mirror of
https://github.com/ROCm/jax.git
synced 2025-04-25 04:56:06 +00:00
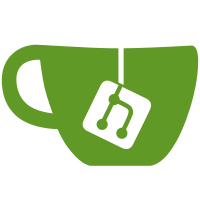
This adds support for shape polymorphism and export for this custom call, and adds the appropriate tests. One of the biggest changes here is to move all the lowing logic for the getrf call into jax (lax/linalg.py) instead of in jaxlib (gpu_solver.py and lapack.py) since the lowering code is now identical for CPU and GPU (the only difference is the handler names). PiperOrigin-RevId: 665829252
871 lines
28 KiB
Python
871 lines
28 KiB
Python
# Copyright 2018 The JAX Authors.
|
|
#
|
|
# Licensed under the Apache License, Version 2.0 (the "License");
|
|
# you may not use this file except in compliance with the License.
|
|
# You may obtain a copy of the License at
|
|
#
|
|
# https://www.apache.org/licenses/LICENSE-2.0
|
|
#
|
|
# Unless required by applicable law or agreed to in writing, software
|
|
# distributed under the License is distributed on an "AS IS" BASIS,
|
|
# WITHOUT WARRANTIES OR CONDITIONS OF ANY KIND, either express or implied.
|
|
# See the License for the specific language governing permissions and
|
|
# limitations under the License.
|
|
|
|
# Shims that allow the XLA CPU backend to call scipy-provided LAPACK kernels
|
|
# via CustomCallWithLayout.
|
|
|
|
from collections.abc import Sequence
|
|
from enum import Enum
|
|
from typing import Optional
|
|
|
|
import numpy as np
|
|
|
|
import jaxlib.mlir.ir as ir # pylint: disable=consider-using-from-import
|
|
import jaxlib.mlir.dialects.stablehlo as hlo
|
|
|
|
from jaxlib import xla_client
|
|
|
|
from .cpu import _lapack
|
|
from .hlo_helpers import (
|
|
custom_call, hlo_u8, hlo_s32,
|
|
ensure_hlo_s32, hlo_add, hlo_min,
|
|
DimensionSize, ShapeTypePair, mk_result_types_and_shapes,
|
|
)
|
|
|
|
for _name, _value in _lapack.registrations().items():
|
|
xla_client.register_custom_call_target(
|
|
_name,
|
|
_value,
|
|
platform="cpu",
|
|
api_version=(1 if _name.endswith("_ffi") else 0),
|
|
)
|
|
|
|
|
|
def _char_attr(c):
|
|
return ir.IntegerAttr.get(ir.IntegerType.get_unsigned(8), ord(c))
|
|
|
|
|
|
def _lapack_int_attr(value):
|
|
return ir.IntegerAttr.get(ir.IntegerType.get_signless(32), value)
|
|
|
|
|
|
def _enum_to_char_attr(e: Enum):
|
|
return ir.IntegerAttr.get(ir.IntegerType.get_unsigned(8), e.value)
|
|
|
|
|
|
def _matrix_side_attr(*, left_side: bool):
|
|
return _char_attr("L" if left_side else "R")
|
|
|
|
|
|
def _matrix_uplo_attr(*, lower: bool):
|
|
return _char_attr("L" if lower else "U")
|
|
|
|
|
|
def _matrix_transpose_attr(*, transpose: bool, conjugate: bool):
|
|
return _char_attr(("C" if conjugate else "T") if transpose else "N")
|
|
|
|
|
|
def _matrix_diagonal_attr(*, unit_diag: bool):
|
|
return _char_attr("U" if unit_diag else "N")
|
|
|
|
|
|
def _svd_computation_attr(
|
|
*, compute_uv: bool, full_matrices: Optional[bool] = True
|
|
):
|
|
mode = "A"
|
|
if full_matrices is None:
|
|
full_matrices = True
|
|
if not compute_uv:
|
|
# We should assert that `full_matrices` is never True here.
|
|
# This should never happen because `full_matrices` can only be computed when
|
|
# `compute_uv` is True. However, at this point there are too many tests that
|
|
# rely on this behavior.
|
|
mode = "N"
|
|
elif not full_matrices:
|
|
mode = "S"
|
|
return _char_attr(mode)
|
|
|
|
|
|
LAPACK_DTYPE_PREFIX = {
|
|
np.float32: "s",
|
|
np.float64: "d",
|
|
np.complex64: "c",
|
|
np.complex128: "z",
|
|
}
|
|
|
|
|
|
def prepare_lapack_call(fn_base, dtype):
|
|
"""Initializes the LAPACK library and returns the LAPACK target name."""
|
|
_lapack.initialize()
|
|
return build_lapack_fn_target(fn_base, dtype)
|
|
|
|
|
|
def build_lapack_fn_target(fn_base: str, dtype) -> str:
|
|
"""Builds the target name for a LAPACK function custom call."""
|
|
try:
|
|
prefix = (
|
|
LAPACK_DTYPE_PREFIX.get(dtype, None) or LAPACK_DTYPE_PREFIX[dtype.type]
|
|
)
|
|
return f"lapack_{prefix}{fn_base}"
|
|
except KeyError as err:
|
|
raise NotImplementedError(err, f"Unsupported dtype {dtype}.") from err
|
|
|
|
|
|
# TODO(phawkins): it would be nice to avoid duplicating code for each type.
|
|
|
|
# ?trsm(left_side, lower, trans_a, diag, m, n, alpha, a, b):
|
|
# triangular solve
|
|
def trsm_hlo(dtype, alpha, a, b,
|
|
left_side=False, lower=False, trans_a=False,
|
|
conj_a=False, diag=False, *,
|
|
b_shape_vals: tuple[DimensionSize, ...]):
|
|
_lapack.initialize()
|
|
b_type = ir.RankedTensorType(b.type)
|
|
|
|
m, n = b_shape_vals[-2:]
|
|
batch_dims_vals = b_shape_vals[:-2]
|
|
num_bd = len(batch_dims_vals)
|
|
batch_size_val = hlo_s32(1)
|
|
for b_v in batch_dims_vals:
|
|
batch_size_val = hlo.multiply(batch_size_val, ensure_hlo_s32(b_v))
|
|
|
|
if dtype == np.float32:
|
|
fn = "blas_strsm"
|
|
elif dtype == np.float64:
|
|
fn = "blas_dtrsm"
|
|
elif dtype == np.complex64:
|
|
fn = "blas_ctrsm"
|
|
elif dtype == np.complex128:
|
|
fn = "blas_ztrsm"
|
|
else:
|
|
raise NotImplementedError(f"Unsupported dtype {dtype}")
|
|
|
|
if conj_a and not trans_a:
|
|
raise NotImplementedError("Conjugation without transposition not supported")
|
|
scalar_layout = []
|
|
layout = (num_bd, num_bd + 1) + tuple(range(num_bd - 1, -1, -1))
|
|
result_types, result_shapes = mk_result_types_and_shapes(
|
|
[(b_shape_vals, b_type.element_type)])
|
|
return custom_call(
|
|
fn,
|
|
result_types=result_types,
|
|
operands=[hlo_s32(int(left_side)), hlo_s32(int(lower)),
|
|
hlo_s32((2 if conj_a else 1) if trans_a else 0), hlo_s32(int(diag)),
|
|
ensure_hlo_s32(m), ensure_hlo_s32(n), batch_size_val,
|
|
alpha, a, b],
|
|
operand_layouts=[scalar_layout] * 8 + [layout] * 2,
|
|
result_layouts=[layout],
|
|
operand_output_aliases={9: 0},
|
|
result_shapes=result_shapes,
|
|
).results
|
|
|
|
|
|
# # ?getrf: LU decomposition
|
|
|
|
def getrf_hlo(dtype, a: ir.Value, *, a_shape_vals: tuple[DimensionSize, ...]):
|
|
a_type = ir.RankedTensorType(a.type)
|
|
assert len(a_shape_vals) >= 2
|
|
batch_dims_vals = a_shape_vals[:-2]
|
|
num_bd = len(a_shape_vals) - 2
|
|
m, n = a_shape_vals[-2:]
|
|
fn = prepare_lapack_call(fn_base="getrf", dtype=dtype)
|
|
|
|
layout = (num_bd, num_bd + 1) + tuple(range(num_bd - 1, -1, -1))
|
|
|
|
i32_type = ir.IntegerType.get_signless(32)
|
|
shape_type_pairs: Sequence[ShapeTypePair] = [
|
|
(a_shape_vals, a_type.element_type),
|
|
(batch_dims_vals + (hlo_min(m, n),), i32_type),
|
|
(batch_dims_vals, i32_type)
|
|
]
|
|
result_types, result_shapes = mk_result_types_and_shapes(shape_type_pairs)
|
|
|
|
scalar_layout = []
|
|
batch_size_val = hlo_s32(1)
|
|
for b_v in batch_dims_vals:
|
|
batch_size_val = hlo.multiply(batch_size_val, ensure_hlo_s32(b_v))
|
|
|
|
return custom_call(
|
|
fn,
|
|
result_types=result_types,
|
|
operands=[batch_size_val, ensure_hlo_s32(m), ensure_hlo_s32(n), a],
|
|
operand_layouts=[scalar_layout] * 3 + [layout],
|
|
result_layouts=[
|
|
layout,
|
|
tuple(range(num_bd, -1, -1)),
|
|
tuple(range(num_bd - 1, -1, -1)),
|
|
],
|
|
operand_output_aliases={3: 0},
|
|
result_shapes=result_shapes,
|
|
).results
|
|
|
|
# # ?geqrf: QR decomposition
|
|
|
|
def geqrf_hlo(dtype, a: ir.Value, *,
|
|
a_shape_vals: tuple[DimensionSize, ...]):
|
|
_lapack.initialize()
|
|
a_type = ir.RankedTensorType(a.type)
|
|
assert len(a_shape_vals) >= 2
|
|
m, n = a_shape_vals[-2:]
|
|
assert type(m) is int
|
|
assert type(n) is int
|
|
|
|
batch_dims_vals = a_shape_vals[:-2]
|
|
num_bd = len(batch_dims_vals)
|
|
|
|
if dtype == np.float32:
|
|
fn = "lapack_sgeqrf"
|
|
lwork = _lapack.lapack_sgeqrf_workspace(m, n)
|
|
elif dtype == np.float64:
|
|
fn = "lapack_dgeqrf"
|
|
lwork = _lapack.lapack_dgeqrf_workspace(m, n)
|
|
elif dtype == np.complex64:
|
|
fn = "lapack_cgeqrf"
|
|
lwork = _lapack.lapack_cgeqrf_workspace(m, n)
|
|
elif dtype == np.complex128:
|
|
fn = "lapack_zgeqrf"
|
|
lwork = _lapack.lapack_zgeqrf_workspace(m, n)
|
|
else:
|
|
raise NotImplementedError(f"Unsupported dtype {dtype}")
|
|
|
|
scalar_layout = []
|
|
layout = (num_bd, num_bd + 1) + tuple(range(num_bd - 1, -1, -1))
|
|
i32_type = ir.IntegerType.get_signless(32)
|
|
|
|
batch_size_val = hlo_s32(1)
|
|
for b_v in batch_dims_vals:
|
|
batch_size_val = hlo.multiply(batch_size_val, ensure_hlo_s32(b_v))
|
|
shape_type_pairs: Sequence[ShapeTypePair] = [
|
|
(a_shape_vals, a_type.element_type),
|
|
(batch_dims_vals + (min(m, n),), a_type.element_type),
|
|
(batch_dims_vals, i32_type),
|
|
([lwork], a_type.element_type),
|
|
]
|
|
result_types, result_shapes = mk_result_types_and_shapes(shape_type_pairs)
|
|
out = custom_call(
|
|
fn,
|
|
result_types=result_types,
|
|
operands=[batch_size_val, hlo_s32(m), hlo_s32(n), hlo_s32(lwork), a],
|
|
operand_layouts=[scalar_layout] * 4 + [layout],
|
|
result_layouts=[
|
|
layout,
|
|
tuple(range(num_bd, -1, -1)),
|
|
tuple(range(num_bd - 1, -1, -1)),
|
|
[0],
|
|
],
|
|
operand_output_aliases={4: 0},
|
|
result_shapes=result_shapes,
|
|
).results
|
|
return out[:3]
|
|
|
|
|
|
# # ?orgqr: product of elementary Householder reflectors:
|
|
def orgqr_hlo(dtype, a: ir.Value, tau, *,
|
|
a_shape_vals: tuple[DimensionSize, ...],
|
|
tau_shape_vals: tuple[DimensionSize, ...]):
|
|
_lapack.initialize()
|
|
a_type = ir.RankedTensorType(a.type)
|
|
dims = a_type.shape
|
|
dims_vals = a_shape_vals
|
|
assert len(dims) >= 2
|
|
m, n = dims[-2:]
|
|
assert m != ir.ShapedType.get_dynamic_size()
|
|
assert n != ir.ShapedType.get_dynamic_size()
|
|
batch_dims_vals = dims_vals[:-2]
|
|
num_bd = len(batch_dims_vals)
|
|
batch_size_val = hlo_s32(1)
|
|
for b_v in batch_dims_vals:
|
|
batch_size_val = hlo.multiply(batch_size_val, ensure_hlo_s32(b_v))
|
|
|
|
k = tau_shape_vals[-1]
|
|
assert type(k) is int
|
|
|
|
if dtype == np.float32:
|
|
fn = "lapack_sorgqr"
|
|
lwork = _lapack.lapack_sorgqr_workspace(m, n, k)
|
|
elif dtype == np.float64:
|
|
fn = "lapack_dorgqr"
|
|
lwork = _lapack.lapack_dorgqr_workspace(m, n, k)
|
|
elif dtype == np.complex64:
|
|
fn = "lapack_cungqr"
|
|
lwork = _lapack.lapack_cungqr_workspace(m, n, k)
|
|
elif dtype == np.complex128:
|
|
fn = "lapack_zungqr"
|
|
lwork = _lapack.lapack_zungqr_workspace(m, n, k)
|
|
else:
|
|
raise NotImplementedError(f"Unsupported dtype {dtype}")
|
|
|
|
scalar_layout = []
|
|
layout = (num_bd, num_bd + 1) + tuple(range(num_bd - 1, -1, -1))
|
|
i32_type = ir.IntegerType.get_signless(32)
|
|
shape_type_pairs: Sequence[ShapeTypePair] = [
|
|
(a_shape_vals, a_type.element_type),
|
|
(batch_dims_vals, i32_type),
|
|
([lwork], a_type.element_type),
|
|
]
|
|
result_types, result_shapes = mk_result_types_and_shapes(shape_type_pairs)
|
|
out = custom_call(
|
|
fn,
|
|
result_types=result_types,
|
|
operands=[batch_size_val, hlo_s32(m), hlo_s32(n), hlo_s32(k),
|
|
hlo_s32(lwork), a, tau],
|
|
operand_layouts=[scalar_layout] * 5 + [
|
|
layout,
|
|
tuple(range(num_bd, -1, -1)),
|
|
],
|
|
result_layouts=[
|
|
layout,
|
|
tuple(range(num_bd - 1, -1, -1)),
|
|
[0],
|
|
],
|
|
operand_output_aliases={5: 0},
|
|
result_shapes=result_shapes,
|
|
).results
|
|
return out[:2]
|
|
|
|
|
|
# ?potrf: Cholesky decomposition
|
|
|
|
def potrf_hlo(ctx, dtype, a: ir.Value, *, lower=False,
|
|
a_shape_vals: tuple[DimensionSize, ...]):
|
|
a_type = ir.RankedTensorType(a.type)
|
|
fn_base = prepare_lapack_call(fn_base="potrf", dtype=dtype)
|
|
batch_dims_vals = a_shape_vals[:-2]
|
|
num_bd = len(batch_dims_vals)
|
|
layout = (num_bd, num_bd + 1) + tuple(range(num_bd - 1, -1, -1))
|
|
info_layout = tuple(range(num_bd - 1, -1, -1))
|
|
|
|
shape_type_pairs: Sequence[ShapeTypePair] = [
|
|
(a_shape_vals, a_type.element_type),
|
|
(batch_dims_vals, ir.IntegerType.get_signless(32))
|
|
]
|
|
result_types, result_shapes = mk_result_types_and_shapes(shape_type_pairs)
|
|
if ctx.is_forward_compat():
|
|
fn = fn_base
|
|
scalar_layout = []
|
|
n = a_shape_vals[-1]
|
|
batch_size_val = hlo_s32(1)
|
|
for b_v in batch_dims_vals:
|
|
batch_size_val = hlo.multiply(batch_size_val, ensure_hlo_s32(b_v))
|
|
out = custom_call(
|
|
fn,
|
|
result_types=result_types,
|
|
operands=[hlo_s32(int(lower)), batch_size_val, ensure_hlo_s32(n), a],
|
|
operand_layouts=[scalar_layout] * 3 + [layout],
|
|
result_layouts=[layout, info_layout],
|
|
operand_output_aliases={3: 0},
|
|
result_shapes=result_shapes,
|
|
).results
|
|
else:
|
|
fn = fn_base + "_ffi"
|
|
out = custom_call(
|
|
fn,
|
|
result_types=result_types,
|
|
operands=[a],
|
|
operand_layouts=[layout],
|
|
result_layouts=[layout, info_layout],
|
|
operand_output_aliases={0: 0},
|
|
result_shapes=result_shapes,
|
|
backend_config={
|
|
"uplo": _matrix_uplo_attr(lower=lower),
|
|
},
|
|
api_version=4,
|
|
).results
|
|
return out[:2]
|
|
|
|
|
|
# # ?gesdd: Singular value decomposition
|
|
|
|
def gesdd_hlo(ctx, dtype, a: ir.Value, *, full_matrices=True, compute_uv=True,
|
|
a_shape_vals: tuple[DimensionSize, ...]):
|
|
a_type = ir.RankedTensorType(a.type)
|
|
assert len(a_shape_vals) >= 2
|
|
m, n = a_shape_vals[-2:]
|
|
assert type(m) is int
|
|
assert type(n) is int
|
|
batch_dims_vals = a_shape_vals[:-2]
|
|
num_bd = len(batch_dims_vals)
|
|
fn_base = prepare_lapack_call(fn_base="gesdd", dtype=dtype)
|
|
i32_type = ir.IntegerType.get_signless(32)
|
|
workspace: list[ShapeTypePair]
|
|
|
|
# TODO(b/344892332): Remove the old kernel after the compatibility period.
|
|
if ctx.is_forward_compat():
|
|
fn = fn_base
|
|
batch_size_val = hlo_s32(1)
|
|
for b_v in batch_dims_vals:
|
|
batch_size_val = hlo.multiply(batch_size_val, ensure_hlo_s32(b_v))
|
|
if dtype == np.float32:
|
|
singular_vals_type = ir.F32Type.get()
|
|
lwork = _lapack.sgesdd_work_size(m, n, compute_uv, full_matrices)
|
|
workspace = [
|
|
([_lapack.gesdd_iwork_size(m, n)], i32_type),
|
|
([lwork], a_type.element_type),
|
|
]
|
|
workspace_layouts = [[0], [0]]
|
|
elif dtype == np.float64:
|
|
singular_vals_type = ir.F64Type.get()
|
|
lwork = _lapack.dgesdd_work_size(m, n, compute_uv, full_matrices)
|
|
workspace = [
|
|
([_lapack.gesdd_iwork_size(m, n)], i32_type),
|
|
([lwork], a_type.element_type),
|
|
]
|
|
workspace_layouts = [[0], [0]]
|
|
elif dtype == np.complex64:
|
|
singular_vals_type = ir.F32Type.get()
|
|
lwork = _lapack.cgesdd_work_size(m, n, compute_uv, full_matrices)
|
|
workspace = [
|
|
([_lapack.gesdd_iwork_size(m, n)], i32_type),
|
|
([_lapack.cgesdd_rwork_size(m, n, int(compute_uv))], ir.F32Type.get()),
|
|
([lwork], a_type.element_type),
|
|
]
|
|
workspace_layouts = [[0], [0], [0]]
|
|
elif dtype == np.complex128:
|
|
singular_vals_type = ir.F64Type.get()
|
|
lwork = _lapack.zgesdd_work_size(m, n, compute_uv, full_matrices)
|
|
workspace = [
|
|
([_lapack.gesdd_iwork_size(m, n)], i32_type),
|
|
([_lapack.cgesdd_rwork_size(m, n, int(compute_uv))], ir.F64Type.get()),
|
|
([lwork], a_type.element_type),
|
|
]
|
|
workspace_layouts = [[0], [0], [0]]
|
|
else:
|
|
raise NotImplementedError(f"Unsupported dtype {dtype}")
|
|
|
|
scalar_layout = []
|
|
layout = (num_bd, num_bd + 1) + tuple(range(num_bd - 1, -1, -1))
|
|
|
|
shape_type_pairs: Sequence[ShapeTypePair] = [
|
|
(a_shape_vals, a_type.element_type),
|
|
(batch_dims_vals + (min(m, n),), singular_vals_type),
|
|
(batch_dims_vals + (m, m if full_matrices else min(m, n)), a_type.element_type),
|
|
(batch_dims_vals + (n if full_matrices else min(m, n), n), a_type.element_type),
|
|
(batch_dims_vals, i32_type),
|
|
] + workspace
|
|
result_types, result_shapes = mk_result_types_and_shapes(shape_type_pairs)
|
|
return custom_call(
|
|
fn,
|
|
result_types=result_types,
|
|
operands=[hlo_s32(int(full_matrices)), hlo_s32(int(compute_uv)), batch_size_val,
|
|
hlo_s32(m), hlo_s32(n), hlo_s32(lwork), a],
|
|
operand_layouts=[scalar_layout] * 6 + [layout],
|
|
result_layouts=[
|
|
layout,
|
|
(num_bd,) + tuple(range(num_bd - 1, -1, -1)),
|
|
layout,
|
|
layout,
|
|
tuple(range(num_bd - 1, -1, -1)),
|
|
] + workspace_layouts,
|
|
operand_output_aliases={6: 0},
|
|
result_shapes=result_shapes
|
|
).results[1:5]
|
|
fn = fn_base + "_ffi"
|
|
mode_attr = _svd_computation_attr(
|
|
compute_uv=compute_uv, full_matrices=full_matrices
|
|
)
|
|
if dtype == np.float32 or dtype == np.complex64:
|
|
singular_vals_type = ir.F32Type.get()
|
|
elif dtype == np.float64 or dtype == np.complex128:
|
|
singular_vals_type = ir.F64Type.get()
|
|
else:
|
|
raise NotImplementedError(f"Unsupported dtype {dtype}")
|
|
|
|
layout = (num_bd, num_bd + 1) + tuple(range(num_bd - 1, -1, -1))
|
|
a_elem_type = a_type.element_type
|
|
shape_type_pairs: Sequence[ShapeTypePair] = [
|
|
(a_shape_vals, a_elem_type),
|
|
(batch_dims_vals + (min(m, n),), singular_vals_type),
|
|
(batch_dims_vals + (m, m if full_matrices else min(m, n)), a_elem_type),
|
|
(batch_dims_vals + (n if full_matrices else min(m, n), n), a_elem_type),
|
|
(batch_dims_vals, i32_type),
|
|
]
|
|
result_types, result_shapes = mk_result_types_and_shapes(shape_type_pairs)
|
|
return custom_call(
|
|
fn,
|
|
result_types=result_types,
|
|
operands=[a],
|
|
operand_layouts=[layout],
|
|
result_layouts=[
|
|
layout,
|
|
(num_bd,) + tuple(range(num_bd - 1, -1, -1)),
|
|
layout,
|
|
layout,
|
|
tuple(range(num_bd - 1, -1, -1)),
|
|
],
|
|
operand_output_aliases={0: 0},
|
|
result_shapes=result_shapes,
|
|
backend_config={
|
|
"mode": mode_attr,
|
|
},
|
|
api_version=4,
|
|
).results[1:]
|
|
|
|
|
|
# # syevd: Symmetric eigendecomposition
|
|
|
|
def syevd_hlo(dtype, a: ir.Value,
|
|
a_shape_vals: tuple[DimensionSize, ...],
|
|
lower=False):
|
|
_lapack.initialize()
|
|
a_type = ir.RankedTensorType(a.type)
|
|
assert len(a_shape_vals) >= 2
|
|
m, n = a_shape_vals[-2:]
|
|
# Non-batch dimensions must be static
|
|
assert type(m) is int and type(n) is int and m == n, a_shape_vals
|
|
|
|
batch_dims_vals = a_shape_vals[:-2]
|
|
num_bd = len(a_shape_vals) - 2
|
|
|
|
i32_type = ir.IntegerType.get_signless(32)
|
|
workspace: list[ShapeTypePair]
|
|
if dtype == np.float32:
|
|
fn = "lapack_ssyevd"
|
|
eigvals_type = ir.F32Type.get()
|
|
workspace = [
|
|
([_lapack.syevd_work_size(n)], a_type.element_type),
|
|
([_lapack.syevd_iwork_size(n)], i32_type),
|
|
]
|
|
elif dtype == np.float64:
|
|
fn = "lapack_dsyevd"
|
|
eigvals_type = ir.F64Type.get()
|
|
workspace = [
|
|
([_lapack.syevd_work_size(n)], a_type.element_type),
|
|
([_lapack.syevd_iwork_size(n)], i32_type),
|
|
]
|
|
elif dtype == np.complex64:
|
|
fn = "lapack_cheevd"
|
|
eigvals_type = ir.F32Type.get()
|
|
workspace = [
|
|
([_lapack.heevd_work_size(n)], a_type.element_type),
|
|
([_lapack.heevd_rwork_size(n)], eigvals_type),
|
|
([_lapack.syevd_iwork_size(n)], i32_type),
|
|
]
|
|
elif dtype == np.complex128:
|
|
fn = "lapack_zheevd"
|
|
eigvals_type = ir.F64Type.get()
|
|
workspace = [
|
|
([_lapack.heevd_work_size(n)], a_type.element_type),
|
|
([_lapack.heevd_rwork_size(n)], eigvals_type),
|
|
([_lapack.syevd_iwork_size(n)], i32_type),
|
|
]
|
|
else:
|
|
raise NotImplementedError(f"Unsupported dtype {dtype}")
|
|
|
|
batch_size_val = hlo_s32(1)
|
|
for b_v in batch_dims_vals:
|
|
batch_size_val = hlo.multiply(batch_size_val, ensure_hlo_s32(b_v))
|
|
|
|
scalar_layout = []
|
|
shape_layout = [0]
|
|
workspace_layouts = [shape_layout] * len(workspace)
|
|
layout = (num_bd, num_bd + 1) + tuple(range(num_bd - 1, -1, -1))
|
|
|
|
result_types, result_shapes = mk_result_types_and_shapes(
|
|
[(a_shape_vals, a_type.element_type),
|
|
(batch_dims_vals + (n,), eigvals_type),
|
|
(batch_dims_vals, i32_type)] + workspace
|
|
)
|
|
|
|
out = custom_call(
|
|
fn,
|
|
result_types=result_types,
|
|
operands=[hlo_s32(1 if lower else 0), batch_size_val, ensure_hlo_s32(n), a],
|
|
operand_layouts=[scalar_layout] * 3 + [layout],
|
|
result_layouts=[
|
|
layout,
|
|
tuple(range(num_bd, -1, -1)),
|
|
tuple(range(num_bd - 1, -1, -1)),
|
|
] + workspace_layouts,
|
|
operand_output_aliases={3: 0},
|
|
result_shapes=result_shapes,
|
|
).results
|
|
return out[:3]
|
|
|
|
|
|
# # geev: Nonsymmetric eigendecomposition (eig)
|
|
|
|
def geev_hlo(dtype, input, *,
|
|
input_shape_vals: tuple[DimensionSize, ...], # input.shape as ir.Values
|
|
jobvl=True, jobvr=True):
|
|
# input_shape_vals are used for when input has dynamic shapes.
|
|
_lapack.initialize()
|
|
input_shape = ir.RankedTensorType(input.type).shape
|
|
assert len(input_shape) >= 2
|
|
n = input_shape_vals[-1]
|
|
batch_dims_vals = input_shape_vals[:-2]
|
|
num_bd = len(batch_dims_vals)
|
|
|
|
layout = (num_bd, num_bd + 1) + tuple(range(num_bd - 1, -1, -1))
|
|
|
|
jobvl_c = ord('V' if jobvl else 'N')
|
|
jobvr_c = ord('V' if jobvr else 'N')
|
|
|
|
i32_type = ir.IntegerType.get_signless(32)
|
|
f32_type = ir.F32Type.get()
|
|
f64_type = ir.F64Type.get()
|
|
c64_type = ir.ComplexType.get(ir.F32Type.get())
|
|
c128_type = ir.ComplexType.get(ir.F64Type.get())
|
|
|
|
workspaces: list[ShapeTypePair]
|
|
eigvals: list[ShapeTypePair]
|
|
if dtype == np.float32:
|
|
fn = "lapack_sgeev"
|
|
real = True
|
|
eigvecs_type = c64_type
|
|
workspaces = [([n, n], f32_type)] * 3
|
|
workspace_layouts = [[0, 1]] * 3
|
|
eigvals = [(batch_dims_vals + (n,), f32_type)] * 2
|
|
eigvals_layouts = [tuple(range(num_bd, -1, -1))] * 2
|
|
elif dtype == np.float64:
|
|
fn = "lapack_dgeev"
|
|
real = True
|
|
eigvecs_type = c128_type
|
|
workspaces = [([n, n], f64_type)] * 3
|
|
workspace_layouts = [[0, 1]] * 3
|
|
eigvals = [(batch_dims_vals + (n,), f64_type)] * 2
|
|
eigvals_layouts = [tuple(range(num_bd, -1, -1))] * 2
|
|
elif dtype == np.complex64:
|
|
fn = "lapack_cgeev"
|
|
real = False
|
|
eigvecs_type = c64_type
|
|
workspaces = [([n, n], c64_type), ([hlo_add(n, n)], f32_type)]
|
|
workspace_layouts = [[0, 1], [0]]
|
|
eigvals = [(batch_dims_vals + (n,), c64_type)]
|
|
eigvals_layouts = [tuple(range(num_bd, -1, -1))]
|
|
elif dtype == np.complex128:
|
|
fn = "lapack_zgeev"
|
|
real = False
|
|
eigvecs_type = c128_type
|
|
workspaces = [([n, n], c128_type), ([hlo_add(n, n)], f64_type)]
|
|
workspace_layouts = [[0, 1], [0]]
|
|
eigvals = [(batch_dims_vals + (n,), c128_type)]
|
|
eigvals_layouts = [tuple(range(num_bd, -1, -1))]
|
|
else:
|
|
raise NotImplementedError(f"Unsupported dtype {dtype}")
|
|
|
|
scalar_layout = []
|
|
info_layout = tuple(range(num_bd - 1, -1, -1))
|
|
|
|
batch_size_val = hlo_s32(1)
|
|
for b_v in batch_dims_vals:
|
|
batch_size_val = hlo.multiply(batch_size_val, ensure_hlo_s32(b_v))
|
|
|
|
shape_type_pairs: Sequence[ShapeTypePair] = workspaces + eigvals + [
|
|
(input_shape_vals, eigvecs_type),
|
|
(input_shape_vals, eigvecs_type),
|
|
(batch_dims_vals, i32_type)]
|
|
result_types, result_shapes = mk_result_types_and_shapes(shape_type_pairs)
|
|
out = custom_call(
|
|
fn,
|
|
result_types=result_types,
|
|
operands=[batch_size_val, ensure_hlo_s32(n),
|
|
hlo_u8(jobvl_c),
|
|
hlo_u8(jobvr_c),
|
|
input],
|
|
operand_layouts=[scalar_layout] * 4 + [layout],
|
|
result_layouts=(workspace_layouts + eigvals_layouts + [layout] * 2 +
|
|
[info_layout]),
|
|
result_shapes=result_shapes,
|
|
).results
|
|
if real:
|
|
return (hlo.complex(out[3], out[4]), out[5], out[6], out[7])
|
|
else:
|
|
return out[2:6]
|
|
|
|
# # gees : Schur factorization
|
|
|
|
def gees_hlo(dtype, a, *, jobvs=True, sort=False, select=None,
|
|
a_shape_vals: tuple[DimensionSize, ...]):
|
|
_lapack.initialize()
|
|
a_type = ir.RankedTensorType(a.type)
|
|
etype = a_type.element_type
|
|
assert len(a_shape_vals) >= 2
|
|
n = a_shape_vals[-1]
|
|
batch_dims_vals = a_shape_vals[:-2]
|
|
num_bd = len(batch_dims_vals)
|
|
layout = (num_bd, num_bd + 1) + tuple(range(num_bd - 1, -1, -1))
|
|
|
|
if sort:
|
|
raise NotImplementedError(
|
|
"The sort feature of LAPACK's gees routine is not implemented.")
|
|
|
|
jobvs = ord('V' if jobvs else 'N')
|
|
sort = ord('S' if sort else 'N')
|
|
|
|
if dtype == np.float32:
|
|
fn = "lapack_sgees"
|
|
elif dtype == np.float64:
|
|
fn = "lapack_dgees"
|
|
elif dtype == np.complex64:
|
|
fn = "lapack_cgees"
|
|
elif dtype == np.complex128:
|
|
fn = "lapack_zgees"
|
|
else:
|
|
raise NotImplementedError(f"Unsupported dtype {dtype}")
|
|
|
|
workspaces: list[ShapeTypePair]
|
|
eigvals: list[ShapeTypePair]
|
|
if not np.issubdtype(dtype, np.complexfloating):
|
|
workspaces = [(a_shape_vals, etype)]
|
|
workspace_layouts = [layout]
|
|
eigvals = [(batch_dims_vals + (n,), etype)] * 2
|
|
eigvals_layouts = [tuple(range(num_bd, -1, -1))] * 2
|
|
else:
|
|
workspaces = [(a_shape_vals, etype),
|
|
([n], ir.ComplexType(etype).element_type),
|
|
]
|
|
workspace_layouts = [layout, [0]]
|
|
eigvals = [(batch_dims_vals + (n,), etype)]
|
|
eigvals_layouts = [tuple(range(num_bd, -1, -1))]
|
|
|
|
i32_type = ir.IntegerType.get_signless(32)
|
|
|
|
scalar_layout = []
|
|
batch_size_val = hlo_s32(1)
|
|
for b_v in batch_dims_vals:
|
|
batch_size_val = hlo.multiply(batch_size_val, ensure_hlo_s32(b_v))
|
|
shape_type_pairs = workspaces + eigvals + [
|
|
(a_shape_vals, etype),
|
|
(batch_dims_vals, i32_type),
|
|
(batch_dims_vals, i32_type)]
|
|
result_types, result_shapes = mk_result_types_and_shapes(shape_type_pairs)
|
|
out = custom_call(
|
|
fn,
|
|
result_types=result_types,
|
|
operands=[
|
|
batch_size_val,
|
|
ensure_hlo_s32(n),
|
|
hlo_u8(jobvs),
|
|
hlo_u8(sort),
|
|
# TODO: figure out how to put the callable select function here
|
|
a
|
|
],
|
|
operand_layouts=[scalar_layout] * 4 + [layout],
|
|
result_layouts=workspace_layouts + eigvals_layouts + [
|
|
layout,
|
|
tuple(range(num_bd - 1, -1, -1)),
|
|
tuple(range(num_bd - 1, -1, -1)),
|
|
],
|
|
operand_output_aliases={4: 0},
|
|
result_shapes=result_shapes,
|
|
).results
|
|
if sort == ord('S'):
|
|
return (out[0], out[3], out[4], out[5])
|
|
else:
|
|
return (out[0], out[3], out[5])
|
|
|
|
|
|
# gehrd: Reduction of a non-symmetric square matrix to upper Hessenberg form.
|
|
def gehrd_hlo(dtype, a):
|
|
_lapack.initialize()
|
|
a_type = ir.RankedTensorType(a.type)
|
|
dims = a_type.shape
|
|
assert len(dims) >= 2
|
|
m, n = dims[-2:]
|
|
assert m == n, (m, n)
|
|
batch_dims = tuple(dims[:-2])
|
|
num_bd = len(batch_dims)
|
|
b = 1
|
|
for d in batch_dims:
|
|
b *= d
|
|
|
|
if dtype == np.float32:
|
|
fn = "lapack_sgehrd"
|
|
lwork = _lapack.lapack_sgehrd_workspace(n, n, 1, n)
|
|
elif dtype == np.float64:
|
|
fn = "lapack_dgehrd"
|
|
lwork = _lapack.lapack_dgehrd_workspace(n, n, 1, n)
|
|
elif dtype == np.complex64:
|
|
fn = "lapack_cgehrd"
|
|
lwork = _lapack.lapack_cgehrd_workspace(n, n, 1, n)
|
|
elif dtype == np.complex128:
|
|
fn = "lapack_zgehrd"
|
|
lwork = _lapack.lapack_zgehrd_workspace(n, n, 1, n)
|
|
else:
|
|
raise NotImplementedError(f"Unsupported dtype {dtype}")
|
|
|
|
layout = (num_bd, num_bd + 1) + tuple(range(num_bd - 1, -1, -1))
|
|
i32_type = ir.IntegerType.get_signless(32)
|
|
out = custom_call(
|
|
fn,
|
|
result_types=[
|
|
a.type,
|
|
ir.RankedTensorType.get(batch_dims + (n - 1,), a_type.element_type),
|
|
ir.RankedTensorType.get(batch_dims, i32_type),
|
|
ir.RankedTensorType.get([lwork], a_type.element_type),
|
|
],
|
|
operands=[hlo_s32(n), hlo_s32(1), hlo_s32(n), hlo_s32(n), hlo_s32(b),
|
|
hlo_s32(lwork), a],
|
|
operand_layouts=[[]] * 6 + [layout],
|
|
result_layouts=[
|
|
layout,
|
|
(num_bd,) + tuple(range(num_bd - 1, -1, -1)),
|
|
tuple(range(num_bd - 1, -1, -1)),
|
|
[0],
|
|
],
|
|
operand_output_aliases={6: 0},
|
|
).results
|
|
return out[:3]
|
|
|
|
|
|
# sytrd: Reduction of a symmetric (Hermitian) matrix to tridiagonal form.
|
|
def sytrd_hlo(dtype, a, *, lower):
|
|
_lapack.initialize()
|
|
a_type = ir.RankedTensorType(a.type)
|
|
dims = a_type.shape
|
|
assert len(dims) >= 2
|
|
m, n = dims[-2:]
|
|
assert m == n, (m, n)
|
|
batch_dims = tuple(dims[:-2])
|
|
num_bd = len(batch_dims)
|
|
b = 1
|
|
for d in batch_dims:
|
|
b *= d
|
|
|
|
if dtype == np.float32:
|
|
fn = "lapack_ssytrd"
|
|
lwork = _lapack.lapack_ssytrd_workspace(n, n)
|
|
diag_type = a_type.element_type
|
|
elif dtype == np.float64:
|
|
fn = "lapack_dsytrd"
|
|
lwork = _lapack.lapack_dsytrd_workspace(n, n)
|
|
diag_type = a_type.element_type
|
|
elif dtype == np.complex64:
|
|
fn = "lapack_chetrd"
|
|
lwork = _lapack.lapack_chetrd_workspace(n, n)
|
|
diag_type = ir.F32Type.get()
|
|
elif dtype == np.complex128:
|
|
fn = "lapack_zhetrd"
|
|
lwork = _lapack.lapack_zhetrd_workspace(n, n)
|
|
diag_type = ir.F64Type.get()
|
|
else:
|
|
raise NotImplementedError(f"Unsupported dtype {dtype}")
|
|
|
|
layout = (num_bd, num_bd + 1) + tuple(range(num_bd - 1, -1, -1))
|
|
i32_type = ir.IntegerType.get_signless(32)
|
|
out = custom_call(
|
|
fn,
|
|
result_types=[
|
|
a.type,
|
|
ir.RankedTensorType.get(batch_dims + (n,), diag_type),
|
|
ir.RankedTensorType.get(batch_dims + (n - 1,), diag_type),
|
|
ir.RankedTensorType.get(batch_dims + (n - 1,), a_type.element_type),
|
|
ir.RankedTensorType.get(batch_dims, i32_type),
|
|
ir.RankedTensorType.get([lwork], a_type.element_type),
|
|
],
|
|
operands=[hlo_s32(n), hlo_s32(1 if lower else 0), hlo_s32(max(1, n)),
|
|
hlo_s32(b), hlo_s32(lwork), a],
|
|
operand_layouts=[[]] * 5 + [layout],
|
|
result_layouts=[
|
|
layout,
|
|
(num_bd,) + tuple(range(num_bd - 1, -1, -1)),
|
|
(num_bd,) + tuple(range(num_bd - 1, -1, -1)),
|
|
(num_bd,) + tuple(range(num_bd - 1, -1, -1)),
|
|
tuple(range(num_bd - 1, -1, -1)),
|
|
[0],
|
|
],
|
|
operand_output_aliases={5: 0},
|
|
).results
|
|
return out[:5]
|