash",
|
|
attn_logits_soft_cap,
|
|
(mask, q, k, v, segment_ids, o, logsumexp),
|
|
do,
|
|
)
|
|
return dq, dk, dv
|
|
|
|
is_grouped = not is_mqa and num_kv_heads < num_q_heads
|
|
assert num_q_heads % num_kv_heads == 0
|
|
head_multiplier = num_q_heads // num_kv_heads
|
|
if is_mqa:
|
|
bwd = jax.vmap(bwd, in_axes=(0, 0, None, None, None, 0, 0, 0))
|
|
else:
|
|
bwd = jax.vmap(bwd, in_axes=(0, 0, 0, 0, None, 0, 0, 0))
|
|
# Interleave the KV heads to match the corresponding Q heads.
|
|
if is_grouped:
|
|
k32 = jnp.repeat(k32, head_multiplier, axis=0)
|
|
v32 = jnp.repeat(v32, head_multiplier, axis=0)
|
|
|
|
dq_ref, dk_ref, dv_ref = bwd(
|
|
mask[:, :, :],
|
|
q32,
|
|
k32,
|
|
v32,
|
|
segment_ids,
|
|
o.astype(jnp.float32),
|
|
logsumexp,
|
|
do.astype(jnp.float32),
|
|
)
|
|
if is_mqa:
|
|
dk_ref, dv_ref = dk_ref.sum(axis=0), dv_ref.sum(axis=0)
|
|
elif is_grouped:
|
|
# Perform the sum reduction across the head_multiplier dimension only.
|
|
# So that the output still has KV heads.
|
|
dk_ref = dk_ref.reshape(num_kv_heads, head_multiplier, *dk_ref.shape[1:])
|
|
dv_ref = dv_ref.reshape(num_kv_heads, head_multiplier, *dv_ref.shape[1:])
|
|
|
|
dk_ref, dv_ref = dk_ref.sum(axis=1), dv_ref.sum(axis=1)
|
|
|
|
self._assert_allclose(dv, dv_ref, atol=2e-2, rtol=3e-2)
|
|
self._assert_allclose(dq, dq_ref, atol=2e-2, rtol=3e-2)
|
|
self._assert_allclose(dk, dk_ref, atol=2e-2, rtol=3e-2)
|
|
|
|
def test_grid_shrinking(self):
|
|
"""Make sure that grid shrinking does not change the attention output."""
|
|
|
|
class IdentityMask(mask_lib._ComputableMask):
|
|
"""Identity mask that is guaranteed to trigger grid shrinking."""
|
|
|
|
def __init__(
|
|
self,
|
|
shape: tuple[int, int],
|
|
shard_count: int = 1,
|
|
):
|
|
def identity_mask_function(q_ids, kv_ids):
|
|
return q_ids == kv_ids
|
|
|
|
super().__init__(
|
|
shape=shape,
|
|
mask_function=identity_mask_function,
|
|
shard_count=shard_count,
|
|
)
|
|
|
|
def __eq__(self, other: object):
|
|
if not isinstance(other, type(self)):
|
|
return NotImplemented
|
|
|
|
return self.shape == other.shape and np.array_equal(
|
|
self.q_sequence, other.q_sequence
|
|
)
|
|
|
|
def __hash__(self):
|
|
return hash((
|
|
type(self),
|
|
self.shape,
|
|
self.q_sequence.tobytes() if self.q_sequence is not None else None,
|
|
))
|
|
|
|
# Use a sequence length greater than the default block size to trigger
|
|
# the grid shrinking logic.
|
|
seq_len = 256
|
|
head_dim = 128
|
|
key = random.key(42)
|
|
k1, k2, k3 = random.split(key, 3)
|
|
q = random.uniform(k1, (1, seq_len, head_dim), dtype=jnp.float32)
|
|
k = random.uniform(k2, (seq_len, head_dim), dtype=jnp.float32)
|
|
v = random.uniform(k3, (seq_len, head_dim), dtype=jnp.float32)
|
|
|
|
identity_mask = mask_lib.MultiHeadMask([IdentityMask((seq_len, seq_len))])
|
|
|
|
process_mask_path = "jax.experimental.pallas.ops.tpu.splash_attention.splash_attention_mask_info.process_mask"
|
|
process_mask_shrink = lambda *args, **kwargs: process_mask(
|
|
*args, **kwargs, shrink_grid=True
|
|
)
|
|
process_mask_no_shrink = lambda *args, **kwargs: process_mask(
|
|
*args, **kwargs, shrink_grid=False
|
|
)
|
|
|
|
with unittest.mock.patch(process_mask_path, process_mask_shrink):
|
|
shrink_out = splash.make_splash_mqa_single_device(identity_mask)(q, k, v)
|
|
|
|
with unittest.mock.patch(process_mask_path, process_mask_no_shrink):
|
|
no_shrink_out = splash.make_splash_mqa_single_device(identity_mask)(
|
|
q, k, v
|
|
)
|
|
|
|
np.testing.assert_array_equal(shrink_out, no_shrink_out)
|
|
|
|
|
|
if __name__ == "__main__":
|
|
absltest.main(testLoader=jtu.JaxTestLoader())
|