mirror of
https://github.com/ROCm/jax.git
synced 2025-04-17 12:26:07 +00:00
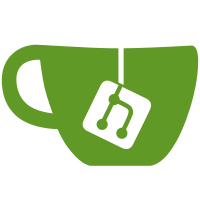
Add print First version with custom_partitioning. The communication during the gradient aren't optimal. Fix the gradient sharding small update Fix the strange replicated computation. Make it work with the new JAX version. Add the structure for custom_p domentation. Small clean up First version of the doc Add comment and typing annotation tab->space Simplify code and add docstring Use the simpler JAX API since 0.4.16 (August 2023). Custom partitioning using custom_partitioning updated docs; dump custom_partitioning HLO doc update more documentation updates; include links to code instead of inlined code fix typos fix more typos fix type annotations in source and update docs minor fixes import fix lint fix added apache license header
529 lines
19 KiB
Python
529 lines
19 KiB
Python
# Copyright 2024 The JAX Authors.
|
|
#
|
|
# Licensed under the Apache License, Version 2.0 (the "License");
|
|
# you may not use this file except in compliance with the License.
|
|
# You may obtain a copy of the License at
|
|
#
|
|
# https://www.apache.org/licenses/LICENSE-2.0
|
|
#
|
|
# Unless required by applicable law or agreed to in writing, software
|
|
# distributed under the License is distributed on an "AS IS" BASIS,
|
|
# WITHOUT WARRANTIES OR CONDITIONS OF ANY KIND, either express or implied.
|
|
# See the License for the specific language governing permissions and
|
|
# limitations under the License.
|
|
|
|
from functools import partial, reduce
|
|
import math
|
|
from typing import Tuple
|
|
|
|
import jax
|
|
import jax.numpy as jnp
|
|
from build import gpu_ops
|
|
from jax import core, dtypes
|
|
from jax.core import ShapedArray
|
|
from jax.experimental.custom_partitioning import custom_partitioning
|
|
from jax.experimental.pjit import pjit
|
|
from jax.interpreters import batching, mlir, xla
|
|
from jax.interpreters.mlir import ir
|
|
from jax.lib import xla_client
|
|
from jax.sharding import Mesh, NamedSharding, PartitionSpec
|
|
from jaxlib.hlo_helpers import custom_call
|
|
from jax._src import dispatch
|
|
|
|
|
|
######################################################################
|
|
# Created Primitives for unsharded RMS norm reference implementation #
|
|
######################################################################
|
|
|
|
# Create _rms_norm_fwd_p for forward operation.
|
|
_rms_norm_fwd_p = core.Primitive("rms_norm_fwd")
|
|
_rms_norm_fwd_p.multiple_results = True
|
|
_rms_norm_fwd_p.def_impl(partial(xla.apply_primitive, _rms_norm_fwd_p))
|
|
|
|
|
|
def rms_norm_fwd(x, weight, eps=1e-05):
|
|
output, invvar = _rms_norm_fwd_p.bind(x, weight, eps=eps)
|
|
return output, (invvar, x, weight)
|
|
|
|
|
|
# Create _rms_norm_bwd_p for backward operation.
|
|
_rms_norm_bwd_p = core.Primitive("rms_norm_bwd")
|
|
_rms_norm_bwd_p.multiple_results = True
|
|
_rms_norm_bwd_p.def_impl(partial(xla.apply_primitive, _rms_norm_bwd_p))
|
|
|
|
|
|
def rms_norm_bwd(eps, res, g):
|
|
invvar, x, weight = res
|
|
grad_input, grad_weight, part_grad = _rms_norm_bwd_p.bind(
|
|
g, invvar, x, weight, eps=eps
|
|
)
|
|
return grad_input, grad_weight
|
|
|
|
|
|
####################
|
|
# Lowering to MLIR #
|
|
####################
|
|
|
|
|
|
# Register functions defined in gpu_ops as custom call target for GPUs
|
|
for _name, _value in gpu_ops.get_rms_norm_registrations().items():
|
|
xla_client.register_custom_call_target(_name, _value, platform="gpu")
|
|
|
|
|
|
def element_type_to_descriptor_type_mapping(element_type):
|
|
_element_type_to_descriptor_type_mapping = {
|
|
ir.BF16Type.get(): gpu_ops.ElementType.BF16,
|
|
ir.F16Type.get(): gpu_ops.ElementType.F16,
|
|
ir.F32Type.get(): gpu_ops.ElementType.F32,
|
|
ir.F64Type.get(): gpu_ops.ElementType.F64,
|
|
}
|
|
return _element_type_to_descriptor_type_mapping.get(element_type)
|
|
|
|
|
|
def default_layouts(*shapes):
|
|
return [range(len(shape) - 1, -1, -1) for shape in shapes]
|
|
|
|
|
|
def _rms_norm_fwd_cuda_lowering(ctx, x, weight, eps):
|
|
x_type = ir.RankedTensorType(x.type)
|
|
x_shape = x_type.shape
|
|
w_type = ir.RankedTensorType(weight.type)
|
|
w_shape = w_type.shape
|
|
iv_element_type = (
|
|
ir.F32Type.get()
|
|
if x_type.element_type in [ir.F16Type.get(), ir.BF16Type.get()]
|
|
else x_type.element_type
|
|
)
|
|
|
|
n2 = math.prod(w_shape)
|
|
n1 = math.prod(x_shape) // n2
|
|
|
|
opaque = gpu_ops.create_rms_norm_descriptor(
|
|
n1,
|
|
n2,
|
|
eps,
|
|
element_type_to_descriptor_type_mapping(x_type.element_type),
|
|
element_type_to_descriptor_type_mapping(w_type.element_type),
|
|
0, # unused
|
|
)
|
|
out = custom_call(
|
|
b"rms_forward_affine_mixed_dtype",
|
|
result_types=[
|
|
ir.RankedTensorType.get(x_shape, w_type.element_type),
|
|
ir.RankedTensorType.get((n1,), iv_element_type),
|
|
],
|
|
operands=[x, weight],
|
|
backend_config=opaque,
|
|
operand_layouts=default_layouts(x_shape, w_shape),
|
|
result_layouts=default_layouts(x_shape, (n1,)),
|
|
).results
|
|
return out
|
|
|
|
|
|
mlir.register_lowering(
|
|
_rms_norm_fwd_p,
|
|
_rms_norm_fwd_cuda_lowering,
|
|
platform="gpu",
|
|
)
|
|
|
|
|
|
def _rms_norm_bwd_cuda_lowering(ctx, grad_output, invvar, x, weight, eps):
|
|
x_type = ir.RankedTensorType(x.type)
|
|
x_shape = x_type.shape
|
|
w_type = ir.RankedTensorType(weight.type)
|
|
w_shape = w_type.shape
|
|
iv_type = ir.RankedTensorType(invvar.type)
|
|
|
|
n2 = reduce(lambda x, y: x * y, w_shape)
|
|
n1 = reduce(lambda x, y: x * y, x_shape) // n2
|
|
|
|
part_grad_shape = ctx.avals_out[-1].shape
|
|
|
|
opaque = gpu_ops.create_rms_norm_descriptor(
|
|
n1,
|
|
n2,
|
|
eps,
|
|
element_type_to_descriptor_type_mapping(x_type.element_type),
|
|
element_type_to_descriptor_type_mapping(w_type.element_type),
|
|
part_grad_shape[0],
|
|
)
|
|
out = custom_call(
|
|
b"rms_backward_affine",
|
|
result_types=[
|
|
ir.RankedTensorType.get(x_shape, x_type.element_type),
|
|
ir.RankedTensorType.get(w_shape, w_type.element_type),
|
|
ir.RankedTensorType.get(part_grad_shape, iv_type.element_type),
|
|
],
|
|
operands=[grad_output, invvar, x, weight],
|
|
backend_config=opaque,
|
|
operand_layouts=default_layouts(x_shape, (n1,), x_shape, w_shape),
|
|
result_layouts=default_layouts(x_shape, w_shape, part_grad_shape),
|
|
).results
|
|
return out
|
|
|
|
|
|
mlir.register_lowering(
|
|
_rms_norm_bwd_p,
|
|
_rms_norm_bwd_cuda_lowering,
|
|
platform="gpu",
|
|
)
|
|
|
|
|
|
#######################
|
|
# Abstract evaluation #
|
|
#######################
|
|
|
|
|
|
def _rms_norm_fwd_abstract(x, weight, eps):
|
|
w_dtype = dtypes.canonicalize_dtype(weight.dtype)
|
|
iv_dtype = dtypes.canonicalize_dtype(x.dtype)
|
|
if iv_dtype in [jnp.float16, jnp.bfloat16]:
|
|
iv_dtype = jnp.float32
|
|
n2 = math.prod(weight.shape)
|
|
n1 = math.prod(x.shape) // n2
|
|
return (
|
|
ShapedArray(x.shape, w_dtype, named_shape=x.named_shape), # output
|
|
ShapedArray((n1,), iv_dtype, named_shape=x.named_shape), # invvar
|
|
)
|
|
|
|
|
|
_rms_norm_fwd_p.def_abstract_eval(_rms_norm_fwd_abstract)
|
|
|
|
|
|
def _rms_norm_bwd_abstract(grad_output, invvar, x, weight, eps):
|
|
iv_dtype = dtypes.canonicalize_dtype(invvar.dtype)
|
|
w_dtype = dtypes.canonicalize_dtype(weight.dtype)
|
|
x_dtype = dtypes.canonicalize_dtype(x.dtype)
|
|
n2 = reduce(lambda x, y: x * y, weight.shape)
|
|
n1 = reduce(lambda x, y: x * y, x.shape) // n2
|
|
part_grad_shape = (16, n2)
|
|
assert dtypes.canonicalize_dtype(grad_output.dtype) == w_dtype
|
|
assert grad_output.shape == x.shape
|
|
assert invvar.shape == (n1,)
|
|
assert (
|
|
iv_dtype == jnp.float32 if x_dtype in [jnp.float16, jnp.bfloat16] else x_dtype
|
|
)
|
|
assert grad_output.named_shape == x.named_shape
|
|
weight_named_shape = (
|
|
weight.named_shape if weight.named_shape else grad_output.named_shape
|
|
)
|
|
return (
|
|
ShapedArray(
|
|
x.shape, x_dtype, named_shape=x.named_shape
|
|
), # grad input
|
|
ShapedArray(
|
|
weight.shape, w_dtype, named_shape=weight_named_shape
|
|
), # grad weight
|
|
ShapedArray(
|
|
part_grad_shape, iv_dtype, named_shape=weight_named_shape
|
|
), # part grad
|
|
)
|
|
|
|
|
|
_rms_norm_bwd_p.def_abstract_eval(_rms_norm_bwd_abstract)
|
|
|
|
|
|
#######################################
|
|
# Top-level interface with custom vjp #
|
|
#######################################
|
|
|
|
|
|
@partial(jax.custom_vjp, nondiff_argnums=(2,))
|
|
def rms_norm(x, weight, eps=1e-05):
|
|
output, _ = rms_norm_fwd(x, weight, eps=eps)
|
|
return output
|
|
|
|
|
|
rms_norm.defvjp(rms_norm_fwd, rms_norm_bwd)
|
|
|
|
###########################################################
|
|
# Create primitives for RMS norm with custom_partitioning #
|
|
###########################################################
|
|
|
|
def _check_valid_batch_dims(bdims):
|
|
"""
|
|
Assert out non-supported bath dims
|
|
"""
|
|
for dim in bdims:
|
|
assert dim in [0, None], \
|
|
"Currently only support batch_dim in [0, None], " \
|
|
f"but got {dim=}"
|
|
|
|
def register_primitive(cls):
|
|
"""
|
|
register jax primitive
|
|
|
|
The order of calls. Each operation is composed of two primitives: Inner and Outer.
|
|
|
|
Inner, only the basic to wrap the custom_call itself.
|
|
- impl to XLA custom_call in C.
|
|
- abstract to know the static shapes
|
|
- lower to StableHLO XLA custom_call.
|
|
Outer, mostly all the rest:
|
|
- impl: Bind to the inner primitive. Not used for real computation, but only for tracing. So we only need to bind.
|
|
- abstract: same
|
|
- lower to StableHLO custom_p. (XLA will call the python callback from it)
|
|
- custom_p
|
|
- vmap: could be added here.
|
|
VJP is based on Outer, but not handled in this function.
|
|
"""
|
|
|
|
def name_of_wrapper_p():
|
|
return cls.name + "_wrapper"
|
|
|
|
inner_p = core.Primitive(cls.name)
|
|
dispatch.prim_requires_devices_during_lowering.add(inner_p)
|
|
inner_p.multiple_results = cls.multiple_results
|
|
inner_p.def_impl(partial(xla.apply_primitive, inner_p))
|
|
inner_p.def_abstract_eval(cls.abstract)
|
|
mlir.register_lowering(inner_p, cls.lowering, platform='cuda')
|
|
cls.inner_primitive = inner_p
|
|
|
|
outer_p = core.Primitive(name_of_wrapper_p())
|
|
dispatch.prim_requires_devices_during_lowering.add(outer_p)
|
|
outer_p.multiple_results = cls.multiple_results
|
|
outer_p.def_impl(cls.impl)
|
|
outer_p.def_abstract_eval(cls.abstract)
|
|
batching.primitive_batchers[outer_p] = cls.batcher
|
|
outer_p_lower = custom_partitioning(cls.impl, static_argnums=cls.impl_static_args)
|
|
outer_p_lower.def_partition(infer_sharding_from_operands=cls.infer_sharding_from_operands,
|
|
partition=cls.partition)
|
|
mlir.register_lowering(outer_p,
|
|
mlir.lower_fun(outer_p_lower, multiple_results=cls.multiple_results))
|
|
cls.outer_primitive = outer_p
|
|
|
|
|
|
class RmsNormFwdClass:
|
|
name = "rms_forward_affine_mixed_dtype"
|
|
multiple_results = True
|
|
impl_static_args = (2,) # eps
|
|
inner_primitive = None
|
|
outer_primitive = None
|
|
|
|
@staticmethod
|
|
def abstract(x_aval, gamma_aval, **kwargs): # pylint: disable=unused-argument
|
|
return _rms_norm_fwd_abstract(x_aval, gamma_aval, **kwargs)
|
|
|
|
@staticmethod
|
|
def lowering(ctx, x, gamma, *, eps):
|
|
return _rms_norm_fwd_cuda_lowering(ctx, x, gamma, eps)
|
|
|
|
@staticmethod
|
|
def impl(x, gamma, eps):
|
|
assert RmsNormFwdClass.inner_primitive is not None
|
|
out, rsigma = RmsNormFwdClass.inner_primitive.bind(x, gamma, eps=eps)
|
|
return out, rsigma
|
|
|
|
@staticmethod
|
|
def batcher(batched_args, batch_dims, *, eps):
|
|
_check_valid_batch_dims(batch_dims)
|
|
assert RmsNormFwdClass.outer_primitive is not None
|
|
x, gamma = batched_args
|
|
x_bdim, _ = batch_dims
|
|
|
|
out_bdims = x_bdim, x_bdim
|
|
return RmsNormFwdClass.outer_primitive.bind(x, gamma, eps=eps), out_bdims
|
|
|
|
@staticmethod
|
|
def infer_sharding_from_operands(eps : float, mesh : jax.sharding.Mesh,
|
|
arg_infos : Tuple[jax._src.api.ShapeDtypeStruct],
|
|
result_infos : Tuple[jax._src.core.ShapedArray]):
|
|
del eps, result_infos # Not needed for this example.
|
|
x_info, weight_info = arg_infos
|
|
assert len(x_info.shape) == 3
|
|
assert len(weight_info.shape) == 2
|
|
# partition() will force all dims to be replicated except the
|
|
# first dim of x that will be kept as is.
|
|
x_spec = arg_infos[0].sharding.spec
|
|
output_sharding = NamedSharding(mesh, PartitionSpec(x_spec[0], None, None))
|
|
invvar_sharding = NamedSharding(mesh, PartitionSpec(x_spec[0]))
|
|
return (output_sharding, invvar_sharding)
|
|
|
|
@staticmethod
|
|
def partition(eps : float, mesh : jax.sharding.Mesh,
|
|
arg_infos : Tuple[jax._src.api.ShapeDtypeStruct],
|
|
result_infos : Tuple[jax._src.api.ShapeDtypeStruct]):
|
|
del result_infos # Not needed for this example.
|
|
x_info, weight_info = arg_infos
|
|
assert len(x_info.shape) == 3
|
|
assert len(weight_info.shape) == 2
|
|
x_spec = arg_infos[0].sharding.spec
|
|
# We only support sharding on the batch dimensions.
|
|
# Force sharding on all others dimensions with None.
|
|
arg_shardings = (NamedSharding(mesh, PartitionSpec(x_spec[0], None, None)),
|
|
NamedSharding(mesh, PartitionSpec(None, None))) # TODO: TE don't force anything.
|
|
invvar_sharding = NamedSharding(mesh, PartitionSpec(x_spec[0]))
|
|
output_shardings = (arg_shardings[0], invvar_sharding)
|
|
# Sharded_impl only accepts positional arugments
|
|
# And they should be Jax traceable variables
|
|
impl = partial(RmsNormFwdClass.impl, eps=eps)
|
|
|
|
return mesh, impl, output_shardings, arg_shardings
|
|
|
|
register_primitive(RmsNormFwdClass)
|
|
|
|
class RmsNormBwdClass:
|
|
name = "rms_norm_bwd"
|
|
multiple_results = True
|
|
impl_static_args = (4,) # eps
|
|
inner_primitive = None
|
|
outer_primitive = None
|
|
|
|
@staticmethod
|
|
def abstract(grad_output, invvar, x, weight, eps): # pylint: disable=unused-argument
|
|
return _rms_norm_bwd_abstract(grad_output, invvar, x, weight, eps)
|
|
|
|
@staticmethod
|
|
def lowering(ctx, grad_output, invvar, x, weight, eps):
|
|
return _rms_norm_bwd_cuda_lowering(ctx, grad_output, invvar, x, weight, eps)
|
|
|
|
@staticmethod
|
|
def impl(grad_output, invvar, x, weight, eps):
|
|
assert RmsNormBwdClass.inner_primitive is not None
|
|
gx, gw, part_grad = RmsNormBwdClass.inner_primitive.bind(grad_output, invvar, x, weight, eps=eps)
|
|
return gx, gw, part_grad
|
|
|
|
@staticmethod
|
|
def batcher(batched_args, batch_dims, *, eps):
|
|
# TODO: Add to the tutorial!
|
|
_check_valid_batch_dims(batch_dims)
|
|
assert RmsNormBwdClass.outer_primitive is not None
|
|
x, gamma = batched_args
|
|
x_bdim, _ = batch_dims
|
|
|
|
out_bdims = x_bdim, x_bdim
|
|
return RmsNormBwdClass.outer_primitive.bind(x, gamma, eps=eps), out_bdims
|
|
|
|
@staticmethod
|
|
def infer_sharding_from_operands(eps : float, mesh : jax.sharding.Mesh,
|
|
arg_infos : Tuple[jax._src.api.ShapeDtypeStruct],
|
|
result_infos : Tuple[jax._src.core.ShapedArray]):
|
|
del eps, result_infos # Not needed for this example.
|
|
g_info, invvar_info, x_info, weight_info = arg_infos
|
|
assert len(g_info.shape) == 3
|
|
assert len(invvar_info.shape) == 1
|
|
assert len(x_info.shape) == 3
|
|
assert len(weight_info.shape) == 2
|
|
# partition() will force all dims to be replicated except the batch dimension.
|
|
x_spec = x_info.sharding.spec
|
|
output_sharding = NamedSharding(mesh, PartitionSpec(x_spec[0], None, None))
|
|
invvar_sharding = NamedSharding(mesh, PartitionSpec(None, None))
|
|
return (output_sharding, invvar_sharding, output_sharding, )
|
|
|
|
@staticmethod
|
|
def partition(eps : float, mesh : jax.sharding.Mesh,
|
|
arg_infos : Tuple[jax._src.api.ShapeDtypeStruct],
|
|
result_infos : Tuple[jax._src.api.ShapeDtypeStruct]):
|
|
del result_infos # Not needed for this example.
|
|
g_info, invvar_info, x_info, weight_info = arg_infos
|
|
assert len(g_info.shape) == 3
|
|
assert len(invvar_info.shape) == 1
|
|
assert len(x_info.shape) == 3
|
|
assert len(weight_info.shape) == 2
|
|
|
|
# We only support sharding on the batch dimensions.
|
|
# Force sharding on all others dimensions with None.
|
|
# Also force gx, x and invvar to have the same batch sharding/replication.
|
|
x_spec = x_info.sharding.spec
|
|
arg_shardings = (NamedSharding(mesh, PartitionSpec(x_spec[0], None, None)),
|
|
NamedSharding(mesh, PartitionSpec(x_spec[0],)),
|
|
NamedSharding(mesh, PartitionSpec(x_spec[0], None, None)),
|
|
NamedSharding(mesh, PartitionSpec(None, None)))
|
|
|
|
output_sharding = NamedSharding(mesh, PartitionSpec(x_spec[0], None, None))
|
|
invvar_sharding = NamedSharding(mesh, PartitionSpec(None, None))
|
|
output_shardings = (output_sharding, invvar_sharding, invvar_sharding)
|
|
|
|
|
|
# Sharded_impl only accepts positional arugments
|
|
# And they should be Jax traceable variables
|
|
def sharded_impl(g, invvar, x, weight):
|
|
grad_input, grad_weight, part_grad = RmsNormBwdClass.impl(
|
|
g, invvar, x, weight, eps=eps
|
|
)
|
|
# We need to sum the weight gradient from all partition.
|
|
# when the input is sharded and weights are replicated
|
|
global_weight = grad_weight
|
|
if x_spec[0]:
|
|
global_weight = jax.lax.psum(grad_weight, x_spec[0])
|
|
return grad_input, global_weight, part_grad
|
|
return mesh, sharded_impl, output_shardings, arg_shardings
|
|
|
|
register_primitive(RmsNormBwdClass)
|
|
|
|
def custom_p_rms_norm_fwd(x, weight, eps=1e-05):
|
|
output, invvar = RmsNormFwdClass.outer_primitive.bind(x, weight, eps=eps)
|
|
return output, (invvar, x, weight)
|
|
|
|
@partial(jax.custom_vjp, nondiff_argnums=(2,))
|
|
def custom_p_rms_norm(x, weight, eps=1e-05):
|
|
output, _ = custom_p_rms_norm_fwd(x, weight, eps=eps)
|
|
return output
|
|
|
|
def custom_p_rms_norm_bwd(eps, res, g):
|
|
invvar, x, weight = res
|
|
grad_input, grad_weight, part_grad = RmsNormBwdClass.outer_primitive.bind(
|
|
g, invvar, x, weight, eps=eps)
|
|
return grad_input, grad_weight
|
|
|
|
custom_p_rms_norm.defvjp(custom_p_rms_norm_fwd, custom_p_rms_norm_bwd)
|
|
|
|
########
|
|
# Test #
|
|
########
|
|
|
|
|
|
import jax
|
|
|
|
per_core_batch_size = 4
|
|
seq_len = 512
|
|
emb_dim = 512
|
|
assert jax.local_device_count() > 1, "Only 1 GPU, the example work, but it is this really what you want?"
|
|
x = jax.random.normal(
|
|
jax.random.PRNGKey(0),
|
|
shape=(jax.local_device_count() * per_core_batch_size, seq_len, emb_dim),
|
|
dtype=jnp.float16,
|
|
)
|
|
norm_shape = x.shape[-2:]
|
|
weight = jnp.ones(norm_shape, dtype=jnp.float16)
|
|
|
|
|
|
def ref_loss(x, weight):
|
|
predictions = rms_norm(x, weight)
|
|
return -jnp.mean(predictions**2)
|
|
|
|
ref_out = jax.grad(ref_loss, argnums=(0, 1))(x, weight)
|
|
|
|
def custom_p_loss(x, weight):
|
|
predictions = custom_p_rms_norm(x, weight)
|
|
return -jnp.mean(predictions**2)
|
|
|
|
with Mesh(jax.local_devices(), ("x",)):
|
|
def run_and_verify(loss):
|
|
pjitted = pjit(
|
|
jax.grad(loss, argnums=(0, 1)),
|
|
# Shard x by batch dimension and replicate weight on all devices.
|
|
in_shardings=(
|
|
PartitionSpec("x", None, None),
|
|
PartitionSpec(None, None),
|
|
),
|
|
# Shard the output by batch dimension and replicate weight grad on all devices.
|
|
out_shardings=(
|
|
PartitionSpec("x", None, None),
|
|
PartitionSpec(None, None),
|
|
),
|
|
)
|
|
hlo = pjitted.lower(x, weight).compile().as_text()
|
|
out = pjitted(x, weight)
|
|
print(hlo)
|
|
assert "all-reduce-done" in hlo, "The gradient will produce wrong value!"
|
|
if "all-gather-start" in hlo:
|
|
print("NOT OPTIMIZED, ALL_GATHER in the graph!")
|
|
return out
|
|
|
|
custom_p_out = run_and_verify(custom_p_loss)
|
|
|
|
|
|
for r, o in zip(ref_out, custom_p_out):
|
|
print(jnp.allclose(r, o, atol=1e-6, rtol=1e-6))
|