mirror of
https://github.com/ROCm/jax.git
synced 2025-04-17 04:16:07 +00:00
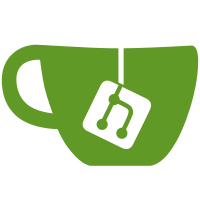
This change is in preparation for deprecating the XlaBuilder APIs for building non-MLIR HLO. In general JAX would be best served by adding a more user-friendly "custom kernel" API that doesn't require the user to build IR directly, but for the moment the best we can do is migrate users to use MLIR/StableHLO utilities instead of classic HLO utilities. Since most users of custom kernels probably want to build a custom-call we can get most of the benefit by providing an ergonomic helper function for building the IR for custom calls that can be called by external primitive lowering rules. This function has two benefits over just building the stablehlo directly: a) it is a JAX API, and we can be more confident the API won't change because of upstream MLIR changes b) the Python API to build stablehlo.custom_call generated by the bindings isn't that easy to use (e.g. it doesn't have sensible defaults). Next step will be to deprecate XlaBuilder and encourage users to switch to lowering rules using this helper. PiperOrigin-RevId: 561042402
426 lines
17 KiB
Python
426 lines
17 KiB
Python
# Copyright 2021 The JAX Authors.
|
|
#
|
|
# Licensed under the Apache License, Version 2.0 (the "License");
|
|
# you may not use this file except in compliance with the License.
|
|
# You may obtain a copy of the License at
|
|
#
|
|
# https://www.apache.org/licenses/LICENSE-2.0
|
|
#
|
|
# Unless required by applicable law or agreed to in writing, software
|
|
# distributed under the License is distributed on an "AS IS" BASIS,
|
|
# WITHOUT WARRANTIES OR CONDITIONS OF ANY KIND, either express or implied.
|
|
# See the License for the specific language governing permissions and
|
|
# limitations under the License.
|
|
|
|
"""ANN (Approximate Nearest Neighbor) computes top-k with a configurable recall rate.
|
|
|
|
This package only optimizes the TPU backend. For other device types it fallbacks
|
|
to sort and slice.
|
|
|
|
Usage::
|
|
|
|
import functools
|
|
import jax
|
|
|
|
# MIPS := maximal inner product search
|
|
# Inputs:
|
|
# qy: f32[qy_size, feature_dim]
|
|
# db: f32[db_size, feature_dim]
|
|
#
|
|
# Returns:
|
|
# (f32[qy_size, k], i32[qy_size, k])
|
|
@functools.partial(jax.jit, static_argnames=["k", "recall_target"])
|
|
def mips(qy, db, k=10, recall_target=0.95):
|
|
dists = jax.lax.dot(qy, db.transpose())
|
|
# Computes max_k along the last dimension
|
|
# returns (f32[qy_size, k], i32[qy_size, k])
|
|
return jax.lax.approx_max_k(dists, k=k, recall_target=recall_target)
|
|
|
|
# Multi-core example
|
|
# Inputs:
|
|
# qy: f32[num_devices, qy_size, feature_dim]
|
|
# db: f32[num_devices, per_device_db_size, feature_dim]
|
|
# db_offset: i32[num_devices]
|
|
# db_size = num_devices * per_device_db_size
|
|
#
|
|
# Returns:
|
|
# (f32[qy_size, num_devices, k], i32[qy_size, num_devices, k])
|
|
@functools.partial(
|
|
jax.pmap,
|
|
# static args: db_size, k, recall_target
|
|
static_broadcasted_argnums=[3, 4, 5],
|
|
out_axes=(1, 1))
|
|
def pmap_mips(qy, db, db_offset, db_size, k, recall_target):
|
|
dists = jax.lax.dot(qy, db.transpose())
|
|
dists, neighbors = jax.lax.approx_max_k(
|
|
dists, k=k, recall_target=recall_target,
|
|
reduction_input_size_override=db_size)
|
|
return (dists, neighbors + db_offset)
|
|
|
|
# i32[qy_size, num_devices, k]
|
|
pmap_neighbors = pmap_mips(qy, db, db_offset, db_size, 10, 0.95)[1]
|
|
# i32[qy_size, num_devices * k]
|
|
neighbors = jax.lax.collapse(pmap_neighbors, start_dimension=1, stop_dimension=3)
|
|
|
|
Todos::
|
|
|
|
* On host top-k aggregation
|
|
* Inaccurate but fast differentiation
|
|
|
|
"""
|
|
|
|
from functools import partial
|
|
from typing import Any
|
|
|
|
import numpy as np
|
|
|
|
|
|
from jax._src import ad_util
|
|
from jax._src import core
|
|
from jax._src import dispatch
|
|
from jax._src import dtypes
|
|
from jax._src.interpreters import ad
|
|
from jax._src.interpreters import batching
|
|
from jax._src.interpreters import mlir
|
|
from jax._src.interpreters import xla
|
|
from jax._src.lax import lax
|
|
from jax._src.lib import xla_client as xc
|
|
from jax._src.lib.mlir import ir
|
|
from jax._src.lib.mlir.dialects import func
|
|
from jax._src.lib.mlir.dialects import hlo
|
|
|
|
|
|
Array = Any
|
|
|
|
|
|
def approx_max_k(operand: Array,
|
|
k: int,
|
|
reduction_dimension: int = -1,
|
|
recall_target: float = 0.95,
|
|
reduction_input_size_override: int = -1,
|
|
aggregate_to_topk: bool = True) -> tuple[Array, Array]:
|
|
"""Returns max ``k`` values and their indices of the ``operand`` in an approximate manner.
|
|
|
|
See https://arxiv.org/abs/2206.14286 for the algorithm details.
|
|
|
|
Args:
|
|
operand : Array to search for max-k. Must be a floating number type.
|
|
k : Specifies the number of max-k.
|
|
reduction_dimension : Integer dimension along which to search. Default: -1.
|
|
recall_target : Recall target for the approximation.
|
|
reduction_input_size_override : When set to a positive value, it overrides
|
|
the size determined by ``operand[reduction_dim]`` for evaluating the
|
|
recall. This option is useful when the given ``operand`` is only a subset
|
|
of the overall computation in SPMD or distributed pipelines, where the
|
|
true input size cannot be deferred by the operand shape.
|
|
aggregate_to_topk : When true, aggregates approximate results to the top-k
|
|
in sorted order. When false, returns the approximate results unsorted. In
|
|
this case, the number of the approximate results is implementation defined
|
|
and is greater or equal to the specified ``k``.
|
|
|
|
Returns:
|
|
Tuple of two arrays. The arrays are the max ``k`` values and the
|
|
corresponding indices along the ``reduction_dimension`` of the input
|
|
``operand``. The arrays' dimensions are the same as the input ``operand``
|
|
except for the ``reduction_dimension``: when ``aggregate_to_topk`` is true,
|
|
the reduction dimension is ``k``; otherwise, it is greater equals to ``k``
|
|
where the size is implementation-defined.
|
|
|
|
We encourage users to wrap ``approx_max_k`` with jit. See the following
|
|
example for maximal inner production search (MIPS):
|
|
|
|
>>> import functools
|
|
>>> import jax
|
|
>>> import numpy as np
|
|
>>> @functools.partial(jax.jit, static_argnames=["k", "recall_target"])
|
|
... def mips(qy, db, k=10, recall_target=0.95):
|
|
... dists = jax.lax.dot(qy, db.transpose())
|
|
... # returns (f32[qy_size, k], i32[qy_size, k])
|
|
... return jax.lax.approx_max_k(dists, k=k, recall_target=recall_target)
|
|
>>>
|
|
>>> qy = jax.numpy.array(np.random.rand(50, 64))
|
|
>>> db = jax.numpy.array(np.random.rand(1024, 64))
|
|
>>> dot_products, neighbors = mips(qy, db, k=10)
|
|
"""
|
|
return approx_top_k_p.bind(
|
|
operand,
|
|
k=k,
|
|
reduction_dimension=reduction_dimension,
|
|
recall_target=recall_target,
|
|
is_max_k=True,
|
|
reduction_input_size_override=reduction_input_size_override,
|
|
aggregate_to_topk=aggregate_to_topk)
|
|
|
|
|
|
def approx_min_k(operand: Array,
|
|
k: int,
|
|
reduction_dimension: int = -1,
|
|
recall_target: float = 0.95,
|
|
reduction_input_size_override: int = -1,
|
|
aggregate_to_topk: bool = True) -> tuple[Array, Array]:
|
|
"""Returns min ``k`` values and their indices of the ``operand`` in an approximate manner.
|
|
|
|
See https://arxiv.org/abs/2206.14286 for the algorithm details.
|
|
|
|
Args:
|
|
operand : Array to search for min-k. Must be a floating number type.
|
|
k : Specifies the number of min-k.
|
|
reduction_dimension: Integer dimension along which to search. Default: -1.
|
|
recall_target: Recall target for the approximation.
|
|
reduction_input_size_override : When set to a positive value, it overrides
|
|
the size determined by ``operand[reduction_dim]`` for evaluating the
|
|
recall. This option is useful when the given operand is only a subset of
|
|
the overall computation in SPMD or distributed pipelines, where the true
|
|
input size cannot be deferred by the ``operand`` shape.
|
|
aggregate_to_topk : When true, aggregates approximate results to the top-k
|
|
in sorted order. When false, returns the approximate results unsorted. In
|
|
this case, the number of the approximate results is implementation defined
|
|
and is greater or equal to the specified ``k``.
|
|
|
|
Returns:
|
|
Tuple of two arrays. The arrays are the least ``k`` values and the
|
|
corresponding indices along the ``reduction_dimension`` of the input
|
|
``operand``. The arrays' dimensions are the same as the input ``operand``
|
|
except for the ``reduction_dimension``: when ``aggregate_to_topk`` is true,
|
|
the reduction dimension is ``k``; otherwise, it is greater equals to ``k``
|
|
where the size is implementation-defined.
|
|
|
|
We encourage users to wrap ``approx_min_k`` with jit. See the following example
|
|
for nearest neighbor search over the squared l2 distance:
|
|
|
|
>>> import functools
|
|
>>> import jax
|
|
>>> import numpy as np
|
|
>>> @functools.partial(jax.jit, static_argnames=["k", "recall_target"])
|
|
... def l2_ann(qy, db, half_db_norms, k=10, recall_target=0.95):
|
|
... dists = half_db_norms - jax.lax.dot(qy, db.transpose())
|
|
... return jax.lax.approx_min_k(dists, k=k, recall_target=recall_target)
|
|
>>>
|
|
>>> qy = jax.numpy.array(np.random.rand(50, 64))
|
|
>>> db = jax.numpy.array(np.random.rand(1024, 64))
|
|
>>> half_db_norm_sq = jax.numpy.linalg.norm(db, axis=1)**2 / 2
|
|
>>> dists, neighbors = l2_ann(qy, db, half_db_norm_sq, k=10)
|
|
|
|
In the example above, we compute ``db^2/2 - dot(qy, db^T)`` instead of
|
|
``qy^2 - 2 dot(qy, db^T) + db^2`` for performance reason. The former uses less
|
|
arithmetics and produces the same set of neighbors.
|
|
"""
|
|
return approx_top_k_p.bind(
|
|
operand,
|
|
k=k,
|
|
reduction_dimension=reduction_dimension,
|
|
recall_target=recall_target,
|
|
is_max_k=False,
|
|
reduction_input_size_override=reduction_input_size_override,
|
|
aggregate_to_topk=aggregate_to_topk)
|
|
|
|
|
|
def _approx_top_k_abstract_eval(operand, *, k, reduction_dimension,
|
|
recall_target, is_max_k,
|
|
reduction_input_size_override,
|
|
aggregate_to_topk):
|
|
if k <= 0:
|
|
raise ValueError(f'k must be positive, got {k}')
|
|
if len(operand.shape) == 0:
|
|
raise TypeError('approx_top_k operand must have >= 1 dimension, got {}'.format(
|
|
operand.shape))
|
|
dims = list(operand.shape)
|
|
if dims[reduction_dimension] < k:
|
|
raise ValueError(
|
|
'k must be smaller than the size of reduction_dim {}, got {}'.format(
|
|
dims[reduction_dimension], k))
|
|
if not dtypes.issubdtype(operand.dtype, np.floating):
|
|
raise ValueError('operand must be a floating type')
|
|
reduction_input_size = dims[reduction_dimension]
|
|
dims[reduction_dimension] = xc.ops.ApproxTopKReductionOutputSize(
|
|
reduction_input_size, len(dims), k, recall_target, aggregate_to_topk,
|
|
reduction_input_size_override)[0]
|
|
return (operand.update(
|
|
shape=dims, dtype=operand.dtype, weak_type=operand.weak_type),
|
|
operand.update(shape=dims, dtype=np.dtype(np.int32)))
|
|
|
|
|
|
def _comparator_builder(op_type, is_max_k):
|
|
c = xc.XlaBuilder(
|
|
'top_k_{}_comparator'.format('gt' if is_max_k else 'lt'))
|
|
p0 = xla.parameter(c, 0, xc.Shape.scalar_shape(op_type))
|
|
p1 = xla.parameter(c, 1, xc.Shape.scalar_shape(op_type))
|
|
xla.parameter(c, 2, xc.Shape.scalar_shape(np.dtype(np.int32)))
|
|
xla.parameter(c, 3, xc.Shape.scalar_shape(np.dtype(np.int32)))
|
|
if is_max_k:
|
|
cmp_result = xc.ops.Gt(p0, p1)
|
|
else:
|
|
cmp_result = xc.ops.Lt(p0, p1)
|
|
return c.build(cmp_result)
|
|
|
|
|
|
def _get_init_val_literal(op_type, is_max_k):
|
|
return np.array(-np.inf if is_max_k else np.inf, dtype=op_type)
|
|
|
|
def _approx_top_k_tpu_translation(ctx, avals_in, avals_out, operand, *, k,
|
|
reduction_dimension, recall_target, is_max_k,
|
|
reduction_input_size_override,
|
|
aggregate_to_topk):
|
|
c = ctx.builder
|
|
op_shape = c.get_shape(operand)
|
|
if not op_shape.is_array():
|
|
raise ValueError(f'operand must be an array, but was {op_shape}')
|
|
op_dims = op_shape.dimensions()
|
|
op_type = op_shape.element_type()
|
|
if reduction_dimension < 0:
|
|
reduction_dimension = len(op_dims) + reduction_dimension
|
|
comparator = _comparator_builder(op_type, is_max_k)
|
|
init_val_literal = _get_init_val_literal(op_type, is_max_k)
|
|
iota = xc.ops.Iota(c, xc.Shape.array_shape(np.dtype(np.int32), op_dims),
|
|
reduction_dimension)
|
|
init_val = xc.ops.Constant(c, init_val_literal)
|
|
init_arg = xc.ops.Constant(c, np.int32(-1))
|
|
out = xc.ops.ApproxTopK(c, [operand, iota], [init_val, init_arg], k,
|
|
reduction_dimension, comparator, recall_target,
|
|
aggregate_to_topk, reduction_input_size_override)
|
|
return xla.xla_destructure(c, out)
|
|
|
|
|
|
def _comparator_builder_mlir(ctx, op_type, is_max_k):
|
|
scalar = ir.RankedTensorType.get([], op_type)
|
|
index = ir.RankedTensorType.get([], ir.IntegerType.get_signless(32))
|
|
ir_types = [scalar, scalar, index, index]
|
|
result_types = [ir.RankedTensorType.get([], ir.IntegerType.get_signless(1))]
|
|
|
|
comparator_type = ir.FunctionType.get(ir_types, result_types)
|
|
with ir.InsertionPoint.at_block_begin(ctx.module_context.module.body):
|
|
comparator = func.FuncOp(
|
|
"top_k_{}_{}_comparator".format('gt' if is_max_k else 'lt', op_type),
|
|
comparator_type)
|
|
ctx.module_context.symbol_table.insert(comparator)
|
|
|
|
entry_block = comparator.add_entry_block()
|
|
with ir.InsertionPoint(entry_block):
|
|
p0, p1, _, _ = entry_block.arguments
|
|
direction = hlo.ComparisonDirectionAttr.get('GT' if is_max_k else 'LT')
|
|
cmp_result = hlo.CompareOp(p0, p1, comparison_direction=direction)
|
|
hlo.ReturnOp(cmp_result)
|
|
|
|
return comparator
|
|
|
|
def _approx_top_k_lowering(ctx, operand, *, k,
|
|
reduction_dimension, recall_target, is_max_k,
|
|
reduction_input_size_override,
|
|
aggregate_to_topk, fallback=False):
|
|
assert ctx.avals_in
|
|
assert all(isinstance(x, core.ShapedArray) for x in ctx.avals_in)
|
|
|
|
op_shape = ctx.avals_in[0].shape
|
|
if len(op_shape) == 0:
|
|
raise ValueError(f'operand must be an array, but was {op_shape}')
|
|
|
|
op_dims = op_shape
|
|
op_type = mlir.dtype_to_ir_type(ctx.avals_in[0].dtype)
|
|
recall_type = ir.F32Type.get()
|
|
if reduction_dimension < 0:
|
|
reduction_dimension = len(op_dims) + reduction_dimension
|
|
|
|
comparator = _comparator_builder_mlir(ctx, op_type, is_max_k)
|
|
iota = mlir.iota(ctx, core.ShapedArray(ctx.avals_in[0].shape, np.int32),
|
|
dimension=reduction_dimension)
|
|
|
|
init_arg = hlo.ConstantOp(ir.DenseElementsAttr.get(np.int32(-1))).result
|
|
init_val_array = _get_init_val_literal(ctx.avals_in[0].dtype, is_max_k)
|
|
init_val = mlir.ir_constant(init_val_array.reshape(()))
|
|
|
|
backend_config = {
|
|
"top_k" : mlir.i64_attr(k),
|
|
"reduction_dim" : mlir.i64_attr(reduction_dimension),
|
|
"recall_target" : mlir.ir.FloatAttr.get(recall_type, recall_target),
|
|
"aggregate_to_topk" : mlir.ir.BoolAttr.get(aggregate_to_topk),
|
|
"reduction_input_size_override" :
|
|
mlir.i64_attr(reduction_input_size_override)}
|
|
if fallback:
|
|
backend_config["is_fallback"] = mlir.ir.BoolAttr.get(fallback)
|
|
|
|
if all(core.is_constant_shape(aval_out.shape) for aval_out in ctx.avals_out):
|
|
result_shapes = None
|
|
else:
|
|
result_shapes = [
|
|
mlir.shape_tensor(mlir.eval_dynamic_shape(ctx, aval_out.shape))
|
|
for aval_out in ctx.avals_out]
|
|
|
|
out = mlir.custom_call(
|
|
"ApproxTopK",
|
|
result_types=[mlir.aval_to_ir_type(aval) for aval in ctx.avals_out],
|
|
operands=[operand, iota, init_val, init_arg],
|
|
called_computations=[comparator.name.value],
|
|
backend_config=backend_config,
|
|
result_shapes=result_shapes)
|
|
|
|
return out.results
|
|
|
|
def _approx_top_k_batch_rule(batch_operands, batch_axes, *, k,
|
|
reduction_dimension, recall_target, is_max_k,
|
|
reduction_input_size_override, aggregate_to_topk):
|
|
assert len(batch_operands) == 1
|
|
assert len(batch_axes) == 1
|
|
operand, = batch_operands
|
|
batch_axis, = batch_axes
|
|
dim_map = [d for d in range(operand.ndim) if d is not batch_axis]
|
|
reduction_dimension = dim_map[reduction_dimension]
|
|
return approx_top_k_p.bind(
|
|
operand,
|
|
k=k,
|
|
reduction_dimension=reduction_dimension,
|
|
recall_target=recall_target,
|
|
is_max_k=is_max_k,
|
|
reduction_input_size_override=reduction_input_size_override,
|
|
aggregate_to_topk=aggregate_to_topk), (batch_axis, batch_axis)
|
|
|
|
|
|
# Slow jvp implementation using gather.
|
|
#
|
|
# TODO(fchern): Some optimization ideas
|
|
# 1. ApproxTopK is internally a variadic reduce, so we can simply call
|
|
# ApproxTopK(operand, tangent, iota) for jvp.
|
|
# 2. vjp cannot benefit from the algorithm above. We must run scatter to
|
|
# distribute the output cotangent to input cotangent. A reasonable way to do
|
|
# this is to run it on CPU.
|
|
def _approx_top_k_jvp(primals, tangents, *, k, reduction_dimension,
|
|
recall_target, is_max_k, reduction_input_size_override,
|
|
aggregate_to_topk):
|
|
operand, = primals
|
|
tangent, = tangents
|
|
if is_max_k:
|
|
val_out, arg_out = approx_max_k(operand, k, reduction_dimension,
|
|
recall_target,
|
|
reduction_input_size_override,
|
|
aggregate_to_topk)
|
|
else:
|
|
val_out, arg_out = approx_min_k(operand, k, reduction_dimension,
|
|
recall_target,
|
|
reduction_input_size_override,
|
|
aggregate_to_topk)
|
|
if type(tangent) is ad_util.Zero:
|
|
tangent_out = ad_util.Zero.from_value(val_out)
|
|
else:
|
|
arg_shape = arg_out.shape
|
|
rank = len(arg_shape)
|
|
if reduction_dimension < 0:
|
|
reduction_dimension += rank
|
|
iotas = [
|
|
lax.broadcasted_iota(arg_out.dtype, arg_shape, i) for i in range(rank)
|
|
]
|
|
idx = tuple(
|
|
arg_out if i == reduction_dimension else iotas[i] for i in range(rank))
|
|
tangent_out = tangent[idx]
|
|
return (val_out, arg_out), (tangent_out, ad_util.Zero.from_value(arg_out))
|
|
|
|
|
|
approx_top_k_p = core.Primitive('approx_top_k')
|
|
approx_top_k_p.multiple_results = True
|
|
approx_top_k_p.def_impl(partial(dispatch.apply_primitive, approx_top_k_p))
|
|
approx_top_k_p.def_abstract_eval(_approx_top_k_abstract_eval)
|
|
mlir.register_lowering(approx_top_k_p,
|
|
partial(_approx_top_k_lowering, fallback=True))
|
|
mlir.register_lowering(approx_top_k_p, _approx_top_k_lowering,
|
|
platform='tpu')
|
|
batching.primitive_batchers[approx_top_k_p] = _approx_top_k_batch_rule
|
|
ad.primitive_jvps[approx_top_k_p] = _approx_top_k_jvp
|