mirror of
https://github.com/ROCm/jax.git
synced 2025-04-16 11:56:07 +00:00
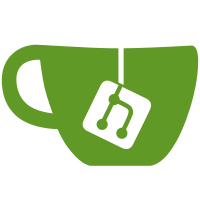
Created debug_info_test.py and moved there some of the tests involving debug_info. In the future we will put here more tests for debugging info, and their helper functions.
10975 lines
342 KiB
Python
10975 lines
342 KiB
Python
# Copyright 2018 The JAX Authors.
|
|
#
|
|
# Licensed under the Apache License, Version 2.0 (the "License");
|
|
# you may not use this file except in compliance with the License.
|
|
# You may obtain a copy of the License at
|
|
#
|
|
# https://www.apache.org/licenses/LICENSE-2.0
|
|
#
|
|
# Unless required by applicable law or agreed to in writing, software
|
|
# distributed under the License is distributed on an "AS IS" BASIS,
|
|
# WITHOUT WARRANTIES OR CONDITIONS OF ANY KIND, either express or implied.
|
|
# See the License for the specific language governing permissions and
|
|
# limitations under the License.
|
|
|
|
from __future__ import annotations
|
|
|
|
import collections
|
|
import collections.abc
|
|
from collections.abc import Callable
|
|
import concurrent.futures
|
|
from contextlib import contextmanager
|
|
import copy
|
|
import enum
|
|
import functools
|
|
from functools import partial
|
|
import gc
|
|
import importlib
|
|
import inspect
|
|
import itertools as it
|
|
import operator
|
|
import operator as op
|
|
import os
|
|
import re
|
|
import subprocess
|
|
import sys
|
|
import traceback
|
|
import types
|
|
from typing import NamedTuple
|
|
import unittest
|
|
import weakref
|
|
|
|
from absl import logging
|
|
from absl.testing import absltest, parameterized
|
|
import jax
|
|
from jax import custom_derivatives as custom_derivatives_public
|
|
from jax import device_put, float0, grad, hessian, jacfwd, jacrev, jit
|
|
from jax import lax
|
|
from jax import tree_util
|
|
from jax._src import api, api_util, dtypes, lib
|
|
from jax._src import array
|
|
from jax._src import config
|
|
from jax._src import core
|
|
from jax._src import custom_derivatives
|
|
from jax._src import linear_util as lu
|
|
from jax._src import test_util as jtu
|
|
from jax._src import xla_bridge
|
|
from jax._src import debugging
|
|
from jax._src import pjit as pjit_lib
|
|
from jax._src.ad_checkpoint import saved_residuals
|
|
from jax._src.interpreters import mlir
|
|
from jax._src.interpreters import partial_eval as pe
|
|
from jax._src.compilation_cache import is_persistent_cache_enabled
|
|
from jax._src.lib import xla_extension
|
|
import jax._src.util as jax_util
|
|
from jax.ad_checkpoint import checkpoint_name, checkpoint as new_checkpoint
|
|
import jax.custom_batching
|
|
import jax.custom_derivatives
|
|
import jax.custom_transpose
|
|
from jax.errors import (UnexpectedTracerError, TracerIntegerConversionError,
|
|
ConcretizationTypeError, TracerBoolConversionError)
|
|
from jax.experimental import pjit
|
|
from jax.interpreters import ad
|
|
from jax.interpreters import batching
|
|
from jax.interpreters import xla
|
|
import jax.numpy as jnp
|
|
from jax.sharding import PartitionSpec as P
|
|
import numpy as np
|
|
|
|
config.parse_flags_with_absl()
|
|
|
|
|
|
def _check_instance(self, x):
|
|
self.assertIsInstance(x, array.ArrayImpl)
|
|
|
|
|
|
class JitTest(jtu.BufferDonationTestCase):
|
|
"""Shared tests between the Python and the C++ jax,jit implementations.
|
|
|
|
Because the Python implementation supports more features, we need to have the
|
|
Python tests that extend the C++ tests (and not the other way around).
|
|
"""
|
|
|
|
def test_jit_repr(self):
|
|
def my_function():
|
|
return
|
|
jitted = jit(my_function)
|
|
self.assertEqual(repr(jitted), f"<PjitFunction of {repr(my_function)}>")
|
|
|
|
def test_fun_name(self):
|
|
def my_function():
|
|
return
|
|
|
|
with self.subTest("function"):
|
|
jitted = jit(my_function)
|
|
self.assertEqual(
|
|
jitted.__getstate__()["function_name"], my_function.__name__
|
|
)
|
|
with self.subTest("default_partial"):
|
|
my_partial = partial(my_function)
|
|
jitted = jit(my_partial)
|
|
self.assertEqual(
|
|
jitted.__getstate__()["function_name"], my_function.__name__
|
|
)
|
|
with self.subTest("nested_default_partial"):
|
|
my_partial = partial(partial(my_function))
|
|
jitted = jit(my_partial)
|
|
self.assertEqual(
|
|
jitted.__getstate__()["function_name"], my_function.__name__
|
|
)
|
|
with self.subTest("named_partial"):
|
|
my_partial = partial(my_function)
|
|
my_partial.__name__ = "my_partial"
|
|
jitted = jit(my_partial)
|
|
self.assertEqual(
|
|
jitted.__getstate__()["function_name"], my_partial.__name__
|
|
)
|
|
with self.subTest("lambda"):
|
|
jitted = jit(lambda: my_function())
|
|
self.assertEqual(jitted.__getstate__()["function_name"], "<lambda>")
|
|
|
|
def test_jit_repr_errors(self):
|
|
class Callable:
|
|
def __call__(self): pass
|
|
def __repr__(self):
|
|
raise ValueError("invalid repr")
|
|
|
|
# repr succeeds when underlying function repr fails.
|
|
jitted = jit(Callable())
|
|
self.assertEqual(repr(jitted), "<PjitFunction>")
|
|
|
|
# repr succeeds when object is malformed.
|
|
del jitted.__wrapped__
|
|
self.assertEqual(repr(jitted), "<PjitFunction>")
|
|
|
|
def test_jit_of_noncallable(self):
|
|
self.assertRaisesRegex(TypeError, "Expected a callable value.*",
|
|
lambda: jit(3))
|
|
|
|
def test_jit_of_generator(self):
|
|
|
|
def gen(x):
|
|
yield x
|
|
|
|
self.assertRaisesRegex(TypeError,
|
|
"Expected a function, got a generator function.*",
|
|
lambda: jit(gen))
|
|
|
|
@parameterized.parameters([
|
|
# Integer support
|
|
(1, 2, 3, 4, 5),
|
|
# Numpy array support
|
|
(
|
|
np.asarray(1, np.int32),
|
|
np.asarray(2, np.int32),
|
|
np.asarray(3, np.int32),
|
|
np.asarray(4, np.int32),
|
|
np.asarray(5, np.int32),
|
|
),
|
|
])
|
|
def test_jit_static_args(self, one, two, three, four, five):
|
|
side = []
|
|
|
|
def f(x, y, z, flag=False, flag2=False):
|
|
del flag2 # unused
|
|
assert flag
|
|
side.append(None)
|
|
return 100 * x + 10 * y + z
|
|
|
|
f1 = jit(f, static_argnums=(3, 4))
|
|
assert f1(one, two, three, True, False) == 123
|
|
assert len(side) == 1
|
|
assert f1(one, two, three, True, False) == 123
|
|
assert len(side) == 1 # Obvious cache hit.
|
|
assert f1(two, one, three, True, False) == 213
|
|
assert len(side) == 1 # Should cache hit because same signature.
|
|
assert f1(two, one, three, True, True) == 213
|
|
assert len(side) == 2
|
|
|
|
side[:] = []
|
|
f2 = jit(f, static_argnums=(0, 2, 3, 4))
|
|
assert f2(1, 2, 3, True, False) == 123
|
|
assert len(side) == 1
|
|
assert f2(1, 3, 3, True, False) == 133
|
|
assert len(side) == 1
|
|
assert f2(2, 2, 3, True, False) == 223
|
|
assert len(side) == 2
|
|
assert f2(2, 4, 3, True, False) == 243
|
|
assert len(side) == 2
|
|
assert f2(2, 4, 3, True, True) == 243
|
|
assert len(side) == 3
|
|
assert f2(2, 5, 3, True, True) == 253
|
|
assert len(side) == 3
|
|
|
|
def test_static_args_equality(self):
|
|
class A():
|
|
|
|
def __hash__(self):
|
|
return 1
|
|
|
|
def __eq__(self, other):
|
|
return isinstance(other, A)
|
|
|
|
side = []
|
|
def f(x, static_arg):
|
|
del static_arg
|
|
side.append(None)
|
|
return x * 100
|
|
|
|
f1 = jit(f, static_argnums=(1,))
|
|
|
|
self.assertEqual(f1(1, A()), 100)
|
|
self.assertLen(side, 1)
|
|
self.assertEqual(f1(1, A()), 100)
|
|
self.assertLen(side, 1)
|
|
f1_cpp = getattr(f1, "_cpp_jitted_f", f1)
|
|
self.assertEqual(f1_cpp._cache_size(), 1)
|
|
|
|
@parameterized.parameters([
|
|
(1, 2, 3),
|
|
(
|
|
np.asarray(1, np.int32),
|
|
np.asarray(2, np.int32),
|
|
np.asarray(3, np.int32),
|
|
),
|
|
])
|
|
def test_jit_kwargs(self, one, two, three):
|
|
side = []
|
|
# For the CPP jit, we need to clear the cache to prevent cache hits between
|
|
# parameterized tests.
|
|
if hasattr(jit, "cache_clear"):
|
|
jit.cache_clear()
|
|
|
|
def f(x, y, z):
|
|
side.append(None)
|
|
return 100 * x + 10 * y + z.astype(y.dtype)
|
|
|
|
f = jit(f)
|
|
assert f(one, two, three) == 123
|
|
assert len(side) == 1
|
|
assert f(one, two, three) == 123
|
|
assert len(side) == 1
|
|
|
|
assert f(one, two, z=three) == 123
|
|
assert len(side) == 2 # actually recompiles from kwarg
|
|
assert f(one, two, z=three) == 123
|
|
assert len(side) == 2 # but should still cache
|
|
|
|
f(one, two, z=np.zeros(3)) # doesn't crash
|
|
if config.enable_x64.value:
|
|
# In the above call, three is of a new type (int64), thus it should
|
|
# trigger a new compilation.
|
|
assert len(side) == 3
|
|
|
|
def test_jit_device(self):
|
|
device = jax.devices()[-1]
|
|
with jtu.ignore_warning(category=DeprecationWarning,
|
|
message="backend and device argument"):
|
|
x = jit(lambda x: x, device=device)(3.)
|
|
_check_instance(self, x)
|
|
self.assertEqual(x.devices(), {device})
|
|
|
|
@parameterized.named_parameters(
|
|
('jit', jax.jit),
|
|
('pjit', pjit.pjit),
|
|
)
|
|
@jtu.skip_on_devices("cpu")
|
|
def test_jit_default_device(self, module):
|
|
if jax.device_count() == 1:
|
|
raise unittest.SkipTest("Test requires multiple devices")
|
|
|
|
system_default_devices = jnp.add(1, 1).devices()
|
|
self.assertLen(system_default_devices, 1)
|
|
system_default_device = list(system_default_devices)[0]
|
|
test_device = jax.devices()[-1]
|
|
self.assertNotEqual(system_default_device, test_device)
|
|
|
|
f = module(lambda x: x + 1)
|
|
self.assertEqual(f(1).devices(), system_default_devices)
|
|
|
|
with jax.default_device(test_device):
|
|
self.assertEqual(jnp.add(1, 1).devices(), {test_device})
|
|
self.assertEqual(f(1).devices(), {test_device})
|
|
|
|
self.assertEqual(jnp.add(1, 1).devices(), system_default_devices)
|
|
self.assertEqual(f(1).devices(), system_default_devices)
|
|
|
|
with jax.default_device(test_device):
|
|
# Explicit `device` or `backend` argument to jit overrides default_device
|
|
with jtu.ignore_warning(category=DeprecationWarning,
|
|
message="backend and device argument"):
|
|
self.assertEqual(
|
|
module(f, device=system_default_device)(1).devices(),
|
|
system_default_devices)
|
|
out = module(f, backend="cpu")(1)
|
|
self.assertEqual(next(iter(out.devices())).platform, "cpu")
|
|
|
|
# Sticky input device overrides default_device
|
|
sticky = jax.device_put(1, system_default_device)
|
|
self.assertEqual(jnp.add(sticky, 1).devices(), system_default_devices)
|
|
self.assertEqual(f(sticky).devices(), system_default_devices)
|
|
|
|
# Test nested default_devices
|
|
with jax.default_device(system_default_device):
|
|
self.assertEqual(f(1).devices(), system_default_devices)
|
|
self.assertEqual(f(1).devices(), {test_device})
|
|
|
|
# Test a few more non-default_device calls for good luck
|
|
self.assertEqual(jnp.add(1, 1).devices(), system_default_devices)
|
|
self.assertEqual(f(sticky).devices(), system_default_devices)
|
|
self.assertEqual(f(1).devices(), system_default_devices)
|
|
|
|
def test_jit_default_platform(self):
|
|
with jax.default_device("cpu"):
|
|
result = jax.jit(lambda x: x + 1)(1)
|
|
self.assertEqual(result.device.platform, "cpu")
|
|
self.assertEqual(result.device, jax.local_devices(backend="cpu")[0])
|
|
|
|
result = jax.jit(lambda x: x + 1)(1)
|
|
self.assertEqual(result.device.platform, jax.default_backend())
|
|
self.assertEqual(result.device, jax.local_devices()[0])
|
|
|
|
def test_complex_support(self):
|
|
self.assertEqual(jit(lambda x: x + 1)(1 + 1j), 2 + 1j)
|
|
|
|
@parameterized.parameters("static_argnums", "donate_argnums")
|
|
def test_jit_argnums_overflow_error(self, argnum_type: str):
|
|
def f(a, b, c):
|
|
...
|
|
|
|
def g(a, /, b, *, c):
|
|
...
|
|
|
|
def h(a, *args):
|
|
...
|
|
|
|
def i():
|
|
...
|
|
|
|
# Simplest cases
|
|
jit(f, **{argnum_type: (0, 1)})
|
|
jit(g, **{argnum_type: (0, 1)})
|
|
jit(f, **{argnum_type: (0, 1, -3)})
|
|
|
|
# Out of bounds without *args
|
|
with self.assertRaises(ValueError):
|
|
jit(f, **{argnum_type: (0, 1, 3)})
|
|
|
|
with self.assertRaises(ValueError):
|
|
jit(f, **{argnum_type: (0, 1, -4)})
|
|
|
|
with self.assertRaises(ValueError):
|
|
jit(g, **{argnum_type: (0, 1, 3)})
|
|
|
|
with self.assertRaises(ValueError):
|
|
jit(g, **{argnum_type: (0, 1, -3)})
|
|
|
|
# Out of bounds with *args
|
|
jit(h, **{argnum_type: (0, 999)})
|
|
jit(h, **{argnum_type: (0, -999)})
|
|
|
|
# No positional arguments
|
|
jit(i, static_argnums=())
|
|
jit(i)
|
|
|
|
@parameterized.parameters("static_argnames", "donate_argnames")
|
|
def test_jit_argnames_validation(self, argnum_type: str):
|
|
def f(a, b, c):
|
|
...
|
|
|
|
def g(a, b, **kwargs):
|
|
...
|
|
|
|
def h(a, /, b, c, *args, **kwargs):
|
|
...
|
|
|
|
# Simplest case
|
|
jit(f, **{argnum_type: ("b", "c")})
|
|
|
|
# Undefined arg without **kwargs
|
|
with self.assertRaises(ValueError):
|
|
jit(f, **{argnum_type: ("b", "c", "not_defined")})
|
|
|
|
# Undefined arg with **kwargs
|
|
jit(g, **{argnum_type: ("a", "b", "not_defined")})
|
|
|
|
jit(h, **{argnum_type: ("b", "c")})
|
|
jit(h, **{argnum_type: ("b", "c", "not_defined")})
|
|
|
|
# Positional only
|
|
with self.assertRaises(ValueError):
|
|
jit(h, **{argnum_type: ("a", "c")})
|
|
|
|
# Var positional
|
|
with self.assertRaises(ValueError):
|
|
jit(h, **{argnum_type: ("args", "c")})
|
|
|
|
def test_jit_with_many_args_works(self):
|
|
|
|
@jit
|
|
def f(args_list):
|
|
return sum(args_list)
|
|
|
|
self.assertEqual(f(list(range(500))), sum(range(500)))
|
|
|
|
# Jit and Donate arguments
|
|
|
|
def test_donate_argnames_signature_fail(self):
|
|
inp = np.arange(4)
|
|
with self.assertRaisesRegex(
|
|
ValueError,
|
|
"Getting the signature of function.*failed. Pass donate_argnums "
|
|
"instead of donate_argnames."):
|
|
jax.jit(np.dot, donate_argnames='a')(inp, inp)
|
|
|
|
@parameterized.named_parameters(
|
|
("argnums", "donate_argnums", (0, 1)),
|
|
("argnames", "donate_argnames", ('x', 'y')),
|
|
)
|
|
def test_jit_donate_warning_raised(self, argnum_type, argnum_val):
|
|
x = jnp.array([1.0, 2.0], jnp.float32)
|
|
y = jnp.array([1, 2], jnp.int32)
|
|
f = jit(lambda x, y: x.sum() + jnp.float32(y.sum()),
|
|
**{argnum_type: argnum_val})
|
|
with self.assertWarnsRegex(UserWarning, "Some donated buffers were not usable"):
|
|
f(x, y)
|
|
|
|
@parameterized.named_parameters(
|
|
("argnums", "donate_argnums", 0),
|
|
("argnames", "donate_argnames", 'x'),
|
|
)
|
|
@jtu.device_supports_buffer_donation()
|
|
def test_jit_donate_invalidates_input(self, argnum_type, argnum_val):
|
|
# We can't just use `lambda x: x` because JAX simplifies this away to an
|
|
# empty XLA computation.
|
|
move = jit(lambda x: x + x - x, **{argnum_type: argnum_val})
|
|
x = jnp.ones([])
|
|
y = move(x)
|
|
self.assertDeleted(x)
|
|
self.assertEqual(y, 1.)
|
|
|
|
@parameterized.named_parameters(
|
|
("donate_argnums", "donate_argnums", (2, 3)),
|
|
("donate_argnames", "donate_argnames", ('c', 'd')),
|
|
)
|
|
@jtu.device_supports_buffer_donation()
|
|
def test_jit_donate_static_argnums(self, argnum_type, argnum_val):
|
|
jit_fun = jit(
|
|
lambda a, b, c, d: ((a + b + c), (a + b + d)),
|
|
static_argnums=(0, 1),
|
|
**{argnum_type: argnum_val})
|
|
|
|
c = jax.device_put(jnp.array([2., 2.]))
|
|
d = jax.device_put(jnp.array([1., 1., 1., 1.]))
|
|
e, f = jit_fun(1, 2, c, d)
|
|
np.testing.assert_allclose(e, jnp.array([5., 5.]))
|
|
np.testing.assert_allclose(f, jnp.array([4., 4., 4., 4.]))
|
|
self.assertDeleted(c)
|
|
self.assertDeleted(d)
|
|
|
|
@jtu.device_supports_buffer_donation()
|
|
def test_jit_donate_argnames_kwargs_static_argnums(self):
|
|
jit_fun = jit(
|
|
lambda a, b, c, d, e: ((a + b + c), (a + b + d), (a + b + e)),
|
|
static_argnums=(0, 1),
|
|
donate_argnames=('d', 'e'))
|
|
|
|
c = jax.device_put(jnp.array([2., 2.]))
|
|
d = jax.device_put(jnp.array([1., 1., 1., 1.]))
|
|
e = jax.device_put(jnp.array([3., 3., 3., 3.]))
|
|
f, g, h = jit_fun(1, 2, c, d=d, e=e)
|
|
np.testing.assert_allclose(f, jnp.array([5., 5.]))
|
|
np.testing.assert_allclose(g, jnp.array([4., 4., 4., 4.]))
|
|
np.testing.assert_allclose(h, jnp.array([6., 6., 6., 6.]))
|
|
self.assertNotDeleted(c)
|
|
self.assertDeleted(d)
|
|
self.assertDeleted(e)
|
|
|
|
def test_device_put_aliasing(self):
|
|
arr = jax.device_put(np.arange(8), jax.devices()[0])
|
|
out = jax.device_put(arr, may_alias=True, donate=False)
|
|
self.assertEqual(id(arr), id(out))
|
|
|
|
out = jax.device_put(arr, may_alias=False, donate=False)
|
|
self.assertNotEqual(id(arr), id(out))
|
|
|
|
with self.assertRaisesRegex(
|
|
ValueError, "may_alias and donate cannot be True at the same time."):
|
|
jax.device_put(arr, may_alias=True, donate=True)
|
|
|
|
out = jax.device_put(arr,
|
|
jax.sharding.SingleDeviceSharding(jax.devices()[0]),
|
|
may_alias=True, donate=False)
|
|
self.assertEqual(id(arr), id(out))
|
|
|
|
out = jax.device_put(arr,
|
|
jax.sharding.SingleDeviceSharding(jax.devices()[0]),
|
|
may_alias=False, donate=False)
|
|
self.assertNotEqual(id(arr), id(out))
|
|
|
|
@parameterized.named_parameters(
|
|
("argnums", "donate_argnums", 0),
|
|
("argnames", "donate_argnames", 'x'),
|
|
)
|
|
@jtu.device_supports_buffer_donation()
|
|
def test_jit_donate_weak_type(self, argnum_type, argnum_val):
|
|
# input has weak-type, output does not have weak-type
|
|
move = jit(lambda x: x.astype(int), **{argnum_type: argnum_val})
|
|
x = jnp.broadcast_to(2, (3,))
|
|
move(x)
|
|
self.assertDeleted(x)
|
|
|
|
@parameterized.named_parameters(
|
|
("argnums", "donate_argnums", (0,)),
|
|
("argnames", "donate_argnames", ('array',)),
|
|
)
|
|
def test_jnp_array_copy(self, argnum_type, argnum_val):
|
|
# https://github.com/jax-ml/jax/issues/3412
|
|
|
|
@partial(jit, **{argnum_type: argnum_val})
|
|
def _test(array):
|
|
return array.at[0].set(77)
|
|
|
|
x = jnp.asarray([0, 1])
|
|
x_copy = jnp.array(x, copy=True)
|
|
with jtu.ignore_warning():
|
|
_test(x) # donation
|
|
|
|
# Gives: RuntimeError: Invalid argument: CopyToHostAsync() called on invalid buffer.
|
|
print(x_copy) # doesn't crash
|
|
|
|
@jtu.device_supports_buffer_donation()
|
|
def test_specify_donate_argnums_and_argnames(self):
|
|
@partial(jax.jit, donate_argnums=0, donate_argnames=('inp2', 'inp3'))
|
|
def f(inp1, inp2, inp3):
|
|
return inp1 * 2, inp2 * 2, inp3 * 2
|
|
|
|
x = jnp.ones((2, 5)) * 4
|
|
y = jnp.ones((2, 5)) * 2
|
|
z = jnp.ones((2, 5))
|
|
|
|
f(x, inp2=y, inp3=z)
|
|
self.assertDeleted(x)
|
|
self.assertDeleted(y)
|
|
self.assertDeleted(z)
|
|
|
|
def test_resolve_argnums_signature_fail(self):
|
|
api_util.resolve_argnums(int, None, None, None, None, None) # doesn't crash
|
|
|
|
@jtu.device_supports_buffer_donation()
|
|
def test_donate_argnames_with_args(self):
|
|
@partial(jax.jit, donate_argnames='inp1')
|
|
def f(inp1):
|
|
return inp1 * 2
|
|
|
|
x = jax.device_put(jnp.ones((2, 5)) * 4, jax.devices()[0])
|
|
f(x)
|
|
self.assertDeleted(x)
|
|
|
|
@jtu.device_supports_buffer_donation()
|
|
def test_donate_argnums_with_kwargs(self):
|
|
@partial(jax.jit, donate_argnums=0)
|
|
def f(inp1):
|
|
return inp1 * 2
|
|
|
|
x = jax.device_put(jnp.ones((2, 5)) * 4, jax.devices()[0])
|
|
f(inp1=x)
|
|
self.assertDeleted(x)
|
|
|
|
def test_donate_args_info_aot(self):
|
|
def fn(x, y):
|
|
return jax.tree.map(lambda i: i * 2, x), y * 2
|
|
|
|
x = jax.device_put({"A": np.array(1.0), "B": np.array(2.0)},
|
|
jax.devices()[0])
|
|
y = jax.device_put(np.array(3.0), jax.devices()[0])
|
|
|
|
f = jax.jit(fn, donate_argnums=1)
|
|
lowered = f.lower(x, y)
|
|
args_info = lowered.args_info[0]
|
|
# x is not donated.
|
|
self.assertFalse(args_info[0]['A'].donated)
|
|
self.assertFalse(args_info[0]['B'].donated)
|
|
# y is donated.
|
|
self.assertTrue(args_info[1].donated)
|
|
|
|
g = jax.jit(fn, donate_argnums=0)
|
|
lowered = g.lower(x, y)
|
|
args_info = lowered.args_info[0]
|
|
# x is donated.
|
|
self.assertTrue(args_info[0]['A'].donated)
|
|
self.assertTrue(args_info[0]['B'].donated)
|
|
# y is not donated.
|
|
self.assertFalse(args_info[1].donated)
|
|
|
|
def test_double_donation(self):
|
|
def add(x, y):
|
|
return x + y
|
|
|
|
f = jax.jit(add, donate_argnums=(0,))
|
|
x = jnp.zeros((10,), jnp.float32)
|
|
|
|
with self.assertRaises(RuntimeError):
|
|
result = f(x, x)
|
|
result.block_until_ready()
|
|
|
|
@parameterized.named_parameters(
|
|
('argnames', {'donate_argnames': ('z', 'y')}),
|
|
('argnums', {'donate_argnums': (0, 1)})
|
|
)
|
|
def test_dict_donation(self, jit_kwargs):
|
|
@partial(jax.jit, **jit_kwargs)
|
|
def f(z, y, x):
|
|
return z, y, x
|
|
|
|
z = {'c': 3.}
|
|
y = {'b': 2.}
|
|
x = {'a': 1.}
|
|
|
|
_, kwargs_info = f.lower(z=z, y=y, x=x).args_info
|
|
self.assertTrue(kwargs_info['z']['c'].donated)
|
|
self.assertTrue(kwargs_info['y']['b'].donated)
|
|
self.assertFalse(kwargs_info['x']['a'].donated)
|
|
|
|
@parameterized.named_parameters(
|
|
('argnames', {'donate_argnames': ('z', 'y')}),
|
|
('argnums', {'donate_argnums': (0, 1)})
|
|
)
|
|
def test_dict_donation_args_kwargs(self, jit_kwargs):
|
|
@partial(jax.jit, **jit_kwargs)
|
|
def f(z, y, x):
|
|
return z, y, x
|
|
|
|
z = {'c': 3.}
|
|
y = {'b': 2.}
|
|
x = {'a': 1.}
|
|
|
|
args_info, kwargs_info = f.lower(z, y=y, x=x).args_info
|
|
self.assertTrue(args_info[0]['c'].donated)
|
|
self.assertTrue(kwargs_info['y']['b'].donated)
|
|
self.assertFalse(kwargs_info['x']['a'].donated)
|
|
|
|
def test_intersecting_static_and_donate_argnames(self):
|
|
with self.assertRaisesRegex(
|
|
ValueError, "static_argnames and donate_argnames cannot intersect"):
|
|
jax.jit(lambda x: x, static_argnames='x', donate_argnames='x')
|
|
|
|
def test_jit_global_cache(self):
|
|
def f(x):
|
|
assert python_should_be_executing
|
|
return x
|
|
|
|
python_should_be_executing = True
|
|
jit(f)(2)
|
|
python_should_be_executing = False
|
|
jit(f)(3)
|
|
|
|
@jtu.thread_unsafe_test() # GC effects aren't predictable with threads
|
|
def test_jit_cache_clear(self):
|
|
@jit
|
|
def f(x, y):
|
|
return x + y
|
|
|
|
client = jax.devices()[0].client
|
|
gc.collect()
|
|
num_live_initial = len(client.live_executables())
|
|
f(1, 2).block_until_ready()
|
|
gc.collect()
|
|
num_live = len(client.live_executables())
|
|
self.assertEqual(num_live_initial + 1, num_live)
|
|
f.clear_cache()
|
|
gc.collect()
|
|
num_live = len(client.live_executables())
|
|
self.assertEqual(num_live_initial, num_live)
|
|
|
|
def test_jit_shallow_copy(self):
|
|
def f(x):
|
|
return copy.copy(x)
|
|
jit(f)(1)
|
|
|
|
def test_jit_deep_copy(self):
|
|
def f(x):
|
|
return copy.deepcopy(x)
|
|
jit(f)(1)
|
|
|
|
def test_disable_jit(self):
|
|
effects = []
|
|
|
|
@jit
|
|
def f(x):
|
|
effects.append(1)
|
|
return x
|
|
|
|
with api.disable_jit():
|
|
f(2)
|
|
f(2)
|
|
assert len(effects) == 2
|
|
|
|
f(2)
|
|
f(2)
|
|
assert len(effects) == 3
|
|
|
|
def test_static_argnum_on_method(self):
|
|
|
|
class A:
|
|
|
|
@functools.partial(jit, static_argnums=(0,))
|
|
def my_func_jit(self, x):
|
|
return x+2
|
|
|
|
A().my_func_jit(3)
|
|
|
|
def test_static_argnum_on_static_method_is_not_supported(self):
|
|
with self.assertRaisesRegex(TypeError, "Expected a callable value"):
|
|
|
|
class A:
|
|
|
|
@functools.partial(jit, static_argnums=(0,))
|
|
@classmethod
|
|
def my_classmethod_jit(cls, x):
|
|
return x+2
|
|
|
|
def test_staticmethod_is_not_supported(self):
|
|
with self.assertRaisesRegex(TypeError,
|
|
"staticmethod arguments are not supported"):
|
|
|
|
class A:
|
|
|
|
@functools.partial(jit)
|
|
@staticmethod
|
|
def my_staticmethod_jit(x):
|
|
return x + 2
|
|
|
|
def test_concurrent_jit(self):
|
|
@jit
|
|
def f(x):
|
|
return x + x - 3.
|
|
|
|
xs = [self.rng().randn(i) for i in range(10)]
|
|
with concurrent.futures.ThreadPoolExecutor() as executor:
|
|
futures = [executor.submit(partial(f, x)) for x in xs]
|
|
ys = [f.result() for f in futures]
|
|
for x, y in zip(xs, ys):
|
|
self.assertAllClose(x * 2 - 3., y)
|
|
|
|
def test_trivial_computations(self):
|
|
x = jnp.array([1, 2, 3])
|
|
y = jit(lambda x: x)(x)
|
|
self.assertNotEqual(x.unsafe_buffer_pointer(), y.unsafe_buffer_pointer())
|
|
|
|
z1, z2 = jit(lambda x: (x, x))(x)
|
|
self.assertNotEqual(z1.unsafe_buffer_pointer(), z2.unsafe_buffer_pointer())
|
|
|
|
x1, x2 = jnp.array([1, 2]), jnp.array([2, 3])
|
|
z1, z2, z3 = jit(lambda x, y: (y, 1, x))(x1, x2)
|
|
self.assertNotEqual(z1.unsafe_buffer_pointer(), x2.unsafe_buffer_pointer())
|
|
self.assertNotEqual(z3.unsafe_buffer_pointer(), x1.unsafe_buffer_pointer())
|
|
self.assertEqual(z2, 1)
|
|
|
|
def test_print_token_buffer_error(self):
|
|
token = jax.lax.create_token()
|
|
with self.assertRaisesRegex(
|
|
RuntimeError, "Cannot convert a token-shape buffer to a numpy array."
|
|
):
|
|
token._buf._value
|
|
|
|
def test_trivial_computations_with_tokens(self):
|
|
@jit
|
|
def noop(arr, token):
|
|
return arr, token
|
|
|
|
arr = jnp.ones(10)
|
|
token = jax.lax.create_token()
|
|
_, out_token = noop(arr, token)
|
|
|
|
self.assertIsInstance(token, core.Token)
|
|
self.assertIsInstance(out_token, core.Token)
|
|
# Different token objects.
|
|
self.assertIsNot(token, out_token)
|
|
|
|
def test_jit_bad_input(self):
|
|
def f(x):
|
|
return x
|
|
|
|
err_str = ("Error interpreting argument to .* as an abstract array. The problematic "
|
|
"value is of type .* and was passed to the function at path x.")
|
|
with self.assertRaisesRegex(TypeError, err_str):
|
|
jit(f)("foo")
|
|
|
|
# Jax type objects aren't valid data arguments.
|
|
with self.assertRaisesRegex(TypeError, err_str):
|
|
jit(f)(jnp.int32)
|
|
|
|
def test_jit_masked_array(self):
|
|
x = np.ma.array([1, 2, 3], mask=[True, False, True])
|
|
f = jit(lambda x: x)
|
|
with self.assertRaisesRegex(ValueError, "numpy masked arrays are not supported"):
|
|
f(x)
|
|
|
|
def test_jit_on_all_devices(self):
|
|
# Verifies we can run the same computation on every device present, even
|
|
# if they are, for example, different models of GPU.
|
|
data = self.rng().rand(1000).astype(np.float32)
|
|
f = jit(jnp.negative)
|
|
for device in jax.local_devices():
|
|
x = device_put(data, device=device)
|
|
np.testing.assert_array_equal(-data, f(x))
|
|
|
|
def test_jit_nested_donate_ignored(self):
|
|
jit_fun = jit(lambda x: jit(lambda y: y**2, donate_argnums=0)(x))
|
|
a = jax.device_put(jnp.array(1))
|
|
|
|
# NOTE(mattjj): stopped raising error here and instead just ignored
|
|
# with self.assertRaisesRegex(ValueError, "nested.*not supported"):
|
|
# jit_fun(a)
|
|
|
|
jit_fun(a) # doesn't crash
|
|
|
|
def test_jit_reference_dropping(self):
|
|
x = jnp.ones(10)
|
|
f = (lambda x: lambda: x)(x) # reference to x in f's closure
|
|
g = jit(f)
|
|
x = weakref.ref(x) # no more strong ref to x in this scope
|
|
assert x() is not None # x is still around
|
|
f() # f runs
|
|
g() # g runs
|
|
g() # g runs a second time
|
|
del f # delete the raw callable
|
|
assert x() is not None # x is still around
|
|
g() # g still runs
|
|
del g # no more references to x
|
|
assert x() is None # x is gone
|
|
|
|
def test_jit_of_nonweakreferenceable_function(self):
|
|
class CallableWithSlots:
|
|
__slots__ = []
|
|
def __call__(self, x):
|
|
return x + 1
|
|
|
|
c = CallableWithSlots()
|
|
with self.assertRaisesRegex(TypeError, "cannot create weak reference.*"):
|
|
weakref.ref(c)
|
|
# Building a jit object does not crash.
|
|
f = jit(c)
|
|
with self.assertRaisesRegex(TypeError, "cannot create weak reference.*"):
|
|
# Calling the jit object will fail, but not because of the C++ JIT. The
|
|
# Python-level jit cache requires weak reference support.
|
|
f(3)
|
|
|
|
def test_jit_raises_on_first_invocation_on_non_hashable_static_argnum(self):
|
|
f = lambda x, y: x + 3
|
|
jitted_f = jit(f, static_argnums=(1,))
|
|
|
|
msg = "Non-hashable static arguments are not supported"
|
|
with self.assertRaisesRegex(ValueError, msg):
|
|
jitted_f(1, np.asarray(1))
|
|
|
|
def test_cpp_jit_raises_on_non_hashable_static_argnum(self):
|
|
f = lambda x, y: x + 3
|
|
jitted_f = jit(f, static_argnums=[1])
|
|
|
|
jitted_f(1, 1)
|
|
|
|
msg = "Non-hashable static arguments are not supported"
|
|
|
|
with self.assertRaisesRegex(ValueError, msg):
|
|
jitted_f(1, np.asarray(1))
|
|
|
|
class HashableWithoutEq:
|
|
|
|
def __hash__(self):
|
|
return 1
|
|
|
|
def __eq__(self, other):
|
|
raise NotImplementedError(
|
|
"A Python error is as is, without stack trace")
|
|
|
|
with self.assertRaisesRegex(
|
|
ValueError,
|
|
re.escape("static arguments should be comparable using __eq__")):
|
|
jitted_f(1, HashableWithoutEq())
|
|
# __eq__ would only be called if we might have a cache hit. Call the
|
|
# function a second time with exactly the same arguments to make sure that
|
|
# we could.
|
|
jitted_f(1, HashableWithoutEq())
|
|
|
|
def test_cpp_jit_raises_other_exceptions_when_hashing_fails(self):
|
|
class A:
|
|
def __hash__(self):
|
|
raise ValueError
|
|
|
|
f = jax.jit(lambda x: x + 1, static_argnums=(0,))
|
|
a = A()
|
|
with self.assertRaisesRegex(ValueError, '^$'): # no extra message
|
|
f(a)
|
|
|
|
def test_cpp_jitted_function_returns_PyBuffer(self):
|
|
jitted_f = jit(lambda a: a + 1)
|
|
jitted_f(1)
|
|
out = jitted_f(2)
|
|
self.assertIsInstance(out.sharding, jax.sharding.SingleDeviceSharding)
|
|
self.assertIsInstance(out, array.ArrayImpl)
|
|
|
|
@parameterized.named_parameters(
|
|
('jit', jax.jit),
|
|
('pjit', pjit.pjit)
|
|
)
|
|
@jtu.skip_on_devices("cpu")
|
|
def test_explicit_backend(self, module):
|
|
f = lambda x: x + 1
|
|
with jtu.ignore_warning(category=DeprecationWarning,
|
|
message="backend and device argument"):
|
|
jitted_f = module(f, backend=jtu.device_under_test())
|
|
jitted_f_cpu = module(f, backend="cpu")
|
|
|
|
result = jitted_f(1.)
|
|
result_cpu = jitted_f_cpu(1.)
|
|
self.assertEqual(list(result.devices())[0].platform, jtu.device_under_test())
|
|
self.assertEqual(list(result_cpu.devices())[0].platform, "cpu")
|
|
|
|
@parameterized.named_parameters(
|
|
('jit', jax.jit),
|
|
('pjit', pjit.pjit)
|
|
)
|
|
@jtu.skip_on_devices("cpu")
|
|
def test_device_to_device_copy_between_backends(self, module):
|
|
# b/186624243
|
|
f = lambda x: x + 1
|
|
with jtu.ignore_warning(category=DeprecationWarning,
|
|
message="backend and device argument"):
|
|
jitted_f = module(f, backend=jtu.device_under_test())
|
|
jitted_f_cpu = module(f, backend="cpu")
|
|
|
|
x = np.arange(30).reshape(1, 10, 3)
|
|
result = jitted_f(x)
|
|
result_cpu = jitted_f_cpu(result)
|
|
result_2 = jitted_f(result_cpu)
|
|
result_cpu_2 = jitted_f_cpu(result_2)
|
|
self.assertAllClose(result_2, x + 3)
|
|
self.assertAllClose(result_cpu_2, x + 4)
|
|
|
|
@jtu.skip_on_devices("cpu")
|
|
@jtu.ignore_warning(category=DeprecationWarning,
|
|
message="backend and device argument")
|
|
def test_mismatched_nested_backends(self):
|
|
@partial(jax.jit, backend=jtu.device_under_test())
|
|
def f(x):
|
|
return jax.jit(lambda x: x + 1, backend="cpu")(x)
|
|
|
|
msg = 'Received incompatible devices for jitted computation'
|
|
with self.assertRaisesRegex(ValueError, msg):
|
|
f(1.)
|
|
|
|
@jax.legacy_prng_key('allow')
|
|
def test_omnistaging(self):
|
|
# See https://github.com/jax-ml/jax/issues/5206
|
|
|
|
# TODO(frostig): remove `wrap` once we always enable_custom_prng
|
|
def wrap(arr):
|
|
arr = np.array(arr, dtype=np.uint32)
|
|
if config.enable_custom_prng.value:
|
|
return jax.random.wrap_key_data(arr)
|
|
else:
|
|
return arr
|
|
|
|
key_list = [None]
|
|
|
|
def init():
|
|
key, subkey = jax.random.split(key_list[0])
|
|
key_list[0] = key
|
|
return jax.random.normal(subkey, ())
|
|
|
|
key_list[0] = wrap([2384771982, 3928867769])
|
|
init()
|
|
jit(init)()
|
|
self.assertIsInstance(key_list[0], core.Tracer)
|
|
del key_list[0]
|
|
|
|
def test_jit_wrapped_attributes(self):
|
|
def f(x: int) -> int:
|
|
"""docstring of f."""
|
|
return x + 1
|
|
f.some_value = 4
|
|
jf = jit(f)
|
|
for attr in ["doc", "name", "module", "qualname", "annotations"]:
|
|
self.assertEqual(
|
|
{attr: getattr(f, f"__{attr}__")},
|
|
{attr: getattr(jf, f"__{attr}__")})
|
|
self.assertEqual(f.some_value, jf.some_value)
|
|
|
|
def test_jit_python_builtin(self):
|
|
x = jnp.array([1, 2])
|
|
expected = x + 1
|
|
jit_add = jit(operator.add, static_argnums=(1,))
|
|
actual = jit_add(x, 1)
|
|
self.assertArraysEqual(expected, actual)
|
|
|
|
def test_infer_argnums_and_argnames(self):
|
|
def f(x, y=1):
|
|
pass
|
|
|
|
sig = inspect.signature(f)
|
|
|
|
argnums, argnames = api_util.infer_argnums_and_argnames(
|
|
sig, argnums=None, argnames=None)
|
|
assert argnums == ()
|
|
assert argnames == ()
|
|
|
|
argnums, argnames = api_util.infer_argnums_and_argnames(
|
|
sig, argnums=0, argnames=None)
|
|
assert argnums == (0,)
|
|
assert argnames == ('x',)
|
|
|
|
argnums, argnames = api_util.infer_argnums_and_argnames(
|
|
sig, argnums=None, argnames='y')
|
|
assert argnums == (1,)
|
|
assert argnames == ('y',)
|
|
|
|
argnums, argnames = api_util.infer_argnums_and_argnames(
|
|
sig, argnums=0, argnames='y') # no validation
|
|
assert argnums == (0,)
|
|
assert argnames == ('y',)
|
|
|
|
def g(x, y, *args):
|
|
pass
|
|
|
|
sig = inspect.signature(g)
|
|
|
|
argnums, argnames = api_util.infer_argnums_and_argnames(
|
|
sig, argnums=(1, 2), argnames=None)
|
|
assert argnums == (1, 2)
|
|
assert argnames == ('y',)
|
|
|
|
def h(x, y, **kwargs):
|
|
pass
|
|
|
|
sig = inspect.signature(h)
|
|
|
|
argnums, argnames = api_util.infer_argnums_and_argnames(
|
|
sig, argnums=None, argnames=('foo', 'bar'))
|
|
assert argnums == ()
|
|
assert argnames == ('foo', 'bar')
|
|
|
|
def test_jit_with_static_argnames(self):
|
|
|
|
def f(x):
|
|
assert x == 'foo'
|
|
return 1
|
|
|
|
f_nums = jit(f, static_argnums=0)
|
|
assert f_nums('foo') == 1
|
|
assert f_nums(x='foo') == 1
|
|
|
|
f_names = jit(f, static_argnames='x')
|
|
assert f_names('foo') == 1
|
|
assert f_names(x='foo') == 1
|
|
|
|
def test_new_static_argnum_on_keyword_arguments(self):
|
|
f = jit(lambda x: x, static_argnums=0)
|
|
y = f(x=4)
|
|
assert y == 4
|
|
|
|
def test_new_static_argnum_with_default_arguments(self):
|
|
f = jit(lambda x=4: x, static_argnums=0)
|
|
y = f()
|
|
assert y == 4
|
|
|
|
def test_jit_with_mismatched_static_argnames(self):
|
|
x_is_tracer, y_is_tracer = False, False
|
|
def f(x, y):
|
|
assert isinstance(x, core.Tracer) == x_is_tracer
|
|
assert isinstance(y, core.Tracer) == y_is_tracer
|
|
return 1
|
|
|
|
# If both static_argnums and static_argnames are provided, they are allowed
|
|
# to disagree and `jit` will respect the user's choices.
|
|
f_nums = jit(f, static_argnums=1, static_argnames=())
|
|
x_is_tracer, y_is_tracer = True, False
|
|
assert f_nums(2, 'foo') == 1
|
|
x_is_tracer, y_is_tracer = True, True
|
|
assert f_nums(1, y=2) == 1
|
|
|
|
f_names = jit(f, static_argnums=(), static_argnames='y')
|
|
x_is_tracer, y_is_tracer = True, True
|
|
assert f_names(2, 3) == 1
|
|
x_is_tracer, y_is_tracer = True, False
|
|
assert f_names(1, y='foo') == 1
|
|
|
|
f_mixed = jit(f, static_argnums=(1,), static_argnames='x')
|
|
x_is_tracer, y_is_tracer = True, False
|
|
assert f_mixed(2, 'foo') == 1
|
|
x_is_tracer, y_is_tracer = True, True
|
|
assert f_mixed(1, y=3) == 1
|
|
x_is_tracer, y_is_tracer = False, True
|
|
assert f_mixed(x='foo', y=3) == 1
|
|
|
|
# TODO(zhangqiaorjc): Test pruning constants after DCE pass prunes primitive
|
|
# applications.
|
|
@parameterized.parameters(2, 3, 4)
|
|
def test_jit_with_pruned_args(self, num_args):
|
|
def f(*args):
|
|
used = np.array(2)
|
|
return args[1] + used
|
|
f_pruned = jit(f)
|
|
args = range(num_args)
|
|
with jtu.count_device_put() as count:
|
|
np.testing.assert_allclose(f_pruned(*args), 3)
|
|
self.assertEqual(count(), 1)
|
|
|
|
def testBuffersAreFreedPromptly(self):
|
|
# Regression test for a bug where garbage collection was delayed too long
|
|
# for NumPy buffers that are aliased zero-copy by the runtime.
|
|
@jit
|
|
def f(x):
|
|
return x + 1
|
|
|
|
refs = []
|
|
x = np.ones((10000,), np.float32)
|
|
for step in range(1000):
|
|
x = f(x)
|
|
refs.append(weakref.ref(x))
|
|
x = np.asarray(x)
|
|
|
|
# We expect most of the input buffers to have been garbage
|
|
# collected in parallel with the execution. We can't call
|
|
# block_until_ready() here because it would force a garbage collection.
|
|
live_refs = len([ref for ref in refs if ref() is not None])
|
|
self.assertLessEqual(live_refs, 100)
|
|
|
|
def test_jit_lower_compile(self):
|
|
def f(x):
|
|
return jnp.sqrt(x ** 2) + 1.
|
|
|
|
f_jit = jit(f)
|
|
lowered = f_jit.lower(1.)
|
|
compiled = lowered.compile()
|
|
self.assertAllClose(compiled(1.), 2.)
|
|
self.assertEqual(lowered.in_avals, compiled.in_avals)
|
|
expected_dtype = np.float64 if config.enable_x64.value else np.float32
|
|
for obj in [lowered, compiled]:
|
|
self.assertEqual(
|
|
obj.in_avals,
|
|
((core.ShapedArray([], expected_dtype, weak_type=True),), {}))
|
|
self.assertEqual(obj.in_tree, jax.tree.flatten(((0,), {}))[1])
|
|
|
|
def test_jit_lower_duck_typing(self):
|
|
f_jit = jit(lambda x: 2 * x)
|
|
f_low = f_jit.lower(jax.ShapeDtypeStruct((), 'float32')) # doesn't crash
|
|
f_exe = f_low.compile()
|
|
self.assertAllClose(f_exe(jnp.float32(1.)), jnp.float32(2.))
|
|
|
|
def test_jit_lower_compile_in_tree_mismatch(self):
|
|
def f(x):
|
|
return jnp.sqrt(x ** 2) + 1.
|
|
|
|
f_jit = jit(f)
|
|
f_low = f_jit.lower(1.)
|
|
f_exe = f_low.compile()
|
|
self.assertRaisesRegex(
|
|
TypeError,
|
|
'Function compiled with input pytree does not match the input pytree it'
|
|
' was called with',
|
|
lambda: f_exe([1.]))
|
|
|
|
def test_jit_lower_compile_trivial(self):
|
|
def f(x): return x
|
|
out = jit(f).lower(1.).compile()(4.)
|
|
self.assertAllClose(out, 4.)
|
|
|
|
def test_jit_lower_compile_sharding_computation(self):
|
|
s = jax.sharding.SingleDeviceSharding(jax.devices()[0])
|
|
def f(x): return jax.lax.with_sharding_constraint(x, s)
|
|
out = jit(f).lower(1.).compile()(4.)
|
|
self.assertAllClose(out, 4.)
|
|
|
|
def test_jit_lower_compile_trivial_in_tree_mismatch(self):
|
|
def f(x): return x
|
|
f_exe = jit(f).lower(1.).compile()
|
|
self.assertRaisesRegex(
|
|
TypeError,
|
|
"Function compiled with input pytree does not match the input pytree it"
|
|
" was called with",
|
|
lambda: f_exe([4.0]),
|
|
)
|
|
|
|
def test_jit_lower_compile_arg_type_mismatch(self):
|
|
def f(x):
|
|
return jnp.sqrt(x ** 2) + 1.
|
|
|
|
x = jnp.array(1, dtype=int)
|
|
x_f32 = x.astype(jnp.float32)
|
|
x_i32 = x.astype(jnp.int32)
|
|
f_exe = jit(f).lower(x_f32).compile()
|
|
self.assertRaisesRegex(
|
|
TypeError,
|
|
r"Argument types differ .*"
|
|
r"The mismatches are:\n"
|
|
r"Argument 'x' compiled with.*float32.*and called with.*int32.*",
|
|
lambda: f_exe(x_i32))
|
|
|
|
def test_jit_lower_compile_multi_arg(self):
|
|
def f(*args):
|
|
x, *_ = args
|
|
return jnp.sqrt(x ** 2) + 1.
|
|
f_exe = jit(f).lower(1., 1.).compile()
|
|
self.assertAllClose(f_exe(1., 1.), 2.)
|
|
|
|
def test_jit_lower_compile_trivial_multi_arg(self):
|
|
def f(*args):
|
|
x, *_ = args
|
|
return x
|
|
f_exe = jit(f).lower(1., 1.).compile()
|
|
self.assertAllClose(f_exe(1., 1.), 1.)
|
|
|
|
def test_jit_lower_donate_argnums_available(self):
|
|
def f(*args):
|
|
x, *_ = args
|
|
return x + 4.
|
|
f_low = jit(f, donate_argnums=(0,)).lower(1., 1.)
|
|
f_com = f_low.compile()
|
|
f_low.donate_argnums == f_com.donate_argnums == (0,)
|
|
|
|
def test_jit_lower_compile_vmap(self):
|
|
f = jit(lambda x: x + 4).lower(1.).compile()
|
|
def err():
|
|
return jax.vmap(lambda x: f(x) + 2)(jnp.ones(3))
|
|
self.assertRaisesRegex(
|
|
TypeError,
|
|
"Cannot apply JAX transformations to a function lowered and compiled "
|
|
"for a particular signature. Detected .*BatchTracer",
|
|
err)
|
|
|
|
def test_jit_lower_as_text(self):
|
|
f = jit(lambda x: x + 4).lower(1.)
|
|
self.assertIsInstance(f.as_text(), str)
|
|
self.assertIsInstance(f.as_text(dialect='hlo'), str)
|
|
self.assertIsInstance(f.as_text(dialect="stablehlo"), str)
|
|
|
|
def test_jit_lower_compiler_ir(self):
|
|
f = jit(lambda x: x + 4).lower(1.)
|
|
self.assertIsNotNone(f.compiler_ir())
|
|
self.assertIsNotNone(f.compiler_ir(dialect='hlo'))
|
|
self.assertIsNotNone(f.compiler_ir(dialect="stablehlo"))
|
|
|
|
def test_jit_lower_trivial_compiler_ir(self):
|
|
f = jit(lambda x: x).lower(1.)
|
|
self.assertIsNotNone(f.compiler_ir())
|
|
self.assertIsNotNone(f.compiler_ir(dialect='hlo'))
|
|
self.assertIsNotNone(f.compiler_ir(dialect="stablehlo"))
|
|
|
|
def test_jit_replica_attributes(self):
|
|
hlo = jit(lambda x: x + 4).lower(1.).as_text("stablehlo")
|
|
self.assertIn("mhlo.num_partitions = 1", hlo)
|
|
self.assertIn("mhlo.num_replicas = 1", hlo)
|
|
|
|
def test_jit_lower_no_pruning(self):
|
|
compiled = jit(lambda x, y: x + y).lower(1., 2.).compile()
|
|
self.assertEqual(compiled._executable._kept_var_idx, {0, 1})
|
|
self.assertLen(compiled._executable.in_avals, 2)
|
|
|
|
compiled = jit(lambda x, y: x).lower(1., 2.).compile()
|
|
self.assertEqual(compiled._executable._kept_var_idx, {0})
|
|
self.assertLen(compiled._executable.in_avals, 1)
|
|
|
|
compiled = jit(lambda x, y: x, keep_unused=True).lower(
|
|
1., 2.).compile()
|
|
self.assertEqual(compiled._executable._kept_var_idx, {0, 1})
|
|
self.assertLen(compiled._executable.in_avals, 2)
|
|
# Also works with jax.jit
|
|
jitted_f = jit(lambda x, y: x, keep_unused=True)
|
|
with jtu.count_pjit_cpp_cache_miss() as count:
|
|
_ = jitted_f(1, 2)
|
|
self.assertEqual(count(), 1)
|
|
|
|
def test_jit_lower_compile_compiler_ir(self):
|
|
f = jit(lambda x: x + 4).lower(1.).compile()
|
|
self.assertIsNotNone(f.runtime_executable())
|
|
|
|
def test_jit_lower_trivial_compile_compiler_ir(self):
|
|
f = jit(lambda x: x).lower(1.).compile()
|
|
self.assertIsNotNone(f.runtime_executable())
|
|
|
|
def test_jit_lower_compile_as_text(self):
|
|
f = jit(lambda x: x).lower(1.).compile()
|
|
g = jit(lambda x: x + 4).lower(1.).compile()
|
|
self.assertIsInstance(f.as_text(), (str, type(None)))
|
|
self.assertIsInstance(g.as_text(), (str, type(None)))
|
|
|
|
def test_jit_lower_cost_analysis(self):
|
|
# TODO(b/261771737): add support for uncompiled cost analysis in C API.
|
|
if "PJRT C API" in xla_bridge.get_backend().platform_version:
|
|
raise unittest.SkipTest("C API does not support uncompiled cost analysis")
|
|
f = jit(lambda x: x).lower(1.)
|
|
g = jit(lambda x: x + 4).lower(1.)
|
|
f.cost_analysis() # doesn't raise
|
|
g.cost_analysis() # doesn't raise
|
|
|
|
def test_jit_lower_compile_cost_analysis(self):
|
|
f = jit(lambda x: x).lower(1.).compile()
|
|
g = jit(lambda x: x + 4).lower(1.).compile()
|
|
self.assertIsNotNone(f.cost_analysis())
|
|
self.assertIsNotNone(g.cost_analysis())
|
|
|
|
def test_jit_lower_compile_memory_analysis(self):
|
|
f = jit(lambda x: x).lower(1.).compile()
|
|
g = jit(lambda x: x + 4).lower(1.).compile()
|
|
f.memory_analysis() # doesn't raise
|
|
g.memory_analysis() # doesn't raise
|
|
|
|
def test_jit_lower_compile_executable(self):
|
|
f = jit(lambda x: x).lower(1.).compile()
|
|
g = jit(lambda x: x + 4).lower(1.).compile()
|
|
self.assertIsNotNone(f.runtime_executable())
|
|
self.assertIsNotNone(g.runtime_executable())
|
|
|
|
def test_jit_lower_compile_with_compiler_options(self):
|
|
def f(x):
|
|
return jnp.sqrt(x ** 2) + 1.
|
|
|
|
f_jit = jit(f)
|
|
lowered = f_jit.lower(1.)
|
|
lowered.compile( # doesn't crash
|
|
compiler_options={
|
|
"xla_embed_ir_in_executable": True,
|
|
"xla_dump_max_hlo_modules": 200,
|
|
"xla_gpu_auto_spmd_partitioning_memory_budget_ratio": 0.5,
|
|
}
|
|
)
|
|
|
|
def test_compile_options_jit(self):
|
|
def f(x):
|
|
return jnp.sqrt(x ** 2) + 1.
|
|
|
|
f_jit = jit(
|
|
f,
|
|
compiler_options={
|
|
"xla_embed_ir_in_executable": True,
|
|
"xla_dump_max_hlo_modules": 200,
|
|
"xla_gpu_auto_spmd_partitioning_memory_budget_ratio": 0.5,
|
|
})(1.0) # doesn't crash.
|
|
|
|
def test_exec_time_optimization_effort_compiler_option(self):
|
|
def f(x):
|
|
return jnp.sqrt(x ** 2) + 1.
|
|
|
|
f_jit = jit(
|
|
f,
|
|
compiler_options={
|
|
"exec_time_optimization_effort": 0.0,
|
|
})(1.0) # doesn't crash.
|
|
|
|
with self.assertRaisesRegex(xla_extension.XlaRuntimeError, "No such"):
|
|
f_jit = jit(
|
|
f,
|
|
compiler_options={
|
|
"exec_time_compilation_effort": 0.0,
|
|
})(1.0)
|
|
|
|
def test_jit_lower_compile_with_compiler_options_invalid(self):
|
|
def f(x):
|
|
return jnp.sqrt(x ** 2) + 1.
|
|
|
|
f_jit = jit(f)
|
|
lowered = f_jit.lower(1.)
|
|
|
|
self.assertRaisesRegex(
|
|
xla_extension.XlaRuntimeError, "No such compile option: 'invalid_key'",
|
|
lambda: lowered.compile(
|
|
compiler_options={"invalid_key": "invalid_value"}))
|
|
|
|
self.assertRaisesRegex(
|
|
xla_extension.XlaRuntimeError, "is not a valid bool value.",
|
|
lambda: lowered.compile(
|
|
compiler_options={"xla_embed_ir_in_executable": "invalid_value"}))
|
|
|
|
def test_jit_compile_with_compiler_options_multiple(self):
|
|
def f(x):
|
|
return jnp.sqrt(x ** 2) + 1.
|
|
|
|
with jtu.count_jit_compilation_cache_miss() as count:
|
|
jit(f, compiler_options={"xla_embed_ir_in_executable": True})(1.)
|
|
jit(f, compiler_options={"xla_embed_ir_in_executable": False})(1.)
|
|
self.assertEqual(count(), 2)
|
|
|
|
# We should still error on invalid options after some valid compiles
|
|
with self.assertRaisesRegex(
|
|
xla_extension.XlaRuntimeError, "No such compile option: 'invalid_key'"):
|
|
jit(f, compiler_options={"invalid_key": "invalid_value"})(1.)
|
|
|
|
def test_lower_compile_with_compiler_options_multiple(self):
|
|
def f(x):
|
|
return jnp.sqrt(x ** 2) + 1.
|
|
|
|
f_jit = jit(f)
|
|
lowered = f_jit.lower(1.)
|
|
|
|
l1 = lowered.compile()
|
|
l2 = lowered.compile(
|
|
compiler_options={"xla_embed_ir_in_executable": True})
|
|
l3 = lowered.compile(
|
|
compiler_options={"xla_embed_ir_in_executable": False})
|
|
|
|
# Ideally we could test that these objects are different only in
|
|
# that they respect the different options. Object identity is a
|
|
# heuristic proxy for that.
|
|
self.assertTrue(l1 is not l2)
|
|
self.assertTrue(l1 is not l3)
|
|
self.assertTrue(l2 is not l3)
|
|
|
|
# We should still error on invalid options after some valid compiles
|
|
self.assertRaisesRegex(
|
|
xla_extension.XlaRuntimeError, "No such compile option: 'invalid_key'",
|
|
lambda: lowered.compile(
|
|
compiler_options={"invalid_key": "invalid_value"}))
|
|
|
|
def test_jit_enum_as_dict_keys_fails(self):
|
|
class E(enum.Enum):
|
|
A = 0
|
|
B = 1
|
|
|
|
@jit
|
|
def f(d) -> float:
|
|
return d[E.A]
|
|
|
|
with self.assertRaisesRegex(
|
|
(TypeError, ValueError),
|
|
"('<' not supported|Comparator raised exception).*"):
|
|
f({E.A: 1.0, E.B: 2.0})
|
|
|
|
def test_jit_static_argnums_requires_type_equality(self):
|
|
# See: https://github.com/jax-ml/jax/pull/9311
|
|
@partial(jit, static_argnums=(0,))
|
|
def f(k):
|
|
assert python_should_be_executing
|
|
return k
|
|
|
|
# Values of 'x' that compare as equal but have different types do not lead
|
|
# to cache hits.
|
|
for x in [1, True, 1.0]:
|
|
python_should_be_executing = True
|
|
self.assertEqual(x, f(x))
|
|
python_should_be_executing = False
|
|
self.assertEqual(x, f(x))
|
|
|
|
def test_caches_depend_on_axis_env(self):
|
|
# https://github.com/jax-ml/jax/issues/9187
|
|
f = lambda: lax.psum(1, "i")
|
|
g = jax.jit(f)
|
|
expected = jax.vmap(f, axis_name="i", axis_size=2, out_axes=None)()
|
|
ans = jax.vmap(g, axis_name="i", axis_size=2, out_axes=None)()
|
|
self.assertEqual(ans, expected)
|
|
|
|
# This second call to g could erroneously get a cache hit.
|
|
expected = jax.vmap(f, axis_name="i", axis_size=3, out_axes=None)()
|
|
ans = jax.vmap(g, axis_name="i", axis_size=3, out_axes=None)()
|
|
self.assertEqual(ans, expected)
|
|
|
|
# Since stackless, the vmap(f) version gets compiled a second time
|
|
@unittest.skip
|
|
def test_caches_dont_depend_on_unnamed_axis_env(self):
|
|
# https://github.com/jax-ml/jax/issues/9187
|
|
f = jax.jit(lambda: jnp.sin(1))
|
|
expected = f()
|
|
with jtu.count_jit_and_pmap_lowerings() as count: # noqa: F841
|
|
ans = jax.vmap(f, axis_size=2, out_axes=None)()
|
|
self.assertEqual(count(), 0) # no compiles
|
|
self.assertArraysAllClose(ans, expected, check_dtypes=True)
|
|
|
|
def test_cache_key_defaults(self):
|
|
# https://github.com/jax-ml/jax/discussions/11875
|
|
f = jit(lambda x: (x ** 2).sum())
|
|
self.assertEqual(f._cache_size(), 0)
|
|
x = jnp.arange(5.0)
|
|
for _ in range(3):
|
|
_ = f(x)
|
|
self.assertEqual(f._cache_size(), 1)
|
|
|
|
def test_jit_nan_times_zero(self):
|
|
# https://github.com/jax-ml/jax/issues/4780
|
|
def f(x):
|
|
return 1 + x * 0
|
|
self.assertAllClose(f(np.nan), np.nan)
|
|
self.assertAllClose(jit(f)(np.nan), np.nan)
|
|
|
|
def test_no_tracing(self):
|
|
@jax.jit
|
|
def f(x):
|
|
return x
|
|
|
|
x = jnp.arange(3)
|
|
y = jnp.arange(4)
|
|
|
|
_ = f(x) # no crash
|
|
|
|
with self.assertRaisesRegex(RuntimeError, 'no_tracing'):
|
|
with jax.no_tracing():
|
|
_ = f(y) # crash!
|
|
|
|
|
|
class APITest(jtu.JaxTestCase):
|
|
|
|
def test_grad_item(self):
|
|
def f(x):
|
|
if x.astype(bool).item():
|
|
return x ** 2
|
|
else:
|
|
return x
|
|
out = jax.grad(f)(2.0)
|
|
self.assertEqual(out, 4)
|
|
|
|
def test_jit_item(self):
|
|
def f(x):
|
|
return x.item()
|
|
x = jnp.array(1.0)
|
|
self.assertEqual(f(x), x)
|
|
with self.assertRaisesRegex(core.ConcretizationTypeError, "Abstract tracer value"):
|
|
jax.jit(f)(x)
|
|
|
|
@parameterized.named_parameters(
|
|
('grad', jax.grad),
|
|
('jacfwd', jax.jacfwd),
|
|
('jacref', jax.jacrev),
|
|
)
|
|
def test_grad_wrap(self, transform):
|
|
# Ensures that transforms wrap transformed functions with the correct signature.
|
|
|
|
@partial(jit, static_argnames=['flag'])
|
|
@transform
|
|
def my_function(x, flag):
|
|
return x if flag else jnp.zeros_like(x)
|
|
|
|
self.assertEqual(my_function(1.0, False), 0.0)
|
|
self.assertEqual(my_function(1.0, True), 1.0)
|
|
|
|
def test_grad_bad_input(self):
|
|
def f(x):
|
|
return x
|
|
|
|
self.assertRaisesRegex(
|
|
TypeError, ".* 'foo' of type <.*'str'> is not a valid JAX type",
|
|
lambda: grad(f)("foo"))
|
|
|
|
def test_grad_argnums(self):
|
|
def f(x, y, z, flag=False):
|
|
assert flag
|
|
return 1.0 * x + 2.0 * y + 3.0 * z
|
|
|
|
assert grad(f)(1.0, 1.0, 1.0, flag=True) == 1.0
|
|
assert grad(f, argnums=1)(1.0, 1.0, 1.0, flag=True) == 2.0
|
|
assert grad(f, argnums=(2, 0))(1.0, 1.0, 1.0, flag=True) == (3.0, 1.0)
|
|
|
|
def test_value_and_grad_argnums(self):
|
|
def f(x, y, z, flag=False):
|
|
assert flag
|
|
return 1.0 * x + 2.0 * y + 3.0 * z
|
|
|
|
y = f(1.0, 1.0, 1.0, flag=True)
|
|
assert api.value_and_grad(f)(1.0, 1.0, 1.0, flag=True) == (y, 1.0)
|
|
assert api.value_and_grad(f, argnums=1)(1.0, 1.0, 1.0, flag=True) == (y, 2.0)
|
|
assert api.value_and_grad(f, argnums=(2, 0))(1.0, 1.0, 1.0, flag=True) == (y, (3.0, 1.0))
|
|
|
|
@jtu.thread_unsafe_test() # Concurrent cache eviction means we may retrace.
|
|
def test_grad_of_jit(self):
|
|
side = []
|
|
|
|
@jit
|
|
def f(x):
|
|
side.append(None)
|
|
return x * x
|
|
|
|
assert grad(f)(1.0) == 2.0
|
|
assert len(side) == 1
|
|
assert grad(f)(2.0) == 4.0
|
|
assert len(side) == 1
|
|
|
|
@jtu.thread_unsafe_test() # Concurrent ache eviction means we may retrace.
|
|
def test_jit_of_grad(self):
|
|
side = []
|
|
|
|
@jit
|
|
def f(x):
|
|
side.append(None)
|
|
return x * x
|
|
|
|
g = jit(grad(f))
|
|
assert g(1.0) == 2.0
|
|
assert len(side) == 1
|
|
assert g(2.0) == 4.0
|
|
assert len(side) == 1
|
|
|
|
@parameterized.named_parameters(
|
|
{"testcase_name": f"_{transform.__name__}", "transform": transform}
|
|
for transform in [grad, jacfwd, jacrev])
|
|
def test_ad_weak_types(self, transform):
|
|
out = transform(lambda x: x)(1.0)
|
|
self.assertTrue(dtypes.is_weakly_typed(out))
|
|
|
|
def test_bad_input(self):
|
|
def f(x):
|
|
return x
|
|
|
|
with self.assertRaisesRegex(TypeError, ".* 'foo' of type <.*'str'> is not a valid JAX type"):
|
|
grad(f)("foo")
|
|
|
|
|
|
err_str = ("Error interpreting argument to .* as an abstract array. The problematic "
|
|
"value is of type .* and was passed to the function at path x.")
|
|
with self.assertRaisesRegex(TypeError, err_str):
|
|
jit(f)("foo")
|
|
|
|
def test_grad_tuple_output(self):
|
|
jtu.check_raises(lambda: grad(lambda x: (x,x))(1.0), TypeError,
|
|
"Gradient only defined for scalar-output functions. ")
|
|
|
|
def test_grad_unit_output(self):
|
|
jtu.check_raises(lambda: grad(lambda x: ())(np.zeros(3)), TypeError,
|
|
"Gradient only defined for scalar-output functions. ")
|
|
|
|
def test_grad_nonscalar_output(self):
|
|
jtu.check_raises(lambda: grad(lambda x: x)(np.zeros(3)), TypeError,
|
|
"Gradient only defined for scalar-output functions. ")
|
|
|
|
def test_unwrapped_numpy(self):
|
|
def f(x):
|
|
return np.exp(x)
|
|
|
|
with self.assertRaisesRegex(Exception, "The numpy.ndarray conversion .*"):
|
|
grad(f)(np.zeros(3))
|
|
|
|
def test_binop_mismatch(self):
|
|
def f(x, y):
|
|
return x + y
|
|
|
|
jtu.check_raises(
|
|
lambda: f(jnp.zeros(3), jnp.zeros(4)),
|
|
TypeError,
|
|
"add got incompatible shapes for broadcasting: (3,), (4,).")
|
|
|
|
jtu.check_raises(
|
|
lambda: grad(f)(np.zeros(3), np.zeros(4)),
|
|
TypeError,
|
|
"add got incompatible shapes for broadcasting: (3,), (4,).")
|
|
|
|
def test_dot_mismatch(self):
|
|
def f(x, y):
|
|
return jnp.dot(x, y)
|
|
|
|
self.assertRaisesRegex(
|
|
TypeError, ("dot_general requires contracting dimensions to have "
|
|
"the same shape, got \\(3L?,\\) and \\(4L?,\\)."),
|
|
lambda: grad(f)(np.zeros(3), np.zeros(4)))
|
|
|
|
def test_abstract_error_message(self):
|
|
for castfun in [float, complex, int]:
|
|
def f(x):
|
|
return castfun(x)
|
|
|
|
self.assertRaisesRegex(
|
|
TypeError,
|
|
f"[Tt]ry using `x.astype\\({castfun.__name__}\\)`",
|
|
lambda: jit(f)(1.0))
|
|
|
|
def test_switch_value_jit(self):
|
|
def f(x):
|
|
y = x > 0
|
|
if y:
|
|
return x
|
|
else:
|
|
return -x
|
|
|
|
assert grad(f)(1.0) == 1.0
|
|
assert grad(f)(-1.0) == -1.0
|
|
with self.assertRaisesRegex(core.ConcretizationTypeError,
|
|
"Attempted boolean conversion"):
|
|
jit(f)(1)
|
|
|
|
def test_list_index_err(self):
|
|
L = [1, 2, 3]
|
|
def f(n):
|
|
return L[n]
|
|
|
|
assert jit(f, static_argnums=(0,))(0) == L[0]
|
|
self.assertRaisesRegex(
|
|
TypeError,
|
|
r"The __index__\(\) method was called on traced array.*",
|
|
lambda: jit(f)(0))
|
|
|
|
def test_range_err(self):
|
|
def f(x, n):
|
|
for i in range(n):
|
|
x = x + i
|
|
return x
|
|
|
|
assert jit(f, static_argnums=(1,))(0, 5) == 10
|
|
self.assertRaisesRegex(
|
|
TypeError,
|
|
r"The __index__\(\) method was called on traced array.*",
|
|
lambda: jit(f)(0, 5))
|
|
|
|
def test_cast_int(self):
|
|
f = lambda x: int(x)
|
|
self.assertRaisesRegex(
|
|
TypeError,
|
|
"('(?:JaxprTracer|DynamicJaxprTracer)' object cannot be interpreted as an integer"
|
|
"|Abstract tracer value encountered where concrete value is expected.*)", lambda: jit(f)(0))
|
|
|
|
def test_casts(self):
|
|
for castfun in [hex, oct]:
|
|
f = lambda x: castfun(x)
|
|
self.assertRaisesRegex(
|
|
TypeError,
|
|
r"The __index__\(\) method was called on traced array.*", lambda: jit(f)(0))
|
|
|
|
def test_unimplemented_interpreter_rules(self):
|
|
foo_p = core.Primitive('foo')
|
|
def foo(x):
|
|
return foo_p.bind(x)
|
|
|
|
jtu.check_raises(lambda: foo(1.0), NotImplementedError,
|
|
"Evaluation rule for 'foo' not implemented")
|
|
|
|
jtu.check_raises(lambda: jit(foo)(1.0), NotImplementedError,
|
|
"Abstract evaluation for 'foo' not implemented")
|
|
|
|
jtu.check_raises(lambda: grad(foo)(1.0), NotImplementedError,
|
|
"Differentiation rule for 'foo' not implemented")
|
|
|
|
foo_p.def_abstract_eval(lambda x: x)
|
|
|
|
jtu.check_raises_regexp(lambda: jit(foo)(1.0), NotImplementedError,
|
|
".* rule for primitive 'foo' not found.*")
|
|
|
|
foo_p.def_impl(lambda x: x)
|
|
ad.defjvp(foo_p, lambda g, x: foo(g))
|
|
|
|
jtu.check_raises(lambda: grad(foo)(1.0), NotImplementedError,
|
|
"Transpose rule (for reverse-mode differentiation) for 'foo' not implemented")
|
|
|
|
def test_wrong_output_abstract_eval(self):
|
|
foo_p = core.Primitive('foo')
|
|
def foo(x):
|
|
return foo_p.bind(x)
|
|
foo_p.def_abstract_eval(lambda x: [x]) # Shouldn't return a list.
|
|
|
|
foo_p.def_impl(lambda x: x)
|
|
jitted = jit(lambda x: foo(x))
|
|
jtu.check_raises(lambda: jitted(1.0), ValueError,
|
|
"foo.abstract_eval() method should return a tuple or")
|
|
|
|
foo2_p = core.Primitive('foo2')
|
|
foo2_p.multiple_results = True
|
|
def foo2(x):
|
|
return foo2_p.bind(x),
|
|
|
|
foo2_p.def_abstract_eval(lambda x: x) # Should return a list.
|
|
foo2_p.def_impl(lambda x: [x])
|
|
jitted = jit(lambda x: foo2(x))
|
|
jtu.check_raises(lambda: jitted(1.0), ValueError,
|
|
"foo2.abstract_eval() method should return a tuple or")
|
|
|
|
def test_is_subclass(self):
|
|
self.assertFalse(issubclass(np.ndarray, jax.Array))
|
|
|
|
def test_is_instance(self):
|
|
def f(x):
|
|
self.assertIsInstance(x, jax.Array)
|
|
self.assertNotIsInstance(x, np.ndarray)
|
|
return x + 2
|
|
jit(f)(3)
|
|
jax.vmap(f)(np.arange(3))
|
|
|
|
def test_device_put_and_get(self):
|
|
x = np.arange(12.).reshape((3, 4)).astype("float32")
|
|
dx = api.device_put(x)
|
|
_check_instance(self, dx)
|
|
self.assertIsInstance(dx, jax.Array)
|
|
self.assertNotIsInstance(dx, np.ndarray)
|
|
x2 = api.device_get(dx)
|
|
self.assertNotIsInstance(x2, jax.Array)
|
|
self.assertIsInstance(x2, np.ndarray)
|
|
assert np.all(x == x2)
|
|
|
|
y = [x, (2 * x, 3 * x)]
|
|
dy = api.device_put(y)
|
|
y2 = api.device_get(dy)
|
|
self.assertIsInstance(y2, list)
|
|
self.assertIsInstance(y2[0], np.ndarray)
|
|
assert np.all(y2[0] == x)
|
|
self.assertIsInstance(y2[1], tuple)
|
|
self.assertIsInstance(y2[1][0], np.ndarray)
|
|
assert np.all(y2[1][0] == 2 * x)
|
|
self.assertIsInstance(y2[1][1], np.ndarray)
|
|
assert np.all(y2[1][1] == 3 * x)
|
|
|
|
def test_device_put_sharding(self):
|
|
mesh = jax.sharding.Mesh(jax.devices(), ('x',))
|
|
s = jax.NamedSharding(mesh, P('x'))
|
|
x = jnp.arange(len(jax.devices()))
|
|
|
|
y = jax.device_put(x, s)
|
|
self.assertEqual(y.sharding, s)
|
|
self.assertArraysAllClose(y, x)
|
|
|
|
# this might hit a special fast path
|
|
z = jax.device_put(y, s)
|
|
self.assertEqual(z.sharding, s)
|
|
self.assertArraysAllClose(z, x)
|
|
self.assertIs(z, y) # no copy
|
|
|
|
w = jax.device_put(z)
|
|
self.assertIs(w, z)
|
|
|
|
u = jax.device_put(y, jax.devices()[0])
|
|
self.assertArraysAllClose(u, y)
|
|
self.assertEqual(u.devices(), {jax.devices()[0]})
|
|
|
|
def test_device_put_sharding_tree(self):
|
|
if jax.device_count() < 2:
|
|
raise unittest.SkipTest("Test requires >= 2 devices")
|
|
|
|
mesh = jax.sharding.Mesh(np.array(jax.devices()[:2]).reshape((2, 1)),
|
|
("x", "y"))
|
|
s1 = jax.NamedSharding(mesh, P("x"))
|
|
s2 = jax.NamedSharding(mesh, P("y"))
|
|
s3 = jax.NamedSharding(mesh, P("x", "y"))
|
|
|
|
x = jnp.arange(2)
|
|
y = jnp.arange(2) + 10
|
|
z = (jnp.arange(2) + 100).reshape((2, 1))
|
|
|
|
out = jax.device_put((x, (y, z)), device=(s1, (s2, s3)))
|
|
self.assertEqual(out[0].sharding, s1)
|
|
self.assertEqual(out[1][0].sharding, s2)
|
|
self.assertEqual(out[1][1].sharding, s3)
|
|
|
|
self.assertArraysAllClose(out[0], x)
|
|
self.assertArraysAllClose(out[1][0], y)
|
|
self.assertArraysAllClose(out[1][1], z)
|
|
|
|
def test_device_put_sharding_tree_prefix(self):
|
|
if jax.device_count() < 2:
|
|
raise unittest.SkipTest("Test requires >= 2 devices")
|
|
|
|
mesh = jax.sharding.Mesh(np.array(jax.devices()[:2]).reshape((2, 1)), ("x", "y"))
|
|
s1 = jax.sharding.NamedSharding(mesh, P("x"))
|
|
s2 = jax.sharding.NamedSharding(mesh, P("y"))
|
|
|
|
x = jnp.arange(2)
|
|
y = jnp.arange(2) + 10
|
|
z = jnp.arange(2) + 100
|
|
|
|
out = jax.device_put((x, (y, z)), device=(s1, s2))
|
|
self.assertEqual(out[0].sharding, s1)
|
|
self.assertEqual(out[1][0].sharding, s2)
|
|
self.assertEqual(out[1][1].sharding, s2)
|
|
|
|
self.assertArraysAllClose(out[0], x)
|
|
self.assertArraysAllClose(out[1][0], y)
|
|
self.assertArraysAllClose(out[1][1], z)
|
|
|
|
def test_device_put_sharding_mismatched_tree_same_leaf_count(self):
|
|
if jax.device_count() < 2:
|
|
raise unittest.SkipTest("Test requires >= 2 devices")
|
|
|
|
mesh = jax.sharding.Mesh(np.array(jax.devices()[:2]).reshape((2, 1)), ("x", "y"))
|
|
s1 = jax.sharding.NamedSharding(mesh, P("x"))
|
|
s2 = jax.sharding.NamedSharding(mesh, P("y"))
|
|
|
|
x = jnp.arange(2)
|
|
y = jnp.arange(2) + 10
|
|
z = jnp.arange(2) + 100
|
|
|
|
with self.assertRaisesRegex(
|
|
ValueError,
|
|
"device_put device specification must be a tree prefix of the "
|
|
r"corresponding value, got specification \(\(NamedSharding\(.*\), "
|
|
r"NamedSharding\(.*\)\), NamedSharding\(.*\)\) for value tree "
|
|
r"PyTreeDef\(\(\*, \(\*, \*\)\)\)."
|
|
):
|
|
jax.device_put((x, (y, z)), device=((s1, s2), s2))
|
|
|
|
def test_device_put_sharding_mismatched_tree_different_leaf_count(self):
|
|
if jax.device_count() < 2:
|
|
raise unittest.SkipTest("Test requires >= 2 devices")
|
|
|
|
mesh = jax.sharding.Mesh(np.array(jax.devices()[:2]).reshape((2, 1)), ("x", "y"))
|
|
s1 = jax.sharding.NamedSharding(mesh, P("x"))
|
|
s2 = jax.sharding.NamedSharding(mesh, P("y"))
|
|
|
|
x = jnp.arange(2)
|
|
y = jnp.arange(2) + 10
|
|
z = jnp.arange(2) + 100
|
|
|
|
with self.assertRaisesRegex(
|
|
ValueError,
|
|
"device_put device specification must be a tree prefix of the "
|
|
r"corresponding value, got specification \(NamedSharding\(.*\), "
|
|
r"NamedSharding\(.*\)\) for value tree PyTreeDef\(\(\*, \*, \*\)\)."
|
|
):
|
|
jax.device_put((x, y, z), device=(s1, s2))
|
|
|
|
def test_device_put_custom_type_not_accepting_none_leaves(self):
|
|
|
|
class CustomNode(list):
|
|
pass
|
|
|
|
def unflatten(unused_aux_data, children):
|
|
self.assertIsNotNone(children[0])
|
|
return CustomNode(children)
|
|
|
|
tree_util.register_pytree_node(CustomNode, lambda x: (x, None), unflatten)
|
|
jax.device_put(CustomNode([0.1]))
|
|
|
|
def test_vmap_inconsistent_sizes_constructs_proper_error_message(self):
|
|
def f(x1, x2, g):
|
|
return g(x1, x2)
|
|
|
|
with self.assertRaisesRegex(
|
|
ValueError,
|
|
"vmap got inconsistent sizes for array axes to be mapped:"
|
|
):
|
|
jax.vmap(f, (0, 0, None))(jnp.ones(2), jnp.ones(3), jnp.add)
|
|
|
|
def test_vmap_inconsistent_sizes_constructs_proper_error_message_kwargs(self):
|
|
# regression test for https://github.com/jax-ml/jax/issues/24406
|
|
def f(x1, x2, a3):
|
|
return x1 + x2 + a3
|
|
|
|
with self.assertRaisesRegex(
|
|
ValueError,
|
|
"vmap got inconsistent sizes for array axes to be mapped:\n"
|
|
r" \* most axes \(2 of them\) had size 2, e.g. axis 0 of argument x1 of type float32\[2\];\n"
|
|
r" \* one axis had size 1: axis 0 of kwargs\['a3'\] of type float32\[1\]",
|
|
):
|
|
jax.vmap(f)(
|
|
jnp.ones(2, dtype=jnp.float32),
|
|
a3=jnp.ones(1, dtype=jnp.float32),
|
|
x2=jnp.ones(2, dtype=jnp.float32)
|
|
)
|
|
|
|
def test_device_get_scalar(self):
|
|
x = np.arange(12.).reshape((3, 4)).astype("float32")
|
|
x = api.device_put(x)
|
|
_check_instance(self, x)
|
|
self.assertIsInstance(x.sharding, jax.sharding.SingleDeviceSharding)
|
|
for s in x.addressable_shards:
|
|
self.assertArraysEqual(s.data, x)
|
|
self.assertEqual(s.replica_id, 0)
|
|
self.assertEqual(s.index, (slice(None), slice(None)))
|
|
y = [x, 2]
|
|
y2 = api.device_get(y)
|
|
self.assertIsInstance(y2, list)
|
|
self.assertIsInstance(y2[0], np.ndarray)
|
|
assert np.all(y2[0] == x)
|
|
self.assertIsInstance(y2[1], int)
|
|
self.assertEqual(y2[1], 2)
|
|
|
|
@parameterized.parameters([(3,)], [(2, 0)])
|
|
def test_device_put_across_devices(self, shape):
|
|
if len(jax.local_devices()) < 2:
|
|
raise unittest.SkipTest("this test requires multiple devices")
|
|
d1, d2 = jax.local_devices()[:2]
|
|
data = self.rng().randn(*shape).astype(np.float32)
|
|
x = api.device_put(data, device=d1)
|
|
self.assertEqual(x.devices(), {d1})
|
|
|
|
y = api.device_put(x, device=d2)
|
|
self.assertEqual(y.devices(), {d2})
|
|
|
|
np.testing.assert_array_equal(data, np.array(y))
|
|
# Make sure these don't crash
|
|
api.device_put(x)
|
|
api.device_put(y)
|
|
|
|
@jtu.skip_on_devices("cpu")
|
|
def test_device_put_across_platforms(self):
|
|
default_device = jax.devices()[0]
|
|
cpu_device = jax.devices("cpu")[0]
|
|
|
|
np_arr = np.array([1,2,3])
|
|
scalar = 1
|
|
device_arr = jnp.array([1,2,3])
|
|
assert device_arr.devices() == {default_device}
|
|
|
|
for val in [np_arr, device_arr, scalar]:
|
|
x = api.device_put(val, device=cpu_device)
|
|
self.assertEqual(x.devices(), {cpu_device})
|
|
|
|
def test_device_put_on_single_device_donated_buffer_fails(self):
|
|
@partial(jax.jit, donate_argnums=0)
|
|
def f(inp1):
|
|
return inp1 * 2
|
|
|
|
x = jnp.zeros((10,), jnp.float32)
|
|
f(x)
|
|
|
|
with self.assertRaises(RuntimeError):
|
|
result = jax.device_put(x, jax.devices()[0])
|
|
result.block_until_ready()
|
|
|
|
with self.assertRaises(RuntimeError):
|
|
result = jax.device_put(x, jax.devices()[-1])
|
|
result.block_until_ready()
|
|
|
|
def test_device_put_on_multi_device_donated_buffer_fails(self):
|
|
@partial(jax.jit, donate_argnums=0)
|
|
def f(inp1):
|
|
return inp1 * 2
|
|
|
|
mesh1 = jax.sharding.Mesh(jax.devices(), ("x",))
|
|
s1 = jax.NamedSharding(mesh1, P("x"))
|
|
|
|
mesh2 = jax.sharding.Mesh(tuple(reversed(jax.devices())), ("x",))
|
|
s2 = jax.NamedSharding(mesh2, P("x"))
|
|
|
|
x = jax.device_put(np.arange(len(jax.devices()), dtype=jnp.float32), s1)
|
|
f(x)
|
|
|
|
with self.assertRaises(RuntimeError):
|
|
result = jax.device_put(x, s1)
|
|
result.block_until_ready()
|
|
|
|
with self.assertRaises(RuntimeError):
|
|
result = jax.device_put(x, s2)
|
|
result.block_until_ready()
|
|
|
|
|
|
@jax.default_matmul_precision("float32")
|
|
def test_jacobian(self):
|
|
R = self.rng().randn
|
|
A = R(4, 3)
|
|
x = R(3)
|
|
|
|
f = lambda x: jnp.dot(A, x)
|
|
assert np.allclose(jacfwd(f)(x), A)
|
|
assert np.allclose(jacrev(f)(x), A)
|
|
|
|
f = lambda x: jnp.tanh(jnp.dot(A, x))
|
|
assert np.allclose(jacfwd(f)(x), jacrev(f)(x))
|
|
|
|
@jax.default_matmul_precision("float32")
|
|
def test_hessian(self):
|
|
R = self.rng().randn
|
|
A = R(4, 4)
|
|
x = R(4)
|
|
|
|
f = lambda x: jnp.dot(x, jnp.dot(A, x))
|
|
assert np.allclose(hessian(f)(x), A + A.T)
|
|
|
|
@jax.default_matmul_precision("float32")
|
|
def test_hessian_holomorphic(self):
|
|
R = self.rng().randn
|
|
A = R(4, 4)
|
|
x = R(4).astype('complex64') * (1 + 2j)
|
|
|
|
f = lambda x: jnp.dot(x, jnp.dot(A.astype(x.dtype), x))
|
|
assert np.allclose(hessian(f, holomorphic=True)(x), A + A.T)
|
|
|
|
@jax.default_matmul_precision("float32")
|
|
def test_hessian_aux(self):
|
|
R = self.rng().randn
|
|
A = R(4, 4)
|
|
x = R(4)
|
|
|
|
f = lambda x: (jnp.dot(x, jnp.dot(A, x)), x)
|
|
h, aux = hessian(f, has_aux=True)(x)
|
|
assert np.allclose(h, A + A.T)
|
|
assert np.allclose(aux, x)
|
|
|
|
def test_std_basis(self):
|
|
basis = api._std_basis(jnp.zeros(3))
|
|
assert getattr(basis, "shape", None) == (3, 3)
|
|
assert np.allclose(basis, np.eye(3))
|
|
|
|
basis = api._std_basis(jnp.zeros((3, 3)))
|
|
assert getattr(basis, "shape", None) == (9, 3, 3)
|
|
assert np.allclose(basis, np.eye(9).reshape(9, 3, 3))
|
|
|
|
basis = api._std_basis([0., (jnp.zeros(3), jnp.zeros((3, 4)))])
|
|
assert isinstance(basis, list) and len(basis) == 2
|
|
assert getattr(basis[0], "shape", None) == (16,)
|
|
assert isinstance(basis[1], tuple) and len(basis[1]) == 2
|
|
assert getattr(basis[1][0], "shape", None) == (16, 3)
|
|
assert getattr(basis[1][1], "shape", None) == (16, 3, 4)
|
|
|
|
@jtu.skip_on_devices("tpu")
|
|
def test_jacobian_on_pytrees(self):
|
|
for jacfun in [jacfwd, jacrev]:
|
|
ans = jacfun(lambda x, y: (x, y))(0., 1.)
|
|
expected = (1., 0.)
|
|
self.assertAllClose(ans, expected, check_dtypes=False)
|
|
|
|
ans = jacfun(lambda x, y: (x, y), 1)(0., 1.)
|
|
expected = (0., 1.)
|
|
self.assertAllClose(ans, expected, check_dtypes=False)
|
|
|
|
ans = jacfun(lambda x, y: (x, y), (0, 1))(0., 1.)
|
|
expected = ((1., 0.),
|
|
(0., 1.),)
|
|
self.assertAllClose(ans, expected, check_dtypes=False)
|
|
|
|
ans = jacfun(lambda x: x[:2])((1., 2., 3.))
|
|
expected = ((1., 0., 0.),
|
|
(0., 1., 0.))
|
|
self.assertAllClose(ans, expected, check_dtypes=False)
|
|
|
|
R = self.rng().randn
|
|
x = jnp.array(R(2))
|
|
y = jnp.array(R(3))
|
|
ans = jacfun(lambda x, y: {'x': x, 'xy': jnp.outer(x, y)})(x, y)
|
|
expected = {'x': np.eye(2),
|
|
'xy': np.kron(np.eye(2), y[:, None]).reshape(2, 3, 2)}
|
|
self.assertAllClose(ans, expected, check_dtypes=False)
|
|
|
|
@jtu.skip_on_devices("tpu")
|
|
def test_hessian_on_pytrees(self):
|
|
ans = hessian(lambda x: jnp.array(x)**2)((1., 2.))
|
|
expected = ((np.array([2., 0.]), np.array([0., 0.])),
|
|
(np.array([0., 0.]), np.array([0., 2.])))
|
|
self.assertAllClose(ans, expected, check_dtypes=False)
|
|
|
|
@jtu.skip_on_devices("tpu")
|
|
def test_issue1372(self):
|
|
def quad(x):
|
|
return jnp.dot(x, x)
|
|
|
|
def f(x, u):
|
|
return quad(x) + quad(u)
|
|
|
|
x, u = jnp.ones(5), jnp.ones(2)
|
|
|
|
rev = jacrev
|
|
fwd = jacfwd
|
|
|
|
# Diagonal entries
|
|
self.assertEqual(rev(rev(f, 0), 0)(x, u).shape, (5, 5))
|
|
self.assertEqual(rev(fwd(f, 0), 0)(x, u).shape, (5, 5))
|
|
self.assertEqual(fwd(rev(f, 0), 0)(x, u).shape, (5, 5))
|
|
self.assertEqual(fwd(fwd(f, 0), 0)(x, u).shape, (5, 5))
|
|
self.assertEqual(rev(rev(f, 1), 1)(x, u).shape, (2, 2))
|
|
self.assertEqual(rev(fwd(f, 1), 1)(x, u).shape, (2, 2))
|
|
self.assertEqual(fwd(rev(f, 1), 1)(x, u).shape, (2, 2))
|
|
self.assertEqual(fwd(fwd(f, 1), 1)(x, u).shape, (2, 2))
|
|
|
|
# Off-diagonal entries by reverse-mode on the outside
|
|
self.assertEqual(rev(rev(f, 1), 0)(x, u).shape, (2, 5))
|
|
self.assertEqual(rev(fwd(f, 1), 0)(x, u).shape, (2, 5))
|
|
self.assertEqual(rev(rev(f, 0), 1)(x, u).shape, (5, 2))
|
|
self.assertEqual(rev(fwd(f, 0), 1)(x, u).shape, (5, 2))
|
|
|
|
# Off-diagonal entries by forward-mode on the outside
|
|
self.assertEqual(fwd(rev(f, 1), 0)(x, u).shape, (2, 5))
|
|
self.assertEqual(fwd(fwd(f, 1), 0)(x, u).shape, (2, 5))
|
|
self.assertEqual(fwd(rev(f, 0), 1)(x, u).shape, (5, 2))
|
|
self.assertEqual(fwd(fwd(f, 0), 1)(x, u).shape, (5, 2))
|
|
|
|
def test_large_device_constant(self):
|
|
ans = jit(lambda x: 2 * x)(jnp.ones(int(2e6))) # doesn't crash
|
|
self.assertAllClose(ans, np.ones(int(2e6)) * 2., check_dtypes=False)
|
|
|
|
def test_grad_and_aux_basic(self):
|
|
g, aux = grad(lambda x: (x**3, [x**2]), has_aux=True)(3.)
|
|
self.assertAllClose(g, grad(lambda x: x**3)(3.))
|
|
self.assertAllClose(aux, [9.], check_dtypes=False)
|
|
|
|
def test_grad_and_aux_error(self):
|
|
with self.assertRaisesRegex(TypeError, "two-element tuple"):
|
|
grad(lambda x: (1, 2, 3), has_aux=True)(1.)
|
|
|
|
with self.assertRaisesRegex(TypeError, "two-element tuple"):
|
|
grad(lambda x: x, has_aux=True)(1.)
|
|
|
|
with self.assertRaisesRegex(TypeError, "two-element tuple"):
|
|
grad(lambda x: (x,), has_aux=True)(1.)
|
|
|
|
def test_grad_and_aux_nested(self):
|
|
def f(x):
|
|
g, aux = grad(lambda x: (x**3, [x**3]), has_aux=True)(x)
|
|
return aux[0]
|
|
|
|
f2 = lambda x: x**3
|
|
|
|
self.assertEqual(grad(f)(4.), grad(f2)(4.))
|
|
self.assertEqual(jit(grad(f))(4.), grad(f2)(4.))
|
|
self.assertEqual(jit(grad(jit(f)))(4.), grad(f2)(4.))
|
|
|
|
def f(x):
|
|
g, aux = grad(lambda x: (x**3, [x**3]), has_aux=True)(x)
|
|
return aux[0] * jnp.sin(x)
|
|
|
|
f2 = lambda x: x**3 * jnp.sin(x)
|
|
|
|
self.assertEqual(grad(f)(4.), grad(f2)(4.))
|
|
self.assertEqual(jit(grad(f))(4.), grad(f2)(4.))
|
|
self.assertEqual(jit(grad(jit(f)))(4.), grad(f2)(4.))
|
|
|
|
def test_grad_and_aux_constant(self):
|
|
g, aux = grad(lambda x: (x**3, [4.]), has_aux=True)(4.)
|
|
self.assertEqual(g, grad(lambda x: x**3)(4.))
|
|
self.assertEqual(aux, [4.])
|
|
|
|
g, aux = grad(lambda x: (x**3, [x**2, 4.]), has_aux=True)(4.)
|
|
self.assertEqual(g, grad(lambda x: x**3)(4.))
|
|
self.assertEqual(aux, [4.**2, 4.])
|
|
|
|
def test_grad_and_aux_no_tracers(self):
|
|
# see https://github.com/jax-ml/jax/issues/1950
|
|
def f(x):
|
|
aux = dict(identity=x, p1=x+1)
|
|
return x ** 2, aux
|
|
|
|
_, aux = jax.grad(f, has_aux=True)(3.)
|
|
self.assertIsInstance(aux, dict)
|
|
for val in aux.values():
|
|
self.assertNotIsInstance(val, core.Tracer)
|
|
|
|
def test_jacfwd_and_aux_basic(self):
|
|
jac, aux = jacfwd(lambda x: (x**3, [x**2]), has_aux=True)(3.)
|
|
self.assertAllClose(jac, jacfwd(lambda x: x**3)(3.))
|
|
self.assertAllClose(aux, [9.], check_dtypes=False)
|
|
|
|
def test_jacrev_and_aux_basic(self):
|
|
jac, aux = jacrev(lambda x: (x**3, [x**2]), has_aux=True)(3.)
|
|
self.assertAllClose(jac, jacrev(lambda x: x**3)(3.))
|
|
self.assertAllClose(aux, [9.], check_dtypes=False)
|
|
|
|
def test_jacfwd_and_aux_nested(self):
|
|
def f(x):
|
|
jac, aux = jacfwd(lambda x: (x**3, [x**3]), has_aux=True)(x)
|
|
return aux[0]
|
|
|
|
f2 = lambda x: x**3
|
|
|
|
self.assertEqual(jacfwd(f)(4.), jacfwd(f2)(4.))
|
|
self.assertEqual(jit(jacfwd(f))(4.), jacfwd(f2)(4.))
|
|
self.assertEqual(jit(jacfwd(jit(f)))(4.), jacfwd(f2)(4.))
|
|
|
|
def f(x):
|
|
jac, aux = jacfwd(lambda x: (x**3, [x**3]), has_aux=True)(x)
|
|
return aux[0] * jnp.sin(x)
|
|
|
|
f2 = lambda x: x**3 * jnp.sin(x)
|
|
|
|
self.assertEqual(jacfwd(f)(4.), jacfwd(f2)(4.))
|
|
self.assertEqual(jit(jacfwd(f))(4.), jacfwd(f2)(4.))
|
|
self.assertEqual(jit(jacfwd(jit(f)))(4.), jacfwd(f2)(4.))
|
|
|
|
def test_jacrev_and_aux_nested(self):
|
|
def f(x):
|
|
jac, aux = jacrev(lambda x: (x**3, [x**3]), has_aux=True)(x)
|
|
return aux[0]
|
|
|
|
f2 = lambda x: x**3
|
|
|
|
self.assertEqual(jacrev(f)(4.), jacrev(f2)(4.))
|
|
self.assertEqual(jit(jacrev(f))(4.), jacrev(f2)(4.))
|
|
self.assertEqual(jit(jacrev(jit(f)))(4.), jacrev(f2)(4.))
|
|
|
|
def f(x):
|
|
jac, aux = jacrev(lambda x: (x**3, [x**3]), has_aux=True)(x)
|
|
return aux[0] * jnp.sin(x)
|
|
|
|
f2 = lambda x: x**3 * jnp.sin(x)
|
|
|
|
self.assertEqual(jacrev(f)(4.), jacrev(f2)(4.))
|
|
self.assertEqual(jit(jacrev(f))(4.), jacrev(f2)(4.))
|
|
self.assertEqual(jit(jacrev(jit(f)))(4.), jacrev(f2)(4.))
|
|
|
|
def test_jvp_and_aux_basic(self):
|
|
fun = lambda x: (x**3, [x**2])
|
|
primals, tangents, aux = api.jvp(fun, (3.,), (4.,), has_aux=True)
|
|
expected_primals, expected_tangents = api.jvp(lambda x: x**3, (3.,), (4.,))
|
|
self.assertAllClose(primals, expected_primals, check_dtypes=True)
|
|
self.assertAllClose(tangents, expected_tangents, check_dtypes=True)
|
|
self.assertEqual(aux, [3.**2])
|
|
|
|
def test_jvp_mismatched_arguments(self):
|
|
self.assertRaisesRegex(
|
|
TypeError,
|
|
("primal and tangent arguments to jax.jvp must have the same tree "
|
|
"structure"),
|
|
lambda: api.jvp(lambda x, y: x * y, (np.float32(2),), ()))
|
|
# If primals and tangents must both be tuples or both lists
|
|
self.assertRaisesRegex(
|
|
TypeError,
|
|
("primal and tangent arguments to jax.jvp must have the same tree "
|
|
"structure"),
|
|
lambda: api.jvp(lambda x, y: x * y, (np.float32(2),), [np.float32(2)]))
|
|
self.assertRaisesRegex(
|
|
TypeError,
|
|
"primal and tangent arguments to jax.jvp do not match.",
|
|
lambda: api.jvp(lambda x: -x, (np.float16(2),), (np.float32(4),)))
|
|
# If primals and tangents are not of the same shape then raise error
|
|
fun = lambda x: x+1
|
|
with self.assertRaisesRegex(
|
|
ValueError, "jvp called with different primal and tangent shapes"):
|
|
api.jvp(fun, (jnp.array([1.,2.,3.]),), (jnp.array([1.,2.,3.,4.]),))
|
|
with self.assertRaisesRegex(
|
|
ValueError, "jvp called with different primal and tangent shapes"):
|
|
api.jvp(fun, (jnp.float32(10.),), (jnp.array([1.,2.,3.], dtype=jnp.float32),))
|
|
with self.assertRaisesRegex(
|
|
ValueError, "jvp called with different primal and tangent shapes"):
|
|
api.jvp(fun, (jnp.array([1.,2.,3.], dtype=jnp.float32),), (jnp.float32(20.),))
|
|
with self.assertRaisesRegex(
|
|
ValueError, "jvp called with different primal and tangent shapes"):
|
|
api.jvp(fun, (jnp.array([1.,2.,3.]),), (20.,))
|
|
|
|
def test_jvp_non_tuple_arguments(self):
|
|
def f(x, y): return x + y
|
|
self.assertRaisesRegex(
|
|
TypeError,
|
|
"primal and tangent arguments to jax.jvp must be tuples or lists; found float and tuple.",
|
|
lambda: api.jvp(f, 0., (1.,)))
|
|
self.assertRaisesRegex(
|
|
TypeError,
|
|
"primal and tangent arguments to jax.jvp must be tuples or lists; found tuple and ndarray.",
|
|
lambda: api.jvp(f, (0.,), np.array([1., 2.])))
|
|
|
|
def test_vjp_mismatched_arguments(self):
|
|
_, pullback = api.vjp(lambda x, y: x * y, np.float32(3), np.float32(4))
|
|
self.assertRaisesRegex(
|
|
ValueError, "unexpected tree structure",
|
|
lambda: pullback((np.float32(7), np.float32(100))))
|
|
self.assertRaisesRegex(
|
|
ValueError, "unexpected JAX type",
|
|
lambda: pullback(np.float16(42)))
|
|
|
|
def test_vjp_bad_cotangent_shape(self):
|
|
x = np.ones((2, 5), dtype=np.float32)
|
|
y = np.ones((5, 3), dtype=np.float32)
|
|
def f_jax(x, y):
|
|
return jnp.matmul(x, y)
|
|
res, pullback = jax.vjp(f_jax, x, y)
|
|
with self.assertRaisesRegex(ValueError, "unexpected JAX type"):
|
|
pullback(np.ones((2, 4), dtype=np.float32))
|
|
|
|
def test_jvp_jit_cached(self):
|
|
"""Bug in caching in presence of JVP and JIT."""
|
|
|
|
def func(x):
|
|
def inner(y):
|
|
return y * x
|
|
|
|
# Must have two calls to the inner jit (the second one hits the cache)
|
|
res1 = api.jit(inner)(4.)
|
|
res2 = api.jit(inner)(5.)
|
|
return res1 + res2
|
|
|
|
self.assertAllClose((45., 9.), api.jvp(func, (5.,), (1.,)))
|
|
|
|
def test_linear_transpose_abstract(self):
|
|
x = types.SimpleNamespace(shape=(3,), dtype=np.dtype(np.float32))
|
|
y = jnp.arange(3, dtype=np.float32)
|
|
transpose_fun = api.linear_transpose(lambda x: 2 * x, x)
|
|
z, = transpose_fun(y)
|
|
self.assertArraysEqual(2 * y, z, check_dtypes=True)
|
|
|
|
def test_linear_transpose_integer(self):
|
|
f = lambda x: 2 * x
|
|
transpose = api.linear_transpose(f, 1)
|
|
actual, = transpose(3)
|
|
expected = 6
|
|
self.assertEqual(actual, expected)
|
|
|
|
def test_linear_transpose_dce(self):
|
|
# https://github.com/jax-ml/jax/issues/15660
|
|
f = jit(lambda x: (2 * x, x > 0))
|
|
g = lambda x: f(x)[0]
|
|
api.linear_transpose(g, 1.)(1.)
|
|
|
|
def test_linear_transpose_error(self):
|
|
with self.assertRaisesRegex(
|
|
TypeError, "linear_transpose only supports"):
|
|
api.linear_transpose(lambda x: 2. * x, 1)
|
|
transpose_fun = api.linear_transpose(lambda x: [x, x], 1.0)
|
|
with self.assertRaisesRegex(TypeError, "cotangent tree does not match"):
|
|
transpose_fun(1.0)
|
|
|
|
transpose_fun = api.linear_transpose(lambda x: jnp.stack([x, x]), 1.0)
|
|
with self.assertRaisesRegex(TypeError, "cotangent type does not match"):
|
|
transpose_fun(1.0)
|
|
|
|
transpose_fun = api.linear_transpose(lambda x: 1j * x, 1.0)
|
|
with self.assertRaisesRegex(TypeError, "cotangent type does not match"):
|
|
transpose_fun(1.0)
|
|
|
|
transpose_fun = api.linear_transpose(lambda x: x, 1.0)
|
|
with self.assertRaisesRegex(TypeError, "cotangent type does not match"):
|
|
transpose_fun(1j)
|
|
|
|
def test_linear_transpose_complex(self):
|
|
f = lambda x: (1 + 2j) * x
|
|
transpose = api.linear_transpose(f, 1j)
|
|
actual, = transpose(3 + 4j)
|
|
expected = -5 + 10j
|
|
self.assertEqual(actual, expected)
|
|
|
|
def test_linear_transpose_zeros(self):
|
|
f = lambda x: x[0]
|
|
transpose = api.linear_transpose(f, [1., 2.])
|
|
actual, = transpose(3.)
|
|
expected = [3., 0.]
|
|
self.assertEqual(actual, expected)
|
|
|
|
def test_complex_grad_raises_error(self):
|
|
self.assertRaises(TypeError, lambda: grad(lambda x: jnp.sin(x))(1 + 2j))
|
|
|
|
def test_holomorphic_grad(self):
|
|
out = grad(lambda x: jnp.sin(x), holomorphic=True)(1 + 2j)
|
|
expected = 2.0327230070196656 - 3.0518977991518j
|
|
self.assertAllClose(out, expected, check_dtypes=False)
|
|
|
|
def test_nonholomorphic_grad(self):
|
|
zs = 0.5j * np.arange(5) + np.arange(5)
|
|
|
|
def f(z):
|
|
return jnp.sum(jnp.cos(jnp.abs(z)))
|
|
|
|
ans = grad(f)(zs)
|
|
expected = np.array([ 0. + 0.j,
|
|
-0.80430663 + 0.40215331j,
|
|
-0.70368982 + 0.35184491j,
|
|
0.1886467 - 0.09432335j,
|
|
0.86873727 - 0.43436864j])
|
|
self.assertAllClose(ans, expected, check_dtypes=False,
|
|
atol=jtu.default_gradient_tolerance,
|
|
rtol=jtu.default_gradient_tolerance)
|
|
|
|
def test_complex_output_jacrev_raises_error(self):
|
|
self.assertRaises(TypeError, lambda: jacrev(lambda x: jnp.sin(x))(1 + 2j))
|
|
|
|
def test_nonholomorphic_jacrev(self):
|
|
# code based on https://github.com/jax-ml/jax/issues/603
|
|
zs = 0.5j * np.arange(5) + np.arange(5)
|
|
|
|
def f(z):
|
|
return jnp.cos(jnp.linalg.norm(2 * z))
|
|
|
|
ans = jacrev(f)(zs)
|
|
expected = grad(f)(zs)
|
|
self.assertAllClose(ans, expected)
|
|
|
|
@jax.numpy_dtype_promotion('standard') # Test explicitly exercises implicit dtype promotion.
|
|
def test_heterogeneous_jacfwd(self):
|
|
# See https://github.com/jax-ml/jax/issues/7157
|
|
# See https://github.com/jax-ml/jax/issues/7780
|
|
x = np.array([2.0], dtype=np.float16)
|
|
y = np.array([3.0], dtype=np.float32)
|
|
a = (x, y)
|
|
|
|
def f(tup):
|
|
jtu._check_dtypes_match(tup, a)
|
|
x, y = tup
|
|
return x, y, x + y
|
|
|
|
actual = jacfwd(f)(a)
|
|
desired = ((np.array(1., dtype=np.float16), np.array(0., dtype=np.float16)),
|
|
(np.array(0., dtype=np.float32), np.array(1., dtype=np.float32)),
|
|
(np.array(1., dtype=np.float32), np.array(1., dtype=np.float32)))
|
|
jtu._check_dtypes_match(actual, desired)
|
|
jtu.check_eq(actual, desired)
|
|
|
|
@jax.numpy_dtype_promotion('standard') # Test explicitly exercises implicit dtype promotion.
|
|
def test_heterogeneous_jacrev(self):
|
|
# See https://github.com/jax-ml/jax/issues/7157
|
|
# See https://github.com/jax-ml/jax/issues/7780
|
|
x = np.array([2.0], dtype=np.float16)
|
|
y = np.array([3.0], dtype=np.float32)
|
|
a = (x, y)
|
|
|
|
def f(tup):
|
|
jtu._check_dtypes_match(tup, a)
|
|
x, y = tup
|
|
return x, y, x + y
|
|
|
|
actual = jacrev(f)(a)
|
|
desired = ((np.array(1., dtype=np.float16), np.array(0., dtype=np.float32)),
|
|
(np.array(0., dtype=np.float16), np.array(1., dtype=np.float32)),
|
|
(np.array(1., dtype=np.float16), np.array(1., dtype=np.float32)))
|
|
jtu._check_dtypes_match(actual, desired)
|
|
jtu.check_eq(actual, desired)
|
|
|
|
def test_heterogeneous_grad(self):
|
|
# See https://github.com/jax-ml/jax/issues/7157
|
|
x = np.array(1.0+1j)
|
|
y = np.array(2.0)
|
|
a = (x, y)
|
|
|
|
def f(tup):
|
|
jtu._check_dtypes_match(tup, a)
|
|
x, y = tup
|
|
return jnp.square(jnp.abs(x)) + y
|
|
|
|
actual = grad(f)(a)
|
|
desired = (np.array(2 - 2j), np.array(1.))
|
|
jtu._check_dtypes_match(actual, desired)
|
|
jtu.check_eq(actual, desired)
|
|
|
|
def test_complex_input_jacfwd_raises_error(self):
|
|
self.assertRaises(TypeError, lambda: jacfwd(lambda x: jnp.sin(x))(1 + 2j))
|
|
|
|
def test_legacy_devicearray_repr(self):
|
|
dx = device_put(3.)
|
|
str(dx.item()) # doesn't crash
|
|
|
|
def test_devicearray_repr(self):
|
|
x = device_put(jnp.zeros(3))
|
|
_check_instance(self, x)
|
|
repr(x) # doesn't crash
|
|
|
|
x = device_put(jnp.full(3, 1 + 1j))
|
|
_check_instance(self, x)
|
|
repr(x) # doesn't crash
|
|
|
|
def test_devicearray_delete(self):
|
|
x = device_put(1.)
|
|
x.delete()
|
|
self.assertRaisesRegex(RuntimeError, "Array has been deleted.",
|
|
lambda: repr(x))
|
|
|
|
def test_devicearray_block_until_ready(self):
|
|
x = device_put(1.)
|
|
y = x.block_until_ready()
|
|
# Tests mostly that block_until_ready() does not produce an error.
|
|
self.assertTrue(y is x)
|
|
|
|
def test_block_until_ready_function(self):
|
|
# Just tests that we don't error...
|
|
pytree = (device_put(1.), np.ones(3))
|
|
pytree = jax.block_until_ready(pytree)
|
|
self.assertAllClose(pytree[0], jnp.array(1.), check_dtypes=False)
|
|
self.assertAllClose(pytree[1], np.ones(3), check_dtypes=False)
|
|
|
|
def test_block_until_ready_numpy_arrays(self):
|
|
pytree = (np.ones(1), np.ones(2))
|
|
pytree = jax.block_until_ready(pytree)
|
|
self.assertAllClose(pytree[0], np.ones(1), check_dtypes=False)
|
|
self.assertAllClose(pytree[1], np.ones(2), check_dtypes=False)
|
|
|
|
def test_block_until_ready_mixed(self):
|
|
pytree = (device_put(1.), device_put(2.), np.ones(3), 4)
|
|
pytree = jax.block_until_ready(pytree)
|
|
self.assertAllClose(pytree[0], jnp.array(1.), check_dtypes=False)
|
|
self.assertAllClose(pytree[1], jnp.array(2.), check_dtypes=False)
|
|
self.assertAllClose(pytree[2], np.ones(3), check_dtypes=False)
|
|
self.assertEqual(pytree[3], 4)
|
|
|
|
@jtu.thread_unsafe_test() # Weakref destruction seems unpredictable with threads
|
|
def test_devicearray_weakref_friendly(self):
|
|
x = device_put(1.)
|
|
y = weakref.ref(x)
|
|
self.assertEqual(y(), 1.)
|
|
del x
|
|
self.assertIsNone(y())
|
|
|
|
def test_namedtuple_transparency(self):
|
|
# See https://github.com/jax-ml/jax/issues/446
|
|
Point = collections.namedtuple("Point", ["x", "y"])
|
|
|
|
def f(pt):
|
|
return jnp.sqrt(pt.x ** 2 + pt.y ** 2)
|
|
|
|
pt = Point(1., 2.)
|
|
|
|
f(pt) # doesn't crash
|
|
g = api.grad(f)(pt)
|
|
self.assertIsInstance(g, Point)
|
|
|
|
f_jit = api.jit(f)
|
|
self.assertAllClose(f(pt), f_jit(pt), check_dtypes=False)
|
|
|
|
def test_namedtuple_subclass_transparency(self):
|
|
# See https://github.com/jax-ml/jax/issues/806
|
|
Point = collections.namedtuple("Point", ["x", "y"])
|
|
|
|
class ZeroPoint(Point):
|
|
def is_zero(self):
|
|
return (self.x == 0) and (self.y == 0)
|
|
|
|
pt = ZeroPoint(0., 0.)
|
|
|
|
def f(pt):
|
|
return 0. if pt.is_zero() else jnp.sqrt(pt.x ** 2 + pt.y ** 2)
|
|
|
|
f(pt) # doesn't crash
|
|
_ = api.grad(f)(pt)
|
|
self.assertIsInstance(pt, ZeroPoint)
|
|
|
|
@parameterized.parameters(1, 2, 3)
|
|
def test_shape_dtype_struct(self, i):
|
|
s = api.ShapeDtypeStruct(shape=(i, 2, 3), dtype=jnp.float32)
|
|
self.assertEqual(s.shape, (i, 2, 3))
|
|
self.assertEqual(s.dtype, jnp.float32)
|
|
self.assertEqual(s.ndim, 3)
|
|
self.assertEqual(s.size, i * 2 * 3)
|
|
self.assertLen(s, i)
|
|
for f in (str, repr):
|
|
self.assertEqual(
|
|
f(s), f"ShapeDtypeStruct(shape=({i}, 2, 3), dtype=float32)")
|
|
|
|
def test_shape_dtype_struct_scalar(self):
|
|
s = api.ShapeDtypeStruct(shape=(), dtype=jnp.float32)
|
|
self.assertEmpty(s.shape)
|
|
self.assertEqual(s.size, 1)
|
|
self.assertEqual(s.ndim, 0)
|
|
with self.assertRaisesRegex(TypeError, "len[(][)] of unsized object"):
|
|
_ = len(s)
|
|
|
|
def test_shape_dtype_struct_hash(self):
|
|
s1 = api.ShapeDtypeStruct(shape=(2, 3), dtype=jnp.float32)
|
|
s2 = api.ShapeDtypeStruct(shape=(2, 3), dtype=jnp.float32)
|
|
s3 = api.ShapeDtypeStruct(shape=(2, 4), dtype=jnp.float32)
|
|
self.assertEqual(hash(s1), hash(s2))
|
|
self.assertNotEqual(hash(s1), hash(s3))
|
|
|
|
def test_shape_dtype_struct_invalid_shape(self):
|
|
with self.assertRaisesRegex(TypeError, "'int' object is not iterable"):
|
|
api.ShapeDtypeStruct(shape=4, dtype='float32')
|
|
|
|
def test_shape_dtype_struct_dtype_none(self):
|
|
with self.assertRaisesRegex(ValueError, "dtype must be specified"):
|
|
api.ShapeDtypeStruct(shape=(), dtype=None)
|
|
|
|
def test_eval_shape(self):
|
|
def fun(x, y):
|
|
return jnp.tanh(jnp.dot(x, y) + 3.)
|
|
|
|
x = jnp.ones((2, 3))
|
|
y = jnp.ones((3, 4))
|
|
out_shape = api.eval_shape(fun, x, y)
|
|
|
|
self.assertEqual(out_shape.shape, (2, 4))
|
|
|
|
def test_eval_shape_constants(self):
|
|
def fun():
|
|
x = jnp.ones((2, 3))
|
|
y = jnp.ones((3, 4))
|
|
return jnp.tanh(jnp.dot(x, y) + 3.)
|
|
|
|
out_shape = api.eval_shape(fun)
|
|
|
|
self.assertEqual(out_shape.shape, (2, 4))
|
|
|
|
def test_eval_shape_tuple_unpacking(self):
|
|
def fun(x, y):
|
|
a, b = x
|
|
return a + b + y
|
|
|
|
x = (jnp.ones(2), jnp.ones(2))
|
|
y = 3.
|
|
out_shape = api.eval_shape(fun, x, y)
|
|
|
|
self.assertEqual(out_shape.shape, (2,))
|
|
|
|
def test_eval_shape_tuple_itemgetting(self):
|
|
def fun(x, y):
|
|
return x[0] + x[1] + y
|
|
|
|
x = (jnp.ones(2), jnp.ones(2))
|
|
y = 3.
|
|
out_shape = api.eval_shape(fun, x, y)
|
|
|
|
self.assertEqual(out_shape.shape, (2,))
|
|
|
|
def test_eval_shape_output_dict(self):
|
|
def fun(x, y):
|
|
return {'hi': x[0] + x[1] + y}
|
|
|
|
x = (jnp.ones(2), jnp.ones(2))
|
|
y = 3.
|
|
out_shape = api.eval_shape(fun, x, y)
|
|
out_shape = jax.tree.map(np.shape, out_shape)
|
|
|
|
self.assertEqual(out_shape, {'hi': (2,)})
|
|
|
|
def test_eval_shape_shape_error(self):
|
|
def fun(x, y):
|
|
return jnp.tanh(jnp.dot(x, y) + 3.)
|
|
|
|
x = jnp.ones((3, 3))
|
|
y = jnp.ones((4, 4))
|
|
|
|
self.assertRaises(TypeError, lambda: api.eval_shape(fun, x, y))
|
|
|
|
def test_eval_shape_trace_cache_share(self):
|
|
def f(x):
|
|
return x
|
|
|
|
inp = np.arange(8)
|
|
|
|
with jtu.count_jit_tracing_cache_miss() as count:
|
|
jax.eval_shape(f, inp)
|
|
jax.jit(f)(inp)
|
|
|
|
self.assertEqual(count(), 1)
|
|
|
|
@jtu.thread_unsafe_test() # jit cache misses aren't thread safe
|
|
def test_jit_infer_params_cache(self):
|
|
def f(x):
|
|
return x
|
|
|
|
f_jit = jax.jit(f)
|
|
|
|
def g(x):
|
|
x = f_jit(x) # noqa: F821
|
|
x = f_jit(x) # noqa: F821
|
|
return x
|
|
|
|
g_jit = jax.jit(g)
|
|
|
|
inp = np.arange(8)
|
|
with jtu.count_jit_infer_params_cache_miss() as count:
|
|
g_jit(inp)
|
|
|
|
self.assertDictEqual(count, {f: 1, g: 1})
|
|
cache_size = pjit_lib._infer_params_cached.cache_info().currsize
|
|
del count, f, f_jit, g, g_jit
|
|
# Cache should only keep a weak reference to f and g.
|
|
self.assertLess(pjit_lib._infer_params_cached.cache_info().currsize,
|
|
cache_size, msg=pjit_lib._infer_params_cached.cache_keys())
|
|
|
|
def test_eval_shape_out_shardings(self):
|
|
s = jax.sharding.SingleDeviceSharding(jax.devices()[0])
|
|
|
|
@partial(jax.jit, out_shardings=s)
|
|
def f(x):
|
|
return x * 2
|
|
|
|
inp = np.arange(8)
|
|
out = f.eval_shape(inp)
|
|
self.assertEqual(out.sharding, s)
|
|
self.assertEqual(out.shape, (inp * 2).shape)
|
|
|
|
def test_eval_shape_duck_typing(self):
|
|
def fun(A, b, x):
|
|
return jnp.dot(A, x) + b
|
|
|
|
class MyArgArray:
|
|
def __init__(self, shape, dtype):
|
|
self.shape = shape
|
|
self.dtype = np.dtype(dtype)
|
|
|
|
A = MyArgArray((3, 4), jnp.float32)
|
|
b = MyArgArray((1, 5), jnp.float32)
|
|
x = MyArgArray((4, 5), jnp.float32)
|
|
out_shape = api.eval_shape(fun, A, b, x)
|
|
|
|
self.assertEqual(out_shape.shape, (3, 5))
|
|
|
|
def test_eval_shape_duck_typing2(self):
|
|
# https://github.com/jax-ml/jax/issues/5683
|
|
class EasyDict(dict):
|
|
def __init__(self, *args, **kwargs):
|
|
super().__init__(*args, **kwargs)
|
|
self.__dict__ = self
|
|
|
|
x = EasyDict(shape=(3,), dtype=np.dtype('float32'))
|
|
out_shape = api.eval_shape(lambda x: x, x) # doesn't crash
|
|
self.assertEqual(out_shape.shape, (3,))
|
|
|
|
def test_issue_871(self):
|
|
T = jnp.array([[1., 2.], [3., 4.], [5., 6.]])
|
|
x = jnp.array([1, 2, 3])
|
|
msg = ("linearized function called on tangent values inconsistent with "
|
|
"the original primal values")
|
|
|
|
y, f_jvp = api.linearize(jnp.sum, x)
|
|
with self.assertRaisesRegex(ValueError, msg):
|
|
f_jvp(T)
|
|
|
|
y, f_jvp = api.linearize(api.jit(jnp.sum), x)
|
|
with self.assertRaisesRegex(ValueError, msg):
|
|
f_jvp(T)
|
|
|
|
def test_grad_of_int_errors(self):
|
|
# Errors without allow_int=True
|
|
dfn = grad(lambda x: x ** 2)
|
|
self.assertRaisesRegex(
|
|
TypeError,
|
|
(r"grad requires real- or complex-valued inputs \(input dtype that is a "
|
|
r"sub-dtype of np.inexact\), but got int.*."),
|
|
lambda: dfn(3))
|
|
|
|
def test_jvp_of_int_identity(self):
|
|
primals = (1,)
|
|
tangents = (np.zeros(shape=(), dtype=float0),)
|
|
|
|
_, out = api.jvp(lambda x: x, primals, tangents)
|
|
self.assertEqual(out, np.zeros(shape=(), dtype=float0))
|
|
|
|
def test_jvp_of_int_add(self):
|
|
primals = (2,)
|
|
tangents = (np.zeros(shape=(), dtype=float0),)
|
|
|
|
_, out_tangent = api.jvp(lambda x: x+1, primals, tangents)
|
|
self.assertEqual(out_tangent, np.zeros(shape=(), dtype=float0))
|
|
|
|
def test_jit_jvp_of_int(self):
|
|
primals = (2,)
|
|
tangents = (np.zeros(shape=(), dtype=float0),)
|
|
|
|
_, out_tangent = api.jvp(jax.jit(lambda x: x+1), primals, tangents)
|
|
self.assertEqual(out_tangent, np.zeros(shape=(), dtype=float0))
|
|
|
|
def test_jvp_of_convert_element_type(self):
|
|
fun = lambda x: x.astype(np.int32) + 1
|
|
primal, tangent = jax.jvp(fun, (2.,), (1.,))
|
|
self.assertAllClose(primal, np.int32(3))
|
|
self.assertEqual(tangent, np.zeros((), dtype=float0))
|
|
|
|
def test_vjp_of_int_index(self):
|
|
primal, fn_vjp = api.vjp(lambda x, i: x[i], np.ones(2)*2, 1)
|
|
tangent_x, tangent_i = fn_vjp(1.)
|
|
self.assertEqual(primal, 2.)
|
|
self.assertAllClose(tangent_x, jnp.array([0., 1.]))
|
|
self.assertEqual(tangent_i, np.zeros(shape=(), dtype=float0))
|
|
|
|
def test_vjp_of_int_shapes(self):
|
|
out, fn_vjp = api.vjp(
|
|
lambda x: lax.reshape(x, (2, 2)), np.ones((4, 1), dtype=int))
|
|
tangent, = fn_vjp(np.zeros((2, 2), dtypes.float0))
|
|
self.assertArraysEqual(tangent, np.zeros(shape=(4, 1), dtype=float0))
|
|
|
|
def test_jit_vjp_of_int(self):
|
|
primal, fn_vjp = api.vjp(lambda x, y: x+y, 2, 1)
|
|
tangent_x, tangent_i = jax.jit(fn_vjp)(np.zeros((), dtypes.float0))
|
|
self.assertEqual(primal, 3)
|
|
self.assertEqual(tangent_x, np.zeros(shape=(), dtype=float0))
|
|
self.assertEqual(tangent_i, np.zeros(shape=(), dtype=float0))
|
|
|
|
def test_vjp_of_int_fulllike(self):
|
|
# Regression test for tangent and cotangent mismatch in convert_element_type
|
|
# transpose rule wrt a ConstVar
|
|
f = lax.full_like
|
|
out, vjp = api.vjp(f, jnp.zeros((2, 2)), 1)
|
|
self.assertAllClose(out, jnp.ones((2, 2)))
|
|
tangent_x, tangent_y = vjp(out)
|
|
self.assertAllClose(tangent_x, jnp.zeros((2, 2)))
|
|
self.assertEqual(tangent_y, np.zeros(shape=(), dtype=float0))
|
|
|
|
def test_grad_of_int(self):
|
|
# Need real-valued output, but testing integer input.
|
|
out = api.grad(lambda x: x+0., allow_int=True)(1)
|
|
self.assertEqual(out, np.zeros(shape=(), dtype=float0))
|
|
|
|
def test_grad_of_bool(self):
|
|
def cond(pred):
|
|
return lax.cond(pred, lambda _: 1., lambda _: 2., 1.)
|
|
value, grd = api.value_and_grad(cond, allow_int=True)(True)
|
|
self.assertEqual(value, 1.)
|
|
self.assertEqual(grd, np.zeros(shape=(), dtype=float0))
|
|
|
|
def test_grad_of_int_index(self):
|
|
grad_x, grad_i = api.grad(lambda x, i: x[i], argnums=(0, 1),
|
|
allow_int=True)(np.ones(2), 1)
|
|
self.assertAllClose(grad_x, jnp.array([0., 1.]))
|
|
self.assertEqual(grad_i, np.zeros(shape=(), dtype=float0))
|
|
|
|
def test_jit_grad_of_int(self):
|
|
grad_f = api.grad(lambda x, i: x[i], argnums=(0, 1), allow_int=True)
|
|
grad_x, grad_i = jax.jit(grad_f)(np.ones(2), 1)
|
|
self.assertAllClose(grad_x, jnp.array([0., 1.]))
|
|
self.assertEqual(grad_i, np.zeros(shape=(), dtype=float0))
|
|
|
|
def test_float0_reshape(self):
|
|
# dtype-agnostic operations are supported
|
|
float0_array = jax.grad(lambda x: jnp.sum(x+0.),
|
|
allow_int=True)(np.ones((2, 4), dtype=int))
|
|
|
|
self.assertArraysEqual(float0_array.reshape((4, 2)),
|
|
np.zeros((4, 2), dtype=float0))
|
|
self.assertArraysEqual(float0_array.transpose(),
|
|
np.zeros((4, 2), dtype=float0))
|
|
|
|
def test_float0_error(self):
|
|
# float0 is incompatible with other dtypes
|
|
float0_array = jax.grad(lambda x: x+0., allow_int=True)(1)
|
|
error_text = "float0s do not support any operations by design"
|
|
|
|
with self.assertRaisesRegex(TypeError, error_text):
|
|
# dispatch via Array
|
|
_ = float0_array + jnp.zeros(())
|
|
|
|
with self.assertRaisesRegex(TypeError, error_text):
|
|
# dispatch via lax
|
|
_ = lax.add(float0_array, jnp.zeros(()))
|
|
|
|
def test_grad_complex_result_errors(self):
|
|
dfn = grad(lambda x: x ** 2 + 1j)
|
|
self.assertRaisesRegex(
|
|
TypeError,
|
|
(r"grad requires real-valued outputs \(output dtype that is a "
|
|
r"sub-dtype of np.floating\), but got complex.*"),
|
|
lambda: dfn(3.))
|
|
|
|
def test_holomorphic_grad_of_float_errors(self):
|
|
dfn = grad(lambda x: x ** 2, holomorphic=True)
|
|
self.assertRaisesRegex(
|
|
TypeError,
|
|
(r"grad with holomorphic=True requires inputs with complex dtype, "
|
|
r"but got float.*"),
|
|
lambda: dfn(3.))
|
|
|
|
def test_holomorphic_jacrev_of_float_errors(self):
|
|
dfn = jacrev(lambda x: x ** 2, holomorphic=True)
|
|
self.assertRaisesRegex(
|
|
TypeError,
|
|
(r"jacrev with holomorphic=True requires inputs with complex dtype, "
|
|
r"but got float.*"),
|
|
lambda: dfn(3.))
|
|
|
|
def test_holomorphic_jacfwd_of_float_errors(self):
|
|
dfn = jacfwd(lambda x: x ** 2, holomorphic=True)
|
|
self.assertRaisesRegex(
|
|
TypeError,
|
|
(r"jacfwd with holomorphic=True requires inputs with complex dtype, "
|
|
r"but got float.*"),
|
|
lambda: dfn(3.))
|
|
|
|
def test_jacfwd_of_complex_errors(self):
|
|
dfn = jacfwd(lambda x: x ** 2)
|
|
self.assertRaisesRegex(
|
|
TypeError,
|
|
(r"jacfwd requires real-valued inputs \(input dtype that is a "
|
|
r"sub-dtype of np.floating\), but got complex.*"),
|
|
lambda: dfn(3. + 1j))
|
|
|
|
def test_compiler_ir(self):
|
|
# TODO(phawkins): merge these tests with the `xla_computation` tests.
|
|
def e(x):
|
|
return jnp.sin(jnp.cos(x))
|
|
hlo = api.jit(e).lower(2.).compiler_ir(dialect="hlo").as_hlo_text()
|
|
self.assertIn(' cosine', hlo)
|
|
self.assertIn(' sine', hlo)
|
|
stablehlo = str(api.jit(e).lower(2.).compiler_ir(dialect="stablehlo"))
|
|
self.assertIn("stablehlo.cosine", stablehlo)
|
|
self.assertIn("stablehlo.sine", stablehlo)
|
|
|
|
def test_concurrent_device_get_and_put(self):
|
|
def f(x):
|
|
for _ in range(100):
|
|
y = jax.device_put(x)
|
|
x = jax.device_get(y)
|
|
return x
|
|
|
|
xs = [self.rng().randn(i) for i in range(10)]
|
|
# Make sure JAX backend is initialised on the main thread since some JAX
|
|
# backends install signal handlers.
|
|
jax.device_put(0)
|
|
with concurrent.futures.ThreadPoolExecutor() as executor:
|
|
futures = [executor.submit(partial(f, x)) for x in xs]
|
|
ys = [f.result() for f in futures]
|
|
for x, y in zip(xs, ys):
|
|
self.assertAllClose(x, y)
|
|
|
|
def test_dtype_from_builtin_types(self):
|
|
for dtype in [bool, int, float, complex]:
|
|
with self.assertNoWarnings():
|
|
x = jnp.array(0, dtype=dtype)
|
|
self.assertEqual(x.dtype, dtypes.canonicalize_dtype(dtype))
|
|
|
|
def test_dtype_warning(self):
|
|
# cf. issue #1230
|
|
if config.enable_x64.value:
|
|
raise unittest.SkipTest("test only applies when x64 is disabled")
|
|
|
|
def check_warning(warn, nowarn):
|
|
with self.assertWarnsRegex(UserWarning, "Explicitly requested dtype"):
|
|
warn()
|
|
with self.assertNoWarnings():
|
|
nowarn()
|
|
|
|
check_warning(lambda: jnp.array([1, 2, 3], dtype="float64"),
|
|
lambda: jnp.array([1, 2, 3], dtype="float32"))
|
|
check_warning(lambda: jnp.array([1, 2, 3], dtype="float64"),
|
|
lambda: jnp.array([1, 2, 3], dtype=float))
|
|
check_warning(lambda: jnp.ones(3, dtype=np.float64),
|
|
lambda: jnp.ones(3))
|
|
check_warning(lambda: jnp.ones(3, dtype=np.float64),
|
|
lambda: jnp.ones(3, dtype=float))
|
|
check_warning(lambda: jnp.ones_like(3, dtype=np.int64),
|
|
lambda: jnp.ones_like(3, dtype=np.int32))
|
|
check_warning(lambda: jnp.zeros(3, dtype="int64"),
|
|
lambda: jnp.zeros(3, dtype="int32"))
|
|
check_warning(lambda: jnp.zeros_like(3, dtype="float64"),
|
|
lambda: jnp.zeros_like(3, dtype="float32"))
|
|
check_warning(lambda: jnp.full((2, 3), 1, dtype="int64"),
|
|
lambda: jnp.full((2, 3), 1))
|
|
check_warning(lambda: jnp.ones(3).astype("float64"),
|
|
lambda: jnp.ones(3).astype("float32"))
|
|
check_warning(lambda: jnp.eye(3, dtype=np.float64),
|
|
lambda: jnp.eye(3))
|
|
check_warning(lambda: jnp.arange(3, dtype=np.float64),
|
|
lambda: jnp.arange(3, dtype=np.float32))
|
|
check_warning(lambda: jnp.linspace(0, 3, dtype=np.float64),
|
|
lambda: jnp.linspace(0, 3, dtype=np.float32))
|
|
check_warning(lambda: jnp.tri(2, dtype="float64"),
|
|
lambda: jnp.tri(2, dtype="float32"))
|
|
check_warning(lambda: jnp.arange(1).astype("float64"),
|
|
lambda: jnp.arange(1).astype(float))
|
|
check_warning(lambda: jnp.arange(1.0).astype("int64"),
|
|
lambda: jnp.arange(1.0).astype(int))
|
|
|
|
def test_error_for_invalid_dtype(self):
|
|
err_str = ("Error interpreting argument to .* as an abstract array. The problematic "
|
|
r"value is of type .* and was passed to the function at path args\[1\].")
|
|
with jax.enable_checks(False):
|
|
with self.assertRaisesRegex(TypeError, err_str):
|
|
lax.add(jnp.array(7), np.array("hello"))
|
|
# TODO(dougalm): re-enable checks at the beginning of `bind`. We just
|
|
# need to know which arguments to a generic primitive are ordinary operands vs functions.
|
|
# with jax.enable_checks(True):
|
|
# with self.assertRaises(AssertionError):
|
|
# lax.add(jnp.array(7), np.array("hello"))
|
|
|
|
def test_vmap_preserves_docstr(self):
|
|
def superfun(a):
|
|
"""Does things with stuff."""
|
|
pass
|
|
|
|
self.assertRegex(api.vmap(superfun).__doc__, "\n".join([
|
|
"Vectorized version of superfun.*",
|
|
"",
|
|
"Original documentation:",
|
|
"",
|
|
superfun.__doc__,
|
|
]))
|
|
|
|
def test_vmap_in_axes_list(self):
|
|
# https://github.com/jax-ml/jax/issues/2367
|
|
dictionary = {'a': 5., 'b': jnp.ones(2)}
|
|
x = jnp.zeros(3)
|
|
y = jnp.arange(3.)
|
|
|
|
def f(dct, x, y):
|
|
return dct['a'] + dct['b'] + x + y
|
|
|
|
out1 = api.vmap(f, (None, 0, 0))(dictionary, x, y)
|
|
out2 = api.vmap(f, [None, 0, 0])(dictionary, x, y)
|
|
self.assertAllClose(out1, out2)
|
|
|
|
def test_vmap_in_axes_non_tuple_error(self):
|
|
# https://github.com/jax-ml/jax/issues/18548
|
|
with self.assertRaisesRegex(
|
|
TypeError,
|
|
re.escape("vmap in_axes must be an int, None, or a tuple of entries corresponding "
|
|
"to the positional arguments passed to the function, but got {'a': 0}.")):
|
|
jax.vmap(lambda x: x['a'], in_axes={'a': 0})
|
|
|
|
def test_vmap_in_axes_wrong_length_tuple_error(self):
|
|
# https://github.com/jax-ml/jax/issues/18548
|
|
with self.assertRaisesRegex(
|
|
ValueError,
|
|
re.escape("vmap in_axes must be an int, None, or a tuple of entries corresponding to the "
|
|
"positional arguments passed to the function, but got len(in_axes)=2, len(args)=1")):
|
|
jax.vmap(lambda x: x['a'], in_axes=(0, {'a': 0}))({'a': jnp.zeros((3, 3))})
|
|
|
|
def test_vmap_in_axes_tree_prefix_error(self):
|
|
# https://github.com/jax-ml/jax/issues/795
|
|
value_tree = jnp.ones(3)
|
|
self.assertRaisesRegex(
|
|
ValueError,
|
|
"vmap in_axes specification must be a tree prefix of the corresponding "
|
|
r"value, got specification \(\[0\],\) for value tree "
|
|
+ re.escape(f"{jax.tree.structure((value_tree,))}."),
|
|
lambda: api.vmap(lambda x: x, in_axes=([0],))(value_tree)
|
|
)
|
|
|
|
def test_vmap_in_axes_leaf_types(self):
|
|
with self.assertRaisesRegex(
|
|
TypeError, r"vmap in_axes must be an int, None, or .*"):
|
|
api.vmap(lambda x: x, in_axes=(jnp.array([1., 2.]),))(jnp.array([1., 2.]))
|
|
|
|
def test_vmap_out_axes_leaf_types(self):
|
|
with self.assertRaisesRegex(
|
|
TypeError, r"vmap out_axes must be an int, None, or .*"):
|
|
api.vmap(lambda x: x, out_axes=(jnp.array([1., 2.]),))(jnp.array([1., 2.]))
|
|
|
|
def test_vmap_unbatched_object_passthrough_issue_183(self):
|
|
# https://github.com/jax-ml/jax/issues/183
|
|
fun = lambda f, x: f(x)
|
|
vfun = api.vmap(fun, (None, 0))
|
|
ans = vfun(lambda x: x + 1, jnp.arange(3))
|
|
self.assertAllClose(ans, np.arange(1, 4), check_dtypes=False)
|
|
|
|
def test_vmap_mismatched_keyword(self):
|
|
# https://github.com/jax-ml/jax/issues/10193
|
|
@jax.vmap
|
|
def f(x, y):
|
|
return x + y
|
|
|
|
with self.assertRaisesRegex(
|
|
ValueError,
|
|
"vmap got inconsistent sizes for array axes to be mapped:\n"
|
|
r" \* one axis had size 1: axis 0 of argument x of type int32\[1\];"
|
|
"\n"
|
|
r" \* one axis had size 2: axis 0 of kwargs\['y'\] of type int32\[2\]"):
|
|
f(jnp.array([1], 'int32'), y=jnp.array([1, 2], 'int32'))
|
|
|
|
def test_vmap_mismatched_axis_sizes_error_message_issue_705(self):
|
|
# https://github.com/jax-ml/jax/issues/705
|
|
def h(a, b):
|
|
return jnp.sum(a) + jnp.sum(b)
|
|
|
|
X = self.rng().randn(10, 4).astype('float32')
|
|
U = self.rng().randn(10, 2).astype('float32')
|
|
|
|
with self.assertRaisesRegex(
|
|
ValueError,
|
|
"vmap got inconsistent sizes for array axes to be mapped:\n"
|
|
r" \* one axis had size 10: axis 0 of argument a of type float32\[10,4\];""\n"
|
|
r" \* one axis had size 2: axis 1 of argument b of type float32\[10,2\]"):
|
|
api.vmap(h, in_axes=(0, 1))(X, U)
|
|
|
|
with self.assertRaisesRegex(
|
|
ValueError,
|
|
"vmap got inconsistent sizes for array axes to be mapped:\n"
|
|
r" \* most axes \(2 of them\) had size 10, e.g. axis 0 of argument x "
|
|
r"of type float32\[10,4\];" "\n"
|
|
r" \* one axis had size 2: axis 1 of argument y of type float32\[10,2\]"):
|
|
api.vmap(lambda x, y, z: None, in_axes=(0, 1, 0))(X, U, X)
|
|
|
|
with self.assertRaisesRegex(
|
|
ValueError,
|
|
"vmap got inconsistent sizes for array axes to be mapped:\n"
|
|
r" \* most axes \(2 of them\) had size 2, e.g. axis 1 of argument b\[0\] "
|
|
r"of type float32\[10,2\];" "\n"
|
|
r" \* one axis had size 10: axis 0 of argument a of type float32\[10,4\]"):
|
|
api.vmap(h, in_axes=(0, 1))(X, [U, U])
|
|
|
|
error = (r"vmap was requested to map its argument along axis 0, which "
|
|
r"implies that its rank should be at least 1, but is only 0 "
|
|
r"\(its shape is \(\)\)")
|
|
with self.assertRaisesRegex(ValueError, error):
|
|
# The mapped inputs cannot be scalars
|
|
api.vmap(lambda x: x)(1.)
|
|
|
|
with self.assertRaisesRegex(
|
|
ValueError, "vmap must have at least one non-None value in in_axes"):
|
|
# If the output is mapped, there must be a non-None in_axes
|
|
api.vmap(lambda x: x, in_axes=None)(jnp.array([1., 2.]))
|
|
|
|
error = (r"vmap was requested to map its argument along axis 1, which "
|
|
r"implies that its rank should be at least 2, but is only 1 "
|
|
r"\(its shape is \(2,\)\)")
|
|
with self.assertRaisesRegex(ValueError, error):
|
|
api.vmap(lambda x: x, in_axes=1)(jnp.array([1., 2.]))
|
|
|
|
# Error is: TypeError: only integer scalar arrays can be converted to a scalar index
|
|
with self.assertRaisesRegex(
|
|
ValueError,
|
|
"vmap out_axes specification must be a tree prefix of the "
|
|
"corresponding value.*"):
|
|
api.vmap(lambda x: x, in_axes=0, out_axes=(2, 3))(jnp.array([1., 2.]))
|
|
|
|
with self.assertRaisesRegex(
|
|
ValueError,
|
|
r"vmap has mapped output \(axis_name='foo'\) but out_axes is None"):
|
|
# If the output is mapped (user-named axis), then there must be some
|
|
# out_axes specified.
|
|
api.vmap(lambda x: x, out_axes=None, axis_name="foo")(jnp.array([1., 2.]))
|
|
|
|
with self.assertRaisesRegex(
|
|
ValueError,
|
|
"at vmap out_axes"):
|
|
# If the output is mapped (unnamed axis), then there must be some out_axes
|
|
# specified.
|
|
api.vmap(lambda x: x, out_axes=None)(jnp.array([1., 2.]))
|
|
|
|
def test_vmap_structured_in_axes(self):
|
|
|
|
A, B, C, D = 2, 3, 4, 5
|
|
K = 6 # batch size
|
|
x = np.ones((K, A, B)) # batch axis in different locations
|
|
y = np.ones((B, K, C))
|
|
z = np.ones((C, D, K))
|
|
|
|
def foo(tree_arg):
|
|
x, (y, z) = tree_arg
|
|
return jnp.dot(x, jnp.dot(y, z))
|
|
|
|
tree = (x, (y, z))
|
|
vfoo = api.vmap(foo, in_axes=((0, (1, 2)),))
|
|
self.assertEqual(vfoo(tree).shape, (6, 2, 5))
|
|
|
|
Point = collections.namedtuple("Point", ["x", "y"])
|
|
tree = (x, Point(y, z))
|
|
vfoo = api.vmap(foo, in_axes=((0, Point(1, 2)),))
|
|
self.assertEqual(vfoo(tree).shape, (6, 2, 5))
|
|
|
|
def foo(tree_arg):
|
|
x, dct = tree_arg
|
|
y, z = dct['a'], dct['b']
|
|
return jnp.dot(x, jnp.dot(y, z))
|
|
|
|
tree = (x, {'a': y, 'b': z})
|
|
vfoo = api.vmap(foo, in_axes=((0, {'a': 1, 'b': 2}),))
|
|
self.assertEqual(vfoo(tree).shape, (6, 2, 5))
|
|
|
|
tree = (x, collections.OrderedDict([('a', y), ('b', z)]))
|
|
vfoo = api.vmap(
|
|
foo, in_axes=((0, collections.OrderedDict([('a', 1), ('b', 2)])),))
|
|
self.assertEqual(vfoo(tree).shape, (6, 2, 5))
|
|
|
|
def test_vmap_in_axes_bool_error(self):
|
|
# https://github.com/jax-ml/jax/issues/6372
|
|
with self.assertRaisesRegex(TypeError, "must be an int"):
|
|
api.vmap(lambda x: x, in_axes=False)(jnp.zeros(3))
|
|
|
|
def test_pmap_in_axes_bool_error(self):
|
|
# https://github.com/jax-ml/jax/issues/6372
|
|
with self.assertRaisesRegex(TypeError, "must be an int"):
|
|
api.pmap(lambda x: x, in_axes=False)(jnp.zeros(1))
|
|
|
|
def test_vmap_empty_arguments(self):
|
|
with self.assertRaisesRegex(
|
|
ValueError,
|
|
"vmap wrapped function must be passed at least one argument "
|
|
r"containing an array, got empty \*args=\(\{\},\) and \*\*kwargs=\{\}"):
|
|
api.vmap(lambda x: x)({})
|
|
|
|
def test_pmap_empty_arguments(self):
|
|
with self.assertRaisesRegex(
|
|
ValueError,
|
|
"pmap wrapped function must be passed at least one argument "
|
|
r"containing an array, got empty \*args=\(\{\},\) and \*\*kwargs=\{\}"):
|
|
api.pmap(lambda x: x)({})
|
|
|
|
def test_pmap_global_cache(self):
|
|
def f(x, y):
|
|
return x, y
|
|
|
|
x = np.ones((1, 1, 1))
|
|
|
|
# All defaults
|
|
with jtu.assert_num_jit_and_pmap_compilations(1):
|
|
for _ in range(2):
|
|
api.pmap(f)(x, x)
|
|
|
|
# With axis name
|
|
with jtu.assert_num_jit_and_pmap_compilations(1):
|
|
for _ in range(2):
|
|
api.pmap(f, 'i')(x, x)
|
|
|
|
# With in_axes and out_axes
|
|
for x_in, y_in, x_out, y_out in it.product(*((0, 1, 2) for _ in range(4))):
|
|
with jtu.assert_num_jit_and_pmap_compilations(1):
|
|
for _ in range(2):
|
|
api.pmap(f, 'i', in_axes=(x_in, y_in), out_axes=(x_out, y_out))(x, x)
|
|
|
|
# Forward-mode AD on the outside
|
|
with jtu.assert_num_jit_and_pmap_compilations(1):
|
|
for _ in range(2):
|
|
api.jvp(api.pmap(f), (x, x), (x, x))
|
|
|
|
# Reverse-mode AD on the outside. One compilation for forward, one for backward.
|
|
with jtu.assert_num_jit_and_pmap_compilations(2):
|
|
for _ in range(2):
|
|
api.vjp(api.pmap(f), x, x)[1]((x, x))
|
|
|
|
def test_device_array_repr(self):
|
|
rep = jnp.ones(()) + 1.
|
|
self.assertStartsWith(repr(rep), 'Array')
|
|
|
|
def test_device_array_hash(self):
|
|
rep = jnp.ones((1,)) + 1.
|
|
_check_instance(self, rep)
|
|
self.assertNotIsInstance(rep, collections.abc.Hashable)
|
|
with self.assertRaisesRegex(TypeError, 'unhashable type'):
|
|
hash(rep)
|
|
|
|
def test_grad_without_enough_args_error_message(self):
|
|
# https://github.com/jax-ml/jax/issues/1696
|
|
def f(x, y): return x + y
|
|
df = api.grad(f, argnums=0)
|
|
self.assertRaisesRegex(
|
|
TypeError,
|
|
"differentiating with respect to argnums=0 requires at least 1 "
|
|
"positional arguments to be passed by the caller, but got only 0 "
|
|
"positional arguments.",
|
|
lambda: partial(df, x=0.)(y=1.))
|
|
|
|
def test_grad_object_array_error(self):
|
|
x = np.array([1, 2, 3], dtype=object)
|
|
with self.assertRaisesRegex(TypeError, ".*is not a valid JAX type"):
|
|
jax.grad(lambda x: x)(x)
|
|
|
|
@jtu.thread_unsafe_test() # logging isn't thread-safe
|
|
def test_jit_compilation_time_logging(self):
|
|
@api.jit
|
|
def f(x):
|
|
return x * 2
|
|
|
|
# make sure some initial warnings & cached operations already happen.
|
|
f(jnp.ones(2))
|
|
|
|
prev_level = logging.get_verbosity()
|
|
try:
|
|
logging.set_verbosity('DEBUG')
|
|
with self.assertLogs(level=logging.DEBUG) as l:
|
|
f(2.)
|
|
finally:
|
|
logging.set_verbosity(prev_level)
|
|
self.assertGreaterEqual(len(l.output), 3) # 3 lines
|
|
self.assertTrue(any('Finished tracing' in line for line in l.output))
|
|
self.assertTrue(any('Compiling f' in line for line in l.output))
|
|
self.assertTrue(any('Finished XLA compilation' in line for line in l.output))
|
|
|
|
def test_grad_of_jit_compilation_caching(self):
|
|
if not hasattr(self, "assertLogs"):
|
|
raise unittest.SkipTest("test requires assertLogs (python 3)")
|
|
|
|
# make sure some initial warnings & cached operations already happen.
|
|
api.grad(api.jit(lambda x: x))(1.0)
|
|
|
|
@api.jit
|
|
def f(x):
|
|
return jnp.sin(x)
|
|
|
|
prev_level = logging.get_verbosity()
|
|
try:
|
|
logging.set_verbosity('DEBUG')
|
|
with self.assertLogs(level=logging.DEBUG) as l:
|
|
ans1 = api.grad(f)(2.)
|
|
ans2 = api.grad(f)(3.)
|
|
finally:
|
|
logging.set_verbosity(prev_level)
|
|
self.assertGreaterEqual(len(l.output), 2 * 3) # one for fwd, one for bwd, 3 lines each
|
|
self.assertAllClose(ans1, np.cos(2.), check_dtypes=False)
|
|
self.assertAllClose(ans2, np.cos(3.), check_dtypes=False)
|
|
|
|
def test_grad_of_jit_compilation_caching2(self):
|
|
# Like the above test, but instead of logging use our compile counters.
|
|
|
|
# make sure some initial convert element type operations are pre-cached.
|
|
api.grad(api.jit(lambda x: x))(1.0)
|
|
|
|
@api.jit
|
|
def f(x):
|
|
return jnp.sin(x)
|
|
|
|
with jtu.count_jit_and_pmap_lowerings() as count: # noqa: F841
|
|
_ = jax.grad(f)(3.)
|
|
self.assertEqual(count(), 2) # one for fwd, one for bwd
|
|
|
|
with jtu.count_jit_and_pmap_lowerings() as count: # noqa: F841
|
|
_ = jax.grad(f)(3.)
|
|
_ = jax.grad(f)(4.)
|
|
self.assertEqual(count(), 0) # cache hits on both fwd and bwd
|
|
|
|
def test_grad_does_not_unflatten_tree_with_none(self):
|
|
# https://github.com/jax-ml/jax/issues/7546
|
|
class CustomNode(list):
|
|
pass
|
|
|
|
def unflatten(unused_aux_data, children):
|
|
self.assertIsNotNone(children[0])
|
|
return CustomNode(children)
|
|
|
|
tree_util.register_pytree_node(CustomNode, lambda x: (x, None), unflatten)
|
|
grad(lambda x: x[0])(CustomNode([0.]))
|
|
|
|
def test_trivial_computations(self):
|
|
x = jnp.array([1, 2, 3])
|
|
y = api.jit(lambda x: x)(x)
|
|
self.assertNotEqual(x.unsafe_buffer_pointer(), y.unsafe_buffer_pointer())
|
|
|
|
z1, z2 = api.jit(lambda x: (x, x))(x)
|
|
self.assertNotEqual(z1.unsafe_buffer_pointer(), z2.unsafe_buffer_pointer())
|
|
|
|
x1, x2 = jnp.array([1, 2]), jnp.array([2, 3])
|
|
z1, z2, z3 = api.jit(lambda x, y: (y, 1, x))(x1, x2)
|
|
self.assertNotEqual(z1.unsafe_buffer_pointer(), x2.unsafe_buffer_pointer())
|
|
self.assertNotEqual(z3.unsafe_buffer_pointer(), x1.unsafe_buffer_pointer())
|
|
self.assertEqual(z2, 1)
|
|
|
|
@jtu.thread_unsafe_test() # monkey-patching mlir.jaxpr_subcomp isn't thread-safe
|
|
def test_nested_jit_hoisting(self):
|
|
@api.jit
|
|
def f(x, y):
|
|
z = 2 * x
|
|
return y + z, 3
|
|
|
|
@api.jit
|
|
def g(x):
|
|
return f(2, x)
|
|
|
|
mlir_jaxpr_subcomp = mlir.jaxpr_subcomp
|
|
|
|
jaxprs = []
|
|
def mlir_jaxpr_subcomp_and_collect(c, jaxpr, *args, **kwargs):
|
|
jaxprs.append(jaxpr)
|
|
return mlir_jaxpr_subcomp(c, jaxpr, *args, **kwargs)
|
|
|
|
try:
|
|
mlir.jaxpr_subcomp = mlir_jaxpr_subcomp_and_collect
|
|
ans = g(3)
|
|
finally:
|
|
mlir.jaxpr_subcomp = mlir_jaxpr_subcomp
|
|
|
|
self.assertEqual(ans, (7, 3))
|
|
self.assertLen(jaxprs, 2)
|
|
outer_jaxpr, inner_jaxpr = jaxprs
|
|
|
|
self.assertLen(outer_jaxpr.eqns, 1)
|
|
prim_name = 'pjit'
|
|
jaxpr_param = 'jaxpr'
|
|
self.assertEqual(outer_jaxpr.eqns[0].primitive.name, f'{prim_name}')
|
|
subjaxpr_1 = outer_jaxpr.eqns[0].params[f"{jaxpr_param}"]
|
|
self.assertEqual(str(subjaxpr_1), str(inner_jaxpr))
|
|
self.assertLen(inner_jaxpr.eqns, 2)
|
|
self.assertEqual(inner_jaxpr.eqns[-2].primitive.name, 'mul')
|
|
self.assertEqual(inner_jaxpr.eqns[-1].primitive.name, 'add')
|
|
|
|
@jtu.thread_unsafe_test() # count_primitive_compiles isn't thread-safe
|
|
def test_primitive_compilation_cache(self):
|
|
with jtu.count_primitive_compiles() as count:
|
|
lax.add(1, 2)
|
|
lax.add(2, 3)
|
|
self.assertEqual(count(), 1)
|
|
|
|
def test_arange_jit(self):
|
|
# see https://github.com/jax-ml/jax/issues/553
|
|
def fun(x):
|
|
r = jnp.arange(x.shape[0])[x]
|
|
return r
|
|
|
|
jit(fun)(jnp.array([0, 1, 2], dtype=jnp.int32)) # doesn't crash
|
|
|
|
def helper_save_tracer(self, x):
|
|
self._saved_tracer = x
|
|
return x
|
|
|
|
def test_escaped_tracers_different_top_level_traces(self):
|
|
api.jit(self.helper_save_tracer)(0.)
|
|
with self.assertRaisesRegex(
|
|
UnexpectedTracerError, "Encountered an unexpected tracer"):
|
|
api.jit(lambda x: self._saved_tracer)(0.)
|
|
|
|
def test_escaped_tracers_cant_lift_sublevels(self):
|
|
api.jit(self.helper_save_tracer)(0.)
|
|
with self.assertRaisesRegex(
|
|
UnexpectedTracerError,
|
|
re.compile(
|
|
"Encountered an unexpected tracer",
|
|
re.DOTALL)):
|
|
api.jit(lambda x: x)(self._saved_tracer)
|
|
|
|
@unittest.skip # TODO(dougalm): rethink what this should do under stackless
|
|
def test_escaped_tracers_tracer_from_higher_level(self):
|
|
api.grad(self.helper_save_tracer)(0.)
|
|
with self.assertRaises(UnexpectedTracerError):
|
|
api.grad(lambda x: x)(self._saved_tracer)
|
|
|
|
def test_escaped_tracers_incompatible_sublevel(self):
|
|
def func1(x):
|
|
api.jit(self.helper_save_tracer)(0.)
|
|
# Use the tracer
|
|
return x + self._saved_tracer
|
|
with self.assertRaisesRegex(
|
|
UnexpectedTracerError,
|
|
re.compile("Encountered an unexpected tracer",
|
|
re.DOTALL)):
|
|
api.jit(func1)(2.)
|
|
|
|
def test_escaped_tracers_cant_lift(self):
|
|
def func1(x):
|
|
api.grad(self.helper_save_tracer)(0.)
|
|
return x + self._saved_tracer
|
|
with self.assertRaisesRegex(
|
|
UnexpectedTracerError,
|
|
re.compile("unexpected tracer")):
|
|
api.grad(func1)(2.)
|
|
|
|
def test_escaped_tracers_not_among_input_tracers(self):
|
|
def func1(x):
|
|
api.grad(self.helper_save_tracer)(x)
|
|
# Use the tracer
|
|
return x + self._saved_tracer
|
|
|
|
msg = "Encountered an unexpected tracer"
|
|
with self.assertRaisesRegex(
|
|
UnexpectedTracerError, re.compile(msg, re.DOTALL)):
|
|
api.jit(func1)(2.0)
|
|
|
|
def test_escaped_tracer_omnistaging(self):
|
|
count = 1
|
|
|
|
@jit
|
|
def f():
|
|
nonlocal count
|
|
count = jnp.add(count, 1)
|
|
f() # leaked a tracer! but currently undetected
|
|
|
|
def f(x, c):
|
|
jnp.add(count, 1)
|
|
return None, None
|
|
|
|
@jit
|
|
def g():
|
|
lax.scan(f, None, None, length=2)
|
|
|
|
with self.assertRaisesRegex(UnexpectedTracerError,
|
|
"was created on line"):
|
|
g()
|
|
|
|
def test_escaped_tracer_omnistaging_top_trace(self):
|
|
count = 1
|
|
|
|
def f(_, __):
|
|
nonlocal count
|
|
count = jnp.add(count, 1)
|
|
return None, None
|
|
|
|
lax.scan(f, None, None, length=2) # leaked a tracer! (of level 1!)
|
|
|
|
with self.assertRaisesRegex(UnexpectedTracerError,
|
|
"was created on line"):
|
|
# The following call will try and raise the ones array to the count tracer
|
|
# level, which is no longer live.
|
|
jax.jit(jnp.add)(jnp.ones(()), count)
|
|
|
|
|
|
def test_escaped_tracer_shape_dtype(self):
|
|
with self.assertRaisesRegex(core.UnexpectedTracerError, r"int32\[4,3\]"):
|
|
jax.jit(self.helper_save_tracer)(jnp.ones((4, 3), dtype=jnp.int32))
|
|
_ = self._saved_tracer+1
|
|
|
|
def test_pmap_static_kwarg_error_message(self):
|
|
# https://github.com/jax-ml/jax/issues/3007
|
|
def f(a, b):
|
|
return a + b
|
|
|
|
g = jax.pmap(f, static_broadcasted_argnums=(1,))
|
|
|
|
msg = (r"pmapped function has static_broadcasted_argnums=\(1,\) but was "
|
|
r"called with only 1 positional argument. All static broadcasted "
|
|
r"arguments must be passed positionally.")
|
|
with self.assertRaisesRegex(ValueError, msg):
|
|
g(jnp.ones((1, 1)), b=1)
|
|
|
|
def test_vmap_unmapped_last(self):
|
|
@partial(jax.vmap, out_axes=-1)
|
|
def f(x):
|
|
return np.zeros((2,))
|
|
f(np.zeros((5,)))
|
|
|
|
# TODO(jakevdp): re-enable this if possible.
|
|
@unittest.skipIf(True, "broken by convert_element_type change.")
|
|
def test_xla_constant_dedup(self):
|
|
y = np.array([7, 14], dtype=np.float32)
|
|
def f(x):
|
|
return x + y + y
|
|
|
|
x = np.array([1, 2], dtype=np.float32)
|
|
hlo_lines = jax.jit(f).lower(x).as_text('hlo').split('\n')
|
|
hlo_lines = {s.strip() for s in hlo_lines}
|
|
self.assertIn('constant.1 = f32[2]{0} constant({7, 14})', hlo_lines)
|
|
self.assertNotIn('constant.2 = f32[2]{0} constant({7, 14})', hlo_lines)
|
|
|
|
def test_eval_context(self):
|
|
@jit
|
|
def f():
|
|
with core.eval_context():
|
|
assert jnp.add(1, 1) == 2
|
|
|
|
f() # doesn't crash
|
|
|
|
def test_linearize_aux(self):
|
|
def fn(x):
|
|
return x * 2 - 3, x > 0
|
|
|
|
f, lin_fn, aux = api.linearize(fn, 3.4, has_aux=True)
|
|
tang = lin_fn(5.)
|
|
|
|
self.assertAllClose(f, 3.8)
|
|
self.assertAllClose(tang, 10.)
|
|
self.assertEqual(aux, True)
|
|
|
|
def test_linearize_aval_error(self):
|
|
# https://github.com/jax-ml/jax/issues/4622
|
|
f = lambda x: x
|
|
|
|
# these should not error
|
|
_, f_jvp = api.linearize(f, 1.)
|
|
f_jvp(1.)
|
|
_, f_jvp = api.linearize(f, np.ones(2, np.int32))
|
|
f_jvp(np.zeros(2, float0))
|
|
|
|
# these should error
|
|
_, f_jvp = api.linearize(f, 1.)
|
|
with self.assertRaisesRegex(ValueError, "tangent values inconsistent"):
|
|
f_jvp(1)
|
|
_, f_jvp = api.linearize(f, np.ones(2, np.int32))
|
|
with self.assertRaisesRegex(ValueError, "tangent values inconsistent"):
|
|
f_jvp(np.ones(2, np.int32))
|
|
|
|
def test_grad_of_token_consuming_primitive(self):
|
|
# https://github.com/jax-ml/jax/issues/5463
|
|
tokentest_p = core.Primitive("tokentest")
|
|
tokentest_p.def_impl(partial(xla.apply_primitive, tokentest_p))
|
|
tokentest_p.def_abstract_eval(lambda x, y: x)
|
|
mlir.register_lowering(tokentest_p, lambda ctx, x, y: [x])
|
|
ad.defjvp(tokentest_p, (lambda g, x, token: x), None)
|
|
|
|
token = jax.lax.create_token(123)
|
|
arr = jnp.ones((3, 2))
|
|
res, vjp_fun = jax.vjp(lambda x: tokentest_p.bind(x, token), arr)
|
|
# Should not crash.
|
|
vjp_fun(arr)
|
|
|
|
def test_jit_returning_token(self):
|
|
x = jax.jit(jax.lax.create_token)(1.0)
|
|
self.assertIsInstance(x, core.Token)
|
|
|
|
def test_jit_capturing_token(self):
|
|
tok = jax.lax.create_token()
|
|
_, y = jax.jit(lambda x: (x + 2, tok))(7)
|
|
self.assertIsInstance(y, core.Token)
|
|
|
|
def test_leak_checker_catches_a_jit_leak(self):
|
|
with jax.checking_leaks():
|
|
lst = []
|
|
|
|
@jit
|
|
def f(x):
|
|
lst.append(x)
|
|
return x
|
|
|
|
with self.assertRaisesRegex(Exception, r"Leaked"):
|
|
f(3)
|
|
|
|
def test_leak_checker_catches_a_pmap_leak(self):
|
|
with jax.checking_leaks():
|
|
lst = []
|
|
|
|
@api.pmap
|
|
def f(x):
|
|
lst.append(x)
|
|
return x
|
|
|
|
with self.assertRaisesRegex(Exception, r"Leaked"):
|
|
f(np.ones(1))
|
|
|
|
def test_leak_checker_catches_a_grad_leak(self):
|
|
with jax.checking_leaks():
|
|
lst = []
|
|
|
|
def f(x):
|
|
lst.append(x)
|
|
return x
|
|
|
|
with self.assertRaisesRegex(Exception, r"Leaked trace"):
|
|
api.grad(f)(3.)
|
|
|
|
def test_leak_checker_avoids_false_positives(self):
|
|
with jax.checking_leaks():
|
|
api.vmap(lambda x: x)(np.arange(3.)) # doesn't crash
|
|
|
|
@jit
|
|
def f(x):
|
|
return x
|
|
f(3) # doesn't crash
|
|
api.vmap(f)(np.arange(3)) # doesn't crash
|
|
api.grad(f)(3.) # doesn't crash
|
|
|
|
@api.pmap
|
|
def f(x):
|
|
return x
|
|
f(np.ones(1)) # doesn't crash
|
|
api.vmap(f)(np.ones((1, 1))) # doesn't crash
|
|
|
|
def test_leak_checker_catches_a_scan_leak(self):
|
|
with jax.checking_leaks():
|
|
lst = []
|
|
|
|
to_scan = lambda c, x: (lst.append(c) or jnp.sin(c), None)
|
|
|
|
with self.assertRaisesRegex(Exception, r"Leaked trace"):
|
|
lax.scan(to_scan, 1., np.arange(3.))
|
|
|
|
def test_leak_checker_avoids_false_positives_scan(self):
|
|
with jax.checking_leaks():
|
|
to_scan = lambda c, x: (jnp.sin(c), None)
|
|
lax.scan(to_scan, 1., np.arange(3.)) # doesn't crash
|
|
|
|
def test_leak_checker_avoids_false_positives_scan_jvp(self):
|
|
with jax.checking_leaks():
|
|
to_scan = lambda c, x: (c, None)
|
|
|
|
def f(x):
|
|
lax.scan(to_scan, x, None, length=1)
|
|
api.jvp(f, (3.,), (1.,)) # doesn't crash
|
|
|
|
def test_leak_checker_avoids_false_positives_scan_vmap(self):
|
|
with jax.checking_leaks():
|
|
to_scan = lambda c, _: (1., None)
|
|
|
|
@api.vmap
|
|
def f(x):
|
|
lax.scan(to_scan, x, None, length=1)
|
|
f(np.arange(5.)) # doesn't crash
|
|
|
|
def test_leak_checker_avoids_false_positives_scan_vmap_2(self):
|
|
with jax.checking_leaks():
|
|
to_scan = lambda c, _: (c, None)
|
|
|
|
@api.vmap
|
|
def f(x):
|
|
lax.scan(to_scan, x, None, length=1)
|
|
f(np.arange(5.)) # doesn't crash
|
|
|
|
def test_leak_checker_catches_a_sublevel_leak(self):
|
|
with jax.checking_leaks():
|
|
@jit
|
|
def f(x):
|
|
lst = []
|
|
@jit
|
|
def g(x):
|
|
lst.append(x)
|
|
return x
|
|
|
|
x = g(x)
|
|
return x
|
|
|
|
msg = r'Leaked trace DynamicJaxprTrace'
|
|
with self.assertRaisesRegex(Exception, f"{msg}"):
|
|
f(3)
|
|
|
|
def test_leak_checker_avoids_false_positive_custom_jvp(self):
|
|
# see https://github.com/jax-ml/jax/issues/5636
|
|
with jax.checking_leaks():
|
|
@jax.custom_jvp
|
|
def t(y):
|
|
return y
|
|
|
|
def t_jvp(p, t):
|
|
pass
|
|
|
|
t.defjvp(t_jvp)
|
|
|
|
@jit
|
|
def s(y):
|
|
return t(y)
|
|
s(3) # doesn't crash
|
|
|
|
def test_leak_checker_internal_error(self):
|
|
def apply_fn(inp):
|
|
fn = jax.checkpoint(lambda x: jax.nn.relu(1.0 * x))
|
|
return jax.vjp(fn, inp)
|
|
|
|
with jax.check_tracer_leaks():
|
|
jax.jit(apply_fn)(1.0) # don't crash
|
|
|
|
def test_leak_checker_reference_chain(self):
|
|
class A:
|
|
def __init__(self, dct):
|
|
self.dct = dct
|
|
|
|
a = A({})
|
|
x = jnp.arange(3)
|
|
|
|
def sketch(x):
|
|
def foo():
|
|
return x
|
|
a.dct['hi'] = [foo]
|
|
return x
|
|
|
|
# TODO(mattjj): full test msg below fails (harmlessly) on CI, investigate
|
|
msg = (
|
|
r"This BatchTracer with object id [0-9]+ was created on line:\n"
|
|
r" .*\n"
|
|
r"<BatchTracer [0-9]+> is referred to by"
|
|
)
|
|
|
|
# msg = (
|
|
# r"This BatchTracer with object id [0-9]+ was created on line:\n"
|
|
# r" .*\n"
|
|
# r"<BatchTracer [0-9]+> is referred to by <function [0-9]+> \(foo\) "
|
|
# r"closed-over variable x\n"
|
|
# r"<function [0-9]+> is referred to by <list [0-9]+>\[0\]\n"
|
|
# r"<list [0-9]+> is referred to by <dict [0-9]+>\['hi'\]\n"
|
|
# r"<dict [0-9]+> is referred to by <A [0-9]+>\.dct\n"
|
|
# )
|
|
|
|
with jax.check_tracer_leaks():
|
|
with self.assertRaisesRegex(Exception, msg):
|
|
jax.vmap(sketch)(x)
|
|
|
|
def test_default_backend(self):
|
|
first_local_device = jax.local_devices()[0]
|
|
self.assertEqual(first_local_device.platform, jax.default_backend())
|
|
|
|
@jtu.skip_on_devices("cpu")
|
|
def test_default_device(self):
|
|
system_default_devices = jnp.add(1, 1).devices()
|
|
self.assertLen(system_default_devices, 1)
|
|
test_device = jax.devices("cpu")[-1]
|
|
|
|
# Sanity check creating array using system default device
|
|
self.assertEqual(jnp.ones(1).devices(), system_default_devices)
|
|
|
|
# Create array with default_device set
|
|
with jax.default_device(test_device):
|
|
# Hits cached primitive path
|
|
self.assertEqual(jnp.ones(1).devices(), {test_device})
|
|
# Uncached
|
|
self.assertEqual(jnp.zeros((1, 2)).devices(), {test_device})
|
|
|
|
# Test that we can reset to system default device
|
|
self.assertEqual(jnp.ones(1).devices(), system_default_devices)
|
|
|
|
def test_dunder_jax_array(self):
|
|
# https://github.com/jax-ml/jax/pull/4725
|
|
|
|
class AlexArray:
|
|
def __init__(self, jax_val):
|
|
self.jax_val = jax_val
|
|
def __jax_array__(self):
|
|
return self.jax_val
|
|
dtype = property(lambda self: self.jax_val.dtype)
|
|
shape = property(lambda self: self.jax_val.shape)
|
|
|
|
x = AlexArray(jnp.array([1., 2., 3.]))
|
|
y = jnp.sin(x)
|
|
self.assertAllClose(y, jnp.sin(jnp.array([1., 2., 3.])))
|
|
y = api.grad(api.jit(lambda x: jnp.sin(x).sum()))(x)
|
|
self.assertAllClose(y, jnp.cos(jnp.array([1., 2., 3.])))
|
|
|
|
x = AlexArray(jnp.array([[1., 2., 3.]]))
|
|
y = api.pmap(jnp.sin)(x)
|
|
self.assertAllClose(y, jnp.sin(jnp.array([[1., 2., 3.]])))
|
|
|
|
x = jnp.array(1)
|
|
a = AlexArray(x)
|
|
for f in [jnp.isscalar, jnp.size, jnp.shape, jnp.dtype]:
|
|
self.assertEqual(f(x), f(a))
|
|
|
|
x = AlexArray(jnp.array(1))
|
|
a1 = jnp.array(x)
|
|
self.assertAllClose(1, a1)
|
|
|
|
a2 = jnp.array(((x, x), [x, x]))
|
|
self.assertAllClose(np.array(((1, 1), (1, 1))), a2)
|
|
|
|
@jtu.thread_unsafe_test() # count_jit_tracing_cache_miss() isn't thread-safe
|
|
def test_eval_shape_weak_type(self):
|
|
# https://github.com/jax-ml/jax/issues/23302
|
|
arr = jax.numpy.array(1)
|
|
|
|
def f(x):
|
|
return jax.numpy.array(x)
|
|
|
|
with jtu.count_jit_tracing_cache_miss() as count:
|
|
jax.eval_shape(f, 1)
|
|
out = jax.eval_shape(f, 1)
|
|
|
|
self.assertEqual(count(), 1)
|
|
self.assertTrue(out.weak_type)
|
|
self.assertEqual(out.weak_type, arr.weak_type)
|
|
|
|
def test_dunder_jax_array_bug(self):
|
|
@jax.tree_util.register_pytree_node_class
|
|
class A:
|
|
x: jax.Array
|
|
|
|
def __init__(self, x: jax.Array):
|
|
self.x = x
|
|
|
|
def tree_flatten(self):
|
|
return ((self.x,), None)
|
|
|
|
@classmethod
|
|
def tree_unflatten(cls, _, children):
|
|
x, = children
|
|
return cls(x)
|
|
|
|
def __jax_array__(self) -> jax.Array:
|
|
return self.x
|
|
|
|
ndim = property(operator.attrgetter('x.ndim'))
|
|
dtype = property(operator.attrgetter('x.dtype'))
|
|
shape = property(operator.attrgetter('x.shape'))
|
|
|
|
a = A(jnp.ones((3, 3)))
|
|
jnp.asarray(a) # don't crash
|
|
|
|
f = jax.jit(jnp.matmul)
|
|
f(a, a) # don't crash
|
|
|
|
def test_constant_handler_mro(self):
|
|
# https://github.com/jax-ml/jax/issues/6129
|
|
|
|
class Foo(enum.IntEnum):
|
|
bar = 1
|
|
|
|
@api.pmap
|
|
def f(_):
|
|
return Foo.bar
|
|
|
|
ans = f(jnp.arange(1)) # doesn't crash
|
|
expected = jnp.arange(1) + 1
|
|
self.assertAllClose(ans, expected, check_dtypes=False)
|
|
|
|
@parameterized.named_parameters([
|
|
{"testcase_name": f"{dtype.__name__}", "dtype": dtype}
|
|
for dtype in jtu.dtypes.all])
|
|
def test_constant_handlers(self, dtype):
|
|
# https://github.com/jax-ml/jax/issues/9380
|
|
@jax.jit
|
|
def f():
|
|
return jnp.exp(dtype(0))
|
|
f() # doesn't error
|
|
|
|
def test_vmap_make_jaxpr_close_over_tracer(self):
|
|
def run(inp):
|
|
def f(x, y):
|
|
return x + y
|
|
g = lambda x: f(x, inp)
|
|
jaxpr = jax.make_jaxpr(g)(1)
|
|
return jax.core.eval_jaxpr(jaxpr.jaxpr, jaxpr.consts, 1)
|
|
|
|
jax.vmap(run)(jnp.arange(2)) # doesn't crash
|
|
|
|
def test_large_python_ints(self):
|
|
with self.assertRaises(OverflowError):
|
|
jnp.multiply(2 ** 100, 3.)
|
|
|
|
out = lax.convert_element_type(2 ** 100, jnp.float32) # doesn't crash
|
|
self.assertArraysEqual(out, np.float32(2 ** 100))
|
|
|
|
def test_dot_precision_context_manager(self):
|
|
x = jnp.zeros((2, 2))
|
|
|
|
with jax.default_matmul_precision(None):
|
|
jnp.dot(x, x) # doesn't crash
|
|
jaxpr = jax.make_jaxpr(jnp.dot)(x, x)
|
|
# self.assertIn('precision=None', str(jaxpr))
|
|
self.assertIs(jaxpr.jaxpr.eqns[0].params['precision'], None)
|
|
|
|
with jax.default_matmul_precision("bfloat16"):
|
|
x @ x # doesn't crash
|
|
jaxpr = jax.make_jaxpr(op.matmul)(x, x)
|
|
self.assertIn('Precision.DEFAULT', str(jaxpr))
|
|
|
|
with jax.default_matmul_precision("tensorfloat32"):
|
|
jnp.dot(x, x) # doesn't crash
|
|
jaxpr = jax.make_jaxpr(jnp.dot)(x, x)
|
|
self.assertIn('Precision.HIGH', str(jaxpr))
|
|
|
|
with jax.default_matmul_precision("float32"):
|
|
jnp.dot(x, x) # doesn't crash
|
|
jaxpr = jax.make_jaxpr(jnp.dot)(x, x)
|
|
self.assertIn('Precision.HIGHEST', str(jaxpr))
|
|
|
|
dot = partial(jnp.dot, precision=lax.Precision.HIGHEST)
|
|
with jax.default_matmul_precision("tensorfloat32"):
|
|
dot(x, x) # doesn't crash
|
|
jaxpr = jax.make_jaxpr(dot)(x, x)
|
|
self.assertIn('Precision.HIGHEST', str(jaxpr))
|
|
|
|
def test_dot_precision_flag(self):
|
|
x = jnp.zeros((2, 2))
|
|
|
|
with config.default_matmul_precision("tensorfloat32"):
|
|
jnp.dot(x, x) # doesn't crash
|
|
jaxpr = jax.make_jaxpr(jnp.dot)(x, x)
|
|
self.assertIn('Precision.HIGH', str(jaxpr))
|
|
|
|
with config.default_matmul_precision("tensorfloat32"):
|
|
jnp.dot(x, x) # doesn't crash
|
|
jaxpr = jax.make_jaxpr(jnp.dot)(x, x)
|
|
self.assertIn('Precision.HIGH', str(jaxpr))
|
|
|
|
@jtu.thread_unsafe_test() # Updating global configs is not thread-safe.
|
|
def test_dot_precision_forces_retrace(self):
|
|
num_traces = 0
|
|
|
|
def g(x):
|
|
nonlocal num_traces
|
|
num_traces += 1
|
|
return jnp.dot(x, x)
|
|
def f_cond(x):
|
|
return lax.cond(True, g, g, x)
|
|
|
|
@jax.jit
|
|
def f_jit(x):
|
|
nonlocal num_traces
|
|
num_traces += 1
|
|
return jnp.dot(x, x)
|
|
|
|
for f in [f_jit, f_cond]:
|
|
# Use _read() to read the flag value rather than threadlocal value.
|
|
precision = config._read("jax_default_matmul_precision")
|
|
try:
|
|
num_traces = 0
|
|
x = jnp.zeros((2, 2))
|
|
f(x)
|
|
self.assertEqual(num_traces, 1)
|
|
f(x)
|
|
self.assertEqual(num_traces, 1)
|
|
with jax.default_matmul_precision("tensorfloat32"):
|
|
f(x)
|
|
self.assertEqual(num_traces, 2)
|
|
config.update("jax_default_matmul_precision", "float32")
|
|
f(x)
|
|
self.assertGreaterEqual(num_traces, 2)
|
|
nt = num_traces
|
|
f(x)
|
|
self.assertEqual(num_traces, nt + 1)
|
|
f(x)
|
|
self.assertEqual(num_traces, nt + 1)
|
|
finally:
|
|
config.update("jax_default_matmul_precision", precision)
|
|
|
|
def test_backward_pass_ref_dropping(self):
|
|
refs = []
|
|
|
|
@jax.custom_vjp
|
|
def f(x):
|
|
return x
|
|
def f_fwd(x):
|
|
return x, None
|
|
def f_rev(_, g):
|
|
assert len(refs) != 2 or refs[0]() is None
|
|
zero = np.zeros(())
|
|
refs.append(weakref.ref(zero))
|
|
return (zero,)
|
|
f.defvjp(f_fwd, f_rev)
|
|
|
|
api.grad(lambda x: f(f(f(x))))(1.)
|
|
|
|
def test_jit_inline(self):
|
|
@partial(api.jit, inline=False)
|
|
def f(x):
|
|
return x * 2
|
|
|
|
jaxpr = api.make_jaxpr(f)(3)
|
|
self.assertIn('pjit', str(jaxpr))
|
|
|
|
@partial(api.jit, inline=True)
|
|
def f(x):
|
|
return x * 2
|
|
|
|
jaxpr = api.make_jaxpr(f)(3)
|
|
self.assertNotIn('pjit', str(jaxpr))
|
|
|
|
# Repro for https://github.com/jax-ml/jax/issues/7229.
|
|
def test_compute_with_large_transfer(self):
|
|
def f(x, delta):
|
|
return x + jnp.asarray(delta, x.dtype)
|
|
|
|
# A large and potentially unaligned array to trigger non-zero-copy and
|
|
# async device array copy.
|
|
xs = self.rng().uniform(0., 1., size=(10, 131, 111, 3)).astype(np.float32)
|
|
for x in xs:
|
|
delta = self.rng().uniform(-0.5, 0.5, size=())
|
|
jitted_f = api.jit(f)
|
|
np.testing.assert_allclose(jitted_f(x, delta), f(x, delta))
|
|
|
|
def test_vjp_fun_jit(self):
|
|
# test that the function returned by vjp can be returned
|
|
# from and passed to jitted functions
|
|
f = lambda x: 2. * x
|
|
|
|
@partial(jit, static_argnums=0)
|
|
def linearize_vjp(f, x):
|
|
_, vjp_fun = api.vjp(f, x)
|
|
return vjp_fun
|
|
|
|
linearized = linearize_vjp(f, 1.)
|
|
actual = jit(lambda f, x: f(x))(linearized, 3.)
|
|
expected = (6.,)
|
|
self.assertEqual(actual, expected)
|
|
|
|
def test_linearize_fun_jit(self):
|
|
# test that the function returned by linearize can be returned
|
|
# from and passed to jitted functions
|
|
f = lambda x: 2. * x
|
|
|
|
@partial(jit, static_argnums=0)
|
|
def linearize(f, x):
|
|
_, jvp_fun = api.linearize(f, x)
|
|
return jvp_fun
|
|
|
|
linearized = linearize(f, 1.)
|
|
actual = jit(lambda f, x: f(x))(linearized, 3.)
|
|
expected = 6.
|
|
self.assertEqual(actual, expected)
|
|
|
|
def test_linear_transpose_fun_jit(self):
|
|
# test that the function returned by linear_transpose can be returned
|
|
# from and passed to jitted functions
|
|
f = lambda x: 2. * x
|
|
|
|
@partial(jit, static_argnums=0)
|
|
def transpose(f, x):
|
|
return api.linear_transpose(f, x)
|
|
|
|
transposed = transpose(f, 1.)
|
|
actual = jit(lambda f, x: f(x))(transposed, 3.)
|
|
expected = (6.,)
|
|
self.assertEqual(actual, expected)
|
|
|
|
def test_leaked_tracer_issue_7613(self):
|
|
# from https://github.com/jax-ml/jax/issues/7613
|
|
import numpy.random as npr
|
|
|
|
def sigmoid(x):
|
|
return 1. / (1. + jnp.exp(-x))
|
|
|
|
x = jnp.ones((1, 50))
|
|
A = jnp.array(npr.randn(50, 50), dtype=x.dtype)
|
|
|
|
@jax.jit
|
|
def loss(A, x):
|
|
h = jax.nn.sigmoid(A * x)
|
|
return jnp.sum((h - x)**2)
|
|
|
|
with jax.checking_leaks():
|
|
_ = jax.grad(loss)(A, x) # doesn't crash
|
|
|
|
def test_vmap_caching(self):
|
|
# https://github.com/jax-ml/jax/issues/7621
|
|
|
|
f = lambda x: jnp.square(x).mean()
|
|
jf = jax.jit(f)
|
|
x = jax.random.uniform(jax.random.key(0), shape=(8, 4))
|
|
|
|
with jtu.count_jit_and_pmap_lowerings() as count: # noqa: F841
|
|
for _ in range(5):
|
|
jax.hessian(jf)(x).block_until_ready()
|
|
|
|
n = count()
|
|
# The exact number of compilations may vary depending on the number of
|
|
# jit decorators in the function above, but it should not grow after an
|
|
# initial warmup phase.
|
|
for _ in range(5):
|
|
jax.hessian(jf)(x).block_until_ready()
|
|
|
|
self.assertEqual(count(), n)
|
|
|
|
def test_jnp_array_doesnt_device_put(self):
|
|
with jtu.count_device_put() as count:
|
|
api.make_jaxpr(lambda: jnp.array(3))()
|
|
self.assertEqual(count(), 0)
|
|
|
|
@jtu.thread_unsafe_test() # Updating global configs is not thread-safe.
|
|
def test_rank_promotion_forces_retrace(self):
|
|
num_traces = 0
|
|
|
|
def g(x):
|
|
nonlocal num_traces
|
|
num_traces += 1
|
|
return x + x
|
|
def f_cond(x):
|
|
return lax.cond(True, g, g, x)
|
|
|
|
@jax.jit
|
|
def f_jit(x):
|
|
nonlocal num_traces
|
|
num_traces += 1
|
|
return x + x
|
|
|
|
for f in [f_jit, f_cond]:
|
|
# Use _read() to read the flag value rather than threadlocal value.
|
|
allow_promotion = jax.numpy_rank_promotion.get_global()
|
|
try:
|
|
config.update("jax_numpy_rank_promotion", "allow")
|
|
num_traces = 0
|
|
@jax.jit
|
|
def f(x):
|
|
nonlocal num_traces
|
|
num_traces += 1
|
|
return x + x
|
|
x = jnp.zeros((2, 2))
|
|
f(x)
|
|
self.assertEqual(num_traces, 1)
|
|
f(x)
|
|
self.assertEqual(num_traces, 1)
|
|
with jax.numpy_rank_promotion("warn"):
|
|
f(x)
|
|
self.assertEqual(num_traces, 2)
|
|
config.update("jax_numpy_rank_promotion", "raise")
|
|
f(x)
|
|
self.assertGreaterEqual(num_traces, 2)
|
|
nt = num_traces
|
|
f(x)
|
|
self.assertEqual(num_traces, nt)
|
|
f(x)
|
|
self.assertEqual(num_traces, nt)
|
|
finally:
|
|
config.update("jax_numpy_rank_promotion", allow_promotion)
|
|
|
|
def test_grad_negative_argnums(self):
|
|
def f(x, y):
|
|
return x.sum() * y.sum()
|
|
|
|
x = jax.random.normal(jax.random.key(0), (16, 16))
|
|
y = jax.random.normal(jax.random.key(1), (16, 16))
|
|
g = jax.grad(f, argnums=-1)
|
|
g(x, y) # doesn't crash
|
|
|
|
def test_jit_negative_static_argnums(self):
|
|
@partial(jax.jit, static_argnums=-1)
|
|
def g(x, y):
|
|
assert isinstance(y, int)
|
|
return x * y
|
|
for i in range(3): # Loop verifies we exercise both Python and C++ dispatch
|
|
self.assertEqual(2 * i, g(2, i), msg=i)
|
|
|
|
def test_fastpath_cache_confusion(self):
|
|
# https://github.com/jax-ml/jax/issues/12542
|
|
@jax.jit
|
|
def a(x):
|
|
return ()
|
|
|
|
@jax.jit
|
|
def b(x):
|
|
return a(x)
|
|
|
|
@jax.jit
|
|
def g(x):
|
|
return x, x
|
|
|
|
@jax.jit
|
|
def h(x):
|
|
return g(x)
|
|
|
|
jaxpr = jax.make_jaxpr(h)(7)
|
|
core.eval_jaxpr(jaxpr.jaxpr, jaxpr.consts, 7)
|
|
|
|
b(8) # don't crash
|
|
|
|
def test_fastpath_cache_confusion2(self):
|
|
@jax.jit
|
|
def a(): # note nullary function, still staged out though
|
|
return ()
|
|
|
|
@jax.jit
|
|
def b(x):
|
|
return a()
|
|
|
|
@jax.jit
|
|
def g(x):
|
|
return x, x
|
|
|
|
@jax.jit
|
|
def h(x):
|
|
return g(x)
|
|
|
|
jaxpr = jax.make_jaxpr(h)(7)
|
|
core.eval_jaxpr(jaxpr.jaxpr, jaxpr.consts, 7)
|
|
|
|
b(8) # don't crash
|
|
|
|
def test_vjp_multiple_arguments_error_message(self):
|
|
# https://github.com/jax-ml/jax/issues/13099
|
|
def foo(x):
|
|
return (x, x)
|
|
_, f_vjp = jax.vjp(foo, 1.0)
|
|
with self.assertRaisesRegex(TypeError, "applied to foo"):
|
|
f_vjp(1.0, 1.0)
|
|
|
|
def test_shapedtypestruct_sharding_error(self):
|
|
with self.assertRaisesRegex(
|
|
ValueError,
|
|
"sharding should be an instance of `jax.sharding.Sharding`."):
|
|
jax.ShapeDtypeStruct((8, 2), np.float32,
|
|
sharding=jax.sharding.PartitionSpec('x'))
|
|
|
|
def test_make_jaxpr_weakref(self):
|
|
class Foo(NamedTuple):
|
|
x: int
|
|
|
|
def __call__(self, y):
|
|
return self.x + y
|
|
|
|
jax.make_jaxpr(Foo(1))(3) # don't crash
|
|
|
|
def test_make_jaxpr_name(self):
|
|
def foo(x, y, z):
|
|
return x + y + z
|
|
jfoo = jax.make_jaxpr(foo)
|
|
self.assertEqual(jfoo.__name__, f"make_jaxpr({foo.__name__})")
|
|
self.assertEqual(jfoo.__qualname__, f"make_jaxpr({foo.__qualname__})")
|
|
self.assertEqual(jfoo.__module__, "jax")
|
|
|
|
@jtu.thread_unsafe_test() # Concurrent cache eviction means we may retrace
|
|
def test_inner_jit_function_retracing(self):
|
|
# https://github.com/jax-ml/jax/issues/7155
|
|
inner_count = outer_count = 0
|
|
|
|
@jax.jit
|
|
def inner_fn(state):
|
|
nonlocal inner_count
|
|
inner_count += 1
|
|
return 2*state
|
|
|
|
@jax.jit
|
|
def outer_fn(x):
|
|
nonlocal outer_count
|
|
outer_count += 1
|
|
old_x = x
|
|
for _ in range(10):
|
|
x = inner_fn(x)
|
|
x = x + old_x
|
|
return x
|
|
|
|
state = jnp.arange(5, dtype=jnp.uint32)
|
|
inner_fn(state)
|
|
outer_fn(state)
|
|
|
|
self.assertEqual(inner_count, 1)
|
|
self.assertEqual(outer_count, 1)
|
|
|
|
def test_grad_conj_symbolic_zeros(self):
|
|
# https://github.com/jax-ml/jax/issues/15400
|
|
f = lambda x: jax.jit(lambda x, y: (x, y))(x, jax.lax.conj(x))[0]
|
|
out = jax.grad(f)(3.0) # doesn't crash
|
|
self.assertAllClose(out, 1., check_dtypes=False)
|
|
|
|
def test_cache_clear_pmap(self):
|
|
@jax.pmap
|
|
def f(i):
|
|
return i * 2
|
|
|
|
f(np.arange(1, dtype='float32')).block_until_ready()
|
|
self.assertEqual(f._cache_size, 1)
|
|
jax.clear_caches()
|
|
self.assertEqual(f._cache_size, 0)
|
|
|
|
def test_invalid_value_device_put(self):
|
|
with self.assertRaisesRegex(ValueError, r".*Received invalid value.*"):
|
|
jax.device_put(jnp.arange(8), 'cpu')
|
|
|
|
@jtu.thread_unsafe_test() # logging is not thread-safe
|
|
def test_clear_cache(self):
|
|
@jax.jit
|
|
def add(x):
|
|
return x * 2
|
|
|
|
inp = jnp.arange(8)
|
|
|
|
with config.log_compiles(True):
|
|
with self.assertLogs(level='WARNING') as cm:
|
|
add(inp)
|
|
jax.clear_caches()
|
|
add(inp)
|
|
tracing_add_count = 0
|
|
for m in cm.output:
|
|
if 'Finished tracing + transforming add for pjit' in m:
|
|
tracing_add_count += 1
|
|
self.assertEqual(tracing_add_count, 2)
|
|
|
|
@jtu.thread_unsafe_test() # logging is not thread-safe
|
|
def test_cache_miss_explanations(self):
|
|
@jax.jit
|
|
def f(x, y):
|
|
return jnp.sin(x) * y['hi']
|
|
|
|
x = jnp.float32(1.)
|
|
y = {'hi': jnp.arange(3., dtype='float32')}
|
|
|
|
expected_log_len = 1 if not is_persistent_cache_enabled() else 3
|
|
|
|
# print on first miss, not on hit
|
|
with config.explain_cache_misses(True):
|
|
with self.assertLogs(level='WARNING') as cm:
|
|
f(x, y)
|
|
f(x, y)
|
|
self.assertLen(cm.output, expected_log_len)
|
|
msg = cm.output[0]
|
|
self.assertIn('TRACING CACHE MISS', msg)
|
|
self.assertIn('never seen function', msg)
|
|
|
|
# shape change
|
|
y_ = {'hi': jnp.arange(4, dtype='float32')}
|
|
with config.explain_cache_misses(True):
|
|
with self.assertLogs(level='WARNING') as cm:
|
|
f(x, y_)
|
|
self.assertLen(cm.output, expected_log_len)
|
|
msg = cm.output[0]
|
|
self.assertIn('never seen input type signature', msg)
|
|
self.assertIn('closest seen input type signature has 1 mismatches', msg)
|
|
self.assertIn('seen f32[3], but now given f32[4]', msg)
|
|
|
|
# weak type change (assuming no x64)
|
|
if not config.enable_x64.value:
|
|
with config.explain_cache_misses(True):
|
|
with self.assertLogs(level='WARNING') as cm:
|
|
f(1., y)
|
|
self.assertLen(cm.output, expected_log_len)
|
|
msg = cm.output[0]
|
|
self.assertIn('weak_type=True', msg)
|
|
self.assertIn('https://jax.readthedocs.io/en/latest/type_promotion.html#weak-types', msg)
|
|
|
|
# kwarg change
|
|
with config.explain_cache_misses(True):
|
|
with self.assertLogs(level='WARNING') as cm:
|
|
f(1, y=y)
|
|
self.assertLen(cm.output, expected_log_len)
|
|
msg = cm.output[0]
|
|
self.assertIn('never seen passing 1 positional args and 1 keyword args', msg)
|
|
|
|
# tracing config change
|
|
with config.explain_cache_misses(True):
|
|
with self.assertLogs(level='WARNING') as cm:
|
|
with jax.numpy_rank_promotion('warn'):
|
|
f(x, y)
|
|
# depending on the backend, we may or may not get persistent cache warnings
|
|
self.assertTrue(1 <= len(cm.output) <= expected_log_len)
|
|
msg = cm.output[0]
|
|
self.assertIn("tracing context doesn't match", msg)
|
|
|
|
@jtu.thread_unsafe_test() # logging is not thread-safe
|
|
def test_cache_miss_explanations_new_function_in_loop(self):
|
|
@jax.jit
|
|
def f(x, y):
|
|
return jnp.sin(x) * y['hi']
|
|
|
|
x = jnp.float32(1.)
|
|
|
|
with config.explain_cache_misses(True):
|
|
with self.assertLogs(level='WARNING') as cm:
|
|
for _ in range(2):
|
|
jax.jit(lambda x: 2 * x)(3)
|
|
if is_persistent_cache_enabled():
|
|
# number of warnings depends on the backend
|
|
self.assertTrue(4 <= len(cm.output) <= 6)
|
|
msg = cm.output[3]
|
|
self.assertIn('another function defined on the same line', msg)
|
|
else:
|
|
self.assertLen(cm.output, 2)
|
|
_, msg = cm.output
|
|
self.assertIn('another function defined on the same line', msg)
|
|
|
|
@jtu.thread_unsafe_test() # logging is not thread-safe
|
|
def test_cache_miss_explanations_unpacks_transforms(self):
|
|
# Tests that the explain_tracing_cache_miss() function does not throw an
|
|
# error when unpacking `transforms` with a length greater than 3.
|
|
@jax.jit
|
|
def f(key):
|
|
return jax.random.truncated_normal(key, 1, 1, dtype=jax.numpy.float32)
|
|
|
|
with config.explain_cache_misses(True):
|
|
with self.assertLogs(level="WARNING") as cm:
|
|
f(jax.random.key(seed=123))
|
|
|
|
if is_persistent_cache_enabled():
|
|
# 5 warnings from tracing cache, 5-10 from persistent cache depending on
|
|
# the backend
|
|
self.assertTrue(10 <= len(cm.output) <= 15)
|
|
self.assertTrue(any("TRACING CACHE MISS" in msg for msg in cm.output))
|
|
else:
|
|
self.assertLen(cm.output, 5)
|
|
for msg in cm.output:
|
|
self.assertIn("TRACING CACHE MISS", msg)
|
|
|
|
def test_cache_miss_explanations_no_source_info(self):
|
|
# ``operator.add`` is a built-in function and does not have source info.
|
|
with config.explain_cache_misses(True):
|
|
jax.jit(operator.add)(42, 24)
|
|
|
|
@parameterized.named_parameters([
|
|
{"testcase_name": f"{np.dtype(dtype)}", "dtype": dtype}
|
|
for dtype in jtu.dtypes.custom_floats])
|
|
def test_jit_custom_floats(self, dtype):
|
|
f = lambda x: x + 1
|
|
args_maker = lambda: [jnp.ones((), dtype=dtype)]
|
|
self._CompileAndCheck(f, args_maker)
|
|
|
|
def test_jvp_asarray_returns_array(self):
|
|
# https://github.com/jax-ml/jax/issues/15676
|
|
p, t = jax.jvp(jax.numpy.asarray, (1.,), (2.,))
|
|
_check_instance(self, p)
|
|
_check_instance(self, t)
|
|
|
|
def test_scalar_conversion_errors(self):
|
|
array_int = jnp.arange(10, dtype=int)
|
|
scalar_float = jnp.float32(0)
|
|
scalar_int = jnp.int32(0)
|
|
empty_int = jnp.arange(0, dtype='int32')
|
|
array1_float = jnp.arange(1, dtype='float32')
|
|
|
|
assertIntError = partial(self.assertRaisesRegex, TypeError,
|
|
"Only integer scalar arrays can be converted to a scalar index.")
|
|
for func in [operator.index, hex, oct]:
|
|
assertIntError(func, array_int)
|
|
assertIntError(func, empty_int)
|
|
assertIntError(func, scalar_float)
|
|
assertIntError(jax.jit(func), array_int)
|
|
assertIntError(jax.jit(func), empty_int)
|
|
assertIntError(jax.jit(func), scalar_float)
|
|
self.assertRaises(TracerIntegerConversionError, jax.jit(func), scalar_int)
|
|
_ = func(scalar_int) # no error
|
|
|
|
assertScalarError = partial(self.assertRaisesRegex, TypeError,
|
|
"Only scalar arrays can be converted to Python scalars.")
|
|
for func in [int, float, complex]:
|
|
assertScalarError(func, array_int)
|
|
assertScalarError(jax.jit(func), array_int)
|
|
self.assertRaises(ConcretizationTypeError, jax.jit(func), scalar_int)
|
|
_ = func(scalar_int) # no error
|
|
assertScalarError(func, array1_float)
|
|
|
|
assertEmptyBoolError = partial(
|
|
self.assertRaisesRegex, ValueError,
|
|
"The truth value of an empty array is ambiguous.")
|
|
assertEmptyBoolError(bool, empty_int)
|
|
assertEmptyBoolError(jax.jit(bool), empty_int)
|
|
|
|
assertBoolError = partial(
|
|
self.assertRaisesRegex, ValueError,
|
|
"The truth value of an array with more than one element is ambiguous.")
|
|
assertBoolError(bool, array_int)
|
|
assertBoolError(jax.jit(bool), array_int)
|
|
self.assertRaises(TracerBoolConversionError, jax.jit(bool), scalar_int)
|
|
_ = bool(scalar_int) # no error
|
|
|
|
@jtu.run_on_devices('cpu')
|
|
def test_asarray_no_copy_np(self):
|
|
x = np.random.uniform(0, 1, (1000, 2000)).astype("float32")
|
|
out = jnp.asarray(x)
|
|
|
|
x_ptr = x.__array_interface__["data"][0]
|
|
# This is because the PJRT CPU client shares memory if it is 16-byte aligned.
|
|
if (x_ptr & 15) != 0:
|
|
self.assertTrue(np.shares_memory(out, x))
|
|
|
|
def test_mesh_creation_error_message(self):
|
|
with self.assertRaisesRegex(ValueError, "ndim of its first argument"):
|
|
jax.sharding.Mesh(jax.devices(), ("x", "y"))
|
|
|
|
@jtu.thread_unsafe_test() # weakref gc doesn't seem predictable
|
|
def test_jit_boundmethod_reference_cycle(self):
|
|
class A:
|
|
def __init__(self):
|
|
self._foo = jax.jit(self.foo)
|
|
def foo(self):
|
|
pass
|
|
a = weakref.ref(A())
|
|
gc.collect()
|
|
assert a() is None
|
|
|
|
def test_forwarding_bug(self):
|
|
# Test for issue #20267.
|
|
def f(x):
|
|
|
|
@jax.jit
|
|
def inner(a, x):
|
|
return a, jnp.exp(x)
|
|
|
|
return inner(0.0, x)[0]
|
|
jax.grad(f)(1.) # don't crash
|
|
|
|
@parameterized.parameters(it.product(range(4), repeat=3))
|
|
@jtu.run_on_devices("cpu")
|
|
def test_jit_forwarding_correctness(self, seed, num_input_fwd, num_output_fwd):
|
|
num_args = 3
|
|
rng = np.random.RandomState(seed)
|
|
in_perm = rng.permutation(num_args)
|
|
out_perm = rng.permutation(num_args)
|
|
|
|
@jax.jit
|
|
def f(inputs):
|
|
inputs = [inputs[i] for i in in_perm]
|
|
outputs = inputs[:num_input_fwd] + [
|
|
jnp.exp(inputs[i]) if i < num_output_fwd else jnp.sin(inputs[i])
|
|
for i in range(num_args - num_input_fwd)]
|
|
return [outputs[i] for i in out_perm]
|
|
|
|
jtu.check_grads(f, (list(jnp.arange(float(num_args))),), order=1,
|
|
modes=['rev'], atol=1e-3, rtol=1e-3)
|
|
|
|
@jtu.run_on_devices("cpu")
|
|
def test_inner_jit_forwarding_happens(self):
|
|
jaxpr = jax.make_jaxpr(lambda: jax.jit(lambda x: x)(3))()
|
|
self.assertLen(jaxpr.jaxpr.outvars, 1)
|
|
self.assertIsInstance(jaxpr.jaxpr.outvars[0], core.Literal)
|
|
self.assertEqual(jaxpr.jaxpr.outvars[0].val, 3)
|
|
|
|
@parameterized.parameters(range(8))
|
|
@jtu.run_on_devices("cpu")
|
|
def test_inner_jit_forwarding_correctness(self, num_input_fwd):
|
|
num_args = 8
|
|
rng = np.random.RandomState(0)
|
|
|
|
@jax.jit
|
|
def f(inputs):
|
|
inputs = [inputs[i] for i in rng.permutation(num_args)]
|
|
outputs = (inputs[:num_input_fwd] +
|
|
[jnp.sin(inputs[i]) for i in range(num_args - num_input_fwd)])
|
|
return [outputs[i] for i in rng.permutation(num_args)]
|
|
|
|
f2 = jax.jit(f)
|
|
inputs = list(jnp.arange(float(num_args)))
|
|
expected = f(inputs)
|
|
ans = f2(inputs)
|
|
for a, b in zip(ans, expected):
|
|
self.assertAllClose(a, b)
|
|
|
|
@unittest.skip # TODO(dougalm): figure out with Matt what to do with this feature
|
|
def test_inner_jit_forwarded_consts_stay_const(self):
|
|
out = jax.jit(lambda: int(jax.jit(lambda x: x)(3)))() # don't crash
|
|
self.assertEqual(out, 3)
|
|
|
|
def test_lowering_platform_aot(self):
|
|
@jax.jit
|
|
def f(x):
|
|
return x * 2
|
|
|
|
f.trace(jnp.arange(8)).lower(lowering_platforms=('tpu',)) # doesn't crash
|
|
|
|
def test_no_double_dots_in_error_message(self):
|
|
@jax.jit
|
|
def f(x):
|
|
return 1 if x > 0 else 0
|
|
|
|
with self.assertRaisesRegex(TracerBoolConversionError, r"with shape bool\[\]\.[^\.]"):
|
|
f(0)
|
|
|
|
def test_inlined_literals_with_error(self):
|
|
@jax.jit
|
|
def f():
|
|
@partial(jax.jit, inline=True)
|
|
def g():
|
|
return jnp.sin(1.)
|
|
if g() > 0:
|
|
return 1.
|
|
return 0.
|
|
|
|
with self.assertRaisesRegex(TracerBoolConversionError, "Attempted boolean"):
|
|
f()
|
|
|
|
def test_inline_return_twice(self):
|
|
# https://github.com/jax-ml/jax/issues/22944
|
|
@jax.jit
|
|
def add_one(x: int) -> int:
|
|
return x + 1
|
|
|
|
def add_one_and_dupe(x: int) -> tuple[int, int]:
|
|
y = add_one(x)
|
|
return (y, y)
|
|
|
|
jit_add_one_dupe = jax.jit(add_one_and_dupe, inline=True)
|
|
jax.eval_shape(jit_add_one_dupe, 0) # don't crash
|
|
|
|
def test_use_direct_linearize(self):
|
|
|
|
def check_invariant_to_use_direct_linearize(f):
|
|
with config.use_direct_linearize(False):
|
|
ans1 = f()
|
|
with config.use_direct_linearize(True):
|
|
ans2 = f()
|
|
|
|
self.assertEqual(ans1, ans2)
|
|
|
|
def sin_of_sin(x):
|
|
return lax.sin(jax.jit(lax.sin)(x))
|
|
|
|
check_invariant_to_use_direct_linearize(lambda: jax.grad(sin_of_sin)(1.0))
|
|
|
|
|
|
class RematTest(jtu.JaxTestCase):
|
|
|
|
@parameterized.named_parameters(
|
|
{"testcase_name": f"{suffix}", "remat": remat}
|
|
for suffix, remat in [
|
|
('', jax.remat),
|
|
('_policy', partial(jax.remat, policy=lambda *_, **__: False)),
|
|
('_new', partial(new_checkpoint, policy=lambda *_, **__: False)),
|
|
])
|
|
@jtu.thread_unsafe_test() # monkey patches sin_p and cos_p
|
|
def test_remat_basic(self, remat):
|
|
@remat
|
|
def g(x):
|
|
return lax.sin(lax.sin(x)), 3.
|
|
|
|
def f(x):
|
|
x, _ = g(x)
|
|
return x
|
|
|
|
ans = f(2.)
|
|
expected = np.sin(np.sin(2.))
|
|
self.assertAllClose(ans, expected, check_dtypes=False)
|
|
|
|
ans, f_lin = api.linearize(f, 2.)
|
|
expected = np.sin(np.sin(2.))
|
|
self.assertAllClose(ans, expected, check_dtypes=False)
|
|
|
|
ans = f_lin(3.)
|
|
expected = np.cos(np.sin(2.)) * np.cos(2.) * 3.
|
|
self.assertAllClose(ans, expected, check_dtypes=False)
|
|
|
|
sin_calls = []
|
|
cos_calls = []
|
|
sin_impl = lax.sin_p.impl
|
|
cos_impl = lax.cos_p.impl
|
|
try:
|
|
lax.sin_p.def_impl(lambda x: sin_calls.append(1) or sin_impl(x))
|
|
lax.cos_p.def_impl(lambda x: cos_calls.append(1) or cos_impl(x))
|
|
f_lin(3.)
|
|
finally:
|
|
lax.sin_p.def_impl(sin_impl)
|
|
lax.cos_p.def_impl(cos_impl)
|
|
self.assertEqual(len(sin_calls), 1)
|
|
self.assertEqual(len(cos_calls), 2)
|
|
|
|
@parameterized.named_parameters(
|
|
{"testcase_name": f"{suffix}", "remat": remat}
|
|
for suffix, remat in [
|
|
('', jax.remat),
|
|
('_policy', partial(jax.remat, policy=lambda *_, **__: False)),
|
|
('_new', partial(new_checkpoint, policy=lambda *_, **__: False)),
|
|
])
|
|
def test_remat_freevars(self, remat):
|
|
def f1(x):
|
|
y = 2 * jnp.sin(x)
|
|
z = jnp.cos(x) * jnp.sin(y)
|
|
return z
|
|
|
|
def f2(x):
|
|
y = 2 * jnp.sin(x)
|
|
z = remat(lambda x: jnp.cos(x) * jnp.sin(y))(x)
|
|
return z
|
|
|
|
ans, f_lin = api.linearize(f2, 2.)
|
|
expected, f_lin_expected = api.linearize(f1, 2.)
|
|
self.assertAllClose(ans, expected, check_dtypes=False)
|
|
|
|
ans = f_lin(3.)
|
|
expected = f_lin_expected(3.)
|
|
self.assertAllClose(ans, expected, check_dtypes=False)
|
|
|
|
@unittest.skip
|
|
def test_remat_grad_python_control_flow_static_argnums(self):
|
|
@partial(jax.remat, static_argnums=(0,))
|
|
def g(x):
|
|
with jax.ensure_compile_time_eval():
|
|
x_pos = x > 0
|
|
if x_pos:
|
|
return lax.sin(x), 3.
|
|
else:
|
|
return lax.cos(x), 4.
|
|
|
|
def f(x):
|
|
x, _ = g(x)
|
|
return x
|
|
|
|
ans = f(2.)
|
|
expected = np.sin(2.)
|
|
self.assertAllClose(ans, expected, check_dtypes=False)
|
|
|
|
ans = api.grad(f)(2.)
|
|
expected = np.cos(2.)
|
|
self.assertAllClose(ans, expected, check_dtypes=False)
|
|
|
|
@unittest.skip
|
|
def test_remat_grad_python_control_flow_unhashable_static_argnums(self):
|
|
@partial(jax.remat, static_argnums=(0,))
|
|
def g(x):
|
|
x = x.val
|
|
with jax.ensure_compile_time_eval():
|
|
x_pos = x > 0
|
|
if x_pos:
|
|
return lax.sin(x), 3.
|
|
else:
|
|
return lax.cos(x), 4.
|
|
|
|
def f(x):
|
|
x, _ = g(x)
|
|
return x
|
|
|
|
class A:
|
|
def __init__(self, val):
|
|
self.val = val
|
|
def __hash__(self):
|
|
raise TypeError
|
|
|
|
ans = f(A(2.))
|
|
expected = np.sin(2.)
|
|
self.assertAllClose(ans, expected, check_dtypes=False)
|
|
|
|
ans = api.grad(lambda x: f(A(x)))(2.)
|
|
expected = np.cos(2.)
|
|
self.assertAllClose(ans, expected, check_dtypes=False)
|
|
|
|
def test_remat_retracing(self):
|
|
# This is *not* a very important behavior; remat doesn't need to provide
|
|
# caching guarantees with the same importance as jit. But even so, in the
|
|
# interest of not redoing tracing work (and thus make jax.remat more
|
|
# feasible to use in eager mode), this test checks that we don't re-trace
|
|
# the remat-decorated function.
|
|
count = 0
|
|
|
|
@jax.remat
|
|
def g(x):
|
|
nonlocal count
|
|
count += 1
|
|
return lax.sin(x), 3.
|
|
|
|
def f(x):
|
|
x, _ = g(x)
|
|
return x
|
|
|
|
for _ in range(10):
|
|
y = f(2.)
|
|
y.block_until_ready()
|
|
self.assertEqual(count, 1)
|
|
|
|
def test_remat_static_agnums_retracing(self):
|
|
# This is *not* a super important behavior; remat doesn't need to provide
|
|
# caching guarantees with the same importance as jit. But even so, in the
|
|
# interest of not redoing tracing work (and thus make jax.remat more
|
|
# feasible to use in eager mode), this test checks that we don't re-trace
|
|
# the remat-decorated function *even with static_argnums*. See also the
|
|
# above test, which doesn't check for static_argnums.
|
|
count = 0
|
|
|
|
@partial(jax.remat, static_argnums=(0,))
|
|
def g(x):
|
|
nonlocal count
|
|
count += 1
|
|
with jax.ensure_compile_time_eval():
|
|
x_pos = x > 0
|
|
if x_pos:
|
|
return lax.sin(x), 3.
|
|
else:
|
|
return lax.cos(x), 4.
|
|
|
|
def f(x):
|
|
x, _ = g(x)
|
|
return x
|
|
|
|
for _ in range(10):
|
|
y = f(2.)
|
|
y.block_until_ready()
|
|
self.assertEqual(count, 1)
|
|
|
|
@parameterized.named_parameters(
|
|
{"testcase_name": f"{suffix}", "remat": remat}
|
|
for suffix, remat in [
|
|
('', jax.remat),
|
|
('_policy', partial(jax.remat, policy=lambda *_, **__: False)),
|
|
('_new', partial(new_checkpoint, policy=lambda *_, **__: False)),
|
|
])
|
|
def test_remat_jit(self, remat):
|
|
@remat
|
|
def g(x):
|
|
return lax.sin(lax.sin(x))
|
|
|
|
def f_(x):
|
|
return g(x)
|
|
f = api.jit(f_)
|
|
|
|
ans = f(2.)
|
|
expected = np.sin(np.sin(2.))
|
|
self.assertAllClose(ans, expected, check_dtypes=False)
|
|
|
|
ans = api.grad(f)(2.)
|
|
expected = np.cos(np.sin(2.)) * np.cos(2.)
|
|
self.assertAllClose(ans, expected, check_dtypes=False)
|
|
|
|
ans = api.jit(api.grad(f_))(2.)
|
|
expected = np.cos(np.sin(2.)) * np.cos(2.)
|
|
self.assertAllClose(ans, expected, check_dtypes=False)
|
|
|
|
@parameterized.named_parameters(
|
|
{"testcase_name": f"{suffix}", "remat": remat}
|
|
for suffix, remat in [
|
|
('', jax.remat),
|
|
('_policy', partial(jax.remat, policy=lambda *_, **__: False)),
|
|
('_new', partial(new_checkpoint, policy=lambda *_, **__: False)),
|
|
])
|
|
def test_remat_vmap(self, remat):
|
|
@remat
|
|
def g(x):
|
|
return lax.sin(lax.sin(x))
|
|
|
|
x = np.arange(3.)
|
|
|
|
ans = api.vmap(g)(x)
|
|
expected = np.sin(np.sin(x))
|
|
self.assertAllClose(ans, expected, check_dtypes=False)
|
|
|
|
ans = api.jacfwd(g)(x)
|
|
expected = np.diag(np.cos(np.sin(x)) * np.cos(x))
|
|
self.assertAllClose(ans, expected, check_dtypes=False)
|
|
|
|
ans = api.jacrev(g)(x)
|
|
expected = np.diag(np.cos(np.sin(x)) * np.cos(x))
|
|
self.assertAllClose(ans, expected, check_dtypes=False)
|
|
|
|
# Make sure that introducing constants in vmap works.
|
|
constant_introducing_p = core.Primitive('introduce_constant')
|
|
constant_introducing_p.def_abstract_eval(core.raise_to_shaped)
|
|
def _constant_introducing_batcher(xs, ds):
|
|
(x,), (d,) = xs, ds
|
|
return (x + np.arange(x.size, dtype=x.dtype).reshape(x.shape)), d
|
|
batching.primitive_batchers[constant_introducing_p] = _constant_introducing_batcher
|
|
|
|
api.vmap(remat(constant_introducing_p.bind))(jnp.ones(20))
|
|
|
|
@parameterized.named_parameters(
|
|
{"testcase_name": f"{suffix}", "remat": remat}
|
|
for suffix, remat in [
|
|
('', jax.remat),
|
|
('_policy', partial(jax.remat, policy=lambda *_, **__: False)),
|
|
('_new', partial(new_checkpoint, policy=lambda *_, **__: False)),
|
|
])
|
|
def test_remat_vmap_not_leading_dim(self, remat):
|
|
@remat
|
|
def g(x):
|
|
return lax.sin(lax.sin(x))
|
|
|
|
x = np.arange(3 * 5.).reshape(3, 5)
|
|
|
|
ans = api.vmap(g, 1, 0)(x)
|
|
expected = np.sin(np.sin(x)).T
|
|
self.assertAllClose(ans, expected, check_dtypes=False)
|
|
|
|
@parameterized.named_parameters(
|
|
{"testcase_name": f"{suffix}", "remat": remat}
|
|
for suffix, remat in [
|
|
('', jax.remat),
|
|
('_policy', partial(jax.remat, policy=lambda *_, **__: False)),
|
|
('_new', partial(new_checkpoint, policy=lambda *_, **__: False)),
|
|
])
|
|
def test_remat_higher_order_autodiff(self, remat):
|
|
def f(x):
|
|
return lax.cos(lax.sin(x))
|
|
g = remat(f)
|
|
|
|
ans = api.grad(api.grad(g))(3.)
|
|
expected = api.grad(api.grad(f))(3.)
|
|
self.assertAllClose(ans, expected, check_dtypes=False)
|
|
|
|
@parameterized.named_parameters(
|
|
{"testcase_name": f"{suffix}", "remat": remat}
|
|
for suffix, remat in [
|
|
('', jax.remat),
|
|
('_new', new_checkpoint),
|
|
])
|
|
def test_remat_scan(self, remat):
|
|
to_scan = lambda c, x: (jnp.sin(c), None)
|
|
|
|
def f_noremat(x):
|
|
y, _ = lax.scan(to_scan, x, np.arange(3.))
|
|
return y
|
|
|
|
def f_yesremat(x):
|
|
y, _ = lax.scan(remat(to_scan), x, np.arange(3.))
|
|
return y
|
|
|
|
ans = f_yesremat(4.)
|
|
expected = f_noremat(4.)
|
|
self.assertAllClose(ans, expected, check_dtypes=False)
|
|
|
|
ans = api.grad(f_yesremat)(4.)
|
|
expected = api.grad(f_noremat)(4.)
|
|
self.assertAllClose(ans, expected, check_dtypes=False)
|
|
|
|
jaxpr = api.make_jaxpr(api.linearize(f_yesremat, 4.)[1])(1.)
|
|
scan_eqn, = jaxpr.jaxpr.eqns
|
|
self.assertIn(' cos ', str(scan_eqn.params['jaxpr']))
|
|
|
|
jaxpr = api.make_jaxpr(api.vjp(f_yesremat, 4.)[1])(1.)
|
|
scan_eqn, = jaxpr.jaxpr.eqns
|
|
self.assertIn(' cos ', str(scan_eqn.params['jaxpr']))
|
|
|
|
@parameterized.named_parameters(
|
|
{"testcase_name": f"{suffix}", "remat": remat}
|
|
for suffix, remat in [
|
|
('', jax.remat),
|
|
('_policy', partial(jax.remat, policy=lambda *_, **__: False)),
|
|
('_new', partial(new_checkpoint, policy=lambda *_, **__: False)),
|
|
])
|
|
@jtu.thread_unsafe_test() # monkey patches sin_p
|
|
def test_remat_no_redundant_flops(self, remat):
|
|
# see https://github.com/jax-ml/jax/pull/1749#issuecomment-558267584
|
|
|
|
@api.jit
|
|
def g(x):
|
|
return f(2., x)
|
|
|
|
@remat
|
|
def f(x, y):
|
|
return jnp.sin(x) * y
|
|
|
|
# We swap out sin_p's impl rule to count how many times it's invoked
|
|
called = []
|
|
sin_impl = lax.sin_p.impl
|
|
try:
|
|
lax.sin_p.def_impl(lambda x: called.append(1) or sin_impl(x))
|
|
api.grad(g)(3.)
|
|
finally:
|
|
lax.sin_p.def_impl(sin_impl)
|
|
num_calls = len(called)
|
|
self.assertLessEqual(num_calls, 1)
|
|
|
|
@parameterized.named_parameters(
|
|
{"testcase_name": f"{suffix}", "remat": remat}
|
|
for suffix, remat in [
|
|
('', jax.remat),
|
|
('_policy', partial(jax.remat, policy=lambda *_, **__: False)),
|
|
('_new', partial(new_checkpoint, policy=lambda *_, **__: False)),
|
|
])
|
|
def test_remat_binomial_checkpointing(self, remat):
|
|
def binom_checkpoint(funs):
|
|
if len(funs) == 1:
|
|
return funs[0]
|
|
else:
|
|
f1 = binom_checkpoint(funs[:len(funs)//2])
|
|
f2 = binom_checkpoint(funs[len(funs)//2:])
|
|
return remat(lambda x: f1(f2(x)))
|
|
|
|
f1 = binom_checkpoint([jnp.sin, jnp.sin, jnp.sin, jnp.sin])
|
|
f2 = lambda x: jnp.sin(jnp.sin(jnp.sin(jnp.sin(x))))
|
|
x = 4.
|
|
self.assertAllClose(f1(x), f2(x), check_dtypes=False)
|
|
self.assertAllClose(api.grad(f1)(x), api.grad(f2)(x), check_dtypes=False)
|
|
|
|
@parameterized.named_parameters(
|
|
{"testcase_name": f"{suffix}", "remat": remat}
|
|
for suffix, remat in [
|
|
('', jax.remat),
|
|
('_new', new_checkpoint),
|
|
])
|
|
def test_remat_symbolic_zeros(self, remat):
|
|
# code from https://github.com/jax-ml/jax/issues/1907
|
|
|
|
key = jax.random.key(0)
|
|
key, split = jax.random.split(key)
|
|
n = 5
|
|
|
|
def func(D0):
|
|
def shift(R, dR, **unused_kwargs):
|
|
return R + dR
|
|
|
|
def apply_fn(R):
|
|
return D0 * R
|
|
|
|
Rinit = jax.random.uniform(split, (n,3), minval=0.0, maxval=5.0,
|
|
dtype=jnp.float32)
|
|
|
|
def move(R,i):
|
|
F = apply_fn(R)
|
|
return shift(R, 0.001 * F), jnp.array([0.])
|
|
|
|
move = remat(move)
|
|
R, temp = lax.scan(move, Rinit, jnp.arange(2))
|
|
return R[0, 0]
|
|
|
|
api.grad(func)(5.0) # doesn't crash
|
|
|
|
@parameterized.named_parameters(
|
|
{"testcase_name": f"{suffix}", "remat": remat}
|
|
for suffix, remat in [
|
|
('', jax.remat),
|
|
('_policy', partial(jax.remat, policy=lambda *_, **__: False)),
|
|
('_new', partial(new_checkpoint, policy=lambda *_, **__: False)),
|
|
])
|
|
def test_remat_jit2(self, remat):
|
|
@api.jit
|
|
def f(x):
|
|
y = 2 * x
|
|
|
|
@remat
|
|
def g():
|
|
return y
|
|
|
|
return g()
|
|
|
|
self.assertAllClose(f(3), 6, check_dtypes=False)
|
|
|
|
@parameterized.named_parameters(
|
|
{"testcase_name": f"{suffix}", "remat": remat}
|
|
for suffix, remat in [
|
|
('', jax.remat),
|
|
('_new', new_checkpoint),
|
|
])
|
|
def test_remat_nontrivial_env(self, remat):
|
|
# simplified from https://github.com/jax-ml/jax/issues/2030
|
|
|
|
@remat
|
|
def foo(state, dt=0.5, c=1):
|
|
u, u_t = state
|
|
u_tt = c**2 * u
|
|
u_t = u_t + u_tt * dt
|
|
return (u, u_t)
|
|
|
|
@partial(api.jit, static_argnums=(1,))
|
|
def _multi_step(state, count, dt, c):
|
|
f = lambda s, _: (foo(s, dt, c), _)
|
|
return lax.scan(f, state, None, count)
|
|
|
|
def multi_step(state, count, dt=1/jnp.sqrt(2), c=1):
|
|
return _multi_step(state, count, dt, c)
|
|
|
|
def loss(u0, target, steps, dt=1/jnp.sqrt(2), c=1):
|
|
init = (u0, jnp.zeros_like(u0))
|
|
(uf, _), _ = multi_step(init, steps, dt, c)
|
|
return ((uf - target) ** 2).mean()
|
|
|
|
target = jnp.zeros((128, 128))
|
|
u0 = jnp.ones_like(target)
|
|
loss(u0, target, 10) # doesn't crash
|
|
|
|
@parameterized.named_parameters(
|
|
{"testcase_name": f"{suffix}", "remat": remat}
|
|
for suffix, remat in [
|
|
('', jax.remat),
|
|
('_policy', partial(jax.remat, policy=lambda *_, **__: False)),
|
|
('_new', partial(new_checkpoint, policy=lambda *_, **__: False)),
|
|
])
|
|
def test_remat_jit3(self, remat):
|
|
# https://github.com/jax-ml/jax/issues/2180
|
|
def f(w, x):
|
|
a = jnp.dot(x, w)
|
|
b = jnp.einsum("btd,bTd->btT", a, a)
|
|
c = jnp.einsum("btT,btd->btd", b, a)
|
|
return jnp.sum(c)
|
|
|
|
w = jnp.ones([1, 1])
|
|
x = jnp.ones([1, 1, 1])
|
|
f = remat(f)
|
|
api.grad(f)(w, x) # doesn't crash
|
|
|
|
@api.jit
|
|
def mul(a, b):
|
|
return a * b
|
|
|
|
def f(w, x):
|
|
a = mul(w, x)
|
|
b = mul(a, a)
|
|
return b
|
|
|
|
w = 1.
|
|
x = 1.
|
|
f = remat(f)
|
|
api.grad(f)(w, x) # doesn't crash
|
|
|
|
@parameterized.named_parameters(
|
|
{"testcase_name": f"{suffix}", "remat": remat}
|
|
for suffix, remat in [
|
|
('', jax.remat),
|
|
('_new', new_checkpoint),
|
|
])
|
|
def test_remat_scan2(self, remat):
|
|
# https://github.com/jax-ml/jax/issues/1963
|
|
|
|
def scan_bug(x0):
|
|
f = lambda x, _: (x + 1, None)
|
|
def scanned_f(x, _):
|
|
return lax.scan(f, x, xs=None, length=1)[0], None
|
|
x, _ = remat(scanned_f)(x0, None)
|
|
return x
|
|
|
|
jax.grad(scan_bug)(1.0) # doesn't crash
|
|
|
|
def test_remat_jit_static_argnum_omnistaging(self):
|
|
# https://github.com/jax-ml/jax/issues/2833
|
|
# NOTE(mattjj): after #3370, this test doesn't actually call remat...
|
|
def named_call(f):
|
|
def named_f(*args):
|
|
f_ = lu.wrap_init(lambda: (f(*args),))
|
|
out, = core.call_p.bind(f_)
|
|
return out
|
|
return named_f
|
|
|
|
def f(a_bool, y):
|
|
if a_bool:
|
|
return y + 1
|
|
else:
|
|
return y
|
|
|
|
api.jit(named_call(f), static_argnums=0)(True, 1) # no crash
|
|
|
|
@parameterized.named_parameters(
|
|
{"testcase_name": f"{suffix}", "remat": remat}
|
|
for suffix, remat in [
|
|
('', jax.remat),
|
|
('_policy', partial(jax.remat, policy=lambda *_, **__: False)),
|
|
('_new', partial(new_checkpoint, policy=lambda *_, **__: False)),
|
|
])
|
|
def test_remat_eval_counter(self, remat):
|
|
# https://github.com/jax-ml/jax/issues/2737
|
|
add_one_p = core.Primitive('add_one')
|
|
add_one = add_one_p.bind
|
|
|
|
num_evals = 0
|
|
|
|
@contextmanager
|
|
def assertEvals(n):
|
|
start = num_evals
|
|
yield
|
|
assert num_evals - start == n
|
|
|
|
def add_one_impl(x):
|
|
nonlocal num_evals
|
|
num_evals += 1
|
|
return x + 1
|
|
add_one_p.def_impl(add_one_impl)
|
|
|
|
def add_one_jvp(pin, tin):
|
|
pout = add_one(pin[0])
|
|
return pout, pout * tin[0]
|
|
ad.primitive_jvps[add_one_p] = add_one_jvp
|
|
|
|
add_one_p.def_abstract_eval(lambda x: x)
|
|
|
|
v = np.zeros((1,))
|
|
|
|
f = remat(add_one)
|
|
g = remat(lambda x: add_one(f(x)))
|
|
|
|
# 2 calls needed to evaluate g
|
|
with assertEvals(2):
|
|
_, vjp = jax.vjp(g, v)
|
|
# 2 calls made while transposing g, 1 call made while transposing f
|
|
with assertEvals(3):
|
|
vjp(v)
|
|
|
|
@jax_util.curry
|
|
def call(f, *args):
|
|
return core.call(
|
|
lu.wrap_init(lambda *args: [f(*args)]),
|
|
*args, name='foo')[0]
|
|
|
|
f = call(add_one)
|
|
g = remat(lambda x: add_one(f(x)))
|
|
|
|
# 2 calls needed to evaluate g
|
|
with assertEvals(2):
|
|
_, vjp = jax.vjp(g, v)
|
|
# 2 calls made while transposing g, no reevaluation for transposition of f
|
|
with assertEvals(2):
|
|
vjp(v)
|
|
|
|
@parameterized.named_parameters(
|
|
{"testcase_name": f"{suffix}", "remat": remat}
|
|
for suffix, remat in [
|
|
('', jax.remat),
|
|
('_policy', partial(jax.remat, policy=lambda *_, **__: False)),
|
|
('_new', partial(new_checkpoint, policy=lambda *_, **__: False)),
|
|
])
|
|
def test_escaped_tracer_remat(self, remat):
|
|
# b/169779185
|
|
def f():
|
|
seq = [jnp.zeros([])]
|
|
def g():
|
|
seq[0] += 1 # this is line 7 btw
|
|
return seq[0]
|
|
|
|
remat(g)()
|
|
remat(lambda: g())() # lambda defeats caching
|
|
|
|
with self.assertRaisesRegex(UnexpectedTracerError, "global state"):
|
|
api.jit(f)()
|
|
|
|
@parameterized.named_parameters(
|
|
{"testcase_name": f"{suffix}", "remat": remat}
|
|
for suffix, remat in [
|
|
('', jax.remat),
|
|
('_policy', partial(jax.remat, policy=lambda *_, **__: False)),
|
|
('_new', partial(new_checkpoint, policy=lambda *_, **__: False)),
|
|
])
|
|
def test_no_cse_widget_on_primals(self, remat):
|
|
@remat
|
|
def g(x):
|
|
return lax.sin(lax.sin(x)), 3.
|
|
|
|
def f(x):
|
|
x, _ = g(x)
|
|
return x
|
|
|
|
text = jax.jit(f).lower(2.).as_text('hlo')
|
|
self.assertNotIn('while', text)
|
|
self.assertNotIn('conditional', text)
|
|
self.assertNotIn('opt-barrier', text)
|
|
|
|
text = jax.jit(grad(f)).lower(2.).as_text('hlo')
|
|
self.assertTrue('while' in text or 'conditional' in text
|
|
or 'opt-barrier' in text)
|
|
|
|
@parameterized.named_parameters(
|
|
{"testcase_name": f"{suffix}", "remat": remat}
|
|
for suffix, remat in [
|
|
('', jax.remat),
|
|
('_new', new_checkpoint),
|
|
])
|
|
def test_no_cse_widget_with_prevent_cse_false(self, remat):
|
|
@partial(remat, prevent_cse=False)
|
|
def g(x):
|
|
return lax.sin(lax.sin(x)), 3.
|
|
|
|
def f(x):
|
|
x, _ = g(x)
|
|
return x
|
|
|
|
text = jax.jit(f).lower(2.).as_text('hlo')
|
|
self.assertNotIn('while', text)
|
|
self.assertNotIn('conditional', text)
|
|
|
|
text = jax.jit(grad(f)).lower(2.).as_text('hlo')
|
|
self.assertNotIn('while', text)
|
|
self.assertNotIn('conditional', text)
|
|
|
|
@parameterized.named_parameters(
|
|
{"testcase_name": f"_{policy_name}_{remat_name}", "remat": remat,
|
|
"policy": policy, "in_jaxpr2": in_jaxpr2, "not_in_jaxpr2": not_in_jaxpr2}
|
|
for remat_name, remat in [
|
|
('old_remat', jax.remat),
|
|
('new_remat', new_checkpoint),
|
|
]
|
|
for policy_name, policy, in_jaxpr2, not_in_jaxpr2 in [
|
|
('save_anything', lambda *_, **__: True, [], [' sin ', ' cos ']),
|
|
('save_nothing', lambda *_, **__: False, [' sin ', ' cos '], []),
|
|
('save_sin', lambda p, *_, **__: str(p) == 'sin', [' cos '], [' sin ']),
|
|
])
|
|
def test_remat_custom_policy(self, remat, policy, in_jaxpr2, not_in_jaxpr2):
|
|
for square in [lambda x: x * x, api.jit(lambda x: x * x)]:
|
|
f = remat(lambda x: jnp.sin(square(jnp.sin(x))), policy=policy)
|
|
y, f_lin = api.linearize(f, 1.)
|
|
ydot = f_lin(2.)
|
|
jaxpr_text = str(f_lin.func.args[0])
|
|
for substr in in_jaxpr2:
|
|
self.assertIn(substr, jaxpr_text)
|
|
for substr in not_in_jaxpr2:
|
|
self.assertNotIn(substr, jaxpr_text)
|
|
y_expected, ydot_expected = api.jvp(lambda x: jnp.sin(square(jnp.sin(x))),
|
|
[1.], [2.])
|
|
self.assertAllClose(y, y_expected)
|
|
self.assertAllClose(ydot, ydot_expected)
|
|
jtu.check_grads(f, (3.,), order=2, modes=['fwd', 'rev'])
|
|
|
|
@parameterized.named_parameters(
|
|
{"testcase_name": f"_{remat_name}", "remat": remat}
|
|
for remat_name, remat in [
|
|
('old_remat', jax.remat),
|
|
('new_remat', new_checkpoint),
|
|
])
|
|
def test_remat_custom_policy_save_cos(self, remat):
|
|
save_cos = lambda prim, *_, **__: str(prim) == 'cos'
|
|
f = remat(lambda x: jnp.sin(jnp.sin(x)), # different function
|
|
policy=save_cos)
|
|
_, f_lin = api.linearize(f, 1.)
|
|
jaxpr_text = str(f_lin.func.args[0])
|
|
self.assertNotIn(' sin ', jaxpr_text)
|
|
self.assertNotIn(' cos ', jaxpr_text)
|
|
jtu.check_grads(f, (3.,), order=2, modes=['fwd', 'rev'])
|
|
|
|
@parameterized.named_parameters(
|
|
{"testcase_name": f"_{remat_name}", "remat": remat}
|
|
for remat_name, remat in [
|
|
('old_remat', jax.remat),
|
|
('new_remat', new_checkpoint),
|
|
])
|
|
def test_remat_checkpoint_dots(self, remat):
|
|
@partial(remat, policy=jax.checkpoint_policies.checkpoint_dots)
|
|
def f(x):
|
|
x = jnp.dot(x, x, precision=lax.Precision.HIGHEST)
|
|
x = jnp.sin(x)
|
|
x = jnp.dot(x, x, precision=lax.Precision.HIGHEST)
|
|
x = jnp.sin(x)
|
|
x = jnp.dot(x, x, precision=lax.Precision.HIGHEST)
|
|
x = jnp.sin(x)
|
|
return x
|
|
|
|
_, f_lin = api.linearize(f, jnp.ones((2, 2)))
|
|
jaxpr_text = str(f_lin.func.args[0])
|
|
self.assertEqual(jaxpr_text.count(' sin '), 2)
|
|
self.assertEqual(jaxpr_text.count(' dot_'), 6)
|
|
jtu.check_grads(f, (jnp.ones((2, 2)),), order=2, modes=['fwd', 'rev'])
|
|
|
|
@parameterized.named_parameters(
|
|
{"testcase_name": f"_{remat_name}", "remat": remat}
|
|
for remat_name, remat in [
|
|
('old_remat', jax.remat),
|
|
('new_remat', new_checkpoint),
|
|
])
|
|
def test_remat_checkpoint_dots_with_no_batch_dims(self, remat):
|
|
@partial(remat, policy=jax.checkpoint_policies.checkpoint_dots_with_no_batch_dims)
|
|
def f(x):
|
|
x = jnp.einsum('ij,jk->ik', x, x, precision=lax.Precision.HIGHEST)
|
|
x = jnp.sin(x)
|
|
x = jnp.einsum('ij,jk->ik', x, x, precision=lax.Precision.HIGHEST)
|
|
x = jnp.sin(x)
|
|
x = jnp.einsum('ij,jk->ik', x, x, precision=lax.Precision.HIGHEST)
|
|
x = jnp.sin(x)
|
|
return x
|
|
|
|
_, f_lin = api.linearize(f, jnp.ones((2, 2)))
|
|
jaxpr_text = str(f_lin.func.args[0])
|
|
self.assertEqual(jaxpr_text.count(' sin '), 2)
|
|
self.assertEqual(jaxpr_text.count(' dot_general'), 6)
|
|
jtu.check_grads(f, (jnp.ones((2, 2)),), order=2, modes=['fwd', 'rev'])
|
|
|
|
@parameterized.named_parameters(
|
|
{"testcase_name": f"_{remat_name}", "remat": remat}
|
|
for remat_name, remat in [
|
|
('old_remat', jax.remat),
|
|
('new_remat', new_checkpoint),
|
|
])
|
|
def test_remat_checkpoint_dots_with_no_batch_dims2(self, remat):
|
|
@partial(remat, policy=jax.checkpoint_policies.checkpoint_dots_with_no_batch_dims)
|
|
def f(x):
|
|
x = jnp.einsum('nij,njk->nik', x, x, precision=lax.Precision.HIGHEST)
|
|
x = jnp.sin(x)
|
|
x = jnp.einsum('nij,njk->nik', x, x, precision=lax.Precision.HIGHEST)
|
|
x = jnp.sin(x)
|
|
x = jnp.einsum('nij,njk->nik', x, x, precision=lax.Precision.HIGHEST)
|
|
x = jnp.sin(x)
|
|
return x
|
|
|
|
_, f_lin = api.linearize(f, jnp.ones((3, 2, 2)))
|
|
jaxpr_text = str(f_lin.func.args[0])
|
|
self.assertEqual(jaxpr_text.count(' sin '), 2)
|
|
self.assertEqual(jaxpr_text.count(' dot_general'), 9)
|
|
jtu.check_grads(f, (jnp.ones((3, 2, 2)),), order=2, modes=['fwd', 'rev'])
|
|
|
|
@parameterized.named_parameters(
|
|
{"testcase_name": f"_{remat_name}", "remat": remat}
|
|
for remat_name, remat in [
|
|
('old_remat', jax.remat),
|
|
('new_remat', new_checkpoint),
|
|
])
|
|
def test_remat_checkpoint_dots_jit(self, remat):
|
|
@api.jit
|
|
@partial(remat, policy=jax.checkpoint_policies.checkpoint_dots)
|
|
def f(x):
|
|
x = jnp.dot(x, x, precision=lax.Precision.HIGHEST)
|
|
x = jnp.sin(x * 1e-3)
|
|
x = jnp.dot(x, x, precision=lax.Precision.HIGHEST)
|
|
x = jnp.sin(x * 1e-3)
|
|
x = jnp.dot(x, x, precision=lax.Precision.HIGHEST)
|
|
x = jnp.sin(x * 1e-3)
|
|
return x
|
|
|
|
_, f_lin = api.linearize(f, jnp.ones((2, 2)))
|
|
jaxpr_text = str(f_lin.func.args[0])
|
|
self.assertEqual(jaxpr_text.count(' sin '), 2)
|
|
self.assertEqual(jaxpr_text.count(' dot_'), 6)
|
|
jtu.check_grads(f, (jnp.ones((2, 2)),), order=2, modes=['fwd', 'rev'])
|
|
|
|
def test_remat_checkpoint_dots_inside_scan(self):
|
|
x = jnp.ones((5,))
|
|
|
|
def f(W):
|
|
@partial(jax.remat, policy=jax.checkpoint_policies.checkpoint_dots)
|
|
def f(x):
|
|
x = jnp.sin(jnp.dot(x, W, precision=lax.Precision.HIGHEST))
|
|
x = jnp.sin(jnp.dot(x, W, precision=lax.Precision.HIGHEST))
|
|
x = jnp.sin(jnp.dot(x, W, precision=lax.Precision.HIGHEST))
|
|
return x
|
|
|
|
def body(x, _): return f(x), None
|
|
return lax.scan(body, x, None, length=2)[0]
|
|
|
|
_, f_vjp = api.vjp(f, jnp.ones((5, 5)))
|
|
jaxpr_text = str(f_vjp.args[0].func.args[1])
|
|
|
|
# Two sine calls in the backward pass because while we don't save sines
|
|
# within the (rematted) body function, we can save the scan carry, which
|
|
# effectively saves one sine. Three cosines for the Jacobian coefficients.
|
|
self.assertEqual(jaxpr_text.count(' sin '), 2)
|
|
self.assertEqual(jaxpr_text.count(' cos '), 3)
|
|
# Six calls to dot_general in the backward pass because we save the primal
|
|
# matmuls and only compure the backward pass ones (two for each primal one).
|
|
self.assertEqual(jaxpr_text.count(' dot_'), 6)
|
|
|
|
jtu.check_grads(api.jit(f), (jnp.ones((5, 5)),), order=2,
|
|
modes=['fwd', 'rev'])
|
|
|
|
def test_remat_custom_jvp_policy(self):
|
|
@jax.custom_jvp
|
|
def sin(x):
|
|
return jnp.sin(x)
|
|
def sin_jvp(primals, tangents):
|
|
x, = primals
|
|
g, = tangents
|
|
return sin(x), jnp.cos(x) * g
|
|
sin.defjvp(sin_jvp)
|
|
|
|
@partial(jax.remat, policy=jax.checkpoint_policies.checkpoint_dots)
|
|
def f(x):
|
|
x = jnp.dot(x, x, precision=lax.Precision.HIGHEST)
|
|
x = sin(x * 1e-3)
|
|
x = jnp.dot(x, x, precision=lax.Precision.HIGHEST)
|
|
x = sin(x * 1e-3)
|
|
x = jnp.dot(x, x, precision=lax.Precision.HIGHEST)
|
|
x = sin(x * 1e-3)
|
|
return x
|
|
|
|
jtu.check_grads(f, (3.,), order=2, modes=['fwd', 'rev'])
|
|
|
|
def g(x):
|
|
return lax.scan(lambda x, _: (f(x), None), x, None, length=2)[0]
|
|
jtu.check_grads(g, (3.,), order=2, modes=['fwd', 'rev'])
|
|
|
|
def test_remat_custom_vjp_policy(self):
|
|
@jax.custom_vjp
|
|
def sin(x):
|
|
return jnp.sin(x)
|
|
def sin_fwd(x):
|
|
return sin(x), x
|
|
def sin_bwd(x, y_bar):
|
|
return (jnp.cos(x) * y_bar,)
|
|
sin.defvjp(sin_fwd, sin_bwd)
|
|
|
|
@partial(jax.remat, policy=jax.checkpoint_policies.checkpoint_dots)
|
|
def f(x):
|
|
@partial(api.named_call, name="dot")
|
|
def dot2(y, z):
|
|
return jnp.dot(x, jnp.dot(y, z, precision=lax.Precision.HIGHEST),
|
|
precision=lax.Precision.HIGHEST)
|
|
|
|
x = dot2(x, x)
|
|
x = sin(x * 1e-3)
|
|
x = dot2(x, x)
|
|
x = sin(x * 1e-3)
|
|
x = dot2(x, x)
|
|
x = sin(x * 1e-3)
|
|
return x
|
|
|
|
jtu.check_grads(f, (3.,), order=2, modes=['rev'])
|
|
|
|
def g(x):
|
|
return lax.scan(lambda x, _: (f(x), None), x, None, length=2)[0]
|
|
jtu.check_grads(g, (3.,), order=2, modes=['rev'])
|
|
|
|
@parameterized.named_parameters(
|
|
{"testcase_name": f"_{remat_name}", "remat": remat}
|
|
for remat_name, remat in [
|
|
('old_remat', jax.remat),
|
|
('new_remat', new_checkpoint),
|
|
])
|
|
def test_remat_dropvar_policy(self, remat):
|
|
def f(x):
|
|
return x, x
|
|
|
|
@partial(remat, policy=jax.checkpoint_policies.checkpoint_dots)
|
|
def g(x):
|
|
x = api.grad(lambda x: f(x)[0])(x)
|
|
return x
|
|
|
|
api.grad(g)(3.)
|
|
|
|
def test_remat_custom_jvp_linear_policy(self):
|
|
@jax.custom_jvp
|
|
def sum(x):
|
|
return jnp.sum(x, axis=0)
|
|
@sum.defjvp
|
|
def sum_jvp(primals, tangents):
|
|
(x,), (xdot,) = primals, tangents
|
|
return sum(x), sum(xdot)
|
|
|
|
@partial(jax.remat, policy=jax.checkpoint_policies.checkpoint_dots)
|
|
def f(x):
|
|
return sum(x)
|
|
jtu.check_grads(f, (jnp.ones(3),), order=2, modes=['fwd', 'rev'])
|
|
|
|
def g(x):
|
|
return lax.scan(lambda _, x: (None, f(x)), None, x)[1]
|
|
jtu.check_grads(g, (jnp.ones((2, 3)),), order=2, modes=['fwd', 'rev'])
|
|
|
|
def test_constants_not_hoisted(self):
|
|
# The old implementation of remat worked by data dependence, and so
|
|
# (potentially large) constants would not be rematerialized and could be
|
|
# wastefully instantiated. This test checks that the newer remat
|
|
# implementation avoids that. See https://github.com/jax-ml/jax/pull/8191.
|
|
|
|
# no residuals from constants created inside jnp.einsum
|
|
@partial(new_checkpoint, policy=lambda *_, **__: False)
|
|
def f(x):
|
|
return jnp.einsum('ii->i', x)
|
|
res_avals = saved_residuals(f, jnp.ones((2, 2)))
|
|
self.assertLen(res_avals, 0)
|
|
|
|
# no residuals from jnp.zeros
|
|
@partial(new_checkpoint, policy=lambda *_, **__: False)
|
|
def f(x):
|
|
return jnp.zeros_like(x) * x
|
|
res_avals = saved_residuals(f, jnp.ones((2, 2)))
|
|
self.assertLen(res_avals, 0)
|
|
|
|
# no residuals from jnp.zeros, but input must be saved
|
|
@partial(new_checkpoint, policy=lambda *_, **__: False)
|
|
def f(x):
|
|
return jnp.zeros_like(x) * jnp.sin(x)
|
|
res_avals = saved_residuals(f, jnp.ones((2, 2)))
|
|
self.assertLen(res_avals, 1)
|
|
|
|
def test_name_saveable_input(self):
|
|
@partial(jax.remat, policy=lambda p, *_, **__: 'mul' in str(p))
|
|
def f(x):
|
|
x = checkpoint_name(x * x, 'foo')
|
|
x = x * x
|
|
return x
|
|
|
|
res = saved_residuals(f, 3.)
|
|
self.assertStartsWith(res[1][1], "named 'foo'")
|
|
|
|
def test_name_denylist(self):
|
|
def f(x):
|
|
y = checkpoint_name(jnp.multiply(2., 2.), 'y')
|
|
z = checkpoint_name(jnp.multiply(2., 2.), 'z')
|
|
w = checkpoint_name(jnp.multiply(2., 2.), 'w')
|
|
u = jnp.multiply(2., 2.)
|
|
return (((x * y) * z) * w) * u
|
|
|
|
policy = jax.checkpoint_policies.save_any_names_but_these('y', 'z', 'w')
|
|
res = saved_residuals(new_checkpoint(f, policy=policy), 1.)
|
|
self.assertLen(res, 0) # can't save anything
|
|
|
|
policy = jax.checkpoint_policies.save_any_names_but_these('z', 'w')
|
|
res = saved_residuals(new_checkpoint(f, policy=policy), 1.)
|
|
self.assertLen(res, 1) # can save only y
|
|
|
|
policy = jax.checkpoint_policies.save_any_names_but_these('w')
|
|
res = saved_residuals(new_checkpoint(f, policy=policy), 1.)
|
|
self.assertLen(res, 2) # can save y and z
|
|
|
|
policy = jax.checkpoint_policies.save_any_names_but_these()
|
|
res = saved_residuals(new_checkpoint(f, policy=policy), 1.)
|
|
self.assertLen(res, 3) # can save y, z, and w
|
|
|
|
def test_name_allowlist(self):
|
|
def f(x):
|
|
y = checkpoint_name(jnp.multiply(2., 2.), 'y')
|
|
z = checkpoint_name(jnp.multiply(2., 2.), 'z')
|
|
w = checkpoint_name(jnp.multiply(2., 2.), 'w')
|
|
u = jnp.multiply(2., 2.)
|
|
return (((x * y) * z) * w) * u
|
|
|
|
policy = jax.checkpoint_policies.save_only_these_names('y', 'z', 'w')
|
|
res = saved_residuals(new_checkpoint(f, policy=policy), 1.)
|
|
self.assertLen(res, 3) # can save y, z, and w
|
|
|
|
policy = jax.checkpoint_policies.save_only_these_names('z', 'w')
|
|
res = saved_residuals(new_checkpoint(f, policy=policy), 1.)
|
|
self.assertLen(res, 2) # can save z and w
|
|
|
|
policy = jax.checkpoint_policies.save_only_these_names('w')
|
|
res = saved_residuals(new_checkpoint(f, policy=policy), 1.)
|
|
self.assertLen(res, 1) # can save w
|
|
|
|
policy = jax.checkpoint_policies.save_only_these_names()
|
|
res = saved_residuals(new_checkpoint(f, policy=policy), 1.)
|
|
self.assertLen(res, 0) # can't save anything!
|
|
|
|
def test_saved_residuals_utility(self):
|
|
def f(x, y):
|
|
x1, x2 = x
|
|
z = checkpoint_name(jnp.sin(3.), 'z')
|
|
return z * ((x1 * x2) * y) * np.array([3.])
|
|
|
|
res = saved_residuals(f, (2., 3.), y=4.)
|
|
self.assertLen(res, 6)
|
|
self.assertEqual(res[0][0].shape, (1,))
|
|
self.assertEqual(res[0][1], "from a constant")
|
|
self.assertEqual(res[1][0].shape, ())
|
|
self.assertEqual(res[1][1], "from the argument x[0]")
|
|
self.assertEqual(res[2][0].shape, ())
|
|
self.assertEqual(res[2][1], "from the argument x[1]")
|
|
self.assertEqual(res[3][0].shape, ())
|
|
self.assertEqual(res[3][1], "from the argument y")
|
|
self.assertEqual(res[4][0].shape, ())
|
|
self.assertStartsWith(res[4][1], "named 'z'")
|
|
self.assertEqual(res[5][0].shape, ())
|
|
|
|
def test_saved_residuals_utility_jit(self):
|
|
@jax.jit
|
|
def f(x, y):
|
|
x1, x2 = x
|
|
z = checkpoint_name(jnp.sin(3.), 'z')
|
|
return z * ((x1 * x2) * y) * np.array([3.])
|
|
|
|
res = saved_residuals(f, (2., 3.), y=4.)
|
|
self.assertLen(res, 6)
|
|
self.assertEqual(res[0][0].shape, ())
|
|
self.assertEqual(res[0][1], "from the argument x[0]")
|
|
self.assertEqual(res[1][0].shape, ())
|
|
self.assertEqual(res[1][1], "from the argument x[1]")
|
|
self.assertEqual(res[2][0].shape, ())
|
|
self.assertEqual(res[2][1], "from the argument y")
|
|
self.assertEqual(res[3][0].shape, ())
|
|
self.assertStartsWith(res[3][1], "output of jitted function 'f'")
|
|
self.assertEqual(res[4][0].shape, ())
|
|
self.assertEqual(res[5][0].shape, (1,))
|
|
self.assertStartsWith(res[5][1], "output of jitted function 'f'")
|
|
|
|
@parameterized.named_parameters(
|
|
{"testcase_name": f"{suffix}", "remat": remat}
|
|
for suffix, remat in [
|
|
('', jax.remat),
|
|
('_policy', partial(jax.remat, policy=lambda *_, **__: False)),
|
|
('_new', partial(new_checkpoint, policy=lambda *_, **__: False)),
|
|
])
|
|
def test_checkpoint_dropvars(self, remat):
|
|
@remat
|
|
def f(x):
|
|
_, x = api.jit(lambda: (x, x))()
|
|
return x
|
|
|
|
_ = api.grad(f)(3.) # doesn't crash
|
|
|
|
def test_dce_keeps_eqns_with_used_outputs_but_no_used_inputs(self):
|
|
@new_checkpoint
|
|
def f(x):
|
|
c = jax.jit(lambda: 3.)()
|
|
return c * x
|
|
|
|
_ = jax.grad(f)(3.) # doesn't crash
|
|
|
|
def test_linearize_caching(self):
|
|
# https://github.com/jax-ml/jax/issues/9661
|
|
identity = jax.checkpoint(jax.jit(lambda x: 2 * x))
|
|
_, f_lin = jax.linearize(identity, 1.)
|
|
with jtu.count_jit_and_pmap_lowerings() as count: # noqa: F841
|
|
for _ in range(20):
|
|
f_lin(1.).block_until_ready()
|
|
self.assertEqual(count(), 1) # cached after first execution
|
|
|
|
def test_vjp_caching(self):
|
|
# https://github.com/jax-ml/jax/issues/9661
|
|
identity = jax.checkpoint(jax.jit(lambda x: 2 * x))
|
|
_, f_vjp = jax.vjp(identity, 1.)
|
|
with jtu.count_pjit_cpp_cache_miss() as count: # noqa: F841
|
|
for _ in range(20):
|
|
f_vjp(1.)[0].block_until_ready()
|
|
self.assertEqual(count(), 2) # fwd execute_trivial, backward_pass on bwd
|
|
|
|
def test_vjp_caching_static_argnums(self):
|
|
identity = jax.remat(lambda x, y: jax.jit(lambda x: 2 * x if y else x)(x),
|
|
static_argnums=(1,))
|
|
_, f_vjp = jax.vjp(lambda x: identity(x, True), 1.)
|
|
with jtu.count_jit_and_pmap_lowerings() as count: # noqa: F841
|
|
for _ in range(20):
|
|
f_vjp(1.)[0].block_until_ready()
|
|
self.assertEqual(count(), 2) # fwd execute_trivial, backward_pass on bwd
|
|
|
|
def test_fwd_caching(self):
|
|
# see above test also
|
|
identity = jax.checkpoint(jax.jit(lambda x: 2 * x))
|
|
with jtu.count_jit_and_pmap_lowerings() as count: # noqa: F841
|
|
for _ in range(20):
|
|
y, _ = jax.vjp(identity, 1.)
|
|
y.block_until_ready()
|
|
self.assertEqual(count(), 1)
|
|
|
|
def test_fwd_caching_static_argnums(self):
|
|
# see above test also
|
|
identity = jax.checkpoint(jax.jit(lambda x: 2 * x), static_argnums=(0,))
|
|
with jtu.count_jit_and_pmap_lowerings() as count: # noqa: F841
|
|
for _ in range(20):
|
|
y = identity(1.)
|
|
y.block_until_ready()
|
|
self.assertEqual(count(), 1)
|
|
|
|
@parameterized.named_parameters(
|
|
{"testcase_name": f"{suffix}", "remat": remat}
|
|
for suffix, remat in [
|
|
('', jax.remat),
|
|
('_new', new_checkpoint),
|
|
])
|
|
def test_remat_of_scan(self, remat):
|
|
to_scan = lambda c, _: (jnp.sin(c), jnp.sin(c))
|
|
f = lambda x: lax.scan(to_scan, x, None, length=3)
|
|
jtu.check_grads(remat(f), (3.,), order=2, modes=['rev'])
|
|
|
|
jaxpr = api.make_jaxpr(api.linearize(remat(f), 4.)[1])(1.)
|
|
self.assertIn(' sin ', str(jaxpr))
|
|
self.assertIn(' cos ', str(jaxpr))
|
|
|
|
@parameterized.named_parameters(
|
|
{"testcase_name": f"{suffix}", "remat": remat}
|
|
for suffix, remat in [
|
|
('', jax.remat),
|
|
('_new', new_checkpoint),
|
|
])
|
|
def test_const_in_jvp_scan(self, remat):
|
|
@jax.custom_jvp
|
|
def f(x):
|
|
return x * jnp.arange(3.)
|
|
@f.defjvp
|
|
def f_jvp(primals, tangents):
|
|
(x,), (xdot,) = primals, tangents
|
|
return f(x), xdot * jnp.arange(3.)
|
|
|
|
@remat
|
|
def g(x):
|
|
def body(c, _):
|
|
return f(c), None
|
|
y, _ = jax.lax.scan(body, x, None, length=1)
|
|
return y.sum()
|
|
|
|
jax.grad(g)(jnp.arange(3.)) # doesn't crash
|
|
|
|
def test_remat_checkpoint_dots_outside_scan(self):
|
|
# see also above test test_remat_checkpoint_dots_inside_scan
|
|
x = jnp.ones((5,))
|
|
|
|
@partial(new_checkpoint, policy=jax.checkpoint_policies.checkpoint_dots)
|
|
def f(W):
|
|
def f(x):
|
|
x = jnp.sin(jnp.dot(x, W, precision=lax.Precision.HIGHEST))
|
|
x = jnp.sin(jnp.dot(x, W, precision=lax.Precision.HIGHEST))
|
|
x = jnp.sin(jnp.dot(x, W, precision=lax.Precision.HIGHEST))
|
|
return x
|
|
|
|
def body(x, _): return f(x), None
|
|
return lax.scan(body, x, None, length=2)[0]
|
|
|
|
_, f_vjp = api.vjp(f, jnp.ones((5, 5)))
|
|
jaxpr = f_vjp.args[0].func.args[1]
|
|
jaxpr_text = str(jaxpr)
|
|
|
|
self.assertEqual(jaxpr_text.count(' sin '), 3)
|
|
self.assertEqual(jaxpr_text.count(' cos '), 3)
|
|
# Six calls to dot_general in the backward pass because we save the primal
|
|
# matmuls and only compure the backward pass ones (two for each primal one).
|
|
self.assertEqual(jaxpr_text.count(' dot_'), 6)
|
|
|
|
jtu.check_grads(api.jit(f), (jnp.ones((5, 5)),), order=2,
|
|
modes=['fwd', 'rev'])
|
|
|
|
def test_remat_of_scan_policy(self):
|
|
save_cos = lambda prim, *_, **__: str(prim) == 'cos'
|
|
to_scan = lambda c, _: (jnp.sin(c), jnp.sin(c))
|
|
f = new_checkpoint(lambda x: lax.scan(to_scan, x, None, length=3),
|
|
policy=save_cos)
|
|
jtu.check_grads(f, (3.,), order=2, modes=['rev'])
|
|
|
|
jaxpr = api.make_jaxpr(api.linearize(f, 4.)[1])(1.)
|
|
jaxpr_text = str(jaxpr)
|
|
self.assertEqual(jaxpr_text.count(' sin '), 0)
|
|
self.assertEqual(jaxpr_text.count(' cos '), 0)
|
|
|
|
def test_remat_of_scan_funky_custom_jvp(self):
|
|
def scan_apply(f, x):
|
|
y, _ = lax.scan(lambda x, _: (f(x), None), x, None, length=1)
|
|
return y
|
|
|
|
@jax.custom_jvp
|
|
def sin(x):
|
|
return jnp.sin(x)
|
|
def sin_jvp(primals, tangents):
|
|
x, = primals
|
|
xdot, = tangents
|
|
y, c = jax.jit(lambda: (jnp.sin(x), jnp.cos(x)))()
|
|
ydot = c * xdot
|
|
return y, ydot
|
|
sin.defjvp(sin_jvp)
|
|
|
|
save_cos = lambda prim, *_, **__: str(prim) == 'cos'
|
|
f = new_checkpoint(partial(scan_apply, sin), policy=save_cos)
|
|
jtu.check_grads(f, (3.,), order=2, modes=['rev'])
|
|
jaxpr = api.make_jaxpr(api.linearize(f, 4.)[1])(1.)
|
|
jaxpr_text = str(jaxpr)
|
|
self.assertEqual(jaxpr_text.count(' sin '), 0)
|
|
self.assertEqual(jaxpr_text.count(' cos '), 0)
|
|
|
|
save_sin = lambda prim, *_, **__: str(prim) == 'sin'
|
|
f = new_checkpoint(partial(scan_apply, sin), policy=save_sin)
|
|
jtu.check_grads(f, (3.,), order=2, modes=['rev'])
|
|
jaxpr = api.make_jaxpr(api.linearize(f, 4.)[1])(1.)
|
|
jaxpr_text = str(jaxpr)
|
|
self.assertEqual(jaxpr_text.count(' sin '), 0)
|
|
self.assertEqual(jaxpr_text.count(' cos '), 1)
|
|
|
|
f = new_checkpoint(partial(scan_apply, sin),
|
|
policy=jax.checkpoint_policies.everything_saveable)
|
|
jtu.check_grads(f, (3.,), order=2, modes=['rev'])
|
|
jaxpr = api.make_jaxpr(api.linearize(f, 4.)[1])(1.)
|
|
jaxpr_text = str(jaxpr)
|
|
self.assertEqual(jaxpr_text.count(' sin '), 0)
|
|
self.assertEqual(jaxpr_text.count(' cos '), 0)
|
|
|
|
f = new_checkpoint(partial(scan_apply, sin),
|
|
policy=jax.checkpoint_policies.nothing_saveable)
|
|
jtu.check_grads(f, (3.,), order=2, modes=['rev'])
|
|
jaxpr = api.make_jaxpr(api.linearize(f, 4.)[1])(1.)
|
|
jaxpr_text = str(jaxpr)
|
|
self.assertEqual(jaxpr_text.count(' sin '), 1) # +1 b/c dce fixed point
|
|
self.assertEqual(jaxpr_text.count(' cos '), 1)
|
|
|
|
f = new_checkpoint(lambda x: scan_apply(sin, scan_apply(sin, x)),
|
|
policy=jax.checkpoint_policies.nothing_saveable)
|
|
jtu.check_grads(f, (3.,), order=2, modes=['rev'])
|
|
jaxpr = api.make_jaxpr(api.linearize(f, 4.)[1])(1.)
|
|
jaxpr_text = str(jaxpr)
|
|
self.assertEqual(jaxpr_text.count(' sin '), 2) # +1 b/c dce fixed point
|
|
self.assertEqual(jaxpr_text.count(' cos '), 2)
|
|
|
|
def test_remat_of_scan_funky_custom_jvp2(self):
|
|
# Like the above test but instead of using jit inside custom_jvp, use scan.
|
|
|
|
def scan_apply(f, x):
|
|
y, _ = lax.scan(lambda x, _: (f(x), None), x, None, length=1)
|
|
return y
|
|
|
|
@jax.custom_jvp
|
|
def sin(x):
|
|
return jnp.sin(x)
|
|
def sin_jvp(primals, tangents):
|
|
x, = primals
|
|
xdot, = tangents
|
|
y, c = scan_apply(lambda xs: (jnp.sin(xs[0]), jnp.cos(xs[1])), (x, x))
|
|
ydot = c * xdot
|
|
return y, ydot
|
|
sin.defjvp(sin_jvp)
|
|
|
|
save_cos = lambda prim, *_, **__: str(prim) == 'cos'
|
|
f = new_checkpoint(partial(scan_apply, sin), policy=save_cos)
|
|
jtu.check_grads(f, (3.,), order=2, modes=['rev'])
|
|
jaxpr = api.make_jaxpr(api.linearize(f, 4.)[1])(1.)
|
|
jaxpr_text = str(jaxpr)
|
|
self.assertEqual(jaxpr_text.count(' sin '), 1) # +1 b/c dce fixed point
|
|
self.assertEqual(jaxpr_text.count(' cos '), 0)
|
|
|
|
save_sin = lambda prim, *_, **__: str(prim) == 'sin'
|
|
f = new_checkpoint(partial(scan_apply, sin), policy=save_sin)
|
|
jtu.check_grads(f, (3.,), order=2, modes=['rev'])
|
|
jaxpr = api.make_jaxpr(api.linearize(f, 4.)[1])(1.)
|
|
jaxpr_text = str(jaxpr)
|
|
self.assertEqual(jaxpr_text.count(' sin '), 0)
|
|
self.assertEqual(jaxpr_text.count(' cos '), 1)
|
|
|
|
f = new_checkpoint(partial(scan_apply, sin),
|
|
policy=jax.checkpoint_policies.everything_saveable)
|
|
jtu.check_grads(f, (3.,), order=2, modes=['rev'])
|
|
jaxpr = api.make_jaxpr(api.linearize(f, 4.)[1])(1.)
|
|
jaxpr_text = str(jaxpr)
|
|
self.assertEqual(jaxpr_text.count(' sin '), 0)
|
|
self.assertEqual(jaxpr_text.count(' cos '), 0)
|
|
|
|
f = new_checkpoint(partial(scan_apply, sin),
|
|
policy=jax.checkpoint_policies.nothing_saveable)
|
|
jtu.check_grads(f, (3.,), order=2, modes=['rev'])
|
|
jaxpr = api.make_jaxpr(api.linearize(f, 4.)[1])(1.)
|
|
jaxpr_text = str(jaxpr)
|
|
self.assertEqual(jaxpr_text.count(' sin '), 1) # +1 b/c dce fixed point
|
|
self.assertEqual(jaxpr_text.count(' cos '), 1)
|
|
|
|
f = new_checkpoint(lambda x: scan_apply(sin, scan_apply(sin, x)),
|
|
policy=jax.checkpoint_policies.nothing_saveable)
|
|
jtu.check_grads(f, (3.,), order=2, modes=['rev'])
|
|
jaxpr = api.make_jaxpr(api.linearize(f, 4.)[1])(1.)
|
|
jaxpr_text = str(jaxpr)
|
|
self.assertEqual(jaxpr_text.count(' sin '), 2) # +1 b/c dce fixed point
|
|
self.assertEqual(jaxpr_text.count(' cos '), 2)
|
|
|
|
@parameterized.named_parameters(
|
|
{"testcase_name": f"{suffix}", "remat": remat}
|
|
for suffix, remat in [
|
|
('', jax.remat),
|
|
('_new', new_checkpoint),
|
|
])
|
|
def test_remat_of_cond(self, remat):
|
|
true_fn = lambda c: (jnp.sin(c), jnp.sin(c))
|
|
false_fn = lambda c: (jnp.sin(c), jnp.sin(c))
|
|
f = lambda x: lax.cond(x > 0., true_fn, false_fn, x)
|
|
jtu.check_grads(remat(f), (3.,), order=2, modes=['rev'])
|
|
|
|
jaxpr = api.make_jaxpr(api.linearize(remat(f), 4.)[1])(1.)
|
|
self.assertNotIn(' sin ', str(jaxpr))
|
|
self.assertIn(' cos ', str(jaxpr))
|
|
|
|
true_fn = lambda c: jnp.sin(jnp.sin(c))
|
|
false_fn = lambda c: c
|
|
f = lambda x: lax.cond(x > 0., true_fn, false_fn, x)
|
|
jtu.check_grads(remat(f), (3.,), order=2, modes=['rev'])
|
|
|
|
jaxpr = api.make_jaxpr(api.linearize(remat(f), 4.)[1])(1.)
|
|
self.assertIn(' sin ', str(jaxpr))
|
|
self.assertIn(' cos ', str(jaxpr))
|
|
|
|
@parameterized.named_parameters(
|
|
{"testcase_name": f"{suffix}", "remat": remat}
|
|
for suffix, remat in [
|
|
('', jax.remat),
|
|
('_new', new_checkpoint),
|
|
])
|
|
def test_const_in_jvp_cond(self, remat):
|
|
@jax.custom_jvp
|
|
def f(x):
|
|
return x * jnp.arange(3.)
|
|
@f.defjvp
|
|
def f_jvp(primals, tangents):
|
|
(x,), (xdot,) = primals, tangents
|
|
return f(x), xdot * jnp.arange(3.)
|
|
|
|
@remat
|
|
def g(x):
|
|
y = jax.lax.cond(x.sum() > 0, f, lambda x: x, x)
|
|
return y.sum()
|
|
|
|
jax.grad(g)(jnp.arange(3.)) # doesn't crash
|
|
|
|
def test_remat_checkpoint_dots_inside_cond(self):
|
|
x = jnp.ones((5,))
|
|
|
|
def f(W):
|
|
@partial(jax.remat, policy=jax.checkpoint_policies.checkpoint_dots)
|
|
def f(x):
|
|
x = jnp.sin(jnp.dot(x, W, precision=lax.Precision.HIGHEST))
|
|
x = jnp.sin(jnp.dot(x, W, precision=lax.Precision.HIGHEST))
|
|
x = jnp.sin(jnp.dot(x, W, precision=lax.Precision.HIGHEST))
|
|
return x
|
|
|
|
return lax.cond(x.sum() > 0, f, lambda x: x, x)
|
|
|
|
_, f_vjp = api.vjp(f, jnp.ones((5, 5)))
|
|
jaxpr_text = str(f_vjp.args[0].func.args[1])
|
|
|
|
self.assertEqual(jaxpr_text.count(' sin '), 2)
|
|
self.assertEqual(jaxpr_text.count(' cos '), 3)
|
|
# Five calls to dot_general in the backward pass because we have two for
|
|
# each forward-pass dot, except for the first which only has one (as we are
|
|
# differentiating with respect to only W and not x).
|
|
self.assertEqual(jaxpr_text.count(' dot_'), 5)
|
|
|
|
jtu.check_grads(api.jit(f), (jnp.ones((5, 5)),), order=2,
|
|
modes=['fwd', 'rev'])
|
|
|
|
def test_remat_checkpoint_dots_outside_cond(self):
|
|
# see also above test test_remat_checkpoint_dots_inside_cond
|
|
# The behavior between the two tests is essentially identical, whereas for
|
|
# scan different things are saved based on this difference in remat
|
|
# placement (because of the carry).
|
|
x = jnp.ones((5,))
|
|
|
|
@partial(new_checkpoint, policy=jax.checkpoint_policies.checkpoint_dots)
|
|
def f(W):
|
|
def f(x):
|
|
x = jnp.sin(jnp.dot(x, W, precision=lax.Precision.HIGHEST))
|
|
x = jnp.sin(jnp.dot(x, W, precision=lax.Precision.HIGHEST))
|
|
x = jnp.sin(jnp.dot(x, W, precision=lax.Precision.HIGHEST))
|
|
return x
|
|
|
|
return lax.cond(x.sum() > 0, f, lambda x: x, x)
|
|
|
|
_, f_vjp = api.vjp(f, jnp.ones((5, 5)))
|
|
jaxpr = f_vjp.args[0].func.args[1]
|
|
jaxpr_text = str(jaxpr)
|
|
|
|
self.assertEqual(jaxpr_text.count(' sin '), 2)
|
|
self.assertEqual(jaxpr_text.count(' cos '), 3)
|
|
self.assertEqual(jaxpr_text.count(' dot_'), 5)
|
|
|
|
jtu.check_grads(api.jit(f), (jnp.ones((5, 5)),), order=2,
|
|
modes=['fwd', 'rev'])
|
|
|
|
def test_remat_of_cond_policy(self):
|
|
save_cos = lambda prim, *_, **__: str(prim) == 'cos'
|
|
f = new_checkpoint(lambda x: lax.cond(x > 0, jnp.sin, lambda x: x, x),
|
|
policy=save_cos)
|
|
jtu.check_grads(f, (3.,), order=2, modes=['rev'])
|
|
|
|
jaxpr = api.make_jaxpr(api.linearize(f, 4.)[1])(1.)
|
|
jaxpr_text = str(jaxpr)
|
|
self.assertEqual(jaxpr_text.count(' sin '), 0)
|
|
self.assertEqual(jaxpr_text.count(' cos '), 0)
|
|
|
|
def test_remat_of_cond_funky_custom_jvp(self):
|
|
def cond_apply(f, x):
|
|
return lax.cond(x.sum() > -jnp.inf, f, lambda x: x, x)
|
|
|
|
@jax.custom_jvp
|
|
def sin(x):
|
|
return jnp.sin(x)
|
|
def sin_jvp(primals, tangents):
|
|
x, = primals
|
|
xdot, = tangents
|
|
y, c = jax.jit(lambda: (jnp.sin(x), jnp.cos(x)))()
|
|
ydot = c * xdot
|
|
return y, ydot
|
|
sin.defjvp(sin_jvp)
|
|
|
|
save_cos = lambda prim, *_, **__: str(prim) == 'cos'
|
|
f = new_checkpoint(partial(cond_apply, sin), policy=save_cos)
|
|
jtu.check_grads(f, (3.,), order=2, modes=['rev'])
|
|
jaxpr = api.make_jaxpr(api.linearize(f, 4.)[1])(1.)
|
|
jaxpr_text = str(jaxpr)
|
|
self.assertEqual(jaxpr_text.count(' sin '), 0)
|
|
self.assertEqual(jaxpr_text.count(' cos '), 0)
|
|
|
|
save_sin = lambda prim, *_, **__: str(prim) == 'sin'
|
|
f = new_checkpoint(partial(cond_apply, sin), policy=save_sin)
|
|
jtu.check_grads(f, (3.,), order=2, modes=['rev'])
|
|
jaxpr = api.make_jaxpr(api.linearize(f, 4.)[1])(1.)
|
|
jaxpr_text = str(jaxpr)
|
|
self.assertEqual(jaxpr_text.count(' sin '), 0)
|
|
self.assertEqual(jaxpr_text.count(' cos '), 1)
|
|
|
|
f = new_checkpoint(partial(cond_apply, sin),
|
|
policy=jax.checkpoint_policies.everything_saveable)
|
|
jtu.check_grads(f, (3.,), order=2, modes=['rev'])
|
|
jaxpr = api.make_jaxpr(api.linearize(f, 4.)[1])(1.)
|
|
jaxpr_text = str(jaxpr)
|
|
self.assertEqual(jaxpr_text.count(' sin '), 0)
|
|
self.assertEqual(jaxpr_text.count(' cos '), 0)
|
|
|
|
f = new_checkpoint(partial(cond_apply, sin),
|
|
policy=jax.checkpoint_policies.nothing_saveable)
|
|
jtu.check_grads(f, (3.,), order=2, modes=['rev'])
|
|
jaxpr = api.make_jaxpr(api.linearize(f, 4.)[1])(1.)
|
|
jaxpr_text = str(jaxpr)
|
|
self.assertEqual(jaxpr_text.count(' sin '), 0)
|
|
self.assertEqual(jaxpr_text.count(' cos '), 1)
|
|
|
|
f = new_checkpoint(lambda x: cond_apply(sin, cond_apply(sin, x)),
|
|
policy=jax.checkpoint_policies.nothing_saveable)
|
|
jtu.check_grads(f, (3.,), order=2, modes=['rev'])
|
|
jaxpr = api.make_jaxpr(api.linearize(f, 4.)[1])(1.)
|
|
jaxpr_text = str(jaxpr)
|
|
self.assertEqual(jaxpr_text.count(' sin '), 1)
|
|
self.assertEqual(jaxpr_text.count(' cos '), 2)
|
|
|
|
def test_remat_of_cond_funky_custom_jvp2(self):
|
|
# Like the above test but instead of using jit inside custom_jvp, use cond.
|
|
|
|
def cond_apply(f, x):
|
|
return lax.cond(True, f, lambda x: x, x)
|
|
|
|
@jax.custom_jvp
|
|
def sin(x):
|
|
return jnp.sin(x)
|
|
def sin_jvp(primals, tangents):
|
|
x, = primals
|
|
xdot, = tangents
|
|
y, c = cond_apply(lambda xs: (jnp.sin(xs[0]), jnp.cos(xs[1])), (x, x))
|
|
ydot = c * xdot
|
|
return y, ydot
|
|
sin.defjvp(sin_jvp)
|
|
|
|
save_cos = lambda prim, *_, **__: str(prim) == 'cos'
|
|
f = new_checkpoint(partial(cond_apply, sin), policy=save_cos)
|
|
jtu.check_grads(f, (3.,), order=2, modes=['rev'])
|
|
jaxpr = api.make_jaxpr(api.linearize(f, 4.)[1])(1.)
|
|
jaxpr_text = str(jaxpr)
|
|
self.assertEqual(jaxpr_text.count(' sin '), 0)
|
|
self.assertEqual(jaxpr_text.count(' cos '), 0)
|
|
|
|
save_sin = lambda prim, *_, **__: str(prim) == 'sin'
|
|
f = new_checkpoint(partial(cond_apply, sin), policy=save_sin)
|
|
jtu.check_grads(f, (3.,), order=2, modes=['rev'])
|
|
jaxpr = api.make_jaxpr(api.linearize(f, 4.)[1])(1.)
|
|
jaxpr_text = str(jaxpr)
|
|
self.assertEqual(jaxpr_text.count(' sin '), 0)
|
|
self.assertEqual(jaxpr_text.count(' cos '), 1)
|
|
|
|
f = new_checkpoint(partial(cond_apply, sin),
|
|
policy=jax.checkpoint_policies.everything_saveable)
|
|
jtu.check_grads(f, (3.,), order=2, modes=['rev'])
|
|
jaxpr = api.make_jaxpr(api.linearize(f, 4.)[1])(1.)
|
|
jaxpr_text = str(jaxpr)
|
|
self.assertEqual(jaxpr_text.count(' sin '), 0)
|
|
self.assertEqual(jaxpr_text.count(' cos '), 0)
|
|
|
|
f = new_checkpoint(partial(cond_apply, sin),
|
|
policy=jax.checkpoint_policies.nothing_saveable)
|
|
jtu.check_grads(f, (3.,), order=2, modes=['rev'])
|
|
jaxpr = api.make_jaxpr(api.linearize(f, 4.)[1])(1.)
|
|
jaxpr_text = str(jaxpr)
|
|
self.assertEqual(jaxpr_text.count(' sin '), 0)
|
|
self.assertEqual(jaxpr_text.count(' cos '), 1)
|
|
|
|
f = new_checkpoint(lambda x: cond_apply(sin, cond_apply(sin, x)),
|
|
policy=jax.checkpoint_policies.nothing_saveable)
|
|
jtu.check_grads(f, (3.,), order=2, modes=['rev'])
|
|
jaxpr = api.make_jaxpr(api.linearize(f, 4.)[1])(1.)
|
|
jaxpr_text = str(jaxpr)
|
|
self.assertEqual(jaxpr_text.count(' sin '), 1)
|
|
self.assertEqual(jaxpr_text.count(' cos '), 2)
|
|
|
|
@parameterized.named_parameters(
|
|
{"testcase_name": f"{suffix}", "remat": remat}
|
|
for suffix, remat in [
|
|
('', jax.remat),
|
|
('_new', new_checkpoint),
|
|
])
|
|
def test_remat_of_while_loop(self, remat):
|
|
def cond_fn(carry):
|
|
i, _ = carry
|
|
return i < 3
|
|
def body_fn(carry):
|
|
i, x = carry
|
|
return i + 1, jnp.sin(x)
|
|
def f(x):
|
|
_, y = lax.while_loop(cond_fn, body_fn, (0, x))
|
|
return y
|
|
|
|
_, f_lin = jax.linearize(remat(f), 3.)
|
|
y_dot = f_lin(1.0)
|
|
expected = jax.grad(lambda x: jnp.sin(jnp.sin(jnp.sin(x))))(3.)
|
|
self.assertArraysAllClose(y_dot, expected, check_dtypes=False)
|
|
|
|
jaxpr = api.make_jaxpr(jax.linearize(remat(f), 4.)[1])(1.)
|
|
self.assertIn(' sin ', str(jaxpr))
|
|
self.assertIn(' cos ', str(jaxpr))
|
|
|
|
def test_remat_of_while_loop_policy(self):
|
|
def cond_fn(carry):
|
|
i, _ = carry
|
|
return i < 3
|
|
def body_fn(carry):
|
|
i, x = carry
|
|
return i + 1, jnp.sin(x)
|
|
def f(x):
|
|
_, y = lax.while_loop(cond_fn, body_fn, (0, x))
|
|
return y
|
|
|
|
# even with a policy, we can't save residuals (w/o dynamic shapes)!
|
|
save_cos = lambda prim, *_, **__: str(prim) == 'cos'
|
|
g = new_checkpoint(f, policy=save_cos)
|
|
jaxpr = api.make_jaxpr(jax.linearize(g, 4.)[1])(1.)
|
|
self.assertIn(' sin ', str(jaxpr))
|
|
self.assertIn(' cos ', str(jaxpr))
|
|
|
|
@jtu.thread_unsafe_test() # logging isn't thread-safe
|
|
def test_remat_residual_logging(self):
|
|
def f(x):
|
|
x = jnp.sin(x)
|
|
x = jnp.cos(x.sum())
|
|
return x
|
|
|
|
x = jnp.arange(3.)
|
|
|
|
f1 = jax.remat(f)
|
|
f2 = jax.remat(f, policy=lambda *_, **__: True)
|
|
f3 = jax.remat(f, policy=lambda p, *_, **__: str(p) == 'cos')
|
|
|
|
prev_level = logging.get_verbosity()
|
|
try:
|
|
logging.set_verbosity('DEBUG')
|
|
with self.assertLogs(level=logging.DEBUG) as l:
|
|
jax.grad(f1)(x)
|
|
finally:
|
|
logging.set_verbosity(prev_level)
|
|
self.assertTrue(any('remat-decorated function saving inputs with shapes:'
|
|
in line for line in l.output))
|
|
self.assertFalse(any('intermediates' in line for line in l.output))
|
|
|
|
prev_level = logging.get_verbosity()
|
|
try:
|
|
logging.set_verbosity('DEBUG')
|
|
with self.assertLogs(level=logging.DEBUG) as l:
|
|
jax.grad(f2)(x)
|
|
finally:
|
|
logging.set_verbosity(prev_level)
|
|
self.assertFalse(any('saving inputs' in line for line in l.output))
|
|
self.assertTrue(any('remat-decorated function saving these intermediates:'
|
|
in line for line in l.output))
|
|
self.assertTrue(any(' sin ' in line for line in l.output))
|
|
self.assertTrue(any(' cos ' in line for line in l.output))
|
|
|
|
prev_level = logging.get_verbosity()
|
|
try:
|
|
logging.set_verbosity('DEBUG')
|
|
with self.assertLogs(level=logging.DEBUG) as l:
|
|
jax.grad(f3)(x)
|
|
finally:
|
|
logging.set_verbosity(prev_level)
|
|
self.assertTrue(any('remat-decorated function saving inputs with shapes:'
|
|
in line for line in l.output))
|
|
self.assertTrue(any('and saving these intermediates:'
|
|
in line for line in l.output))
|
|
self.assertFalse(any(' sin ' in line for line in l.output))
|
|
self.assertTrue(any(' cos ' in line for line in l.output))
|
|
|
|
def test_excess_precision_hell(self):
|
|
finfo = jnp.finfo('bfloat16')
|
|
eps = finfo.eps
|
|
|
|
@jax.custom_vjp
|
|
def dot(x):
|
|
return jnp.dot(x, x)
|
|
def dot_fwd(x):
|
|
return dot(x), None
|
|
def dot_bwd(_, g):
|
|
return g,
|
|
dot.defvjp(dot_fwd, dot_bwd)
|
|
|
|
@jax.custom_vjp
|
|
def foo(x):
|
|
return jnp.float32(1.) * x.astype('float32')
|
|
def foo_fwd(x):
|
|
return foo(x), x
|
|
def foo_bwd(x, _):
|
|
return jnp.float32(1.) * x.astype('float32'),
|
|
foo.defvjp(foo_fwd, foo_bwd)
|
|
|
|
@jax.jit
|
|
@partial(jax.remat, policy=lambda *_, **__: True)
|
|
def f(x):
|
|
x = dot(x)
|
|
return foo(x)
|
|
|
|
x = (jnp.bfloat16(1) + eps) * jnp.eye(2, dtype='bfloat16')
|
|
y, vjp = jax.vjp(f, x)
|
|
y_, = vjp(jnp.ones_like(y))
|
|
self.assertAllClose(y, y_, atol=0, rtol=0)
|
|
|
|
def test_concreteness_error_includes_user_code(self):
|
|
@jax.remat
|
|
def f(x):
|
|
if x > 0:
|
|
return x
|
|
else:
|
|
return jnp.sin(x)
|
|
|
|
try:
|
|
f(3.)
|
|
except TracerBoolConversionError:
|
|
self.assertIn('x > 0', traceback.format_exc())
|
|
else:
|
|
assert False
|
|
|
|
def test_concreteness_error_includes_user_code_with_static_argnums(self):
|
|
@partial(jax.remat, static_argnums=(1,))
|
|
def f(x, _):
|
|
if x > 0:
|
|
return x
|
|
else:
|
|
return jnp.sin(x)
|
|
|
|
try:
|
|
f(3., 1.)
|
|
except TracerBoolConversionError:
|
|
self.assertIn('x > 0', traceback.format_exc())
|
|
else:
|
|
assert False
|
|
|
|
|
|
@jtu.with_config(jax_pprint_use_color=False)
|
|
class JaxprTest(jtu.JaxTestCase):
|
|
|
|
def test_scalar_literals(self):
|
|
jaxpr = api.make_jaxpr(lambda x: x + 2)(42)
|
|
self.assertLen(jaxpr.jaxpr.constvars, 0)
|
|
|
|
def test_abstract_inputs(self):
|
|
jaxpr = api.make_jaxpr(lambda x: x + 2.)(
|
|
types.SimpleNamespace(shape=(), dtype=np.dtype(np.float32)))
|
|
self.assertEqual(jaxpr.in_avals[0].shape, ())
|
|
self.assertEqual(jaxpr.in_avals[0].dtype, np.float32)
|
|
|
|
def test_const(self):
|
|
def fun(x):
|
|
return (x, 1., np.zeros(1, dtype=jnp.float32))
|
|
|
|
expected = "{ lambda a:f32[1]; b:f32[]. let in (b, 1.0, a) }"
|
|
jaxpr = api.make_jaxpr(fun)(jnp.float32(0.))
|
|
self.assertMultiLineStrippedEqual(expected, str(jaxpr))
|
|
|
|
def test_cond(self):
|
|
def f(x):
|
|
return lax.cond(x >= 0.,
|
|
x + 1.,
|
|
lambda xt: xt + x,
|
|
x + 2.,
|
|
lambda xf: xf - x)
|
|
expected = """{ lambda ; a:f32[]. let
|
|
b:bool[] = ge a 0.0
|
|
c:f32[] = add a 1.0
|
|
d:f32[] = add a 2.0
|
|
e:i32[] = convert_element_type[new_dtype=int32 weak_type=False] b
|
|
f:f32[] = cond[
|
|
branches=(
|
|
{ lambda ; g_:f32[] h:f32[] i:f32[] j:f32[]. let
|
|
k:f32[] = sub j h
|
|
in (k,) }
|
|
{ lambda ; l:f32[] m_:f32[] n:f32[] o:f32[]. let
|
|
p:f32[] = add n l
|
|
in (p,) }
|
|
)
|
|
] e a a c d
|
|
in (f,) }"""
|
|
jaxpr = api.make_jaxpr(f)(jnp.float32(3.))
|
|
self.assertMultiLineStrippedEqual(expected, str(jaxpr))
|
|
|
|
def test_make_jaxpr_static_argnums(self):
|
|
def f(x, y):
|
|
return x + y
|
|
|
|
jaxpr = api.make_jaxpr(f, static_argnums=(1,))(2, 3)
|
|
self.assertIn('3', str(jaxpr))
|
|
|
|
def test_make_jaxpr_return_shape(self):
|
|
_, shape_tree = api.make_jaxpr(lambda x: (x + 1, jnp.zeros(2, jnp.float32)),
|
|
return_shape=True)(jnp.int32(1))
|
|
expected = (api.ShapeDtypeStruct(shape=(), dtype=jnp.int32),
|
|
api.ShapeDtypeStruct(shape=(2,), dtype=jnp.float32))
|
|
self.assertEqual(shape_tree, expected)
|
|
|
|
def test_make_jaxpr_axis_env(self):
|
|
def f(x):
|
|
return x - lax.psum(x, 'i')
|
|
jaxpr = api.make_jaxpr(f, axis_env=[('i', 4)])(2)
|
|
self.assertIn('psum', str(jaxpr))
|
|
|
|
def test_weak_type_jit_invariance(self):
|
|
y = jnp.broadcast_to(3., (3,))
|
|
self.assertTrue(y.aval.weak_type)
|
|
|
|
def f():
|
|
return lax.convert_element_type(y, 'float32')
|
|
|
|
self.assertEqual(f().aval.weak_type, api.jit(f)().aval.weak_type)
|
|
|
|
def test_elide_trivial_convert_element_types(self):
|
|
# since we apply convert_element_type to a numpy.ndarray, the primitive is
|
|
# still bound and thus would appear in the jaxpr if we didn't clean it up
|
|
if config.enable_x64.value:
|
|
x = np.arange(3, dtype='float64')
|
|
else:
|
|
x = np.arange(3, dtype='float32')
|
|
|
|
cet = partial(lax.convert_element_type, new_dtype=x.dtype)
|
|
jaxpr = api.make_jaxpr(lambda: cet(cet(cet(x))))()
|
|
self.assertLen(jaxpr.eqns, 0)
|
|
|
|
def test_elide_trivial_broadcasts(self):
|
|
# since we apply broadcast to a numpy.ndarray, the primitive is still bound
|
|
# and thus would appear in the jaxpr if we didn't clean it up
|
|
jaxpr = api.make_jaxpr(lambda: lax.broadcast(np.float32(3), ()))()
|
|
self.assertLen(jaxpr.jaxpr.eqns, 0)
|
|
|
|
def test_convert_element_type_literal_constant_folding(self):
|
|
# this convert_element_type is nontrivial, but because it's on a scalar we
|
|
# constant-fold it
|
|
cet = partial(lax.convert_element_type, new_dtype='float16')
|
|
jaxpr = api.make_jaxpr(lambda: cet(3.))()
|
|
self.assertLen(jaxpr.eqns, 0)
|
|
|
|
def test_eqn_repr_with_no_lhs(self):
|
|
def f(x):
|
|
jax.debug.print("{}", x)
|
|
return x
|
|
jaxpr = jax.make_jaxpr(f)(np.int32(0))
|
|
self.assertEqual(jaxpr.eqns[0].primitive, debugging.debug_callback_p)
|
|
self.assertStartsWith(str(jaxpr.eqns[0]), "debug_callback[", )
|
|
|
|
|
|
class DCETest(jtu.JaxTestCase):
|
|
|
|
def assert_dce_result(self, jaxpr: core.Jaxpr, used_outputs: list[bool],
|
|
expected_used_inputs: list[bool],
|
|
expected_num_eqns: int | None = None,
|
|
check_diff: bool = True):
|
|
jaxpr_dce, used_inputs = pe.dce_jaxpr(jaxpr, used_outputs)
|
|
core.check_jaxpr(jaxpr_dce)
|
|
self.assertEqual(used_inputs, expected_used_inputs)
|
|
if expected_num_eqns is not None:
|
|
all_jaxprs = it.chain([jaxpr_dce], core.subjaxprs(jaxpr_dce))
|
|
num_eqns = sum(len(subjaxpr.eqns) for subjaxpr in all_jaxprs)
|
|
self.assertEqual(num_eqns, expected_num_eqns, msg=str(jaxpr_dce))
|
|
|
|
rand_ = jtu.rand_small(np.random.RandomState(0))
|
|
rand = lambda v: rand_(v.aval.shape, v.aval.dtype)
|
|
consts = [rand(v) for v in jaxpr.constvars]
|
|
inputs = [rand(v) for v in jaxpr.invars ]
|
|
inputs_dce = [x for x, used in zip(inputs, used_inputs) if used]
|
|
full_outs = core.eval_jaxpr(jaxpr , consts, *inputs)
|
|
expected_outs_dce = [y for y, used in zip(full_outs, used_outputs) if used]
|
|
outs = core.eval_jaxpr(jaxpr_dce, consts, *inputs_dce)
|
|
self.assertAllClose(outs, expected_outs_dce)
|
|
|
|
if check_diff and expected_num_eqns != 0:
|
|
f = lambda *args: core.eval_jaxpr(jaxpr_dce, consts, *args)
|
|
jtu.check_grads(f, inputs_dce, order=2, modes=['rev'])
|
|
|
|
def test_dce_jaxpr_scan_nontrivial_fixedpoint_carry(self):
|
|
# The idea is that each element of the output carry tuple depends on the
|
|
# corresponding carried input as well as the one to the left. The extensive
|
|
# inputs and outputs aren't used here; just the carry depending on itself.
|
|
def f(lst):
|
|
def body(c, _):
|
|
return [c[0]] + [c1 + c2 for c1, c2 in zip(c[:-1], c[1:])], None
|
|
out, _ = jax.lax.scan(body, lst, None, length=len(lst))
|
|
return out
|
|
jaxpr = api.make_jaxpr(f)([1., 2., 3., 4.]).jaxpr
|
|
self.assertLen(jaxpr.eqns, 1)
|
|
self.assertLen(jaxpr.eqns[0].params['jaxpr'].jaxpr.eqns, 3)
|
|
|
|
# If we use all but the last element, all but the first input is used, and
|
|
# only one eqn is pruned.
|
|
self.assert_dce_result(
|
|
jaxpr, used_outputs=[True, True, True, False],
|
|
expected_used_inputs=[True, True, True, False],
|
|
expected_num_eqns=1 + 2) # one outer scan eqn, two adds in the body
|
|
|
|
# Same as above if we just pull on the third element.
|
|
self.assert_dce_result(
|
|
jaxpr, used_outputs=[False, False, True, False],
|
|
expected_used_inputs=[True, True, True, False],
|
|
expected_num_eqns=1 + 2) # one outer scan eqn, two adds in the body
|
|
|
|
# If we use all but the last two elements, the last two inputs are not used,
|
|
# and two eqns can be pruned.
|
|
self.assert_dce_result(
|
|
jaxpr, used_outputs=[True, True, False, False],
|
|
expected_used_inputs=[True, True, False, False],
|
|
expected_num_eqns=1 + 1) # one outer scan eqn, one add in body
|
|
|
|
# If we only use the last element, no eqns can be pruned.
|
|
self.assert_dce_result(
|
|
jaxpr, used_outputs=[False, False, False, True],
|
|
expected_used_inputs=[True, True, True, True],
|
|
expected_num_eqns=1 + 3) # one outer scan eqn, three adds in body
|
|
|
|
def test_dce_jaxpr_scan_nontrivial_fixedpoint_carry_2(self):
|
|
# This is much like the above test, except with a more interesting
|
|
# dependence structure among the carry elements. Also add a const and
|
|
# extensive input.
|
|
hidden_sequence = [1, 2, 3, 5, 8]
|
|
def f(lst):
|
|
def body(c, _):
|
|
_ = jnp.sin(np.array([3., 1., 4.]))
|
|
sub_c = [c[i] for i in hidden_sequence]
|
|
sub_c = [sub_c[0]] + [c1 * c2 for c1, c2 in zip(sub_c[:-1], sub_c[1:])]
|
|
new_c = list(c)
|
|
for i, elt in zip(hidden_sequence, sub_c):
|
|
new_c[i] = elt
|
|
return new_c, None
|
|
out, _ = jax.lax.scan(body, lst, np.arange(len(lst), dtype='float32'))
|
|
return out
|
|
jaxpr = api.make_jaxpr(f)([1., 2., 3., 4., 5., 6., 7., 8., 9., 10.]).jaxpr
|
|
self.assertLen(jaxpr.eqns, 1)
|
|
self.assertLen(jaxpr.eqns[0].params['jaxpr'].jaxpr.eqns, 5)
|
|
|
|
# If we use the value at index 8 only, all the hidden sequence must be kept
|
|
# and no eqns can be pruned.
|
|
used_outputs = [False] * 10
|
|
used_outputs[8] = True
|
|
expected_used_inputs = [False] * 10
|
|
for i in hidden_sequence:
|
|
expected_used_inputs[i] = True
|
|
self.assert_dce_result(
|
|
jaxpr, used_outputs=used_outputs,
|
|
expected_used_inputs=expected_used_inputs,
|
|
expected_num_eqns=1 + 4)
|
|
|
|
# If we use the value at any indices not in the hidden sequence, none of the
|
|
# hidden sequence must be kept and we can prune all body eqns.
|
|
used_outputs = [False] * 10
|
|
expected_used_inputs = [False] * 10
|
|
used_outputs[9] = expected_used_inputs[9] = True
|
|
self.assert_dce_result(
|
|
jaxpr, used_outputs=used_outputs,
|
|
expected_used_inputs=expected_used_inputs,
|
|
expected_num_eqns=1) # 1 b/c scan doesn't have fwding rule
|
|
used_outputs[7] = expected_used_inputs[7] = True
|
|
used_outputs[6] = expected_used_inputs[6] = True
|
|
self.assert_dce_result(
|
|
jaxpr, used_outputs=used_outputs,
|
|
expected_used_inputs=expected_used_inputs,
|
|
expected_num_eqns=1)
|
|
|
|
# If we use the value at index 3 only, some of the hidden sequence must be
|
|
# kept but the rest pruned.
|
|
used_outputs = [False] * 10
|
|
used_outputs[3] = True
|
|
expected_used_inputs = [False] * 10
|
|
expected_used_inputs[1] = expected_used_inputs[2] = \
|
|
expected_used_inputs[3] = True
|
|
self.assert_dce_result(
|
|
jaxpr, used_outputs=used_outputs,
|
|
expected_used_inputs=expected_used_inputs,
|
|
expected_num_eqns=1 + 2)
|
|
|
|
def test_dce_jaxpr_scan_nontrivial_fixedpoint_extensive_output(self):
|
|
# Here we test how using the extensive output affects the carry.
|
|
def f(lst):
|
|
def body(c, _):
|
|
return [c[-1], *c[:-1]], c[-1]
|
|
_, ys = jax.lax.scan(body, lst, None, length=len(lst))
|
|
return ys
|
|
jaxpr = api.make_jaxpr(f)([1., 2., 3., 4.]).jaxpr
|
|
self.assertLen(jaxpr.eqns, 1)
|
|
|
|
# If we only use the extensive output, all carry elements are needed, and we
|
|
# need to keep the scan itself.
|
|
self.assert_dce_result(
|
|
jaxpr, used_outputs=[True],
|
|
expected_used_inputs=[True, True, True, True],
|
|
expected_num_eqns=1)
|
|
|
|
# If we don't use the extensive output, no carry elements are needed, and we
|
|
# don't need to keep the scan.
|
|
self.assert_dce_result(
|
|
jaxpr, used_outputs=[False],
|
|
expected_used_inputs=[False, False, False, False],
|
|
expected_num_eqns=0)
|
|
|
|
def test_dce_jaxpr_scan_extensive_input(self):
|
|
# Here we test an extensive input affecting the carry.
|
|
def cumprod(xs):
|
|
def body(c, x):
|
|
return c * x, c
|
|
c, ys = jax.lax.scan(body, jnp.float32(1.), xs)
|
|
return c, ys
|
|
jaxpr = api.make_jaxpr(cumprod)(jnp.arange(1., 5., dtype='float32')).jaxpr
|
|
|
|
# If we only use the carry output or extensive output, we need the input.
|
|
self.assert_dce_result(
|
|
jaxpr, used_outputs=[True, False],
|
|
expected_used_inputs=[True],
|
|
expected_num_eqns=2)
|
|
self.assert_dce_result(
|
|
jaxpr, used_outputs=[False, True],
|
|
expected_used_inputs=[True],
|
|
expected_num_eqns=2)
|
|
|
|
# If we don't use either output, the scan is eliminated.
|
|
self.assert_dce_result(
|
|
jaxpr, used_outputs=[False, False],
|
|
expected_used_inputs=[False],
|
|
expected_num_eqns=0)
|
|
|
|
def test_dce_jaxpr_scan_overpruning(self):
|
|
# This is a regression test for a specific issue.
|
|
@jax.remat
|
|
def scanned_f(c, x):
|
|
out = jnp.tanh(c * x)
|
|
return out, out
|
|
|
|
def f(xs):
|
|
return lax.scan(scanned_f, jnp.array(1., 'float32'), xs)
|
|
|
|
xs = jnp.arange(10., dtype='float32')
|
|
jaxpr = api.make_jaxpr(lambda xs: api.linearize(f, xs)[1])(xs).jaxpr
|
|
|
|
jaxpr, used_inputs = pe.dce_jaxpr(jaxpr, [True] * len(jaxpr.outvars))
|
|
self.assertLen(jaxpr.eqns, 1)
|
|
self.assertLen(jaxpr.eqns[-1].params['jaxpr'].jaxpr.eqns, 2)
|
|
|
|
def test_dce_jaxpr_scan_const_in_jvp(self):
|
|
# The main point of this test is to check for a crash.
|
|
@jax.custom_jvp
|
|
def f(x):
|
|
return x * np.arange(3.)
|
|
@f.defjvp
|
|
def f_jvp(primals, tangents):
|
|
(x,), (xdot,) = primals, tangents
|
|
return f(x), xdot * np.arange(3.)
|
|
|
|
def g(x):
|
|
def body(c, _):
|
|
return f(c), None
|
|
y, _ = jax.lax.scan(body, x, None, length=1)
|
|
return y
|
|
|
|
jaxpr = api.make_jaxpr(lambda x, xdot: api.jvp(g, (x,), (xdot,))
|
|
)(np.arange(3.), np.arange(3.)).jaxpr
|
|
|
|
self.assert_dce_result(
|
|
jaxpr, used_outputs=[True, True],
|
|
expected_used_inputs=[True, True])
|
|
|
|
self.assert_dce_result(
|
|
jaxpr, used_outputs=[True, False],
|
|
expected_used_inputs=[True, False])
|
|
|
|
def test_dce_jaxpr_scan_results(self):
|
|
# This doesn't test whether DCE is doing nontrivial work; instead it tests
|
|
# whether the result after applying DCE computes different values. If
|
|
# dce_jaxpr were an identity function, it'd pass this test!
|
|
def f(cs, xs):
|
|
def body(c, x):
|
|
return (c[0], c[0] + c[1], jnp.arange(3.)), x
|
|
cs, xs = jax.lax.scan(body, cs, xs)
|
|
return cs[::2], xs[::2]
|
|
|
|
cs = 1., 2., jnp.arange(3.)
|
|
xs = jnp.arange(3.), jnp.arange(3.) + 5
|
|
jaxpr_ = jax.make_jaxpr(f)(cs, xs)
|
|
jaxpr, consts = jaxpr_.jaxpr, jaxpr_.consts
|
|
jaxpr_pruned, used_inputs = pe.dce_jaxpr(jaxpr, [True] * len(jaxpr.outvars))
|
|
|
|
args = (*cs, *xs)
|
|
result1 = core.eval_jaxpr(jaxpr , consts, *cs, *xs)
|
|
pruned_args = [x for x, used in zip(args, used_inputs) if used]
|
|
result2 = core.eval_jaxpr(jaxpr_pruned, consts, *pruned_args)
|
|
self.assertAllClose(result1, result2)
|
|
|
|
def test_dce_jaxpr_cond_trivial(self):
|
|
x = jnp.array(1., dtype='float32')
|
|
|
|
# start with 7 eqns, use both outputs so nothing can be pruned
|
|
def f(x1, x2):
|
|
return lax.cond(x1 > 0,
|
|
lambda x1, x2: (jnp.sin(x1), jnp.sin(x2)),
|
|
lambda x1, x2: (jnp.sin(x1), jnp.sin(x2)),
|
|
x1, x2)
|
|
jaxpr = jax.make_jaxpr(f)(x, x).jaxpr
|
|
self.assert_dce_result(jaxpr, [True, True], [True, True], 7)
|
|
|
|
# use neither output so everything can be pruned
|
|
self.assert_dce_result(jaxpr, [False, False], [False, False], 0)
|
|
|
|
def test_dce_jaxpr_cond_nontrivial(self):
|
|
x = jnp.array(1., dtype='float32')
|
|
|
|
# start with 7 eqns, don't use an output so an eqn can be trimmed on each
|
|
# side and x2 _can_ be pruned
|
|
def f(x1, x2):
|
|
return lax.cond(x1 > 0,
|
|
lambda x1, x2: (jnp.sin(x1), jnp.sin(x2)),
|
|
lambda x1, x2: (jnp.sin(x1), jnp.sin(x1)),
|
|
x1, x2)
|
|
jaxpr = jax.make_jaxpr(f)(x, x).jaxpr
|
|
self.assert_dce_result(jaxpr, [True, False], [True, False], 5)
|
|
|
|
# start with 7 eqns, don't use an output so an eqn can be trimmed on each
|
|
# side, but x2 _can't_ be pruned b/c of a swap
|
|
def f(x1, x2):
|
|
return lax.cond(x1 > 0,
|
|
lambda x1, x2: (jnp.sin(x1), jnp.sin(x2)),
|
|
lambda x1, x2: (jnp.sin(x2), jnp.sin(x1)),
|
|
x1, x2)
|
|
jaxpr = jax.make_jaxpr(f)(x, x).jaxpr
|
|
self.assert_dce_result(jaxpr, [True, False], [True, True], 5)
|
|
|
|
# start with 7 eqns, only use x1 on one side and x2 on the other, so we
|
|
# can't prune any inputs or eqns
|
|
def f(x1, x2):
|
|
return lax.cond(x1 > 0,
|
|
lambda x1, x2: (jnp.sin(x1), jnp.sin(x1)),
|
|
lambda x1, x2: (jnp.sin(x2), jnp.sin(x2)),
|
|
x1, x2)
|
|
jaxpr = jax.make_jaxpr(f)(x, x).jaxpr
|
|
self.assert_dce_result(jaxpr, [True, True], [True, True], 7)
|
|
# use only one output, so we can prune eqns but not inputs
|
|
self.assert_dce_result(jaxpr, [True, False], [True, True], 5)
|
|
|
|
|
|
class CustomJVPTest(jtu.JaxTestCase):
|
|
|
|
def test_basic(self):
|
|
@jax.custom_jvp
|
|
def f(x):
|
|
return jnp.sin(x)
|
|
def f_jvp(primals, tangents):
|
|
x, = primals
|
|
g, = tangents
|
|
return f(x), 2 * jnp.cos(x) * g
|
|
f.defjvp(f_jvp)
|
|
|
|
x = 3.
|
|
self.assertAllClose(f(x), jnp.sin(x))
|
|
self.assertAllClose(api.jvp(f, (x,), (1.,)),
|
|
(jnp.sin(x), 2 * jnp.cos(x)))
|
|
self.assertAllClose(api.grad(f)(x), 2 * jnp.cos(x))
|
|
|
|
def test_invariance(self):
|
|
@jax.custom_jvp
|
|
def f(x):
|
|
return jnp.cos(2 * x) / 2.
|
|
def f_jvp(primals, tangents):
|
|
x, = primals
|
|
g, = tangents
|
|
return (f(x), 3 * g)
|
|
f.defjvp(f_jvp)
|
|
def f2(x):
|
|
y, _ = api.jvp(f, (x,), (x,))
|
|
return y
|
|
def f3(x):
|
|
y, _ = api.jvp(f2, (x,), (x,))
|
|
return y
|
|
x = 1.
|
|
self.assertAllClose(api.jvp(f, (x,), (x,)),
|
|
api.jvp(f2, (x,), (x,)),
|
|
check_dtypes=False)
|
|
self.assertAllClose(api.jvp(f, (x,), (x,)),
|
|
api.jvp(f3, (x,), (x,)),
|
|
check_dtypes=False)
|
|
|
|
def test_python_control_flow(self):
|
|
@jax.custom_jvp
|
|
def f(x):
|
|
if x > 0:
|
|
return jnp.sin(x)
|
|
else:
|
|
return jnp.cos(x)
|
|
def f_jvp(primals, tangents):
|
|
x, = primals
|
|
g, = tangents
|
|
if x > 0:
|
|
return f(x), 2 * g
|
|
else:
|
|
return f(x), 3 * g
|
|
f.defjvp(f_jvp)
|
|
x = 2.
|
|
self.assertAllClose(f(x), jnp.sin(x))
|
|
self.assertAllClose(f(-x), jnp.cos(-x))
|
|
self.assertAllClose(api.jvp(f, (x,), (1.,)),
|
|
(jnp.sin(x), 2.),
|
|
check_dtypes=False)
|
|
self.assertAllClose(api.jvp(f, (-x,), (1.,)),
|
|
(jnp.cos(-x), 3.),
|
|
check_dtypes=False)
|
|
self.assertAllClose(api.grad(f)(x), 2., check_dtypes=False)
|
|
self.assertAllClose(api.grad(f)(-x), 3., check_dtypes=False)
|
|
|
|
def test_vmap(self):
|
|
@jax.custom_jvp
|
|
def f(x):
|
|
assert jnp.ndim(x) == 0
|
|
return jnp.sin(x)
|
|
def f_jvp(primals, tangents):
|
|
x, = primals
|
|
g, = tangents
|
|
assert jnp.ndim(x) == jnp.ndim(g) == 0
|
|
return f(x), 2 * jnp.cos(x) * g
|
|
f.defjvp(f_jvp)
|
|
|
|
x = jnp.arange(3.)
|
|
xx = jnp.arange(6.).reshape(2, 3)
|
|
|
|
# vmap of f
|
|
self.assertAllClose(api.vmap(f)(x), jnp.sin(x))
|
|
self.assertAllClose(api.vmap(api.vmap(f))(xx), jnp.sin(xx))
|
|
|
|
# vmap of jvp of f
|
|
self.assertAllClose(api.vmap(lambda x: api.jvp(f, (x,), (x,)))(x),
|
|
(jnp.sin(x), 2 * jnp.cos(x) * x))
|
|
self.assertAllClose(api.vmap(api.vmap(lambda x: api.jvp(f, (x,), (x,))))(xx),
|
|
(jnp.sin(xx), 2 * jnp.cos(xx) * xx))
|
|
|
|
# jvp of vmap of f
|
|
self.assertAllClose(api.jvp(api.vmap(f), (x,), (x,)),
|
|
(jnp.sin(x), 2 * jnp.cos(x) * x))
|
|
self.assertAllClose(api.jvp(api.vmap(api.vmap(f)), (xx,), (xx,)),
|
|
(jnp.sin(xx), 2 * jnp.cos(xx) * xx))
|
|
|
|
# vmap of jvp of vmap of f
|
|
self.assertAllClose(api.vmap(lambda x: api.jvp(api.vmap(f), (x,), (x,)))(xx),
|
|
(jnp.sin(xx), 2 * jnp.cos(xx) * xx))
|
|
|
|
def test_jit(self):
|
|
@jax.custom_jvp
|
|
def f(x):
|
|
return jnp.sin(x)
|
|
def f_jvp(primals, tangents):
|
|
x, = primals
|
|
g, = tangents
|
|
return f(x), 2 * jnp.cos(x) * g
|
|
f.defjvp(f_jvp)
|
|
|
|
x = 3.
|
|
|
|
# jit
|
|
self.assertAllClose(api.jit(f)(x), jnp.sin(x))
|
|
self.assertAllClose(api.jit(api.jit(f))(x), jnp.sin(x))
|
|
|
|
# jit of jvp
|
|
self.assertAllClose(api.jit(lambda x: api.jvp(f, (x,), (x,)))(x),
|
|
(jnp.sin(x), 2 * jnp.cos(x) * x),
|
|
check_dtypes=False)
|
|
|
|
# jvp of jit
|
|
self.assertAllClose(api.jvp(api.jit(f), (x,), (x,)),
|
|
(jnp.sin(x), 2 * jnp.cos(x) * x),
|
|
check_dtypes=False)
|
|
|
|
def test_pytrees(self):
|
|
@jax.custom_jvp
|
|
def f(x):
|
|
return {'b': jnp.sin(x['a'])}
|
|
def f_jvp(primals, tangents):
|
|
x, = primals
|
|
g, = tangents
|
|
return f(x), {'b': 2 * jnp.cos(x['a']) * g['a']}
|
|
f.defjvp(f_jvp)
|
|
x = {'a': 3.}
|
|
self.assertAllClose(f(x)['b'], jnp.sin(x['a']))
|
|
self.assertAllClose(api.jvp(f, (x,), (x,)),
|
|
({'b': jnp.sin(x['a'])},
|
|
{'b': 2 * jnp.cos(x['a']) * x['a']}),
|
|
check_dtypes=False)
|
|
|
|
def test_kwargs(self):
|
|
# from https://github.com/jax-ml/jax/issues/1938
|
|
@jax.custom_jvp
|
|
def my_fun(x, y, c=1.):
|
|
return c * (x + y)
|
|
def my_jvp(primals, tangents):
|
|
x, y, c = primals
|
|
t_x, t_y, t_c = tangents
|
|
return my_fun(x, y, c), t_c
|
|
my_fun.defjvp(my_jvp)
|
|
f = lambda x, y: jnp.square(my_fun(x, y, c=2.)).sum()
|
|
f(10., 5.) # doesn't crash
|
|
api.jvp(f, (10., 5.), (1., 1.)) # doesn't crash
|
|
|
|
def test_initial_style(self):
|
|
@jax.custom_jvp
|
|
def f(x):
|
|
return 3 * x
|
|
def f_jvp(primals, tangents):
|
|
x, = primals
|
|
g, = tangents
|
|
return f(x), 2 * g
|
|
f.defjvp(f_jvp)
|
|
|
|
def foo(x):
|
|
out, _ = lax.scan(lambda c, _: (f(c), None), x, None, length=1)
|
|
return out
|
|
|
|
ans = api.grad(foo)(3.)
|
|
expected = 2.
|
|
self.assertAllClose(ans, expected, check_dtypes=False)
|
|
|
|
ans = api.grad(api.jit(foo))(3.)
|
|
expected = 2.
|
|
self.assertAllClose(ans, expected, check_dtypes=False)
|
|
|
|
ans = api.jit(api.grad(foo))(3.)
|
|
expected = 2.
|
|
self.assertAllClose(ans, expected, check_dtypes=False)
|
|
|
|
ans = api.grad(api.grad(foo))(3.)
|
|
expected = 0.
|
|
self.assertAllClose(ans, expected, check_dtypes=False)
|
|
|
|
ans = api.grad(api.grad(api.jit(foo)))(3.)
|
|
expected = 0.
|
|
self.assertAllClose(ans, expected, check_dtypes=False)
|
|
|
|
ans = api.grad(api.jit(api.grad(foo)))(3.)
|
|
expected = 0.
|
|
self.assertAllClose(ans, expected, check_dtypes=False)
|
|
|
|
ans = api.jit(api.grad(api.grad(foo)))(3.)
|
|
expected = 0.
|
|
self.assertAllClose(ans, expected, check_dtypes=False)
|
|
|
|
def test_initial_style_vmap(self):
|
|
@jax.custom_jvp
|
|
def f(x):
|
|
assert jnp.ndim(x) == 0
|
|
return 3 * x
|
|
def f_jvp(primals, tangents):
|
|
x, = primals
|
|
g, = tangents
|
|
return f(x), 2 * g
|
|
f.defjvp(f_jvp)
|
|
|
|
def foo(x):
|
|
out, _ = lax.scan(lambda c, _: (f(c), None), x, None, length=1)
|
|
return out
|
|
|
|
ans = api.vmap(foo)(jnp.ones(3))
|
|
expected = 3. * jnp.ones(3)
|
|
self.assertAllClose(ans, expected, check_dtypes=False)
|
|
|
|
ans = api.vmap(api.jit(foo))(jnp.ones(3))
|
|
expected = 3. * jnp.ones(3)
|
|
self.assertAllClose(ans, expected, check_dtypes=False)
|
|
|
|
ans = api.jit(api.vmap(foo))(jnp.ones(3))
|
|
expected = 3. * jnp.ones(3)
|
|
self.assertAllClose(ans, expected, check_dtypes=False)
|
|
|
|
ans = api.grad(lambda x: api.vmap(foo)(x).sum())(jnp.ones(3))
|
|
expected = 2. * jnp.ones(3)
|
|
self.assertAllClose(ans, expected, check_dtypes=False)
|
|
|
|
ans = api.grad(lambda x: api.vmap(api.jit(foo))(x).sum())(jnp.ones(3))
|
|
expected = 2. * jnp.ones(3)
|
|
self.assertAllClose(ans, expected, check_dtypes=False)
|
|
|
|
ans = api.grad(lambda x: api.jit(api.vmap(foo))(x).sum())(jnp.ones(3))
|
|
expected = 2. * jnp.ones(3)
|
|
self.assertAllClose(ans, expected, check_dtypes=False)
|
|
|
|
ans = api.grad(api.jit(lambda x: api.vmap(foo)(x).sum()))(jnp.ones(3))
|
|
expected = 2. * jnp.ones(3)
|
|
self.assertAllClose(ans, expected, check_dtypes=False)
|
|
|
|
ans = api.jit(api.grad(lambda x: api.vmap(foo)(x).sum()))(jnp.ones(3))
|
|
expected = 2. * jnp.ones(3)
|
|
self.assertAllClose(ans, expected, check_dtypes=False)
|
|
|
|
def test_initial_style_vmap_with_collective(self):
|
|
|
|
@jax.custom_jvp
|
|
def f(x):
|
|
return lax.psum(x, 'foo')
|
|
|
|
@f.defjvp
|
|
def f_jvp(xs, ts):
|
|
x, = xs
|
|
t, = ts
|
|
return lax.psum(x, 'foo'), t
|
|
|
|
def g(x):
|
|
jaxpr = api.make_jaxpr(f)(x)
|
|
return core.eval_jaxpr(jaxpr.jaxpr, [], x)[0]
|
|
|
|
v = api.vmap(lambda _, x: g(x), axis_name='foo', in_axes=(0, None),
|
|
out_axes=None)(jnp.arange(4.), 2.)
|
|
self.assertAllClose(v, 8.)
|
|
|
|
def test_closed_over_tracers_error_message(self):
|
|
def f(x):
|
|
@jax.custom_jvp
|
|
def g(y):
|
|
return x + y
|
|
def g_jvp(primals, tangents):
|
|
return g(x), 2 * primals[0]
|
|
g.defjvp(g_jvp)
|
|
return g(1.)
|
|
|
|
self.assertRaises(UnexpectedTracerError, lambda: api.jvp(f, (3.,), (1.,)))
|
|
self.assertRaises(UnexpectedTracerError, lambda: api.grad(f)(3.))
|
|
|
|
def test_nondiff_arg(self):
|
|
@partial(jax.custom_jvp, nondiff_argnums=(0,))
|
|
def app(f, x):
|
|
return f(x)
|
|
def app_jvp(f, primals, tangents):
|
|
(x,), (t,) = primals, tangents
|
|
return app(f, x), 3 * t
|
|
app.defjvp(app_jvp)
|
|
|
|
ans = app(lambda x: 2 * x, 1)
|
|
expected = 2
|
|
self.assertAllClose(ans, expected, check_dtypes=False)
|
|
|
|
ans = api.jvp(lambda x: app(lambda y: 2 * y, x), (1.,), (1.,))
|
|
expected = (2., 3.)
|
|
self.assertAllClose(ans, expected, check_dtypes=False)
|
|
|
|
def test_nondiff_arg_jit_tracer(self):
|
|
# This test would pass with "final-style" JIT tracing, but that was
|
|
# misleading: it doesn't work with "initial-style" staging, i.e. control
|
|
# flow primitives like jax.lax.scan or even pjit. The behavior isn't very
|
|
# useful either: instead of using nondiff_argnums here, a user can just pass
|
|
# such inputs as ordinary arguments, and ignore the corresponding tangents.
|
|
# Then nondiff_argnums can be reserved for (1) non jaxtype data (like a
|
|
# string- or callable-valued argument which parameterizes the function or
|
|
# rule) or (2) static data (e.g. integers which parameterize shapes).
|
|
raise unittest.SkipTest("behavior no longer supported")
|
|
|
|
@partial(jax.custom_jvp, nondiff_argnums=(0,))
|
|
def f(x, y):
|
|
return x * y
|
|
def f_jvp(x, primals, tangents):
|
|
(y,), (t_y,) = primals, tangents
|
|
return f(x, y), 5 * t_y
|
|
f.defjvp(f_jvp)
|
|
|
|
@jit
|
|
def g(x, y):
|
|
return f(x, y)
|
|
|
|
ans = api.jvp(lambda y: g(2., y), (3.,), (1.,))
|
|
expected = (6., 5.)
|
|
self.assertAllClose(ans, expected, check_dtypes=False)
|
|
|
|
def test_nondiff_arg_vmap_tracer(self):
|
|
@partial(jax.custom_jvp, nondiff_argnums=(0,))
|
|
def f(x, y):
|
|
return x * y
|
|
def f_jvp(x, primals, tangents):
|
|
(y,), (t_y,) = primals, tangents
|
|
return f(x, y), 5 * t_y
|
|
f.defjvp(f_jvp)
|
|
|
|
g = jax.vmap(f)
|
|
|
|
ans = api.jvp(lambda y: g(jnp.array([2.]), y),
|
|
(jnp.array([3.]),), (jnp.array([1.]),))
|
|
expected = (jnp.array([6.]), jnp.array([5.]))
|
|
self.assertAllClose(ans, expected, check_dtypes=False)
|
|
|
|
def test_nondiff_arg_hiding_jvp_tracer(self):
|
|
def f(x):
|
|
@partial(jax.custom_jvp, nondiff_argnums=(0,))
|
|
def g(h, x):
|
|
return h(x)
|
|
@g.defjvp
|
|
def g_jvp(h, primals, tangents):
|
|
x, = primals
|
|
t, = tangents
|
|
return g(h, x), 2. * t
|
|
h = lambda y: x + y # capture x
|
|
return g(h, x)
|
|
|
|
with self.assertRaises(UnexpectedTracerError):
|
|
api.jvp(f, (2.,), (1.,))
|
|
|
|
def test_vmap_axes(self):
|
|
raise unittest.SkipTest("TODO") # TODO(mattjj): write test
|
|
|
|
def test_pmap(self):
|
|
raise unittest.SkipTest("TODO") # TODO(mattjj): write test
|
|
|
|
def test_missing_jvp_rule_error_message(self):
|
|
@jax.custom_jvp
|
|
def foo(x):
|
|
return x ** 2
|
|
|
|
self.assertRaisesRegex(
|
|
AttributeError,
|
|
r"No JVP defined for custom_jvp function foo using defjvp.",
|
|
lambda: foo(2))
|
|
self.assertRaisesRegex(
|
|
AttributeError,
|
|
r"No JVP defined for custom_jvp function foo using defjvp.",
|
|
lambda: api.jvp(foo, (2.,), (1.,)))
|
|
self.assertRaisesRegex(
|
|
AttributeError,
|
|
r"No JVP defined for custom_jvp function foo using defjvp.",
|
|
lambda: api.grad(foo)(2.))
|
|
|
|
def test_jvp_rule_inconsistent_pytree_structures_error_message(self):
|
|
@jax.custom_jvp
|
|
def f(x):
|
|
return (x**2,)
|
|
|
|
@f.defjvp
|
|
def foo_jvp(primals, tangents):
|
|
x, = primals
|
|
t, = tangents
|
|
return f(x), [2 * x * t, x]
|
|
|
|
f(2.) # doesn't crash
|
|
self.assertRaisesRegex(
|
|
TypeError,
|
|
re.escape(
|
|
"Custom JVP rule foo_jvp for function f "
|
|
"must produce primal and tangent outputs "
|
|
"with equal container (pytree) structures, but got "
|
|
"{} and {} respectively.".format(
|
|
jax.tree.structure((1,)),
|
|
jax.tree.structure([1, 2]))
|
|
),
|
|
lambda: api.jvp(f, (2.,), (1.,)))
|
|
|
|
def test_primal_tangent_aval_disagreement_error_message(self):
|
|
@jax.custom_jvp
|
|
def f(x):
|
|
return x ** 2
|
|
|
|
@f.defjvp
|
|
def foo_jvp(primals, tangents):
|
|
x, = primals
|
|
t, = tangents
|
|
return f(x), jnp.reshape(t, (1,))
|
|
|
|
f(2.) # doesn't crash
|
|
self.assertRaisesRegex(
|
|
TypeError,
|
|
re.escape(
|
|
"Custom JVP rule must produce primal and tangent outputs "
|
|
"with corresponding shapes and dtypes. "
|
|
"Expected float32[] (tangent type of float32[]) but got float32[1]."),
|
|
lambda: api.jvp(f, (jnp.float32(2.),), (jnp.float32(1.),)))
|
|
|
|
|
|
def test_jvp_rule_doesnt_return_pair_error_message(self):
|
|
# https://github.com/jax-ml/jax/issues/2516
|
|
|
|
@jax.custom_jvp
|
|
def f(x):
|
|
return x ** 2
|
|
|
|
@f.defjvp
|
|
def foo_jvp(primals, tangents):
|
|
x, = primals
|
|
t, = tangents
|
|
return t
|
|
|
|
f(2.) # doesn't crash
|
|
self.assertRaisesRegex(
|
|
TypeError,
|
|
re.escape(
|
|
"Custom JVP rule foo_jvp for function f "
|
|
"must produce a pair (list or tuple of length two) "
|
|
"representing primal and tangent outputs, but got 1.0"),
|
|
lambda: api.jvp(f, (2.,), (1.,)))
|
|
|
|
def test_jvp_rule_primal_out_type_doesnt_match_primal_error_message(self):
|
|
# https://github.com/lucidrains/flash-attention-jax/issues/7
|
|
|
|
def scan_apply(f, x):
|
|
y, _ = jax.lax.scan(lambda x, _: (f(x), None), x, None, length=1)
|
|
return y
|
|
|
|
@jax.custom_jvp
|
|
def f(x):
|
|
return x
|
|
|
|
@f.defjvp
|
|
def f_jvp(primals, tangents):
|
|
(x,), (xdot,) = primals, tangents
|
|
return (x, x), (xdot, xdot)
|
|
|
|
x = jnp.float32(1.)
|
|
self.assertRaisesRegex(
|
|
TypeError,
|
|
re.escape(
|
|
"Custom JVP rule f_jvp for function f must produce a pair "
|
|
"(list or tuple of length two) where the first element represents "
|
|
"the primal output (equal in value to the output of the "
|
|
"custom_jvp-decorated function f, and in particular of the "
|
|
"same container/pytree structure), but instead the JVP rule "
|
|
"output's first element had container/pytree structure:\n"
|
|
" (float32[], float32[])\n"
|
|
"while the custom_jvp-decorated function f had output "
|
|
"container/pytree structure:\n"
|
|
" float32[]."
|
|
),
|
|
lambda: jax.jvp(lambda x: scan_apply(f, x), (x,), (x,)))
|
|
|
|
@f.defjvp
|
|
def f_jvp2(primals, tangents):
|
|
(x,), (xdot,) = primals, tangents
|
|
return jnp.zeros((3, *x.shape), x.dtype), xdot
|
|
|
|
self.assertRaisesRegex(
|
|
TypeError,
|
|
re.escape(
|
|
"Custom JVP rule f_jvp2 for function f must produce a pair "
|
|
"(list or tuple of length two) where the first element represents "
|
|
"the primal output (equal in value to the output of the "
|
|
"custom_jvp-decorated function f, and in particular "
|
|
"with leaves of the same shape/dtype), but instead the JVP rule "
|
|
"output's first element had shapes/dtypes of:\n"
|
|
" float32[3]\n"
|
|
"while the custom_jvp-decorated function f had output shapes/dtypes"
|
|
" of:\n"
|
|
" float32[]"
|
|
),
|
|
lambda: jax.jvp(lambda x: scan_apply(f, x), (x,), (x,)))
|
|
|
|
def test_multiple_rule_invocations(self):
|
|
@jax.custom_jvp
|
|
def expit(x):
|
|
return 1 / (1 + lax.exp(-x))
|
|
|
|
@expit.defjvp
|
|
def _expit_jvp(primals, tangents):
|
|
(x,), (t,) = primals, tangents
|
|
ans = expit(x)
|
|
t_out = t * ans * (1 - ans)
|
|
return ans, t_out
|
|
|
|
def scanned_fun(c, _):
|
|
return [expit(c[0])] + [c[i-1] + c[i] for i in range(1, len(c))], None
|
|
|
|
def foo(x):
|
|
zero = jnp.zeros_like(x)
|
|
c, _ = lax.scan(scanned_fun, [x, zero, zero, zero, zero], None, length=10)
|
|
return c[-1]
|
|
|
|
# just make sure these don't crash
|
|
foo(3.)
|
|
grad(foo)(3.)
|
|
grad(lambda x: jax.vmap(foo)(x).sum())(jnp.arange(3.))
|
|
|
|
def test_hard_stuff(self):
|
|
arr = jnp.ones((5, 2, 2))
|
|
api.jit(jax.vmap(jnp.linalg.det))(arr) # doesn't crash
|
|
|
|
def test_hard_stuff2(self):
|
|
@jax.custom_jvp
|
|
def f(x):
|
|
return np.zeros(x.shape, x.dtype)
|
|
|
|
@f.defjvp
|
|
def f_jvp(primals, tangents):
|
|
x, = primals
|
|
t, = tangents
|
|
return f(x), t
|
|
|
|
# don't crash
|
|
jax.jit(jax.vmap(f))(jnp.arange(3.))
|
|
jax.jit(jax.vmap(jax.grad(f)))(jnp.arange(3.))
|
|
jax.jit(jax.grad(lambda x: jax.vmap(f)(x).sum()))(jnp.arange(3.))
|
|
jax.grad(lambda x: jax.vmap(f)(x).sum())(jnp.arange(3.))
|
|
jax.jvp(jax.vmap(f), (jnp.arange(3.),), (jnp.ones(3),))
|
|
|
|
def test_hard_stuff3(self):
|
|
@jax.custom_jvp
|
|
def relu(x):
|
|
return jnp.maximum(x, 0)
|
|
|
|
@relu.defjvp
|
|
def _relu_jvp(primals, tangents):
|
|
x, = primals
|
|
t, = tangents
|
|
return relu(x), lax.select(x > 0, t, lax.full_like(t, 0))
|
|
|
|
def scanned_fun(c, _):
|
|
return [relu(c[0])] + [c[i-1] + c[i] for i in range(1, len(c))], None
|
|
|
|
def f(x):
|
|
zero = jnp.zeros_like(x)
|
|
c, _ = lax.scan(scanned_fun, [x, zero, zero, zero, zero], None, length=10)
|
|
return c[-1]
|
|
|
|
# don't crash
|
|
jax.jit(jax.vmap(f))(jnp.arange(3.))
|
|
jax.jit(jax.vmap(jax.grad(f)))(jnp.arange(3.))
|
|
jax.jit(jax.grad(lambda x: jax.vmap(f)(x).sum()))(jnp.arange(3.))
|
|
jax.grad(lambda x: jax.vmap(f)(x).sum())(jnp.arange(3.))
|
|
jax.jvp(jax.jit(jax.vmap(f)), (jnp.arange(3.),), (jnp.ones(3),))
|
|
|
|
def test_eval_shape(self):
|
|
@jax.custom_jvp
|
|
def expit(x):
|
|
return 1 / (1 + lax.exp(-x))
|
|
|
|
@expit.defjvp
|
|
def _expit_jvp(primals, tangents):
|
|
(x,), (t,) = primals, tangents
|
|
ans = expit(x)
|
|
t_out = t * ans * (1 - ans)
|
|
return ans, t_out
|
|
|
|
# don't crash
|
|
api.eval_shape(expit, jnp.ones((2, 3)))
|
|
api.eval_shape(api.grad(lambda x: expit(x).sum()), jnp.ones((2, 3)))
|
|
|
|
def test_jaxpr_zeros(self):
|
|
# from https://github.com/jax-ml/jax/issues/2657
|
|
@jax.custom_jvp
|
|
def f(A, b):
|
|
return A @ b
|
|
|
|
def f_jvp(primals, tangents):
|
|
A, b = primals
|
|
dA, db = tangents
|
|
z = f(A, b)
|
|
dz = A @ db + dA @ b
|
|
return z, dz
|
|
|
|
f.defjvp(f_jvp)
|
|
|
|
def experiment(theta):
|
|
def step(q, _):
|
|
z = f(jnp.eye(3), jnp.ones(3) * theta)
|
|
q += z[0]
|
|
return q, q
|
|
|
|
q = 0.
|
|
q, _ = lax.scan(step, q, None, 4)
|
|
return q
|
|
|
|
grad(experiment)(1.) # doesn't crash
|
|
|
|
def test_linear_in_scan(self):
|
|
@jax.custom_jvp
|
|
def f(x):
|
|
return -x
|
|
|
|
@f.defjvp
|
|
def f_jvp(primals, tangents):
|
|
x, = primals
|
|
x_dot, = tangents
|
|
return f(x), f(x_dot)
|
|
|
|
def foo(x):
|
|
out, _ = lax.scan(lambda c, _: (f(c), None), x, None, length=1)
|
|
return out
|
|
|
|
ans = api.grad(foo)(3.)
|
|
expected = -1.
|
|
self.assertAllClose(ans, expected, check_dtypes=False)
|
|
|
|
def test_custom_jvps_first_rule_is_none(self):
|
|
# https://github.com/jax-ml/jax/issues/3389
|
|
@jax.custom_jvp
|
|
def f(x, y):
|
|
return x ** 2 * y
|
|
|
|
f.defjvps(None, lambda x_dot, primal_out, x, y: 2 * x * y * x_dot)
|
|
ans = grad(f, 1)(2., 3.) # doesn't crash
|
|
expected = 12.
|
|
self.assertAllClose(ans, expected, check_dtypes=False)
|
|
|
|
def test_concurrent_initial_style(self):
|
|
# https://github.com/jax-ml/jax/issues/3843
|
|
def unroll(param, sequence):
|
|
def scan_f(prev_state, inputs):
|
|
return prev_state, jax.nn.sigmoid(param * inputs)
|
|
return jnp.sum(jax.lax.scan(scan_f, None, sequence)[1])
|
|
|
|
def run():
|
|
return jax.grad(unroll)(jnp.array(1.0), jnp.array([1.0]))
|
|
|
|
expected = run()
|
|
|
|
# we just don't want this to crash
|
|
n_workers = 2
|
|
with concurrent.futures.ThreadPoolExecutor(max_workers=n_workers) as e:
|
|
futures = []
|
|
for _ in range(n_workers):
|
|
futures.append(e.submit(run))
|
|
results = [f.result() for f in futures]
|
|
for ans in results:
|
|
self.assertAllClose(ans, expected)
|
|
|
|
def test_nondiff_argnums_vmap_tracer(self):
|
|
# https://github.com/jax-ml/jax/issues/3964
|
|
@partial(jax.custom_jvp, nondiff_argnums=(0, 2))
|
|
def sample(shape, param, seed):
|
|
return jax.random.uniform(key=seed, shape=shape, minval=param)
|
|
|
|
@sample.defjvp
|
|
def sample_jvp(shape, seed, primals, tangents):
|
|
param, = primals
|
|
dparam, = tangents
|
|
dparam = jnp.broadcast_to(dparam, shape)
|
|
samples = sample(shape, param, seed)
|
|
return samples, samples * dparam # dummy jvp for proof of concept
|
|
|
|
# check these don't crash
|
|
jax.vmap(lambda seed: sample((2,3), 1., seed))(
|
|
jax.random.split(jax.random.key(1), 10))
|
|
jax.jvp(lambda x: sample((2, 3), x, jax.random.key(1)),
|
|
(1.,), (1.,))
|
|
|
|
def test_fun_with_nested_calls_2(self):
|
|
def call(f, *args):
|
|
f = jax.custom_jvp(f)
|
|
f.defjvp(lambda primals, tangents: (f(*primals), sum(tangents)))
|
|
return f(*args)
|
|
|
|
def fun_with_nested_calls_2(x):
|
|
def bar(y):
|
|
def baz(w):
|
|
q = call(lambda x: y, x)
|
|
q = q + call(lambda: y)
|
|
q = q + call(lambda y: w + y, y)
|
|
q = call(lambda w: call(jnp.sin, x) * y, 1.0) + q
|
|
return q
|
|
return api.jit(baz)(x)
|
|
return call(bar, x)
|
|
|
|
# test these don't crash
|
|
self.assertAllClose(api.jit(fun_with_nested_calls_2)(3.),
|
|
fun_with_nested_calls_2(3.))
|
|
api.vmap(fun_with_nested_calls_2)(jnp.arange(3.))
|
|
|
|
def test_closure_with_vmap(self):
|
|
# https://github.com/jax-ml/jax/issues/3822
|
|
alpha = np.float32(2.)
|
|
|
|
def sample(seed):
|
|
@jax.custom_jvp
|
|
def f(alpha):
|
|
return jax.random.gamma(seed, alpha, shape=[])
|
|
|
|
@f.defjvp
|
|
def f_jvp(primal, tangent):
|
|
alpha = primal
|
|
dalpha = tangent
|
|
sample = f(alpha)
|
|
partial_alpha = lax.random_gamma_grad(alpha, sample)
|
|
return sample, partial_alpha * dalpha
|
|
return f(alpha)
|
|
|
|
api.vmap(sample)(jax.random.split(jax.random.key(1), 3)) # don't crash
|
|
|
|
def test_closure_with_vmap2(self):
|
|
# https://github.com/jax-ml/jax/issues/8783
|
|
def h(z):
|
|
def f(x):
|
|
@jax.custom_jvp
|
|
def g(y):
|
|
return x * y
|
|
|
|
# NOTE: rule closes over vmap tracer
|
|
@g.defjvp
|
|
def g_jvp(primals, tangents):
|
|
(y,), (ydot,) = primals, tangents
|
|
return x * y, x * ydot
|
|
|
|
return g(z) # NOTE: no vmapped arg
|
|
|
|
return jax.vmap(f)(jnp.arange(3., dtype='float32'))
|
|
|
|
primals, tangents = jax.jvp(h, (jnp.float32(1.),), (jnp.float32(2.),))
|
|
self.assertAllClose(primals , jnp.arange(3., dtype='float32'))
|
|
self.assertAllClose(tangents, 2 * jnp.arange(3., dtype='float32'))
|
|
|
|
def test_float0(self):
|
|
scalar_float0 = jnp.zeros((), dtype=float0)
|
|
@jax.custom_jvp
|
|
def f(x, y):
|
|
return x, y
|
|
def f_jvp(primals, _):
|
|
x, y = primals
|
|
return (x, y), (2., custom_derivatives_public.zero_from_primal(y))
|
|
f.defjvp(f_jvp)
|
|
|
|
primals = (2., 3)
|
|
tangents = (np.ones(()), scalar_float0)
|
|
expected_tangents = (2., scalar_float0)
|
|
self.assertAllClose(api.jvp(f, primals, tangents),
|
|
(primals, expected_tangents))
|
|
|
|
def test_float0_initial_style(self):
|
|
scalar_float0 = jnp.zeros((), dtype=float0)
|
|
@jax.custom_jvp
|
|
def f(x, y):
|
|
return x, y
|
|
def f_jvp(primals, _):
|
|
x, y = primals
|
|
return (x, y), (2., custom_derivatives_public.zero_from_primal(y))
|
|
f.defjvp(f_jvp)
|
|
|
|
def foo(x, y):
|
|
out, _ = lax.scan(lambda c, _: (f(*c), None), (x, y), None, length=1)
|
|
return out
|
|
|
|
primals = (2., 3)
|
|
tangents = (np.ones(()), scalar_float0)
|
|
expected_tangents = (2., scalar_float0)
|
|
|
|
self.assertAllClose(api.jvp(foo, primals, tangents),
|
|
(primals, expected_tangents))
|
|
|
|
def test_remat(self):
|
|
@jax.custom_jvp
|
|
def f(x):
|
|
return jnp.sin(x)
|
|
def f_jvp(primals, tangents):
|
|
x, = primals
|
|
g, = tangents
|
|
return f(x), 2 * jnp.cos(x) * g
|
|
f.defjvp(f_jvp)
|
|
|
|
@jax.remat
|
|
def g(x):
|
|
return f(f(x))
|
|
|
|
ans = g(2.)
|
|
expected = np.sin(np.sin(2.))
|
|
self.assertAllClose(ans, expected, check_dtypes=False)
|
|
|
|
ans = api.grad(g)(2.)
|
|
expected = 4. * api.grad(lambda x: jnp.sin(jnp.sin(x)))(2.)
|
|
self.assertAllClose(ans, expected, check_dtypes=False)
|
|
|
|
def test_remat_higher_order(self):
|
|
@jax.custom_jvp
|
|
def f(x):
|
|
return jnp.sin(x)
|
|
def f_jvp(primals, tangents):
|
|
x, = primals
|
|
g, = tangents
|
|
return f(x), 2 * jnp.cos(x) * g
|
|
f.defjvp(f_jvp)
|
|
|
|
def g(x):
|
|
return f(f(x))
|
|
|
|
ans = api.grad(api.grad(new_checkpoint(g)))(2.)
|
|
expected = api.grad(api.grad(g))(2.)
|
|
self.assertAllClose(ans, expected, check_dtypes=False)
|
|
|
|
ans = api.grad(new_checkpoint(api.grad(g)))(2.)
|
|
expected = api.grad(api.grad(g))(2.)
|
|
self.assertAllClose(ans, expected, check_dtypes=False)
|
|
|
|
ans = api.grad(api.grad(api.grad(new_checkpoint(g))))(2.)
|
|
expected = api.grad(api.grad(api.grad(g)))(2.)
|
|
self.assertAllClose(ans, expected, check_dtypes=False)
|
|
|
|
def test_initial_style_vmap_2(self):
|
|
# This is like test_initial_style_vmap except the primal function closes
|
|
# over an array constant.
|
|
y = jnp.arange(1., 4.)
|
|
|
|
@jax.custom_jvp
|
|
def f(x):
|
|
assert jnp.ndim(x) == 0
|
|
return 3 * x * jnp.sum(y)
|
|
def f_jvp(primals, tangents):
|
|
x, = primals
|
|
g, = tangents
|
|
return f(x), 2 * g
|
|
f.defjvp(f_jvp)
|
|
|
|
def foo(x):
|
|
out, _ = lax.scan(lambda c, _: (f(c), None), x, None, length=1)
|
|
return out
|
|
|
|
ans = api.grad(lambda x: api.vmap(foo)(x).sum())(jnp.ones(3))
|
|
expected = 2. * jnp.ones(3)
|
|
self.assertAllClose(ans, expected, check_dtypes=False)
|
|
|
|
ans = api.grad(lambda x: api.vmap(api.jit(foo))(x).sum())(jnp.ones(3))
|
|
expected = 2. * jnp.ones(3)
|
|
self.assertAllClose(ans, expected, check_dtypes=False)
|
|
|
|
ans = api.grad(lambda x: api.jit(api.vmap(foo))(x).sum())(jnp.ones(3))
|
|
expected = 2. * jnp.ones(3)
|
|
self.assertAllClose(ans, expected, check_dtypes=False)
|
|
|
|
ans = api.grad(api.jit(lambda x: api.vmap(foo)(x).sum()))(jnp.ones(3))
|
|
expected = 2. * jnp.ones(3)
|
|
self.assertAllClose(ans, expected, check_dtypes=False)
|
|
|
|
ans = api.jit(api.grad(lambda x: api.vmap(foo)(x).sum()))(jnp.ones(3))
|
|
expected = 2. * jnp.ones(3)
|
|
self.assertAllClose(ans, expected, check_dtypes=False)
|
|
|
|
def test_custom_jvp_vmap_broadcasting_interaction(self):
|
|
# https://github.com/jax-ml/jax/issues/6452
|
|
def f2(y, z):
|
|
v1 = z
|
|
v2 = jnp.sum(y) + z
|
|
return jnp.logaddexp(v1, v2)
|
|
|
|
def f1(y, z):
|
|
v = api.vmap(lambda _y: f2(_y, z))(y)
|
|
return jnp.sum(v)
|
|
|
|
y = jnp.ones((3, 2))
|
|
f = lambda z: f1(y, z)
|
|
z = 0.1
|
|
val, g = api.value_and_grad(f)(z)
|
|
self.assertEqual(val.shape, ())
|
|
self.assertEqual(g.shape, ())
|
|
|
|
def test_custom_jvp_vmap_broadcasting_interaction_2(self):
|
|
# https://github.com/jax-ml/jax/issues/5849
|
|
@jax.custom_jvp
|
|
def transform(box, R):
|
|
if jnp.isscalar(box) or box.size == 1:
|
|
return R * box
|
|
elif box.ndim == 2:
|
|
return jnp.einsum('ij,j->i', box, R)
|
|
raise ValueError()
|
|
|
|
@transform.defjvp
|
|
def transform_jvp(primals, tangents):
|
|
box, R = primals
|
|
dbox, dR = tangents
|
|
return (transform(box, R), dR + transform(dbox, R))
|
|
|
|
def periodic_general(box):
|
|
def displacement_fn(Ra, Rb, **kwargs):
|
|
_box = kwargs.get('box', box)
|
|
return transform(_box, Ra - Rb)
|
|
|
|
return displacement_fn
|
|
|
|
N = 250
|
|
|
|
scalar_box = 1.0
|
|
displacement = periodic_general(scalar_box)
|
|
|
|
key = jax.random.key(0)
|
|
R = jax.random.uniform(key, (N, 2))
|
|
|
|
def energy_fn(box):
|
|
d = partial(displacement, box=box)
|
|
d = api.vmap(api.vmap(d, (None, 0)), (0, None))
|
|
return jnp.sum(d(R, R) ** 2)
|
|
|
|
self.assertEqual(grad(energy_fn)(scalar_box).shape, ())
|
|
|
|
def test_custom_jvp_implicit_broadcasting(self):
|
|
# https://github.com/jax-ml/jax/issues/6357
|
|
if config.enable_x64.value:
|
|
raise unittest.SkipTest("test only applies when x64 is disabled")
|
|
|
|
@jax.custom_jvp
|
|
def projection_unit_simplex(x: jax.Array) -> jax.Array:
|
|
"""Projection onto the unit simplex."""
|
|
s = 1.0
|
|
n_features = x.shape[0]
|
|
u = jnp.sort(x)[::-1]
|
|
cssv = jnp.cumsum(u) - s
|
|
ind = jnp.arange(n_features, dtype=x.dtype) + 1
|
|
cond = u - cssv / ind > 0
|
|
idx = jnp.count_nonzero(cond)
|
|
threshold = cssv[idx - 1] / idx.astype(x.dtype)
|
|
return jax.nn.relu(x - threshold)
|
|
|
|
|
|
@projection_unit_simplex.defjvp
|
|
def projection_unit_simplex_jvp(primals, tangents):
|
|
x, = primals
|
|
x_dot, = tangents
|
|
primal_out = projection_unit_simplex(x)
|
|
supp = (primal_out > 0).astype(x_dot.dtype)
|
|
card = jnp.count_nonzero(supp).astype(x_dot.dtype)
|
|
tangent_out = supp * x_dot - (jnp.dot(supp, x_dot) / card) * supp
|
|
return primal_out, tangent_out
|
|
|
|
rng = self.rng()
|
|
x = rng.rand(5).astype(np.float32)
|
|
|
|
J_rev = jax.jacrev(projection_unit_simplex)(x)
|
|
J_fwd = jax.jacfwd(projection_unit_simplex)(x)
|
|
|
|
p = projection_unit_simplex(x)
|
|
support = (p > 0).astype(jnp.float32)
|
|
cardinality = jnp.count_nonzero(support).astype(support.dtype)
|
|
J_true = jnp.diag(support) - jnp.outer(support, support) / cardinality
|
|
self.assertAllClose(J_true, J_fwd)
|
|
self.assertAllClose(J_true, J_rev)
|
|
|
|
proj = jax.vmap(projection_unit_simplex)
|
|
|
|
def fun(X):
|
|
return jnp.sum(proj(X) ** 2)
|
|
|
|
rng = self.rng()
|
|
X = rng.rand(4, 5).astype(np.float32)
|
|
U = rng.rand(4, 5)
|
|
U /= np.sqrt(np.sum(U ** 2))
|
|
U = U.astype(np.float32)
|
|
|
|
eps = 1e-3
|
|
dir_deriv_num = (fun(X + eps * U) - fun(X - eps * U)) / (2 * eps)
|
|
dir_deriv = jnp.vdot(jax.grad(fun)(X), U)
|
|
self.assertAllClose(dir_deriv, dir_deriv_num, atol=1e-3)
|
|
|
|
def test_vmap_inside_defjvp(self):
|
|
# https://github.com/jax-ml/jax/issues/3201
|
|
seed = 47
|
|
key = jax.random.key(seed)
|
|
mat = jax.random.normal(key, (2, 3))
|
|
|
|
@jax.custom_jvp
|
|
def f(mat, aux):
|
|
num_rows, num_cols = mat.shape
|
|
return jnp.ones((num_rows, 1)) / num_cols
|
|
|
|
@f.defjvp
|
|
def f_jvp(primals, tangents):
|
|
mat, aux = primals
|
|
vec, _ = tangents
|
|
output = f(*primals)
|
|
num_rows, num_cols = mat.shape
|
|
size = num_rows * num_cols
|
|
# -----
|
|
bd_mat = mat.reshape(1, 1, num_rows, num_cols)
|
|
bd_mat = jnp.tile(bd_mat, reps=(num_rows, num_cols))
|
|
bd_mat = bd_mat.reshape(size, num_rows, num_cols)
|
|
# -----
|
|
rowsum = jnp.sum(mat, axis=1, keepdims=True)
|
|
colsum = jnp.sum(mat, axis=0, keepdims=True)
|
|
bd_rowsum = jnp.tile(rowsum, reps=(1, num_rows))
|
|
bd_colsum = jnp.tile(colsum, reps=(num_cols, 1))
|
|
# -----
|
|
bd_vec = vec.reshape(size, 1)
|
|
# -----
|
|
def operate(mx, val):
|
|
buf = 0
|
|
for i in range(2):
|
|
buf = buf + jnp.matmul(mx, bd_colsum) / jnp.power(aux, i)
|
|
buf = jnp.matmul(bd_rowsum, buf)
|
|
return buf * val[None, :]
|
|
# -----
|
|
# Vertorizing will raise shape error
|
|
bd_buf = jax.vmap(operate, in_axes=(0, 0), out_axes=0)(bd_mat, bd_vec)
|
|
# -----
|
|
bd_buf = bd_buf / aux
|
|
jvp = jnp.sum(bd_buf, axis=0)
|
|
jvp = jnp.mean(jvp, axis=1, keepdims=True)
|
|
# -----
|
|
# JVP ends successfully, but still raise an error
|
|
return (output, jvp)
|
|
|
|
jax.grad(lambda mat, aux: jnp.sum(f(mat, aux)))(mat, 0.5) # doesn't crash
|
|
|
|
def test_custom_jvp_unbroadcasting(self):
|
|
# https://github.com/jax-ml/jax/issues/3056
|
|
a = jnp.array([1., 1.])
|
|
|
|
@jax.custom_jvp
|
|
def f(x):
|
|
return a * x
|
|
|
|
@f.defjvp
|
|
def f_jvp(primals, tangents):
|
|
x, = primals
|
|
dx, = tangents
|
|
return a * x, a * dx
|
|
|
|
shape = grad(lambda x: jnp.sum(f(x)))(jnp.array(1.)).shape
|
|
self.assertEqual(shape, ())
|
|
|
|
def test_maybe_perturbed_internal_helper_function(self):
|
|
# This is a unit test for an internal API. We include it so as not to
|
|
# regress https://github.com/jax-ml/jax/issues/9567. For an explanation of
|
|
# this helper function, see https://github.com/jax-ml/jax/issues/6415.
|
|
def f(x):
|
|
def g(y, _):
|
|
z = y * x
|
|
self.assertTrue(custom_derivatives._maybe_perturbed(z))
|
|
return y, None
|
|
g(1, None)
|
|
return lax.scan(g, 1, xs=None, length=1)[0]
|
|
|
|
jax.jvp(f, (1.0,), (1.0,)) # assertions inside f
|
|
|
|
def test_maybe_perturbed_int_regression(self):
|
|
# see https://github.com/jax-ml/jax/discussions/9951
|
|
|
|
@jax.jit
|
|
def f():
|
|
x = jnp.array(1)
|
|
_, aux_args = custom_derivatives.closure_convert(lambda: x)
|
|
self.assertEmpty(aux_args)
|
|
f()
|
|
|
|
def test_sinc_constant_function_batching(self):
|
|
# https://github.com/jax-ml/jax/pull/10756
|
|
batch_data = jnp.arange(15.).reshape(5, 3)
|
|
|
|
@jax.vmap
|
|
def f(x):
|
|
return jax.lax.map(jnp.sinc, x)
|
|
g = lambda param: f(param * batch_data).sum()
|
|
|
|
@jax.vmap
|
|
def f_ref(x):
|
|
return jnp.stack([jnp.sinc(x_) for x_ in x])
|
|
g_ref = lambda param: f_ref(param * batch_data).sum()
|
|
|
|
grad = jax.grad(g )(0.1) # doesn't crash
|
|
grad_ref = jax.grad(g_ref)(0.1)
|
|
self.assertAllClose(grad, grad_ref, check_dtypes=False)
|
|
|
|
@parameterized.named_parameters(
|
|
('jit_vmap', True, True),
|
|
('jit', True, False),
|
|
('vmap', False, True),
|
|
('', False, False),
|
|
)
|
|
def test_symbolic_zero_custom_jvp(self, maybe_jit, maybe_vmap):
|
|
def f(static_scalar, static_array, dyn_scalar, dyn_array):
|
|
out1 = static_scalar + dyn_scalar
|
|
out2 = static_array + dyn_array
|
|
return out1, out2
|
|
|
|
def _pack(x):
|
|
return lax.broadcast(x, (1,))
|
|
|
|
def _unpack(x):
|
|
(x,) = x
|
|
return x
|
|
|
|
def _vmap(fun):
|
|
def _fun(*args):
|
|
args = jax.tree.map(_pack, args)
|
|
out = jax.vmap(fun)(*args)
|
|
out = jax.tree.map(_unpack, out)
|
|
return out
|
|
return _fun
|
|
|
|
f = jax.custom_jvp(f)
|
|
|
|
@partial(f.defjvp, symbolic_zeros=True)
|
|
def f_jvp(primals, tangents):
|
|
static_scalar, *_ = primals
|
|
t_static, t_static_arr, t_dyn_scalar, t_dyn_array = tangents
|
|
self.assertIs(type(t_static) , custom_derivatives_public.SymbolicZero)
|
|
self.assertIs(type(t_static_arr), custom_derivatives_public.SymbolicZero)
|
|
self.assertEqual(t_static.shape, ())
|
|
self.assertEqual(t_static_arr.shape, (2,))
|
|
return f(*primals), (static_scalar + 90, t_dyn_array + 91)
|
|
|
|
def g(dyn_scalar, dyn_array):
|
|
if maybe_vmap:
|
|
f_ = _vmap(f)
|
|
else:
|
|
f_ = f
|
|
return f_(1., jnp.array([2., 3.]), dyn_scalar, dyn_array)
|
|
|
|
def run(primal_ins, tangent_ins):
|
|
return jax.jvp(g, primal_ins, tangent_ins)
|
|
|
|
if maybe_jit:
|
|
run = jax.jit(run)
|
|
|
|
primal_ins = (4., jnp.array([5., 6.]))
|
|
tangent_ins = (7., jnp.array([8., 9.]))
|
|
primal_outs, tangent_outs = run(primal_ins, tangent_ins)
|
|
primal_out1, primal_out2 = primal_outs
|
|
tangent_out1, tangent_out2 = tangent_outs
|
|
scalar_type = jax.Array if maybe_jit or maybe_vmap else float
|
|
self.assertIsInstance(primal_out1, scalar_type)
|
|
self.assertAllClose(primal_out1, 5.)
|
|
self.assertIsInstance(tangent_out1, scalar_type)
|
|
self.assertAllClose(tangent_out1, 91.)
|
|
self.assertIsInstance(primal_out2, jax.Array)
|
|
self.assertArraysAllClose(primal_out2, jnp.array([7., 9.]))
|
|
self.assertIsInstance(tangent_out2, jax.Array)
|
|
self.assertArraysAllClose(tangent_out2, jnp.array([99., 100.]))
|
|
|
|
def test_symbolic_zero_custom_jvp_vmap_output(self):
|
|
@jax.custom_jvp
|
|
def f(x, y):
|
|
return x * y
|
|
|
|
@partial(f.defjvp, symbolic_zeros=True)
|
|
def f_jvp(primals, tangents):
|
|
x, y = primals
|
|
x_dot, y_dot = tangents
|
|
self.assertIs(type(y_dot), custom_derivatives_public.SymbolicZero)
|
|
return f(x, y), y_dot
|
|
|
|
jax.grad(lambda x, y: jax.vmap(f)(x, y).sum())(jnp.ones(3), jnp.ones(3))
|
|
|
|
def test_symbolic_zeros_memoization_caching(self):
|
|
# Tests multiple zero patterns for partial_eval._memoize, and also tests
|
|
# that we're okay with stores being occupied with equal values.
|
|
|
|
@jax.custom_jvp
|
|
def f(x, y):
|
|
return x * y
|
|
|
|
@partial(f.defjvp, symbolic_zeros=True)
|
|
def f_jvp(primals, tangents):
|
|
x, y = primals
|
|
x_dot, y_dot = tangents
|
|
return f(x, y), y_dot
|
|
|
|
f_ = core.jaxpr_as_fun(jax.make_jaxpr(f)(2., 3.))
|
|
_ = jax.linearize(f_, 2., 3.)
|
|
_ = jax.linearize(lambda x: f_(x, 3.), 2.) # don't crash!
|
|
|
|
def test_symbolic_zeros_under_jit(self):
|
|
# https://github.com/jax-ml/jax/issues/14833
|
|
Zero = jax.custom_derivatives.SymbolicZero
|
|
|
|
@jax.custom_jvp
|
|
def f(x, y):
|
|
return x * y
|
|
|
|
@partial(f.defjvp, symbolic_zeros=True)
|
|
def fjvp(primals, tangents):
|
|
x, y = primals
|
|
tx, ty = tangents
|
|
assert type(tx) is not Zero or type(ty) is not Zero
|
|
return f(x, y), (
|
|
ty if type(tx) is Zero else
|
|
tx if type(ty) is Zero else
|
|
tx + ty)
|
|
|
|
jax.jacfwd(jax.jit(f))(0.1, 0.2) # don't crash
|
|
|
|
def test_custom_jvp_functools_partial(self):
|
|
def fun(x, y, a):
|
|
return x + y * a
|
|
|
|
fun_wrapped = functools.partial(fun, a = 0.1)
|
|
|
|
def jvp_fn(primals, tangents):
|
|
return jax.jvp(fun_wrapped, primals, tangents)
|
|
|
|
fn = jax.custom_jvp(fun_wrapped)
|
|
fn.defjvp(jvp_fn)
|
|
|
|
self.assertEqual((1.0, 0.1), jax.grad(lambda args: fn(*args))((1.0, 2.0)))
|
|
|
|
def test_run_rules_more_than_once(self):
|
|
# https://github.com/jax-ml/jax/issues/16614
|
|
|
|
@jax.custom_jvp
|
|
def f(x, y):
|
|
return x
|
|
|
|
@partial(f.defjvp, symbolic_zeros=True)
|
|
def f_jvp(primals, tangents):
|
|
x, _ = primals
|
|
x_dot, _ = tangents
|
|
return x, x_dot
|
|
|
|
def body(x_y, _):
|
|
x, y = x_y
|
|
return (f(x, y), x), None
|
|
|
|
@jax.grad
|
|
def g(x):
|
|
(out, _), _ = lax.scan(body, (x, 1.), xs=None, length=2)
|
|
return out
|
|
|
|
g(1.) # doesn't crash
|
|
|
|
|
|
class CustomVJPTest(jtu.JaxTestCase):
|
|
|
|
def test_basic(self):
|
|
@jax.custom_vjp
|
|
def f(x):
|
|
return jnp.sin(x)
|
|
def f_fwd(x):
|
|
return f(x), jnp.cos(x)
|
|
def f_rev(cos_x, g):
|
|
return (2 * cos_x * g,)
|
|
f.defvjp(f_fwd, f_rev)
|
|
|
|
x = 3.
|
|
self.assertAllClose(f(x), jnp.sin(x))
|
|
self.assertAllClose(api.grad(f)(x), 2 * jnp.cos(x))
|
|
self.assertAllClose(api.value_and_grad(f)(x),
|
|
(jnp.sin(x), 2 * jnp.cos(x)))
|
|
|
|
def test_invariance(self):
|
|
@jax.custom_vjp
|
|
def f(x):
|
|
return jnp.cos(2 * x) / 2.
|
|
def f_fwd(x):
|
|
return (f(x), x)
|
|
def f_rev(x, g):
|
|
return (g * 3,)
|
|
f.defvjp(f_fwd, f_rev)
|
|
def f2(x):
|
|
y, _ = api.value_and_grad(f)(x)
|
|
return y
|
|
def f3(x):
|
|
y, _ = api.value_and_grad(f2)(x)
|
|
return y
|
|
x = 1.
|
|
self.assertAllClose(f(x), f2(x), check_dtypes=False)
|
|
self.assertAllClose(f(x), f3(x), check_dtypes=False)
|
|
self.assertAllClose(api.grad(f)(x), api.grad(f2)(x),
|
|
check_dtypes=False)
|
|
self.assertAllClose(api.grad(f)(x), api.grad(f3)(x),
|
|
check_dtypes=False)
|
|
|
|
def test_python_control_flow(self):
|
|
@jax.custom_vjp
|
|
def f(x):
|
|
if x > 0:
|
|
return jnp.sin(x)
|
|
else:
|
|
return jnp.cos(x)
|
|
def f_fwd(x):
|
|
if x > 0:
|
|
return f(x), x
|
|
else:
|
|
return f(x), x
|
|
def f_rev(x, g):
|
|
if x > 0:
|
|
return (2 * g,)
|
|
else:
|
|
return (3 * g,)
|
|
f.defvjp(f_fwd, f_rev)
|
|
x = 2.
|
|
self.assertAllClose(f(x), jnp.sin(x))
|
|
self.assertAllClose(f(-x), jnp.cos(-x))
|
|
self.assertAllClose(api.value_and_grad(f)(x), (jnp.sin(x), 2.),
|
|
check_dtypes=False)
|
|
self.assertAllClose(api.value_and_grad(f)(-x), (jnp.cos(-x), 3.),
|
|
check_dtypes=False)
|
|
|
|
def test_vmap(self):
|
|
@jax.custom_vjp
|
|
def f(x):
|
|
assert jnp.ndim(x) == 0
|
|
return jnp.sin(x)
|
|
def f_fwd(x):
|
|
assert jnp.ndim(x) == 0
|
|
return f(x), jnp.cos(x)
|
|
def f_rev(cos_x, g):
|
|
return (2 * cos_x * g,)
|
|
f.defvjp(f_fwd, f_rev)
|
|
|
|
x = jnp.arange(3.)
|
|
xx = jnp.arange(6.).reshape(2, 3)
|
|
|
|
# vmap of f
|
|
self.assertAllClose(api.vmap(f)(x), jnp.sin(x))
|
|
self.assertAllClose(api.vmap(api.vmap(f))(xx), jnp.sin(xx))
|
|
|
|
# vmap of grad of f
|
|
self.assertAllClose(api.vmap(api.grad(f))(x), 2 * jnp.cos(x))
|
|
self.assertAllClose(api.vmap(api.value_and_grad(f))(x),
|
|
(jnp.sin(x), 2 * jnp.cos(x)))
|
|
self.assertAllClose(api.vmap(api.vmap(api.grad(f)))(xx), 2 * jnp.cos(xx))
|
|
self.assertAllClose(api.vmap(api.vmap(api.value_and_grad(f)))(xx),
|
|
(jnp.sin(xx), 2 * jnp.cos(xx)))
|
|
|
|
# grad of vmap of f
|
|
self.assertAllClose(api.grad(lambda x: api.vmap(f)(x).sum())(x),
|
|
2 * jnp.cos(x))
|
|
self.assertAllClose(api.grad(lambda x: api.vmap(api.vmap(f))(x).sum())(xx),
|
|
2 * jnp.cos(xx))
|
|
|
|
# vmap of grad of vmap of f
|
|
self.assertAllClose(api.vmap(api.grad(lambda x: api.vmap(f)(x).sum()))(xx),
|
|
2 * jnp.cos(xx))
|
|
|
|
def test_jit(self):
|
|
@jax.custom_vjp
|
|
def f(x):
|
|
return jnp.sin(x)
|
|
def f_fwd(x):
|
|
return f(x), jnp.cos(x)
|
|
def f_rev(cos_x, g):
|
|
return (2 * cos_x * g,)
|
|
f.defvjp(f_fwd, f_rev)
|
|
|
|
x = 3.
|
|
|
|
# jit
|
|
self.assertAllClose(api.jit(f)(x), jnp.sin(x))
|
|
self.assertAllClose(api.jit(api.jit(f))(x), jnp.sin(x))
|
|
|
|
# jit of grad
|
|
self.assertAllClose(api.jit(api.grad(f))(x), 2 * jnp.cos(x),
|
|
check_dtypes=False)
|
|
|
|
# grad of jit
|
|
self.assertAllClose(api.grad(api.jit(f))(x), 2 * jnp.cos(x),
|
|
check_dtypes=False)
|
|
|
|
def test_pytrees(self):
|
|
@jax.custom_vjp
|
|
def f(x):
|
|
return {'b': jnp.sin(x['a'])}
|
|
def f_fwd(x):
|
|
return f(x), {'r': jnp.cos(x['a'])}
|
|
def f_bwd(res, g):
|
|
cos_x = res['r']
|
|
return ({'a': 2 * cos_x * g['b']},)
|
|
f.defvjp(f_fwd, f_bwd)
|
|
x = {'a': 3.}
|
|
self.assertAllClose(f(x)['b'], jnp.sin(x['a']))
|
|
self.assertAllClose(api.grad(lambda x: f(x)['b'])(x),
|
|
{'a': 2 * jnp.cos(x['a'])})
|
|
|
|
def test_jvp_error(self):
|
|
@jax.custom_vjp
|
|
def f(x):
|
|
return jnp.sin(x)
|
|
def f_fwd(x):
|
|
return f(x), jnp.cos(x)
|
|
def f_rev(cos_x, g):
|
|
return (2 * cos_x * g,)
|
|
f.defvjp(f_fwd, f_rev)
|
|
|
|
self.assertRaisesRegex(
|
|
TypeError,
|
|
r"can't apply forward-mode autodiff \(jvp\) to a custom_vjp function.",
|
|
lambda: api.jvp(f, (3.,), (1.,)))
|
|
self.assertRaisesRegex(
|
|
TypeError,
|
|
r"can't apply forward-mode autodiff \(jvp\) to a custom_vjp function.",
|
|
lambda: api.jvp(api.vmap(f), (jnp.arange(3.),), (jnp.ones(3),)))
|
|
self.assertRaisesRegex(
|
|
TypeError,
|
|
r"can't apply forward-mode autodiff \(jvp\) to a custom_vjp function.",
|
|
lambda: api.jvp(jit(f), (3.,), (1.,)))
|
|
|
|
def test_kwargs(self):
|
|
# from https://github.com/jax-ml/jax/issues/1938
|
|
@jax.custom_vjp
|
|
def my_fun(x, y, c=1.):
|
|
return c * (x + y)
|
|
my_fun.defvjp(lambda x, y, c=1.: (my_fun(c, y, c), None),
|
|
lambda _, g: (g, g, g))
|
|
f = lambda x, y: jnp.square(my_fun(x, y, c=2.)).sum()
|
|
f(10., 5.) # doesn't crash
|
|
api.grad(f)(10., 5.) # doesn't crash
|
|
|
|
def test_initial_style(self):
|
|
@jax.custom_vjp
|
|
def f(x):
|
|
return jnp.sin(x)
|
|
def f_fwd(x):
|
|
return f(x), jnp.cos(x)
|
|
def f_rev(cos_x, g):
|
|
return (2 * cos_x * g,)
|
|
f.defvjp(f_fwd, f_rev)
|
|
|
|
def foo(x):
|
|
out, _ = lax.scan(lambda c, _: (f(c), None), x, None, length=1)
|
|
return out
|
|
|
|
ans = api.grad(foo)(3.)
|
|
expected = 2. * jnp.cos(3.)
|
|
self.assertAllClose(ans, expected, check_dtypes=False)
|
|
|
|
ans = api.grad(api.grad(foo))(3.)
|
|
expected = -2. * jnp.sin(3.)
|
|
self.assertAllClose(ans, expected)
|
|
|
|
def test_initial_style_vmap(self):
|
|
@jax.custom_vjp
|
|
def f(x):
|
|
assert jnp.ndim(x) == 0
|
|
return 3 * x
|
|
def f_fwd(x):
|
|
return f(x), jnp.cos(x)
|
|
def f_rev(cos_x, g):
|
|
return (2 * cos_x * g,)
|
|
f.defvjp(f_fwd, f_rev)
|
|
|
|
def foo(x):
|
|
out, _ = lax.scan(lambda c, _: (f(c), None), x, None, length=1)
|
|
return out
|
|
|
|
ans = api.vmap(foo)(jnp.arange(3.))
|
|
expected = 3. * jnp.arange(3.)
|
|
self.assertAllClose(ans, expected, check_dtypes=False)
|
|
|
|
ans = api.grad(lambda x: api.vmap(foo)(x).sum())(jnp.arange(3.))
|
|
expected = 2. * jnp.cos(jnp.arange(3.))
|
|
self.assertAllClose(ans, expected, check_dtypes=False)
|
|
|
|
def test_nondiff_arg(self):
|
|
@partial(jax.custom_vjp, nondiff_argnums=(0,))
|
|
def app(f, x):
|
|
return f(x)
|
|
def app_fwd(f, x):
|
|
return app(f, x), jnp.cos(x)
|
|
def app_rev(f, cos_x, g):
|
|
return (cos_x * g,)
|
|
app.defvjp(app_fwd, app_rev)
|
|
|
|
ans = app(lambda x: 2 * x, 1)
|
|
expected = 2
|
|
self.assertAllClose(ans, expected, check_dtypes=False)
|
|
|
|
ans = api.value_and_grad(lambda x: app(lambda y: 2 * y, x))(1.)
|
|
expected = (2., jnp.cos(1.))
|
|
self.assertAllClose(ans, expected, check_dtypes=False)
|
|
|
|
def test_closed_over_jit_tracer(self):
|
|
# See the comment in CustomJVPTest.test_nondiff_arg_jit_tracer.
|
|
raise unittest.SkipTest("behavior no longer supported")
|
|
|
|
# This test is similar to test_nondiff_arg_tracer except it uses lexical
|
|
# closure rather than the nondiff_argnums mechanism. We decided to disallow
|
|
# tracers in nondiff_argnums to greatly simplify bookkeeping while still
|
|
# supporting the cases for which it is necessary.
|
|
def outer(x):
|
|
@jax.custom_vjp
|
|
def f(y):
|
|
return x * y
|
|
def f_fwd(y):
|
|
return f(y), jnp.cos(y)
|
|
def f_rev(cos_y, g):
|
|
return (cos_y * g,)
|
|
f.defvjp(f_fwd, f_rev)
|
|
return f
|
|
|
|
@jit
|
|
def g(x, y):
|
|
return outer(x)(y)
|
|
|
|
ans = g(2, 3.)
|
|
expected = 6.
|
|
self.assertAllClose(ans, expected, check_dtypes=False)
|
|
|
|
ans = api.grad(g, 1)(2., 3.)
|
|
expected = jnp.cos(3.)
|
|
self.assertAllClose(ans, expected, check_dtypes=False)
|
|
|
|
def test_closed_over_vmap_tracer(self):
|
|
def outer(x):
|
|
@jax.custom_vjp
|
|
def f(y):
|
|
return x * y
|
|
def f_fwd(y):
|
|
return f(y), jnp.cos(y)
|
|
def f_rev(cos_y, g):
|
|
return (cos_y * g,)
|
|
f.defvjp(f_fwd, f_rev)
|
|
return f
|
|
|
|
@api.vmap
|
|
def g(x):
|
|
return outer(x)(3.)
|
|
|
|
ans = g(np.arange(3.))
|
|
expected = np.arange(3.) * 3
|
|
self.assertAllClose(ans, expected, check_dtypes=False)
|
|
|
|
def test_closed_over_tracer3(self):
|
|
def outer(x):
|
|
@jax.custom_vjp
|
|
def f(y):
|
|
return x * y
|
|
def f_fwd(y):
|
|
return f(y), (x, jnp.cos(y))
|
|
def f_rev(res, g):
|
|
x, cos_y = res
|
|
return (cos_y * g * x,)
|
|
f.defvjp(f_fwd, f_rev)
|
|
return api.grad(f)
|
|
|
|
@api.vmap
|
|
def g(x):
|
|
return outer(x)(3.)
|
|
|
|
ans = g(np.arange(3.))
|
|
expected = np.cos(3.) * np.arange(3.)
|
|
self.assertAllClose(ans, expected, check_dtypes=False)
|
|
|
|
def test_nondiff_arg_tracer_error(self):
|
|
# This is similar to the old (now skipped) test_nondiff_arg_tracer, except
|
|
# we're testing for the error message that usage pattern now raises.
|
|
|
|
@partial(jax.custom_vjp, nondiff_argnums=(0,))
|
|
def f(x, y):
|
|
return x * y
|
|
def f_fwd(x, y):
|
|
return f(x, y), jnp.cos(y)
|
|
def f_rev(x, cos_y, g):
|
|
return (cos_y * g,)
|
|
f.defvjp(f_fwd, f_rev)
|
|
|
|
@jit
|
|
def g(x, y):
|
|
return f(x, y)
|
|
|
|
with self.assertRaisesRegex(UnexpectedTracerError, "custom_vjp"):
|
|
_ = g(2, 3.)
|
|
with self.assertRaisesRegex(UnexpectedTracerError, "custom_vjp"):
|
|
_ = api.grad(g, 1)(2., 3.)
|
|
|
|
def test_vmap_axes(self):
|
|
raise unittest.SkipTest("TODO") # TODO(mattjj): write test
|
|
|
|
def test_pmap(self):
|
|
raise unittest.SkipTest("TODO") # TODO(mattjj): write test
|
|
|
|
def test_missing_vjp_rule_error(self):
|
|
@jax.custom_vjp
|
|
def foo(x):
|
|
return x ** 2
|
|
|
|
self.assertRaisesRegex(
|
|
AttributeError,
|
|
r"No VJP defined for custom_vjp function foo using defvjp.",
|
|
lambda: foo(2))
|
|
self.assertRaisesRegex(
|
|
AttributeError,
|
|
r"No VJP defined for custom_vjp function foo using defvjp.",
|
|
lambda: api.grad(foo)(2.))
|
|
|
|
def test_vjp_rule_inconsistent_pytree_structures_error(self):
|
|
@jax.custom_vjp
|
|
def f(x):
|
|
return x
|
|
|
|
def foo_fwd(x):
|
|
return x, None
|
|
|
|
def foo_bwd(_, g):
|
|
return (g, g)
|
|
|
|
f.defvjp(foo_fwd, foo_bwd)
|
|
|
|
f(2) # doesn't crash
|
|
self.assertRaisesRegex(
|
|
TypeError,
|
|
re.escape(
|
|
"Custom VJP bwd rule must produce an output with the same container "
|
|
"(pytree) structure as the args tuple of the primal function, "
|
|
"and in particular must produce a tuple of length equal to the "
|
|
"number of arguments to the primal function, but got bwd output "
|
|
"structure {} for primal input structure {}.".format(
|
|
jax.tree.structure((1, 1)),
|
|
jax.tree.structure((1,)))
|
|
),
|
|
lambda: api.grad(f)(2.))
|
|
|
|
def test_vjp_bwd_returns_non_tuple_error(self):
|
|
@jax.custom_vjp
|
|
def f(x):
|
|
return x
|
|
|
|
def foo_fwd(x):
|
|
return x, None
|
|
|
|
def foo_bwd(_, g):
|
|
return 2. * g # Should be a tuple
|
|
|
|
f.defvjp(foo_fwd, foo_bwd)
|
|
with self.assertRaisesRegex(TypeError, "Custom VJP bwd rule .* must produce a tuple"):
|
|
api.grad(f)(3.)
|
|
|
|
def test_fwd_rule_primal_out_type_doesnt_match_primal_error_message(self):
|
|
# https://github.com/lucidrains/flash-attention-jax/issues/7
|
|
|
|
def scan_apply(f, x):
|
|
y, _ = jax.lax.scan(lambda x, _: (f(x), None), x, None, length=1)
|
|
return y
|
|
|
|
@jax.custom_vjp
|
|
def f(x):
|
|
return x
|
|
|
|
def f_fwd(x):
|
|
return (x, x), None
|
|
|
|
def f_bwd(_, y_bar):
|
|
return (y_bar,)
|
|
|
|
f.defvjp(f_fwd, f_bwd)
|
|
|
|
self.assertRaisesRegex(
|
|
TypeError,
|
|
re.escape(
|
|
"Custom VJP fwd rule f_fwd for function f must produce a pair "
|
|
"(list or tuple of length two) where the first element represents "
|
|
"the primal output (equal to the output of the "
|
|
"custom_vjp-decorated function f) and the second element "
|
|
"represents residuals (i.e. values stored from the forward "
|
|
"pass for use on the backward pass), but instead the fwd rule "
|
|
"output's first element had container/pytree structure:\n"
|
|
" (float32[], float32[])\n"
|
|
"while the custom_vjp-decorated function f had output "
|
|
"container/pytree structure:\n"
|
|
" float32[]."
|
|
),
|
|
lambda: jax.grad(lambda x: scan_apply(f, x))(jnp.float32(1.)))
|
|
|
|
def f_fwd2(x):
|
|
return jnp.zeros((3, *x.shape), x.dtype), None
|
|
|
|
def f_bwd2(_, y_bar):
|
|
return (y_bar,)
|
|
|
|
f.defvjp(f_fwd2, f_bwd2)
|
|
|
|
self.assertRaisesRegex(
|
|
TypeError,
|
|
re.escape(
|
|
"Custom VJP fwd rule f_fwd2 for function f must produce a pair "
|
|
"(list or tuple of length two) where the first element represents "
|
|
"the primal output (equal to the output of the "
|
|
"custom_vjp-decorated function f) and the second element "
|
|
"represents residuals (i.e. values stored from the forward "
|
|
"pass for use on the backward pass), but instead the fwd rule "
|
|
"output's first element had shapes/dtypes of:\n"
|
|
" float32[3]\n"
|
|
"while the custom_vjp-decorated function f had output "
|
|
"shapes/dtypes of:\n"
|
|
" float32[]"
|
|
),
|
|
lambda: jax.grad(lambda x: scan_apply(f, x))(jnp.float32(1.)))
|
|
|
|
def test_issue2511(self):
|
|
arr = jnp.ones((5, 2, 2))
|
|
foo = lambda x: api.vmap(jnp.linalg.det, (0,))(x)
|
|
api.jit(foo)(arr) # doesn't crash
|
|
|
|
def test_lowering_out_of_traces(self):
|
|
# https://github.com/jax-ml/jax/issues/2578
|
|
|
|
class F(collections.namedtuple("F", ["a"])):
|
|
def __call__(self, x):
|
|
return jax.nn.relu(self.a) * x
|
|
|
|
@jax.jit
|
|
def g(f, x):
|
|
return f(x)
|
|
|
|
jax.grad(g, argnums=(1,))(F(2.0), 0.) # doesn't crash
|
|
|
|
def test_clip_gradient(self):
|
|
# https://github.com/jax-ml/jax/issues/2784
|
|
@jax.custom_vjp
|
|
def _clip_gradient(lo, hi, x):
|
|
return x # identity function when not differentiating
|
|
|
|
def clip_gradient_fwd(lo, hi, x):
|
|
return x, (lo, hi,)
|
|
|
|
def clip_gradient_bwd(res, g):
|
|
lo, hi = res
|
|
return (None, None, jnp.clip(g, lo, hi),)
|
|
|
|
_clip_gradient.defvjp(clip_gradient_fwd, clip_gradient_bwd)
|
|
|
|
def clip_gradient(x):
|
|
lo = -0.1
|
|
hi = x + 0.1
|
|
return _clip_gradient(lo, hi, x)
|
|
|
|
g = jax.grad(clip_gradient)(0.1) # doesn't crash
|
|
self.assertAllClose(g, jnp.array(0.2))
|
|
|
|
def test_nestable_vjp(self):
|
|
# Verify that https://github.com/jax-ml/jax/issues/3667 is resolved.
|
|
def f(x):
|
|
return x ** 2
|
|
|
|
@jax.custom_vjp
|
|
def g(x):
|
|
return f(x)
|
|
|
|
def g_fwd(x):
|
|
y, f_vjp = api.vjp(f, x)
|
|
return y, f_vjp
|
|
|
|
def g_bwd(f_vjp, y_bar):
|
|
return f_vjp(y_bar)
|
|
|
|
g.defvjp(g_fwd, g_bwd)
|
|
|
|
# Check that VJP can be nested in simple situations. For this to pass,
|
|
# vjp has to return a PyTree.
|
|
_, g_vjp = api.vjp(g, 1.0)
|
|
y, = g_vjp(1.0)
|
|
self.assertAllClose(y, jnp.array(2.0))
|
|
|
|
# Check that VJP can be nested in complex situations. For this to pass,
|
|
# vjp can't treat the closed-over tracer x as a static argument.
|
|
@jit
|
|
def z(x):
|
|
_, g_vjp = api.vjp(g, x)
|
|
return g_vjp
|
|
y, = z(1.0)(3.0)
|
|
self.assertAllClose(y, jnp.array(6.0))
|
|
|
|
def test_initial_style_vmap_2(self):
|
|
# https://github.com/jax-ml/jax/issues/4173
|
|
x = jnp.ones((10, 3))
|
|
|
|
# Create the custom function
|
|
@jax.custom_vjp
|
|
def custom_fun(x):
|
|
return x.sum()
|
|
|
|
def forward(x):
|
|
return x.sum(), (jnp.ones_like(x),)
|
|
|
|
def backward(res, g):
|
|
return g * res[0],
|
|
|
|
custom_fun.defvjp(forward, backward)
|
|
|
|
def train_fun(x):
|
|
|
|
def summed_fun(x):
|
|
return api.vmap(custom_fun)(x).sum()
|
|
|
|
return api.grad(summed_fun)(x)
|
|
|
|
def scan_body(carry, inputs):
|
|
x = carry
|
|
return carry, train_fun(x)
|
|
|
|
scan_range = jnp.arange(4)
|
|
lax.scan(scan_body, x, scan_range) # don't crash
|
|
|
|
def test_initial_style_vmap_3(self):
|
|
# This is like test_initial_style_vmap except the primal function closes
|
|
# over an array constant.
|
|
y = jnp.arange(1., 4.)
|
|
|
|
@jax.custom_vjp
|
|
def f(x):
|
|
assert jnp.ndim(x) == 0
|
|
return 3 * x * jnp.sum(y)
|
|
def f_fwd(x):
|
|
return f(x), jnp.cos(x)
|
|
def f_rev(cos_x, g):
|
|
return (2 * cos_x * g,)
|
|
f.defvjp(f_fwd, f_rev)
|
|
|
|
def foo(x):
|
|
out, _ = lax.scan(lambda c, _: (f(c), None), x, None, length=1)
|
|
return out
|
|
|
|
ans = api.vmap(foo)(jnp.arange(3.))
|
|
expected = 3. * jnp.arange(3.) * 6
|
|
self.assertAllClose(ans, expected, check_dtypes=False)
|
|
|
|
ans = api.grad(lambda x: api.vmap(foo)(x).sum())(jnp.arange(3.))
|
|
expected = 2. * jnp.cos(jnp.arange(3.))
|
|
self.assertAllClose(ans, expected, check_dtypes=False)
|
|
|
|
def test_initial_style_vmap_with_collective(self):
|
|
|
|
@jax.custom_vjp
|
|
def f(x):
|
|
return lax.psum(x, 'foo')
|
|
|
|
def f_fwd(x):
|
|
return lax.psum(x, 'foo'), None
|
|
|
|
def f_bwd(res, dx):
|
|
return dx
|
|
f.defvjp(f_fwd, f_bwd)
|
|
|
|
def g(x):
|
|
jaxpr = api.make_jaxpr(f)(x)
|
|
return core.eval_jaxpr(jaxpr.jaxpr, [], x)[0]
|
|
|
|
out = api.vmap(lambda _, x: g(x), axis_name='foo', in_axes=(0, None),
|
|
out_axes=None)(jnp.arange(4.), 2.)
|
|
self.assertAllClose(out, 8.)
|
|
|
|
def test_bwd_closes_over_tracer(self):
|
|
def f(y):
|
|
@jax.custom_vjp
|
|
def f(x):
|
|
return 2. * jnp.sin(x)
|
|
|
|
def fwd(x):
|
|
return f(x), ()
|
|
|
|
def bwd(_, g):
|
|
return (2. * jnp.cos(y) * g,) # capture!
|
|
|
|
f.defvjp(fwd, bwd)
|
|
|
|
return jax.grad(f)(1.)
|
|
|
|
ans = jax.jit(f)(2.)
|
|
self.assertAllClose(ans, 2. * jnp.cos(2.))
|
|
|
|
ans = jax.vmap(f)(jnp.arange(3.))
|
|
self.assertAllClose(ans, 2. * jnp.cos(jnp.arange(3.)))
|
|
|
|
ans = jax.jit(jax.vmap(f))(jnp.arange(3.))
|
|
self.assertAllClose(ans, 2. * jnp.cos(jnp.arange(3.)))
|
|
|
|
ans = jax.vmap(jax.jit(f))(jnp.arange(3.))
|
|
self.assertAllClose(ans, 2. * jnp.cos(jnp.arange(3.)))
|
|
|
|
ans = jax.grad(f)(4.)
|
|
self.assertAllClose(ans, -2. * jnp.sin(4.))
|
|
|
|
def test_fwd_closes_over_tracer(self):
|
|
def f(y):
|
|
@jax.custom_vjp
|
|
def f(x):
|
|
return 2. * jnp.sin(x)
|
|
|
|
def fwd(x):
|
|
return f(x), y
|
|
|
|
def bwd(y, g):
|
|
return (2. * jnp.cos(y) * g,) # capture!
|
|
|
|
f.defvjp(fwd, bwd)
|
|
|
|
return jax.grad(f)(1.)
|
|
|
|
ans = jax.jit(f)(2.)
|
|
self.assertAllClose(ans, 2. * jnp.cos(2.))
|
|
|
|
ans = jax.vmap(f)(jnp.arange(3.))
|
|
self.assertAllClose(ans, 2. * jnp.cos(jnp.arange(3.)))
|
|
|
|
ans = jax.jit(jax.vmap(f))(jnp.arange(3.))
|
|
self.assertAllClose(ans, 2. * jnp.cos(jnp.arange(3.)))
|
|
|
|
ans = jax.vmap(jax.jit(f))(jnp.arange(3.))
|
|
self.assertAllClose(ans, 2. * jnp.cos(jnp.arange(3.)))
|
|
|
|
ans = jax.grad(f)(4.)
|
|
self.assertAllClose(ans, -2. * jnp.sin(4.))
|
|
|
|
def test_float0(self):
|
|
@jax.custom_vjp
|
|
def f(x, _):
|
|
return x
|
|
def f_fwd(x, _):
|
|
# we need a defined (non-float0) tangent to trigger the rule
|
|
return x, (2., 1)
|
|
def f_rev(*_):
|
|
return (2., 1)
|
|
f.defvjp(f_fwd, f_rev)
|
|
|
|
x = 2.
|
|
y = 3
|
|
self.assertEqual(api.grad(f, allow_int=True, argnums=(0, 1))(x, y),
|
|
(2., np.zeros(shape=(), dtype=float0)))
|
|
|
|
def test_float0_initial_style(self):
|
|
@jax.custom_vjp
|
|
def f(x):
|
|
return x
|
|
def f_fwd(x):
|
|
return x, (2., x)
|
|
def f_rev(*_):
|
|
return ((2., jnp.zeros(shape=(), dtype=float0)),)
|
|
f.defvjp(f_fwd, f_rev)
|
|
|
|
def foo(x, y):
|
|
out, _ = lax.scan(lambda c, _: (f(c), None), (x, y), None, length=1)
|
|
return out[0]
|
|
|
|
x = 2.
|
|
y = 3
|
|
self.assertEqual(api.grad(foo, allow_int=True, argnums=(0, 1))(x, y),
|
|
(2., np.zeros(shape=(), dtype=float0)))
|
|
|
|
def test_remat(self):
|
|
@jax.custom_vjp
|
|
def f(x):
|
|
return jnp.sin(x)
|
|
def f_fwd(x):
|
|
return f(x), jnp.cos(x)
|
|
def f_rev(cos_x, g):
|
|
return (2 * cos_x * g,)
|
|
f.defvjp(f_fwd, f_rev)
|
|
|
|
@jax.remat
|
|
def g(x):
|
|
return f(f(x))
|
|
|
|
ans = g(2.)
|
|
expected = np.sin(np.sin(2.))
|
|
self.assertAllClose(ans, expected, check_dtypes=False)
|
|
|
|
ans = api.grad(g)(2.)
|
|
expected = 4. * api.grad(lambda x: jnp.sin(jnp.sin(x)))(2.)
|
|
self.assertAllClose(ans, expected, check_dtypes=False)
|
|
|
|
def test_remat_higher_order(self):
|
|
@jax.custom_vjp
|
|
def f(x):
|
|
return jnp.sin(x)
|
|
def f_fwd(x):
|
|
return f(x), jnp.cos(x)
|
|
def f_rev(cos_x, g):
|
|
return (2 * cos_x * g,)
|
|
f.defvjp(f_fwd, f_rev)
|
|
|
|
def g(x):
|
|
return f(f(x))
|
|
|
|
ans = api.grad(api.grad(jax.remat(g)))(2.)
|
|
expected = api.grad(api.grad(g))(2.)
|
|
self.assertAllClose(ans, expected, check_dtypes=False)
|
|
|
|
ans = api.grad(jax.remat(api.grad(g)))(2.)
|
|
expected = api.grad(api.grad(g))(2.)
|
|
self.assertAllClose(ans, expected, check_dtypes=False)
|
|
|
|
ans = api.grad(api.grad(api.grad(jax.remat(g))))(2.)
|
|
expected = api.grad(api.grad(api.grad(g)))(2.)
|
|
self.assertAllClose(ans, expected, check_dtypes=False)
|
|
|
|
def test_bwd_nones(self):
|
|
@jax.custom_vjp
|
|
def f(x, y):
|
|
return x * jnp.sin(y)
|
|
def f_fwd(x, y):
|
|
return f(x, y), jnp.cos(y)
|
|
def f_rev(cos, g):
|
|
return (None, 2 * cos * g)
|
|
f.defvjp(f_fwd, f_rev)
|
|
|
|
ans = api.grad(lambda x: f(x, x))(3.)
|
|
expected = 2 * jnp.cos(3.)
|
|
self.assertAllClose(ans, expected, check_dtypes=False)
|
|
|
|
def test_bwd_nones_vmap(self):
|
|
@jax.custom_vjp
|
|
def f(x, y):
|
|
return x * jnp.sin(y)
|
|
def f_fwd(x, y):
|
|
return f(x, y), jnp.cos(y)
|
|
def f_rev(cos, g):
|
|
return (None, 2 * cos * g)
|
|
f.defvjp(f_fwd, f_rev)
|
|
|
|
ans = api.grad(lambda x: api.vmap(f)(x, x).sum())(jnp.arange(3.))
|
|
expected = 2 * jnp.cos(jnp.arange(3.))
|
|
self.assertAllClose(ans, expected, check_dtypes=False)
|
|
|
|
def test_bwd_nones_pytree(self):
|
|
@jax.custom_vjp
|
|
def f(xs, y):
|
|
x1, x2 = xs
|
|
return x1 * x2 * jnp.sin(y)
|
|
def f_fwd(xs, y):
|
|
return f(xs, y), jnp.cos(y)
|
|
def f_rev(cos, g):
|
|
return (None, 2 * cos * g)
|
|
f.defvjp(f_fwd, f_rev)
|
|
|
|
ans = api.grad(lambda x: f((x, x), x))(3.)
|
|
expected = 2 * jnp.cos(3.)
|
|
self.assertAllClose(ans, expected, check_dtypes=False)
|
|
|
|
def test_custom_vjp_closure_4521(self):
|
|
# https://github.com/jax-ml/jax/issues/4521
|
|
@jax.custom_vjp
|
|
def g(x, y):
|
|
return None
|
|
def g_fwd(x, y):
|
|
return None, y
|
|
def g_bwd(residuals, z_bar):
|
|
assert False
|
|
|
|
g.defvjp(g_fwd, g_bwd)
|
|
|
|
def f(xs, y):
|
|
v_g = api.vmap(g, in_axes=(0, None), out_axes=None)
|
|
v_g(xs, y)
|
|
|
|
def scan_body(xs, _):
|
|
y = jnp.zeros(1)
|
|
_, vjp_f = api.vjp(f, xs, y)
|
|
vjp_f(None)
|
|
return xs, None
|
|
|
|
lax.scan(scan_body, jnp.ones(5), None, 100) # doesn't crash
|
|
|
|
def test_float0_bwd_none(self):
|
|
@jax.custom_vjp
|
|
def f(i, x):
|
|
return jnp.sin(x)
|
|
def f_fwd(i, x):
|
|
return f(i, x), jnp.cos(x)
|
|
def f_rev(cos_x, g):
|
|
return (None, 2 * cos_x * g)
|
|
f.defvjp(f_fwd, f_rev)
|
|
|
|
ans = api.grad(f, 1)(jnp.array([1, 2]), 3.) # doesn't crash
|
|
expected = 2 * jnp.cos(3.)
|
|
self.assertAllClose(ans, expected, check_dtypes=False)
|
|
|
|
def test_custom_gradient(self):
|
|
@jax.custom_gradient
|
|
def f(x):
|
|
return x ** 2, lambda g: (g * x,)
|
|
|
|
self.assertAllClose(f(3.), 9., check_dtypes=False)
|
|
self.assertAllClose(api.grad(f)(3.), 3., check_dtypes=False)
|
|
self.assertAllClose(api.grad(api.grad(f))(3.), 1., check_dtypes=False)
|
|
|
|
def test_custom_gradient_2(self):
|
|
@jax.custom_gradient
|
|
def f(x, y):
|
|
return x * y, lambda g: (y, x)
|
|
|
|
self.assertAllClose(f(3., 4.), 12., check_dtypes=False)
|
|
self.assertAllClose(api.grad(f, argnums=(0, 1))(3., 4.), (4., 3.),
|
|
check_dtypes=False)
|
|
|
|
def test_custom_gradient_3(self):
|
|
@jax.custom_gradient
|
|
def f(x):
|
|
vjp = lambda g: (jnp.cos(x) * jnp.arange(3., 6.),)
|
|
return jnp.sum(jnp.sin(x)), vjp
|
|
|
|
self.assertAllClose(f(jnp.arange(3)), jnp.sum(jnp.sin(jnp.arange(3.))),
|
|
check_dtypes=False)
|
|
self.assertAllClose(
|
|
api.grad(f)(jnp.arange(3.)),
|
|
api.grad(lambda x: jnp.sum(jnp.sin(x)))(jnp.arange(3.)) * jnp.arange(3., 6.),
|
|
check_dtypes=False)
|
|
|
|
def test_custom_gradient_can_return_singleton_value_in_vjp(self):
|
|
@jax.custom_gradient
|
|
def f(x):
|
|
return x ** 2, lambda g: g * x
|
|
|
|
self.assertAllClose(f(3.), 9., check_dtypes=False)
|
|
self.assertAllClose(api.grad(f)(3.), 3., check_dtypes=False)
|
|
self.assertAllClose(api.grad(api.grad(f))(3.), 1., check_dtypes=False)
|
|
|
|
def test_closure_convert(self):
|
|
def cos_after(fn, x):
|
|
converted_fn, aux_args = jax.closure_convert(fn, x)
|
|
self.assertLessEqual(len(aux_args), 1)
|
|
return _cos_after(converted_fn, x, *aux_args)
|
|
|
|
@partial(jax.custom_vjp, nondiff_argnums=(0,))
|
|
def _cos_after(fn, x, *args):
|
|
return jnp.cos(fn(x, *args))
|
|
|
|
def fwd(fn, x, *args):
|
|
y = _cos_after(fn, x, *args)
|
|
return y, (x, args)
|
|
|
|
def rev(fn, res, g):
|
|
x, args = res
|
|
x_bar = 17. * x
|
|
args_bars = [42. * a for a in args]
|
|
return (x_bar, *args_bars)
|
|
|
|
_cos_after.defvjp(fwd, rev)
|
|
|
|
def dist(c, x):
|
|
return jnp.sum((x - c) ** 2.)
|
|
|
|
def solve(c, x):
|
|
def closure(x):
|
|
return dist(c, x)
|
|
return cos_after(closure, x)
|
|
|
|
c, x = 2. * jnp.ones(2), jnp.ones(2)
|
|
expected = jnp.cos(dist(c, x))
|
|
self.assertAllClose(solve(c, x), expected, check_dtypes=False)
|
|
g_c, g_x = api.grad(solve, argnums=(0, 1))(c, x)
|
|
self.assertAllClose(g_c, 42. * c, check_dtypes=False)
|
|
self.assertAllClose(g_x, 17. * x, check_dtypes=False)
|
|
|
|
def test_closure_convert_mixed_consts(self):
|
|
# Like test_closure_convert, but close over values that
|
|
# participate in AD as well as values that do not.
|
|
# See https://github.com/jax-ml/jax/issues/6415
|
|
|
|
def cos_after(fn, x):
|
|
converted_fn, aux_args = jax.closure_convert(fn, x)
|
|
self.assertLessEqual(len(aux_args), 1)
|
|
return _cos_after(converted_fn, x, *aux_args)
|
|
|
|
@partial(jax.custom_vjp, nondiff_argnums=(0,))
|
|
def _cos_after(fn, x, *args):
|
|
return jnp.cos(fn(x, *args))
|
|
|
|
def fwd(fn, x, *args):
|
|
y = _cos_after(fn, x, *args)
|
|
return y, (x, args)
|
|
|
|
def rev(fn, res, g):
|
|
x, args = res
|
|
x_bar = 17. * x
|
|
args_bars = [42. * a for a in args]
|
|
return (x_bar, *args_bars)
|
|
|
|
_cos_after.defvjp(fwd, rev)
|
|
|
|
def dist(c, s, x):
|
|
return jnp.sum(s * (x - c) ** 2.)
|
|
|
|
def solve(c, s, x):
|
|
def closure(x):
|
|
return dist(c, s, x)
|
|
return cos_after(closure, x)
|
|
|
|
c, s, x = 2. * jnp.ones(2), 3. * jnp.ones(2), jnp.ones(2)
|
|
expected = jnp.cos(dist(c, s, x))
|
|
self.assertAllClose(solve(c, s, x), expected, check_dtypes=False)
|
|
g_c, g_x = api.grad(solve, argnums=(0, 2))(c, s, x)
|
|
self.assertAllClose(g_c, 42. * c, check_dtypes=False)
|
|
self.assertAllClose(g_x, 17. * x, check_dtypes=False)
|
|
|
|
def test_closure_convert_pytree_mismatch(self):
|
|
# See https://github.com/jax-ml/jax/issues/23588
|
|
def f(x, z):
|
|
return z * x
|
|
|
|
x, z = 2.0, 3.0
|
|
_, vjp = api.vjp(f, x, z)
|
|
vjp_pure, vjp_aux_args = jax.closure_convert(vjp, x)
|
|
vjp_pure(x, *vjp_aux_args)
|
|
with self.assertRaisesRegex(
|
|
TypeError, "The inputs to the closure produced by closure_convert"):
|
|
vjp_pure(x, vjp_aux_args)
|
|
|
|
def test_float0_cotangents_automatically_handled(self):
|
|
@jax.custom_vjp
|
|
def f(x, y):
|
|
return x
|
|
|
|
def f_fwd(x, y):
|
|
return x, None
|
|
|
|
def f_bwd(_, zbar):
|
|
return (0., 1)
|
|
|
|
f.defvjp(f_fwd, f_bwd)
|
|
|
|
jax.jit(lambda x: jax.vjp(f, 0., x)[1](1.))(1) # doesn't crash
|
|
|
|
def test_custom_vjp_scan_batching_edge_case(self):
|
|
# https://github.com/jax-ml/jax/issues/5832
|
|
@jax.custom_vjp
|
|
def mul(x, coeff): return x * coeff
|
|
def mul_fwd(x, coeff): return mul(x, coeff), (x, coeff)
|
|
def mul_bwd(res, g):
|
|
x, coeff = res
|
|
g_x = g * coeff
|
|
g_coeff = (x * g).sum()
|
|
return g_x, g_coeff
|
|
mul.defvjp(mul_fwd, mul_bwd)
|
|
|
|
def scan_over_mul(x, coeff):
|
|
def f_(x, t):
|
|
return mul(x, coeff), None
|
|
y, _ = jax.lax.scan(f_, x, jnp.arange(3))
|
|
return y
|
|
|
|
key = jax.random.key(0)
|
|
key1, key2 = jax.random.split(key, 2)
|
|
x_batch = jax.random.normal(key1, (3, 2))
|
|
covector_batch = jax.random.normal(key2, (3, 2))
|
|
coeff = jnp.array(1., dtype=x_batch.dtype)
|
|
|
|
batched_scan_over_mul = jax.vmap(scan_over_mul, in_axes=(0, None), out_axes=0)
|
|
res, vjp_fun = jax.vjp(batched_scan_over_mul, x_batch, coeff)
|
|
vjp_fun(covector_batch) # doesn't crash
|
|
|
|
jtu.check_grads(batched_scan_over_mul, (x_batch, coeff), order=2,
|
|
modes=['rev'])
|
|
|
|
def test_closure_with_vmap2(self):
|
|
# https://github.com/jax-ml/jax/issues/8783
|
|
def h(z):
|
|
def f(x):
|
|
@jax.custom_vjp
|
|
def g(y):
|
|
return x * y
|
|
|
|
def g_fwd(y):
|
|
return x * y, (x, x * y, y)
|
|
def g_rev(res, w_bar):
|
|
x, *_ = res
|
|
return (x * w_bar,)
|
|
g.defvjp(g_fwd, g_rev)
|
|
|
|
return g(z)
|
|
|
|
return jax.vmap(f)(jnp.arange(3., dtype='float32')).sum()
|
|
|
|
jtu.check_grads(h, (jnp.float32(3.14),), order=1, modes=['rev'])
|
|
|
|
def test_pytrees_not_required_to_contain_nones(self):
|
|
class A(list):
|
|
pass
|
|
|
|
def unflatten(_, children):
|
|
assert children[0] is not None
|
|
return A(children)
|
|
|
|
tree_util.register_pytree_node(A, lambda x: (x, None), unflatten)
|
|
|
|
@jax.custom_vjp
|
|
def f(x):
|
|
return x[0]
|
|
def f_fwd(x):
|
|
return x[0], None
|
|
def f_bwd(_, g):
|
|
return A([g]),
|
|
f.defvjp(f_fwd, f_bwd)
|
|
|
|
jax.grad(f)(A([1.])) # doesn't crash
|
|
|
|
def test_vmap_vjp_called_twice(self):
|
|
# https://github.com/jax-ml/jax/pull/14728
|
|
@jax.custom_vjp
|
|
def f(x):
|
|
return x
|
|
f.defvjp(lambda x: (x, None), lambda _, y_bar: (y_bar,))
|
|
|
|
_, f_vjp = jax.vjp(jax.vmap(f), jnp.array([3.]))
|
|
f_vjp(jnp.array([3.]))
|
|
f_vjp(jnp.array([3.])) # doesn't crash
|
|
|
|
def test_symbolic_zero_custom_vjp_basic(self):
|
|
ZERO = custom_derivatives_public.SymbolicZero
|
|
|
|
@jax.custom_vjp
|
|
def f(x, y, z):
|
|
return x, x
|
|
|
|
def fwd(x, y, z):
|
|
self.assertIsInstance(x, jax.custom_derivatives.CustomVJPPrimal)
|
|
self.assertIsInstance(y, jax.custom_derivatives.CustomVJPPrimal)
|
|
self.assertIsInstance(z, jax.custom_derivatives.CustomVJPPrimal)
|
|
self.assertTrue(x.perturbed)
|
|
self.assertFalse(y.perturbed)
|
|
self.assertFalse(z.perturbed)
|
|
return (x.value, x.value), None
|
|
|
|
def fwd_all(x, y, z):
|
|
self.assertIsInstance(x, jax.custom_derivatives.CustomVJPPrimal)
|
|
self.assertIsInstance(y, jax.custom_derivatives.CustomVJPPrimal)
|
|
self.assertIsInstance(z, jax.custom_derivatives.CustomVJPPrimal)
|
|
self.assertTrue(x.perturbed)
|
|
self.assertTrue(y.perturbed)
|
|
self.assertTrue(z.perturbed)
|
|
return (x.value, x.value), None
|
|
|
|
def bwd_all(_, g):
|
|
x1, x2 = g
|
|
self.assertFalse(type(x1) is ZERO)
|
|
self.assertFalse(type(x2) is ZERO)
|
|
return x1, x1, x2
|
|
|
|
def bwd_fst(_, g):
|
|
x1, x2 = g
|
|
self.assertFalse(type(x1) is ZERO)
|
|
self.assertIs(type(x2), ZERO)
|
|
return x1, x1, x2
|
|
|
|
def bwd_snd(_, g):
|
|
x1, x2 = g
|
|
self.assertIs(type(x1), ZERO)
|
|
self.assertFalse(type(x2) is ZERO)
|
|
return x1, x1, x2
|
|
|
|
x, y, z = 4., 5., 6.
|
|
i = np.array(7, np.int32)
|
|
zero = np.array(0.)
|
|
|
|
f.defvjp(fwd, bwd_all, symbolic_zeros=True)
|
|
h = jax.jit(f)
|
|
jax.jacrev(h)(x, y, z)
|
|
jax.jacrev(lambda x: h(x, y, z))(x)
|
|
jax.jacrev(h, argnums=(0, 1, 2), allow_int=True)(x, i, i)
|
|
|
|
f.defvjp(fwd_all, bwd_fst, symbolic_zeros=True)
|
|
fst_f = lambda *xs: f(*xs)[0]
|
|
_, vjp = jax.vjp(fst_f, x, y, z)
|
|
_, _, gz = vjp(x)
|
|
self.assertArraysAllClose(gz, zero)
|
|
|
|
f.defvjp(fwd_all, bwd_snd, symbolic_zeros=True)
|
|
snd_f = lambda *xs: f(*xs)[1]
|
|
_, vjp = jax.vjp(snd_f, x, y, z)
|
|
gx, gy, _ = vjp(x)
|
|
self.assertArraysAllClose(gx, zero)
|
|
self.assertArraysAllClose(gy, zero)
|
|
|
|
f.defvjp(fwd, bwd_snd, symbolic_zeros=True)
|
|
_, vjp = jax.vjp(lambda x: snd_f(x, y, z), x)
|
|
gx, = vjp(x)
|
|
self.assertArraysAllClose(gx, zero)
|
|
|
|
def test_symbolic_zero_custom_vjp_bwd_shape_error(self):
|
|
@jax.custom_vjp
|
|
def f(x, y, z):
|
|
return x, y, z
|
|
|
|
def fwd(x, y, z):
|
|
return f(x.value, y.value, z.value), None
|
|
|
|
def bwd(_, gs):
|
|
x_bar, y_bar, z_bar = gs
|
|
return y_bar, x_bar, z_bar # swapped!
|
|
|
|
f.defvjp(fwd, bwd, symbolic_zeros=True)
|
|
|
|
with self.assertRaisesRegex(
|
|
ValueError,
|
|
r'Consider just returning a None here'):
|
|
jax.grad(lambda x, y, z: f(x, y, z)[2].sum())(
|
|
jnp.ones(1), jnp.ones(2), jnp.ones(3))
|
|
|
|
@parameterized.named_parameters(
|
|
('jit_vmap', True, True),
|
|
('jit', True, False),
|
|
('vmap', False, True),
|
|
('', False, False),
|
|
)
|
|
def test_symbolic_zero_custom_vjp(self, maybe_jit, maybe_vmap):
|
|
# below:
|
|
# * static_scalar will be static in and out
|
|
# * static_array will be static in, but dynamic out
|
|
# * dyn_scalar and dyn_array will be dynamic in and out
|
|
|
|
ZERO = custom_derivatives_public.SymbolicZero
|
|
|
|
def f(static_scalar, static_array, dyn_scalar, dyn_array):
|
|
out1 = static_scalar + dyn_scalar
|
|
out2 = static_array + dyn_array
|
|
return static_scalar, static_array, out1, out2
|
|
|
|
def _pack(x):
|
|
return lax.broadcast(x, (1,))
|
|
|
|
def _unpack(x):
|
|
(x,) = x
|
|
return x
|
|
|
|
def _vmap(fun):
|
|
def _fun(*args):
|
|
args = jax.tree.map(_pack, args)
|
|
out = jax.vmap(fun)(*args)
|
|
out = jax.tree.map(_unpack, out)
|
|
return out
|
|
return _fun
|
|
|
|
f = jax.custom_vjp(f)
|
|
|
|
def fwd(*args):
|
|
xs, pert = [x.value for x in args], [x.perturbed for x in args]
|
|
self.assertFalse(pert[0])
|
|
self.assertFalse(pert[1])
|
|
self.assertTrue(pert[2])
|
|
self.assertTrue(pert[3])
|
|
return f(*xs), xs
|
|
|
|
def bwd(res, g):
|
|
static_scalar, *_ = res
|
|
t_static, t_static_arr, t_dyn_scalar, t_dyn_array = g
|
|
self.assertIs(type(t_static), ZERO)
|
|
self.assertFalse(type(t_static_arr) is ZERO)
|
|
self.assertFalse(type(t_dyn_scalar) is ZERO)
|
|
self.assertFalse(type(t_dyn_array) is ZERO)
|
|
self.assertEqual(t_static.shape, ())
|
|
self.assertEqual(t_static_arr.shape, (2,))
|
|
return (static_scalar + 90,
|
|
t_static_arr + 91,
|
|
t_dyn_scalar + 92,
|
|
t_dyn_array + 93)
|
|
|
|
f.defvjp(fwd, bwd, symbolic_zeros=True)
|
|
|
|
def g(dyn_scalar, dyn_array):
|
|
if maybe_vmap:
|
|
f_ = _vmap(f)
|
|
else:
|
|
f_ = f
|
|
outs = f_(1., jnp.array([2., 3.]), dyn_scalar, dyn_array)
|
|
return outs[1:]
|
|
|
|
def run(primal_ins, cotangent_outs):
|
|
primal_outs, vjp = jax.vjp(g, *primal_ins)
|
|
cotangent_ins = vjp(cotangent_outs)
|
|
return primal_outs, cotangent_ins
|
|
|
|
if maybe_jit:
|
|
run = jax.jit(run)
|
|
|
|
scalar_type = jax.Array if maybe_jit or maybe_vmap else float
|
|
primal_ins = (4., jnp.array([5., 6.]))
|
|
cotangent_outs = (jnp.array([10., 11.]), 7., jnp.array([8., 9.]))
|
|
primal_outs, cotangent_ins = run(primal_ins, cotangent_outs)
|
|
|
|
primal_out1, primal_out2, primal_out3 = primal_outs
|
|
self.assertIsInstance(primal_out1, jax.Array)
|
|
self.assertAllClose(primal_out1, jnp.array([2., 3.]))
|
|
self.assertIsInstance(primal_out2, scalar_type)
|
|
self.assertAllClose(primal_out2, 5.)
|
|
self.assertIsInstance(primal_out3, jax.Array)
|
|
self.assertAllClose(primal_out3, jnp.array([7., 9.]))
|
|
|
|
ct_in1, ct_in2 = cotangent_ins
|
|
self.assertIsInstance(ct_in1, scalar_type)
|
|
self.assertAllClose(ct_in1, 99.)
|
|
self.assertIsInstance(ct_in2, jax.Array)
|
|
self.assertArraysAllClose(ct_in2, jnp.array([101., 102.]))
|
|
|
|
def test_symbolic_zero_custom_vjp_vmap_output(self):
|
|
@jax.custom_vjp
|
|
def f(x, y):
|
|
return x, y
|
|
|
|
def fwd(x, y):
|
|
self.assertTrue(x.perturbed)
|
|
self.assertFalse(y.perturbed)
|
|
return f(x.value, y.value), None
|
|
|
|
def bwd(_, g):
|
|
_, ct_y = g
|
|
self.assertIs(type(ct_y), custom_derivatives_public.SymbolicZero)
|
|
return g
|
|
|
|
f.defvjp(fwd, bwd, symbolic_zeros=True)
|
|
jax.grad(lambda x, y: jax.vmap(f)(x, y)[0].sum())(jnp.ones(3), jnp.ones(3))
|
|
|
|
def test_symbolic_zero_custom_vjp_custom_pytree(self):
|
|
tree_values = custom_derivatives_public.custom_vjp_primal_tree_values
|
|
|
|
@tree_util.register_pytree_node_class
|
|
class Box:
|
|
def __init__(self_, strict, val):
|
|
if strict:
|
|
# make sure we aren't getting special arguments that should only
|
|
# come up when symbolic_zeros is True
|
|
self.assertFalse(hasattr(val, 'perturbed'))
|
|
self_.strict = strict
|
|
self_.x = val
|
|
|
|
def tree_flatten(self_):
|
|
return [self_.x], self_.strict
|
|
|
|
@classmethod
|
|
def tree_unflatten(cls, strict, xs):
|
|
x, = xs
|
|
return cls(strict, x)
|
|
|
|
x, y = Box(False, jnp.array(72.)), jnp.array(73.)
|
|
|
|
@jax.custom_vjp
|
|
def f(box, y):
|
|
return box.x * y
|
|
|
|
def fwd0(box, y):
|
|
self.assertTrue(box.x.perturbed)
|
|
self.assertFalse(y.perturbed)
|
|
box, y = map(tree_values, [box, y])
|
|
return f(box, y), (box, y)
|
|
|
|
def bwd0(res, g):
|
|
box, y = res
|
|
return y * g, box.x * g
|
|
|
|
def fwd1(box, y):
|
|
self.assertFalse(box.x.perturbed)
|
|
self.assertTrue(y.perturbed)
|
|
box, y = map(tree_values, [box, y])
|
|
return f(box, y), (box, y)
|
|
|
|
def bwd1(res, g):
|
|
box, y = res
|
|
return y * g, box.x * g
|
|
|
|
f.defvjp(fwd0, bwd0, symbolic_zeros=True)
|
|
jax.grad(f, argnums=0)(x, y)
|
|
f.defvjp(fwd1, bwd1, symbolic_zeros=True)
|
|
jax.grad(f, argnums=1)(x, y)
|
|
|
|
def fwd_strict(box, y):
|
|
return f(box, y), (box, y)
|
|
|
|
def bwd_strict(res, g):
|
|
box, y = res
|
|
return y * g, box.x * g
|
|
|
|
f.defvjp(fwd_strict, bwd_strict)
|
|
jax.grad(f)(x, y)
|
|
|
|
def test_symbolic_zeros_memoization_caching(self):
|
|
# Tests multiple zero patterns for partial_eval._memoize, and also tests
|
|
# that we're okay with stores being occupied with equal values.
|
|
@jax.custom_vjp
|
|
def f(x, y):
|
|
return x * y
|
|
|
|
def f_fwd(x, y):
|
|
return x.value, None
|
|
|
|
def f_bwd(_, z_bar):
|
|
return z_bar, None
|
|
|
|
f.defvjp(f_fwd, f_bwd, symbolic_zeros=True)
|
|
|
|
f_ = core.jaxpr_as_fun(jax.make_jaxpr(f)(2., 3.))
|
|
_ = jax.linearize(f_, 2., 3.)
|
|
_ = jax.linearize(lambda x: f_(x, 3.), 2.) # don't crash!
|
|
|
|
def test_run_rules_more_than_once(self):
|
|
# https://github.com/jax-ml/jax/issues/16614
|
|
|
|
@jax.custom_vjp
|
|
def f(x, y):
|
|
return x + y
|
|
|
|
def f_fwd(x, y):
|
|
if y.perturbed:
|
|
res = None
|
|
else:
|
|
res = []
|
|
return x.value + y.value, res
|
|
|
|
def f_bwd(res, ct):
|
|
return ct, ct
|
|
|
|
f.defvjp(f_fwd, f_bwd, symbolic_zeros=True)
|
|
|
|
def body(x_y, _):
|
|
x, y = x_y
|
|
return (f(x, y), x), None
|
|
|
|
@jax.grad
|
|
def g(x):
|
|
(out, _), _ = lax.scan(body, (x, 1.), xs=None, length=2)
|
|
return out
|
|
|
|
g(1.) # doesn't crash
|
|
|
|
def test_nones_representing_zeros_in_subtrees_returned_by_bwd(self):
|
|
# https://github.com/jax-ml/jax/issues/8356
|
|
@jax.custom_vjp
|
|
def f(x):
|
|
return x[0]
|
|
|
|
def f_fwd(x):
|
|
return f(x), None
|
|
|
|
def f_bwd(_, z_bar):
|
|
return (z_bar, (None, None)),
|
|
|
|
f.defvjp(f_fwd, f_bwd)
|
|
|
|
jax.grad(f)((1.0, (2.0, 3.0))) # don't crash
|
|
|
|
def test_pytree_nones_returned_by_bwd(self):
|
|
@jax.custom_vjp
|
|
def f(x):
|
|
return x[0]
|
|
|
|
def f_fwd(x):
|
|
return f(x), None
|
|
|
|
def f_bwd(_, z_bar):
|
|
return (z_bar, (None, None)),
|
|
|
|
f.defvjp(f_fwd, f_bwd)
|
|
|
|
jax.grad(f)((1.0, (2.0, None))) # don't crash
|
|
|
|
def test_bwd_rule_shape_mismatch(self):
|
|
@jax.custom_vjp
|
|
def foo(x, y):
|
|
return x
|
|
|
|
def foo_fwd(x, y):
|
|
return x, None
|
|
|
|
def foo_bwd(_, g):
|
|
return jnp.zeros(3), jnp.zeros(3)
|
|
|
|
foo.defvjp(foo_fwd, foo_bwd)
|
|
|
|
with self.assertRaisesRegex(
|
|
ValueError,
|
|
r'output\[1\] the bwd rule produced an output of shape/dtype float..\[3\]'):
|
|
jax.grad(lambda x, y: foo(x, y * y).sum(), 1)(jnp.ones(3), jnp.ones(4))
|
|
|
|
def test_bwd_rule_shape_mismatch_disable(self):
|
|
# TODO(mattjj): remove this test when the config option is removed
|
|
@jax.custom_vjp
|
|
def foo(x, y):
|
|
return x
|
|
|
|
def foo_fwd(x, y):
|
|
return x, None
|
|
|
|
def foo_bwd(_, g):
|
|
return jnp.zeros(3), jnp.zeros(3)
|
|
|
|
foo.defvjp(foo_fwd, foo_bwd)
|
|
|
|
with config.custom_vjp_disable_shape_check(True):
|
|
jax.grad(lambda x, y: foo(x, y).sum(), 1)(jnp.ones(3), jnp.ones(4))
|
|
|
|
def test_bwd_rule_can_produce_list_or_tuple(self):
|
|
@jax.custom_vjp
|
|
def f(x, y):
|
|
return x * y
|
|
|
|
def f_fwd(x, y):
|
|
return f(x, y), (x, y)
|
|
|
|
def f_bwd(xy, g):
|
|
x, y = xy
|
|
return [g * y, x * g] # list, not tuple
|
|
|
|
f.defvjp(f_fwd, f_bwd)
|
|
|
|
jax.grad(f)(1., 2.) # don't crash
|
|
|
|
def test_optimize_remat(self):
|
|
def fun(x):
|
|
# This array is included to make sure that we handle consts appropriately
|
|
return np.array([1.0])*x
|
|
|
|
def fwd(x):
|
|
return np.array([2.0])*x*x/np.array([1.0]), (x,)
|
|
|
|
fwd = custom_derivatives.optimize_remat_of_custom_vjp_fwd(fun, fwd)
|
|
x = jnp.linspace(0, 5.0, 10)
|
|
self.assertAllClose(jax.jit(fwd)(x)[0], 2*x*x) # Shouldn't hit custom DCE
|
|
self.assertAllClose(jax.jit(lambda x: fwd(x)[0])(x), x) # Should be DCEed
|
|
|
|
def test_optimize_remat_vmap(self):
|
|
def fun(x):
|
|
return (np.array([1.0])*x)[0]
|
|
def fwd(x):
|
|
return (np.array([2.0])*x*x/np.array([1.0]))[0], (x,)
|
|
fwd = custom_derivatives.optimize_remat_of_custom_vjp_fwd(fun, fwd)
|
|
x = jnp.linspace(0, 5.0, 10)
|
|
self.assertAllClose(jax.jit(jax.vmap(fwd))(x)[0], 2*x*x)
|
|
self.assertAllClose(jax.jit(lambda x: jax.vmap(fwd)(x)[0])(x), x)
|
|
|
|
def test_optimize_remat_cond(self):
|
|
def fun(x):
|
|
return x
|
|
def fwd(x):
|
|
return x*x, (x,)
|
|
fwd = custom_derivatives.optimize_remat_of_custom_vjp_fwd(fun, fwd)
|
|
|
|
def g(x):
|
|
return jax.lax.cond(True, fwd, lambda x: (2.0 * x, (x,)), x)
|
|
x = jnp.linspace(0, 5.0, 10)
|
|
self.assertAllClose(jax.jit(g)(x)[0], x*x)
|
|
self.assertAllClose(jax.jit(lambda x: g(x)[0])(x), x)
|
|
|
|
def test_optimize_remat_jvp(self):
|
|
def fun(x):
|
|
return x**2
|
|
def fwd_(x):
|
|
return x*x, (x,)
|
|
fwd = custom_derivatives.optimize_remat_of_custom_vjp_fwd(fun, fwd_)
|
|
calc = jax.jvp(fwd, (3.2,), (1.0,))
|
|
expected = jax.jvp(fwd_, (3.2,), (1.0,))
|
|
self.assertAllClose(calc, expected)
|
|
|
|
@jax.jit
|
|
def g(x, t):
|
|
(y, r), (y_dot, r_dot) = jax.jvp(fwd, (x,), (t,))
|
|
return y, y_dot
|
|
calc = g(3.2, 1.0)
|
|
expected = jax.jvp(fun, (3.2,), (1.0,))
|
|
self.assertAllClose(calc, expected)
|
|
|
|
def test_optimize_remat_gh21303(self):
|
|
@jax.custom_vjp
|
|
def f(x):
|
|
return jnp.tan(x)
|
|
|
|
def f_fwd(x):
|
|
return jnp.sin(x), (x,)
|
|
|
|
def f_bwd(res, g):
|
|
x, = res
|
|
cos_x = jnp.cos(x)
|
|
return (cos_x * g,)
|
|
|
|
f.defvjp(f_fwd, f_bwd, optimize_remat=True)
|
|
|
|
def temp(x):
|
|
out = jax.remat(f)(x)
|
|
out = out ** 2
|
|
return out
|
|
|
|
v, g = jax.value_and_grad(temp)(3.2)
|
|
self.assertAllClose(v, jnp.tan(3.2)**2)
|
|
|
|
def test_optimize_remat_multiple_args(self):
|
|
def f_(x, y):
|
|
return jnp.sin(x) * y
|
|
|
|
@jax.custom_vjp
|
|
def f(x, y):
|
|
return f_(x, y)
|
|
|
|
def f_fwd(x, y):
|
|
return f(x, y), (jnp.cos(x), jnp.sin(x), y)
|
|
|
|
def f_bwd(res, g):
|
|
cos_x, sin_x, y = res
|
|
return (cos_x * g * y, sin_x * g)
|
|
|
|
f.defvjp(f_fwd, f_bwd, optimize_remat=True)
|
|
x, y = 3.2, 1.0
|
|
self.assertAllClose(jax.grad(f)(x, y), jax.grad(f_)(x, y))
|
|
|
|
def test_optimize_remat_kwargs(self):
|
|
@jax.custom_vjp
|
|
def f(x, y):
|
|
return jnp.sin(x) * y
|
|
|
|
def f_fwd(x, y, *, keyword=False):
|
|
del keyword
|
|
return f(x, y), (jnp.cos(x), jnp.sin(x), y)
|
|
|
|
def f_bwd(res, g):
|
|
cos_x, sin_x, y = res
|
|
return (cos_x * g * y, sin_x * g)
|
|
|
|
f.defvjp(f_fwd, f_bwd, optimize_remat=True)
|
|
x, y = 3.2, 1.0
|
|
jax.grad(f)(x, y) # Doesn't error
|
|
|
|
def test_optimize_remat_custom_vmap(self):
|
|
# See https://github.com/jax-ml/jax/pull/23000
|
|
@jax.custom_vjp
|
|
def f(x, y):
|
|
return jnp.sin(x) * y
|
|
|
|
@jax.custom_batching.custom_vmap
|
|
def f_fwd(x, y):
|
|
return f(x, y), (jnp.cos(x), jnp.sin(x), y)
|
|
|
|
@f_fwd.def_vmap
|
|
def f_fwd_vmap(_, in_batched, x, y):
|
|
# Insert a new const here to test the optimize_remat batching rule.
|
|
out = np.array([2.0])*f(x, y)
|
|
out_batched = (True, (True, True, True))
|
|
return (out, (jnp.cos(x), jnp.sin(x), y)), out_batched
|
|
|
|
def f_bwd(res, g):
|
|
cos_x, sin_x, y = res
|
|
return (cos_x * g * y, sin_x * g)
|
|
|
|
f.defvjp(f_fwd, f_bwd, optimize_remat=True)
|
|
x, y = jnp.linspace(0.0, 1.0, 5), jnp.linspace(2.0, 5.0, 5)
|
|
jax.jit(jax.vmap(jax.grad(f)))(x, y) # Doesn't error
|
|
|
|
|
|
def transpose_unary(f, x_example):
|
|
def transposed(y):
|
|
x, = api.linear_transpose(f, x_example)(y)
|
|
return x
|
|
return transposed
|
|
|
|
|
|
# This class wraps jax.custom_transpose.custom_transpose in order to pass in a
|
|
# particular tree of output type on each call. Otherwise it forwards
|
|
# all attribute access.
|
|
class _custom_transpose:
|
|
def __init__(self, out_types, fun):
|
|
self.out_types = out_types
|
|
self.fun = jax.custom_transpose.custom_transpose(fun)
|
|
|
|
def __getattr__(self, name):
|
|
return getattr(self.fun, name)
|
|
|
|
def __call__(self, *args):
|
|
return self.fun(self.out_types, *args)
|
|
|
|
|
|
# This function is meant to be used as a decorator that delegates to
|
|
# custom_transpose but makes it easy to specify output argument types
|
|
# by example. If used directly a decorator (i.e. not invoked with
|
|
# example arguments), assumes a scalar-valued function.
|
|
#
|
|
# TODO(frostig): remove this (and its uses) once custom_transpose offers
|
|
# an option of inferring output types.
|
|
def custom_transpose(example_out):
|
|
if isinstance(example_out, Callable):
|
|
out_type = core.get_aval(0.).to_tangent_aval()
|
|
return _custom_transpose(out_type, example_out)
|
|
return partial(
|
|
_custom_transpose,
|
|
jax.tree.map(
|
|
lambda x: core.get_aval(x).to_tangent_aval(), example_out))
|
|
|
|
|
|
class CustomTransposeTest(jtu.JaxTestCase):
|
|
|
|
def test_linear_call(self):
|
|
def f(x, y):
|
|
def fn(r, x): return x / r
|
|
def tp(r, t): return t / r
|
|
return x + jax.custom_derivatives.linear_call(fn, tp, y, x)
|
|
|
|
def f_ref(x, y):
|
|
return x + x / y
|
|
|
|
x = jnp.ones(2) * 6.
|
|
y = jnp.ones(2) * 3.
|
|
self.assertAllClose(f(x, y), f_ref(x, y))
|
|
|
|
f1 = lambda x: f(x, y)
|
|
f1_ref = lambda x: f_ref(x, y)
|
|
self.assertAllClose(transpose_unary(f1, x)(x),
|
|
transpose_unary(f1_ref, x)(x))
|
|
|
|
def test_linear_call_incorrect_transpose(self):
|
|
def f(x, y):
|
|
def fn(r, x): return x / r
|
|
def tp(r, t): return t / (2. * r) # nb: not the true transpose
|
|
return x + jax.custom_derivatives.linear_call(fn, tp, y, x)
|
|
|
|
def f_ref(x, y):
|
|
return x + x / y
|
|
|
|
x = jnp.ones(2) * 6.
|
|
y = jnp.ones(2) * 3.
|
|
self.assertAllClose(f(x, y), f_ref(x, y))
|
|
|
|
f1 = lambda x: f(x, y)
|
|
f1_ref = lambda x: f_ref(x, 2. * y) # nb: double the reference divisor
|
|
self.assertAllClose(transpose_unary(f1, x)(x),
|
|
transpose_unary(f1_ref, x)(x))
|
|
|
|
def test_linear_call_transpose_transpose_transpose(self):
|
|
def fn(r, x): return x / r
|
|
def tp(r, t): return t / (2. * r) # nb: untrue transpose
|
|
def f_(x, y):
|
|
return x + jax.custom_derivatives.linear_call(fn, tp, y, x)
|
|
|
|
x = jnp.ones(2) * 6.
|
|
y = jnp.ones(2) * 3.
|
|
f = lambda x: f_(x, y)
|
|
ft = transpose_unary(f, x)
|
|
ftt = transpose_unary(ft, x)
|
|
fttt = transpose_unary(ftt, x)
|
|
self.assertAllClose(ft(x), x + tp(y, x))
|
|
self.assertAllClose(f(x), ftt(x))
|
|
self.assertAllClose(ft(x), fttt(x))
|
|
|
|
def test_linear_call_scalar_to_vector(self):
|
|
def f(c, x):
|
|
def fn(_, x):
|
|
return [x, x]
|
|
|
|
def tp(_, t):
|
|
t1, t2 = t
|
|
return t1 + t2
|
|
|
|
return jax.custom_derivatives.linear_call(fn, tp, (), c * x)
|
|
|
|
def f_ref(c, x):
|
|
return [c * x, c * x]
|
|
|
|
c, x = 2., 3.
|
|
t = [4., 5.]
|
|
self.assertAllClose(f(c, x), f_ref(c, x))
|
|
self.assertAllClose(transpose_unary(partial(f, c), x)(t),
|
|
transpose_unary(partial(f_ref, c), x)(t))
|
|
|
|
def test_linear_call_nested(self):
|
|
# identity function with an untrue transpose of 0
|
|
def id_(x):
|
|
def f(_, x): return x
|
|
def t(_, t): return 0.
|
|
return jax.custom_derivatives.linear_call(f, t, (), x)
|
|
|
|
# identity function with an untrue transpose of 7, and where both
|
|
# forward and transpose have custom transpositions that should
|
|
# never end up invoked.
|
|
def f(x):
|
|
def f_(_, x): return id_(x)
|
|
def t_(_, t): return id_(7.)
|
|
return jax.custom_derivatives.linear_call(f_, t_, (), x)
|
|
|
|
x = 5.
|
|
id_t = transpose_unary(id_, x)
|
|
id_tt = transpose_unary(id_t, x)
|
|
ft = transpose_unary(f, x)
|
|
ftt = transpose_unary(ft, x)
|
|
fttt = transpose_unary(ftt, x)
|
|
|
|
self.assertAllClose(id_(x), x)
|
|
self.assertAllClose(id_t(x), 0.)
|
|
self.assertAllClose(id_tt(x), x)
|
|
|
|
self.assertAllClose(f(x), x)
|
|
self.assertAllClose(ft(x), 7.)
|
|
self.assertAllClose(ftt(x), x)
|
|
self.assertAllClose(fttt(x), 7.)
|
|
|
|
def test_linear_call_jit(self):
|
|
def f(x, y):
|
|
def fn(r, x): return x / r
|
|
def tp(r, t): return t / r
|
|
return x + jax.custom_derivatives.linear_call(fn, tp, y, x)
|
|
|
|
x = jnp.ones(2) * 6.
|
|
y = jnp.ones(2) * 3.
|
|
self.assertAllClose(f(x, y), jax.jit(f)(x, y))
|
|
|
|
f1 = lambda x: f(x, y)
|
|
self.assertAllClose(transpose_unary(f1, x)(x),
|
|
jax.jit(transpose_unary(f1, x))(x))
|
|
|
|
def test_basic(self):
|
|
def f(x, y):
|
|
@custom_transpose(jnp.ones(2))
|
|
def fn(r, x): return x / r
|
|
@fn.def_transpose
|
|
def tp(r, t): return t / r
|
|
|
|
return x + fn(y, x)
|
|
|
|
def f_ref(x, y):
|
|
return x + x / y
|
|
|
|
x = jnp.ones(2) * 6.
|
|
y = jnp.ones(2) * 3.
|
|
self.assertAllClose(f(x, y), f_ref(x, y))
|
|
|
|
f1 = lambda x: f(x, y)
|
|
f1_ref = lambda x: f_ref(x, y)
|
|
self.assertAllClose(transpose_unary(f1, x)(x),
|
|
transpose_unary(f1_ref, x)(x))
|
|
|
|
def test_incorrect_transpose(self):
|
|
def f(x, y):
|
|
@custom_transpose(jnp.ones(2))
|
|
def fn(r, x): return x / r
|
|
@fn.def_transpose
|
|
def tp(r, t): return t / (2. * r) # nb: not the true transpose
|
|
|
|
return x + fn(y, x)
|
|
|
|
def f_ref(x, y):
|
|
return x + x / y
|
|
|
|
x = jnp.ones(2) * 6.
|
|
y = jnp.ones(2) * 3.
|
|
self.assertAllClose(f(x, y), f_ref(x, y))
|
|
|
|
f1 = lambda x: f(x, y)
|
|
f1_ref = lambda x: f_ref(x, 2. * y) # nb: double the reference divisor
|
|
self.assertAllClose(transpose_unary(f1, x)(x),
|
|
transpose_unary(f1_ref, x)(x))
|
|
|
|
def test_transpose_transpose_transpose(self):
|
|
@custom_transpose(jnp.ones(2))
|
|
def fn(r, x): return x / r
|
|
@custom_transpose(jnp.ones(2))
|
|
def tp(r, t): return t / (2. * r) # nb: untrue transpose
|
|
|
|
fn.def_transpose(tp)
|
|
tp.def_transpose(fn)
|
|
|
|
def f_(x, y):
|
|
return x + fn(y, x)
|
|
|
|
x = jnp.ones(2) * 6.
|
|
y = jnp.ones(2) * 3.
|
|
f = lambda x: f_(x, y)
|
|
ft = transpose_unary(f, x)
|
|
ftt = transpose_unary(ft, x)
|
|
fttt = transpose_unary(ftt, x)
|
|
self.assertAllClose(ft(x), x + tp(y, x))
|
|
self.assertAllClose(f(x), ftt(x))
|
|
self.assertAllClose(ft(x), fttt(x))
|
|
|
|
def test_scalar_to_vector(self):
|
|
def f(c, x):
|
|
@custom_transpose([0., 0.])
|
|
def fn(_, x):
|
|
return [x, x]
|
|
|
|
@fn.def_transpose
|
|
def tp(_, t):
|
|
t1, t2 = t
|
|
return t1 + t2
|
|
|
|
return fn((), c * x)
|
|
|
|
def f_ref(c, x):
|
|
return [c * x, c * x]
|
|
|
|
c, x = 2., 3.
|
|
t = [4., 5.]
|
|
self.assertAllClose(f(c, x), f_ref(c, x))
|
|
self.assertAllClose(transpose_unary(partial(f, c), x)(t),
|
|
transpose_unary(partial(f_ref, c), x)(t))
|
|
|
|
def test_nested(self):
|
|
# identity function with an untrue transpose of 0
|
|
def id_(x):
|
|
f = custom_transpose(lambda _, x: x)
|
|
t = custom_transpose(lambda _, t: 0.)
|
|
f.def_transpose(t)
|
|
t.def_transpose(f)
|
|
return f((), x)
|
|
|
|
# identity function with an untrue transpose of 7, and where both
|
|
# forward and transpose have custom transpositions that should
|
|
# never end up invoked.
|
|
def f(x):
|
|
f_ = custom_transpose(lambda _, x: id_(x))
|
|
t_ = custom_transpose(lambda _, t: id_(7.))
|
|
f_.def_transpose(t_)
|
|
t_.def_transpose(f_)
|
|
return f_((), x)
|
|
|
|
x = 5.
|
|
id_t = transpose_unary(id_, x)
|
|
id_tt = transpose_unary(id_t, x)
|
|
ft = transpose_unary(f, x)
|
|
ftt = transpose_unary(ft, x)
|
|
fttt = transpose_unary(ftt, x)
|
|
|
|
self.assertAllClose(id_(x), x)
|
|
self.assertAllClose(id_t(x), 0.)
|
|
self.assertAllClose(id_tt(x), x)
|
|
|
|
self.assertAllClose(f(x), x)
|
|
self.assertAllClose(ft(x), 7.)
|
|
self.assertAllClose(ftt(x), x)
|
|
self.assertAllClose(fttt(x), 7.)
|
|
|
|
def test_one_degree(self):
|
|
T = lambda f: transpose_unary(f, 0.)
|
|
|
|
@custom_transpose
|
|
def f(_, z): return 2. * z
|
|
@f.def_transpose
|
|
def ft(_, z): return 3. * z
|
|
|
|
f = partial(f, ())
|
|
self.assertAllClose(2., f(1.))
|
|
self.assertAllClose(3., T(f)(1.))
|
|
self.assertAllClose(3., T(T(f))(1.))
|
|
self.assertAllClose(3., T(T(T(f)))(1.))
|
|
self.assertAllClose(3., T(T(T(T(f))))(1.)) # ...
|
|
|
|
def test_two_degrees(self):
|
|
T = lambda f: transpose_unary(f, 0.)
|
|
|
|
@custom_transpose
|
|
def f(_, z): return 2. * z
|
|
|
|
@f.def_transpose
|
|
@custom_transpose
|
|
def ft(_, z): return 3. * z
|
|
|
|
@ft.def_transpose
|
|
def ftt(_, z): return 7. * z
|
|
|
|
f = partial(f, ())
|
|
self.assertAllClose(2., f(1.))
|
|
self.assertAllClose(3., T(f)(1.))
|
|
self.assertAllClose(7., T(T(f))(1.))
|
|
self.assertAllClose(7., T(T(T(f)))(1.))
|
|
self.assertAllClose(7., T(T(T(T(f))))(1.)) # ...
|
|
|
|
def test_symmetric(self):
|
|
T = lambda f: transpose_unary(f, 0.)
|
|
|
|
@custom_transpose
|
|
def f(_, z): return 2. * z
|
|
@custom_transpose
|
|
def g(_, z): return 3. * z
|
|
|
|
f.def_transpose(g)
|
|
g.def_transpose(f)
|
|
|
|
f = partial(f, ())
|
|
self.assertAllClose(2., f(1.))
|
|
self.assertAllClose(3., T(f)(1.))
|
|
self.assertAllClose(2., T(T(f))(1.))
|
|
self.assertAllClose(3., T(T(T(f)))(1.))
|
|
self.assertAllClose(2., T(T(T(T(f))))(1.)) # ...
|
|
|
|
def test_recursive(self):
|
|
T = lambda f: transpose_unary(f, 0.)
|
|
|
|
@custom_transpose
|
|
def f(c, z): return c * z
|
|
|
|
@f.def_transpose
|
|
def ft(c, z): return f(c + 1., z)
|
|
|
|
g = partial(f, 1.)
|
|
self.assertAllClose(1., g(1.))
|
|
self.assertAllClose(2., T(g)(1.))
|
|
self.assertAllClose(3., T(T(g))(1.))
|
|
self.assertAllClose(4., T(T(T(g)))(1.))
|
|
self.assertAllClose(5., T(T(T(T(g))))(1.)) # ...
|
|
|
|
def test_jvp_lin(self):
|
|
def f(x, y):
|
|
@custom_transpose(jnp.ones(2))
|
|
def fn(r, x): return x / r
|
|
@fn.def_transpose
|
|
def tp(r, t): return t / r
|
|
return x + fn(y, x)
|
|
|
|
def f_ref(x, y): return x + x / y
|
|
|
|
x, y, tx = 6., 3., 1.
|
|
g = lambda x: f(x, y)
|
|
g_ref = lambda x: f_ref(x, y)
|
|
self.assertAllClose(api.jvp(g, [x], [tx]), api.jvp(g_ref, [x], [tx]))
|
|
|
|
def test_jvp_res(self):
|
|
raise unittest.SkipTest('unimplemented') # TODO(frostig)
|
|
|
|
def f(x, y):
|
|
@custom_transpose(jnp.ones(2))
|
|
def fn(r, x): return x / r
|
|
@fn.def_transpose
|
|
def tp(r, t): return t / r
|
|
return x + fn(y, x)
|
|
|
|
def f_ref(x, y): return x + x / y
|
|
|
|
x, y, ty = 6., 3., 1.
|
|
g = lambda y: f(x, y)
|
|
g_ref = lambda y: f_ref(x, y)
|
|
self.assertAllClose(api.jvp(g, [y], [ty]), api.jvp(g_ref, [y], [ty]))
|
|
|
|
def test_jvp_both(self):
|
|
raise unittest.SkipTest('unimplemented') # TODO(frostig)
|
|
|
|
def f(x, y):
|
|
@custom_transpose(jnp.ones(2))
|
|
def fn(r, x): return x / r
|
|
@fn.def_transpose
|
|
def tp(r, t): return t / r
|
|
return x + fn(y, x)
|
|
|
|
def f_ref(x, y): return x + x / y
|
|
|
|
x, y, tx, ty = 6., 3., 1., 1.
|
|
self.assertAllClose(api.jvp(f, [x, y], [tx, ty]),
|
|
api.jvp(f_ref, [x, y], [tx, ty]))
|
|
|
|
def test_make_jaxpr(self):
|
|
def f(x, y):
|
|
@custom_transpose(jnp.ones(2))
|
|
def fn(r, x): return x / r
|
|
@fn.def_transpose
|
|
def tp(r, t): return 2 * t / r
|
|
|
|
return x + fn(y, x)
|
|
|
|
x = jnp.ones(2) * 6.
|
|
y = jnp.ones(2) * 3.
|
|
f_ = lambda x: f(x, y)
|
|
f_t = transpose_unary(f_, x)
|
|
|
|
jaxpr = api.make_jaxpr(f_)(x)
|
|
self.assertIn('custom_transpose_call', str(jaxpr))
|
|
|
|
jaxpr_t = api.make_jaxpr(f_t)(x)
|
|
self.assertNotIn('custom_transpose_call', str(jaxpr_t))
|
|
|
|
def test_jit(self):
|
|
def f(x, y):
|
|
@custom_transpose(jnp.ones(2))
|
|
def fn(r, x): return x / r
|
|
@fn.def_transpose
|
|
def tp(r, t): return 2 * t / r
|
|
|
|
return x + fn(y, x)
|
|
|
|
x = jnp.ones(2) * 6.
|
|
y = jnp.ones(2) * 3.
|
|
self.assertAllClose(f(x, y), jax.jit(f)(x, y))
|
|
|
|
f_ = lambda x: f(x, y)
|
|
f_t = transpose_unary(f_, x)
|
|
g_ = jax.jit(f_)
|
|
g_t = transpose_unary(g_, x)
|
|
self.assertAllClose(f_(x), jax.jit(f_)(x))
|
|
self.assertAllClose(f_t(x), jax.jit(f_t)(x))
|
|
self.assertAllClose(f_(x), g_(x))
|
|
self.assertAllClose(f_t(x), g_t(x))
|
|
|
|
def test_jit_recursive(self):
|
|
def f(x, y):
|
|
@custom_transpose(jnp.ones(2))
|
|
def fn(r, x): return x / r
|
|
@fn.def_transpose
|
|
def tp(r, t): return 2 * fn(r, t)
|
|
|
|
return x + fn(y, x)
|
|
|
|
x = jnp.ones(2) * 6.
|
|
y = jnp.ones(2) * 3.
|
|
self.assertAllClose(f(x, y), jax.jit(f)(x, y))
|
|
|
|
f_ = lambda x: f(x, y)
|
|
f_t = transpose_unary(f_, x)
|
|
g_ = jax.jit(f_)
|
|
g_t = transpose_unary(g_, x)
|
|
self.assertAllClose(f_(x), jax.jit(f_)(x))
|
|
self.assertAllClose(f_t(x), jax.jit(f_t)(x))
|
|
self.assertAllClose(f_(x), g_(x))
|
|
self.assertAllClose(f_t(x), g_t(x))
|
|
|
|
def test_cond(self):
|
|
def f(x, y):
|
|
@custom_transpose(jnp.ones(2))
|
|
def fn(r, x): return x / r
|
|
@fn.def_transpose
|
|
def tp(r, t): return 2 * t / r
|
|
|
|
return x + fn(y, x)
|
|
|
|
def cond_wrap(f):
|
|
return lambda i, x: lax.cond(i > 0, f, lambda x: x, x)
|
|
|
|
i = 7.
|
|
x = jnp.ones(2) * 6.
|
|
y = jnp.ones(2) * 3.
|
|
|
|
f_ = lambda x: f(x, y)
|
|
f_t = transpose_unary(f_, x)
|
|
g_ = partial(cond_wrap(f_), i)
|
|
g_t = transpose_unary(g_, x)
|
|
|
|
self.assertAllClose(f_(x), g_(x))
|
|
self.assertAllClose(f_t(x), g_t(x))
|
|
|
|
def test_cond_recursive(self):
|
|
def f(x, y):
|
|
@custom_transpose(jnp.ones(2))
|
|
def fn(r, x): return x / r
|
|
@fn.def_transpose
|
|
def tp(r, t): return 2 * fn(r, t)
|
|
|
|
return x + fn(y, x)
|
|
|
|
def cond_wrap(f):
|
|
return lambda i, x: lax.cond(i > 0, f, lambda x: x, x)
|
|
|
|
i = 7.
|
|
x = jnp.ones(2) * 6.
|
|
y = jnp.ones(2) * 3.
|
|
|
|
f_ = lambda x: f(x, y)
|
|
f_t = transpose_unary(f_, x)
|
|
g_ = partial(cond_wrap(f_), i)
|
|
g_t = transpose_unary(g_, x)
|
|
|
|
self.assertAllClose(f_(x), g_(x))
|
|
self.assertAllClose(f_t(x), g_t(x))
|
|
|
|
|
|
class CustomVmapTest(jtu.JaxTestCase):
|
|
|
|
def test_basic(self):
|
|
@jax.custom_batching.custom_vmap
|
|
def f(x): return jnp.sin(x)
|
|
|
|
@f.def_vmap
|
|
def rule(axis_size, in_batched, xs):
|
|
xs_batched, = in_batched
|
|
self.assertEqual(xs_batched, True)
|
|
self.assertEqual(axis_size, xs.shape[0])
|
|
return jnp.cos(xs), xs_batched
|
|
|
|
x, xs = jnp.array(1.), jnp.arange(3)
|
|
y = f(x)
|
|
self.assertAllClose(y, jnp.sin(x))
|
|
ys = api.vmap(f)(xs)
|
|
self.assertAllClose(ys, jnp.cos(xs))
|
|
|
|
@jax.numpy_dtype_promotion('standard')
|
|
def test_closure(self):
|
|
z = jnp.array([2., 1., 3.])
|
|
|
|
@jax.custom_batching.custom_vmap
|
|
def f(x): return z + jnp.sin(x)
|
|
|
|
@f.def_vmap
|
|
def rule(axis_size, in_batched, *args):
|
|
self.assertEqual(len(in_batched), 1)
|
|
self.assertEqual(len(args), 1)
|
|
xs, = args
|
|
xs_batched, = in_batched
|
|
self.assertEqual(xs_batched, True)
|
|
self.assertEqual(axis_size, xs.shape[0])
|
|
return z + jnp.cos(xs), xs_batched
|
|
|
|
x, xs = jnp.array(1.), jnp.arange(3)
|
|
y = f(x)
|
|
self.assertAllClose(y, z + jnp.sin(x))
|
|
ys = api.vmap(f)(xs)
|
|
self.assertAllClose(ys, z + jnp.cos(xs))
|
|
|
|
def test_rule_multi_output(self):
|
|
@jax.custom_batching.custom_vmap
|
|
def f(x): return jnp.sin(x), jnp.cos(x)
|
|
|
|
@f.def_vmap
|
|
def rule(axis_size, in_batched, xs):
|
|
return (jnp.cos(xs), jnp.sin(xs)), tuple(in_batched * 2)
|
|
|
|
x, xs = jnp.array(1.), jnp.arange(3)
|
|
y1, y2 = f(x)
|
|
self.assertAllClose(y1, jnp.sin(x))
|
|
self.assertAllClose(y2, jnp.cos(x))
|
|
ys1, ys2 = api.vmap(f)(xs)
|
|
self.assertAllClose(ys1, jnp.cos(xs))
|
|
self.assertAllClose(ys2, jnp.sin(xs))
|
|
|
|
def test_nary(self):
|
|
@jax.custom_batching.custom_vmap
|
|
def f(x, y): return jnp.sin(x) + y ** 2.
|
|
|
|
@f.def_vmap
|
|
def rule(axis_size, in_batched, xs, ys):
|
|
self.assertEqual(in_batched, [True, True])
|
|
self.assertEqual(axis_size, 3)
|
|
self.assertEqual(axis_size, xs.shape[0])
|
|
self.assertEqual(axis_size, ys.shape[0])
|
|
return jnp.cos(xs) + ys ** 2., True
|
|
|
|
xs, ys = jnp.arange(3.0), jnp.arange(3.0)
|
|
zs = api.vmap(f)(xs, ys)
|
|
self.assertAllClose(zs, jnp.cos(xs) + ys ** 2.)
|
|
|
|
def test_nary_mixed_batching(self):
|
|
@jax.custom_batching.custom_vmap
|
|
def vector_dot(u, v):
|
|
self.assertEqual(u.ndim, 1)
|
|
self.assertEqual(v.ndim, 1)
|
|
return u @ v
|
|
|
|
size = 4
|
|
vlen = 3
|
|
in_batched_log = []
|
|
|
|
@vector_dot.def_vmap
|
|
def vector_dot_vmap_rule(axis_size, in_batched, u, v):
|
|
in_batched_log.append(in_batched)
|
|
self.assertEqual(axis_size, size)
|
|
u_batched, v_batched = in_batched
|
|
if u_batched:
|
|
self.assertEqual(u.ndim, 2)
|
|
self.assertEqual(u.shape[0], size)
|
|
else:
|
|
self.assertEqual(u.ndim, 1)
|
|
self.assertEqual(u.shape[0], vlen)
|
|
if v_batched:
|
|
self.assertEqual(v.ndim, 2)
|
|
self.assertEqual(v.shape[0], size)
|
|
else:
|
|
self.assertEqual(v.ndim, 1)
|
|
self.assertEqual(v.shape[0], vlen)
|
|
if u_batched and v_batched:
|
|
out = jnp.sum(u * v, axis=1)
|
|
else:
|
|
out = u @ v if u_batched else v @ u
|
|
return out, u_batched or v_batched
|
|
|
|
f = vector_dot
|
|
v = lambda *shape: jnp.ones(shape)
|
|
|
|
y = api.vmap(f, in_axes=(0, None))(v(4, 3), v(3))
|
|
self.assertAllClose(y, v(4, 3) @ v(3))
|
|
y = api.vmap(f, in_axes=(1, None))(v(3, 4), v(3))
|
|
self.assertAllClose(y, v(3, 4).T @ v(3))
|
|
y = api.vmap(f, in_axes=(None, 0))(v(3), v(4, 3))
|
|
self.assertAllClose(y, v(3) @ v(4, 3).T)
|
|
y = api.vmap(f, in_axes=(0, 0))(v(4, 3), v(4, 3))
|
|
self.assertAllClose(y, jnp.sum(v(4, 3) * v(4, 3), axis=1))
|
|
self.assertEqual(in_batched_log[0], [True, False])
|
|
self.assertEqual(in_batched_log[1], [True, False])
|
|
self.assertEqual(in_batched_log[2], [False, True])
|
|
self.assertEqual(in_batched_log[3], [True, True])
|
|
|
|
def test_rule_input_signature(self):
|
|
@jax.custom_batching.custom_vmap
|
|
def f(x): return jnp.sin(x)
|
|
|
|
rule_args = []
|
|
|
|
@f.def_vmap
|
|
def rule(axis_size, in_batched, xs):
|
|
rule_args.append((axis_size, in_batched))
|
|
return jnp.cos(xs), in_batched[0]
|
|
|
|
xs = jnp.arange(3)
|
|
_ = api.vmap(f)(xs)
|
|
(axis_size, in_batched), = rule_args
|
|
self.assertIs(type(axis_size), int)
|
|
self.assertIs(type(in_batched), list)
|
|
self.assertEqual(len(in_batched), 1)
|
|
|
|
def test_rule_output_vs_batching_output_mismatch(self):
|
|
@jax.custom_batching.custom_vmap
|
|
def f(x): return jnp.sin(x)
|
|
|
|
@f.def_vmap
|
|
def test_rule_abc(axis_size, in_batched, xs):
|
|
return [jnp.sin(xs), jnp.cos(xs)], in_batched
|
|
|
|
xs = jnp.arange(3)
|
|
self.assertRaisesRegex(
|
|
ValueError,
|
|
'structure of output value and output batching specification '
|
|
r'returned by custom vmap rule \(test_rule_abc\) do not match.*',
|
|
lambda: api.vmap(f)(xs))
|
|
|
|
def test_rule_vs_call_output_mismatch(self):
|
|
@jax.custom_batching.custom_vmap
|
|
def f(x): return jnp.sin(x)
|
|
|
|
@f.def_vmap
|
|
def test_rule_abc2(axis_size, in_batched, xs):
|
|
return [jnp.sin(xs)], in_batched
|
|
|
|
xs = jnp.arange(3)
|
|
self.assertRaisesRegex(
|
|
ValueError,
|
|
r'structure of output returned by custom vmap rule \(test_rule_abc2\) '
|
|
r'does not match that of original custom-vmapped function.*',
|
|
lambda: api.vmap(f)(xs))
|
|
|
|
def test_jvp_basic(self):
|
|
@jax.custom_batching.custom_vmap
|
|
def f(x): return jnp.sin(x)
|
|
|
|
@f.def_vmap
|
|
def rule(axis_size, in_batched, xs):
|
|
self.assertEqual(axis_size, 3)
|
|
self.assertEqual(in_batched, [True])
|
|
return jnp.cos(xs), in_batched[0]
|
|
|
|
f_jvp = lambda x, tx: api.jvp(f, [x], [tx])
|
|
|
|
x, tx = jnp.array(1.), jnp.array(2.)
|
|
xs, txs = jnp.arange(3.), jnp.arange(3.) * 2.
|
|
|
|
y, ty = f_jvp(x, tx)
|
|
self.assertAllClose(y, jnp.sin(x))
|
|
self.assertAllClose(ty, jnp.cos(x) * tx)
|
|
|
|
ys, tys = api.vmap(f_jvp)(xs, txs)
|
|
self.assertAllClose(ys, jnp.cos(xs))
|
|
self.assertAllClose(tys, -jnp.sin(xs) * txs)
|
|
|
|
ys, tys = api.jvp(api.vmap(f), [xs], [txs])
|
|
self.assertAllClose(ys, jnp.cos(xs))
|
|
self.assertAllClose(tys, -jnp.sin(xs) * txs)
|
|
|
|
@jax.numpy_dtype_promotion('standard')
|
|
def test_jvp_closure(self):
|
|
z = jnp.array([2., 1., 3.])
|
|
def bcast(x): return z + x - z
|
|
|
|
@jax.custom_batching.custom_vmap
|
|
def f(x): return z + jnp.sin(x)
|
|
|
|
@f.def_vmap
|
|
def rule(axis_size, in_batched, xs):
|
|
self.assertEqual(axis_size, 3)
|
|
self.assertEqual(in_batched, [True])
|
|
return z + jnp.cos(xs), in_batched[0]
|
|
|
|
f_jvp = lambda x, tx: api.jvp(f, [x], [tx])
|
|
|
|
x, tx = jnp.array(1.), jnp.array(2.)
|
|
xs, txs = jnp.arange(3.), jnp.arange(3.) * 2.
|
|
|
|
y, ty = f_jvp(x, tx)
|
|
self.assertAllClose(y, z + jnp.sin(x))
|
|
self.assertAllClose(ty, bcast(jnp.cos(x)) * tx)
|
|
|
|
ys, tys = api.vmap(f_jvp)(xs, txs)
|
|
self.assertAllClose(ys, z + jnp.cos(xs))
|
|
self.assertAllClose(tys, bcast(-jnp.sin(xs)) * txs)
|
|
|
|
ys, tys = api.jvp(api.vmap(f), [xs], [txs])
|
|
self.assertAllClose(ys, z + jnp.cos(xs))
|
|
self.assertAllClose(tys, bcast(-jnp.sin(xs)) * txs)
|
|
|
|
def test_jvp_nary(self):
|
|
@jax.custom_batching.custom_vmap
|
|
def f(x, y): return jnp.sin(x) + y
|
|
|
|
@f.def_vmap
|
|
def rule(axis_size, in_batched, xs, ys):
|
|
self.assertEqual(axis_size, 3)
|
|
self.assertEqual(in_batched, [True, True])
|
|
return jnp.cos(xs) + ys, True
|
|
|
|
f_jvp = lambda x, y, tx, ty: api.jvp(f, [x, y], [tx, ty])
|
|
|
|
x, y, tx, ty = jnp.arange(4.)
|
|
xs, ys, txs, tys = 4. + jnp.arange(3. * 4).reshape((4, 3))
|
|
|
|
zs, tzs = api.vmap(f_jvp)(xs, ys, txs, tys)
|
|
self.assertAllClose(zs, jnp.cos(xs) + ys)
|
|
self.assertAllClose(tzs, -jnp.sin(xs) * txs + tys)
|
|
|
|
zs, tzs = api.jvp(api.vmap(f), [xs, ys], [txs, tys])
|
|
self.assertAllClose(zs, jnp.cos(xs) + ys)
|
|
self.assertAllClose(tzs, -jnp.sin(xs) * txs + tys)
|
|
|
|
def test_jvp_extra_batched_tangents(self):
|
|
@jax.custom_batching.custom_vmap
|
|
def f(x): return jnp.sin(x)
|
|
|
|
@f.def_vmap
|
|
def rule(axis_size, in_batched, xs):
|
|
self.assertEqual(axis_size, 3)
|
|
self.assertEqual(in_batched, [False])
|
|
return jnp.cos(xs), in_batched[0]
|
|
|
|
f_jvp = lambda x, tx: api.jvp(f, [x], [tx])
|
|
|
|
txs = 2. + jnp.arange(3.)
|
|
x = jnp.array(1, dtype=txs.dtype)
|
|
y, tys = api.vmap(f_jvp, in_axes=(None, 0), out_axes=(None, 0))(x, txs)
|
|
self.assertAllClose(y, jnp.cos(x))
|
|
self.assertAllClose(tys, -jnp.sin(x) * txs)
|
|
|
|
def test_jacfwd(self):
|
|
# jacfwd is another way to exercise extra-batched tangents
|
|
|
|
@jax.custom_batching.custom_vmap
|
|
def f(x): return jnp.sin(x)
|
|
|
|
@f.def_vmap
|
|
def rule(axis_size, in_batched, xs):
|
|
self.assertEqual(axis_size, 3)
|
|
self.assertEqual(in_batched, [False])
|
|
return jnp.cos(xs), in_batched[0]
|
|
|
|
x = jnp.arange(3.) + .72
|
|
j = api.jacfwd(f)(x)
|
|
self.assertAllClose(j, -jnp.diag(jnp.sin(x)))
|
|
|
|
def test_jvp_extra_batched_primals(self):
|
|
@jax.custom_batching.custom_vmap
|
|
def f(x): return jnp.sin(x)
|
|
|
|
@f.def_vmap
|
|
def rule(axis_size, in_batched, xs):
|
|
self.assertEqual(axis_size, 3)
|
|
self.assertEqual(in_batched, [False])
|
|
return jnp.cos(xs), in_batched[0]
|
|
|
|
f_jvp = lambda x, tx: api.jvp(f, [x], [tx])
|
|
|
|
xs = jnp.arange(3.)
|
|
tx = jnp.array(4, dtype=xs.dtype)
|
|
ys, tys = api.vmap(f_jvp, in_axes=(0, None))(xs, tx)
|
|
self.assertAllClose(ys, jnp.cos(xs))
|
|
self.assertAllClose(tys, -jnp.sin(xs) * tx)
|
|
|
|
def test_jvp_extra_batched_primals_with_linear_vmap_rule(self):
|
|
# When a function is linear, its Jacobian is constant. JAX's JVP
|
|
# of linear functions takes advantage of this: when mapping over a
|
|
# batch of primals relative to a fixed (i.e. symbolically
|
|
# replicated) tangent, output tangents remain replicated as well
|
|
# (i.e. JAX will not broadcast them). This is true in general, and
|
|
# this test checks that vmapped JVPs continue to behave this way
|
|
# when custom_vmap is involved and the custom vmap rule is linear.
|
|
|
|
@jax.custom_batching.custom_vmap
|
|
def f_linear(x): return 7. * x
|
|
|
|
@f_linear.def_vmap
|
|
def linear_rule(axis_size, in_batched, xs):
|
|
return 11. * xs, in_batched[0]
|
|
|
|
@jax.custom_batching.custom_vmap
|
|
def f_nonlinear(x): return jnp.sin(x)
|
|
|
|
@f_nonlinear.def_vmap
|
|
def nonlinear_rule(axis_size, in_batched, xs):
|
|
return jnp.cos(xs), in_batched[0]
|
|
|
|
f_lin_jvp = lambda x, tx: api.jvp(f_linear, [x], [tx])
|
|
f_non_jvp = lambda x, tx: api.jvp(f_nonlinear, [x], [tx])
|
|
xs = jnp.arange(3.)
|
|
tx = jnp.array(4., dtype=xs.dtype)
|
|
|
|
# doesn't err
|
|
_ = api.vmap(f_lin_jvp, in_axes=(0, None), out_axes=(0, None))(xs, tx)
|
|
|
|
# does err
|
|
self.assertRaisesRegex(
|
|
ValueError, "at vmap out_axes",
|
|
lambda: api.vmap(
|
|
f_non_jvp, in_axes=(0, None), out_axes=(0, None))(xs, tx))
|
|
|
|
def test_jvp_dataflow_violation(self):
|
|
# The jvp-of-custom-vmap machinery should not assume the standard
|
|
# dataflow constraint on the JVP of the custom vmap rule (primal
|
|
# outputs independent of tangent inputs). Both jvp and vmap are
|
|
# "forward" transformations under which, at present, we don't
|
|
# enforce the JVP dependence diagram. Because output primals can
|
|
# depend on input tangents, extra-batched input tangents can
|
|
# create batched output primals, as this test checks.
|
|
|
|
@jax.custom_jvp
|
|
def cos_with_invalid_dataflow_jvp(x): return jnp.cos(x)
|
|
|
|
@cos_with_invalid_dataflow_jvp.defjvp
|
|
def invalid_dataflow_jvp(x, tx):
|
|
[x], [tx] = x, tx
|
|
return jnp.cos(x * tx), tx
|
|
|
|
@jax.custom_batching.custom_vmap
|
|
def f(x): return jnp.sin(x)
|
|
|
|
@f.def_vmap
|
|
def rule(axis_size, in_batched, xs):
|
|
return cos_with_invalid_dataflow_jvp(xs), in_batched[0]
|
|
|
|
f_jvp = lambda x, tx: api.jvp(f, [x], [tx])
|
|
txs = 2. + jnp.arange(3.)
|
|
x = jnp.array(1, dtype=txs.dtype)
|
|
|
|
# doesn't err
|
|
ys, tys = api.vmap(f_jvp, in_axes=(None, 0))(x, txs)
|
|
self.assertAllClose(ys, jnp.cos(x * txs))
|
|
self.assertAllClose(tys, txs)
|
|
|
|
# does err
|
|
self.assertRaisesRegex(
|
|
ValueError, "at vmap out_axes",
|
|
lambda: api.vmap(
|
|
f_jvp, in_axes=(None, 0), out_axes=(None, 0))(x, txs))
|
|
|
|
def test_tree(self):
|
|
tree_sin = partial(jax.tree.map, jnp.sin)
|
|
tree_cos = partial(jax.tree.map, jnp.cos)
|
|
|
|
x, xs = jnp.array(1.), jnp.arange(3)
|
|
x = (x, [x + 1, x + 2], [x + 3], x + 4)
|
|
xs = (xs, [xs + 1, xs + 2], [xs + 3], xs + 4)
|
|
in_batched_ref = jax.tree.map(lambda _: True, x)
|
|
|
|
@jax.custom_batching.custom_vmap
|
|
def f(xs): return tree_sin(xs)
|
|
|
|
@f.def_vmap
|
|
def rule(axis_size, in_batched, xs):
|
|
self.assertEqual(in_batched, [in_batched_ref])
|
|
sz, = {z.shape[0] for z in jax.tree.leaves(xs)}
|
|
self.assertEqual(axis_size, sz)
|
|
return tree_cos(xs), in_batched[0]
|
|
|
|
y = f(x)
|
|
self.assertAllClose(y, tree_sin(x))
|
|
ys = api.vmap(f)(xs)
|
|
self.assertAllClose(ys, tree_cos(xs))
|
|
|
|
def test_tree_with_nones(self):
|
|
tree_sin = partial(jax.tree.map, jnp.sin)
|
|
tree_cos = partial(jax.tree.map, jnp.cos)
|
|
|
|
x, xs = jnp.array(1.), jnp.arange(3)
|
|
x = (x, [x + 1, None], [x + 3], None)
|
|
xs = (xs, [xs + 1, None], [xs + 3], None)
|
|
in_batched_ref = jax.tree.map(lambda _: True, x)
|
|
|
|
@jax.custom_batching.custom_vmap
|
|
def f(xs): return tree_sin(xs)
|
|
|
|
@f.def_vmap
|
|
def rule(axis_size, in_batched, xs):
|
|
self.assertEqual(in_batched, [in_batched_ref])
|
|
sz, = {z.shape[0] for z in jax.tree.leaves(xs)}
|
|
self.assertEqual(axis_size, sz)
|
|
return tree_cos(xs), in_batched[0]
|
|
|
|
y = f(x)
|
|
self.assertAllClose(y, tree_sin(x))
|
|
ys = api.vmap(f)(xs)
|
|
self.assertAllClose(ys, tree_cos(xs))
|
|
|
|
def test_jit(self):
|
|
@jax.custom_batching.custom_vmap
|
|
def f(x): return jnp.sin(x)
|
|
|
|
@f.def_vmap
|
|
def rule(axis_size, in_batched, xs):
|
|
self.assertEqual(in_batched, [True])
|
|
self.assertEqual(axis_size, xs.shape[0])
|
|
return jnp.cos(xs), in_batched[0]
|
|
|
|
x, xs = jnp.array(1.), jnp.arange(3)
|
|
self.assertAllClose(f(x), jit(f)(x))
|
|
self.assertAllClose(jit(api.vmap(f))(xs), api.vmap(f)(xs))
|
|
self.assertAllClose(api.vmap(jit(f))(xs), api.vmap(f)(xs))
|
|
|
|
def test_sequential_vmap_basic(self):
|
|
@jax.custom_batching.sequential_vmap
|
|
def f(x):
|
|
return x + 1.
|
|
|
|
def vmap_ref(xs):
|
|
return lax.map(f, xs)
|
|
|
|
xs = jnp.arange(3.)
|
|
jaxpr = api.make_jaxpr(api.vmap(f))(xs)
|
|
jaxpr_ref = api.make_jaxpr(vmap_ref)(xs)
|
|
|
|
self.assertEqual(str(jaxpr), str(jaxpr_ref))
|
|
|
|
def test_sequential_vmap_nary_same_batching(self):
|
|
@jax.custom_batching.sequential_vmap
|
|
def f(x, y):
|
|
return x + y
|
|
|
|
def vmap_ref(xs, ys):
|
|
return lax.map(lambda args: f(*args), (xs, ys))
|
|
|
|
xs, ys = jnp.arange(3.), 4. + jnp.arange(3.)
|
|
jaxpr = api.make_jaxpr(api.vmap(f))(xs, ys)
|
|
jaxpr_ref = api.make_jaxpr(vmap_ref)(xs, ys)
|
|
|
|
self.assertEqual(str(jaxpr), str(jaxpr_ref))
|
|
|
|
def test_sequential_vmap_nary_mixed_batching(self):
|
|
@jax.custom_batching.sequential_vmap
|
|
def f(x, y):
|
|
return x + y
|
|
|
|
def vmap_ref(xs, y):
|
|
return lax.map(lambda x: f(x, y), xs)
|
|
|
|
xs, y = jnp.arange(3.), 4.
|
|
jaxpr = api.make_jaxpr(api.vmap(f, in_axes=(0, None)))(xs, y)
|
|
jaxpr_ref = api.make_jaxpr(vmap_ref)(xs, y)
|
|
|
|
self.assertEqual(str(jaxpr), str(jaxpr_ref))
|
|
|
|
@parameterized.named_parameters(
|
|
("1", 1),
|
|
("8", 4),
|
|
("12", 8),
|
|
("16", 16),
|
|
)
|
|
def test_batch_map_basic(self, batch_size: int):
|
|
def f(x):
|
|
self.assertEqual(x.shape, ())
|
|
return x**2
|
|
|
|
x = np.arange(16)
|
|
y = jax.lax.map(f, x, batch_size=batch_size)
|
|
|
|
np.testing.assert_array_equal(y, x**2)
|
|
|
|
@parameterized.named_parameters(
|
|
("1", 1),
|
|
("8", 4),
|
|
("12", 8),
|
|
("16", 16),
|
|
)
|
|
def test_batch_map_pytrees(self, batch_size: int):
|
|
f = lambda x: {'b': x['a'] ** 2}
|
|
inputs = {'a': np.arange(16)}
|
|
expected = np.arange(16) ** 2
|
|
|
|
outputs = jax.lax.map(f, inputs, batch_size=batch_size)
|
|
self.assertAllClose(outputs['b'], expected)
|
|
|
|
outputs = jax.lax.map(
|
|
f, inputs, batch_size=batch_size
|
|
)
|
|
self.assertAllClose(outputs['b'], expected)
|
|
|
|
def test_batch_divides_axis(self):
|
|
def f(t):
|
|
x, a = t
|
|
self.assertEqual(x.shape, (4,))
|
|
return (x + a)**2
|
|
|
|
x = jax.random.randint(jax.random.key(0), (16, 4), -10, 10)
|
|
a = jax.random.randint(jax.random.key(1), (16, 4), -10, 10)
|
|
|
|
@jax.jit
|
|
def g(x, a):
|
|
return jax.lax.map(f, (x, a), batch_size=8)
|
|
|
|
y = g(x, a)
|
|
|
|
self.assertAllClose(y, (x + a)**2)
|
|
|
|
def test_undefined_rule(self):
|
|
@jax.custom_batching.custom_vmap
|
|
def f(x): return jnp.sin(x)
|
|
|
|
with self.assertRaisesRegex(
|
|
AttributeError, "No batching rule defined for custom_vmap function f"):
|
|
f(0.5)
|
|
|
|
def test_kwargs(self):
|
|
@jax.custom_batching.custom_vmap
|
|
def f(x): return jnp.sin(x)
|
|
|
|
@f.def_vmap
|
|
def rule(axis_size, in_batched, xs):
|
|
xs_batched, = in_batched
|
|
self.assertEqual(xs_batched, True)
|
|
self.assertEqual(axis_size, xs.shape[0])
|
|
return jnp.cos(xs), xs_batched
|
|
|
|
x, xs = jnp.array(1.), jnp.arange(3)
|
|
y = f(x=x)
|
|
self.assertAllClose(y, jnp.sin(x))
|
|
ys = api.vmap(f)(x=xs)
|
|
self.assertAllClose(ys, jnp.cos(xs))
|
|
|
|
def test_partial_eval_raises(self):
|
|
@jax.custom_batching.custom_vmap
|
|
def f(x):
|
|
return jnp.sin(x)
|
|
|
|
@f.def_vmap
|
|
def rule(axis_size, in_batched, xs):
|
|
del axis_size # unused
|
|
return jnp.cos(xs), in_batched[0]
|
|
|
|
with self.assertRaisesRegex(
|
|
ValueError,
|
|
"Linearization failed to produce known values for all output primals",
|
|
):
|
|
jax.grad(f)(0.5)
|
|
|
|
def test_compose_custom_vjp(self):
|
|
@jax.custom_vjp
|
|
@jax.custom_batching.custom_vmap
|
|
def f(x, y):
|
|
return jnp.sin(x) * y
|
|
|
|
@f.def_vmap
|
|
def f_vmap_rule(axis_size, in_batched, xs, ys):
|
|
return jnp.cos(xs) * ys, True
|
|
|
|
def f_fwd(x, y):
|
|
return f(x, y), (jnp.cos(x), jnp.sin(x), y)
|
|
|
|
def f_bwd(res, g):
|
|
cos_x, sin_x, y = res
|
|
return (cos_x * g * y, sin_x * g)
|
|
|
|
f.defvjp(f_fwd, f_bwd)
|
|
|
|
xs = jnp.linspace(0, 1, 5)
|
|
ys = jnp.linspace(-0.1, 0.1, 5)
|
|
self.assertAllClose(jax.vmap(f)(xs, ys), jnp.cos(xs) * ys)
|
|
jax.grad(f)(xs[0], ys[0]) # Doesn't crash.
|
|
|
|
def test_compose_custom_vjp_bwd_rule(self):
|
|
# This tests the case where both the forward and backward rules are wrapped
|
|
# in custom_vmap.
|
|
@jax.custom_batching.sequential_vmap
|
|
def fun_fwd(x, y):
|
|
return jnp.sin(x) * y, (x, y)
|
|
|
|
@jax.custom_batching.sequential_vmap
|
|
def fun_bwd(res, ct):
|
|
x, y = res
|
|
return x * ct, y * ct
|
|
|
|
fun = jax.custom_vjp(lambda *args: fun_fwd(*args)[0])
|
|
fun.defvjp(fun_fwd, fun_bwd)
|
|
|
|
xs = jnp.linspace(0, 1, 5)
|
|
y = jnp.array(0.5, dtype=xs.dtype)
|
|
f = jax.vmap(jax.jit(fun), in_axes=(0, None))
|
|
out, f_vjp = jax.vjp(f, xs, y)
|
|
f_vjp(out) # Doesn't crash.
|
|
|
|
|
|
class CustomApiTest(jtu.JaxTestCase):
|
|
"""Test interactions among the custom_{vmap,jvp,vjp,transpose,*} APIs"""
|
|
|
|
def test_method_forwarding(self):
|
|
@jax.custom_batching.custom_vmap
|
|
@jax.custom_jvp
|
|
@jax.custom_transpose.custom_transpose
|
|
def f(x): return 2. * x
|
|
|
|
# none of these err:
|
|
@f.def_vmap
|
|
def f_batch(sz, b, xs): return 2. * xs
|
|
@f.defjvp
|
|
def f_jvp(x, tx): return 2. * x, 2. * tx
|
|
@f.def_transpose
|
|
def f_transpose(x): return 2. * x
|
|
|
|
def test_def_method_forwarding_all_permutations(self):
|
|
for wraps in it.permutations([
|
|
jax.custom_jvp, jax.custom_transpose.custom_transpose, jax.custom_batching.custom_vmap]):
|
|
f = lambda x: x + 1.
|
|
for wrap in wraps:
|
|
f = wrap(f)
|
|
for methods in it.permutations(['defjvp', 'def_vmap', 'def_transpose']):
|
|
for method in methods:
|
|
self.assertIsInstance(getattr(f, method), Callable)
|
|
|
|
for decorators in it.permutations([
|
|
jax.custom_vjp, jax.custom_transpose.custom_transpose, jax.custom_batching.custom_vmap]):
|
|
f = lambda x: x + 1.
|
|
for decorator in decorators:
|
|
f = decorator(f)
|
|
for methods in it.permutations(['defvjp', 'def_vmap', 'def_transpose']):
|
|
for method in methods:
|
|
self.assertIsInstance(getattr(f, method), Callable)
|
|
|
|
|
|
class BufferDonationTest(jtu.BufferDonationTestCase):
|
|
|
|
@jtu.device_supports_buffer_donation()
|
|
def test_pmap_donate_argnums_invalidates_input(self):
|
|
move = api.pmap(lambda x: x + x - x, donate_argnums=0)
|
|
n = jax.local_device_count()
|
|
x = api.pmap(lambda x: x)(jnp.ones([n]))
|
|
y = move(x)
|
|
self.assertDeleted(x)
|
|
np.testing.assert_allclose(y, [1.] * n)
|
|
|
|
@jtu.device_supports_buffer_donation()
|
|
def test_pmap_nested_donate_ignored(self):
|
|
pmap_fun = jit(lambda x: api.pmap(lambda y: y ** 2, donate_argnums=0)(x))
|
|
a = api.pmap(lambda x: x)(jnp.array([1]))
|
|
|
|
# NOTE(mattjj): stopped raising error here and instead just ignored
|
|
# with self.assertRaisesRegex(ValueError, "nested.*not supported"):
|
|
# pmap_fun(a)
|
|
|
|
pmap_fun(a) # doesn't crash
|
|
|
|
|
|
class NamedCallTest(jtu.JaxTestCase):
|
|
|
|
def test_non_jaxtype_arg(self):
|
|
# For the test to fail without the invalid JaxType filter we need to pass
|
|
# in a valid JaxType that forces the invalid Jaxtype to be raised to an
|
|
# abstract value.
|
|
def f(not_a_jaxtype, a_jaxtype):
|
|
# then Jax needs to try and evaluate the abstractified non-JaxType
|
|
if not_a_jaxtype:
|
|
return a_jaxtype
|
|
return 0
|
|
|
|
f = api.named_call(f, name="test")
|
|
out = jax.jit(f, static_argnums=(0,))("not a Jaxtype", 1)
|
|
self.assertEqual(out, 1)
|
|
|
|
@parameterized.parameters(jax.jit, jax.grad, jax.vmap, jax.remat)
|
|
def test_jax_transforms(self, transform):
|
|
f = jnp.sum
|
|
x = jnp.array([1.])
|
|
|
|
unnamed_out = transform(f)(x)
|
|
named_out = transform(api.named_call(f, name="test"))(x)
|
|
|
|
self.assertEqual(unnamed_out, named_out)
|
|
|
|
def test_static_argnums(self):
|
|
f = api.named_call(lambda x, y: y if x else None, name="test")
|
|
f = jax.jit(f, static_argnums=(0,))
|
|
out = f(True, 5)
|
|
self.assertEqual(out, 5)
|
|
|
|
def test_partial_eval(self):
|
|
f = api.named_call(lambda x, y: y if x else None, name="test")
|
|
f = jax.jit(functools.partial(f, True))
|
|
out = f(5)
|
|
self.assertEqual(out, 5)
|
|
|
|
@parameterized.parameters(
|
|
[dict(func=func, jit=jit)
|
|
for func in ['identity_trivial', 'identity', 'closure_trivial', 'closure',
|
|
'asarray', 'device_put']
|
|
for jit in jtu.JIT_IMPLEMENTATION
|
|
if not (jit._name == "noop" and func in ('identity', 'identity_trivial'))
|
|
],
|
|
)
|
|
def test_integer_overflow(self, jit, func):
|
|
funcdict = {
|
|
'identity_trivial': lambda x: x, # may hit trivial dispatch path
|
|
'identity': lambda x: x + 0,
|
|
'closure_trivial': lambda x: jax.jit(lambda: x)(),
|
|
'closure': lambda x: jax.jit(lambda: x + 0)(),
|
|
'asarray': lambda x: jnp.asarray(x), # add lambdas so no cross-test cache
|
|
'device_put': lambda x: api.device_put(x),
|
|
}
|
|
|
|
f = jit(funcdict[func])
|
|
|
|
int_dtype = dtypes.canonicalize_dtype(jnp.int64)
|
|
int_max = np.iinfo(int_dtype).max
|
|
int_min = np.iinfo(int_dtype).min
|
|
|
|
# check before any jit cache entries
|
|
self.assertRaises(OverflowError, f, int_max + 1)
|
|
self.assertRaises(OverflowError, f, int_min - 1)
|
|
|
|
self.assertEqual(f(int_max).dtype, int_dtype)
|
|
self.assertEqual(f(int_min).dtype, int_dtype)
|
|
self.assertAllClose(f(int_max), int_max)
|
|
self.assertAllClose(f(int_min), int_min)
|
|
|
|
# check after any cache entries
|
|
self.assertRaises(OverflowError, f, int_max + 1)
|
|
self.assertRaises(OverflowError, f, int_min - 1)
|
|
if func in ('trivial', 'identity'):
|
|
self.assertRaisesRegex(
|
|
OverflowError, 'An overflow.*whose argument path is x.', f,
|
|
int_max + 1)
|
|
|
|
|
|
class BackendsTest(jtu.JaxTestCase):
|
|
|
|
@unittest.skipIf(not sys.executable, "test requires sys.executable")
|
|
@jtu.run_on_devices("cpu")
|
|
def test_no_backend_warning_on_cpu_if_platform_specified(self):
|
|
warning_not_expected = (
|
|
"import jax; "
|
|
"jax.config.update('jax_platform_name', 'cpu'); "
|
|
"jax.numpy.arange(10)")
|
|
|
|
result = subprocess.run([sys.executable, '-c', warning_not_expected],
|
|
check=True, capture_output=True)
|
|
assert "may be present" not in result.stderr.decode()
|
|
|
|
|
|
class CleanupTest(jtu.JaxTestCase):
|
|
def test_call_wrapped_second_phase_cleanup(self):
|
|
try:
|
|
jax.vmap(lambda x: x, out_axes=None)(jnp.arange(3))
|
|
except:
|
|
assert core.trace_state_clean() # this is the hard one
|
|
assert core.trace_state_clean()
|
|
|
|
|
|
class EnvironmentInfoTest(jtu.JaxTestCase):
|
|
@parameterized.parameters([True, False])
|
|
@jtu.thread_unsafe_test()
|
|
def test_print_environment_info(self, return_string):
|
|
# Flush stdout buffer before checking.
|
|
sys.stdout.flush()
|
|
with jtu.capture_stdout() as stdout:
|
|
result = jax.print_environment_info(return_string=return_string)
|
|
if return_string:
|
|
self.assertEmpty(stdout())
|
|
else:
|
|
self.assertIsNone(result)
|
|
result = stdout()
|
|
assert f"jax: {jax.__version__}" in result
|
|
assert f"jaxlib: {lib.version_str}" in result
|
|
assert f"numpy: {np.__version__}" in result
|
|
|
|
class AutodidaxTest(jtu.JaxTestCase):
|
|
def test_autodidax_smoketest(self):
|
|
autodidax_file = os.path.join(
|
|
os.path.dirname(os.path.dirname(__file__)),
|
|
'docs',
|
|
'autodidax.py')
|
|
if not os.path.exists(autodidax_file):
|
|
self.skipTest("Cannot locate autodidax.py")
|
|
spec = importlib.util.spec_from_file_location('autodidax', autodidax_file)
|
|
autodidax_module = importlib.util.module_from_spec(spec)
|
|
spec.loader.exec_module(autodidax_module)
|
|
|
|
class GarbageCollectionTest(jtu.JaxTestCase):
|
|
|
|
@jtu.thread_unsafe_test() # GC isn't predictable
|
|
def test_xla_gc_callback(self):
|
|
# https://github.com/jax-ml/jax/issues/14882
|
|
x_np = np.arange(10, dtype='int32')
|
|
x_jax = jax.device_put(x_np)
|
|
x_np_weakref = weakref.ref(x_np)
|
|
|
|
del x_np
|
|
del x_jax
|
|
gc.collect()
|
|
|
|
assert x_np_weakref() is None
|
|
|
|
|
|
class OverrideLoweringTest(jtu.JaxTestCase):
|
|
|
|
def test_sharding_constraint_as_noop(self):
|
|
def f(x):
|
|
return jax.lax.with_sharding_constraint(
|
|
x, jax.sharding.SingleDeviceSharding(jax.devices()[0]))
|
|
|
|
def wsc_as_noop(ctx, operand, *args, **kwargs):
|
|
del ctx, args, kwargs
|
|
return [operand]
|
|
|
|
rules = ((jax.lax.sharding_constraint_p, wsc_as_noop),)
|
|
lowered_ir = (
|
|
jax.jit(f)
|
|
.trace(jax.ShapeDtypeStruct((2, 4), dtype=jnp.bfloat16))
|
|
.lower(_private_parameters=mlir.LoweringParameters(
|
|
override_lowering_rules=rules))
|
|
.as_text()
|
|
)
|
|
self.assertNotIn("stablehlo.custom_call @Sharding", lowered_ir)
|
|
|
|
|
|
if __name__ == '__main__':
|
|
absltest.main(testLoader=jtu.JaxTestLoader())
|