mirror of
https://github.com/ROCm/jax.git
synced 2025-04-16 03:46:06 +00:00
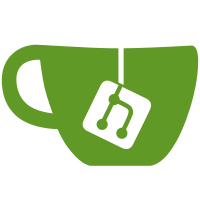
Most of the functionality is for the JAX native serialization case. This relies on newly added functionality to xla_extension.refine_polymorphic_shapes that handles custom calls @static_assertion. As a beneficial side-effect now we get shape constraint checking for jax2tf graph serialization when the resulting function is executed in graph mode.
2192 lines
90 KiB
Python
2192 lines
90 KiB
Python
# Copyright 2021 The JAX Authors.
|
|
#
|
|
# Licensed under the Apache License, Version 2.0 (the "License");
|
|
# you may not use this file except in compliance with the License.
|
|
# You may obtain a copy of the License at
|
|
#
|
|
# https://www.apache.org/licenses/LICENSE-2.0
|
|
#
|
|
# Unless required by applicable law or agreed to in writing, software
|
|
# distributed under the License is distributed on an "AS IS" BASIS,
|
|
# WITHOUT WARRANTIES OR CONDITIONS OF ANY KIND, either express or implied.
|
|
# See the License for the specific language governing permissions and
|
|
# limitations under the License.
|
|
|
|
# Lowering and execution path that converts jaxprs into MLIR.
|
|
from __future__ import annotations
|
|
|
|
import collections
|
|
import dataclasses
|
|
import functools
|
|
from functools import partial
|
|
import io
|
|
import itertools
|
|
import operator
|
|
import re
|
|
import typing
|
|
from typing import (Any, Callable, Iterator, NamedTuple, Optional,
|
|
Protocol, Sequence, Union)
|
|
import warnings
|
|
|
|
from jax._src import ad_util
|
|
from jax._src import core
|
|
from jax._src import dtypes
|
|
from jax._src import effects as effects_lib
|
|
from jax._src import linear_util as lu
|
|
from jax._src import pickle_util
|
|
from jax._src import sharding_impls
|
|
from jax._src import source_info_util
|
|
from jax._src import util
|
|
from jax._src import xla_bridge as xb
|
|
from jax._src.config import config
|
|
from jax._src.interpreters import partial_eval as pe
|
|
from jax._src.interpreters import xla
|
|
from jax._src.lib import xla_client as xc
|
|
from jax._src.lib import xla_extension
|
|
from jax._src.lib import xla_extension_version
|
|
from jax._src.lib.mlir import dialects
|
|
from jax._src.lib.mlir import ir
|
|
from jax._src.lib.mlir.dialects import func as func_dialect
|
|
from jax._src.lib.mlir.dialects import hlo
|
|
from jax._src.sharding_impls import XLACompatibleSharding
|
|
import numpy as np
|
|
|
|
|
|
map, unsafe_map = util.safe_map, map
|
|
zip, unsafe_zip = util.safe_zip, zip
|
|
|
|
T = typing.TypeVar("T")
|
|
|
|
Value = Any # = ir.Value
|
|
|
|
# mypy implicitly sets this variable to true when type checking.
|
|
MYPY = False
|
|
|
|
lowerable_effects: effects_lib.EffectTypeSet = effects_lib.lowerable_effects
|
|
|
|
|
|
# IR Helpers
|
|
|
|
def dense_int_elements(xs) -> ir.DenseIntElementsAttr:
|
|
return ir.DenseIntElementsAttr.get(np.asarray(xs, np.int64))
|
|
|
|
def dense_bool_elements(xs: Sequence[bool]) -> ir.DenseElementsAttr:
|
|
a = np.packbits(np.array(xs, np.bool_), bitorder='little')
|
|
# TODO(b/209005197): Work around for MLIR crash for non-splat single element
|
|
# buffers.
|
|
if len(xs) == 1:
|
|
a = np.array(0 if a.item() == 0 else 0xff, np.uint8)
|
|
return ir.DenseElementsAttr.get(
|
|
a, type=ir.IntegerType.get_signless(1), shape=[len(xs)])
|
|
|
|
def i32_attr(i): return ir.IntegerAttr.get(ir.IntegerType.get_signless(32), i)
|
|
def i64_attr(i): return ir.IntegerAttr.get(ir.IntegerType.get_signless(64), i)
|
|
|
|
def shape_tensor(sizes: Sequence[Union[int, ir.RankedTensorType]]
|
|
) -> ir.RankedTensorType:
|
|
int1d = aval_to_ir_type(core.ShapedArray((1,), np.int32))
|
|
i32_type = aval_to_ir_type(core.ShapedArray((), np.int32))
|
|
def lower_dim(d):
|
|
if type(d) is int:
|
|
return ir_constant(np.array([d], np.int32))
|
|
else:
|
|
if d.type != i32_type:
|
|
d = hlo.ConvertOp(i32_type, d)
|
|
return hlo.ReshapeOp(int1d, d).result
|
|
ds = map(lower_dim, sizes)
|
|
if not ds:
|
|
return ir_constant(np.array([], np.int32))
|
|
elif len(ds) == 1:
|
|
return ds[0]
|
|
else:
|
|
return hlo.ConcatenateOp(ds, i64_attr(0)).result
|
|
|
|
|
|
def delegate_lowering(ctx, lowering_fun, *args, **ctx_override_kwargs):
|
|
"""Side-effects on `ctx`"""
|
|
ctx_new = ctx.replace(**ctx_override_kwargs)
|
|
out = lowering_fun(ctx_new, *args)
|
|
ctx.set_tokens_out(ctx_new.tokens_out)
|
|
return out
|
|
|
|
|
|
# IR Types
|
|
|
|
# Non-canonicalized dtype to IR type mapping.
|
|
_dtype_to_ir_type : dict[np.dtype, Callable[[], ir.Type]] = {
|
|
np.dtype(dtypes.float0): partial(ir.IntegerType.get_signless, 1),
|
|
np.dtype(np.bool_): partial(ir.IntegerType.get_signless, 1),
|
|
np.dtype(np.int8): partial(ir.IntegerType.get_signless, 8),
|
|
np.dtype(np.int16): partial(ir.IntegerType.get_signless, 16),
|
|
np.dtype(np.int32): partial(ir.IntegerType.get_signless, 32),
|
|
np.dtype(np.int64): partial(ir.IntegerType.get_signless, 64),
|
|
np.dtype(np.uint8): partial(ir.IntegerType.get_unsigned, 8),
|
|
np.dtype(np.uint16): partial(ir.IntegerType.get_unsigned, 16),
|
|
np.dtype(np.uint32): partial(ir.IntegerType.get_unsigned, 32),
|
|
np.dtype(np.uint64): partial(ir.IntegerType.get_unsigned, 64),
|
|
np.dtype(dtypes.float8_e4m3b11fnuz): ir.Float8E4M3B11FNUZType.get,
|
|
np.dtype(dtypes.float8_e4m3fn): ir.Float8E4M3FNType.get,
|
|
np.dtype(dtypes.float8_e5m2): ir.Float8E5M2Type.get,
|
|
np.dtype(dtypes.bfloat16): ir.BF16Type.get,
|
|
np.dtype(np.float16): ir.F16Type.get,
|
|
np.dtype(np.float32): ir.F32Type.get,
|
|
np.dtype(np.float64): ir.F64Type.get,
|
|
np.dtype(np.complex64): lambda: ir.ComplexType.get(ir.F32Type.get()),
|
|
np.dtype(np.complex128): lambda: ir.ComplexType.get(ir.F64Type.get()),
|
|
}
|
|
|
|
if dtypes.int4 is not None:
|
|
_dtype_to_ir_type.update({
|
|
np.dtype(dtypes.int4): partial(ir.IntegerType.get_signless, 4),
|
|
np.dtype(dtypes.uint4): partial(ir.IntegerType.get_unsigned, 4),
|
|
})
|
|
|
|
|
|
def dtype_to_ir_type(dtype: Union[core.bint, np.dtype, np.generic]) -> ir.Type:
|
|
if isinstance(dtype, core.bint):
|
|
# TODO Support different-size underlying dtypes to take advantage of the
|
|
# bound for packing?
|
|
dtype = np.dtype(np.int32)
|
|
assert isinstance(dtype, (np.dtype, np.generic)), type(dtype)
|
|
dtype = np.dtype(dtype)
|
|
try:
|
|
ir_type_factory = _dtype_to_ir_type[dtype]
|
|
except KeyError as err:
|
|
raise TypeError(
|
|
f"No dtype_to_ir_type handler for dtype: {dtype}") from err
|
|
return ir_type_factory()
|
|
|
|
def _array_ir_types(aval: Union[core.ShapedArray, core.DShapedArray]
|
|
) -> Sequence[ir.Type]:
|
|
aval = core.physical_aval(aval) # type: ignore
|
|
if not core.is_constant_shape(aval.shape):
|
|
return _dynamic_array_ir_types(aval) # type: ignore
|
|
return (ir.RankedTensorType.get(aval.shape, dtype_to_ir_type(aval.dtype)),)
|
|
|
|
def _dynamic_array_ir_types(aval: core.ShapedArray) -> Sequence[ir.Type]:
|
|
dyn_size = ir.ShapedType.get_dynamic_size()
|
|
shape = [d if type(d) is int else dyn_size for d in aval.shape]
|
|
return (ir.RankedTensorType.get(shape, dtype_to_ir_type(aval.dtype)),)
|
|
|
|
ir_type_handlers: dict[type[core.AbstractValue],
|
|
Callable[[Any], Sequence[ir.Type]]] = {}
|
|
|
|
def aval_to_ir_types(aval: core.AbstractValue) -> Sequence[ir.Type]:
|
|
"""Converts a JAX aval to zero or more MLIR IR types.
|
|
|
|
In general, a JAX value may be represented by multiple IR values, so this
|
|
function returns multiple types."""
|
|
try:
|
|
return ir_type_handlers[type(aval)](aval)
|
|
except KeyError as err:
|
|
raise TypeError(f"No ir_type_handler for aval type: {type(aval)}") from err
|
|
|
|
ir_type_handlers[core.ShapedArray] = _array_ir_types
|
|
ir_type_handlers[core.ConcreteArray] = _array_ir_types
|
|
ir_type_handlers[core.AbstractToken] = lambda _: [hlo.TokenType.get()]
|
|
ir_type_handlers[core.DShapedArray] = _dynamic_array_ir_types
|
|
|
|
def aval_to_ir_type(aval: core.AbstractValue) -> ir.Type:
|
|
"""Convenience wrapper around aval_to_ir_types for single types.
|
|
|
|
For some common cases, e.g. dense arrays, we know JAX values are represented
|
|
by a single IR value."""
|
|
types = aval_to_ir_types(aval)
|
|
if len(types) != 1:
|
|
raise TypeError(f"aval_to_ir_type called on {aval} which corresponds to "
|
|
f"multiple IR types {types}")
|
|
return types[0]
|
|
|
|
|
|
# Constants
|
|
|
|
class ConstantHandler(Protocol):
|
|
def __call__(self, val: Any, canonicalize_types: bool) -> Sequence[ir.Value]:
|
|
"""Builds an IR representation for a constant `val`.
|
|
|
|
A JAX value is represented by zero or more IR values."""
|
|
|
|
_constant_handlers : dict[type, ConstantHandler] = {}
|
|
|
|
def register_constant_handler(type_: type, handler_fun: ConstantHandler):
|
|
_constant_handlers[type_] = handler_fun
|
|
|
|
def get_constant_handler(type_: type) -> ConstantHandler:
|
|
return _constant_handlers[type_]
|
|
|
|
def ir_constants(val: Any,
|
|
canonicalize_types: bool = True) -> Sequence[ir.Value]:
|
|
"""Translate a Python `val` to an IR constant, canonicalizing its dtype.
|
|
|
|
Args:
|
|
val: a Python value to be translated to a constant.
|
|
|
|
Returns:
|
|
A representation of the constant as a list of IR values.
|
|
"""
|
|
for t in type(val).__mro__:
|
|
handler = _constant_handlers.get(t)
|
|
if handler:
|
|
out = handler(val, canonicalize_types)
|
|
assert all(isinstance(v, ir.Value) for v in out), (type(val), out)
|
|
return out
|
|
if hasattr(val, '__jax_array__'):
|
|
return ir_constants(val.__jax_array__(), canonicalize_types)
|
|
raise TypeError(f"No constant handler for type: {type(val)}")
|
|
|
|
def ir_constant(val: Any, canonicalize_types: bool = True) -> ir.Value:
|
|
"""Convenience wrapper around ir_constants for singleton values."""
|
|
values = ir_constants(val, canonicalize_types=canonicalize_types)
|
|
if len(values) != 1:
|
|
raise TypeError(f"ir_constant called on {val} which corresponds to "
|
|
f"multiple IR values {values}")
|
|
return values[0]
|
|
|
|
|
|
def _numpy_array_constant(x: np.ndarray, canonicalize_types
|
|
) -> Sequence[ir.Value]:
|
|
if canonicalize_types:
|
|
x = np.asarray(x, dtypes.canonicalize_dtype(x.dtype))
|
|
element_type = dtype_to_ir_type(x.dtype)
|
|
shape = x.shape
|
|
if x.dtype == np.bool_:
|
|
nelems = x.size
|
|
x = np.packbits(x, bitorder='little')
|
|
# TODO(b/209005197): Work around for MLIR crash for non-splat single element
|
|
# buffers.
|
|
if nelems == 1:
|
|
x = np.array(0 if x.item() == 0 else 0xff, np.uint8)
|
|
elif x.dtype == dtypes.bfloat16:
|
|
x = x.view(np.uint16)
|
|
elif x.dtype in [dtypes.float8_e4m3b11fnuz, dtypes.float8_e4m3fn, dtypes.float8_e5m2]:
|
|
x = x.view(np.uint8)
|
|
x = np.ascontiguousarray(x)
|
|
attr = ir.DenseElementsAttr.get(x, type=element_type, shape=shape)
|
|
return (hlo.ConstantOp(attr).result,)
|
|
|
|
|
|
def _masked_array_constant_handler(*args, **kwargs):
|
|
raise ValueError("numpy masked arrays are not supported as direct inputs to JAX functions. "
|
|
"Use arr.filled() to convert the value to a standard numpy array.")
|
|
|
|
register_constant_handler(np.ma.MaskedArray, _masked_array_constant_handler)
|
|
|
|
def _ndarray_constant_handler(val: np.ndarray, canonicalize_types
|
|
) -> Sequence[ir.Value]:
|
|
"""Constant handler for ndarray literals, handling zero-size strides.
|
|
|
|
In most cases this function calls _numpy_array_constant(val) except it has
|
|
special handling of arrays with any strides of size zero: for those, it
|
|
generates appropriate calls to NumpyArrayConstant, Broadcast, and Transpose
|
|
to avoid staging in large literals that might arise from np.zeros or np.ones
|
|
or the output of lax.broadcast (which uses np.broadcast_to which in turn
|
|
uses size-zero strides).
|
|
|
|
Args:
|
|
val: an ndarray.
|
|
|
|
Returns:
|
|
An XLA ComputationDataHandle / XlaOp representing the constant ndarray
|
|
staged into the XLA Computation.
|
|
"""
|
|
if dtypes.result_type(val) == dtypes.float0:
|
|
return _numpy_array_constant(np.zeros(val.shape, dtype=np.bool_),
|
|
canonicalize_types=False)
|
|
elif np.any(np.equal(0, val.strides)) and val.size > 0:
|
|
zero_stride_axes, = np.where(np.equal(0, val.strides))
|
|
other_axes, = np.where(np.not_equal(0, val.strides))
|
|
collapsed_val = val[tuple(0 if ax in zero_stride_axes else slice(None) # type: ignore
|
|
for ax in range(val.ndim))] # type: ignore
|
|
if canonicalize_types:
|
|
collapsed_val = np.asarray(
|
|
collapsed_val, dtypes.canonicalize_dtype(collapsed_val.dtype))
|
|
out = hlo.BroadcastInDimOp(
|
|
ir.RankedTensorType.get(
|
|
val.shape, dtype_to_ir_type(collapsed_val.dtype)),
|
|
_numpy_array_constant(collapsed_val, canonicalize_types=False)[0],
|
|
dense_int_elements(other_axes)).result
|
|
return (out,)
|
|
else:
|
|
return _numpy_array_constant(val, canonicalize_types)
|
|
|
|
register_constant_handler(np.ndarray, _ndarray_constant_handler)
|
|
|
|
for _scalar_type in [np.int8, np.int16, np.int32, np.int64,
|
|
np.uint8, np.uint16, np.uint32, np.uint64,
|
|
np.float16, np.float32, np.float64,
|
|
np.complex64, np.complex128,
|
|
np.bool_, np.longlong, dtypes.bfloat16]:
|
|
register_constant_handler(_scalar_type, _ndarray_constant_handler) # type: ignore
|
|
|
|
def _python_scalar_handler(dtype, val, canonicalize_dtypes):
|
|
return _numpy_array_constant(np.array(val, dtype), canonicalize_dtypes)
|
|
|
|
for ptype, dtype in dtypes.python_scalar_dtypes.items():
|
|
register_constant_handler(ptype, partial(_python_scalar_handler, dtype))
|
|
|
|
def _token_constant_handler(val, canonicalize_types):
|
|
return [hlo.CreateTokenOp().result]
|
|
register_constant_handler(core.Token, _token_constant_handler)
|
|
|
|
# Source locations
|
|
|
|
def _traceback_to_location(tb: xc.Traceback) -> ir.Location:
|
|
"""Converts a full traceback to a callsite() MLIR location."""
|
|
frame_locs = []
|
|
for code, lasti in zip(*tb.raw_frames()):
|
|
frame = source_info_util.raw_frame_to_frame(code, lasti)
|
|
if source_info_util.is_user_filename(frame.file_name):
|
|
file_loc = ir.Location.file(
|
|
xla.get_canonical_source_file(frame),
|
|
frame.start_line,
|
|
frame.start_column,
|
|
)
|
|
name_loc = ir.Location.name(frame.function_name, childLoc=file_loc)
|
|
frame_locs.append(name_loc)
|
|
|
|
if len(frame_locs) == 0:
|
|
return ir.Location.unknown()
|
|
else:
|
|
if len(frame_locs) == 1:
|
|
return frame_locs[0]
|
|
|
|
return ir.Location.callsite(frame_locs[0], frame_locs[1:])
|
|
|
|
def _source_info_to_location(
|
|
primitive: core.Primitive, params: dict,
|
|
source_info: source_info_util.SourceInfo,
|
|
name_stack: source_info_util.NameStack) -> ir.Location:
|
|
eqn_str = (f'{str(source_info.name_stack)}/'
|
|
f'{core.str_eqn_compact(primitive.name, params)}')
|
|
if config.jax_include_full_tracebacks_in_locations:
|
|
if source_info.traceback is None:
|
|
loc = ir.Location.unknown()
|
|
else:
|
|
loc = _traceback_to_location(source_info.traceback)
|
|
else:
|
|
frame = source_info_util.user_frame(source_info)
|
|
if frame is None:
|
|
loc = ir.Location.unknown()
|
|
else:
|
|
loc = ir.Location.file(xla.get_canonical_source_file(frame),
|
|
frame.start_line, frame.start_column)
|
|
loc = ir.Location.name(eqn_str, childLoc=loc)
|
|
# TODO(phawkins): also include primitive.name as the operator type.
|
|
return loc
|
|
|
|
|
|
# Translation rules
|
|
def make_ir_context() -> ir.Context:
|
|
"""Creates an MLIR context suitable for JAX IR."""
|
|
context = ir.Context()
|
|
|
|
# If threading is enabled, each MLIR context will keep alive a thread pool.
|
|
# Since we cache MLIR modules (and hence contexts), this means we might keep
|
|
# several threads alive for each cache entry. This is a terrible idea. However
|
|
# we don't do any heavy computation on MLIR modules from Python anyway, so we
|
|
# just disable threading.
|
|
context.enable_multithreading(False)
|
|
|
|
dialects.mhlo.register_mhlo_dialect(context)
|
|
dialects.chlo.register_dialect(context)
|
|
dialects.hlo.register_dialect(context)
|
|
return context
|
|
|
|
|
|
AxisContext = Union[
|
|
sharding_impls.SPMDAxisContext,
|
|
sharding_impls.ReplicaAxisContext,
|
|
sharding_impls.ShardingContext,
|
|
]
|
|
|
|
class ShapePolyLoweringState:
|
|
# The names of the dimension variables, sorted by name. This is the order in
|
|
# which they are passed to the IR functions that need them. This is only
|
|
# used for native serialization with polymorphic shapes when
|
|
# --jax_dynamic_shapes is off.
|
|
dim_vars: Sequence[str]
|
|
# Whether the module uses dimension variables, either in its inputs or
|
|
# from an inner call to a polymorphic Exported.
|
|
uses_dim_vars: bool
|
|
|
|
def __init__(self, dim_vars: Sequence[str]):
|
|
self.dim_vars = dim_vars
|
|
self.uses_dim_vars = (len(dim_vars) > 0)
|
|
|
|
|
|
@dataclasses.dataclass
|
|
class ModuleContext:
|
|
"""Module-wide context information for MLIR lowering."""
|
|
context: ir.Context
|
|
module: ir.Module
|
|
ip: ir.InsertionPoint
|
|
symbol_table: ir.SymbolTable
|
|
backend_or_name: Optional[Union[str, xb.XlaBackend]]
|
|
platform: str
|
|
axis_context: AxisContext
|
|
name_stack: source_info_util.NameStack
|
|
keepalives: list[Any]
|
|
channel_iterator: Iterator[int]
|
|
host_callbacks: list[Any]
|
|
# Keep state for the lowering of shape polymorphism
|
|
shape_poly_state: ShapePolyLoweringState
|
|
|
|
# Cached primitive lowerings.
|
|
cached_primitive_lowerings: dict[Any, func_dialect.FuncOp]
|
|
cached_call_jaxpr_lowerings: dict[Any, func_dialect.FuncOp]
|
|
|
|
# A mapping between primitives and user-defined LoweringRules.
|
|
# When lowering a primitive, give priorioty to the rule in this map over
|
|
# existing Jax rules.
|
|
override_lowering_rules: Optional[tuple[tuple[core.Primitive, LoweringRule]]]
|
|
|
|
@property
|
|
def axis_env(self) -> sharding_impls.AxisEnv:
|
|
return self.axis_context.axis_env
|
|
|
|
def __init__(
|
|
self,
|
|
backend_or_name: Optional[Union[str, xb.XlaBackend]],
|
|
platform: str,
|
|
axis_context: AxisContext,
|
|
name_stack: source_info_util.NameStack,
|
|
keepalives: list[Any],
|
|
channel_iterator: Iterator[int],
|
|
host_callbacks: list[Any],
|
|
context: Optional[ir.Context] = None,
|
|
module: Optional[ir.Module] = None,
|
|
ip: Optional[ir.InsertionPoint] = None,
|
|
symbol_table: Optional[ir.SymbolTable] = None,
|
|
cached_primitive_lowerings: Optional[dict[Any,
|
|
func_dialect.FuncOp]] = None,
|
|
cached_call_jaxpr_lowerings: Optional[dict[Any,
|
|
func_dialect.FuncOp]] = None,
|
|
override_lowering_rules: Optional[
|
|
tuple[tuple[core.Primitive, LoweringRule]]] = None,
|
|
shape_poly_state = None):
|
|
assert platform is not None
|
|
self.context = context or make_ir_context()
|
|
self.module = module or ir.Module.create(loc=ir.Location.unknown(self.context))
|
|
self.ip = ip or ir.InsertionPoint(self.module.body)
|
|
self.symbol_table = symbol_table or ir.SymbolTable(self.module.operation)
|
|
self.backend_or_name = backend_or_name
|
|
self.platform = platform
|
|
self.axis_context = axis_context
|
|
self.name_stack = name_stack
|
|
self.cached_primitive_lowerings = ({} if cached_primitive_lowerings is None
|
|
else cached_primitive_lowerings)
|
|
self.channel_iterator = channel_iterator
|
|
self.keepalives = keepalives
|
|
self.host_callbacks = host_callbacks
|
|
self.cached_call_jaxpr_lowerings = ({}
|
|
if cached_call_jaxpr_lowerings is None
|
|
else cached_call_jaxpr_lowerings)
|
|
self.override_lowering_rules = override_lowering_rules
|
|
self.shape_poly_state = shape_poly_state or ShapePolyLoweringState(())
|
|
|
|
@property
|
|
def backend(self) -> xb.XlaBackend:
|
|
if self.backend_or_name is None or isinstance(self.backend_or_name, str):
|
|
return xb.get_backend(self.backend_or_name)
|
|
return self.backend_or_name
|
|
|
|
def new_channel(self) -> int:
|
|
return next(self.channel_iterator)
|
|
|
|
def add_host_callback(self, host_callback: Any) -> None:
|
|
self.host_callbacks.append(host_callback)
|
|
|
|
def add_keepalive(self, keepalive: Any) -> None:
|
|
self.keepalives.append(keepalive)
|
|
|
|
def replace(self, **kw): return dataclasses.replace(self, **kw)
|
|
|
|
|
|
@dataclasses.dataclass
|
|
class LoweringRuleContext:
|
|
"""Per-rule context information for MLIR lowering."""
|
|
module_context: ModuleContext
|
|
primitive: Optional[core.Primitive]
|
|
avals_in: Sequence[core.AbstractValue]
|
|
avals_out: Any # Usually Sequence[core.AbstractValue], but sometimes None.
|
|
tokens_in: TokenSet
|
|
tokens_out: Optional[TokenSet] # Mutable store for output containers
|
|
axis_size_env: Optional[dict[core.Var, ir.Value]] = None # Dynamic axis sizes
|
|
dim_var_values: Sequence[ir.Value] = () # The values for the dimension variables
|
|
# in same order as module_context.shape_poly_state.dim_vars
|
|
|
|
def set_tokens_out(self, tokens_out: TokenSet):
|
|
assert self.tokens_out is None, 'Should only set `tokens_out` once.'
|
|
self.tokens_out = tokens_out
|
|
|
|
def replace(self, **kw): return dataclasses.replace(self, **kw)
|
|
|
|
|
|
if not MYPY:
|
|
class LoweringRule(Protocol):
|
|
def __call__(self, ctx: LoweringRuleContext,
|
|
*args: Union[ir.Value, Sequence[ir.Value]],
|
|
**kw) -> Sequence[Union[ir.Value, Sequence[ir.Value]]]:
|
|
"""Converts a JAX primitive invocation into MLIR."""
|
|
else:
|
|
LoweringRule = Any
|
|
|
|
_lowerings: dict[core.Primitive, LoweringRule] = {}
|
|
_platform_specific_lowerings: dict[str, dict[core.Primitive, LoweringRule]]
|
|
_platform_specific_lowerings = collections.defaultdict(dict)
|
|
|
|
def register_lowering(prim: core.Primitive, rule: LoweringRule,
|
|
platform: Optional[str] = None):
|
|
if platform is None:
|
|
_lowerings[prim] = rule
|
|
else:
|
|
# For backward compatibility reasons, we allow rules to be registered
|
|
# under "gpu" even though the platforms are now called "cuda" and "rocm".
|
|
# TODO(phawkins): fix up users to specify either "cuda" or "rocm" and remove
|
|
# this expansion.
|
|
for p in xb.expand_platform_alias(platform):
|
|
_platform_specific_lowerings[p][prim] = rule
|
|
return rule
|
|
|
|
|
|
def _unwrap_singleton_ir_values(x): return x[0] if len(x) == 1 else x
|
|
def wrap_singleton_ir_values(x: Union[ir.Value, Sequence[ir.Value]]
|
|
) -> Sequence[ir.Value]:
|
|
"""Adds a consistent tuples to a mixture of tupled and untuple values."""
|
|
return (x,) if isinstance(x, ir.Value) else tuple(x)
|
|
|
|
def flatten_lowering_ir_args(
|
|
xs: Sequence[Union[ir.Value, Sequence[ir.Value]]]
|
|
) -> Sequence[Sequence[ir.Value]]:
|
|
return util.flatten(map(wrap_singleton_ir_values, xs))
|
|
|
|
_module_name_regex = re.compile(r"[^\w.-]")
|
|
|
|
def sharded_aval(aval: core.AbstractValue,
|
|
sharding: Optional[XLACompatibleSharding]) -> core.AbstractValue:
|
|
"""Returns the new aval sharded based on sharding proto."""
|
|
if sharding is None:
|
|
return aval
|
|
if isinstance(aval, core.AbstractToken):
|
|
return aval
|
|
if not isinstance(aval, (core.ShapedArray, core.DShapedArray)):
|
|
raise NotImplementedError
|
|
return aval.update(sharding.shard_shape(aval.shape))
|
|
|
|
|
|
def eval_dynamic_shape(ctx: LoweringRuleContext,
|
|
shape: core.Shape) -> tuple[Union[int, Value], ...]:
|
|
if config.jax_dynamic_shapes:
|
|
return tuple(ctx.axis_size_env.get(d, d) for d in shape) # type: ignore
|
|
else:
|
|
ctx = ctx.replace(
|
|
primitive="eval_dynamic_shape",
|
|
avals_in=[core.dim_value_aval()] * len(ctx.module_context.shape_poly_state.dim_vars))
|
|
|
|
res = lower_fun(
|
|
partial(core.evaluate_shape, shape, ctx.module_context.shape_poly_state.dim_vars),
|
|
multiple_results=True)(ctx, *ctx.dim_var_values)
|
|
return tuple(operator.index(d) if core.is_constant_dim(d) else d_ir
|
|
for d, d_ir in zip(shape, util.flatten(res))) # type: ignore
|
|
|
|
# TODO: replace usage of eval_dynamic_shape_as_vals with eval_dynamic_shape_as_ivals
|
|
def eval_dynamic_shape_as_vals(ctx: LoweringRuleContext,
|
|
shape: core.Shape) -> tuple[Value, ...]:
|
|
"""Evaluates the dynamic shapes as int32 values."""
|
|
def convert_dim(d: Union[int, Value]):
|
|
if type(d) is int:
|
|
return ir_constant(np.array(d, dtype=np.int32))
|
|
else:
|
|
i32_type = aval_to_ir_type(core.ShapedArray((), np.int32))
|
|
if d.type != i32_type: # type: ignore
|
|
return hlo.ConvertOp(i32_type, d).result
|
|
else:
|
|
return d
|
|
return tuple(convert_dim(v) for v in eval_dynamic_shape(ctx, shape))
|
|
|
|
|
|
def eval_dynamic_shape_as_ivals(
|
|
ctx: LoweringRuleContext, shape: core.Shape
|
|
) -> tuple[Union[int, Value], ...]:
|
|
"""Evaluates the dynamic shapes as int or ir.int32 values."""
|
|
def convert_dim(d: Union[int, Value]) -> Union[int, ir.Value]:
|
|
if type(d) is int:
|
|
return d
|
|
else:
|
|
i32_type = aval_to_ir_type(core.ShapedArray((), np.int32))
|
|
if d.type != i32_type: # type: ignore
|
|
return hlo.ConvertOp(i32_type, d).result
|
|
else:
|
|
return d
|
|
return tuple(convert_dim(v) for v in eval_dynamic_shape(ctx, shape))
|
|
|
|
def eval_dynamic_shape_as_tensor(ctx: LoweringRuleContext,
|
|
shape: core.Shape) -> Value:
|
|
"""Evaluates the dynamic shapes as one 1d int32 tensor."""
|
|
return shape_tensor(eval_dynamic_shape(ctx, shape))
|
|
|
|
class LoweringResult(NamedTuple):
|
|
module: ir.Module
|
|
keepalive: Optional[Any]
|
|
host_callbacks: list[Any]
|
|
shape_poly_state: ShapePolyLoweringState
|
|
|
|
|
|
_platforms_with_donation = ["cpu", "cuda", "rocm", "tpu"]
|
|
|
|
|
|
def _to_logical_op_sharding(
|
|
aval: core.AbstractValue, sharding: Optional[XLACompatibleSharding],
|
|
) -> Optional[xc.HloSharding]:
|
|
if sharding is None:
|
|
return None
|
|
assert isinstance(sharding, sharding_impls.XLACompatibleSharding)
|
|
assert isinstance(aval, (core.ShapedArray, core.DShapedArray))
|
|
return sharding._to_xla_hlo_sharding(aval.ndim)
|
|
|
|
|
|
def lower_jaxpr_to_module(
|
|
module_name: str,
|
|
jaxpr: core.ClosedJaxpr,
|
|
ordered_effects: list[core.Effect],
|
|
backend_or_name: Optional[Union[str, xb.XlaBackend]],
|
|
platform: str,
|
|
axis_context: AxisContext,
|
|
name_stack: source_info_util.NameStack,
|
|
donated_args: Sequence[bool],
|
|
replicated_args: Optional[Sequence[bool]] = None,
|
|
arg_shardings: Optional[Sequence[Optional[XLACompatibleSharding]]] = None,
|
|
result_shardings: Optional[Sequence[Optional[XLACompatibleSharding]]] = None,
|
|
arg_names: Optional[Sequence[Optional[str]]] = None,
|
|
result_names: Optional[Sequence[Optional[str]]] = None,
|
|
num_replicas: int = 1,
|
|
num_partitions: int = 1,
|
|
override_lowering_rules: Optional[
|
|
tuple[tuple[core.Primitive, LoweringRule]]] = None,
|
|
) -> LoweringResult:
|
|
"""Lowers a top-level jaxpr to an MLIR module.
|
|
|
|
Handles the quirks of the argument/return value passing conventions of the
|
|
runtime.
|
|
"""
|
|
platform = xb.canonicalize_platform(platform)
|
|
if not xb.is_known_platform(platform):
|
|
raise ValueError(f"Unknown platform {platform}")
|
|
input_output_aliases = None
|
|
in_avals = (jaxpr.in_avals if arg_shardings is None else
|
|
map(sharded_aval, jaxpr.in_avals, arg_shardings))
|
|
out_avals = (jaxpr.out_avals if result_shardings is None else
|
|
map(sharded_aval, jaxpr.out_avals, result_shardings))
|
|
if platform in _platforms_with_donation:
|
|
input_output_aliases, donated_args = _set_up_aliases(
|
|
in_avals, out_avals, donated_args)
|
|
unlowerable_effects = lowerable_effects.filter_not_in(jaxpr.effects)
|
|
if unlowerable_effects:
|
|
raise ValueError(f'Cannot lower jaxpr with effects: {jaxpr.effects}')
|
|
if any(donated_args):
|
|
unused_donations = [str(a) for a, d in zip(in_avals, donated_args) if d]
|
|
msg = "See an explanation at https://jax.readthedocs.io/en/latest/faq.html#buffer-donation."
|
|
if platform not in _platforms_with_donation:
|
|
msg = f"Donation is not implemented for {platform}.\n{msg}"
|
|
warnings.warn(f"Some donated buffers were not usable: {', '.join(unused_donations)}.\n{msg}")
|
|
|
|
# HLO channels need to start at 1
|
|
channel_iter = itertools.count(1)
|
|
# Create a keepalives list that will be mutated during the lowering.
|
|
keepalives: list[Any] = []
|
|
host_callbacks: list[Any] = []
|
|
|
|
dim_vars: Sequence[str]
|
|
if not config.jax_dynamic_shapes:
|
|
# Find the dimension variables
|
|
all_dim_poly = [d for aval in jaxpr.in_avals if hasattr(aval, "shape")
|
|
for d in aval.shape if not core.is_constant_dim(d)]
|
|
dim_vars = tuple(sorted(functools.reduce(lambda acc, new: acc.union(new.get_vars()),
|
|
all_dim_poly, set())))
|
|
else:
|
|
dim_vars = ()
|
|
|
|
arg_op_shardings = (
|
|
map(_to_logical_op_sharding, jaxpr.in_avals, arg_shardings)
|
|
if arg_shardings is not None else arg_shardings)
|
|
result_op_shardings = (
|
|
map(_to_logical_op_sharding, jaxpr.out_avals, result_shardings)
|
|
if result_shardings is not None else result_shardings)
|
|
|
|
ctx = ModuleContext(backend_or_name, platform, axis_context, name_stack,
|
|
keepalives, channel_iter, host_callbacks,
|
|
override_lowering_rules=override_lowering_rules,
|
|
shape_poly_state=ShapePolyLoweringState(dim_vars))
|
|
with ctx.context, ir.Location.unknown(ctx.context):
|
|
# Remove module name characters that XLA would alter. This ensures that
|
|
# XLA computation preserves the module name.
|
|
attrs = ctx.module.operation.attributes
|
|
module_name = _module_name_regex.sub("_", module_name)
|
|
attrs["sym_name"] = ir.StringAttr.get(module_name)
|
|
attrs["mhlo.num_replicas"] = i32_attr(num_replicas)
|
|
attrs["mhlo.num_partitions"] = i32_attr(num_partitions)
|
|
lower_jaxpr_to_fun(
|
|
ctx, "main", jaxpr, ordered_effects, public=True, create_tokens=True,
|
|
replace_tokens_with_dummy=True,
|
|
num_output_tokens=0,
|
|
replicated_args=replicated_args,
|
|
arg_shardings=arg_op_shardings,
|
|
result_shardings=result_op_shardings,
|
|
input_output_aliases=input_output_aliases,
|
|
arg_names=arg_names,
|
|
result_names=result_names)
|
|
|
|
try:
|
|
if not ctx.module.operation.verify():
|
|
module_string = module_to_string(ctx.module)
|
|
raise ValueError(
|
|
f"Cannot lower jaxpr with verifier errors: {module_string}")
|
|
except ir.MLIRError as e:
|
|
module_string = module_to_string(ctx.module)
|
|
raise ValueError(
|
|
f"Cannot lower jaxpr with verifier errors: {module_string}") from e
|
|
|
|
return LoweringResult(ctx.module, ctx.keepalives, ctx.host_callbacks,
|
|
ctx.shape_poly_state)
|
|
|
|
def module_to_string(module: ir.Module) -> str:
|
|
output = io.StringIO()
|
|
module.operation.print(file=output, enable_debug_info=True,
|
|
print_generic_op_form=False)
|
|
return output.getvalue()
|
|
|
|
def module_to_bytecode(module: ir.Module) -> bytes:
|
|
output = io.BytesIO()
|
|
module.operation.write_bytecode(file=output)
|
|
return output.getvalue()
|
|
|
|
|
|
def _set_up_aliases(avals_in, avals_out, donated_args):
|
|
input_output_aliases = [None] * len(avals_in)
|
|
# To match-up in-avals to out-avals we only care about the number of
|
|
# bytes, so we strip off unrelated aval metadata (eg. the named shape)
|
|
strip_metadata = lambda a: a.strip_named_shape().strip_weak_type()
|
|
avals_in = map(strip_metadata, avals_in)
|
|
avals_out = map(strip_metadata, avals_out)
|
|
|
|
donations = collections.defaultdict(collections.deque)
|
|
for i, (aval, donated) in enumerate(zip(avals_in, donated_args)):
|
|
if donated:
|
|
donations[aval].append(i)
|
|
|
|
out_donated_args = list(donated_args)
|
|
for i, aval in enumerate(avals_out):
|
|
if donations.get(aval, ()):
|
|
input_id = donations[aval].popleft()
|
|
input_output_aliases[input_id] = i
|
|
out_donated_args[input_id] = False
|
|
|
|
return input_output_aliases, out_donated_args
|
|
|
|
Token = Sequence[ir.Value]
|
|
|
|
def token_type() -> Sequence[ir.Type]:
|
|
return [hlo.TokenType.get()]
|
|
|
|
def create_token() -> Token:
|
|
return wrap_singleton_ir_values(hlo.CreateTokenOp().result)
|
|
|
|
class TokenSet:
|
|
"""An immutable container of tokens to be used to lower effectful jaxprs. When lowering
|
|
effectful jaxprs, we need to thread HLO tokens to sequence them. Each effect
|
|
will need its own token that will be threaded in and out of the effectful
|
|
primitives. A `TokenSet` encapsulates a set of HLO tokens that will be
|
|
used by the lowering rules.
|
|
"""
|
|
_tokens: typing.OrderedDict[core.Effect, Token]
|
|
|
|
def __init__(self, *args, **kwargs):
|
|
self._tokens = collections.OrderedDict(*args, **kwargs)
|
|
|
|
def __len__(self):
|
|
return len(self._tokens)
|
|
|
|
def get(self, effect: core.Effect) -> Token:
|
|
return self._tokens[effect]
|
|
|
|
@classmethod
|
|
def create(cls, effects: Sequence[core.Effect]) -> TokenSet:
|
|
"""Creates a `TokenSet` corresponding to a list of `core.Effect`s."""
|
|
tokens = [create_token() for _ in effects]
|
|
return TokenSet(zip(effects, tokens))
|
|
|
|
def items(self) -> Sequence[tuple[core.Effect, Token]]:
|
|
return tuple(self._tokens.items())
|
|
|
|
def effects(self) -> set[core.Effect]:
|
|
return set(self._tokens.keys())
|
|
|
|
def subset(self, effects: Sequence[core.Effect]) -> TokenSet:
|
|
"""Return a subset of the `TokenSet` restricted to a set of `core.Effect`s."""
|
|
return TokenSet((eff, self._tokens[eff]) for eff in effects)
|
|
|
|
def update_tokens(self, tokens: TokenSet) -> TokenSet:
|
|
"""Returns a new `TokenSet` with tokens replaced with ones from the input `TokenSet`."""
|
|
new_tokens = []
|
|
for eff in self.effects():
|
|
if eff in tokens._tokens:
|
|
new_tokens.append((eff, tokens._tokens[eff]))
|
|
else:
|
|
new_tokens.append((eff, self._tokens[eff]))
|
|
return TokenSet(new_tokens)
|
|
|
|
def dummy_token_type() -> Sequence[ir.Type]:
|
|
return aval_to_ir_types(core.ShapedArray((0,), np.bool_))
|
|
|
|
def dummy_token() -> Sequence[ir.Value]:
|
|
return ir_constants(np.zeros(0, np.bool_))
|
|
|
|
def lower_jaxpr_to_fun(
|
|
ctx: ModuleContext,
|
|
name: str,
|
|
jaxpr: core.ClosedJaxpr,
|
|
effects: Sequence[core.Effect],
|
|
*,
|
|
create_tokens: bool = False,
|
|
public: bool = False,
|
|
replace_tokens_with_dummy: bool = False,
|
|
replicated_args: Optional[Sequence[bool]] = None,
|
|
arg_shardings: Optional[Sequence[Optional[xc.HloSharding]]] = None,
|
|
result_shardings: Optional[Sequence[Optional[xc.HloSharding]]] = None,
|
|
use_sharding_annotations: bool = True,
|
|
input_output_aliases: Optional[Sequence[Optional[int]]] = None,
|
|
num_output_tokens: int = 0,
|
|
api_name: str = "jit",
|
|
arg_names: Optional[Sequence[Optional[str]]] = None,
|
|
result_names: Optional[Sequence[Optional[str]]] = None,
|
|
) -> func_dialect.FuncOp:
|
|
"""Lowers jaxpr and its callees to an IR function.
|
|
|
|
Assumes that an MLIR context, location, and insertion point are set.
|
|
|
|
Args:
|
|
ctx: the lowering context.
|
|
name: the function name. The name will be uniquified by the symbol table,
|
|
so it is ok to use the same name multiple times.
|
|
jaxpr: the jaxpr to lower.
|
|
effects: a sequence of `core.Effect`s corresponding to an ordering of tokens
|
|
that will be created in or used by the lowered function.
|
|
create_tokens: if true, the HLO will create tokens and ignore dummy input tokens.
|
|
public: if true, the function's visibility is set to "public".
|
|
replace_tokens_with_dummy: if true, token arguments/return values are
|
|
replaced with bool arrays of size [0].
|
|
replicated_args: if present, annotates arguments as replicated.
|
|
arg_shardings: sharding annotations for each argument (optional).
|
|
result_shardings: sharding annotations for each result (optional).
|
|
use_sharding_annotations: if True, use "mhlo.sharding" annotations on
|
|
parameters and return values to express sharding. If False, use
|
|
hlo.custom_call operators with sharding annotations.
|
|
TODO(b/228598865): remove this option when "mhlo.sharding" annotations are
|
|
propagated on non-entry functions during MLIR->HLO conversion.
|
|
input_output_aliases: optional sequence that maps argument numbers to the
|
|
corresponding output that should alias them.
|
|
api_name: The name of the higher level primitive which should show up in the
|
|
name stack.
|
|
Returns:
|
|
MLIR func op
|
|
"""
|
|
def aval_to_types(aval):
|
|
if replace_tokens_with_dummy and aval is core.abstract_token:
|
|
aval = core.ShapedArray((), np.dtype(np.bool_))
|
|
return aval_to_ir_types(aval)
|
|
|
|
num_dim_vars = len(ctx.shape_poly_state.dim_vars)
|
|
dim_var_avals = [core.ShapedArray((), dtypes.canonicalize_dtype(np.int64))] * num_dim_vars
|
|
dim_var_types = map(aval_to_types, dim_var_avals)
|
|
|
|
# Function inputs: *dim_var_values, *tokens, *actual_inputs
|
|
input_types = map(aval_to_types, jaxpr.in_avals)
|
|
output_types = map(aval_to_types, jaxpr.out_avals)
|
|
num_tokens = len(effects)
|
|
|
|
if create_tokens:
|
|
# If we create the tokens they won't be inputs to the MLIR function.
|
|
token_types = [dummy_token_type() for _ in effects]
|
|
output_token_types = [dummy_token_type() for _ in range(num_output_tokens)]
|
|
else:
|
|
# If we aren't creating tokens they will be the initial inputs to the
|
|
# MLIR function.
|
|
output_token_types = []
|
|
token_types = [token_type() for _ in effects]
|
|
token_avals = [core.AbstractToken] * num_tokens
|
|
# Order of arguments: dim vars, tokens, array inputs
|
|
input_avals = dim_var_avals + token_avals + jaxpr.in_avals
|
|
input_types = [*dim_var_types, *token_types, *input_types]
|
|
output_avals = [core.AbstractToken] * (len(output_token_types) + num_tokens) + jaxpr.out_avals
|
|
output_types = [*output_token_types, *token_types, *output_types]
|
|
if input_output_aliases is not None:
|
|
token_input_output_aliases = [None] * (num_dim_vars + num_tokens)
|
|
input_output_aliases = [*token_input_output_aliases, *input_output_aliases]
|
|
# Update the existing aliases to account for the new output values
|
|
input_output_aliases = [None if a is None
|
|
else a + num_output_tokens + num_tokens
|
|
for a in input_output_aliases]
|
|
if arg_shardings is not None:
|
|
token_shardings = [None] * (num_dim_vars + num_tokens)
|
|
arg_shardings = [*token_shardings, *arg_shardings]
|
|
if result_shardings is not None:
|
|
token_shardings = [None] * (num_tokens + num_output_tokens)
|
|
result_shardings = [*token_shardings, *result_shardings]
|
|
if replicated_args is not None:
|
|
token_replicated_args = [False] * (num_dim_vars + num_tokens)
|
|
replicated_args = [*token_replicated_args, *replicated_args]
|
|
flat_input_types = util.flatten(input_types)
|
|
flat_output_types = util.flatten(output_types)
|
|
ftype = ir.FunctionType.get(flat_input_types, flat_output_types)
|
|
func_op = func_dialect.FuncOp(name, ftype, ip=ctx.ip)
|
|
func_op.attributes["sym_visibility"] = ir.StringAttr.get(
|
|
"public" if public else "private")
|
|
ctx.symbol_table.insert(func_op)
|
|
ir_arg_shardings = None
|
|
if arg_shardings is not None:
|
|
in_avals = [None] * (num_dim_vars + num_tokens) + list(jaxpr.in_avals)
|
|
ir_arg_shardings = util.flatten(
|
|
[[_to_physical_op_sharding(a, s)] * len(types)
|
|
for a, s, types in zip(in_avals, arg_shardings, input_types)])
|
|
del in_avals
|
|
ir_result_shardings = None
|
|
if result_shardings is not None:
|
|
out_avals = [None] * (num_tokens + num_output_tokens) + list(jaxpr.out_avals)
|
|
ir_result_shardings = util.flatten(
|
|
[[_to_physical_op_sharding(a, s)] * len(types)
|
|
for a, s, types in zip(out_avals, result_shardings, output_types)])
|
|
del out_avals
|
|
|
|
if (
|
|
replicated_args is not None
|
|
or ir_arg_shardings is not None
|
|
or input_output_aliases is not None
|
|
or arg_names is not None
|
|
or num_tokens > 0
|
|
):
|
|
arg_attrs: list[dict[str, ir.Attribute]] = [
|
|
{} for _ in range(len(flat_input_types))]
|
|
|
|
if replicated_args is not None:
|
|
replicated_ir_args = [[replicated] * len(types) for replicated, types
|
|
in zip(replicated_args, input_types)]
|
|
for attrs, replicated in zip(arg_attrs, util.flatten(replicated_ir_args)):
|
|
if replicated:
|
|
attrs["mhlo.is_same_data_across_replicas"] = ir.UnitAttr.get()
|
|
|
|
if use_sharding_annotations and ir_arg_shardings is not None:
|
|
for attrs, sharding in zip(arg_attrs, ir_arg_shardings):
|
|
if sharding is not None:
|
|
attrs["mhlo.sharding"] = get_sharding_attr(sharding)
|
|
|
|
if input_output_aliases is not None:
|
|
output_ids = util.unflatten(list(range(len(flat_output_types))),
|
|
map(len, output_types))
|
|
aliases: list[Optional[int]] = []
|
|
for types, alias in zip(input_types, input_output_aliases):
|
|
if alias is None:
|
|
aliases.extend([None] * len(types))
|
|
else:
|
|
aliases.extend(output_ids[alias])
|
|
|
|
for attrs, alias in zip(arg_attrs, aliases):
|
|
if alias is not None:
|
|
attrs["tf.aliasing_output"] = i32_attr(alias)
|
|
|
|
if num_tokens > 0:
|
|
token_arg_attrs = arg_attrs[num_dim_vars:num_dim_vars + num_tokens]
|
|
for attrs in token_arg_attrs:
|
|
attrs["jax.token"] = ir.BoolAttr.get(True)
|
|
|
|
if arg_names:
|
|
named_arg_attrs = arg_attrs[num_dim_vars + num_tokens:]
|
|
for attrs, name_ in zip(named_arg_attrs, arg_names):
|
|
if name_:
|
|
attrs['jax.arg_info'] = ir.StringAttr.get(name_)
|
|
|
|
func_op.arg_attrs = ir.ArrayAttr.get(
|
|
[ir.DictAttr.get(attrs) for attrs in arg_attrs])
|
|
|
|
result_attrs: list[dict[str, ir.Attribute]] = [
|
|
{} for _ in range(len(flat_output_types))]
|
|
|
|
if num_tokens > 0:
|
|
token_result_attrs = result_attrs[:num_tokens]
|
|
for attrs in token_result_attrs:
|
|
attrs["jax.token"] = ir.BoolAttr.get(True)
|
|
|
|
if result_names:
|
|
named_result_attrs = result_attrs[num_tokens:]
|
|
if len(named_result_attrs) == len(result_names):
|
|
for attrs, name_ in zip(named_result_attrs, result_names):
|
|
attrs['jax.result_info'] = ir.StringAttr.get(name_)
|
|
|
|
if use_sharding_annotations and ir_result_shardings is not None:
|
|
for attrs, sharding in zip(result_attrs, ir_result_shardings):
|
|
if sharding is not None:
|
|
attrs['mhlo.sharding'] = get_sharding_attr(sharding)
|
|
|
|
func_op.result_attrs = ir.ArrayAttr.get(
|
|
[ir.DictAttr.get(attrs) for attrs in result_attrs])
|
|
|
|
entry_block = func_op.add_entry_block()
|
|
with ir.InsertionPoint(entry_block):
|
|
flat_args = entry_block.arguments
|
|
# We separate out the dimension variable inputs, the token inputs and
|
|
# the regular inputs. The dimension variables and token inputs
|
|
# will be passed to `jaxpr_subcomp` separately from the `args`.
|
|
dim_var_values, _, _ = util.split_list(flat_args, [num_dim_vars, num_tokens])
|
|
# A lowering context just for function body entry/exit code.
|
|
entry_lowering_ctx = LoweringRuleContext(
|
|
ctx, None, [], None, TokenSet.create([]), None, None, dim_var_values)
|
|
if not use_sharding_annotations and ir_arg_shardings is not None:
|
|
flat_args = [
|
|
a if s is None else wrap_with_sharding_op(entry_lowering_ctx, a, a_aval, s)
|
|
for a, s, a_aval in zip(flat_args, ir_arg_shardings, input_avals)]
|
|
|
|
_, token_args, unflattened_args = util.split_list(util.unflatten(flat_args, map(len, input_types)),
|
|
[num_dim_vars, num_tokens])
|
|
if create_tokens:
|
|
tokens_in = TokenSet.create(effects)
|
|
else:
|
|
tokens_in = TokenSet(zip(effects, token_args))
|
|
args: list[list[ir.Value]] = []
|
|
for aval, arg in zip(jaxpr.in_avals, unflattened_args):
|
|
if replace_tokens_with_dummy and aval is core.abstract_token:
|
|
args.append(hlo.CreateTokenOp().results)
|
|
else:
|
|
args.append(arg)
|
|
callee_name_stack = ctx.name_stack.extend(util.wrap_name(name, api_name))
|
|
out_vals, tokens_out = jaxpr_subcomp(ctx.replace(name_stack=callee_name_stack),
|
|
jaxpr.jaxpr, tokens_in, map(ir_constants, jaxpr.consts),
|
|
*args, dim_var_values=dim_var_values)
|
|
outs = []
|
|
if create_tokens:
|
|
for _ in range(num_output_tokens):
|
|
outs.append(dummy_token())
|
|
for _ in effects:
|
|
outs.append(dummy_token())
|
|
else:
|
|
for eff in effects:
|
|
outs.append(tokens_out.get(eff))
|
|
for aval, out in zip(jaxpr.out_avals, out_vals):
|
|
if replace_tokens_with_dummy and aval is core.abstract_token:
|
|
outs.append(ir_constants(np.zeros((), np.bool_)))
|
|
else:
|
|
outs.append(out)
|
|
flat_outputs = util.flatten(outs)
|
|
if not use_sharding_annotations and ir_result_shardings is not None:
|
|
flat_outputs = [
|
|
o if s is None else wrap_with_sharding_op(entry_lowering_ctx, o, o_aval, s)
|
|
for o, s, o_aval in zip(flat_outputs, ir_result_shardings, output_avals)]
|
|
|
|
func_dialect.ReturnOp(flat_outputs)
|
|
|
|
return func_op
|
|
|
|
|
|
def _to_physical_op_sharding(
|
|
aval: Optional[core.AbstractValue], sharding: Optional[xc.HloSharding]
|
|
) -> Optional[xc.OpSharding]:
|
|
if (isinstance(aval, core.ShapedArray) and dtypes.is_opaque_dtype(aval.dtype)
|
|
and sharding is not None):
|
|
return aval.dtype._rules.physical_hlo_sharding(aval, sharding).to_proto()
|
|
return None if sharding is None else sharding.to_proto() # type: ignore
|
|
|
|
|
|
def _emit_lowering_rule_as_fun(lowering_rule,
|
|
ctx: LoweringRuleContext) -> func_dialect.FuncOp:
|
|
"""Emits the contents of a lowering rule as a private function."""
|
|
num_dim_vars = len(ctx.module_context.shape_poly_state.dim_vars)
|
|
# TODO(necula) maybe only pass the dim_vars if they are needed?
|
|
dim_var_types = map(aval_to_ir_types, [core.ShapedArray((), dtypes.canonicalize_dtype(np.int64))] * num_dim_vars)
|
|
|
|
input_types = map(aval_to_ir_types, ctx.avals_in)
|
|
output_types = map(aval_to_ir_types, ctx.avals_out)
|
|
effs = list(ctx.tokens_in.effects())
|
|
token_types = [token_type() for _ in effs]
|
|
input_types = [*dim_var_types, *token_types, *input_types]
|
|
output_types = [*token_types, *output_types]
|
|
|
|
flat_input_types = util.flatten(input_types)
|
|
flat_output_types = util.flatten(output_types)
|
|
ftype = ir.FunctionType.get(flat_input_types, flat_output_types)
|
|
assert ctx.primitive is not None
|
|
func_op = func_dialect.FuncOp(ctx.primitive.name, ftype,
|
|
ip=ctx.module_context.ip)
|
|
func_op.attributes["sym_visibility"] = ir.StringAttr.get("private")
|
|
ctx.module_context.symbol_table.insert(func_op)
|
|
entry_block = func_op.add_entry_block()
|
|
with ir.InsertionPoint(entry_block):
|
|
unflattened_args = util.unflatten(entry_block.arguments,
|
|
map(len, input_types))
|
|
dim_var_values, token_args, unflattened_args = util.split_list(unflattened_args, [num_dim_vars, len(ctx.tokens_in)])
|
|
sub_ctx = ctx.replace(tokens_in=TokenSet(zip(effs, token_args)),
|
|
dim_var_values=dim_var_values)
|
|
outs = lowering_rule(sub_ctx, *_unwrap_singleton_ir_values(unflattened_args))
|
|
if sub_ctx.tokens_out:
|
|
outs = [*[sub_ctx.tokens_out.get(eff) for eff in effs], outs]
|
|
func_dialect.ReturnOp(util.flatten(map(wrap_singleton_ir_values, outs)))
|
|
return func_op
|
|
|
|
def jaxpr_subcomp(ctx: ModuleContext, jaxpr: core.Jaxpr,
|
|
tokens: TokenSet,
|
|
consts: Sequence[Sequence[ir.Value]],
|
|
*args: Sequence[ir.Value],
|
|
dim_var_values: Sequence[ir.Value]
|
|
) -> tuple[Sequence[Sequence[ir.Value]], TokenSet]:
|
|
"""Lowers a jaxpr into MLIR, inlined into an existing function.
|
|
|
|
Assumes that an MLIR context, location, and insertion point are set.
|
|
|
|
dim_var_values: the list of dimension variables values in the current
|
|
IR function, in the order of ctx.shape_poly_state.dim_vars.
|
|
"""
|
|
assert ctx.platform != "gpu"
|
|
def read(v: core.Atom) -> Sequence[ir.Value]:
|
|
if type(v) is core.Literal:
|
|
return ir_constants(v.val, canonicalize_types=True)
|
|
else:
|
|
assert isinstance(v, core.Var)
|
|
return env[v]
|
|
|
|
def aval(v: core.Atom) -> core.AbstractValue:
|
|
if type(v) is core.Literal:
|
|
return xla.abstractify(v.val)
|
|
else:
|
|
return v.aval
|
|
|
|
def write(v: core.Var, node: Sequence[ir.Value]):
|
|
assert node is not None
|
|
env[v] = tuple(node)
|
|
|
|
def get_lowering(primitive: core.Primitive) -> Optional[LoweringRule]:
|
|
if ctx.override_lowering_rules is None:
|
|
return None
|
|
for p, rule in ctx.override_lowering_rules:
|
|
if primitive is p:
|
|
return rule
|
|
return None
|
|
|
|
env: dict[core.Var, tuple[ir.Value, ...]] = {}
|
|
|
|
assert len(args) == len(jaxpr.invars), (jaxpr, args)
|
|
assert len(consts) == len(jaxpr.constvars), (jaxpr, consts)
|
|
assert all(isinstance(v, ir.Value) for vs in consts for v in vs), consts
|
|
assert len(ctx.shape_poly_state.dim_vars) == len(dim_var_values), (ctx.shape_poly_state.dim_vars, dim_var_values)
|
|
map(write, jaxpr.constvars, consts)
|
|
map(write, jaxpr.invars, args)
|
|
last_used = core.last_used(jaxpr)
|
|
for eqn in jaxpr.eqns:
|
|
in_nodes = map(read, eqn.invars)
|
|
assert isinstance(ctx.name_stack, source_info_util.NameStack), type(ctx.name_stack)
|
|
source_info = eqn.source_info.replace(
|
|
name_stack=ctx.name_stack + eqn.source_info.name_stack)
|
|
loc = _source_info_to_location(eqn.primitive, eqn.params, source_info,
|
|
ctx.name_stack)
|
|
with source_info_util.user_context(eqn.source_info.traceback), loc:
|
|
override_rule = get_lowering(eqn.primitive)
|
|
if override_rule is not None:
|
|
rule = override_rule
|
|
elif eqn.primitive in _platform_specific_lowerings[ctx.platform]:
|
|
rule = _platform_specific_lowerings[ctx.platform][eqn.primitive]
|
|
elif eqn.primitive in xla._backend_specific_translations[ctx.platform]:
|
|
rule = xla_fallback_lowering(eqn.primitive)
|
|
elif eqn.primitive in _lowerings:
|
|
rule = _lowerings[eqn.primitive]
|
|
elif eqn.primitive in xla._translations:
|
|
rule = xla_fallback_lowering(eqn.primitive)
|
|
else:
|
|
raise NotImplementedError(
|
|
f"MLIR translation rule for primitive '{eqn.primitive.name}' not "
|
|
f"found for platform {ctx.platform}")
|
|
|
|
eqn_ctx = ctx.replace(name_stack=source_info.name_stack)
|
|
effects = list(effects_lib.ordered_effects.filter_in(eqn.effects))
|
|
tokens_in = tokens.subset(effects)
|
|
avals_in = map(aval, eqn.invars)
|
|
rule_ctx = LoweringRuleContext(
|
|
module_context=eqn_ctx, primitive=eqn.primitive, avals_in=avals_in,
|
|
avals_out=map(aval, eqn.outvars), tokens_in=tokens_in,
|
|
tokens_out=None, dim_var_values=dim_var_values)
|
|
if config.jax_dynamic_shapes:
|
|
axis_size_env = {d: read(d)[0]
|
|
for a in avals_in if type(a) is core.DShapedArray
|
|
for d in a.shape if type(d) is core.Var}
|
|
rule_ctx = rule_ctx.replace(axis_size_env=axis_size_env)
|
|
ans = rule(rule_ctx, *map(_unwrap_singleton_ir_values, in_nodes),
|
|
**eqn.params)
|
|
if effects:
|
|
# If there were ordered effects in the primitive, there should be output
|
|
# tokens we need for subsequent ordered effects.
|
|
tokens_out = rule_ctx.tokens_out
|
|
if tokens_out is None:
|
|
raise ValueError(
|
|
f'Lowering rule for `{eqn.primitive}` needs to set `tokens_out` '
|
|
f'because it has effects: {eqn.effects}.')
|
|
if tokens_out.effects() != tokens_in.effects():
|
|
raise ValueError(
|
|
f'Lowering rule for `{eqn.primitive}` '
|
|
'returns incorrect set of output tokens. '
|
|
f'Expected: {tuple(tokens_in.effects())} vs. Actual: {tuple(tokens_out.effects())}')
|
|
tokens = tokens.update_tokens(tokens_out)
|
|
|
|
try:
|
|
out_nodes = tuple(map(wrap_singleton_ir_values, ans))
|
|
except TypeError as e:
|
|
raise ValueError("Output of translation rule must be iterable: "
|
|
f"{eqn}, got output {ans}") from e
|
|
|
|
assert all(isinstance(v, tuple) for v in out_nodes), (ans, eqn)
|
|
assert all(isinstance(v, ir.Value) for w in out_nodes for v in w), (
|
|
ans, "lowering function returned a bad output", eqn)
|
|
assert len(ans) == len(eqn.outvars), (ans, eqn)
|
|
map(write, eqn.outvars, out_nodes)
|
|
core.clean_up_dead_vars(eqn, env, last_used)
|
|
return map(read, jaxpr.outvars), tokens
|
|
|
|
def _ir_consts(consts):
|
|
unique_consts = {id(const): const for const in consts}
|
|
ir_consts = {
|
|
id_: ir_constants(const) for id_, const in unique_consts.items()}
|
|
return [ir_consts[id(const)] for const in consts]
|
|
|
|
def lower_fun(fun: Callable, multiple_results: bool = True) -> Callable:
|
|
"""Converts a traceable JAX function `fun` into a lowering rule.
|
|
|
|
The returned function does not use `avals_out`, so callers may pass any value
|
|
as `avals_out`."""
|
|
def f_lowered(ctx, *args, **params):
|
|
f = fun if multiple_results else lambda *args, **kw: (fun(*args, **kw),)
|
|
wrapped_fun = lu.wrap_init(f, params)
|
|
|
|
if config.jax_dynamic_shapes:
|
|
# We might be applying this function to arguments with dynamic shapes,
|
|
# i.e. there might be Vars in the shape tuples of ctx.avals_in. In that
|
|
# case, we need to form a jaxpr with leading binders for those axis size
|
|
# arguments (by computing an InputType and using trace_to_jaxpr_dynamic2),
|
|
# and we need to call jaxpr_subcomp with these arguments made explicit.
|
|
args = (*ctx.axis_size_env.values(), *args)
|
|
idx = {d: core.DBIdx(i) for i, d in enumerate(ctx.axis_size_env)}
|
|
i32_aval = core.ShapedArray((), np.dtype('int32'))
|
|
implicit_args = [(i32_aval, False)] * len(ctx.axis_size_env)
|
|
explicit_args = [(a.update(shape=tuple(idx.get(d, d) for d in a.shape))
|
|
if type(a) is core.DShapedArray else a, True)
|
|
for a in ctx.avals_in]
|
|
wrapped_fun = lu.annotate(wrapped_fun, (*implicit_args, *explicit_args))
|
|
jaxpr, _, consts = pe.trace_to_jaxpr_dynamic2(wrapped_fun)
|
|
else:
|
|
jaxpr, _, consts = pe.trace_to_jaxpr_dynamic(wrapped_fun, ctx.avals_in)
|
|
# TODO(frostig,mattjj): check ctx.avals_out against jaxpr avals out?
|
|
|
|
out, tokens = jaxpr_subcomp(
|
|
ctx.module_context, jaxpr, ctx.tokens_in, _ir_consts(consts),
|
|
*map(wrap_singleton_ir_values, args), dim_var_values=ctx.dim_var_values)
|
|
ctx.set_tokens_out(tokens)
|
|
return out
|
|
|
|
return f_lowered
|
|
|
|
|
|
def _lower_jaxpr_to_fun_cached(ctx, fn_name, call_jaxpr, effects,
|
|
arg_names=None, result_names=None):
|
|
if not call_jaxpr.consts and arg_names is result_names is None:
|
|
# Cacheable.
|
|
key = (fn_name, call_jaxpr.jaxpr, tuple(effects))
|
|
try:
|
|
func_op = ctx.cached_call_jaxpr_lowerings[key]
|
|
except KeyError:
|
|
func_op = lower_jaxpr_to_fun(
|
|
ctx, fn_name, call_jaxpr, effects, arg_names=arg_names,
|
|
result_names=result_names)
|
|
ctx.cached_call_jaxpr_lowerings[key] = func_op
|
|
else:
|
|
func_op = lower_jaxpr_to_fun(
|
|
ctx, fn_name, call_jaxpr, effects, arg_names=arg_names,
|
|
result_names=result_names)
|
|
return func_op
|
|
|
|
|
|
def _call_lowering(fn_name, stack_name, call_jaxpr, backend, ctx, avals_in,
|
|
avals_out, tokens_in, *args,
|
|
dim_var_values: Sequence[ir.Value],
|
|
arg_names=None, result_names=None):
|
|
if isinstance(call_jaxpr, core.Jaxpr):
|
|
call_jaxpr = core.ClosedJaxpr(call_jaxpr, ())
|
|
xla.check_backend_matches(backend, ctx.platform)
|
|
effects = list(tokens_in.effects())
|
|
output_types = map(aval_to_ir_types, avals_out)
|
|
output_types = [token_type()] * len(effects) + output_types
|
|
flat_output_types = util.flatten(output_types)
|
|
symbol_name = _lower_jaxpr_to_fun_cached(
|
|
ctx, fn_name, call_jaxpr, effects, arg_names=arg_names,
|
|
result_names=result_names).name.value
|
|
tokens = [tokens_in.get(eff) for eff in effects]
|
|
args = tuple([*dim_var_values, *tokens, *args])
|
|
call = func_dialect.CallOp(flat_output_types,
|
|
ir.FlatSymbolRefAttr.get(symbol_name),
|
|
flatten_lowering_ir_args(args))
|
|
out_nodes = util.unflatten(call.results, map(len, output_types))
|
|
tokens, out_nodes = util.split_list(out_nodes, [len(effects)])
|
|
tokens_out = tokens_in.update_tokens(TokenSet(zip(effects, tokens)))
|
|
return out_nodes, tokens_out
|
|
|
|
def core_call_lowering(ctx, *args, name, backend=None, call_jaxpr):
|
|
out_nodes, tokens = _call_lowering(
|
|
name, name, call_jaxpr, backend, ctx.module_context,
|
|
ctx.avals_in, ctx.avals_out, ctx.tokens_in, *args,
|
|
dim_var_values=ctx.dim_var_values)
|
|
ctx.set_tokens_out(tokens)
|
|
return out_nodes
|
|
|
|
register_lowering(core.call_p, partial(core_call_lowering, name="core_call"))
|
|
register_lowering(core.closed_call_p,
|
|
partial(core_call_lowering, name="core_closed_call"))
|
|
|
|
def broadcast_in_dim(ctx: LoweringRuleContext, op, aval_out: core.AbstractValue, *,
|
|
broadcast_dimensions) -> ir.Value:
|
|
# broadcast_dimension[i] is the axis of the result where the axis i of
|
|
# op is broadcast.
|
|
# Lower a possibly-dynamic broadcast_in_dim
|
|
if dtypes.is_opaque_dtype(aval_out.dtype): # type: ignore
|
|
elt_shape = aval_out.dtype._rules.physical_element_aval( # type: ignore
|
|
aval_out.dtype).shape # type: ignore
|
|
trailing_dims = [aval_out.ndim + i for i in range(len(elt_shape))] # type: ignore
|
|
broadcast_dimensions = [*broadcast_dimensions, *trailing_dims]
|
|
physical_aval_out = core.physical_aval(aval_out)
|
|
return broadcast_in_dim(
|
|
ctx, op, physical_aval_out, broadcast_dimensions=broadcast_dimensions)
|
|
else:
|
|
if not core.is_constant_shape(aval_out.shape): # type: ignore
|
|
shape = eval_dynamic_shape_as_tensor(ctx, aval_out.shape) # type: ignore
|
|
return hlo.DynamicBroadcastInDimOp(
|
|
aval_to_ir_type(aval_out), op,
|
|
shape,
|
|
dense_int_elements(broadcast_dimensions),
|
|
).result
|
|
else:
|
|
assert all(d != ir.ShapedType.get_dynamic_size()
|
|
for d in aval_out.shape), aval_out # type: ignore
|
|
return hlo.BroadcastInDimOp(
|
|
aval_to_ir_type(aval_out), op,
|
|
dense_int_elements(broadcast_dimensions)).result
|
|
|
|
def multi_broadcast_in_dim(ctx: LoweringRuleContext,
|
|
ops: Sequence[ir.Value],
|
|
ops_avals: Sequence[core.AbstractValue],
|
|
out_shape: core.Shape) -> Sequence[ir.Value]:
|
|
"""Broadcasts multiple ops to the out_shape."""
|
|
out = []
|
|
for op, op_aval in zip(ops, ops_avals):
|
|
op_aval_shape = op_aval.shape # type: ignore
|
|
if core.definitely_equal_shape(op_aval_shape, out_shape): # type: ignore
|
|
out.append(op)
|
|
else:
|
|
assert len(op_aval_shape) <= len(out_shape), (op_aval_shape, out_shape)
|
|
broadcast_dimensions = list(range(len(out_shape) - len(op_aval_shape), len(out_shape)))
|
|
out.append(broadcast_in_dim(ctx, op,
|
|
core.ShapedArray(out_shape, op_aval.dtype), # type: ignore
|
|
broadcast_dimensions=broadcast_dimensions))
|
|
return out
|
|
|
|
def reshape(ctx: LoweringRuleContext, op, aval_out: core.AbstractValue) -> ir.Value:
|
|
aval_out = core.physical_aval(aval_out)
|
|
if not core.is_constant_shape(aval_out.shape): # type: ignore
|
|
shape = eval_dynamic_shape_as_tensor(ctx, aval_out.shape) # type: ignore
|
|
return hlo.DynamicReshapeOp(
|
|
aval_to_ir_type(aval_out), op, shape,
|
|
).result
|
|
else:
|
|
return hlo.ReshapeOp(aval_to_ir_type(aval_out), op).result
|
|
|
|
def slice_op(ctx: LoweringRuleContext, x, aval_out, *,
|
|
start_indices, limit_indices, strides) -> ir.Value:
|
|
if dtypes.is_opaque_dtype(aval_out.dtype):
|
|
elt_shape = aval_out.dtype._rules.physical_element_aval(
|
|
aval_out.dtype).shape
|
|
trailing_zeros = [0] * len(elt_shape)
|
|
trailing_ones = [1] * len(elt_shape)
|
|
start_indices = (*start_indices, *trailing_zeros)
|
|
limit_indices = (*limit_indices, *elt_shape)
|
|
strides = (*strides, *trailing_ones)
|
|
physical_aval_out = core.physical_aval(aval_out)
|
|
return slice_op(ctx, x, physical_aval_out, start_indices=start_indices,
|
|
limit_indices=limit_indices, strides=strides)
|
|
else:
|
|
if any(not core.is_constant_shape(s) for s in (start_indices, limit_indices, strides)):
|
|
start_indices = eval_dynamic_shape_as_tensor(ctx, start_indices)
|
|
limit_indices = eval_dynamic_shape_as_tensor(ctx, limit_indices)
|
|
strides = eval_dynamic_shape_as_tensor(ctx, strides)
|
|
return hlo.RealDynamicSliceOp(
|
|
aval_to_ir_type(aval_out),
|
|
x, start_indices, limit_indices, strides).result
|
|
else:
|
|
return hlo.SliceOp(x,
|
|
dense_int_elements(start_indices),
|
|
dense_int_elements(limit_indices),
|
|
dense_int_elements(strides)).result
|
|
|
|
def dynamic_slice(ctx: LoweringRuleContext, aval_out, x, *,
|
|
start_indices) -> ir.Value:
|
|
x_aval = ctx.avals_in[0]
|
|
if dtypes.is_opaque_dtype(aval_out.dtype):
|
|
elt_shape = aval_out.dtype._rules.physical_element_aval(
|
|
aval_out.dtype).shape
|
|
index_avals = ctx.avals_in[1:]
|
|
dtype = dtypes.canonicalize_dtype(
|
|
index_avals[0].dtype if index_avals else 'int64') # type: ignore
|
|
trailing_zeros = [ir_constant(np.array(0, dtype))] * len(elt_shape)
|
|
start_indices = (*start_indices, *trailing_zeros)
|
|
aval_out = core.physical_aval(aval_out)
|
|
x_aval = core.physical_aval(x_aval)
|
|
|
|
slice_sizes = aval_out.shape
|
|
if not core.is_constant_shape(slice_sizes):
|
|
# lax.dynamic_slice clamps the start indices, but we are going to
|
|
# lower to RealDynamicSliceOp, which is a version of SliceOp, and does
|
|
# not have the clamping behavior. We clamp start ourselves.
|
|
slice_sizes = eval_dynamic_shape_as_tensor(ctx, slice_sizes)
|
|
clamped_start = hlo.ClampOp(
|
|
shape_tensor([0] * len(start_indices)),
|
|
shape_tensor(start_indices),
|
|
hlo.SubtractOp(
|
|
eval_dynamic_shape_as_tensor(ctx, x_aval.shape), # type: ignore
|
|
slice_sizes))
|
|
return hlo.RealDynamicSliceOp(
|
|
aval_to_ir_type(aval_out), x,
|
|
clamped_start,
|
|
hlo.AddOp(clamped_start, slice_sizes).result,
|
|
shape_tensor([1] * len(start_indices))
|
|
).result
|
|
else:
|
|
return hlo.DynamicSliceOp(x, start_indices,
|
|
dense_int_elements(slice_sizes)).result
|
|
|
|
def dynamic_update_slice(ctx: LoweringRuleContext, aval_out, x, update, *,
|
|
start_indices) -> ir.Value:
|
|
if dtypes.is_opaque_dtype(aval_out.dtype):
|
|
elt_shape = aval_out.dtype._rules.physical_element_aval(
|
|
aval_out.dtype).shape
|
|
index_avals = ctx.avals_in[2:]
|
|
dtype = dtypes.canonicalize_dtype(
|
|
index_avals[0].dtype if index_avals else 'int64') # type: ignore
|
|
zeros = [ir_constant(np.array(0, dtype=dtype))] * len(elt_shape)
|
|
start_indices = (*start_indices, *zeros)
|
|
physical_aval_out = core.physical_aval(aval_out)
|
|
return dynamic_update_slice(ctx, physical_aval_out, x, update,
|
|
start_indices=start_indices)
|
|
else:
|
|
# TODO(necula): handle dynamic shapes
|
|
return hlo.DynamicUpdateSliceOp(x, update, start_indices).result
|
|
|
|
def pad(ctx: LoweringRuleContext, aval_out,
|
|
x, padding_value,
|
|
padding_low, padding_high, padding_interior) -> ir.Value:
|
|
if all(core.is_constant_shape(s) for s in (padding_low,
|
|
padding_high, padding_interior)):
|
|
return hlo.PadOp(x, padding_value,
|
|
dense_int_elements(padding_low),
|
|
dense_int_elements(padding_high),
|
|
dense_int_elements(padding_interior)).result
|
|
else:
|
|
padding_low = eval_dynamic_shape_as_tensor(ctx, padding_low)
|
|
padding_high = eval_dynamic_shape_as_tensor(ctx, padding_high)
|
|
padding_interior = eval_dynamic_shape_as_tensor(ctx, padding_interior)
|
|
return hlo.DynamicPadOp(
|
|
aval_to_ir_type(aval_out),
|
|
x, padding_value, padding_low, padding_high, padding_interior).result
|
|
|
|
def iota(ctx: LoweringRuleContext, aval_out, *, dimension: int):
|
|
if not core.is_constant_shape(aval_out.shape):
|
|
shape = eval_dynamic_shape_as_tensor(ctx, aval_out.shape)
|
|
return hlo.DynamicIotaOp(
|
|
aval_to_ir_type(aval_out),
|
|
shape,
|
|
i64_attr(dimension),
|
|
).result
|
|
else:
|
|
return hlo.IotaOp(aval_to_ir_type(aval_out),
|
|
i64_attr(dimension)).result
|
|
|
|
def full_like_aval(ctx: LoweringRuleContext, value, aval: core.ShapedArray) -> ir.Value:
|
|
"""Returns an IR constant shaped full of `value` shaped like `aval`."""
|
|
zero = ir_constant(np.array(value, aval.dtype))
|
|
return broadcast_in_dim(ctx, zero, aval, broadcast_dimensions=())
|
|
|
|
def zeros_like_lowering(ctx, x):
|
|
aval, = ctx.avals_in
|
|
assert isinstance(aval, core.ShapedArray), aval
|
|
return [full_like_aval(ctx, 0, aval)]
|
|
register_lowering(ad_util.zeros_like_p, zeros_like_lowering)
|
|
|
|
def add_jaxvals_lowering(ctx, x, y):
|
|
return hlo.AddOp(x, y).results
|
|
register_lowering(ad_util.add_jaxvals_p, add_jaxvals_lowering)
|
|
|
|
register_lowering(ad_util.stop_gradient_p, lambda ctx, x: [x])
|
|
|
|
|
|
def compare_hlo(x, y, direction: str, comparison_type: Optional[str] = None):
|
|
"""Creates CompareOp."""
|
|
if comparison_type is None:
|
|
elem_type = ir.RankedTensorType(x.type).element_type
|
|
if ir.IntegerType.isinstance(elem_type):
|
|
comparison_type = ("UNSIGNED" if ir.IntegerType.is_unsigned(elem_type)
|
|
else "SIGNED")
|
|
else:
|
|
comparison_type = "FLOAT"
|
|
|
|
return hlo.CompareOp(
|
|
x,
|
|
y,
|
|
hlo.ComparisonDirectionAttr.get(direction),
|
|
compare_type=hlo.ComparisonTypeAttr.get(comparison_type))
|
|
|
|
def _minmax_hlo(op, cmp, x, y):
|
|
"""Min/max that compares complex values lexicographically as pairs."""
|
|
tensor_type = ir.RankedTensorType(x.type)
|
|
if ir.ComplexType.isinstance(tensor_type.element_type):
|
|
rx = hlo.RealOp(x).result
|
|
ry = hlo.RealOp(y).result
|
|
real_eq = compare_hlo(rx, ry, "EQ", "FLOAT")
|
|
real_cmp = compare_hlo(rx, ry, cmp, "FLOAT")
|
|
imag_cmp = compare_hlo(
|
|
hlo.ImagOp(x).result,
|
|
hlo.ImagOp(y).result, cmp, "FLOAT")
|
|
which = hlo.SelectOp(real_eq, imag_cmp, real_cmp).result
|
|
return hlo.SelectOp(which, x, y)
|
|
else:
|
|
return op(x, y)
|
|
|
|
min_hlo = partial(_minmax_hlo, hlo.MinOp, "LT")
|
|
max_hlo = partial(_minmax_hlo, hlo.MaxOp, "GT")
|
|
|
|
|
|
def convert_hlo(ctx: LoweringRuleContext, x, aval_in, aval_out):
|
|
"""Variant of convert that has HLO semantics.
|
|
|
|
In particular, treat casts to boolean as x != 0, rather than truncating
|
|
integer values (b/209440332)."""
|
|
if (not dtypes.is_opaque_dtype(aval_out.dtype) and
|
|
aval_out.dtype == np.dtype(np.bool_)):
|
|
if dtypes.issubdtype(aval_in.dtype, np.inexact):
|
|
compare_type = "FLOAT"
|
|
elif dtypes.issubdtype(aval_in.dtype, np.signedinteger):
|
|
compare_type = "SIGNED"
|
|
else:
|
|
compare_type = "UNSIGNED"
|
|
return compare_hlo(x, full_like_aval(ctx, 0, aval_in), "NE",
|
|
compare_type).result
|
|
return hlo.ConvertOp(aval_to_ir_type(aval_out), x).result
|
|
|
|
def _wrap_with_spmd_op(name: str,
|
|
ctx: LoweringRuleContext,
|
|
x: ir.Value,
|
|
aval_out: core.AbstractValue,
|
|
sharding_proto: xc.OpSharding,
|
|
unspecified_dims: Optional[set[int]] = None):
|
|
# unspecified_dims indicate dimensions whose shardings are not specified and
|
|
# XLA sharding propagation can change them.
|
|
if unspecified_dims:
|
|
backend_config = "unspecified_dims=[" + ",".join(
|
|
[str(i) for i in sorted(unspecified_dims)]) + "]"
|
|
else:
|
|
backend_config = ""
|
|
result_type = aval_to_ir_type(aval_out)
|
|
out_shape = core.physical_aval(aval_out).shape # type: ignore
|
|
if core.is_constant_shape(out_shape):
|
|
result_shapes = None
|
|
else:
|
|
result_shapes = [eval_dynamic_shape_as_tensor(ctx, out_shape)]
|
|
|
|
op = custom_call(name, [result_type], [x],
|
|
backend_config=backend_config,
|
|
has_side_effect=False,
|
|
api_version=1,
|
|
result_shapes=result_shapes)
|
|
set_sharding(op, sharding_proto)
|
|
return op.result
|
|
|
|
|
|
wrap_with_sharding_op = partial(_wrap_with_spmd_op, "Sharding")
|
|
wrap_with_full_to_shard_op = partial(_wrap_with_spmd_op, "SPMDFullToShardShape")
|
|
wrap_with_shard_to_full_op = partial(_wrap_with_spmd_op, "SPMDShardToFullShape")
|
|
|
|
def set_sharding(op, sharding_proto: xc.OpSharding):
|
|
op.attributes["mhlo.sharding"] = get_sharding_attr(sharding_proto)
|
|
|
|
|
|
def get_sharding_attr(sharding_proto: xc.OpSharding):
|
|
# If there are very large numbers of devices, use the proto representation.
|
|
# The MHLO to HLO conversion supports both, and the proto representation is
|
|
# more compact.
|
|
if len(sharding_proto.tile_assignment_devices) > 100:
|
|
return ir.StringAttr.get(sharding_proto.SerializeToString())
|
|
else:
|
|
return ir.StringAttr.get(repr(xc.HloSharding.from_proto(sharding_proto)))
|
|
|
|
|
|
# MLIR lowerings for lax primitives
|
|
|
|
def cache_lowering(f):
|
|
"""Decorator that causes the contents of a lowering rule to be reused.
|
|
|
|
The lowering will be emitted out-of-line in a separate function, together with
|
|
a call to that function. If the same primitive is called with the same shapes
|
|
and parameters, a new call to the original function will be added, without
|
|
emitting a new function.
|
|
"""
|
|
@functools.wraps(f)
|
|
def cached_lowering(ctx, *args, **params):
|
|
assert ctx.primitive is not None
|
|
key = (ctx.primitive, tuple(ctx.avals_in), tuple(ctx.avals_out),
|
|
tuple(params.items()))
|
|
try:
|
|
func = ctx.module_context.cached_primitive_lowerings.get(key)
|
|
except TypeError:
|
|
# If the parameters aren't hashable, give up on caching.
|
|
# TODO(phawkins): switch to requiring hashability, when XLA fallback
|
|
# computations have been ported to MLIR.
|
|
return f(ctx, *args, **params)
|
|
if func is None:
|
|
func = _emit_lowering_rule_as_fun(partial(f, **params), ctx)
|
|
ctx.module_context.cached_primitive_lowerings[key] = func
|
|
|
|
output_types = map(aval_to_ir_types, ctx.avals_out)
|
|
args = tuple(ctx.dim_var_values) + args
|
|
flat_output_types = util.flatten(output_types)
|
|
call = func_dialect.CallOp(flat_output_types,
|
|
ir.FlatSymbolRefAttr.get(func.name.value),
|
|
flatten_lowering_ir_args(args))
|
|
return util.unflatten(call.results, map(len, output_types))
|
|
return cached_lowering
|
|
|
|
|
|
def xla_computation_to_mlir_module(xla_computation: xc.XlaComputation
|
|
) -> ir.Module:
|
|
module_str = xc._xla.mlir.xla_computation_to_mlir_module(xla_computation)
|
|
return ir.Module.parse(module_str)
|
|
|
|
def merge_mlir_modules(dst_module: ir.Module,
|
|
sym_name: str,
|
|
src_module: ir.Module) -> str:
|
|
"""
|
|
Args:
|
|
dst_module: the module into which the contents of src_module should be
|
|
moved. Nothing in dst_module will be renamed.
|
|
sym_name: the desired name for the "main" function of src_module after
|
|
merging. This is a hint: the true name may be different because of symbol
|
|
uniquification, and the true name is returned by this function.
|
|
src_module: the module whose contents are to be alpha-renamed, set to
|
|
private visibility, and merged into dst_module. src_module must contain
|
|
exactly one symbol named "main".
|
|
|
|
Functions in src_module will be renamed such that they do not collide with
|
|
functions in dst_module.
|
|
|
|
This function mutates `src_module`. On return, `src_module` is left in an
|
|
undefined state.
|
|
|
|
Returns:
|
|
the name of src_module's main() function, after renaming.
|
|
"""
|
|
assert dst_module.context == src_module.context
|
|
|
|
src_symtab = ir.SymbolTable(src_module.operation)
|
|
dst_symtab = ir.SymbolTable(dst_module.operation)
|
|
used_names = set()
|
|
|
|
# Rename all symbols in src_module that clash with names in dst_module, or
|
|
# are the "main" symbol.
|
|
renamings = {}
|
|
for op in src_module.body.operations:
|
|
name = op.name.value
|
|
should_rename = name in dst_symtab or name == "main"
|
|
if should_rename:
|
|
base_name = sym_name if name == "main" else name
|
|
new_name = base_name
|
|
i = 0
|
|
# Replacements are chosen such that the new names are present in neither
|
|
# src_module, dst_module, or the set of fresh names we've already used.
|
|
# Since we rename names one at a time, if new names were in src_module,
|
|
# they might themselves collide with a later renaming.
|
|
while (new_name in src_symtab or new_name in dst_symtab or
|
|
new_name in used_names):
|
|
new_name = f"{base_name}_{i}"
|
|
i += 1
|
|
renamings[name] = new_name
|
|
used_names.add(new_name)
|
|
|
|
# Apply the symbol renamings to symbol definitions.
|
|
private = ir.StringAttr.get("private")
|
|
for op in src_module.body.operations:
|
|
if op.name.value in renamings:
|
|
src_symtab.set_symbol_name(op, renamings[op.name.value])
|
|
op.attributes["sym_visibility"] = private
|
|
|
|
# Apply the symbol renamings to symbol uses.
|
|
for old_name, new_name in renamings.items():
|
|
for op in src_module.body.operations:
|
|
src_symtab.replace_all_symbol_uses(old_name, new_name, op)
|
|
|
|
for op in src_module.body.operations:
|
|
dst_module.body.append(op)
|
|
|
|
return renamings["main"]
|
|
|
|
|
|
def xla_fallback_lowering(prim: core.Primitive):
|
|
@cache_lowering
|
|
def fallback(ctx: LoweringRuleContext, *args, **params):
|
|
module_ctx = ctx.module_context
|
|
axis_ctx = module_ctx.axis_context
|
|
if isinstance(axis_ctx, sharding_impls.SPMDAxisContext):
|
|
axis_env = axis_ctx.unsafe_axis_env
|
|
else:
|
|
axis_env = module_ctx.axis_env
|
|
|
|
if any(hasattr(a, "shape") and
|
|
not core.is_constant_shape(a.shape) for a in (ctx.avals_in + ctx.avals_out)):
|
|
raise NotImplementedError(
|
|
f"Shape polymorphism for xla_fallback_lowering is not implemented ({ctx.primitive}); b/261682623")
|
|
|
|
xla_computation = xla.primitive_subcomputation(
|
|
module_ctx.platform, axis_env, prim, ctx.avals_in,
|
|
ctx.avals_out, **params)
|
|
xla_module = xla_computation_to_mlir_module(xla_computation)
|
|
callee_name = merge_mlir_modules(
|
|
module_ctx.module, f"xla_fallback_{prim.name}", xla_module)
|
|
output_types = map(aval_to_ir_types, ctx.avals_out)
|
|
flat_output_types = util.flatten(output_types)
|
|
output_type = (ir.TupleType.get_tuple(flat_output_types)
|
|
if prim.multiple_results else flat_output_types[0])
|
|
|
|
call = func_dialect.CallOp([output_type],
|
|
ir.FlatSymbolRefAttr.get(callee_name),
|
|
flatten_lowering_ir_args(args)).result
|
|
if not prim.multiple_results:
|
|
return [call]
|
|
flat_results = [hlo.GetTupleElementOp(call, i32_attr(i)).result
|
|
for i in range(len(flat_output_types))]
|
|
|
|
return util.unflatten(flat_results, map(len, output_types))
|
|
return fallback
|
|
|
|
|
|
DEVICE_TO_DEVICE_TYPE = 1
|
|
SEND_TO_HOST_TYPE = 2
|
|
RECV_FROM_HOST_TYPE = 3
|
|
|
|
_dtype_to_xla_type_string_map = {
|
|
np.dtype("bool"): "pred",
|
|
np.dtype("float16"): "f16",
|
|
np.dtype("float32"): "f32",
|
|
np.dtype("float64"): "f64",
|
|
np.dtype("int8"): "s8",
|
|
np.dtype("uint8"): "u8",
|
|
np.dtype("int16"): "s16",
|
|
np.dtype("uint16"): "u16",
|
|
np.dtype("int32"): "s32",
|
|
np.dtype("uint32"): "u32",
|
|
np.dtype("int64"): "s64",
|
|
np.dtype("uint64"): "u64",
|
|
dtypes._bfloat16_dtype: "bf16",
|
|
np.dtype("complex64"): "c64",
|
|
np.dtype("complex128"): "c128",
|
|
}
|
|
|
|
def _dtype_to_xla_type_string(dtype: np.dtype) -> str:
|
|
if dtype not in _dtype_to_xla_type_string_map:
|
|
raise NotImplementedError(dtype)
|
|
return _dtype_to_xla_type_string_map[dtype]
|
|
|
|
def send_to_host(channel: int, token: hlo.TokenType, operand: Any,
|
|
aval: core.ShapedArray, name: str, *,
|
|
sharding: Optional[xc.OpSharding] = None) -> ir.Value:
|
|
channel_handle = hlo.ChannelHandle.get(channel, SEND_TO_HOST_TYPE)
|
|
send_op = hlo.SendOp([operand], token, channel_handle,
|
|
is_host_transfer=ir.BoolAttr.get(True))
|
|
dtype_str = _dtype_to_xla_type_string(aval.dtype)
|
|
if dtype_str in {"f64", "s64", "u64", "c64", "c128"}:
|
|
raise NotImplementedError("64-bit types not supported.")
|
|
send_op.attributes["mhlo.frontend_attributes"] = ir.DictAttr.get(
|
|
dict(
|
|
_xla_host_transfer_handler_name=ir.StringAttr.get(str(name)),
|
|
_xla_host_transfer_original_type=ir.StringAttr.get(dtype_str),
|
|
_xla_host_transfer_rendezvous=ir.StringAttr.get(str(name))))
|
|
if sharding is not None:
|
|
set_sharding(send_op, sharding)
|
|
return send_op.result
|
|
|
|
|
|
def receive_from_host(channel: int, token: hlo.TokenType,
|
|
out_aval: core.ShapedArray, name: str, *,
|
|
sharding: Optional[xc.OpSharding] = None) -> ir.Value:
|
|
channel_handle = hlo.ChannelHandle.get(channel, RECV_FROM_HOST_TYPE)
|
|
recv_op = hlo.RecvOp([aval_to_ir_type(out_aval),
|
|
hlo.TokenType.get()], token, channel_handle,
|
|
is_host_transfer=ir.BoolAttr.get(True))
|
|
dtype_str = _dtype_to_xla_type_string(out_aval.dtype)
|
|
if dtype_str in {"f64", "s64", "u64", "c64", "c128"}:
|
|
raise NotImplementedError("64-bit types not supported.")
|
|
recv_op.attributes["mhlo.frontend_attributes"] = ir.DictAttr.get(
|
|
dict(
|
|
_xla_host_transfer_handler_name=ir.StringAttr.get(str(name)),
|
|
_xla_host_transfer_original_type=ir.StringAttr.get(dtype_str),
|
|
_xla_host_transfer_rendezvous=ir.StringAttr.get(str(name))))
|
|
if sharding is not None:
|
|
set_sharding(recv_op, sharding)
|
|
# Token should be at the end of the results
|
|
result, token = recv_op.results
|
|
return token, result
|
|
|
|
|
|
def _emit_tpu_python_callback(
|
|
backend: xb.XlaBackend,
|
|
ctx: LoweringRuleContext,
|
|
callback,
|
|
token: Optional[Any],
|
|
operands: Sequence[ir.Value],
|
|
operand_avals: list[core.ShapedArray],
|
|
operand_shapes: list[xc.Shape],
|
|
result_avals: list[core.ShapedArray],
|
|
result_shapes: list[xc.Shape],
|
|
*,
|
|
sharding: Optional[xc.OpSharding] = None
|
|
) -> tuple[list[ir.Value], Any, Any]:
|
|
token = token or hlo.CreateTokenOp().result
|
|
_wrapped_callback = callback
|
|
|
|
send_channels = []
|
|
if not operand_avals:
|
|
# If there are no operands to the callback, we need to insert a dummy send
|
|
# op or the callback will never be triggered!
|
|
# TODO(sharadmv,chky): Enable this fix in the runtime as opposed to in
|
|
# MLIR builder.
|
|
callback_without_args = _wrapped_callback
|
|
def _wrapped_callback(*args): # pylint: disable=function-redefined
|
|
del args
|
|
return callback_without_args()
|
|
send_channel = ctx.module_context.new_channel()
|
|
dummy_send_aval = core.ShapedArray((1,), np.float32)
|
|
dummy_send_val = ir_constant(np.zeros(1, np.float32))
|
|
operand_shapes = [*operand_shapes,
|
|
xla.aval_to_xla_shapes(dummy_send_aval)[0]]
|
|
token = send_to_host(send_channel, token, dummy_send_val, dummy_send_aval,
|
|
callback.__name__, sharding=sharding)
|
|
send_channels.append(send_channel)
|
|
else:
|
|
for operand, operand_aval in zip(operands, operand_avals):
|
|
if any(s == 0 for s in operand_aval.shape):
|
|
raise NotImplementedError(
|
|
"Callbacks with zero-dimensional values not supported on TPU.")
|
|
channel = ctx.module_context.new_channel()
|
|
token = send_to_host(channel, token, operand, operand_aval,
|
|
callback.__name__, sharding=sharding)
|
|
send_channels.append(channel)
|
|
|
|
recv_channels = []
|
|
outputs = []
|
|
for result_aval in result_avals:
|
|
if any(s == 0 for s in result_aval.shape):
|
|
raise NotImplementedError(
|
|
"Callbacks with zero-dimensional values not supported on TPU.")
|
|
channel = ctx.module_context.new_channel()
|
|
assert isinstance(result_aval, core.ShapedArray)
|
|
token, out = receive_from_host(channel, token, result_aval,
|
|
callback.__name__, sharding=sharding)
|
|
outputs.append(out)
|
|
recv_channels.append(channel)
|
|
if xla_extension_version < 161:
|
|
opaque = backend.make_python_callback_from_host_send_and_recv(
|
|
_wrapped_callback, operand_shapes, result_shapes, send_channels,
|
|
recv_channels) # type: ignore # pylint: disable=missing-parameter
|
|
else:
|
|
opaque = backend.make_python_callback_from_host_send_and_recv(
|
|
_wrapped_callback, operand_shapes, result_shapes, send_channels,
|
|
recv_channels, pickle_util.dumps) # type: ignore # pylint: disable=missing-parameter
|
|
ctx.module_context.add_host_callback(opaque)
|
|
return outputs, token, opaque
|
|
|
|
def _layout_to_mlir_layout(minor_to_major: Optional[Sequence[int]]):
|
|
if minor_to_major is None:
|
|
# Needed for token layouts
|
|
layout = np.zeros((0,), dtype="int64")
|
|
else:
|
|
layout = np.array(minor_to_major, dtype="int64")
|
|
return ir.DenseIntElementsAttr.get(layout, type=ir.IndexType.get())
|
|
|
|
def _aval_to_default_layouts(aval):
|
|
avals = [core.physical_aval(aval)]
|
|
# Row major order is default for `NumPy`.
|
|
return [list(range(aval.ndim - 1, -1, -1)) for aval in avals]
|
|
|
|
def emit_python_callback(
|
|
ctx: LoweringRuleContext, callback, token: Optional[Any],
|
|
operands: Sequence[ir.Value], operand_avals: list[core.ShapedArray],
|
|
result_avals: list[core.ShapedArray],
|
|
has_side_effect: bool, *, sharding: Optional[xc.OpSharding] = None,
|
|
operand_layouts: Optional[Sequence[Optional[Sequence[int]]]] = None,
|
|
result_layouts: Optional[Sequence[Optional[Sequence[int]]]] = None,
|
|
) -> tuple[list[ir.Value], Any, Any]:
|
|
"""Emits MLIR that calls back to a provided Python function."""
|
|
platform = ctx.module_context.platform
|
|
if platform not in {"cpu", "cuda", "rocm", "tpu"}:
|
|
raise ValueError(
|
|
f"`EmitPythonCallback` not supported on {platform} backend.")
|
|
backend = ctx.module_context.backend
|
|
result_shapes = util.flatten(
|
|
[xla.aval_to_xla_shapes(result_aval) for result_aval in result_avals])
|
|
operand_shapes = util.flatten(
|
|
[xla.aval_to_xla_shapes(op_aval) for op_aval in operand_avals])
|
|
# Handling layouts
|
|
if operand_layouts is None:
|
|
operand_layouts = util.concatenate(
|
|
map(_aval_to_default_layouts, operand_avals))
|
|
operand_mlir_layouts = map(_layout_to_mlir_layout, operand_layouts)
|
|
if result_layouts is None:
|
|
result_layouts = util.concatenate(map(_aval_to_default_layouts, result_avals))
|
|
result_mlir_layouts = map(_layout_to_mlir_layout, result_layouts)
|
|
|
|
# First we apply checks to ensure output shapes and dtypes match the expected
|
|
# ones.
|
|
def _wrapped_callback(*args):
|
|
out_vals = callback(*args)
|
|
if len(out_vals) != len(result_avals):
|
|
raise RuntimeError(
|
|
"Mismatched number of outputs from callback. "
|
|
"Expected: {}, Actual: {}".format(len(result_avals), len(out_vals)))
|
|
for i, (out_val, out_aval) in enumerate(zip(out_vals, result_avals)):
|
|
if out_val.shape != out_aval.shape:
|
|
raise RuntimeError(
|
|
f"Incorrect output shape for return value {i}: "
|
|
"Expected: {}, Actual: {}".format(out_aval.shape, out_val.shape))
|
|
if out_val.dtype != out_aval.dtype:
|
|
raise RuntimeError(
|
|
f"Incorrect output dtype for return value {i}: "
|
|
"Expected: {}, Actual: {}".format(out_aval.dtype, out_val.dtype))
|
|
return out_vals
|
|
|
|
if platform == "tpu":
|
|
return _emit_tpu_python_callback(backend, ctx, _wrapped_callback, token,
|
|
operands, operand_avals, operand_shapes, result_avals, result_shapes,
|
|
sharding=sharding)
|
|
result_types = util.flatten([aval_to_ir_types(aval) for aval in result_avals])
|
|
if token:
|
|
|
|
callback_without_token = _wrapped_callback
|
|
def _wrapped_callback(token, *args): # type: ignore # pylint: disable=function-redefined
|
|
return (token, *callback_without_token(*args))
|
|
|
|
operand_shapes = [
|
|
xla.aval_to_xla_shapes(core.abstract_token)[0], *operand_shapes
|
|
]
|
|
result_shapes = [
|
|
xla.aval_to_xla_shapes(core.abstract_token)[0], *result_shapes
|
|
]
|
|
operands = [token, *operands]
|
|
result_types = [token_type()[0], *result_types]
|
|
operand_mlir_layouts = [_layout_to_mlir_layout(None), *operand_mlir_layouts]
|
|
result_mlir_layouts = [_layout_to_mlir_layout(None), *result_mlir_layouts]
|
|
callback_descriptor, keepalive = (
|
|
backend.get_emit_python_callback_descriptor(_wrapped_callback,
|
|
operand_shapes,
|
|
result_shapes))
|
|
descriptor_operand = ir_constant(
|
|
callback_descriptor, canonicalize_types=False)
|
|
callback_operands = [descriptor_operand, *operands]
|
|
if operand_mlir_layouts is not None:
|
|
operand_mlir_layouts = [_layout_to_mlir_layout([]), *operand_mlir_layouts]
|
|
result_type = ir.TupleType.get_tuple(result_types)
|
|
call_target_name = ("xla_python_gpu_callback"
|
|
if platform in {"cuda", "rocm"} else "xla_python_cpu_callback")
|
|
result = hlo.CustomCallOp(
|
|
[result_type],
|
|
callback_operands,
|
|
call_target_name=ir.StringAttr.get(call_target_name),
|
|
has_side_effect=ir.BoolAttr.get(has_side_effect),
|
|
api_version=i32_attr(2),
|
|
called_computations=ir.ArrayAttr.get([]),
|
|
backend_config=ir.StringAttr.get(str(callback_descriptor)),
|
|
operand_layouts=(
|
|
None if operand_mlir_layouts is None
|
|
else ir.ArrayAttr.get(operand_mlir_layouts)),
|
|
result_layouts=(
|
|
None if result_mlir_layouts is None
|
|
else ir.ArrayAttr.get(result_mlir_layouts)))
|
|
if sharding is not None:
|
|
set_sharding(result, sharding)
|
|
results = [
|
|
hlo.GetTupleElementOp(result, i32_attr(i)).result
|
|
for i in range(len(result_types))
|
|
]
|
|
if token:
|
|
token, *results = results
|
|
return results, token, keepalive
|
|
|
|
def build_xla_computation_helper(
|
|
closed_jaxpr: core.ClosedJaxpr, *, name: str, platform: str,
|
|
backend_or_name: str, axis_context: AxisContext) -> xc.XlaComputation:
|
|
"""Helper to generate pmap-style XLA computations for custom partitioners."""
|
|
if closed_jaxpr.effects:
|
|
raise NotImplementedError
|
|
lowering_result = lower_jaxpr_to_module(name, closed_jaxpr,
|
|
backend_or_name=backend_or_name, ordered_effects=[],
|
|
name_stack=source_info_util.NameStack(),
|
|
donated_args=[False] * len(closed_jaxpr.jaxpr.invars),
|
|
axis_context=axis_context, platform=platform)
|
|
return xc._xla.mlir.mlir_module_to_xla_computation(
|
|
module_to_string(lowering_result.module), use_tuple_args=False,
|
|
return_tuple=False)
|
|
|
|
def custom_call(
|
|
call_target_name: str,
|
|
out_types: Sequence[ir.Type],
|
|
operands: Sequence[ir.Value],
|
|
*,
|
|
backend_config: Union[str, dict[str, ir.Attribute]] = "",
|
|
has_side_effect: bool = False,
|
|
result_shapes: Optional[Sequence[ir.Value]] = None,
|
|
called_computations: Sequence[str] = (),
|
|
api_version: int = 2,
|
|
extra_attributes: dict[str, ir.Attribute] = {},
|
|
) -> ir.Operation:
|
|
"""Wraps a hlo.CustomCall.
|
|
|
|
Args:
|
|
result_shapes: tensors that represent the result shapes, to be used when
|
|
the results have dynamic shapes. If not-None, its length must match the
|
|
number of the results.
|
|
called_computations: the list of function names called by the custom call.
|
|
"""
|
|
if backend_config is None:
|
|
backend_config_attr = ir.StringAttr.get("")
|
|
elif isinstance(backend_config, str):
|
|
backend_config_attr = ir.StringAttr.get(backend_config)
|
|
elif isinstance(backend_config, dict):
|
|
# TODO(necula): it seems that the CustomCallOp constructor requires that
|
|
# backend_config_attr be a string attribute, even though in some cases we
|
|
# need it to be a DictAttr, e.g., for ApproxTopK on TPU.
|
|
# "Verification failed: 'stablehlo.custom_call' op attribute 'backend_config' failed to satisfy constraint: string attribute"
|
|
# To workaround this limitation we first set it to the empty string and we
|
|
# use an unregistered attribute mhlo.backend_config to hold the DictAttr.
|
|
# We must also use api_version=1 to ensure that mhlo.backend_config is
|
|
# handled properly.
|
|
backend_config_attr = ir.StringAttr.get("")
|
|
api_version = 1
|
|
else:
|
|
raise ValueError("custom_call backend_config unexpected type: " + str(backend_config))
|
|
attributes = dict(
|
|
call_target_name=ir.StringAttr.get(call_target_name),
|
|
has_side_effect=ir.BoolAttr.get(has_side_effect),
|
|
backend_config=backend_config_attr,
|
|
api_version=i32_attr(api_version),
|
|
called_computations=ir.ArrayAttr.get([
|
|
ir.FlatSymbolRefAttr.get(name) for name in called_computations]),
|
|
)
|
|
attributes.update(extra_attributes)
|
|
|
|
if result_shapes is not None:
|
|
# We add the result_shapes at the end of the operands, and must pass
|
|
# the indices_of_output_operands attribute. This attribute is not yet
|
|
# accepted by the CustomCall constructor, so we use build_generic
|
|
attributes["indices_of_shape_operands"] = ir.DenseIntElementsAttr.get(
|
|
np.asarray(list(range(len(operands), len(operands) + len(result_shapes))),
|
|
dtype=np.int64))
|
|
operands = list(operands) + list(result_shapes)
|
|
|
|
op = hlo.CustomCallOp.build_generic(results=out_types, operands=operands, attributes=attributes)
|
|
if isinstance(backend_config, dict):
|
|
backend_config_attr = ir.DictAttr.get(backend_config)
|
|
op.operation.attributes["mhlo.backend_config"] = backend_config_attr
|
|
return op
|
|
|
|
|
|
def reduce_window(
|
|
ctx: LoweringRuleContext, *,
|
|
# Base name to be used for the reducer function
|
|
reducer_name: str,
|
|
# Compute the reducer body given the reducer.
|
|
reducer_body: Callable[[ir.Block], Sequence[ir.Value]],
|
|
operands: Sequence[ir.Value],
|
|
init_values: Sequence[ir.Value],
|
|
init_values_avals: Sequence[core.AbstractValue],
|
|
out_avals: Sequence[core.AbstractValue],
|
|
window_dimensions, window_strides, padding, base_dilation, window_dilation):
|
|
"""Builds a ReduceWindowOp, with support for dynamic shapes."""
|
|
|
|
scalar_types = [aval_to_ir_type(aval) for aval in init_values_avals]
|
|
if any(not core.is_constant_shape(s)
|
|
for s in [window_dimensions, window_dilation, window_strides, base_dilation, *padding]):
|
|
# d_padding will be an array i32[N, 2] with pad_lo and pad_hi for each
|
|
# spatial dimension.
|
|
int2d = aval_to_ir_type(core.ShapedArray((1, 2), np.int32))
|
|
def prep_one_pad(pad_lo_hi: tuple[core.DimSize, core.DimSize]):
|
|
pads = eval_dynamic_shape_as_tensor(ctx, pad_lo_hi) # i32[2]
|
|
return hlo.ReshapeOp(int2d, pads)
|
|
d_padding = hlo.ConcatenateOp(list(map(prep_one_pad, padding)),
|
|
i64_attr(0)).result
|
|
# Build the reducer
|
|
reducer_type = ir.FunctionType.get(scalar_types + scalar_types,
|
|
scalar_types)
|
|
with ir.InsertionPoint.at_block_begin(ctx.module_context.module.body):
|
|
reducer = func_dialect.FuncOp(reducer_name, reducer_type)
|
|
ctx.module_context.symbol_table.insert(reducer)
|
|
entry_block = reducer.add_entry_block()
|
|
with ir.InsertionPoint(entry_block):
|
|
res = reducer_body(entry_block)
|
|
hlo.ReturnOp(res)
|
|
|
|
rw = custom_call(
|
|
"stablehlo.dynamic_reduce_window",
|
|
list(map(aval_to_ir_type, out_avals)),
|
|
[
|
|
*operands, *init_values,
|
|
eval_dynamic_shape_as_tensor(ctx, window_dimensions),
|
|
eval_dynamic_shape_as_tensor(ctx, window_strides),
|
|
eval_dynamic_shape_as_tensor(ctx, base_dilation),
|
|
eval_dynamic_shape_as_tensor(ctx, window_dilation),
|
|
d_padding],
|
|
called_computations=[reducer.name.value],
|
|
)
|
|
else: # Static shapes
|
|
rw = hlo.ReduceWindowOp(
|
|
list(map(aval_to_ir_type, out_avals)),
|
|
operands, init_values,
|
|
dense_int_elements(window_dimensions),
|
|
window_strides=dense_int_elements(window_strides),
|
|
base_dilations=dense_int_elements(base_dilation),
|
|
window_dilations=dense_int_elements(window_dilation),
|
|
padding=ir.DenseIntElementsAttr.get(np.asarray(padding, np.int64),
|
|
shape=(len(padding), 2)))
|
|
reducer = rw.regions[0].blocks.append(*(scalar_types + scalar_types))
|
|
with ir.InsertionPoint(reducer):
|
|
res = reducer_body(reducer)
|
|
hlo.ReturnOp(res)
|
|
return rw.results
|
|
|
|
|
|
def refine_polymorphic_shapes(module: ir.Module) -> ir.Module:
|
|
"""Refines the polymorphic shapes inside a module.
|
|
|
|
Given a module with static input shapes, but using dynamic shapes due to
|
|
shape polymorphism, runs shape refinement to resolve all the dynamic shapes.
|
|
Then verifies that there are no more dynamic shapes in the module.
|
|
"""
|
|
if xc.mlir_api_version >= 53:
|
|
refined_module_str = xla_extension.mlir.refine_polymorphic_shapes(
|
|
module_to_bytecode(module), enable_shape_assertions=True,
|
|
validate_static_shapes=True)
|
|
elif xc.mlir_api_version == 52:
|
|
refined_module_str = xla_extension.mlir.refine_polymorphic_shapes(
|
|
module_to_bytecode(module), enable_shape_assertions=True)
|
|
elif xc.mlir_api_version >= 50:
|
|
refined_module_str = xla_extension.mlir.refine_polymorphic_shapes(
|
|
module_to_bytecode(module))
|
|
else:
|
|
raise NotImplementedError("refine_polymorphic_shapes needs jaxlib 0.4.12")
|
|
|
|
context = make_ir_context()
|
|
with context:
|
|
return ir.Module.parse(refined_module_str)
|