mirror of
https://github.com/ROCm/jax.git
synced 2025-04-15 19:36:06 +00:00
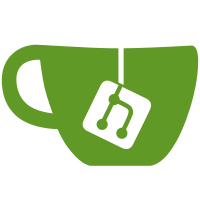
In some environments this appears to import the config module rather than the config object.
2904 lines
96 KiB
Python
2904 lines
96 KiB
Python
# Copyright 2018 The JAX Authors.
|
|
#
|
|
# Licensed under the Apache License, Version 2.0 (the "License");
|
|
# you may not use this file except in compliance with the License.
|
|
# You may obtain a copy of the License at
|
|
#
|
|
# https://www.apache.org/licenses/LICENSE-2.0
|
|
#
|
|
# Unless required by applicable law or agreed to in writing, software
|
|
# distributed under the License is distributed on an "AS IS" BASIS,
|
|
# WITHOUT WARRANTIES OR CONDITIONS OF ANY KIND, either express or implied.
|
|
# See the License for the specific language governing permissions and
|
|
# limitations under the License.
|
|
|
|
|
|
import collections
|
|
import contextlib
|
|
from functools import partial
|
|
import itertools
|
|
import operator
|
|
import re
|
|
import unittest
|
|
|
|
from absl.testing import absltest
|
|
from absl.testing import parameterized
|
|
|
|
import numpy as np
|
|
|
|
import jax
|
|
from jax._src import core
|
|
from jax import dtypes
|
|
from jax.errors import UnexpectedTracerError
|
|
from jax import lax
|
|
from jax import random
|
|
from jax._src import test_util as jtu
|
|
from jax import tree_util
|
|
from jax._src.util import unzip2
|
|
from jax.ad_checkpoint import checkpoint as new_checkpoint, checkpoint_policies
|
|
import jax.numpy as jnp # scan tests use numpy
|
|
import jax.scipy as jsp
|
|
from jax._src.lax import control_flow as lax_control_flow
|
|
from jax._src.lax.control_flow import for_loop
|
|
from jax._src.maps import xmap
|
|
|
|
jax.config.parse_flags_with_absl()
|
|
|
|
|
|
# Some tests are useful for testing both lax.cond and lax.switch. This function
|
|
# provides a lax.cond-compatible interface to a two-branch lax.switch. Several
|
|
# tests in this file are parameterized such that they either call into lax.cond
|
|
# or into this function.
|
|
def cond_via_switch(pred, true_fun, false_fun, op, *args):
|
|
if len(args) > 0:
|
|
assert len(args) == 1
|
|
true_op, _true_fun, false_op, _false_fun = true_fun, false_fun, op, args[0]
|
|
op = (false_op, true_op)
|
|
false_fun = lambda op: _false_fun(op[0])
|
|
true_fun = lambda op: _true_fun(op[1])
|
|
index = lax.convert_element_type(pred, np.int32)
|
|
return lax.switch(index, [false_fun, true_fun], op)
|
|
|
|
def cond_with_new_checkpoint(pred, true_fun, false_fun, op, *args):
|
|
if args:
|
|
true_op, _true_fun, false_op, _false_fun = true_fun, false_fun, op, args[0]
|
|
op = (false_op, true_op)
|
|
false_fun = lambda op: _false_fun(op[0])
|
|
true_fun = lambda op: _true_fun(op[1])
|
|
index = lax.convert_element_type(pred, np.int32)
|
|
fn = lambda index, op: lax.switch(index, [false_fun, true_fun], op)
|
|
return new_checkpoint(fn)(index, op)
|
|
|
|
COND_IMPLS = [
|
|
(lax.cond, 'cond'),
|
|
(cond_via_switch, 'switch'),
|
|
(cond_with_new_checkpoint, 'new_checkpoint'),
|
|
]
|
|
|
|
|
|
# We wanted to try all scan tests with the scan partial evaluation rule that
|
|
# happens under ad_checkpoint.checkpoint, so we make a scan wrapper which
|
|
# wraps a ad_checkpoint.checkpoint around the computation.
|
|
def scan_with_new_checkpoint(f, *args, **kwargs):
|
|
return new_checkpoint(partial(lax.scan, f, **kwargs),
|
|
policy=checkpoint_policies.nothing_saveable)(*args)
|
|
def scan_with_new_checkpoint2(f, *args, **kwargs):
|
|
return new_checkpoint(partial(lax.scan, f, **kwargs),
|
|
policy=checkpoint_policies.everything_saveable)(*args)
|
|
|
|
def scan_with_for(f, *args, **kwargs):
|
|
return for_loop.scan(f, *args, **kwargs)
|
|
|
|
def scan_with_remat_for(f, *args, **kwargs):
|
|
return jax.remat(lambda *args: for_loop.scan(f, *args, **kwargs))(*args)
|
|
|
|
SCAN_IMPLS_WITH_FOR = [
|
|
(lax.scan, 'unroll1'),
|
|
(partial(lax.scan, unroll=2), 'unroll2'),
|
|
(partial(lax.scan, _split_transpose=True), 'split_transpose'),
|
|
(scan_with_new_checkpoint , 'new_checkpoint'),
|
|
(scan_with_new_checkpoint2, 'new_checkpoint2'),
|
|
(scan_with_for, 'for_loop'),
|
|
(scan_with_remat_for, 'for_loop_remat'),
|
|
]
|
|
|
|
def while_loop_new_checkpoint(cond_fun, body_fun, init_val):
|
|
return new_checkpoint(partial(lax.while_loop, cond_fun, body_fun))(init_val)
|
|
|
|
WHILE_LOOP_IMPLS = [
|
|
(lax.while_loop, 'while_loop'),
|
|
(while_loop_new_checkpoint, 'new_checkpoint'),
|
|
]
|
|
|
|
|
|
def while_loop_reference(cond, body, carry):
|
|
while cond(carry):
|
|
carry = body(carry)
|
|
return carry
|
|
|
|
|
|
def scan_reference(f, init, xs):
|
|
carry = init
|
|
ys = []
|
|
for x in xs:
|
|
(carry, y) = f(carry, x)
|
|
ys.append(lax.reshape(y, (1,) + np.shape(y)))
|
|
ys = lax.concatenate(ys, 0)
|
|
return carry, ys
|
|
|
|
|
|
ignore_jit_of_pmap_warning = partial(
|
|
jtu.ignore_warning, message=".*jit-of-pmap.*")
|
|
|
|
|
|
class LaxControlFlowTest(jtu.JaxTestCase):
|
|
|
|
def setUp(self):
|
|
super().setUp()
|
|
lax_control_flow._initial_style_open_jaxpr.cache_clear()
|
|
lax_control_flow._initial_style_jaxpr.cache_clear()
|
|
lax_control_flow._initial_style_jaxprs_with_common_consts.cache_clear()
|
|
|
|
def testCallableErrors(self):
|
|
not_callable = 42
|
|
with self.assertRaisesRegex(TypeError, "lax.fori_loop.*callable.*"):
|
|
lax.fori_loop(0, 1, not_callable, 0)
|
|
with self.assertRaisesRegex(TypeError, "lax.while_loop.*callable.*"):
|
|
lax.while_loop(not_callable, not_callable, 0)
|
|
with self.assertRaisesRegex(TypeError, "lax.switch:.*callable.*"):
|
|
lax.switch(0, [not_callable])
|
|
with self.assertRaisesRegex(TypeError, "lax.cond.*callable.*"):
|
|
lax.cond(0, not_callable, not_callable)
|
|
with self.assertRaisesRegex(TypeError, "lax.scan.*callable.*"):
|
|
lax.scan(not_callable, 0, 1)
|
|
with self.assertRaisesRegex(TypeError, "lax.associative_scan.*callable.*"):
|
|
lax.associative_scan(not_callable, 0)
|
|
|
|
def testWhileWithTuple(self):
|
|
limit = 10
|
|
|
|
def loop_cond(state):
|
|
pos, _ = state
|
|
return lax.lt(pos, limit)
|
|
|
|
def loop_body(state):
|
|
pos, count = state
|
|
return (lax.add(pos, 1), lax.add(count, 1))
|
|
|
|
def loop(init):
|
|
result = lax.while_loop(loop_cond, loop_body, (init, 0))
|
|
_, count = result
|
|
return count
|
|
|
|
cloop = jax.jit(loop)
|
|
|
|
self.assertEqual(loop(2), limit - 2)
|
|
self.assertEqual(cloop(2), limit - 2)
|
|
self.assertEqual(cloop(2), limit - 2)
|
|
self.assertEqual(cloop(3), limit - 3)
|
|
|
|
def testWhileWithManyArgs(self):
|
|
nargs = 256
|
|
|
|
def loop_cond(state):
|
|
return lax.lt(state[0], 2)
|
|
|
|
def loop_body(state):
|
|
return tuple(lax.add(s, 1) for s in state)
|
|
|
|
_ = lax.while_loop(loop_cond, loop_body, (0,) * nargs)
|
|
|
|
def testNestedWhile(self):
|
|
|
|
def outer_loop(num): # pylint: disable=missing-docstring
|
|
def cond_fun(state):
|
|
num, i, _ = state
|
|
return lax.lt(i, num)
|
|
|
|
def body_fun(state):
|
|
num, i, count = state
|
|
return (num, lax.add(i, 1), inner_loop(i, count))
|
|
|
|
init_val = (num, 0, 0)
|
|
_, i, count = lax.while_loop(cond_fun, body_fun, init_val)
|
|
return (i, count)
|
|
|
|
def inner_loop(i, count): # pylint: disable=missing-docstring
|
|
def cond_fun(state):
|
|
i, j, _ = state
|
|
return lax.le(j, i)
|
|
|
|
def body_fun(state):
|
|
i, j, count = state
|
|
return (i, lax.add(j, 1), lax.add(count, 1))
|
|
|
|
init_val = (i, 0, count)
|
|
_, _, count = lax.while_loop(cond_fun, body_fun, init_val)
|
|
return count
|
|
|
|
cloop = jax.jit(outer_loop)
|
|
|
|
self.assertEqual(outer_loop(3), (3, 6))
|
|
self.assertEqual(cloop(3), (3, 6))
|
|
self.assertEqual(cloop(3), (3, 6))
|
|
self.assertEqual(cloop(2), (2, 3))
|
|
self.assertEqual(cloop(4), (4, 10))
|
|
|
|
def testWhileWithClosure(self):
|
|
|
|
def loop(init, local_limit, inc):
|
|
|
|
def loop_cond(state):
|
|
pos, _ = state
|
|
return lax.lt(pos, local_limit)
|
|
|
|
def loop_body(state):
|
|
effect[0] = True
|
|
pos, count = state
|
|
return (lax.add(pos, 1), lax.add(count, inc))
|
|
|
|
result = lax.while_loop(loop_cond, loop_body, (init, 0))
|
|
_, count = result
|
|
return count
|
|
|
|
cloop = jax.jit(loop)
|
|
|
|
limit = 10
|
|
effect = [False]
|
|
self.assertEqual(loop(2, limit, 1), limit - 2)
|
|
assert effect[0]
|
|
effect[0] = False
|
|
self.assertEqual(cloop(2, limit, 1), limit - 2)
|
|
assert effect[0]
|
|
effect[0] = False
|
|
self.assertEqual(cloop(2, limit, 1), limit - 2)
|
|
self.assertEqual(cloop(3, limit, 1), limit - 3)
|
|
assert not effect[0]
|
|
|
|
def testWhileWithClosureJit(self):
|
|
|
|
def loop(init, local_limit, inc):
|
|
|
|
def loop_cond(state):
|
|
pos, _ = state
|
|
return lax.lt(pos, local_limit)
|
|
|
|
def loop_body(state):
|
|
effect[0] = True
|
|
pos, count = state
|
|
f = lambda pos, inc: (lax.add(pos, 1), lax.add(count, inc))
|
|
return jax.jit(f)(pos, inc)
|
|
|
|
result = lax.while_loop(loop_cond, loop_body, (init, 0))
|
|
_, count = result
|
|
return count
|
|
|
|
cloop = jax.jit(loop)
|
|
|
|
limit = 10
|
|
effect = [False]
|
|
self.assertEqual(loop(2, limit, 1), limit - 2)
|
|
assert effect[0]
|
|
effect[0] = False
|
|
self.assertEqual(cloop(2, limit, 1), limit - 2)
|
|
assert effect[0]
|
|
effect[0] = False
|
|
self.assertEqual(cloop(2, limit, 1), limit - 2)
|
|
self.assertEqual(cloop(3, limit, 1), limit - 3)
|
|
assert not effect[0]
|
|
|
|
def testWhileTypeErrors(self):
|
|
"""Test typing error messages for while."""
|
|
tuple_treedef = jax.tree.structure((1., 1.))
|
|
leaf_treedef = jax.tree.structure(0.)
|
|
with self.assertRaisesRegex(TypeError,
|
|
re.escape(f"cond_fun must return a boolean scalar, but got pytree {tuple_treedef}.")):
|
|
lax.while_loop(lambda c: (1., 1.), lambda c: c, 0.)
|
|
with self.assertRaisesRegex(TypeError,
|
|
re.escape("cond_fun must return a boolean scalar, but got output type(s) [ShapedArray(float32[])].")):
|
|
lax.while_loop(lambda c: np.float32(1.), lambda c: c, np.float32(0.))
|
|
with self.assertRaisesRegex(TypeError,
|
|
re.escape("body_fun output and input must have same type structure, "
|
|
f"got {tuple_treedef} and {leaf_treedef}.")):
|
|
lax.while_loop(lambda c: True, lambda c: (1., 1.), 0.)
|
|
with self.assertRaisesWithLiteralMatch(TypeError,
|
|
("body_fun output and input must have identical types, got\n"
|
|
"('ShapedArray(bool[])', "
|
|
"'DIFFERENT ShapedArray(bool[]) vs. "
|
|
"ShapedArray(float32[])').")):
|
|
lax.while_loop(lambda c: True, lambda c: (True, True),
|
|
(np.bool_(True), np.float32(0.)))
|
|
|
|
def testNestedWhileWithDynamicUpdateSlice(self):
|
|
num = 5
|
|
|
|
def update_entry(arr, val, i, j):
|
|
val = lax.reshape(val, [1, 1])
|
|
return lax.dynamic_update_slice(arr, val, (i, j))
|
|
|
|
def outer_loop(arr): # pylint: disable=missing-docstring
|
|
|
|
def cond_fun(state):
|
|
i, num, _, _ = state
|
|
return lax.lt(i, num)
|
|
|
|
def body_fun(state):
|
|
i, num, arr, out = state
|
|
return (lax.add(i, 1), num, arr, inner_loop(i, arr, out))
|
|
|
|
out = np.zeros(arr.shape, dtype=arr.dtype)
|
|
init_val = (0, num, arr, out)
|
|
_, _, _, out = lax.while_loop(cond_fun, body_fun, init_val)
|
|
return out
|
|
|
|
def inner_loop(i, arr, out): # pylint: disable=missing-docstring
|
|
|
|
def cond_fun(state):
|
|
i, j, _, _ = state
|
|
return lax.le(j, i)
|
|
|
|
def body_fun(state):
|
|
i, j, arr, out = state
|
|
arr_i = lax.dynamic_index_in_dim(arr, i, 0, False)
|
|
arr_i_j = lax.dynamic_index_in_dim(arr_i, j, 0, False)
|
|
out = update_entry(out, arr_i_j, i, j)
|
|
return (i, lax.add(j, 1), arr, out)
|
|
|
|
init_val = (i, 0, arr, out)
|
|
_, _, _, out = lax.while_loop(cond_fun, body_fun, init_val)
|
|
return out
|
|
|
|
cloop = jax.jit(outer_loop)
|
|
arr = self.rng().randn(5, 5)
|
|
self.assertAllClose(outer_loop(arr), np.tril(arr), check_dtypes=False)
|
|
self.assertAllClose(cloop(arr), np.tril(arr), check_dtypes=False)
|
|
self.assertAllClose(cloop(arr), np.tril(arr), check_dtypes=False)
|
|
|
|
def testLoopWithConjunctionCondition(self):
|
|
def sum_first_n(arr, num): # pylint: disable=missing-docstring
|
|
def cond_fun(state):
|
|
arr, num, i, _ = state
|
|
return lax.bitwise_and(lax.lt(i, num), lax.lt(i, arr.shape[0]))
|
|
|
|
def body_fun(state):
|
|
arr, num, i, total = state
|
|
arr_i = lax.dynamic_index_in_dim(arr, i, 0, False)
|
|
return (arr, num, i + 1, total + arr_i)
|
|
|
|
init_val = (arr, num, 0, 0.)
|
|
_, _, _, total = lax.while_loop(cond_fun, body_fun, init_val)
|
|
return total
|
|
|
|
cfun = jax.jit(sum_first_n)
|
|
x = self.rng().randn(10).astype(jnp.float_)
|
|
|
|
for num in [0, 5, 10, 15]:
|
|
self.assertAllClose(sum_first_n(x, num), np.sum(x[:num]),
|
|
check_dtypes=False)
|
|
self.assertAllClose(cfun(x, num), np.sum(x[:num]), check_dtypes=False)
|
|
self.assertAllClose(cfun(x, num), np.sum(x[:num]), check_dtypes=False)
|
|
|
|
def testWhileLoopBatched(self):
|
|
def fun(x):
|
|
return lax.while_loop(lambda x: x < 3, lambda x: x + 2, x)
|
|
|
|
ans = jax.vmap(fun)(np.array([0, 1, 2, 3]))
|
|
expected = np.array([4, 3, 4, 3])
|
|
self.assertAllClose(ans, expected, check_dtypes=False)
|
|
|
|
fun = jax.jit(fun)
|
|
ans = jax.vmap(fun)(np.array([0, 1, 2, 3]))
|
|
expected = np.array([4, 3, 4, 3])
|
|
self.assertAllClose(ans, expected, check_dtypes=False)
|
|
|
|
def testWhileLoopAxisIndexBatched(self):
|
|
def fun(x):
|
|
return lax.while_loop(lambda x: x < lax.axis_index('i'), lambda x: x + 2, x)
|
|
|
|
ans = jax.vmap(fun, axis_name='i')(np.array([0, 0, 0, 0], dtype='int32'))
|
|
expected = np.array([0, 2, 2, 4])
|
|
self.assertAllClose(ans, expected, check_dtypes=False)
|
|
|
|
fun = jax.jit(fun)
|
|
ans = jax.vmap(fun, axis_name='i')(np.array([0, 0, 0, 0], dtype='int32'))
|
|
expected = np.array([0, 2, 2, 4])
|
|
self.assertAllClose(ans, expected, check_dtypes=False)
|
|
|
|
ans = jax.vmap(lambda _, x: fun(x), axis_name='i', in_axes=(0, None))(
|
|
np.array([0, 0, 0, 0]), 0)
|
|
expected = np.array([0, 2, 2, 4], dtype='int32')
|
|
self.assertAllClose(ans, expected, check_dtypes=False)
|
|
|
|
def testWhileLoopBatchedWithConstBody(self):
|
|
def f(x):
|
|
def body_fn(_): return jnp.asarray(0., dtype=jnp.float32)
|
|
def cond_fn(_): return jnp.logical_not(False) == False
|
|
return jax.lax.while_loop(cond_fn, body_fn, x)
|
|
x = jnp.arange(5, dtype=jnp.float32)
|
|
self.assertAllClose(jax.vmap(f)(x), x)
|
|
|
|
def testWhileLoopCondConstsBatched(self):
|
|
def fun(x, y):
|
|
return lax.while_loop(lambda x: x < y, lambda x: x + 2, x)
|
|
|
|
ans = jax.vmap(fun, in_axes=(None, 0))(0, np.array([2, 3]))
|
|
expected = np.array([2, 4])
|
|
self.assertAllClose(ans, expected, check_dtypes=False)
|
|
|
|
def testWhileLoopBodyConstsBatched(self):
|
|
def fun(x, y):
|
|
return lax.while_loop(lambda x: x < 3, lambda x: x + y, x)
|
|
|
|
ans = jax.vmap(fun, in_axes=(None, 0))(0, jnp.array([2, 3]))
|
|
expected = np.array([4, 3])
|
|
self.assertAllClose(ans, expected, check_dtypes=False)
|
|
|
|
def testWhileLoopTupleBatched(self):
|
|
def cond_fun(loop_carry):
|
|
x, y = loop_carry
|
|
return x + y < 5
|
|
|
|
def body_fun(loop_carry):
|
|
x, y = loop_carry
|
|
x = x + 1
|
|
return x, y
|
|
|
|
def fun(x, y):
|
|
return lax.while_loop(cond_fun, body_fun, (x, y))
|
|
|
|
ans = jax.vmap(fun)(np.array([0, 0]), np.array([1, 2]))
|
|
expected = (np.array([4, 3]), np.array([1, 2]))
|
|
self.assertAllClose(ans, expected, check_dtypes=False)
|
|
|
|
def test_issue_3204(self):
|
|
# Error during XLA code generation for vmap of nested loops
|
|
def test(a, b):
|
|
val = 0
|
|
i = 0
|
|
j = 0
|
|
|
|
condfun_1 = lambda inp: inp[1] < a + 1
|
|
condfun_2 = lambda inp: inp[2] < b + 1
|
|
|
|
def bodyfun_1(inp):
|
|
val, i, j = inp
|
|
j = 0
|
|
|
|
def bodyfun_2(inp):
|
|
val, i, j = inp
|
|
val += i + j
|
|
j += 1
|
|
return (val, i, j)
|
|
|
|
result = lax.while_loop(condfun_2, bodyfun_2, (val, i, j))
|
|
val = result[0]
|
|
i += 1
|
|
return (val, i, j)
|
|
|
|
result = lax.while_loop(condfun_1, bodyfun_1, (val, i, j))
|
|
return result[0]
|
|
|
|
arr = np.arange(5)
|
|
vmap_test = jax.vmap(test, (0, 0))
|
|
vmap_test(arr, arr)
|
|
|
|
def testForiLoopErrors(self):
|
|
"""Test typing error messages for fori_loop."""
|
|
with self.assertRaisesRegex(
|
|
TypeError, "arguments to fori_loop must have equal types"):
|
|
lax.fori_loop(np.int16(0), jnp.int32(10), (lambda i, c: c), jnp.float32(7))
|
|
|
|
def testForiLoopScalarLimits(self):
|
|
"""Test that scalar limits passed to fori_loop do not cause typing errors."""
|
|
body = lambda i, c: c + 1
|
|
init = jnp.float32(10)
|
|
|
|
result = lax.fori_loop(np.int16(0), 10, body, init)
|
|
self.assertEqual(result, init + 10)
|
|
|
|
result = lax.fori_loop(0, np.int16(10), body, init)
|
|
self.assertEqual(result, init + 10)
|
|
|
|
def test_fori_loop_supports_unrolling(self):
|
|
"""Test that we can unroll static fori_loops."""
|
|
body = lambda i, c: c + 1
|
|
init = jnp.float32(10)
|
|
|
|
result = lax.fori_loop(np.int16(0), 10, body, init,
|
|
unroll=3)
|
|
self.assertEqual(result, init + 10)
|
|
|
|
result = lax.fori_loop(0, np.int16(10), body, init,
|
|
unroll=2)
|
|
self.assertEqual(result, init + 10)
|
|
|
|
def test_fori_loop_supports_unrolling_with_bool(self):
|
|
"""Test that we can unroll static fori_loops."""
|
|
body = lambda i, c: c + 1
|
|
init = jnp.float32(10)
|
|
|
|
result = lax.fori_loop(np.int16(0), 10, body, init,
|
|
unroll=True)
|
|
self.assertEqual(result, init + 10)
|
|
|
|
result = lax.fori_loop(0, np.int16(10), body, init,
|
|
unroll=False)
|
|
self.assertEqual(result, init + 10)
|
|
|
|
def test_fori_loop_with_dynamic_indices_cannot_unroll(self):
|
|
"""Test that we can't unroll dynamic fori_loops."""
|
|
body = lambda i, c: c + 1
|
|
init = jnp.float32(10)
|
|
|
|
@jax.jit
|
|
def f(upper):
|
|
return lax.fori_loop(np.int16(0), upper, body, init,
|
|
unroll=3)
|
|
|
|
with self.assertRaisesRegex(ValueError, "Can only use `unroll`"):
|
|
f(10)
|
|
|
|
@parameterized.named_parameters(
|
|
{
|
|
"testcase_name": f"_{jit=}_{upper=}_{unroll=}",
|
|
"jit": jit,
|
|
"upper": upper,
|
|
"unroll": unroll,
|
|
}
|
|
for jit in (False, True)
|
|
for upper in (0, -1)
|
|
for unroll in (False, True)
|
|
)
|
|
def test_fori_loop_returns_init_with_nonpositive_length(
|
|
self, jit, upper, unroll
|
|
):
|
|
"""Test that `length <= 0` behaves like Python `range`."""
|
|
fori_loop_with_static_upper_and_lower = partial(
|
|
lax.fori_loop, 0, upper, lambda i, c: c + 1, unroll=unroll
|
|
)
|
|
if jit:
|
|
fori_loop_with_static_upper_and_lower = jax.jit(
|
|
fori_loop_with_static_upper_and_lower
|
|
)
|
|
init = jnp.float32(10)
|
|
self.assertEqual(fori_loop_with_static_upper_and_lower(init), init)
|
|
|
|
def testForiLoopBatched(self):
|
|
def body_fun(i, loop_carry):
|
|
x, y = loop_carry
|
|
x = x + 1
|
|
y = y + 2
|
|
return x, y
|
|
|
|
def fun(x):
|
|
return lax.fori_loop(0, 10, body_fun, (x, 0))
|
|
|
|
ans = jax.vmap(fun)(np.array([0, 1]))
|
|
expected = (np.array([10, 11]), np.array([20, 20]))
|
|
self.assertAllClose(ans, expected, check_dtypes=False)
|
|
|
|
def testForiLoopBatchedIssue1190(self):
|
|
cond_fun = lambda carry: carry[0] < 4
|
|
body_fun = lambda carry: (carry[0] + 1, carry[1] + 1)
|
|
f = lambda x: lax.while_loop(cond_fun, body_fun, (0, x))
|
|
jaxpr = jax.make_jaxpr(jax.vmap(f))(jnp.arange(3))
|
|
eqn = jaxpr.jaxpr.eqns[0]
|
|
self.assertIs(eqn.primitive, lax.while_p)
|
|
self.assertEqual(eqn.params['cond_jaxpr'].in_avals[0].shape, ())
|
|
|
|
def testForiLoopBasic(self):
|
|
def body_fun(i, tot):
|
|
return lax.add(tot, i)
|
|
|
|
def count(num):
|
|
return lax.fori_loop(0, num, body_fun, 0)
|
|
|
|
self.assertEqual(count(2), 1)
|
|
self.assertEqual(count(3), 3)
|
|
self.assertEqual(count(4), 6)
|
|
|
|
for args_maker in [lambda: [2], lambda: [3], lambda: [4]]:
|
|
self._CompileAndCheck(count, args_maker)
|
|
|
|
def testForiLoopClosure(self):
|
|
def count(num):
|
|
def body_fun(i, tot):
|
|
return lax.add(num, lax.add(tot, i))
|
|
return lax.fori_loop(0, num, body_fun, 0)
|
|
|
|
cfun = jax.jit(count)
|
|
|
|
self.assertEqual(count(2), 1 + 2**2)
|
|
self.assertEqual(count(2), cfun(2))
|
|
self.assertEqual(count(3), 3 + 3**2)
|
|
self.assertEqual(count(3), cfun(3))
|
|
self.assertEqual(count(4), 6 + 4**2)
|
|
self.assertEqual(count(4), cfun(4))
|
|
|
|
def testForiLoopTupleState(self):
|
|
def sum_first_n(arr, num):
|
|
def body_fun(i, state):
|
|
arr, total = state
|
|
arr_i = lax.dynamic_index_in_dim(arr, i, 0, False)
|
|
return (arr, lax.add(total, arr_i))
|
|
|
|
init_val = (arr, arr.dtype.type(0))
|
|
_, total = lax.fori_loop(0, lax.min(arr.shape[0], num), body_fun,
|
|
init_val)
|
|
return total
|
|
|
|
cfun = jax.jit(sum_first_n)
|
|
x = self.rng().randn(10).astype(jnp.float_)
|
|
|
|
for num in [0, 5, 10, 15]:
|
|
self.assertAllClose(sum_first_n(x, num), np.sum(x[:num]),
|
|
check_dtypes=False)
|
|
self.assertAllClose(cfun(x, num), np.sum(x[:num]), check_dtypes=False)
|
|
self.assertAllClose(cfun(x, num), np.sum(x[:num]), check_dtypes=False)
|
|
|
|
def testForiLoopDictState(self):
|
|
def sum_first_n(arr, num):
|
|
def body_fun(i, state):
|
|
arr, total = state['arr'], state['total']
|
|
arr_i = lax.dynamic_index_in_dim(arr, i, 0, False)
|
|
return {'arr': arr, 'total': lax.add(total, arr_i)}
|
|
|
|
init_val = {'arr': arr, 'total': arr.dtype.type(0)}
|
|
out_val = lax.fori_loop(0, lax.min(arr.shape[0], num), body_fun, init_val)
|
|
return out_val['total']
|
|
|
|
cfun = jax.jit(sum_first_n)
|
|
x = self.rng().randn(10).astype(jnp.float_)
|
|
|
|
for num in [0, 5, 10, 15]:
|
|
self.assertAllClose(sum_first_n(x, num), np.sum(x[:num]),
|
|
check_dtypes=False)
|
|
self.assertAllClose(cfun(x, num), np.sum(x[:num]), check_dtypes=False)
|
|
self.assertAllClose(cfun(x, num), np.sum(x[:num]), check_dtypes=False)
|
|
|
|
def testForiLoopEmptyTupleInState(self):
|
|
def sum_first_n(arr, num):
|
|
def body_fun(i, state):
|
|
arr, total, _ = state
|
|
arr_i = lax.dynamic_index_in_dim(arr, i, 0, False)
|
|
return (arr, lax.add(total, arr_i), ())
|
|
|
|
init_val = (arr, arr.dtype.type(0), ())
|
|
_, tot, _ = lax.fori_loop(0, lax.min(arr.shape[0], num), body_fun, init_val)
|
|
return tot
|
|
|
|
cfun = jax.jit(sum_first_n)
|
|
x = self.rng().randn(10).astype(jnp.float_)
|
|
|
|
for num in [0, 5, 10, 15]:
|
|
self.assertAllClose(sum_first_n(x, num), np.sum(x[:num]),
|
|
check_dtypes=False)
|
|
self.assertAllClose(cfun(x, num), np.sum(x[:num]), check_dtypes=False)
|
|
self.assertAllClose(cfun(x, num), np.sum(x[:num]), check_dtypes=False)
|
|
|
|
def testForiLoopIssue8152(self):
|
|
y = lax.fori_loop(lower=0, upper=0, body_fun=lambda x, i: x + i, init_val=1.)
|
|
self.assertAllClose(y, 1., check_dtypes=False)
|
|
|
|
# trivial fori_loop should work - even when jit is disabled
|
|
with jax.disable_jit():
|
|
y = lax.fori_loop(lower=0, upper=0, body_fun=lambda x, i: x + i, init_val=1.)
|
|
self.assertAllClose(y, 1., check_dtypes=False)
|
|
|
|
# scan with length 0 should work with jit, but raise an error without
|
|
def should_raise_wo_jit():
|
|
carry, out = lax.scan(lambda c, x: (c + x, x), 0., np.array([]))
|
|
return carry
|
|
self.assertAllClose(should_raise_wo_jit(), 0., check_dtypes=False)
|
|
with jax.disable_jit():
|
|
self.assertRaises(ValueError, should_raise_wo_jit)
|
|
|
|
def testCond(self):
|
|
def fun(x):
|
|
if x < 3:
|
|
return (x, x)
|
|
else:
|
|
y = lax.mul(2, x)
|
|
return y, lax.mul(2, y)
|
|
|
|
@jax.jit
|
|
def cfun(x):
|
|
def false_fun(x):
|
|
y = lax.mul(2, x)
|
|
return y, lax.mul(2, y)
|
|
return lax.cond(lax.lt(x, 3), lambda x: (x, x), false_fun, x)
|
|
|
|
self.assertEqual(fun(0), cfun(0))
|
|
self.assertEqual(fun(0), (0, 0))
|
|
self.assertEqual(fun(1), cfun(1))
|
|
self.assertEqual(fun(1), (1, 1))
|
|
self.assertEqual(fun(2), cfun(2))
|
|
self.assertEqual(fun(2), (2, 2))
|
|
self.assertEqual(fun(3), cfun(3))
|
|
self.assertEqual(fun(3), (6, 12))
|
|
self.assertEqual(fun(4), cfun(4))
|
|
self.assertEqual(fun(4), (8, 16))
|
|
|
|
def testCondPredIsNone(self):
|
|
# see https://github.com/google/jax/issues/11574
|
|
def f(pred, x):
|
|
return lax.cond(pred, lambda x: x + 1, lambda x: x + 2, x)
|
|
|
|
self.assertRaisesRegex(TypeError, "cond predicate is None",
|
|
lambda: f(None, 1.))
|
|
self.assertRaisesRegex(TypeError, "cond predicate is None",
|
|
lambda: jax.jit(f)(None, 1.))
|
|
|
|
def testCondTwoOperands(self):
|
|
# see https://github.com/google/jax/issues/8469
|
|
add, mul = lax.add, lax.mul
|
|
|
|
def fun(x):
|
|
return add(x, x) if x == 0 else mul(x, x)
|
|
|
|
def cfun(x):
|
|
return lax.cond(x == 0, add, mul, x, x)
|
|
|
|
self.assertEqual(fun(0), cfun(0))
|
|
self.assertEqual(fun(1), cfun(1))
|
|
cfun = jax.jit(cfun)
|
|
self.assertEqual(fun(0), cfun(0))
|
|
self.assertEqual(fun(1), cfun(1))
|
|
|
|
def testCondThreeOperands(self):
|
|
add = lambda x, y, z: x + y + z
|
|
mul = lambda x, y, z: x * y * z
|
|
|
|
def fun(x):
|
|
return add(x, x, x) if x == 0 else mul(x, x, x)
|
|
|
|
def cfun(x):
|
|
return lax.cond(x == 0, add, mul, x, x, x)
|
|
|
|
self.assertEqual(fun(0), cfun(0))
|
|
self.assertEqual(fun(1), cfun(1))
|
|
cfun = jax.jit(cfun)
|
|
self.assertEqual(fun(0), cfun(0))
|
|
self.assertEqual(fun(1), cfun(1))
|
|
|
|
def testCondCallableOperands(self):
|
|
# see https://github.com/google/jax/issues/16413
|
|
|
|
@tree_util.register_pytree_node_class
|
|
class Foo:
|
|
def __init__(self, x):
|
|
self.x = x
|
|
|
|
def __call__(self, *xs):
|
|
assert False
|
|
return xs
|
|
|
|
def tree_flatten(self):
|
|
return (self.x,), None
|
|
|
|
@classmethod
|
|
def tree_unflatten(cls, _, xs):
|
|
return cls(*xs)
|
|
|
|
f_00 = lambda a, b: a + b
|
|
f_01 = lambda a, b: a + b.x
|
|
f_10 = lambda a, b: a.x + b
|
|
f_11 = lambda a, b: a.x + b.x
|
|
|
|
# these don't raise
|
|
a = lax.cond(True, f_00, f_00, 3, 4)
|
|
b = lax.cond(True, f_01, f_01, 3, Foo(4))
|
|
c = lax.cond(True, f_10, f_10, Foo(3), 4)
|
|
d = lax.cond(True, f_11, f_11, Foo(3), Foo(4))
|
|
self.assertEqual(a, b)
|
|
self.assertEqual(a, c)
|
|
self.assertEqual(a, d)
|
|
|
|
def testSwitch(self):
|
|
def branch(x):
|
|
y = lax.mul(2, x)
|
|
return y, lax.mul(2, y)
|
|
|
|
branches = [lambda x: (x, x),
|
|
branch,
|
|
lambda x: (x, -x)]
|
|
|
|
def fun(x):
|
|
if x <= 0:
|
|
return branches[0](x)
|
|
elif x == 1:
|
|
return branches[1](x)
|
|
else:
|
|
return branches[2](x)
|
|
|
|
def cfun(x):
|
|
return lax.switch(x, branches, x)
|
|
|
|
self.assertEqual(fun(-1), cfun(-1))
|
|
self.assertEqual(fun(0), cfun(0))
|
|
self.assertEqual(fun(1), cfun(1))
|
|
self.assertEqual(fun(2), cfun(2))
|
|
self.assertEqual(fun(3), cfun(3))
|
|
|
|
cfun = jax.jit(cfun)
|
|
|
|
self.assertEqual(fun(-1), cfun(-1))
|
|
self.assertEqual(fun(0), cfun(0))
|
|
self.assertEqual(fun(1), cfun(1))
|
|
self.assertEqual(fun(2), cfun(2))
|
|
self.assertEqual(fun(3), cfun(3))
|
|
|
|
def testSwitchMultiOperands(self):
|
|
branches = [lax.add, lax.mul]
|
|
|
|
def fun(x):
|
|
i = 0 if x <= 0 else 1
|
|
return branches[i](x, x)
|
|
|
|
def cfun(x):
|
|
return lax.switch(x, branches, x, x)
|
|
|
|
self.assertEqual(fun(-1), cfun(-1))
|
|
self.assertEqual(fun(0), cfun(0))
|
|
self.assertEqual(fun(1), cfun(1))
|
|
self.assertEqual(fun(2), cfun(2))
|
|
cfun = jax.jit(cfun)
|
|
self.assertEqual(fun(-1), cfun(-1))
|
|
self.assertEqual(fun(0), cfun(0))
|
|
self.assertEqual(fun(1), cfun(1))
|
|
self.assertEqual(fun(2), cfun(2))
|
|
|
|
def testSwitchResidualsMerge(self):
|
|
def get_conds(fun):
|
|
jaxpr = jax.make_jaxpr(jax.grad(fun))(0., 0)
|
|
return [eqn for eqn in jaxpr.jaxpr.eqns if eqn.primitive.name == 'cond']
|
|
|
|
def branch_invars_len(cond_eqn):
|
|
lens = [len(jaxpr.jaxpr.invars) for jaxpr in cond_eqn.params['branches']]
|
|
assert len(set(lens)) == 1
|
|
return lens[0]
|
|
|
|
def branch_outvars_len(cond_eqn):
|
|
lens = [len(jaxpr.jaxpr.outvars) for jaxpr in cond_eqn.params['branches']]
|
|
assert len(set(lens)) == 1
|
|
return lens[0]
|
|
|
|
branches1 = [
|
|
lambda x: jnp.sin(x),
|
|
lambda x: jnp.cos(x)] # branch residuals overlap, should be reused
|
|
branches2 = branches1 + [
|
|
lambda x: jnp.sinh(x)] # another overlapping residual, expect reuse
|
|
branches3 = branches2 + [
|
|
lambda x: jnp.sin(x) + jnp.cos(x)] # requires one more residual slot
|
|
def fun1(x, i):
|
|
return lax.switch(i + 1, branches1, x)
|
|
def fun2(x, i):
|
|
return lax.switch(i + 1, branches2, x)
|
|
def fun3(x, i):
|
|
return lax.switch(i + 1, branches3, x)
|
|
|
|
fwd1, bwd1 = get_conds(fun1)
|
|
fwd2, bwd2 = get_conds(fun2)
|
|
fwd3, bwd3 = get_conds(fun3)
|
|
|
|
fwd1_num_out = branch_outvars_len(fwd1)
|
|
fwd2_num_out = branch_outvars_len(fwd2)
|
|
fwd3_num_out = branch_outvars_len(fwd3)
|
|
assert fwd1_num_out == fwd2_num_out
|
|
assert fwd3_num_out == fwd2_num_out + 1
|
|
|
|
bwd1_num_in = branch_invars_len(bwd1)
|
|
bwd2_num_in = branch_invars_len(bwd2)
|
|
bwd3_num_in = branch_invars_len(bwd3)
|
|
assert bwd1_num_in == bwd2_num_in
|
|
assert bwd3_num_in == bwd2_num_in + 1
|
|
|
|
def testOneBranchSwitch(self):
|
|
branch = lambda x: -x
|
|
f = lambda i, x: lax.switch(i, [branch], x)
|
|
x = 7.
|
|
self.assertEqual(f(-1, x), branch(x))
|
|
self.assertEqual(f(0, x), branch(x))
|
|
self.assertEqual(f(1, x), branch(x))
|
|
cf = jax.jit(f)
|
|
self.assertEqual(cf(-1, x), branch(x))
|
|
self.assertEqual(cf(0, x), branch(x))
|
|
self.assertEqual(cf(1, x), branch(x))
|
|
cf = jax.jit(f, static_argnums=0)
|
|
self.assertEqual(cf(-1, x), branch(x))
|
|
self.assertEqual(cf(0, x), branch(x))
|
|
self.assertEqual(cf(1, x), branch(x))
|
|
|
|
def testIssue1379(self):
|
|
def fun(pred):
|
|
return lax.cond(pred, lambda x: (True, x), lambda x: (False, x), pred)
|
|
|
|
@jax.jit
|
|
def cfun(pred):
|
|
return fun(pred)
|
|
|
|
self.assertEqual(fun(0), cfun(0), (False,0))
|
|
self.assertEqual(fun(0.), cfun(0.), (False,0.))
|
|
self.assertEqual(fun(1), cfun(1), (True,1))
|
|
self.assertEqual(fun(1.), cfun(1.), (True,1.))
|
|
|
|
# test that proper errors are raised for wrong types
|
|
for pred in ["abc", [], [1,2]]:
|
|
for f in [fun, cfun]:
|
|
self.assertRaises(TypeError, f, pred)
|
|
|
|
@parameterized.named_parameters(
|
|
{"testcase_name": f"_{name}", "cond": cond}
|
|
for cond, name in COND_IMPLS)
|
|
def testNestedCond(self, cond):
|
|
def fun(x):
|
|
if x < 2:
|
|
return lax.mul(2, x)
|
|
else:
|
|
if x < 5:
|
|
return lax.mul(3, x)
|
|
else:
|
|
return lax.mul(4, x)
|
|
|
|
@jax.jit
|
|
def cfun(x):
|
|
return cond(
|
|
lax.lt(x, 2),
|
|
lambda x: lax.mul(2, x),
|
|
lambda x: cond(lax.lt(x, 5),
|
|
x, lambda x: lax.mul(3, x),
|
|
4, lambda y: lax.mul(y, x)),
|
|
x)
|
|
|
|
self.assertEqual(cfun(1), 2)
|
|
self.assertEqual(cfun(3), 9)
|
|
self.assertEqual(cfun(6), 24)
|
|
self.assertEqual(cfun(1), fun(1))
|
|
self.assertEqual(cfun(3), fun(3))
|
|
self.assertEqual(cfun(6), fun(6))
|
|
|
|
def testCondTypeErrors(self):
|
|
"""Test typing error messages for cond."""
|
|
with self.assertRaisesRegex(TypeError,
|
|
re.escape("Pred type must be either boolean or number, got <function")):
|
|
lax.cond(lambda x: True, lambda top: 2., lambda fop: 3., 1.)
|
|
with self.assertRaisesRegex(TypeError,
|
|
re.escape("Pred must be a scalar, got foo of type <class 'str'>")):
|
|
lax.cond("foo", lambda top: 2., lambda fop: 3., 1.)
|
|
with self.assertRaisesRegex(TypeError,
|
|
re.escape("Pred must be a scalar, got (1.0, 1.0) of type <class 'tuple'>")):
|
|
lax.cond((1., 1.), lambda top: 2., lambda fop: 3., 1.)
|
|
with self.assertRaisesRegex(TypeError,
|
|
re.escape("true_fun and false_fun output must have same type structure, "
|
|
f"got {jax.tree.structure(2.)} and {jax.tree.structure((3., 3.))}.")):
|
|
lax.cond(True, lambda top: 2., lambda fop: (3., 3.), 1.)
|
|
with self.assertRaisesRegex(
|
|
TypeError,
|
|
"true_fun and false_fun output must have identical types, got\n"
|
|
r"DIFFERENT ShapedArray\(float32\[1\]\) vs. "
|
|
r"ShapedArray\(float32\[\].*\)."):
|
|
lax.cond(True,
|
|
lambda top: jnp.array([1.], jnp.float32),
|
|
lambda fop: jnp.float32(1.),
|
|
1.)
|
|
|
|
def testSwitchErrors(self):
|
|
"""Test typing error messages for switch."""
|
|
with self.assertRaisesRegex(TypeError,
|
|
re.escape("Index type must be an integer, got <function")):
|
|
lax.switch(lambda x: True, [lambda _: 2., lambda _: 3.], 1.)
|
|
with self.assertRaisesRegex(TypeError,
|
|
re.escape("Index type must be an integer, got foo.")):
|
|
lax.switch("foo", [lambda _: 2., lambda _: 3.], 1.)
|
|
with self.assertRaisesRegex(TypeError,
|
|
re.escape("Branch index must be scalar, got (1.0, 1.0) of shape (2,).")):
|
|
lax.switch((1., 1.), [lambda _: 2., lambda _: 3.], 1.)
|
|
with self.assertRaisesRegex(ValueError,
|
|
re.escape("Empty branch sequence")):
|
|
lax.switch(0, [], 1.)
|
|
with self.assertRaisesRegex(TypeError,
|
|
re.escape("branch 0 and 1 outputs must have same type structure, "
|
|
f"got {jax.tree.structure(2.)} and {jax.tree.structure((3., 3.))}.")):
|
|
lax.switch(1, [lambda _: 2., lambda _: (3., 3.)], 1.)
|
|
with self.assertRaisesRegex(
|
|
TypeError,
|
|
"branch 0 and 1 outputs must have identical types, got\n"
|
|
r"DIFFERENT ShapedArray\(float32\[1\]\) "
|
|
r"vs. ShapedArray\(float32\[\].*\)."):
|
|
lax.switch(1, [lambda _: jnp.array([1.], jnp.float32),
|
|
lambda _: jnp.float32(1.)],
|
|
1.)
|
|
|
|
def testCondOneBranchConstant(self):
|
|
def fun(x):
|
|
if x < 3:
|
|
return 5.
|
|
else:
|
|
return x
|
|
|
|
@jax.jit
|
|
def cfun(x):
|
|
return lax.cond(lax.lt(x, 3), lambda x: 5, lambda x: x, x)
|
|
|
|
self.assertEqual(fun(0), cfun(0))
|
|
self.assertEqual(cfun(0), 5)
|
|
self.assertEqual(fun(4), cfun(4))
|
|
self.assertEqual(cfun(4), 4)
|
|
|
|
def testCondOneBranchConstantTuple(self):
|
|
def fun(x):
|
|
if x < 3:
|
|
return (1., 2., 3.)
|
|
else:
|
|
return (x, 2., 4.)
|
|
|
|
@jax.jit
|
|
def cfun(x):
|
|
return lax.cond(lax.lt(x, 3),
|
|
lambda x: (1, 2., 3.),
|
|
lambda x: (x, 2., 4.),
|
|
x)
|
|
|
|
self.assertEqual(fun(0), cfun(0))
|
|
self.assertEqual(cfun(0), (1, 2., 3.))
|
|
self.assertEqual(fun(4), cfun(4))
|
|
self.assertEqual(cfun(4), (4, 2., 4.))
|
|
|
|
def testCondBatched(self):
|
|
def fun(x, y, z):
|
|
pred = lax.lt(x, 3)
|
|
true_fun = lambda y: y
|
|
false_fun = lambda z: lax.neg(z)
|
|
return lax.cond(pred, y, true_fun, z, false_fun)
|
|
|
|
# these cases stay as cond
|
|
x = jnp.array(2)
|
|
y = jnp.array([1, 2])
|
|
z = jnp.array([3, 4])
|
|
ans = jax.vmap(fun, (None, 0, 0))(x, y, z)
|
|
jaxpr = jax.make_jaxpr(jax.vmap(fun, (None, 0, 0)))(x, y, z)
|
|
expected = np.array([1, 2])
|
|
self.assertAllClose(ans, expected, check_dtypes=False)
|
|
assert "select" not in str(jaxpr)
|
|
|
|
x = jnp.array(4)
|
|
ans = jax.vmap(fun, (None, 0, 0))(x, y, z)
|
|
jaxpr = jax.make_jaxpr(jax.vmap(fun, (None, 0, 0)))(x, y, z)
|
|
expected = np.array([-3, -4])
|
|
self.assertAllClose(ans, expected, check_dtypes=False)
|
|
assert "select" not in str(jaxpr)
|
|
|
|
fun = jax.jit(fun)
|
|
ans = jax.vmap(fun, (None, 0, 0))(x, y, z)
|
|
expected = np.array([-3, -4])
|
|
self.assertAllClose(ans, expected, check_dtypes=False)
|
|
|
|
z = jnp.array(5)
|
|
ans = jax.vmap(fun, (None, 0, None))(x, y, z)
|
|
jaxpr = jax.make_jaxpr(jax.vmap(fun, (None, 0, None)))(x, y, z)
|
|
expected = np.array([-5, -5])
|
|
self.assertAllClose(ans, expected, check_dtypes=False)
|
|
assert "select" not in str(jaxpr)
|
|
|
|
# these cases become select
|
|
x = jnp.array([2, 4])
|
|
ans = jax.vmap(fun, (0, 0, None))(x, y, z)
|
|
jaxpr = jax.make_jaxpr(jax.vmap(fun, (0, 0, None)))(x, y, z)
|
|
expected = np.array([1, -5])
|
|
self.assertAllClose(ans, expected, check_dtypes=False)
|
|
assert "select" in str(jaxpr)
|
|
|
|
z = jnp.array([3, 4])
|
|
ans = jax.vmap(fun)(x, y, z)
|
|
jaxpr = jax.make_jaxpr(jax.vmap(fun))(x, y, z)
|
|
expected = np.array([1, -4])
|
|
self.assertAllClose(ans, expected, check_dtypes=False)
|
|
assert "select" in str(jaxpr)
|
|
|
|
def testSwitchBatched(self):
|
|
def fun(index, x, y, z):
|
|
branches = [lambda xyz: xyz[0],
|
|
lambda xyz: lax.neg(xyz[1]),
|
|
lambda xyz: lax.sign(xyz[2])]
|
|
return lax.switch(index, branches, (x, y, z))
|
|
|
|
# these cases stay as cond
|
|
x = jnp.array(0)
|
|
y = jnp.array([1, 2])
|
|
z = jnp.array([3, 4])
|
|
w = jnp.array(9)
|
|
ans = jax.vmap(fun, (None, 0, 0, None))(x, y, z, w)
|
|
jaxpr = jax.make_jaxpr(jax.vmap(fun, (None, 0, 0, None)))(x, y, z, w)
|
|
expected = np.array([1, 2])
|
|
self.assertAllClose(ans, expected, check_dtypes=False)
|
|
assert "select" not in str(jaxpr)
|
|
|
|
x = jnp.array(1)
|
|
ans = jax.vmap(fun, (None, 0, 0, None))(x, y, z, w)
|
|
jaxpr = jax.make_jaxpr(jax.vmap(fun, (None, 0, 0, None)))(x, y, z, w)
|
|
expected = np.array([-3, -4])
|
|
self.assertAllClose(ans, expected, check_dtypes=False)
|
|
assert "select" not in str(jaxpr)
|
|
|
|
fun = jax.jit(fun)
|
|
ans = jax.vmap(fun, (None, 0, 0, None))(x, y, z, w)
|
|
expected = np.array([-3, -4])
|
|
self.assertAllClose(ans, expected, check_dtypes=False)
|
|
|
|
z = jnp.array(5)
|
|
ans = jax.vmap(fun, (None, 0, None, None))(x, y, z, w)
|
|
jaxpr = jax.make_jaxpr(jax.vmap(fun, (None, 0, None, None)))(x, y, z, w)
|
|
expected = np.array([-5, -5])
|
|
self.assertAllClose(ans, expected, check_dtypes=False)
|
|
assert "select" not in str(jaxpr)
|
|
|
|
# these cases become select
|
|
x = jnp.array([0, 1])
|
|
ans = jax.vmap(fun, (0, 0, None, None))(x, y, z, w)
|
|
jaxpr = jax.make_jaxpr(jax.vmap(fun, (0, 0, None, None)))(x, y, z, w)
|
|
expected = np.array([1, -5])
|
|
self.assertAllClose(ans, expected, check_dtypes=False)
|
|
assert "select" in str(jaxpr)
|
|
|
|
z = jnp.array([3, 4])
|
|
w = jnp.array([9, 9])
|
|
ans = jax.vmap(fun)(x, y, z, w)
|
|
jaxpr = jax.make_jaxpr(jax.vmap(fun))(x, y, z, w)
|
|
expected = np.array([1, -4])
|
|
self.assertAllClose(ans, expected, check_dtypes=False)
|
|
assert "select" in str(jaxpr)
|
|
|
|
def testCondJVP(self):
|
|
def fun_ref(x):
|
|
if x < 3:
|
|
return (x, x)
|
|
else:
|
|
y = 2 * x
|
|
return y, 2 * y
|
|
|
|
def fun(x):
|
|
def false_fun(x):
|
|
y = 2 * x
|
|
return y, 2 * y
|
|
return lax.cond(x < 3, lambda x: (x, x), false_fun, x)
|
|
|
|
x = 3.14
|
|
ans = jax.jvp(fun, (x,), (x,))
|
|
expected = jax.jvp(fun_ref, (x,), (x,))
|
|
self.assertAllClose(ans, expected, check_dtypes=False)
|
|
jtu.check_grads(fun, (x,), order=2, modes=["fwd"])
|
|
|
|
x = 2.72
|
|
ans = jax.jvp(fun, (x,), (x,))
|
|
expected = jax.jvp(fun_ref, (x,), (x,))
|
|
self.assertAllClose(ans, expected, check_dtypes=False)
|
|
jtu.check_grads(fun, (x,), order=2, modes=["fwd"])
|
|
|
|
def testSwitchJVP(self):
|
|
def branch(x):
|
|
y = 2 * x
|
|
return y, 2 * y
|
|
|
|
branches = [lambda x: (x, x),
|
|
branch,
|
|
lambda x: (x, -x)]
|
|
|
|
def fun_ref(x):
|
|
idx = x // 1
|
|
if idx <= 0:
|
|
return branches[0](x)
|
|
elif idx == 1:
|
|
return branches[1](x)
|
|
else:
|
|
return branches[2](x)
|
|
|
|
def fun(x):
|
|
idx = lax.convert_element_type(x // 1, np.int32)
|
|
return lax.switch(idx, branches, x)
|
|
|
|
for x in [-0.7, 0.7, 1.7, 2.7, 3.7]:
|
|
ans = jax.jvp(fun, (x,), (x,))
|
|
expected = jax.jvp(fun_ref, (x,), (x,))
|
|
self.assertAllClose(ans, expected, check_dtypes=False)
|
|
jtu.check_grads(fun, (x,), order=2, modes=["fwd"])
|
|
|
|
@parameterized.named_parameters(
|
|
{"testcase_name": f"_{name}", "cond": cond}
|
|
for cond, name in COND_IMPLS)
|
|
def testCondJVP2(self, cond):
|
|
def fun_ref(x):
|
|
if x < 3:
|
|
return 2.
|
|
else:
|
|
return 2. * x
|
|
|
|
def fun(x):
|
|
return cond(x < 3, None, lambda _: 2., x, lambda x: 2. * x)
|
|
|
|
x = 3.14
|
|
ans = jax.jvp(fun, (x,), (x,))
|
|
expected = jax.jvp(fun_ref, (x,), (x,))
|
|
self.assertAllClose(ans, expected, check_dtypes=False)
|
|
jtu.check_grads(fun, (x,), order=2, modes=["fwd"])
|
|
|
|
x = 2.72
|
|
ans = jax.jvp(fun, (x,), (x,))
|
|
expected = jax.jvp(fun_ref, (x,), (x,))
|
|
self.assertAllClose(ans, expected, check_dtypes=False)
|
|
jtu.check_grads(fun, (x,), order=2, modes=["fwd"])
|
|
|
|
def testCondGrad(self):
|
|
def f_ref(x):
|
|
return 3. * x if x < 2 else jnp.sin(x)
|
|
|
|
@jax.jit
|
|
def f(x):
|
|
return lax.cond(x < 2, lambda x: 3. * x, lambda x: jnp.sin(x), x)
|
|
|
|
x = 2.14
|
|
ans = jax.grad(f)(x)
|
|
expected = jax.grad(f_ref)(x)
|
|
self.assertAllClose(ans, expected, check_dtypes=False)
|
|
jtu.check_grads(f, (x,), order=2, modes=["fwd", "rev"])
|
|
|
|
x = 1.72
|
|
ans = jax.grad(f)(x)
|
|
expected = jax.grad(f_ref)(x)
|
|
self.assertAllClose(ans, expected, check_dtypes=False)
|
|
jtu.check_grads(f, (x,), order=2, modes=["fwd", "rev"])
|
|
|
|
def testCondGradVmapNan(self):
|
|
eps = 1e-3
|
|
|
|
def safe1(x):
|
|
return lax.cond(x < eps, lambda _: eps, lambda _: jnp.sqrt(x), ())
|
|
|
|
out = jax.grad(lambda x: jax.vmap(safe1)(x).sum())(np.zeros(10))
|
|
self.assertFalse(np.isnan(out).any())
|
|
|
|
def testSwitchGrad(self):
|
|
branches = [lambda x: 3. * x,
|
|
lambda x: jnp.sin(x),
|
|
lambda x: -x]
|
|
|
|
def f_ref(x):
|
|
idx = x // 1
|
|
if idx <= 0:
|
|
return branches[0](x)
|
|
elif idx == 1:
|
|
return branches[1](x)
|
|
else:
|
|
return branches[2](x)
|
|
|
|
@jax.jit
|
|
def f(x):
|
|
idx = lax.convert_element_type(x // 1, np.int32)
|
|
return lax.switch(idx, branches, x)
|
|
|
|
for x in [-0.7, 0.7, 1.7, 2.7, 3.7]:
|
|
ans = jax.grad(f)(x)
|
|
expected = jax.grad(f_ref)(x)
|
|
self.assertAllClose(ans, expected, check_dtypes=False)
|
|
jtu.check_grads(f, (x,), order=2, modes=["fwd", "rev"])
|
|
|
|
def testSwitchGradWithWeakTypeMismatch(self): # issue #4696, PR #4896
|
|
dtype = dtypes.canonicalize_dtype(np.float64)
|
|
dtype = jnp.float32 if dtype == jnp.float32 else jnp.float64
|
|
|
|
branches = [
|
|
lambda x: x, # This preserves the weak type of x.
|
|
lambda x: x + dtype(1), # This strips the weak type of x.
|
|
]
|
|
|
|
def f_ref(x):
|
|
i = x.astype(jnp.int32)
|
|
return branches[i](x)
|
|
|
|
def f(x):
|
|
return lax.switch(x.astype(jnp.int32), branches, x)
|
|
|
|
for x in [0., 1.]:
|
|
ans = jax.grad(f)(x)
|
|
expected = jax.grad(f_ref)(x)
|
|
self.assertAllClose(ans, expected, check_dtypes=False)
|
|
|
|
@parameterized.named_parameters(
|
|
{"testcase_name": f"_{name}", "cond": cond}
|
|
for cond, name in COND_IMPLS)
|
|
def testCondGrad2(self, cond):
|
|
def f_ref(x):
|
|
z = jnp.array([1., 2.], x.dtype) * x if x[0] < 2 else jnp.sin(x)
|
|
return z.sum()
|
|
|
|
def _f(x):
|
|
return cond(
|
|
x[0] < 2,
|
|
lambda x: jnp.array([1., 2.], x.dtype) * x,
|
|
lambda x: jnp.sin(x),
|
|
x)
|
|
|
|
f = lambda x: jax.jit(_f)(x).sum()
|
|
|
|
x = 2.14 * jnp.ones(2)
|
|
ans = jax.grad(f)(x)
|
|
expected = jax.grad(f_ref)(x)
|
|
self.assertAllClose(ans, expected, check_dtypes=False)
|
|
jtu.check_grads(f, (x,), order=2, modes=["fwd", "rev"])
|
|
|
|
x = 1.72 * jnp.ones(2)
|
|
ans = jax.grad(f)(x)
|
|
expected = jax.grad(f_ref)(x)
|
|
self.assertAllClose(ans, expected, check_dtypes=False)
|
|
jtu.check_grads(f, (x,), order=2, modes=["fwd", "rev"],
|
|
rtol={jnp.float32: 1e-2, jnp.float64: 2e-3})
|
|
|
|
@parameterized.named_parameters(
|
|
{"testcase_name": f"_{name}", "cond": cond}
|
|
for cond, name in COND_IMPLS)
|
|
def testCondGrad3(self, cond):
|
|
def fun_ref(x):
|
|
if x < 3:
|
|
return 2.
|
|
else:
|
|
return 2. * x
|
|
|
|
def fun(x):
|
|
return cond(x < 3, None, lambda _: 2., x, lambda x: 2. * x)
|
|
|
|
x = 3.14
|
|
ans = jax.grad(fun)(x)
|
|
expected = jax.grad(fun_ref)(x)
|
|
self.assertAllClose(ans, expected, check_dtypes=False)
|
|
jtu.check_grads(fun, (x,), order=2, modes=["fwd", "rev"])
|
|
|
|
x = 2.72
|
|
ans = jax.grad(fun)(x)
|
|
expected = jax.grad(fun_ref)(x)
|
|
self.assertAllClose(ans, expected, check_dtypes=False)
|
|
jtu.check_grads(fun, (x,), order=2, modes=["fwd", "rev"])
|
|
|
|
@parameterized.named_parameters(
|
|
{"testcase_name": f"_{name}", "cond": cond}
|
|
for cond, name in COND_IMPLS)
|
|
def testCondGrad4(self, cond):
|
|
if cond is cond_with_new_checkpoint and jtu.test_device_matches(['tpu']):
|
|
raise unittest.SkipTest("tpu bug") # TODO(parkers): tpu bug exhibited here
|
|
def fun_ref(x, y):
|
|
if x < 3:
|
|
return 2. * jnp.sin(y)
|
|
else:
|
|
return 2. * jnp.cos(x)
|
|
|
|
@jax.jit
|
|
def fun(x, y):
|
|
return cond(
|
|
x < 3,
|
|
None, lambda _: 2. * jnp.sin(y),
|
|
x, lambda x: 2. * x)
|
|
|
|
y = 5.8
|
|
x = 3.14
|
|
ans = jax.grad(fun, 1)(x, y)
|
|
expected = jax.grad(fun_ref, 1)(x, y)
|
|
self.assertAllClose(ans, expected, check_dtypes=False)
|
|
jtu.check_grads(fun, (x, y), order=2, modes=["fwd", "rev"])
|
|
|
|
x = 2.72
|
|
ans = jax.grad(fun, 1)(x, y)
|
|
expected = jax.grad(fun_ref, 1)(x, y)
|
|
self.assertAllClose(ans, expected, check_dtypes=False)
|
|
jtu.check_grads(fun, (x, y), order=2, modes=["fwd", "rev"])
|
|
|
|
def testCondLinearize(self):
|
|
def f(x):
|
|
return lax.cond(x < 2, lambda x: 3. * x, lambda x: jnp.sin(x), x)
|
|
y, f_lin = jax.linearize(f, 1.)
|
|
self.assertAllClose(y, 3., check_dtypes=False)
|
|
self.assertAllClose(f_lin(2.), 6., check_dtypes=False)
|
|
y, f_lin = jax.linearize(f, 4.)
|
|
self.assertAllClose(y, jnp.sin(4.), check_dtypes=False)
|
|
self.assertAllClose(f_lin(2.), jnp.cos(4.) * 2., check_dtypes=False)
|
|
|
|
def testSwitchLinearize(self):
|
|
branches = [lambda x: 3. * x,
|
|
lambda x: jnp.sin(x),
|
|
lambda x: -x]
|
|
def f(x):
|
|
idx = lax.convert_element_type(x // 1, np.int32)
|
|
return lax.switch(idx, branches, x)
|
|
|
|
# branch 0
|
|
y, f_lin = jax.linearize(f, -1.)
|
|
self.assertAllClose(y, -3., check_dtypes=False)
|
|
self.assertAllClose(f_lin(2.), 6., check_dtypes=False)
|
|
y, f_lin = jax.linearize(f, 0.)
|
|
self.assertAllClose(y, 0., check_dtypes=False)
|
|
self.assertAllClose(f_lin(2.), 6., check_dtypes=False)
|
|
|
|
# branch 1
|
|
y, f_lin = jax.linearize(f, 1.)
|
|
self.assertAllClose(y, jnp.sin(1.), check_dtypes=False)
|
|
self.assertAllClose(f_lin(2.), jnp.cos(1.) * 2., check_dtypes=False)
|
|
|
|
# branch 2
|
|
y, f_lin = jax.linearize(f, 2.)
|
|
self.assertAllClose(y, -2., check_dtypes=False)
|
|
self.assertAllClose(f_lin(2.), -2., check_dtypes=False)
|
|
y, f_lin = jax.linearize(f, 3.)
|
|
self.assertAllClose(y, -3., check_dtypes=False)
|
|
self.assertAllClose(f_lin(2.), -2., check_dtypes=False)
|
|
|
|
@parameterized.named_parameters(
|
|
{"testcase_name": f"_{name}", "cond": cond}
|
|
for cond, name in COND_IMPLS)
|
|
def testCondLinearize2(self, cond):
|
|
def f_ref(x):
|
|
z = jnp.array([1., 2.], x.dtype) * x if x[0] < 2 else jnp.cos(jnp.sin(x))
|
|
return z.sum()
|
|
|
|
def f(x):
|
|
return cond(
|
|
x[0] < 2,
|
|
lambda x: jnp.array([1., 2.], x.dtype) * x,
|
|
lambda x: jnp.cos(jnp.sin(x)),
|
|
x).sum()
|
|
|
|
x = 2.14 * jnp.ones(2)
|
|
y, f_lin = jax.linearize(f, x)
|
|
y_ref, f_lin_ref = jax.linearize(f_ref, x)
|
|
self.assertAllClose(y, y_ref, check_dtypes=False)
|
|
self.assertAllClose(f_lin(x), f_lin_ref(x), check_dtypes=False)
|
|
|
|
x = -2.14 * jnp.ones(2)
|
|
y, f_lin = jax.linearize(f, x)
|
|
y_ref, f_lin_ref = jax.linearize(f_ref, x)
|
|
self.assertAllClose(y, y_ref, check_dtypes=False)
|
|
self.assertAllClose(f_lin(x), f_lin_ref(x), check_dtypes=False)
|
|
|
|
f = jax.jit(f)
|
|
x = 2.14 * jnp.ones(2)
|
|
y, f_lin = jax.linearize(f, x)
|
|
y_ref, f_lin_ref = jax.linearize(f_ref, x)
|
|
self.assertAllClose(y, y_ref, check_dtypes=False)
|
|
self.assertAllClose(f_lin(x), f_lin_ref(x), check_dtypes=False)
|
|
|
|
def testCondJit(self):
|
|
def f(x):
|
|
return lax.cond(x < 2, lambda x: 3. * x, lambda x: jnp.sin(x), x)
|
|
y = jax.jit(f)(1.)
|
|
expected = f(1.)
|
|
self.assertAllClose(y, expected, check_dtypes=False)
|
|
y = jax.jit(f)(4.)
|
|
expected = f(4.)
|
|
self.assertAllClose(y, expected, check_dtypes=False)
|
|
|
|
def testSwitchJit(self):
|
|
branches = [lambda x: 3. * x,
|
|
lambda x: jnp.sin(x),
|
|
lambda x: -x]
|
|
def f(x):
|
|
idx = lax.convert_element_type(x // 1, np.int32)
|
|
return lax.switch(idx, branches, x)
|
|
for x in [-1., 0., 1., 2., 3.]:
|
|
y = jax.jit(f)(x)
|
|
expected = f(x)
|
|
self.assertAllClose(y, expected, check_dtypes=False)
|
|
|
|
@parameterized.named_parameters(
|
|
{"testcase_name": f"_{name}", "cond": cond}
|
|
for cond, name in COND_IMPLS)
|
|
def testCondJitDisabled(self, cond):
|
|
def f_ref(x):
|
|
return 3. * x if x < 2 else jnp.sin(x)
|
|
def f(x):
|
|
return cond(x < 2, lambda x: 3. * x, lambda x: jnp.sin(x), x)
|
|
|
|
with jax.disable_jit():
|
|
y = f(1.)
|
|
expected = f_ref(1.)
|
|
self.assertAllClose(y, expected, check_dtypes=False)
|
|
|
|
with jax.disable_jit():
|
|
y = jax.jit(f)(1.)
|
|
expected = f(1.)
|
|
self.assertAllClose(y, expected, check_dtypes=False)
|
|
|
|
@parameterized.named_parameters(
|
|
{"testcase_name": f"_{name}", "cond": cond}
|
|
for cond, name in COND_IMPLS)
|
|
def testCondWithConsts(self, cond):
|
|
def f(x):
|
|
return cond(x < 2,
|
|
lambda x: np.array([1., 2.]) * x,
|
|
lambda x: np.array([3., 4.]) * jnp.sin(x),
|
|
x)
|
|
|
|
def f_ref(x):
|
|
if x < 2:
|
|
return np.array([1., 2.]) * x
|
|
else:
|
|
return np.array([3., 4.]) * np.sin(x)
|
|
|
|
y = f(1.)
|
|
expected = f_ref(1.)
|
|
self.assertAllClose(y, expected, check_dtypes=False)
|
|
y = f(4.)
|
|
expected = f_ref(4.)
|
|
self.assertAllClose(y, expected, check_dtypes=False)
|
|
|
|
@parameterized.named_parameters(
|
|
{"testcase_name": f"_{name}", "cond": cond}
|
|
for cond, name in COND_IMPLS)
|
|
def testCondJitWithConsts(self, cond):
|
|
def f(x):
|
|
return cond(x < 2,
|
|
lambda x: np.array([1., 2.]) * x,
|
|
lambda x: np.array([3., 4.]) * jnp.sin(x),
|
|
x)
|
|
|
|
y = jax.jit(f)(1.)
|
|
expected = f(1.)
|
|
self.assertAllClose(y, expected, check_dtypes=False)
|
|
y = jax.jit(f)(4.)
|
|
expected = f(4.)
|
|
self.assertAllClose(y, expected, check_dtypes=False)
|
|
|
|
@parameterized.named_parameters(
|
|
{"testcase_name": f"_{name}", "cond": cond}
|
|
for cond, name in COND_IMPLS)
|
|
def testCondVmapGrad(self, cond):
|
|
# https://github.com/google/jax/issues/2264
|
|
def f_1(x): return x ** 2
|
|
def f_2(x): return x ** 3
|
|
|
|
def f(x): return cond(x > 0, f_1, f_2, x)
|
|
def g(x): return jnp.where(x > 0, f_1(x), f_2(x))
|
|
|
|
x = jnp.linspace(-1, 1, 20)
|
|
ans = jax.vmap(jax.grad(f))(x)
|
|
expected = jax.vmap(jax.grad(g))(x)
|
|
self.assertAllClose(ans, expected, check_dtypes=False)
|
|
|
|
@jax.legacy_prng_key('allow')
|
|
def testIssue1263(self):
|
|
def f(rng, x):
|
|
cond = random.bernoulli(rng)
|
|
return lax.cond(cond, x, lambda x: x, jnp.abs(x) - 1., lambda x: x)
|
|
|
|
def body_fn(i, state):
|
|
rng, x = state
|
|
key, subkey = random.split(rng)
|
|
return key, f(subkey, x)
|
|
|
|
def g(rng, x):
|
|
return lax.fori_loop(0, 10, body_fn, (rng, x))
|
|
|
|
jax.vmap(g)(random.split(random.PRNGKey(0), 3), jnp.ones((3, 4)))
|
|
|
|
def testIssue514(self):
|
|
# just check this doesn't crash
|
|
lax.cond(True,
|
|
(0, 0), lambda x: (x[0], 0),
|
|
(1, 1), lambda x: x)
|
|
|
|
def testIssue649(self):
|
|
from jax import lax
|
|
|
|
def body(x):
|
|
a, b = x
|
|
return (7, b + 1)
|
|
|
|
def cond(x):
|
|
a, b = x
|
|
return b < 10
|
|
|
|
out = lax.while_loop(cond, body, (33, 4))
|
|
self.assertEqual(out, (7, 10))
|
|
|
|
@parameterized.named_parameters(
|
|
{"testcase_name": f"_{jit_scan=}_{jit_f=}_impl={scan_name}",
|
|
"jit_scan": jit_scan, "jit_f": jit_f, "scan": scan_impl,
|
|
"impl_name": scan_name}
|
|
for jit_scan in [False, True]
|
|
for jit_f in [False, True]
|
|
for scan_impl, scan_name in SCAN_IMPLS_WITH_FOR)
|
|
def testScanImpl(self, jit_scan, jit_f, scan, impl_name):
|
|
rng = self.rng()
|
|
|
|
d = rng.randn(2)
|
|
def f(c, a):
|
|
assert a.shape == (3,)
|
|
assert c.shape == (4,)
|
|
b = jnp.cos(jnp.sum(jnp.sin(a)) + jnp.sum(jnp.cos(c)) + jnp.sum(d))
|
|
c = jnp.sin(c * b)
|
|
assert b.shape == ()
|
|
return c, b
|
|
|
|
if jit_f:
|
|
f = jax.jit(f)
|
|
if jit_scan:
|
|
scan = jax.jit(scan, static_argnums=(0,))
|
|
|
|
as_ = rng.randn(5, 3)
|
|
c = rng.randn(4)
|
|
|
|
ans = scan(f, c, as_)
|
|
expected = scan_reference(f, c, as_)
|
|
rtol = {np.float64: 1.4e-15}
|
|
atol = {np.float64: 8e-15}
|
|
if impl_name == "for":
|
|
rtol[np.float32] = 8e-5
|
|
atol[np.float32] = 3e-5
|
|
self.assertAllClose(
|
|
ans,
|
|
expected,
|
|
check_dtypes=False,
|
|
rtol=rtol,
|
|
atol=atol)
|
|
|
|
@parameterized.named_parameters(
|
|
{"testcase_name": f"_{jit_scan=}_{jit_f=}_impl={scan_name}",
|
|
"jit_scan": jit_scan, "jit_f": jit_f, "scan": scan_impl}
|
|
for jit_scan in [False, True]
|
|
for jit_f in [False, True]
|
|
for scan_impl, scan_name in SCAN_IMPLS_WITH_FOR)
|
|
def testScanJVP(self, jit_scan, jit_f, scan):
|
|
rng = self.rng()
|
|
|
|
d = rng.randn(2)
|
|
def f(c, a):
|
|
assert a.shape == (3,)
|
|
assert c.shape == (4,)
|
|
b = jnp.cos(jnp.sum(jnp.sin(a)) + jnp.sum(jnp.cos(c)) + jnp.sum(d))
|
|
c = jnp.sin(c * b)
|
|
assert b.shape == ()
|
|
return c, b
|
|
|
|
if jit_f:
|
|
f = jax.jit(f)
|
|
if jit_scan:
|
|
scan = jax.jit(scan, static_argnums=(0,))
|
|
|
|
as_ = rng.randn(5, 3)
|
|
c = rng.randn(4)
|
|
|
|
ans = jax.jvp( lambda c, as_: scan(f, c, as_), (c, as_), (c, as_))
|
|
expected = jax.jvp(lambda c, as_: scan_reference(f, c, as_), (c, as_), (c, as_))
|
|
tol = {np.float64: 1e-12, np.float32: 1e-4}
|
|
self.assertAllClose(ans, expected, check_dtypes=False, rtol=tol, atol=tol)
|
|
|
|
jtu.check_grads(partial(scan, f), (c, as_), order=2, modes=["fwd"])
|
|
|
|
@parameterized.named_parameters(
|
|
{"testcase_name": f"_{jit_scan=}_{jit_f=}_impl={scan_name}",
|
|
"jit_scan": jit_scan, "jit_f": jit_f, "scan": scan_impl}
|
|
for jit_scan in [False, True]
|
|
for jit_f in [False, True]
|
|
for scan_impl, scan_name in SCAN_IMPLS_WITH_FOR)
|
|
def testScanLinearize(self, jit_scan, jit_f, scan):
|
|
rng = self.rng()
|
|
|
|
d = rng.randn(2)
|
|
def f(c, a):
|
|
assert a.shape == (3,)
|
|
assert c.shape == (4,)
|
|
b = jnp.cos(jnp.sum(jnp.sin(a)) + jnp.sum(jnp.cos(c)) + jnp.sum(d))
|
|
c = jnp.sin(c * b)
|
|
assert b.shape == ()
|
|
return c, b
|
|
|
|
if jit_f:
|
|
f = jax.jit(f)
|
|
if jit_scan:
|
|
scan = jax.jit(scan, static_argnums=(0,))
|
|
|
|
as_ = rng.randn(5, 3)
|
|
c = rng.randn(4)
|
|
|
|
if scan is scan_with_new_checkpoint2:
|
|
rtol = {np.float64: 1e-12, np.float32: 1e-4}
|
|
elif scan is scan_with_for:
|
|
rtol = {np.float64: 1e-12, np.float32: 1e-4}
|
|
else:
|
|
rtol = {np.float64: 1e-14, np.float32: 1e-4}
|
|
|
|
ans = jax.linearize(lambda c, as_: scan(f, c, as_), c, as_)[1](c, as_)
|
|
expected = jax.linearize(lambda c, as_: scan_reference(f, c, as_), c, as_)[1](c, as_)
|
|
self.assertAllClose(ans, expected, check_dtypes=False, rtol=rtol)
|
|
|
|
@parameterized.named_parameters(
|
|
{"testcase_name": f"_{jit_scan=}_{jit_f=}_impl={scan_name}",
|
|
"jit_scan": jit_scan, "jit_f": jit_f, "scan": scan_impl}
|
|
for jit_scan in [False, True]
|
|
for jit_f in [False, True]
|
|
for scan_impl, scan_name in SCAN_IMPLS_WITH_FOR)
|
|
@jtu.skip_on_flag("jax_skip_slow_tests", True)
|
|
def testScanGrad(self, jit_scan, jit_f, scan):
|
|
rng = self.rng()
|
|
|
|
d = rng.randn(2)
|
|
def f(c, a):
|
|
assert a.shape == (3,)
|
|
assert c.shape == (4,)
|
|
b = jnp.sum(jnp.sin(a)) + jnp.sum(jnp.sin(c)) + jnp.sum(jnp.sin(d))
|
|
c = jnp.sin(c * b)
|
|
assert b.shape == ()
|
|
return c, b
|
|
|
|
if scan is scan_with_new_checkpoint:
|
|
rtol = {np.float32: 5e-5, np.float64: 1e-13}
|
|
atol = 1e-5
|
|
elif scan is scan_with_for:
|
|
rtol = {np.float32: 2e-5, np.float64: 1e-13}
|
|
atol = {np.float32: 6e-2, np.float64: 1e-13}
|
|
else:
|
|
rtol = {np.float32: 2e-4, np.float64: 1e-13}
|
|
atol = {np.float32: 8e-5, np.float64: 1e-13}
|
|
|
|
if jit_f:
|
|
f = jax.jit(f)
|
|
if jit_scan:
|
|
scan = jax.jit(scan, static_argnums=(0,))
|
|
|
|
as_ = rng.randn(5, 3)
|
|
c = rng.randn(4)
|
|
|
|
ans = jax.grad(lambda c, as_: list( scan(f, c, as_))[0].sum())(c, as_)
|
|
expected = jax.grad(lambda c, as_: list(scan_reference(f, c, as_))[0].sum())(c, as_)
|
|
self.assertAllClose(ans, expected, check_dtypes=False, rtol=rtol, atol=atol)
|
|
|
|
rtol = 5e-3 if scan is not scan_with_new_checkpoint2 else 5e-2
|
|
atol = 5e-2 if jtu.test_device_matches(["tpu"]) else 1e-3
|
|
jtu.check_grads(partial(scan, f), (c, as_), order=2, modes=["rev"],
|
|
atol=atol, rtol=rtol)
|
|
|
|
@jtu.skip_on_devices("tpu") # TPU lacks precision for this test.
|
|
@jtu.skip_on_flag("jax_skip_slow_tests", True)
|
|
def testScanRnn(self):
|
|
r = self.rng()
|
|
|
|
n_in = 4
|
|
n_hid = 2
|
|
n_out = 1
|
|
length = 3
|
|
|
|
W_trans = r.randn(n_hid, n_hid + n_in).astype(jnp.float_)
|
|
W_out = r.randn(n_out, n_hid + n_in).astype(jnp.float_)
|
|
params = W_trans, W_out
|
|
|
|
inputs = r.randn(length, n_in).astype(jnp.float_)
|
|
targets = r.randn(length, n_out).astype(jnp.float_)
|
|
|
|
def step(params, state, input):
|
|
W_trans, W_out = params
|
|
stacked = jnp.concatenate([state, input])
|
|
output = jnp.tanh(jnp.dot(W_out, stacked))
|
|
next_state = jnp.tanh(jnp.dot(W_trans, stacked))
|
|
return next_state, output
|
|
|
|
def rnn(params, inputs):
|
|
init_state = jnp.zeros(n_hid)
|
|
_, outputs = lax.scan(partial(step, params), init_state, inputs)
|
|
return outputs
|
|
|
|
@jax.jit
|
|
def loss(params, inputs, targets):
|
|
predictions = rnn(params, inputs)
|
|
return jnp.sum((predictions - targets)**2)
|
|
|
|
# evaluation doesn't crash
|
|
loss(params, inputs, targets)
|
|
|
|
# jvp evaluation doesn't crash
|
|
jax.jvp(lambda params: loss(params, inputs, targets), (params,), (params,))
|
|
|
|
# jvp numerical check passes
|
|
jtu.check_grads(loss, (params, inputs, targets), order=2, modes=["fwd"],
|
|
rtol={np.float32: 2e-2, np.float64: 1e-6})
|
|
|
|
# linearize works
|
|
_, expected = jax.jvp(loss, (params, inputs, targets),
|
|
(params, inputs, targets))
|
|
_, linfun = jax.linearize(loss, params, inputs, targets)
|
|
ans = linfun(params, inputs, targets)
|
|
self.assertAllClose(ans, expected, check_dtypes=False)
|
|
|
|
# gradient evaluation doesn't crash
|
|
jax.grad(loss)(params, inputs, targets)
|
|
|
|
# gradient check passes
|
|
jtu.check_grads(loss, (params, inputs, targets), order=2, rtol=2e-2)
|
|
|
|
# we can vmap to batch things
|
|
batch_size = 7
|
|
batched_inputs = r.randn(batch_size, length, n_in).astype(jnp.float_)
|
|
batched_targets = r.randn(batch_size, length, n_out).astype(jnp.float_)
|
|
batched_loss = jax.vmap(lambda x, y: loss(params, x, y))
|
|
losses = batched_loss(batched_inputs, batched_targets)
|
|
expected = np.stack(list(map(lambda x, y: loss(params, x, y),
|
|
batched_inputs, batched_targets)))
|
|
self.assertAllClose(losses, expected, check_dtypes=False, rtol=1e-2)
|
|
|
|
@parameterized.named_parameters(
|
|
{"testcase_name": f"_impl={scan_name}", "scan": scan_impl}
|
|
for scan_impl, scan_name in SCAN_IMPLS_WITH_FOR)
|
|
def testIssue711(self, scan):
|
|
# Tests reverse-mode differentiation through a scan for which the scanned
|
|
# function also involves reverse-mode differentiation.
|
|
# See https://github.com/google/jax/issues/711
|
|
def harmonic_bond(conf, params):
|
|
return jnp.sum(conf * params)
|
|
|
|
def minimize_structure(test_params):
|
|
energy_fn = partial(harmonic_bond, params=test_params)
|
|
|
|
def apply_carry(carry, _):
|
|
i, x = carry
|
|
new_x = x - 0.1 * jax.grad(energy_fn)(x)
|
|
new_carry = (i+1, new_x)
|
|
return new_carry, _
|
|
|
|
x0 = jnp.array([1., 2., 3.])
|
|
carry_final, _ = scan(apply_carry, (0, x0), jnp.zeros((75, 0)))
|
|
_, x_final = carry_final
|
|
return x_final
|
|
|
|
initial_params = 0.5
|
|
minimize_structure(initial_params) # doesn't crash
|
|
|
|
def loss(test_params):
|
|
x_final = minimize_structure(test_params)
|
|
return jnp.sum(jnp.sin(1.0 - x_final))
|
|
|
|
jax.grad(loss)(0.25) # doesn't crash
|
|
|
|
def testIssue744(self):
|
|
Point = collections.namedtuple('Point', ['x', 'y'])
|
|
p0 = Point(x=jnp.array(1), y=jnp.array(2))
|
|
|
|
def plus_one(p, iter_idx):
|
|
return Point(p.x+1, p.y+1), iter_idx
|
|
|
|
self.assertRaisesRegex(
|
|
ValueError,
|
|
'scan got value with no leading axis to scan over.*',
|
|
lambda: lax.scan(plus_one, p0, list(range(5))))
|
|
|
|
def testScanBodyOutputError(self):
|
|
with self.assertRaisesRegex(
|
|
TypeError,
|
|
re.escape("scan body output must be a pair, got ShapedArray(float32[]).")):
|
|
lax.scan(lambda c, x: np.float32(0.), 0, jnp.arange(5.))
|
|
|
|
def testScanBodyCarryPytreeMismatchErrors(self):
|
|
with self.assertRaisesRegex(
|
|
TypeError,
|
|
re.escape("Scanned function carry input and carry output must have "
|
|
"the same pytree structure, but they differ:\n"
|
|
" * the input carry c is a tuple of length 2")):
|
|
lax.scan(lambda c, x: ((0, 0, 0), x), (1, (2, 3)), jnp.arange(5.))
|
|
|
|
with self.assertRaisesRegex(
|
|
TypeError,
|
|
re.escape("Scanned function carry input and carry output must have the "
|
|
"same pytree structure, but they differ:\n"
|
|
" * the input carry x is a tuple of length 2")):
|
|
lax.scan(lambda x, _: ((x[0].astype('float32'),), None),
|
|
(jnp.array(0, 'int32'),) * 2, None, length=1)
|
|
|
|
with self.assertRaisesRegex(
|
|
TypeError,
|
|
re.escape("Scanned function carry input and carry output must have the "
|
|
"same pytree structure, but they differ:\n"
|
|
" * the input carry x is a <class 'tuple'> but the corres")):
|
|
jax.lax.scan(lambda x, _: ([x[0].astype('float32'),] * 2, None),
|
|
(jnp.array(0, 'int32'),) * 2, None, length=1)
|
|
|
|
with self.assertRaisesRegex(
|
|
TypeError,
|
|
re.escape("Scanned function carry input and carry output must have the "
|
|
"same pytree structure, but they differ:\n"
|
|
" * the input carry x is a <class 'dict'> with 1 child but")):
|
|
jax.lax.scan(lambda x, _: ({'a': x['a'], 'b': x['a']}, None),
|
|
{'a': jnp.array(0, 'int32')}, None, length=1)
|
|
|
|
with self.assertRaisesRegex(
|
|
TypeError,
|
|
re.escape("Scanned function carry input and carry output must have the "
|
|
"same pytree structure, but they differ:\n"
|
|
" * the input carry component x[0] is a <class 'dict'> with "
|
|
"1 child but the corresponding component of the carry "
|
|
"output is a <class 'dict'> with 2 children")):
|
|
jax.lax.scan(lambda x, _: (({'a': x[0]['a'], 'b': x[0]['a']},) * 2, None),
|
|
({'a': jnp.array(0, 'int32')},) * 2, None, length=1)
|
|
|
|
def testScanBodyCarryTypeMismatchErrors(self):
|
|
with self.assertRaisesRegex(
|
|
TypeError,
|
|
re.escape("Scanned function carry input and carry output must have equal "
|
|
"types (e.g. shapes and dtypes of arrays), but they differ:\n"
|
|
" * the input carry x has type int32[] but the corresponding "
|
|
"output carry component has type float32[], so the dtypes do "
|
|
"not match"
|
|
)):
|
|
jax.lax.scan(lambda x, _: (x.astype('float32'), None),
|
|
jnp.array(0, 'int32'), None, length=1)
|
|
|
|
with self.assertRaisesRegex(
|
|
TypeError,
|
|
re.escape("Scanned function carry input and carry output must have equal "
|
|
"types (e.g. shapes and dtypes of arrays), but they differ:\n"
|
|
" * the input carry component x[1] has type int32[] but the "
|
|
"corresponding output carry component has type float32[], "
|
|
"so the dtypes do not match"
|
|
)):
|
|
jax.lax.scan(lambda x, _: ((x[0], x[1].astype('float32')), None),
|
|
(jnp.array(0, 'int32'),) * 2, None, length=1)
|
|
|
|
with self.assertRaisesRegex(
|
|
TypeError,
|
|
re.escape("Scanned function carry input and carry output must have equal "
|
|
"types (e.g. shapes and dtypes of arrays), but they differ:\n"
|
|
" * the input carry component x[0] has type int32[] but the "
|
|
"corresponding output carry component has type float32[], "
|
|
"so the dtypes do not match\n\n"
|
|
" * the input carry component x[1] has type int32[] but the "
|
|
"corresponding output carry component has type float32[1,1], "
|
|
"so the dtypes do not match and also the shapes do not match"
|
|
)):
|
|
jax.lax.scan(lambda x, _: ((x[0].astype('float32'),
|
|
x[1].astype('float32').reshape(1, 1),
|
|
x[2]), None),
|
|
(jnp.array(0, 'int32'),) * 3, None, length=1)
|
|
|
|
@parameterized.named_parameters(
|
|
{"testcase_name": f"_{scan_name}",
|
|
"scan": scan_impl}
|
|
for scan_impl, scan_name in SCAN_IMPLS_WITH_FOR)
|
|
def testScanHigherOrderDifferentiation(self, scan):
|
|
d = 0.75
|
|
def f(c, a):
|
|
b = jnp.sin(c * jnp.sum(jnp.cos(d * a)))
|
|
c = 0.9 * jnp.cos(d * jnp.sum(jnp.sin(c * a)))
|
|
return c, b
|
|
|
|
as_ = jnp.arange(6.).reshape((3, 2))
|
|
c = jnp.array(1, dtype=as_.dtype)
|
|
|
|
jtu.check_grads(lambda c, as_: scan(f, c, as_), (c, as_),
|
|
modes=["rev"], order=2, rtol={np.float32: 6e-3})
|
|
|
|
@parameterized.named_parameters(
|
|
{"testcase_name": f"_{jit_scan=}_{jit_f=}_{in_axes=}_impl={scan_name}",
|
|
"jit_scan": jit_scan, "jit_f": jit_f, "in_axes": in_axes,
|
|
"scan": scan_impl}
|
|
for jit_scan in [False, True]
|
|
for jit_f in [False, True]
|
|
for scan_impl, scan_name in SCAN_IMPLS_WITH_FOR
|
|
for in_axes in itertools.product([None, 0, 1], [None, 0, 1, 2])
|
|
if in_axes != (None, None))
|
|
def testScanVmap(self, jit_scan, jit_f, in_axes, scan):
|
|
rng = self.rng()
|
|
|
|
d = rng.randn(2)
|
|
def f(c, a):
|
|
assert a.shape == (3,)
|
|
assert c.shape == (4,)
|
|
b = jnp.cos(jnp.sum(jnp.sin(a)) + jnp.sum(jnp.cos(c)) + jnp.sum(d))
|
|
c = jnp.sin(c * b)
|
|
assert b.shape == ()
|
|
return c, b
|
|
|
|
if jit_f:
|
|
f = jax.jit(f)
|
|
if jit_scan:
|
|
scan = jax.jit(scan, static_argnums=(0,))
|
|
|
|
as_shape = [5, 3]
|
|
c_shape = [4]
|
|
|
|
c_bdim, as_bdim = in_axes
|
|
if c_bdim is not None:
|
|
c_shape.insert(c_bdim, 7)
|
|
if as_bdim is not None:
|
|
as_shape.insert(as_bdim, 7)
|
|
|
|
as_ = rng.randn(*as_shape)
|
|
c = rng.randn(*c_shape)
|
|
|
|
ans = jax.vmap(lambda c, as_: scan(f, c, as_), in_axes)(c, as_)
|
|
expected = jax.vmap(lambda c, as_: scan_reference(f, c, as_), in_axes)(c, as_)
|
|
self.assertAllClose(ans, expected, check_dtypes=False,
|
|
rtol=1e-5, atol=1e-5)
|
|
|
|
def testScanVmapTuples(self):
|
|
def f(c, a):
|
|
a1, a2 = a
|
|
c1, c2 = c
|
|
b = jnp.sum(jnp.cos(a1)) * jnp.sum(c2 * a2)
|
|
c = c1 * jnp.sin(jnp.sum(a1 * a2)), c2 * jnp.cos(jnp.sum(a1))
|
|
return c, b
|
|
|
|
in_axes = (0, (1, 2))
|
|
|
|
r = self.rng()
|
|
as_ = (r.randn(3, 7), r.randn(3, 4, 7))
|
|
c = (r.randn(7, 2), r.randn(7))
|
|
|
|
expected_c_out, expected_bs = [], []
|
|
for i in range(7):
|
|
c_out, bs = lax.scan(f, (c[0][i], c[1][i]), (as_[0][:,i], as_[1][:,:,i]))
|
|
expected_c_out.append(c_out)
|
|
expected_bs.append(bs)
|
|
expected_c_out_0, expected_c_out_1 = unzip2(expected_c_out)
|
|
expected_c_out = (jnp.stack(expected_c_out_0), jnp.stack(expected_c_out_1))
|
|
expected_bs = jnp.stack(expected_bs)
|
|
expected = expected_c_out, expected_bs
|
|
|
|
ans = jax.vmap(lambda c, as_: lax.scan(f, c, as_), in_axes)(c, as_)
|
|
self.assertAllClose(ans, expected, check_dtypes=False)
|
|
|
|
@parameterized.named_parameters(
|
|
{"testcase_name": f"_impl={scan_name}", "scan": scan_impl}
|
|
for scan_impl, scan_name in SCAN_IMPLS_WITH_FOR)
|
|
def testScanVmapFixpoint(self, scan):
|
|
def f(carry_init):
|
|
def scan_body(c, x):
|
|
# The carry is a 4-tuple, the last element starts batched,
|
|
# and the carry is shifted left at each iteration.
|
|
return ((c[1], c[2], c[3], 0.), None)
|
|
return scan(scan_body, (0., 1., 2., carry_init), jnp.zeros(2))
|
|
carry_init = jnp.array([3., 4., 5.])
|
|
carry_out, _ = jax.vmap(f)(carry_init)
|
|
self.assertAllClose(carry_out[3], jnp.array([0., 0., 0.]), check_dtypes=False)
|
|
self.assertAllClose(carry_out[2], jnp.array([0., 0., 0.]), check_dtypes = False)
|
|
# After two shifts, we get the carry_init
|
|
self.assertAllClose(carry_out[1], carry_init, check_dtypes=False)
|
|
self.assertAllClose(carry_out[0], jnp.array([2., 2., 2.]), check_dtypes = False)
|
|
|
|
def testIssue757(self):
|
|
# code from https://github.com/google/jax/issues/757
|
|
def fn(a):
|
|
return jnp.cos(a)
|
|
|
|
def loop(val):
|
|
iterations = 10
|
|
|
|
def apply_carry(x, i):
|
|
return jax.grad(fn, argnums=(0,))(x)[0], i
|
|
|
|
final_val, _ = lax.scan(apply_carry, val, jnp.arange(iterations))
|
|
return final_val
|
|
|
|
arg = 0.5
|
|
jax.jit(jax.jacfwd(loop, argnums=(0,)))(arg) # doesn't crash
|
|
|
|
def testIssue804(self):
|
|
num_devices = jax.device_count()
|
|
f = partial(lax.scan, lambda c, x: (c + lax.psum(x, "i") , c), 0.)
|
|
jax.pmap(f, axis_name="i")(jnp.ones((num_devices, 4))) # doesn't crash
|
|
|
|
def testMap(self):
|
|
f = lambda x: x ** 2
|
|
xs = jnp.arange(10)
|
|
expected = xs ** 2
|
|
actual = lax.map(f, xs)
|
|
self.assertAllClose(actual, expected)
|
|
|
|
def testMapEmpty(self):
|
|
# https://github.com/google/jax/issues/2412
|
|
ans = lax.map(lambda x: x * x, jnp.array([]))
|
|
expected = jnp.array([])
|
|
self.assertAllClose(ans, expected)
|
|
|
|
def testCaching(self):
|
|
def cond(x):
|
|
assert python_should_be_executing
|
|
return x < 5
|
|
|
|
def body(x):
|
|
assert python_should_be_executing
|
|
return x + 2
|
|
|
|
python_should_be_executing = True
|
|
lax.while_loop(cond, body, 0)
|
|
|
|
python_should_be_executing = False
|
|
lax.while_loop(cond, body, 0)
|
|
|
|
# This second caching test shows a different kind of caching that we haven't
|
|
# implemented (but could!), namely that Python functions that are distinct
|
|
# objects but are equivalent functions trigger cache hits. This kind of
|
|
# caching could be salient when using lambda functions with control flow:
|
|
#
|
|
# lax.while_loop(lambda x: x < 5, lambda x: x + 2, 0)
|
|
# lax.while_loop(lambda x: x < 5, lambda x: x + 2, 0)
|
|
#
|
|
# To get a cache hit on the second line we'd need to form a jaxpr and
|
|
# compare them for equality (including the literals on identity). We could
|
|
# implement that by adding a __hash__/__eq__ to core.Jaxpr and
|
|
# core.ClosedJaxpr (see #1221).
|
|
@unittest.skip("not implemented")
|
|
def testCaching2(self):
|
|
def cond(x):
|
|
assert python_should_be_executing
|
|
return x < 5
|
|
|
|
def body(x):
|
|
assert python_should_be_executing
|
|
return x + 2
|
|
|
|
python_should_be_executing = True
|
|
lax.while_loop(cond, body, 0)
|
|
|
|
def cond(x):
|
|
assert python_should_be_executing
|
|
return x < 5
|
|
|
|
def body(x):
|
|
assert python_should_be_executing
|
|
return x + 2
|
|
|
|
python_should_be_executing = False
|
|
lax.while_loop(cond, body, 0)
|
|
|
|
def test_caches_depend_on_axis_env(self):
|
|
# https://github.com/google/jax/issues/9187
|
|
scanned_f = lambda _, __: (lax.psum(1, 'i'), None)
|
|
f = lambda: lax.scan(scanned_f, 0, None, length=1)[0]
|
|
ans = jax.vmap(f, axis_name='i', axis_size=2, out_axes=None)()
|
|
self.assertEqual(ans, 2)
|
|
ans = jax.vmap(f, axis_name='i', axis_size=3, out_axes=None)()
|
|
self.assertEqual(ans, 3)
|
|
|
|
def testWhileCondConstant(self):
|
|
out = lax.while_loop(lambda _: False, lambda _: (), ()) # doesn't crash
|
|
self.assertEqual(out, ())
|
|
|
|
@parameterized.named_parameters(
|
|
{"testcase_name": f"_{jit_loop=}_{jit_body=}_{jit_cond=}",
|
|
"jit_loop": jit_loop, "jit_body": jit_body, "jit_cond": jit_cond}
|
|
for jit_loop in [False, True]
|
|
for jit_body in [False, True]
|
|
for jit_cond in [False, True])
|
|
def testWhileJVP(self, jit_loop=True, jit_body=False, jit_cond=True):
|
|
cond = lambda x: x[0, 2] <= 8
|
|
body = lambda x: x * x
|
|
|
|
if jit_cond:
|
|
cond = jax.jit(cond)
|
|
if jit_body:
|
|
body = jax.jit(body)
|
|
|
|
loop = partial(lax.while_loop, cond, body)
|
|
if jit_loop:
|
|
loop = jax.jit(loop)
|
|
|
|
loop_ref = partial(while_loop_reference, cond, body)
|
|
|
|
x = jnp.arange(9.).reshape((3, 3))
|
|
ans = jax.jvp(loop, (x,), (x,))
|
|
expected = jax.jvp(loop_ref, (x,), (x,))
|
|
self.assertAllClose(ans, expected, check_dtypes=False)
|
|
|
|
jtu.check_grads(loop, (x,), order=2, modes=["fwd"])
|
|
|
|
@parameterized.named_parameters(
|
|
{"testcase_name": f"_{jit_loop=}_{jit_body=}_{jit_cond=}_impl={while_name}",
|
|
"jit_loop": jit_loop, "jit_body": jit_body, "jit_cond": jit_cond,
|
|
"while_loop": while_impl}
|
|
for jit_loop in [False, True]
|
|
for jit_body in [False, True]
|
|
for jit_cond in [False, True]
|
|
for while_impl, while_name in WHILE_LOOP_IMPLS)
|
|
def testWhileLinearize(self, while_loop, jit_loop=True, jit_body=False,
|
|
jit_cond=True):
|
|
cond = lambda x: x[0, 2] <= 8
|
|
body = lambda x: x * x
|
|
|
|
if jit_cond:
|
|
cond = jax.jit(cond)
|
|
if jit_body:
|
|
body = jax.jit(body)
|
|
|
|
loop = partial(while_loop, cond, body)
|
|
if jit_loop:
|
|
loop = jax.jit(loop)
|
|
|
|
loop_ref = partial(while_loop_reference, cond, body)
|
|
|
|
x = jnp.arange(9.).reshape((3, 3))
|
|
y, f_lin = jax.linearize(loop, x)
|
|
ydot = f_lin(x)
|
|
y_expected, ydot_expected = jax.jvp(loop_ref, (x,), (x,))
|
|
self.assertAllClose(y, y_expected, check_dtypes=False)
|
|
self.assertAllClose(ydot, ydot_expected, check_dtypes=False)
|
|
|
|
def testWhileJVPViaForiLoop(self):
|
|
f = lambda x: lax.fori_loop(0, 3, lambda i, x: x * 2, x)
|
|
self.assertAllClose(f(2.), 16., check_dtypes=False)
|
|
self.assertAllClose(jax.jvp(f, (2.,), (1.,)), (16., 8.), check_dtypes=False)
|
|
jtu.check_grads(f, (2.,), order=2, modes=["fwd"])
|
|
|
|
f = lambda x: lax.fori_loop(0, 3, lambda i, x: x * (i + 1), x)
|
|
self.assertAllClose(f(2.), 12., check_dtypes=False)
|
|
self.assertAllClose(jax.jvp(f, (2.,), (1.,)), (12., 6.), check_dtypes=False)
|
|
jtu.check_grads(f, (2.,), order=2, modes=["fwd"])
|
|
|
|
def testWhileJVPWithGrowingNonzeroTangents(self):
|
|
rng = self.rng()
|
|
|
|
def cond(state):
|
|
i, x, y, z = state
|
|
return i < 2
|
|
|
|
def body(state):
|
|
i, x, y, z = state
|
|
y = x * x
|
|
z = y * y
|
|
return i + 1, x, y, z
|
|
|
|
y, z = rng.randn(2), rng.randn(2)
|
|
def loop(loop_impl, x):
|
|
return loop_impl(cond, body, (0, x, y, z))[1]
|
|
|
|
loop_lax = partial(loop, lax.while_loop)
|
|
loop_ref = partial(loop, while_loop_reference)
|
|
|
|
x = rng.randn(2)
|
|
ans = jax.jvp(loop_lax, (x,), (x,))
|
|
expected = jax.jvp(loop_ref, (x,), (x,))
|
|
self.assertAllClose(ans, expected, check_dtypes=False)
|
|
|
|
jtu.check_grads(loop_lax, (x,), order=2, modes=["fwd"])
|
|
|
|
def testStaticForiGrad(self):
|
|
func = lambda x: lax.fori_loop(x, x + 2., lambda i, c: c, x)
|
|
jax.grad(func)(1.) # doesn't crash
|
|
jax.linearize(func, 1.) # doesn't crash
|
|
|
|
@parameterized.named_parameters(
|
|
dict(testcase_name=f"_{loop=}", loop=loop)
|
|
for loop in ["while", "fori_inside_cond", "fori_inside_scan"])
|
|
def testWhileGradError(self, loop: str = "fori_inside_scan"):
|
|
# Raise error for vjp for loops
|
|
if loop == "while":
|
|
func = lambda x: lax.while_loop(lambda i: i < 5., lambda i: i + 1., x)
|
|
elif loop == "fori_inside_jit":
|
|
func = jax.jit(lambda x: lax.fori_loop(x, x + 2., lambda i, c: c, x))
|
|
elif loop == "fori_inside_cond":
|
|
func = lambda x: lax.cond(
|
|
True,
|
|
x, lambda x: lax.fori_loop(x, x + 2., lambda i, c: c, x),
|
|
1., lambda x: x)
|
|
elif loop == "fori_inside_scan":
|
|
func = lambda x: lax.scan(
|
|
lambda c, x: (lax.fori_loop(x, x + 2., lambda i, c1: c1 * c, x), None),
|
|
x, np.ones(2))[0]
|
|
else:
|
|
assert False
|
|
|
|
with self.assertRaisesRegex(ValueError, "Reverse-mode differentiation does not work for lax.while_loop"):
|
|
jax.grad(func)(1.)
|
|
|
|
jax.linearize(func, 1.) # Linearization works
|
|
|
|
@jax.legacy_prng_key('allow')
|
|
def testIssue1316(self):
|
|
def f(carry, _):
|
|
c, key = carry
|
|
key, _ = random.split(key)
|
|
return (c, key), ()
|
|
|
|
key = random.PRNGKey(0)
|
|
jax.grad(lambda c: lax.scan(f, (c, key), np.ones(3))[0][0])(0.) # doesn't crash
|
|
|
|
def testIssue1361(self):
|
|
@jax.jit
|
|
def jit_run_scan(x):
|
|
def fun(carry, _):
|
|
x, _ = carry
|
|
return (2 * x, 0.), None
|
|
(x, _), _ = lax.scan(fun, (x, 0.), jnp.arange(3))
|
|
return x
|
|
|
|
jax.grad(lambda x: jit_run_scan(x))(0.) # doesn't crash
|
|
|
|
def testIssue810(self):
|
|
def loss(A):
|
|
def step(x, i):
|
|
return jnp.matmul(A, x), None
|
|
init_x = jnp.zeros(A.shape[-1:])
|
|
last_x, _ = lax.scan(step, init_x, jnp.arange(10))
|
|
return jnp.sum(last_x)
|
|
|
|
A = jnp.zeros((3, 3))
|
|
# The second DUS was unnecessarily replicating A across time.
|
|
# We check XLA because _scan_impl is "underneath" the jaxpr language.
|
|
s = str(jax.xla_computation(jax.grad(loss))(A).as_hlo_text())
|
|
assert s.count("dynamic-update-slice(") < 2
|
|
|
|
def testScanLengthArg(self):
|
|
def arange(n):
|
|
return lax.scan(lambda c, _: (c + 1, c), 0, None, length=n)[1]
|
|
|
|
ans = arange(10)
|
|
expected = np.arange(10)
|
|
self.assertAllClose(ans, expected, check_dtypes=False)
|
|
|
|
@ignore_jit_of_pmap_warning()
|
|
def test_while_loop_of_pmap(self):
|
|
# Avoid accuracy issue caused by too many devices.
|
|
DEVICE_LIMITATION = 4
|
|
devices = jax.devices()
|
|
count = jax.device_count()
|
|
if jax.device_count() >= DEVICE_LIMITATION:
|
|
devices = devices[:DEVICE_LIMITATION]
|
|
count = DEVICE_LIMITATION
|
|
|
|
# code from jsnoek@
|
|
def body(i, x):
|
|
result = jax.pmap(lambda z: lax.psum(jnp.sin(z), 'i'), devices=devices, axis_name='i')(x)
|
|
return result + x
|
|
f_loop = lambda x: lax.fori_loop(0, 3, body, x) # noqa: F821
|
|
ans = f_loop(jnp.ones(count))
|
|
del body, f_loop
|
|
|
|
def body2(i, x):
|
|
result = jnp.broadcast_to(jnp.sin(x).sum(), x.shape)
|
|
return result + x
|
|
g_loop = lambda x: lax.fori_loop(0, 3, body2, x)
|
|
expected = g_loop(jnp.ones(count))
|
|
|
|
self.assertAllClose(ans, expected, check_dtypes=False)
|
|
|
|
@ignore_jit_of_pmap_warning()
|
|
def test_while_loop_of_pmap_error_message(self):
|
|
|
|
def body(i, x):
|
|
result = jax.pmap(lambda z: lax.psum(jnp.sin(z), 'i'), axis_name='i')(x)
|
|
return result + x
|
|
f_loop = lambda x: lax.fori_loop(0, 3, body, x)
|
|
|
|
too_big = 2 * jax.device_count()
|
|
|
|
self.assertRaisesRegex(
|
|
ValueError,
|
|
re.escape(
|
|
"compiling computation `scan` that requires {} "
|
|
"replicas, but only {} XLA devices are available."
|
|
.format(too_big, jax.device_count())),
|
|
lambda: f_loop(jnp.ones(too_big)))
|
|
|
|
@parameterized.named_parameters(
|
|
{"testcase_name": f"_{scan_name}",
|
|
"scan": scan_impl}
|
|
for scan_impl, scan_name in SCAN_IMPLS_WITH_FOR)
|
|
def test_scan_reverse(self, scan):
|
|
def cumsum(x, reverse):
|
|
return scan(lambda c, x: (c + x, c + x), 0, x, reverse=reverse)[1]
|
|
|
|
x = np.array([3, 1, 4, 1, 5, 9])
|
|
self.assertAllClose(np.cumsum(x), cumsum(x, False), check_dtypes=False)
|
|
self.assertAllClose(np.cumsum(x[::-1])[::-1], cumsum(x, True), check_dtypes=False)
|
|
|
|
with jax.disable_jit():
|
|
self.assertAllClose(np.cumsum(x), cumsum(x, False), check_dtypes=False)
|
|
with jax.disable_jit():
|
|
self.assertAllClose(np.cumsum(x[::-1])[::-1], cumsum(x, True), check_dtypes=False)
|
|
|
|
def test_scan_unroll(self):
|
|
d = jnp.ones(2)
|
|
def f(c, a):
|
|
assert a.shape == (3,)
|
|
assert c.shape == (4,)
|
|
b = jnp.cos(jnp.sum(jnp.sin(a)) + jnp.sum(jnp.cos(c)) + jnp.sum(d))
|
|
c = jnp.sin(c * b)
|
|
assert b.shape == ()
|
|
return c, b
|
|
|
|
xs = jnp.ones((5, 3))
|
|
c = jnp.ones(4)
|
|
|
|
scan = lambda c, xs: lax.scan(f, c, xs)
|
|
scan_unrolled = lambda c, xs: lax.scan(f, c, xs, unroll=2)
|
|
|
|
# jaxprs should be the same size
|
|
self.assertEqual(
|
|
len(str(jax.make_jaxpr(scan)(c, xs))),
|
|
len(str(jax.make_jaxpr(scan_unrolled)(c, xs))))
|
|
|
|
# but HLO should grow due to unrolling
|
|
self.assertLess(
|
|
len(str(jax.xla_computation(scan)(c, xs).as_hlo_text())),
|
|
len(str(jax.xla_computation(scan_unrolled)(c, xs).as_hlo_text())))
|
|
|
|
def test_disable_jit_cond_with_vmap(self):
|
|
# https://github.com/google/jax/issues/3093
|
|
def fn(t):
|
|
return lax.cond(t > 0, 0, lambda x: 0, 0, lambda x: 1)
|
|
fn = jax.vmap(fn)
|
|
|
|
with jax.disable_jit():
|
|
_ = fn(jnp.array([1])) # doesn't crash
|
|
|
|
def test_disable_jit_while_loop_with_vmap(self):
|
|
# https://github.com/google/jax/issues/2823
|
|
def trivial_while(y):
|
|
return lax.while_loop(lambda x: x < 10.0, lambda x: x + 1.0, y)
|
|
with jax.disable_jit():
|
|
jax.vmap(trivial_while)(jnp.array([3.0,4.0])) # doesn't crash
|
|
|
|
def test_vmaps_of_while_loop(self):
|
|
# https://github.com/google/jax/issues/3164
|
|
def f(x, n): return lax.fori_loop(0, n, lambda _, x: x + 1, x)
|
|
x, n = jnp.arange(3), jnp.arange(4)
|
|
jax.vmap(jax.vmap(f, (None, 0)), (0, None))(x, n) # doesn't crash
|
|
|
|
@parameterized.named_parameters(
|
|
{"testcase_name": f"_{shape}_{axis=}",
|
|
"shape": shape, "axis": axis}
|
|
for shape in [
|
|
[0], [1], [2], [3], [5], [10], [1000],
|
|
[2, 3], [7, 5], [5, 6, 7]
|
|
]
|
|
for axis in range(-len(shape), len(shape) - 1))
|
|
def testAssociativeScanUnstructured(self, shape, axis):
|
|
data = np.arange(np.prod(shape)).reshape(shape) + 7
|
|
expected = np.cumsum(data, axis=axis)
|
|
result = lax.associative_scan(operator.add, data, axis=axis)
|
|
self.assertAllClose(result, expected, check_dtypes=False)
|
|
|
|
def testAssociativeScanUnstructured1000Reverse(self):
|
|
data = np.arange(1000) + 32
|
|
expected = np.cumsum(data[::-1])[::-1]
|
|
result = lax.associative_scan(operator.add, data, reverse=True)
|
|
self.assertAllClose(result, expected, check_dtypes=False)
|
|
|
|
def testAssociativeScanStructured3(self):
|
|
pair = collections.namedtuple('pair', ('first', 'second'))
|
|
data = pair(first=np.array([0., 1., 2.]),
|
|
second=np.array([0., 10., 20.]))
|
|
|
|
def fn(a, b):
|
|
return pair(first=a.first + b.first,
|
|
second=a.second + b.second)
|
|
|
|
result = lax.associative_scan(fn, elems=data)
|
|
self.assertAllClose(result.first, np.array([0., 1., 3.]),
|
|
check_dtypes=False)
|
|
self.assertAllClose(result.second, np.array([0., 10., 30.]),
|
|
check_dtypes=False)
|
|
|
|
def testAssociativeScanOfBools(self):
|
|
x = jnp.array([False, True, True, True, False, True])
|
|
y = lax.associative_scan(lax.bitwise_xor, x)
|
|
self.assertArraysEqual(np.array([False, True, False, True, True, False]), y)
|
|
|
|
@parameterized.named_parameters({"testcase_name": f"_{shape}", "shape": shape}
|
|
for shape in [2, 43, 100])
|
|
def testAssociativeScanSolvingRegressionTest(self, shape):
|
|
# This test checks that the batching rule doesn't raise for a batch
|
|
# sensitive function (solve).
|
|
ms = np.repeat(np.eye(2).reshape(1, 2, 2), shape, axis=0)
|
|
vs = np.ones((shape, 2))
|
|
|
|
@jax.vmap
|
|
def fn(a, b):
|
|
m1, v1 = a
|
|
m2, v2 = b
|
|
return m1 + m2, jsp.linalg.solve(m1, v2) + jsp.linalg.solve(m2, v1)
|
|
|
|
_ = lax.associative_scan(fn, elems=(ms, vs))
|
|
|
|
def test_scan_typecheck_param(self):
|
|
d = jnp.ones(2)
|
|
def f(c, a):
|
|
b = jnp.cos(jnp.sum(a) + jnp.sum(c) + jnp.sum(d))
|
|
c = jnp.sin(c * b)
|
|
return c, b
|
|
|
|
xs = jnp.ones((5, 3))
|
|
c = jnp.ones(4)
|
|
scan_fun = lambda c, xs: lax.scan(f, c, xs)
|
|
|
|
def new_jaxpr():
|
|
jaxpr = jax.make_jaxpr(scan_fun)(c, xs).jaxpr
|
|
scan = next(eqn for eqn in jaxpr.eqns if eqn.primitive.name == 'scan')
|
|
return jaxpr, scan
|
|
|
|
jaxpr, eqn = new_jaxpr()
|
|
eqn.params['reverse'] = 4
|
|
self.assertRaisesRegex(
|
|
core.JaxprTypeError,
|
|
re.escape('invalid scan param reverse of type int, bool required: 4'),
|
|
lambda: core.check_jaxpr(jaxpr))
|
|
|
|
jaxpr, eqn = new_jaxpr()
|
|
eqn.params['num_consts'] = -3
|
|
self.assertRaisesRegex(
|
|
core.JaxprTypeError,
|
|
re.escape('invalid scan param num_consts of type int, '
|
|
'non-negative int required: -3'),
|
|
lambda: core.check_jaxpr(jaxpr))
|
|
|
|
def test_cond_typecheck_param(self):
|
|
def new_jaxpr():
|
|
jaxpr = jax.make_jaxpr(
|
|
lambda x: lax.switch(0, [jnp.sin, jnp.cos], x))(1.).jaxpr
|
|
cond = next(eqn for eqn in jaxpr.eqns if eqn.primitive.name == 'cond')
|
|
return jaxpr, cond
|
|
|
|
jaxpr, eqn = new_jaxpr()
|
|
eqn.params['branches'] = (4, 2)
|
|
self.assertRaisesRegex(
|
|
core.JaxprTypeError,
|
|
re.escape('invalid cond param branches of type tuple, '
|
|
'tuple of ClosedJaxpr required: (4, 2)'),
|
|
lambda: core.check_jaxpr(jaxpr))
|
|
|
|
jaxpr, eqn = new_jaxpr()
|
|
eqn.params['linear'] = (4, 2)
|
|
self.assertRaisesRegex(
|
|
core.JaxprTypeError,
|
|
re.escape('invalid cond param linear of type tuple, '
|
|
'tuple of bool required: (4, 2)'),
|
|
lambda: core.check_jaxpr(jaxpr))
|
|
|
|
jaxpr, eqn = new_jaxpr()
|
|
eqn.params['linear'] = 'multi\nline'
|
|
self.assertRaisesRegex(
|
|
core.JaxprTypeError,
|
|
r'invalid cond param linear of type str, '
|
|
r'tuple of bool required:\r?\nmulti\r?\nline',
|
|
lambda: core.check_jaxpr(jaxpr))
|
|
|
|
def test_cond_transformation_rule_with_consts(self):
|
|
# https://github.com/google/jax/pull/9731
|
|
|
|
@jax.custom_jvp
|
|
def f(x):
|
|
return x
|
|
|
|
@f.defjvp
|
|
def f_jvp(primals, tangents):
|
|
(x,), (xdot,) = primals, tangents
|
|
const = np.arange(3, dtype=x.dtype)
|
|
return x * const, xdot * const
|
|
|
|
g = lambda x: jax.lax.cond(True, f, lambda x: x, x)
|
|
x = np.arange(3, dtype='float32')
|
|
jax.jvp(g, (x,), (x,)) # doesn't crash
|
|
|
|
def test_cond_excessive_compilation(self):
|
|
# Regression test for https://github.com/google/jax/issues/14058
|
|
def f(x):
|
|
return x + 1
|
|
|
|
def g(x):
|
|
return x + 2
|
|
|
|
with jtu.count_jit_and_pmap_compiles() as count:
|
|
for x in range(10):
|
|
lax.cond(x, f, g, x)
|
|
# Should observe a maximum of 4 compiles: convert_element_type, f, g, cond
|
|
# In #14058, this was observed to be 31 compiles.
|
|
self.assertLess(count[0], 5)
|
|
|
|
@parameterized.named_parameters(
|
|
{"testcase_name": f"_dtype={dtype.__name__}", "dtype": dtype}
|
|
for dtype in jtu.dtypes.all_integer)
|
|
def test_scan_init_weak_type(self, dtype):
|
|
def func(carry, x):
|
|
return carry + x, x
|
|
init_weak = 0 # Python scalars are weakly-typed.
|
|
x = jnp.ones(5, dtype=dtype)
|
|
carry, result = lax.scan(func, init_weak, x)
|
|
self.assertEqual(carry, x.sum(dtype=carry.dtype))
|
|
self.assertArraysEqual(result, x)
|
|
|
|
@parameterized.named_parameters(
|
|
{"testcase_name": f"_dtype={dtype.__name__}", "dtype": dtype}
|
|
for dtype in jtu.dtypes.all_integer)
|
|
def test_while_loop_init_weak_type(self, dtype):
|
|
# This tests whether lax.while_loop can properly handle weakly-typed
|
|
# initial values.
|
|
def cond_fun(val):
|
|
return val < 2
|
|
def body_fun(val):
|
|
return val + increment
|
|
increment = jnp.array(1, dtype=dtype)
|
|
init_weak = 0 # Python scalars are weakly-typed.
|
|
result = lax.while_loop(cond_fun, body_fun, init_weak)
|
|
self.assertArraysEqual(result, jnp.full_like(increment, 2))
|
|
|
|
@parameterized.named_parameters(
|
|
{"testcase_name": f"{suffix}", "remat": remat}
|
|
for suffix, remat in [
|
|
('', None),
|
|
('new_remat', new_checkpoint),
|
|
])
|
|
def test_scan_vjp_forwards_extensive_residuals(self, remat):
|
|
# https://github.com/google/jax/issues/4510
|
|
def cumprod(x):
|
|
s = jnp.ones((2, 32), jnp.float32)
|
|
return lax.scan(lambda s, x: (x*s, s), s, x)
|
|
if remat is not None:
|
|
cumprod = remat(cumprod)
|
|
|
|
rng = self.rng()
|
|
x = jnp.asarray(rng.randn(32, 2, 32).astype('float32'))
|
|
_, vjp_fun = jax.vjp(cumprod, x)
|
|
|
|
# Need to spelunk into vjp_fun. This is fragile, and if it causes problems
|
|
# just skip this test and make an issue for mattjj.
|
|
*_, ext_res = vjp_fun.args[0].args[0]
|
|
self.assertIs(ext_res, x)
|
|
|
|
if remat is not None:
|
|
# TODO(mattjj): make the numpy.ndarray test pass w/ remat
|
|
raise unittest.SkipTest("new-remat-of-scan doesn't convert numpy.ndarray")
|
|
|
|
x = rng.randn(32, 2, 32).astype('float32') # numpy.ndarray, not Array
|
|
_, vjp_fun = jax.vjp(cumprod, x)
|
|
*_, ext_res = vjp_fun.args[0].args[0]
|
|
self.assertIsInstance(ext_res, jax.Array)
|
|
|
|
def test_scan_vmap_collectives(self):
|
|
def scan_f(state, x):
|
|
s = lax.psum(state, 'i') * x
|
|
return state, s
|
|
|
|
def scan(state, xs):
|
|
return lax.scan(scan_f, state, xs)
|
|
|
|
scan_v = jax.vmap(scan, in_axes=0, out_axes=0, axis_name='i')
|
|
self.assertAllClose(
|
|
scan_v(jnp.ones([1]), jnp.arange(5.).reshape((1, 5))),
|
|
(jnp.array([1.]), jnp.array([[0., 1., 2., 3., 4.]])), check_dtypes=False)
|
|
|
|
def test_xla_cpu_gpu_loop_cond_bug(self):
|
|
# https://github.com/google/jax/issues/5900
|
|
def deriv(f):
|
|
return lambda x, *args: jax.linearize(lambda x: f(x, *args), x)[1](1.0)
|
|
|
|
def _while_loop(cond_fun, body_fun, init_val, max_iter):
|
|
def _iter(val):
|
|
next_val = body_fun(val)
|
|
next_cond = True
|
|
return next_val, next_cond
|
|
|
|
def _fun(tup, _):
|
|
val, cond = tup
|
|
return jax.lax.cond(cond, _iter, lambda x: (x, False), val), _
|
|
|
|
init = (init_val, cond_fun(init_val))
|
|
return jax.lax.scan(_fun, init, None, length=max_iter)[0][0]
|
|
|
|
def my_pow(x, y):
|
|
def body_fun(val):
|
|
return val * x
|
|
def cond_fun(val):
|
|
return True
|
|
return _while_loop(cond_fun, body_fun, 1.0, y)
|
|
|
|
self.assertAllClose(deriv(my_pow)(3.0, 1), 1.0, check_dtypes=False)
|
|
|
|
def test_unexpected_tracer_error(self):
|
|
with self.assertRaisesRegex(UnexpectedTracerError, "for while_loop"):
|
|
lst = []
|
|
def side_effecting_body(val):
|
|
lst.append(val)
|
|
return val+1
|
|
lax.while_loop(lambda x: x < 2, side_effecting_body, 1)
|
|
lst[0] += 1
|
|
|
|
with self.assertRaisesRegex(UnexpectedTracerError, "for scan"):
|
|
lst = []
|
|
def side_effecting_scan(carry, val):
|
|
lst.append(val)
|
|
return carry, val+1
|
|
lax.scan(side_effecting_scan, None, jnp.ones((2, 2)))
|
|
lst[0] += 1
|
|
|
|
def test_while_loop_fixed_point_with_nested_named_axes(self):
|
|
def f(x):
|
|
z = x + lax.axis_index('a').astype(x.dtype)
|
|
y = x + lax.axis_index('b').astype(x.dtype)
|
|
def cond(carry):
|
|
i, x = carry
|
|
return x < 5
|
|
def body(carry):
|
|
i, x = carry
|
|
return i + 1, x + lax.psum(y, 'b')
|
|
return lax.while_loop(cond, body, (0, z))[1]
|
|
xmap(f, axis_sizes=dict(a=2, b=10), out_axes=(['a']), in_axes={})(1.)
|
|
|
|
def test_while_loop_fixed_point_with_batched_pred_and_consts(self):
|
|
def f(i, x):
|
|
def cond(carry):
|
|
i, x = carry
|
|
return i < 5
|
|
def body(carry):
|
|
i, z = carry
|
|
# Close over const with batch dim = 1
|
|
return i + 1, z + x
|
|
return lax.while_loop(cond, body, (i, jnp.ones(3)))[1]
|
|
jax.vmap(f, in_axes=(0, 1))(jnp.arange(4), jnp.ones((3, 4)))
|
|
|
|
def test_cond_ad_batched_unit(self):
|
|
# see issue #9985
|
|
def cond_id(x):
|
|
return lax.cond(x < 0., lambda x: x, lambda x: x, x)
|
|
jax.vmap(jax.jacrev(lambda x: cond_id(cond_id(x))))(jnp.ones(1))
|
|
|
|
@parameterized.named_parameters(
|
|
{"testcase_name": f"impl={scan_name}", "scan": scan_impl}
|
|
for scan_impl, scan_name in SCAN_IMPLS_WITH_FOR)
|
|
def test_scan_hoisting_consts(self, scan):
|
|
A = jnp.arange(4.).reshape(2, 2)
|
|
B = jnp.arange(4.).reshape(2, 2) + 1.
|
|
|
|
def f(x):
|
|
def body(c, _):
|
|
c1, c2, c3 = c
|
|
return (jnp.dot(A, c1), jnp.dot(B, c2), jnp.dot(jnp.sin(B), c3)), None
|
|
init_carry = (x * jnp.ones(2), x * jnp.ones(2), x * jnp.ones(2))
|
|
(c1, c2, c3), _ = scan(body, init_carry, None, length=3)
|
|
return jnp.sum(c1) + jnp.sum(c2) + jnp.sum(c3)
|
|
|
|
jax.grad(f)(1.) # doesn't crash
|
|
|
|
def test_custom_jvp_tangent_cond_transpose(self):
|
|
# https://github.com/google/jax/issues/14026
|
|
def mask_fun(arr, choice):
|
|
out = (1 - choice) * arr.sum() + choice * (1 - arr.sum())
|
|
return out
|
|
|
|
def switch_fun(arr, choice):
|
|
choice = jnp.floor(choice).astype(jnp.int32)
|
|
out = jax.lax.switch(choice, [lambda x: x.sum(), lambda x: 1 - x.sum()], arr)
|
|
return out
|
|
|
|
test_arr = jnp.arange(3.)
|
|
test_val = 0.
|
|
|
|
expected1 = jax.grad(mask_fun)(test_arr, test_val)
|
|
expected2 = jax.grad(switch_fun)(test_arr, test_val)
|
|
|
|
def good_switchfun_jvp(primals, tangents):
|
|
arr, choice = primals
|
|
arr_dot, choice_dot = tangents
|
|
return switch_fun(arr, choice), mask_fun(arr_dot, choice)
|
|
|
|
def bad_switchfun_jvp(primals, tangents):
|
|
arr, choice = primals
|
|
arr_dot, choice_dot = tangents
|
|
return switch_fun(arr, choice), switch_fun(arr_dot, choice)
|
|
|
|
good_custom_switchfun = jax.custom_jvp(switch_fun)
|
|
good_custom_switchfun.defjvp(good_switchfun_jvp)
|
|
expected3 = jax.grad(good_custom_switchfun)(test_arr, test_val)
|
|
|
|
bad_custom_switchfun = jax.custom_jvp(switch_fun)
|
|
bad_custom_switchfun.defjvp(bad_switchfun_jvp)
|
|
actual = jax.grad(bad_custom_switchfun)(test_arr, test_val)
|
|
|
|
self.assertAllClose(expected1, expected2)
|
|
self.assertAllClose(expected2, expected3)
|
|
self.assertAllClose(expected3, actual)
|
|
|
|
def test_platform_dependent(self):
|
|
def f(x):
|
|
return lax.platform_dependent(x, cpu=jnp.sin, default=jnp.cos)
|
|
|
|
x = np.arange(3, dtype=np.float32)
|
|
res = f(x)
|
|
self.assertAllClose(
|
|
res,
|
|
np.sin(x) if jtu.device_under_test() == "cpu" else np.cos(x))
|
|
|
|
def test_platform_dependent_no_args(self):
|
|
def f(x):
|
|
return lax.platform_dependent(cpu=lambda: jnp.sin(x),
|
|
default=lambda: jnp.cos(x))
|
|
|
|
x = np.arange(3, dtype=np.float32)
|
|
res = f(x)
|
|
self.assertAllClose(
|
|
res,
|
|
np.sin(x) if jtu.device_under_test() == "cpu" else np.cos(x))
|
|
|
|
def test_platform_dependent_lowering(self):
|
|
def f(x):
|
|
return lax.platform_dependent(x, cpu=jnp.sin, default=jnp.cos)
|
|
|
|
x = np.arange(3, dtype=np.float32)
|
|
lowered = jax.jit(f).lower(x)
|
|
stablehlo = lowered.as_text()
|
|
self.assertIn("stablehlo.case", stablehlo)
|
|
self.assertIn("stablehlo.sine", stablehlo)
|
|
self.assertIn("stablehlo.cosine", stablehlo)
|
|
|
|
# The HLO has been canonicalized and contains only the branch we need
|
|
hlo = lowered.as_text("hlo")
|
|
if jtu.device_under_test() == "cpu":
|
|
self.assertIn(" sine", hlo)
|
|
self.assertNotIn(" cosine", hlo)
|
|
else:
|
|
self.assertNotIn(" sine", hlo)
|
|
self.assertIn(" cosine", hlo)
|
|
|
|
def test_platform_dependent_multiple_identical_branches(self):
|
|
x = np.arange(3, dtype=np.float32)
|
|
def f(x):
|
|
return lax.platform_dependent(
|
|
x,
|
|
cpu=jnp.sin,
|
|
tpu=jnp.sin,
|
|
default=lambda x: x)
|
|
res = f(x)
|
|
self.assertAllClose(
|
|
res,
|
|
np.sin(x) if jtu.device_under_test() in ["cpu", "tpu"] else x)
|
|
# We only lower the common branches once
|
|
stablehlo = jax.jit(f).lower(x).as_text()
|
|
sines = re.findall(r"stablehlo.sine", stablehlo)
|
|
self.assertEqual(1, len(sines))
|
|
|
|
def test_platform_dependent_no_default(self):
|
|
ctx = contextlib.ExitStack()
|
|
if jtu.device_under_test() != "tpu":
|
|
ctx.enter_context(
|
|
self.assertRaisesRegex(NotImplementedError,
|
|
"translation rule .* not found for platform"))
|
|
with ctx:
|
|
lax.platform_dependent(
|
|
3.,
|
|
tpu=lambda x: x + 2.)
|
|
|
|
def test_platform_dependent_batched(self):
|
|
def f(x):
|
|
return lax.platform_dependent(x, cpu=jnp.sin, default=jnp.cos)
|
|
|
|
xs = np.arange(3, dtype=np.float32)
|
|
self.assertAllClose(
|
|
jax.vmap(f)(xs),
|
|
np.sin(xs) if jtu.device_under_test() == "cpu" else np.cos(xs))
|
|
# We can still fold the un-needed branch
|
|
hlo = jax.jit(jax.vmap(f)).lower(xs).as_text('hlo')
|
|
expect_a_sine = (jtu.device_under_test() == "cpu")
|
|
self.assertEqual(expect_a_sine, " sine(" in hlo)
|
|
self.assertEqual(not expect_a_sine, " cosine(" in hlo)
|
|
|
|
def test_platform_dependent_grad(self):
|
|
# For a function "lax.dot(x, x)", we choose two branches with very different
|
|
# implementations (a dot and a scan), and therefore different residuals,
|
|
# so that we can verify whether the residuals are as we expect (we don't
|
|
# get residuals from a different platform.
|
|
x = np.arange(8, dtype=np.float32)
|
|
def f_impl_dot(x): # x: f32[8]
|
|
return jnp.dot(x, x)
|
|
def f_impl_scan(x):
|
|
def scan_body(carry, x_i):
|
|
return (carry + x_i * x_i, None)
|
|
return lax.scan(scan_body, np.float32(0.), x)[0]
|
|
|
|
def f(x):
|
|
return jnp.sin(lax.platform_dependent(x,
|
|
cpu=f_impl_dot,
|
|
default=f_impl_scan))
|
|
self.assertAllClose(
|
|
jax.grad(f)(x),
|
|
jax.grad(lambda x: jnp.sin(f_impl_dot(x)))(x))
|
|
|
|
# Check that we do not have contamination of computations across platforms
|
|
hlo = jax.jit(jax.grad(f)).lower(x).as_text('hlo')
|
|
expect_a_dot = (jtu.device_under_test() == "cpu")
|
|
self.assertEqual(expect_a_dot, " dot(" in hlo)
|
|
self.assertEqual(not expect_a_dot, " while(" in hlo)
|
|
|
|
|
|
if __name__ == '__main__':
|
|
absltest.main(testLoader=jtu.JaxTestLoader())
|