mirror of
https://github.com/ROCm/jax.git
synced 2025-04-16 20:06:05 +00:00
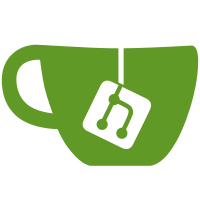
This feature has been in the queue for a long time (see https://github.com/jax-ml/jax/issues/1259), and some folks have found that they can use `pure_callback` to call the CPU version as a workaround. It has recently come up that there can be issues when using `pure_callback` with JAX calls in the body (https://github.com/jax-ml/jax/issues/24255; this should be investigated separately). This change adds a native solution for computing `lax.linalg.eig` on GPU. By default, this is implemented by calling LAPACK on host directly because this has good performance for small to moderately sized problems (less than about 2048^2). For larger matrices, a GPU-backed implementation based on [MAGMA](https://icl.utk.edu/magma/) can have significantly better performance. (I should note that I haven't done a huge amount of benchmarking yet, but this was the breakeven point used by PyTorch, and I find roughly similar behavior so far.) We don't want to add MAGMA as a required dependency, but if a user has installed it, JAX can use it when the `jax_gpu_use_magma` configuration variable is set to `"on"`. By default, we try to dlopen `libmagma.so`, but the path to a non-standard installation location can be specified using the `JAX_GPU_MAGMA_PATH` environment variable. PiperOrigin-RevId: 697631402
91 lines
2.1 KiB
Python
91 lines
2.1 KiB
Python
# Copyright 2018 The JAX Authors.
|
|
#
|
|
# Licensed under the Apache License, Version 2.0 (the "License");
|
|
# you may not use this file except in compliance with the License.
|
|
# You may obtain a copy of the License at
|
|
#
|
|
# https://www.apache.org/licenses/LICENSE-2.0
|
|
#
|
|
# Unless required by applicable law or agreed to in writing, software
|
|
# distributed under the License is distributed on an "AS IS" BASIS,
|
|
# WITHOUT WARRANTIES OR CONDITIONS OF ANY KIND, either express or implied.
|
|
# See the License for the specific language governing permissions and
|
|
# limitations under the License.
|
|
|
|
# Shared CUDA/ROCM GPU kernels.
|
|
|
|
load(
|
|
"//jaxlib:jax.bzl",
|
|
"cc_proto_library",
|
|
"jax_visibility",
|
|
"xla_py_proto_library",
|
|
)
|
|
|
|
licenses(["notice"])
|
|
|
|
package(
|
|
default_applicable_licenses = [],
|
|
default_visibility = ["//jax:internal"],
|
|
)
|
|
|
|
exports_files(srcs = [
|
|
"blas.cc",
|
|
"blas_handle_pool.cc",
|
|
"blas_handle_pool.h",
|
|
"blas_kernels.cc",
|
|
"blas_kernels.h",
|
|
"gpu_kernel_helpers.cc",
|
|
"gpu_kernel_helpers.h",
|
|
"gpu_kernels.cc",
|
|
"hybrid.cc",
|
|
"hybrid_kernels.cc",
|
|
"hybrid_kernels.h",
|
|
"linalg.cc",
|
|
"linalg_kernels.cc",
|
|
"linalg_kernels.cu.cc",
|
|
"linalg_kernels.h",
|
|
"make_batch_pointers.cu.cc",
|
|
"make_batch_pointers.h",
|
|
"prng.cc",
|
|
"prng_kernels.cc",
|
|
"prng_kernels.cu.cc",
|
|
"prng_kernels.h",
|
|
"rnn.cc",
|
|
"rnn_kernels.cc",
|
|
"rnn_kernels.h",
|
|
"solver.cc",
|
|
"solver_handle_pool.cc",
|
|
"solver_handle_pool.h",
|
|
"solver_interface.cc",
|
|
"solver_interface.h",
|
|
"solver_kernels.cc",
|
|
"solver_kernels.h",
|
|
"solver_kernels_ffi.cc",
|
|
"solver_kernels_ffi.h",
|
|
"sparse.cc",
|
|
"sparse_kernels.cc",
|
|
"sparse_kernels.h",
|
|
"triton.cc",
|
|
"triton_kernels.cc",
|
|
"triton_kernels.h",
|
|
"triton_utils.cc",
|
|
"triton_utils.h",
|
|
"vendor.h",
|
|
])
|
|
|
|
proto_library(
|
|
name = "triton_proto",
|
|
srcs = ["triton.proto"],
|
|
)
|
|
|
|
cc_proto_library(
|
|
name = "triton_cc_proto",
|
|
deps = [":triton_proto"],
|
|
)
|
|
|
|
xla_py_proto_library(
|
|
name = "triton_py_pb2",
|
|
visibility = jax_visibility("triton_proto_py_users"),
|
|
deps = [":triton_proto"],
|
|
)
|