mirror of
https://github.com/ROCm/jax.git
synced 2025-04-19 05:16:06 +00:00
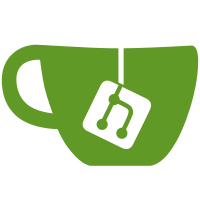
Note that all primitives are now lowered to libdevice calls. Previously, some of them were lowered to the MLIR arith dialect, and some to libdevice calls, without any apparent reason for doing so. PiperOrigin-RevId: 601259707
1229 lines
38 KiB
Python
1229 lines
38 KiB
Python
# Copyright 2024 The JAX Authors.
|
|
#
|
|
# Licensed under the Apache License, Version 2.0 (the "License");
|
|
# you may not use this file except in compliance with the License.
|
|
# You may obtain a copy of the License at
|
|
#
|
|
# https://www.apache.org/licenses/LICENSE-2.0
|
|
#
|
|
# Unless required by applicable law or agreed to in writing, software
|
|
# distributed under the License is distributed on an "AS IS" BASIS,
|
|
# WITHOUT WARRANTIES OR CONDITIONS OF ANY KIND, either express or implied.
|
|
# See the License for the specific language governing permissions and
|
|
# limitations under the License.
|
|
|
|
"""Compatibility layer on top of Triton Python APIs."""
|
|
|
|
# TODO(slebedev): Enable type checking.
|
|
# mypy: ignore-errors
|
|
|
|
from __future__ import annotations
|
|
|
|
from collections.abc import Mapping, Sequence
|
|
from functools import partial, wraps
|
|
import threading
|
|
|
|
from jaxlib.mlir import ir
|
|
from jaxlib.mlir.dialects import arith as arith_dialect
|
|
from jaxlib.mlir.dialects import math as math_dialect
|
|
from jaxlib.mlir.dialects import scf as scf_dialect
|
|
import numpy as np
|
|
import triton.compiler.backends.cuda as cb
|
|
import triton.language as tl
|
|
|
|
from . import dialect as tt_dialect
|
|
|
|
|
|
_tls = threading.local()
|
|
|
|
|
|
def new_ir_context() -> ir.Context:
|
|
ctx = ir.Context()
|
|
tt_dialect.register_dialect(ctx)
|
|
ctx.load_all_available_dialects()
|
|
return ctx
|
|
|
|
|
|
class builder:
|
|
|
|
@classmethod
|
|
@property
|
|
def current(cls) -> "builder":
|
|
return _tls.builder
|
|
|
|
def __init__(self, cuda_options: cb.CUDAOptions):
|
|
self.context = new_ir_context()
|
|
self.loc = ir.Location.unknown(self.context)
|
|
self.options = cuda_options
|
|
|
|
def __enter__(self):
|
|
_tls.builder = self
|
|
self.context.__enter__()
|
|
self.loc.__enter__()
|
|
return self
|
|
|
|
def __exit__(self, *exc_info):
|
|
self.loc.__exit__(*exc_info)
|
|
self.context.__exit__(*exc_info)
|
|
del _tls.builder
|
|
|
|
def create_module(self, *args):
|
|
raise NotImplementedError
|
|
|
|
def set_insertion_point_to_start(self, *args):
|
|
raise NotImplementedError
|
|
|
|
def set_insertion_point_to_end(self, *args):
|
|
raise NotImplementedError
|
|
|
|
def set_insertion_point_after(self, *args):
|
|
raise NotImplementedError
|
|
|
|
def get_insertion_block(self, *args):
|
|
raise NotImplementedError
|
|
|
|
def get_insertion_point(self, *args):
|
|
raise NotImplementedError
|
|
|
|
def restore_insertion_point(self, *args):
|
|
raise NotImplementedError
|
|
|
|
def set_loc(self, *args):
|
|
raise NotImplementedError
|
|
|
|
def get_loc(self, *args):
|
|
raise NotImplementedError
|
|
|
|
def get_bool_attr(self, v: bool) -> ir.BoolAttr:
|
|
return ir.BoolAttr.get(v)
|
|
|
|
def get_int32_attr(self, v: int) -> ir.IntegerAttr:
|
|
return ir.IntegerAttr.get(ir.IntegerType.get_signless(32), v)
|
|
|
|
def get_int1(self, v: bool) -> arith_dialect.ConstantOp:
|
|
return arith_dialect.ConstantOp(self.get_int1_ty(), v)
|
|
|
|
def get_int8(self, v: int) -> arith_dialect.ConstantOp:
|
|
return arith_dialect.ConstantOp(self.get_int8_ty(), v)
|
|
|
|
def get_int16(self, v: int) -> arith_dialect.ConstantOp:
|
|
return arith_dialect.ConstantOp(self.get_int16_ty(), v)
|
|
|
|
def get_int32(self, v: int) -> arith_dialect.ConstantOp:
|
|
return arith_dialect.ConstantOp(self.get_int32_ty(), v)
|
|
|
|
def get_int64(self, v: int) -> arith_dialect.ConstantOp:
|
|
return arith_dialect.ConstantOp(self.get_int64_ty(), v)
|
|
|
|
get_uint8 = get_int8
|
|
get_uint16 = get_int16
|
|
get_uint32 = get_int32
|
|
get_uint64 = get_int64
|
|
|
|
def get_bf16(self, v: float) -> arith_dialect.ConstantOp:
|
|
return arith_dialect.ConstantOp(ir.BF16Type.get(), float(v))
|
|
|
|
def get_fp16(self, v: float) -> arith_dialect.ConstantOp:
|
|
return arith_dialect.ConstantOp(ir.F16Type.get(), float(v))
|
|
|
|
def get_fp32(self, v: float) -> arith_dialect.ConstantOp:
|
|
return arith_dialect.ConstantOp(ir.F32Type.get(), float(v))
|
|
|
|
def get_fp64(self, v: float) -> arith_dialect.ConstantOp:
|
|
return arith_dialect.ConstantOp(ir.F64Type.get(), float(v))
|
|
|
|
def get_null_value(self, t: ir.Type) -> ir.Value:
|
|
if isinstance(t, ir.IntegerType):
|
|
return arith_dialect.ConstantOp(t, 0)
|
|
elif isinstance(t, _FLOAT_TYPES):
|
|
return arith_dialect.ConstantOp(t, 0.0)
|
|
raise NotImplementedError
|
|
|
|
def get_all_ones_values(self, t: ir.Type) -> ir.Value:
|
|
if isinstance(t, ir.IntegerType):
|
|
return arith_dialect.ConstantOp(t, 0xFFFFFFFFFFFFFFFF)
|
|
raise NotImplementedError
|
|
|
|
def get_void_ty(self) -> ir.Type:
|
|
return ir.NoneType.get()
|
|
|
|
def get_int1_ty(self) -> ir.Type:
|
|
return ir.IntegerType.get_signless(1)
|
|
|
|
def get_int8_ty(self) -> ir.Type:
|
|
return ir.IntegerType.get_signless(8)
|
|
|
|
def get_int16_ty(self) -> ir.Type:
|
|
return ir.IntegerType.get_signless(16)
|
|
|
|
def get_int32_ty(self) -> ir.Type:
|
|
return ir.IntegerType.get_signless(32)
|
|
|
|
def get_int64_ty(self) -> ir.Type:
|
|
return ir.IntegerType.get_signless(64)
|
|
|
|
def get_fp8e4nv_ty(self) -> ir.Type:
|
|
return ir.Float8E4M3FNUZType.get()
|
|
|
|
def get_fp8e4b15_ty(self) -> ir.Type:
|
|
return ir.Float8E4M3B11FNUZType.get()
|
|
|
|
def get_fp8e4b15x4_ty(self) -> ir.Type:
|
|
return ir.Float8E4M3FNType.get()
|
|
|
|
def get_fp8e5_ty(self) -> ir.Type:
|
|
return ir.Float8E5M2Type.get()
|
|
|
|
def get_half_ty(self) -> ir.Type:
|
|
return ir.F16Type.get()
|
|
|
|
def get_bf16_ty(self) -> ir.Type:
|
|
return ir.BF16Type.get()
|
|
|
|
def get_float_ty(self) -> ir.Type:
|
|
return ir.F32Type.get()
|
|
|
|
def get_double_ty(self) -> ir.Type:
|
|
return ir.F64Type.get()
|
|
|
|
def get_ptr_ty(self, t: ir.Type, addr_space: int) -> ir.Type:
|
|
return tt_dialect.PointerType.get(t, addr_space)
|
|
|
|
def get_block_ty(
|
|
self, t: ir.Type, shape: Sequence[int]
|
|
) -> ir.RankedTensorType:
|
|
return ir.RankedTensorType.get(shape, t)
|
|
|
|
def get_function_ty(
|
|
self, in_types: Sequence[ir.Type], out_types: Sequence[ir.Type]
|
|
) -> type[ir.FunctionType]:
|
|
return ir.FunctionType.get(in_types, out_types)
|
|
|
|
def get_or_insert_function(self, *args):
|
|
raise NotImplementedError
|
|
|
|
def create_block(self, *args):
|
|
raise NotImplementedError
|
|
|
|
def create_block_with_parent(self, *args):
|
|
raise NotImplementedError
|
|
|
|
def new_block(self):
|
|
raise NotImplementedError
|
|
|
|
def ret(self, vs: Sequence[ir.Value]) -> tt_dialect.ReturnOp:
|
|
return tt_dialect.ReturnOp(vs)
|
|
|
|
def call(
|
|
self, func: tt_dialect.FuncOp, args: Sequence[ir.Value]
|
|
) -> tt_dialect.CallOp:
|
|
func_type: ir.FunctionType = func.function_type
|
|
return tt_dialect.CallOp(func_type.results, func.function_type, args)
|
|
|
|
def create_cond_branch(self, *args):
|
|
raise NotImplementedError
|
|
|
|
def create_branch(self, *args):
|
|
raise NotImplementedError
|
|
|
|
def create_for_op(
|
|
self,
|
|
lb: ir.Value,
|
|
ub: ir.Value,
|
|
step: ir.Value,
|
|
init_args: Sequence[ir.Value],
|
|
) -> scf_dialect.ForOp:
|
|
return scf_dialect.ForOp(lb, ub, step, init_args)
|
|
|
|
def create_if_op(
|
|
self, ret_types: Sequence[ir.Type], condition: ir.Value, with_else: bool
|
|
) -> scf_dialect.IfOp:
|
|
return scf_dialect.IfOp(condition, ret_types, hasElse=with_else)
|
|
|
|
def create_yield_op(self, yields: Sequence[ir.Value]) -> scf_dialect.YieldOp:
|
|
return scf_dialect.YieldOp(yields)
|
|
|
|
def create_while_op(
|
|
self, ret_types: Sequence[ir.Type], init_args: Sequence[ir.Value]
|
|
) -> scf_dialect.WhileOp:
|
|
return scf_dialect.WhileOp(ret_types, init_args)
|
|
|
|
def create_condition_op(
|
|
self, cond: ir.Value, args: Sequence[ir.Value]
|
|
) -> scf_dialect.ConditionOp:
|
|
return scf_dialect.ConditionOp(cond, args)
|
|
|
|
def create_fp_to_fp(self, src: ir.Value, dst_type: ir.Type) -> ir.Value:
|
|
return tt_dialect.fp_to_fp(dst_type, src)
|
|
|
|
def create_bitcast(self, src: ir.Value, dst_type: ir.Type) -> ir.Value:
|
|
return tt_dialect.bitcast(dst_type, src)
|
|
|
|
def create_si_to_fp(self, src: ir.Value, dst_type: ir.Type) -> ir.Value:
|
|
return arith_dialect.sitofp(dst_type, src)
|
|
|
|
def create_ui_to_fp(self, src: ir.Value, dst_type: ir.Type) -> ir.Value:
|
|
return arith_dialect.uitofp(dst_type, src)
|
|
|
|
def create_fp_to_si(self, src: ir.Value, dst_type: ir.Type) -> ir.Value:
|
|
return arith_dialect.fptosi(dst_type, src)
|
|
|
|
def create_fp_to_ui(self, src: ir.Value, dst_type: ir.Type) -> ir.Value:
|
|
return arith_dialect.fptoui(dst_type, src)
|
|
|
|
def create_fp_ext(self, src: ir.Value, dst_type: ir.Type) -> ir.Value:
|
|
return arith_dialect.extf(dst_type, src)
|
|
|
|
def create_fp_trunc(self, src: ir.Value, dst_type: ir.Type) -> ir.Value:
|
|
return arith_dialect.truncf(dst_type, src)
|
|
|
|
def create_int_cast(
|
|
self, src: ir.Value, dst_type: ir.Type, is_signed: bool
|
|
) -> ir.Value:
|
|
src_type = src.type
|
|
if ir.RankedTensorType.isinstance(
|
|
src_type
|
|
) and ir.RankedTensorType.isinstance(dst_type):
|
|
src_element_type = ir.RankedTensorType(src_type).element_type
|
|
dst_element_type = ir.RankedTensorType(dst_type).element_type
|
|
else:
|
|
src_element_type = src_type
|
|
dst_element_type = dst_type
|
|
src_width = ir.IntegerType(src_element_type).width
|
|
dst_width = ir.IntegerType(dst_element_type).width
|
|
if src_width == dst_width:
|
|
return arith_dialect.bitcast(dst_type, src)
|
|
elif src_width > dst_width:
|
|
return arith_dialect.trunci(dst_type, src)
|
|
elif is_signed:
|
|
return arith_dialect.extsi(dst_type, src)
|
|
else:
|
|
return arith_dialect.extui(dst_type, src)
|
|
|
|
def create_to_index(self, input: ir.Value) -> ir.Value:
|
|
return arith_dialect.index_cast(ir.IndexType.get(), input)
|
|
|
|
def create_index_to_si(self, input: ir.Value) -> ir.Value:
|
|
return arith_dialect.index_cast(self.get_int64_ty(), input)
|
|
|
|
def create_fmul(self, lhs: ir.Value, rhs: ir.Value) -> ir.Value:
|
|
return arith_dialect.mulf(lhs, rhs)
|
|
|
|
def create_fdiv(self, lhs: ir.Value, rhs: ir.Value) -> ir.Value:
|
|
return arith_dialect.divf(lhs, rhs)
|
|
|
|
def create_frem(self, lhs: ir.Value, rhs: ir.Value) -> ir.Value:
|
|
return arith_dialect.remf(lhs, rhs)
|
|
|
|
def create_fadd(self, lhs: ir.Value, rhs: ir.Value) -> ir.Value:
|
|
return arith_dialect.addf(lhs, rhs)
|
|
|
|
def create_fsub(self, lhs: ir.Value, rhs: ir.Value) -> ir.Value:
|
|
return arith_dialect.subf(lhs, rhs)
|
|
|
|
def create_mul(self, lhs: ir.Value, rhs: ir.Value) -> ir.Value:
|
|
return arith_dialect.muli(lhs, rhs)
|
|
|
|
def create_sdiv(self, lhs: ir.Value, rhs: ir.Value) -> ir.Value:
|
|
return arith_dialect.divsi(lhs, rhs)
|
|
|
|
def create_udiv(self, lhs: ir.Value, rhs: ir.Value) -> ir.Value:
|
|
return arith_dialect.divui(lhs, rhs)
|
|
|
|
def create_srem(self, lhs: ir.Value, rhs: ir.Value) -> ir.Value:
|
|
return arith_dialect.remsi(lhs, rhs)
|
|
|
|
def create_urem(self, lhs: ir.Value, rhs: ir.Value) -> ir.Value:
|
|
return arith_dialect.remui(lhs, rhs)
|
|
|
|
def create_add(self, lhs: ir.Value, rhs: ir.Value) -> ir.Value:
|
|
return arith_dialect.addi(lhs, rhs)
|
|
|
|
def create_sub(self, lhs: ir.Value, rhs: ir.Value) -> ir.Value:
|
|
return arith_dialect.subi(lhs, rhs)
|
|
|
|
def create_shl(self, lhs: ir.Value, rhs: ir.Value) -> ir.Value:
|
|
return arith_dialect.shli(lhs, rhs)
|
|
|
|
def create_lshr(self, lhs: ir.Value, rhs: ir.Value) -> ir.Value:
|
|
return arith_dialect.shrui(lhs, rhs)
|
|
|
|
def create_ashr(self, lhs: ir.Value, rhs: ir.Value) -> ir.Value:
|
|
return arith_dialect.shrsi(lhs, rhs)
|
|
|
|
def create_minsi(self, lhs: ir.Value, rhs: ir.Value) -> ir.Value:
|
|
return arith_dialect.minsi(lhs, rhs)
|
|
|
|
def create_minui(self, lhs: ir.Value, rhs: ir.Value) -> ir.Value:
|
|
return arith_dialect.minui(lhs, rhs)
|
|
|
|
def create_minimumf(self, lhs: ir.Value, rhs: ir.Value) -> ir.Value:
|
|
return arith_dialect.minimumf(lhs, rhs)
|
|
|
|
def create_minnumf(self, lhs: ir.Value, rhs: ir.Value) -> ir.Value:
|
|
return arith_dialect.minnumf(lhs, rhs)
|
|
|
|
def create_maxsi(self, lhs: ir.Value, rhs: ir.Value) -> ir.Value:
|
|
return arith_dialect.maxsi(lhs, rhs)
|
|
|
|
def create_maxui(self, lhs: ir.Value, rhs: ir.Value) -> ir.Value:
|
|
return arith_dialect.maxui(lhs, rhs)
|
|
|
|
def create_maximumf(self, lhs: ir.Value, rhs: ir.Value) -> ir.Value:
|
|
return arith_dialect.maximumf(lhs, rhs)
|
|
|
|
def create_maxnumf(self, lhs: ir.Value, rhs: ir.Value) -> ir.Value:
|
|
return arith_dialect.maxnumf(lhs, rhs)
|
|
|
|
def create_addptr(self, ptr: ir.Value, offset: ir.Value) -> ir.Value:
|
|
return tt_dialect.addptr(ptr.type, ptr, offset)
|
|
|
|
def create_icmpSLE(self, lhs: ir.Value, rhs: ir.Value) -> ir.Value:
|
|
return arith_dialect.cmpi(arith_dialect.CmpIPredicate.sle, lhs, rhs)
|
|
|
|
def create_icmpSLT(self, lhs: ir.Value, rhs: ir.Value) -> ir.Value:
|
|
return arith_dialect.cmpi(arith_dialect.CmpIPredicate.slt, lhs, rhs)
|
|
|
|
def create_icmpSGE(self, lhs: ir.Value, rhs: ir.Value) -> ir.Value:
|
|
return arith_dialect.cmpi(arith_dialect.CmpIPredicate.sge, lhs, rhs)
|
|
|
|
def create_icmpSGT(self, lhs: ir.Value, rhs: ir.Value) -> ir.Value:
|
|
return arith_dialect.cmpi(arith_dialect.CmpIPredicate.sgt, lhs, rhs)
|
|
|
|
def create_icmpULE(self, lhs: ir.Value, rhs: ir.Value) -> ir.Value:
|
|
return arith_dialect.cmpi(arith_dialect.CmpIPredicate.ule, lhs, rhs)
|
|
|
|
def create_icmpULT(self, lhs: ir.Value, rhs: ir.Value) -> ir.Value:
|
|
return arith_dialect.cmpi(arith_dialect.CmpIPredicate.ult, lhs, rhs)
|
|
|
|
def create_icmpUGE(self, lhs: ir.Value, rhs: ir.Value) -> ir.Value:
|
|
return arith_dialect.cmpi(arith_dialect.CmpIPredicate.uge, lhs, rhs)
|
|
|
|
def create_icmpUGT(self, lhs: ir.Value, rhs: ir.Value) -> ir.Value:
|
|
return arith_dialect.cmpi(arith_dialect.CmpIPredicate.ugt, lhs, rhs)
|
|
|
|
def create_icmpEQ(self, lhs: ir.Value, rhs: ir.Value) -> ir.Value:
|
|
return arith_dialect.cmpi(arith_dialect.CmpIPredicate.eq, lhs, rhs)
|
|
|
|
def create_icmpNE(self, lhs: ir.Value, rhs: ir.Value) -> ir.Value:
|
|
return arith_dialect.cmpi(arith_dialect.CmpIPredicate.ne, lhs, rhs)
|
|
|
|
def create_fcmpOLT(self, lhs: ir.Value, rhs: ir.Value) -> ir.Value:
|
|
return arith_dialect.cmpf(arith_dialect.CmpFPredicate.OLT, lhs, rhs)
|
|
|
|
def create_fcmpOGT(self, lhs: ir.Value, rhs: ir.Value) -> ir.Value:
|
|
return arith_dialect.cmpf(arith_dialect.CmpFPredicate.OGT, lhs, rhs)
|
|
|
|
def create_fcmpOLE(self, lhs: ir.Value, rhs: ir.Value) -> ir.Value:
|
|
return arith_dialect.cmpf(arith_dialect.CmpFPredicate.OLE, lhs, rhs)
|
|
|
|
def create_fcmpOGE(self, lhs: ir.Value, rhs: ir.Value) -> ir.Value:
|
|
return arith_dialect.cmpf(arith_dialect.CmpFPredicate.OGE, lhs, rhs)
|
|
|
|
def create_fcmpOEQ(self, lhs: ir.Value, rhs: ir.Value) -> ir.Value:
|
|
return arith_dialect.cmpf(arith_dialect.CmpFPredicate.OEQ, lhs, rhs)
|
|
|
|
def create_fcmpONE(self, lhs: ir.Value, rhs: ir.Value) -> ir.Value:
|
|
return arith_dialect.cmpf(arith_dialect.CmpFPredicate.ONE, lhs, rhs)
|
|
|
|
def create_fcmpULT(self, lhs: ir.Value, rhs: ir.Value) -> ir.Value:
|
|
return arith_dialect.cmpf(arith_dialect.CmpFPredicate.ULT, lhs, rhs)
|
|
|
|
def create_fcmpUGT(self, lhs: ir.Value, rhs: ir.Value) -> ir.Value:
|
|
return arith_dialect.cmpf(arith_dialect.CmpFPredicate.UGT, lhs, rhs)
|
|
|
|
def create_fcmpULE(self, lhs: ir.Value, rhs: ir.Value) -> ir.Value:
|
|
return arith_dialect.cmpf(arith_dialect.CmpFPredicate.ULE, lhs, rhs)
|
|
|
|
def create_fcmpUGE(self, lhs: ir.Value, rhs: ir.Value) -> ir.Value:
|
|
return arith_dialect.cmpf(arith_dialect.CmpFPredicate.UGE, lhs, rhs)
|
|
|
|
def create_fcmpUEQ(self, lhs: ir.Value, rhs: ir.Value) -> ir.Value:
|
|
return arith_dialect.cmpf(arith_dialect.CmpFPredicate.UEQ, lhs, rhs)
|
|
|
|
def create_fcmpUNE(self, lhs: ir.Value, rhs: ir.Value) -> ir.Value:
|
|
return arith_dialect.cmpf(arith_dialect.CmpFPredicate.UNE, lhs, rhs)
|
|
|
|
def create_and(self, lhs: ir.Value, rhs: ir.Value) -> ir.Value:
|
|
return arith_dialect.andi(lhs, rhs)
|
|
|
|
def create_xor(self, lhs: ir.Value, rhs: ir.Value) -> ir.Value:
|
|
return arith_dialect.xori(lhs, rhs)
|
|
|
|
def create_or(self, lhs: ir.Value, rhs: ir.Value) -> ir.Value:
|
|
return arith_dialect.ori(lhs, rhs)
|
|
|
|
def create_load(
|
|
self,
|
|
ptr: ir.Value,
|
|
cache_modifier: tt_dialect.CacheModifier,
|
|
eviction_policy: tt_dialect.EvictionPolicy,
|
|
is_volatile: bool,
|
|
) -> ir.Value:
|
|
if ir.RankedTensorType.isinstance(ptr.type):
|
|
ptr_type = ir.RankedTensorType(ptr.type)
|
|
element_type = tt_dialect.PointerType(ptr_type.element_type)
|
|
result_type = ir.RankedTensorType.get(
|
|
ptr_type.shape,
|
|
element_type.pointee_type,
|
|
ptr_type.encoding,
|
|
)
|
|
else:
|
|
ptr_type = tt_dialect.PointerType(ptr.type)
|
|
result_type = ptr_type.pointee_type
|
|
return tt_dialect.load(
|
|
result_type, ptr, cache_modifier, eviction_policy, is_volatile
|
|
)
|
|
|
|
def create_store(
|
|
self,
|
|
ptr: ir.Value,
|
|
value: ir.Value,
|
|
cache_modifier: tt_dialect.CacheModifier,
|
|
eviction_policy: tt_dialect.EvictionPolicy,
|
|
) -> ir.Value:
|
|
return tt_dialect.store(
|
|
ptr, value, cache=cache_modifier, evict=eviction_policy
|
|
)
|
|
|
|
def create_tensor_pointer_load(
|
|
self,
|
|
ptr: ir.Value,
|
|
boundary_check: Sequence[int],
|
|
padding_option: Sequence[tt_dialect.PaddingOption],
|
|
cache_modifier: tt_dialect.CacheModifier,
|
|
eviction_policy: tt_dialect.EvictionPolicy,
|
|
is_volatile: bool,
|
|
) -> ir.Value:
|
|
return tt_dialect.load(
|
|
ptr.type,
|
|
ptr,
|
|
cache_modifier,
|
|
eviction_policy,
|
|
is_volatile,
|
|
boundary_check=boundary_check,
|
|
padding=padding_option,
|
|
)
|
|
|
|
def create_tensor_pointer_store(
|
|
self,
|
|
ptr: ir.Value,
|
|
value: ir.Value,
|
|
boundary_check: Sequence[int],
|
|
cache_modifier: tt_dialect.CacheModifier,
|
|
eviction_policy: tt_dialect.EvictionPolicy,
|
|
) -> ir.Value:
|
|
return tt_dialect.store(
|
|
ptr,
|
|
value,
|
|
boundary_check=boundary_check,
|
|
cache=cache_modifier,
|
|
evict=eviction_policy,
|
|
)
|
|
|
|
def create_masked_load(
|
|
self,
|
|
ptr: ir.Value,
|
|
mask: ir.Value,
|
|
other: ir.Value | None,
|
|
cache_modifier: tt_dialect.CacheModifier,
|
|
eviction_policy: tt_dialect.EvictionPolicy,
|
|
is_volatile: bool,
|
|
) -> ir.Value:
|
|
if ir.RankedTensorType.isinstance(ptr.type):
|
|
ptr_type = ir.RankedTensorType(ptr.type)
|
|
element_type = tt_dialect.PointerType(ptr_type.element_type)
|
|
result_type = ir.RankedTensorType.get(
|
|
ptr_type.shape,
|
|
element_type.pointee_type,
|
|
ptr_type.encoding,
|
|
)
|
|
else:
|
|
ptr_type = tt_dialect.PointerType(ptr.type)
|
|
result_type = ptr_type.pointee_type
|
|
return tt_dialect.load(
|
|
result_type,
|
|
ptr,
|
|
cache_modifier,
|
|
eviction_policy,
|
|
is_volatile,
|
|
mask=mask,
|
|
other=other,
|
|
)
|
|
|
|
def create_masked_store(
|
|
self,
|
|
ptr: ir.Value,
|
|
value: ir.Value,
|
|
mask: ir.Value,
|
|
cache_modifier: tt_dialect.CacheModifier,
|
|
eviction_policy: tt_dialect.EvictionPolicy,
|
|
) -> ir.Value:
|
|
return tt_dialect.store(
|
|
ptr,
|
|
value,
|
|
mask=mask,
|
|
cache=cache_modifier,
|
|
evict=eviction_policy,
|
|
)
|
|
|
|
def create_cat(self, lhs: ir.Value, rhs: ir.Value) -> ir.Value:
|
|
assert ir.RankedTensorType.isinstance(lhs.type)
|
|
assert ir.RankedTensorType.isinstance(rhs.type)
|
|
lhs_type = ir.RankedTensorType(lhs.type)
|
|
rhs_type = ir.RankedTensorType(rhs.type)
|
|
assert len(lhs_type.shape) == 1 and len(rhs_type.shape) == 1
|
|
result_type = ir.RankedTensorType.get(
|
|
[lhs_type.shape[0] + rhs_type.shape[0]],
|
|
lhs_type.element_type,
|
|
lhs_type.encoding,
|
|
)
|
|
return tt_dialect.cat(result_type, lhs, rhs)
|
|
|
|
def create_interleave(self, lhs: ir.Value, rhs: ir.Value) -> ir.Value:
|
|
raise NotImplementedError
|
|
|
|
def create_trans(self, arg: ir.Value) -> ir.Value:
|
|
return tt_dialect.trans(arg)
|
|
|
|
def create_broadcast(self, arg: ir.Value, shape: Sequence[int]) -> ir.Value:
|
|
assert ir.RankedTensorType.isinstance(arg.type)
|
|
arg_type = ir.RankedTensorType(arg.type)
|
|
result_type = ir.RankedTensorType.get(
|
|
shape, arg_type.element_type, arg_type.encoding
|
|
)
|
|
return tt_dialect.broadcast(result_type, arg)
|
|
|
|
def create_splat(self, arg: ir.Value, shape: Sequence[int]) -> ir.Value:
|
|
result_type = ir.RankedTensorType.get(shape, arg.type)
|
|
return tt_dialect.splat(result_type, arg)
|
|
|
|
def create_atomic_cas(
|
|
self,
|
|
ptr: ir.Value,
|
|
cmp: ir.Value,
|
|
val: ir.Value,
|
|
sem: tt_dialect.MemSemantic,
|
|
scope: tt_dialect.MemSyncScope,
|
|
) -> ir.Value:
|
|
if ir.RankedTensorType.isinstance(ptr.type):
|
|
ptr_type = ir.RankedTensorType(ptr.type)
|
|
element_type = tt_dialect.PointerType(ptr_type.element_type)
|
|
result_type = ir.RankedTensorType.get(
|
|
ptr_type.shape, element_type.pointee_type, ptr_type.encoding
|
|
)
|
|
else:
|
|
result_type = tt_dialect.PointerType(ptr.type).pointee_type
|
|
return tt_dialect.atomic_cas(result_type, ptr, cmp, val, sem, scope)
|
|
|
|
def create_atomic_rmw(
|
|
self,
|
|
rmw_op: tt_dialect.RMWOp,
|
|
ptr: ir.Value,
|
|
val: ir.Value,
|
|
mask: ir.Value,
|
|
sem: tt_dialect.MemSemantic,
|
|
scope: tt_dialect.MemSyncScope,
|
|
) -> ir.Value:
|
|
if ir.RankedTensorType.isinstance(ptr.type):
|
|
ptr_type = ir.RankedTensorType(ptr.type)
|
|
element_type = tt_dialect.PointerType(ptr_type.element_type)
|
|
result_type = ir.RankedTensorType.get(
|
|
ptr_type.shape, element_type.pointee_type, ptr_type.encoding
|
|
)
|
|
else:
|
|
result_type = tt_dialect.PointerType(ptr.type).pointee_type
|
|
return tt_dialect.atomic_rmw(
|
|
result_type, rmw_op, ptr, val, sem, scope, mask=mask
|
|
)
|
|
|
|
def create_extern_elementwise(
|
|
self,
|
|
lib_name: str,
|
|
lib_path: str,
|
|
symbol: str,
|
|
args: Sequence[ir.Value],
|
|
return_type: ir.Type,
|
|
is_pure: bool,
|
|
) -> ir.Value:
|
|
return tt_dialect.extern_elementwise(
|
|
return_type, args, lib_name, lib_path, symbol, is_pure
|
|
)
|
|
|
|
def create_get_num_programs(self, axis: int) -> ir.Value:
|
|
return tt_dialect.get_num_programs(axis)
|
|
|
|
def create_dot(
|
|
self,
|
|
a: ir.Value,
|
|
b: ir.Value,
|
|
c: ir.Value,
|
|
allow_tf32: bool,
|
|
max_num_imprecise_acc: int,
|
|
) -> ir.Value:
|
|
return tt_dialect.dot(a, b, c, allow_tf32, max_num_imprecise_acc)
|
|
|
|
def create_reduce(
|
|
self, operands: Sequence[ir.Value], axis: int
|
|
) -> tt_dialect.ReduceOp:
|
|
return_types = _infer_reduce_op_return_types(operands, axis)
|
|
return tt_dialect.ReduceOp(return_types, operands, axis)
|
|
|
|
def create_reduce_ret(self, *args: ir.Value) -> ir.Value:
|
|
return tt_dialect.reduce_return(args)
|
|
|
|
def create_scan(
|
|
self, operands: Sequence[ir.Value], axis: int
|
|
) -> tt_dialect.ScanOp:
|
|
return tt_dialect.ScanOp([op.type for op in operands], operands, axis)
|
|
|
|
def create_scan_ret(self, *args: ir.Value) -> ir.Value:
|
|
return tt_dialect.scan_return(args)
|
|
|
|
def create_ptr_to_int(self, val: ir.Value, t: ir.Type) -> ir.Value:
|
|
return tt_dialect.ptr_to_int(t, val)
|
|
|
|
def create_int_to_ptr(self, val: ir.Value, t: ir.Type) -> ir.Value:
|
|
return tt_dialect.int_to_ptr(t, val)
|
|
|
|
def create_select(
|
|
self, condition: ir.Value, true_value: ir.Value, false_value: ir.Value
|
|
) -> ir.Value:
|
|
return arith_dialect.select(condition, true_value, false_value)
|
|
|
|
def create_inline_asm(self, *args):
|
|
raise NotImplementedError
|
|
|
|
def create_print(self, prefix: str, values: Sequence[ir.Value]) -> None:
|
|
tt_dialect.print_(prefix, values)
|
|
|
|
def create_assert(
|
|
self,
|
|
condition: ir.Value,
|
|
message: str,
|
|
file_name: str,
|
|
func_name: str,
|
|
line_no: int,
|
|
) -> None:
|
|
tt_dialect.assert_(condition, message, file_name, func_name, line_no)
|
|
|
|
def create_undef(self, t: ir.Type) -> ir.Value:
|
|
raise NotImplementedError
|
|
|
|
def create_barrier(self):
|
|
# TODO(slebedev): This needs Triton GPU dialect.
|
|
raise NotImplementedError
|
|
|
|
def create_make_block_ptr(
|
|
self,
|
|
base: ir.Value,
|
|
shape: Sequence[ir.Value],
|
|
strides: Sequence[ir.Value],
|
|
offsets: Sequence[ir.Value],
|
|
tensor_shape: Sequence[int],
|
|
order: Sequence[int],
|
|
) -> ir.Value:
|
|
# TODO(slebedev): How to compute result=?
|
|
raise NotImplementedError
|
|
|
|
def create_advance(
|
|
self, ptr: ir.Value, offsets: Sequence[ir.Value]
|
|
) -> ir.Value:
|
|
return tt_dialect.advance(ptr.type, ptr, offsets)
|
|
|
|
|
|
# The following reimplements return type inference for some Triton operations.
|
|
# We cannot avoid doing that atm, because MLIR Python bindings do not support
|
|
# neither
|
|
# * transparent return type inference for operations with regions; nor
|
|
# * manual return type inference for dialects with usePropertiesForAttributes.
|
|
|
|
|
|
def _infer_reduce_op_return_types(
|
|
operands: Sequence[ir.Value], axis: int
|
|
) -> Sequence[ir.Type]:
|
|
return_types = []
|
|
for op in operands:
|
|
op_type = ir.RankedTensorType(op.type)
|
|
shape = list(op_type.shape)
|
|
del shape[axis]
|
|
if not shape:
|
|
return_types.append(op_type.element_type)
|
|
elif op_encoding := op_type.encoding:
|
|
encoding = tt_dialect.infer_reduce_op_encoding(op_encoding, axis)
|
|
if encoding is not None:
|
|
raise RuntimeError("Failed to infer return type encoding for ReduceOp")
|
|
return_types.append(
|
|
ir.RankedTensorType.get(shape, op_type.element_type, encoding)
|
|
)
|
|
else:
|
|
return_types.append(ir.RankedTensorType.get(shape, op_type.element_type))
|
|
return return_types
|
|
|
|
|
|
_FLOAT_TYPES = (
|
|
ir.Float8E4M3FNUZType,
|
|
ir.Float8E4M3FNType,
|
|
ir.Float8E4M3B11FNUZType,
|
|
ir.Float8E5M2Type,
|
|
ir.BF16Type,
|
|
ir.F16Type,
|
|
ir.F32Type,
|
|
ir.F64Type,
|
|
)
|
|
|
|
dtype = tl.core.dtype
|
|
|
|
block_type = tl.core.block_type
|
|
function_type = tl.core.function_type
|
|
pointer_type = tl.core.pointer_type
|
|
|
|
bfloat16 = tl.core.bfloat16
|
|
float16 = tl.core.float16
|
|
float32 = tl.core.float32
|
|
float64 = tl.core.float64
|
|
int32 = tl.core.int32
|
|
int64 = tl.core.int64
|
|
|
|
|
|
def wrap_with_builder(fn):
|
|
@wraps(fn)
|
|
def inner(*args, **kwargs):
|
|
if tl.core.is_builtin(fn):
|
|
v = fn(*args, **kwargs, _builder=builder.current)
|
|
else:
|
|
v = fn(*args, **kwargs, builder=builder.current)
|
|
if isinstance(v, tl.core.tensor):
|
|
return _to_tensor(v)
|
|
return v
|
|
|
|
return inner
|
|
|
|
|
|
constexpr = tl.core.constexpr
|
|
|
|
|
|
def _to_tensor(v) -> "tensor":
|
|
t = tl.core._to_tensor(v, builder.current)
|
|
return tensor(t.handle, t.type)
|
|
|
|
|
|
class tensor(tl.core.tensor):
|
|
|
|
def __add__(self, other):
|
|
return semantic.add(self, _to_tensor(other))
|
|
|
|
def __radd__(self, other):
|
|
return self + other
|
|
|
|
def __sub__(self, other):
|
|
return semantic.sub(self, _to_tensor(other))
|
|
|
|
def __rsub__(self, other):
|
|
return semantic.sub(_to_tensor(other), self)
|
|
|
|
def __mul__(self, other):
|
|
return semantic.mul(self, _to_tensor(other))
|
|
|
|
def __rmul__(self, other):
|
|
return self * other
|
|
|
|
def __truediv__(self, other):
|
|
return semantic.truediv(self, _to_tensor(other))
|
|
|
|
def __rtruediv__(self, other):
|
|
return semantic.truediv(_to_tensor(other), self)
|
|
|
|
def __floordiv__(self, other):
|
|
return semantic.floordiv(self, _to_tensor(other))
|
|
|
|
def __rfloordiv__(self, other):
|
|
return semantic.floordiv(_to_tensor(other), self)
|
|
|
|
def __mod__(self, other):
|
|
return semantic.mod(self, _to_tensor(other))
|
|
|
|
def __rmod__(self, other):
|
|
return semantic.mod(_to_tensor(other), self)
|
|
|
|
def __neg__(self):
|
|
return semantic.minus(self)
|
|
|
|
def __invert__(self):
|
|
return semantic.invert(self)
|
|
|
|
# TODO(slebedev): Override other comparison methods.
|
|
def __eq__(self, other):
|
|
return semantic.equal(self, _to_tensor(other))
|
|
|
|
def __getitem__(self, slices) -> tensor:
|
|
if isinstance(slices, (slice, constexpr)):
|
|
slices = [slices]
|
|
t = self
|
|
for axis, s in enumerate(slices):
|
|
if s is None or isinstance(s, constexpr) and s.value is None:
|
|
t = expand_dims(t, axis)
|
|
elif (
|
|
isinstance(s, slice)
|
|
and s.start is s.stop is s.step is None
|
|
):
|
|
pass
|
|
else:
|
|
raise IndexError(f"unsupported tensor index: {s}")
|
|
return t
|
|
|
|
to = wrap_with_builder(tl.tensor.to)
|
|
|
|
|
|
def program_id(axis: int) -> tensor:
|
|
if axis not in range(3):
|
|
raise ValueError(f"axis must be in [0, 3), but got: {axis}")
|
|
return tensor(tt_dialect.get_program_id(axis), tl.int32)
|
|
|
|
|
|
load = wrap_with_builder(tl.core.load)
|
|
store = wrap_with_builder(tl.core.store)
|
|
|
|
|
|
def arange(start: int, end: int) -> tensor:
|
|
if end <= start:
|
|
raise ValueError(
|
|
f"end must be greater than start, but got: {end} <= {start}"
|
|
)
|
|
if max(start, end) >= 2**32:
|
|
raise ValueError("start and end must fit in int32")
|
|
ty = block_type(tl.int32, [end - start])
|
|
ir_ty = ir.RankedTensorType.get(
|
|
[end - start], ir.IntegerType.get_signless(32)
|
|
)
|
|
return tensor(tt_dialect.make_range(ir_ty, start, end), ty)
|
|
|
|
|
|
def broadcast_to(x: object, shape: Sequence[int | constexpr]) -> tensor:
|
|
x = _to_tensor(x)
|
|
if not x.type.is_block():
|
|
return splat(x, shape)
|
|
elif x.shape == shape:
|
|
return x
|
|
shape = [dim.__index__() for dim in shape]
|
|
x_ir_type = ir.RankedTensorType(x.handle.type)
|
|
result_ir_type = ir.RankedTensorType.get(
|
|
shape, x_ir_type.element_type, x_ir_type.encoding
|
|
)
|
|
return tensor(
|
|
tt_dialect.broadcast(result_ir_type, x.handle),
|
|
block_type(x.dtype, shape),
|
|
)
|
|
|
|
|
|
def splat(x: object, shape: Sequence[int | constexpr]) -> tensor:
|
|
x = _to_tensor(x)
|
|
if x.type.is_block():
|
|
raise ValueError("cannot splat a block tensor")
|
|
if len(shape) == 0:
|
|
return x
|
|
shape = [dim.__index__() for dim in shape]
|
|
result_ir_type = ir.RankedTensorType.get(shape, x.handle.type)
|
|
return tensor(
|
|
tt_dialect.splat(result_ir_type, x.handle), block_type(x.dtype, shape)
|
|
)
|
|
|
|
|
|
def expand_dims(x: object, axis: int) -> tensor:
|
|
x = _to_tensor(x)
|
|
dst_shape = [dim.__index__() for dim in x.shape]
|
|
dst_shape.insert(axis, 1)
|
|
if not x.type.is_block():
|
|
return splat(input, dst_shape)
|
|
return tensor(
|
|
tt_dialect.expand_dims(x.handle, axis),
|
|
block_type(x.dtype, dst_shape),
|
|
)
|
|
|
|
|
|
def reshape(x: tensor, dst_shape: Sequence[int]) -> tensor:
|
|
x_ir_type = ir.RankedTensorType(x.handle.type)
|
|
result_ir_type = ir.RankedTensorType.get(
|
|
dst_shape, x_ir_type.element_type, x_ir_type.encoding
|
|
)
|
|
return tensor(
|
|
tt_dialect.reshape(result_ir_type, x.handle, allow_reorder=False),
|
|
block_type(x.dtype, dst_shape),
|
|
)
|
|
|
|
|
|
dot = wrap_with_builder(tl.core.dot)
|
|
|
|
atomic_xchg = wrap_with_builder(tl.core.atomic_xchg)
|
|
atomic_add = wrap_with_builder(tl.core.atomic_add)
|
|
atomic_max = wrap_with_builder(tl.core.atomic_max)
|
|
atomic_min = wrap_with_builder(tl.core.atomic_min)
|
|
atomic_and = wrap_with_builder(tl.core.atomic_and)
|
|
atomic_or = wrap_with_builder(tl.core.atomic_or)
|
|
atomic_xor = wrap_with_builder(tl.core.atomic_xor)
|
|
atomic_cas = wrap_with_builder(tl.atomic_cas)
|
|
|
|
|
|
def abs(x: object) -> tensor:
|
|
x = _to_tensor(x)
|
|
dtype = x.dtype
|
|
if dtype.is_floating():
|
|
return tensor(math_dialect.absf(x.handle), x.type)
|
|
elif dtype.is_int_signed():
|
|
return tensor(math_dialect.absi(x.handle), x.type)
|
|
elif dtype.is_int_unsigned():
|
|
return x
|
|
else:
|
|
raise ValueError(f"unsupported dtype: {dtype}")
|
|
|
|
|
|
def exp(x: object) -> tensor:
|
|
x = _to_tensor(x)
|
|
if x.dtype != float32 and x.dtype != float64:
|
|
raise ValueError(f"unsupported dtype: {x.dtype}")
|
|
return tensor(math_dialect.exp(x.handle), x.type)
|
|
|
|
|
|
def log(x: object) -> tensor:
|
|
x = _to_tensor(x)
|
|
if x.dtype != float32 and x.dtype != float64:
|
|
raise ValueError(f"unsupported dtype: {x.dtype}")
|
|
return tensor(math_dialect.log(x.handle), x.type)
|
|
|
|
|
|
def sqrt(x: object) -> tensor:
|
|
x = _to_tensor(x)
|
|
if x.dtype != float32 and x.dtype != float64:
|
|
raise ValueError(f"unsupported dtype: {x.dtype}")
|
|
return tensor(math_dialect.sqrt(x.handle), x.type)
|
|
|
|
|
|
def sin(x: object) -> tensor:
|
|
x = _to_tensor(x)
|
|
if x.dtype != float32 and x.dtype != float64:
|
|
raise ValueError(f"unsupported dtype: {x.dtype}")
|
|
return tensor(math_dialect.sin(x.handle), x.type)
|
|
|
|
|
|
def cos(x: object) -> tensor:
|
|
x = _to_tensor(x)
|
|
if x.dtype != float32 and x.dtype != float64:
|
|
raise ValueError(f"unsupported dtype: {x.dtype}")
|
|
return tensor(math_dialect.cos(x.handle), x.type)
|
|
|
|
|
|
def multiple_of(x: tensor, values: Sequence[int]) -> tl.tensor:
|
|
assert max(1, len(x.shape)) == len(values)
|
|
set_attr(
|
|
x.handle,
|
|
"tt.divisibility",
|
|
ir.DenseIntElementsAttr.get(
|
|
np.fromiter(map(int, values), dtype=np.uint32)
|
|
),
|
|
)
|
|
return x
|
|
|
|
|
|
def max_contiguous(x: tensor, values: Sequence[int]) -> tl.tensor:
|
|
assert len(x.shape) == len(values)
|
|
set_attr(
|
|
x.handle,
|
|
"tt.contiguity",
|
|
ir.DenseIntElementsAttr.get(
|
|
np.fromiter(map(int, values), dtype=np.uint32)
|
|
),
|
|
)
|
|
return x
|
|
|
|
|
|
def set_attr(v: ir.Value, name: str, attr: ir.Attribute) -> None:
|
|
if not ir.BlockArgument.isinstance(v):
|
|
v.owner.attributes[name] = attr
|
|
return
|
|
|
|
arg = ir.BlockArgument(v)
|
|
name += f"_arg{arg.arg_number}"
|
|
owner = arg.owner
|
|
is_entry = owner.region.blocks[0] == owner
|
|
if not is_entry:
|
|
return
|
|
if (op := owner.owner.operation) and not isinstance(op, tt_dialect.FuncOp):
|
|
op.attributes[name] = attr
|
|
|
|
|
|
_LIBDEVICE_PATH = tl.math.libdevice_path()
|
|
|
|
|
|
def libdevice_extern_elementwise(
|
|
table: Mapping[tuple[dtype, ...], tuple[str, dtype]],
|
|
is_pure: bool = True,
|
|
):
|
|
def inner(arg: tensor):
|
|
try:
|
|
symbol, dtype = table[(arg.dtype,)]
|
|
except KeyError:
|
|
raise NotImplementedError(f"unsupported dtypes: {(arg.dtype,)}") from None
|
|
|
|
return_type = dtype
|
|
if arg.type.is_block():
|
|
return_type = block_type(dtype, arg.shape)
|
|
return tensor(
|
|
tt_dialect.extern_elementwise(
|
|
return_type.to_ir(builder.current),
|
|
[arg.handle],
|
|
libname="libdevice",
|
|
libpath=_LIBDEVICE_PATH,
|
|
symbol=symbol,
|
|
pure=is_pure,
|
|
),
|
|
return_type,
|
|
)
|
|
|
|
return inner
|
|
|
|
|
|
class math:
|
|
sin = libdevice_extern_elementwise({
|
|
(float32,): ("__nv_sinf", float32),
|
|
(float64,): ("__nv_sin", float64),
|
|
})
|
|
cos = libdevice_extern_elementwise({
|
|
(float32,): ("__nv_cosf", float32),
|
|
(float64,): ("__nv_cos", float64),
|
|
})
|
|
tan = libdevice_extern_elementwise({
|
|
(float32,): ("__nv_tanf", float32),
|
|
(float64,): ("__nv_tan", float64),
|
|
})
|
|
asin = libdevice_extern_elementwise({
|
|
(float32,): ("__nv_asinf", float32),
|
|
(float64,): ("__nv_asin", float64),
|
|
})
|
|
acos = libdevice_extern_elementwise({
|
|
(float32,): ("__nv_acosf", float32),
|
|
(float64,): ("__nv_acos", float64),
|
|
})
|
|
atan = libdevice_extern_elementwise({
|
|
(float32,): ("__nv_atanf", float32),
|
|
(float64,): ("__nv_atan", float64),
|
|
})
|
|
atan2 = libdevice_extern_elementwise({
|
|
(float32,): ("__nv_atan2f", float32),
|
|
(float64,): ("__nv_atan2", float64),
|
|
})
|
|
sinh = libdevice_extern_elementwise({
|
|
(float32,): ("__nv_sinhf", float32),
|
|
(float64,): ("__nv_sinh", float64),
|
|
})
|
|
cosh = libdevice_extern_elementwise({
|
|
(float32,): ("__nv_coshf", float32),
|
|
(float64,): ("__nv_cosh", float64),
|
|
})
|
|
tanh = libdevice_extern_elementwise({
|
|
(float32,): ("__nv_tanhf", float32),
|
|
(float64,): ("__nv_tanh", float64),
|
|
})
|
|
asinh = libdevice_extern_elementwise({
|
|
(float32,): ("__nv_asinhf", float32),
|
|
(float64,): ("__nv_asinh", float64),
|
|
})
|
|
acosh = libdevice_extern_elementwise({
|
|
(float32,): ("__nv_acosf", float32),
|
|
(float64,): ("__nv_acosh", float64),
|
|
})
|
|
atanh = libdevice_extern_elementwise({
|
|
(float32,): ("__nv_atanhf", float32),
|
|
(float64,): ("__nv_atanh", float64),
|
|
})
|
|
|
|
cbrt = libdevice_extern_elementwise({
|
|
(float32,): ("__nv_cbrtf", float32),
|
|
(float64,): ("__nv_cbrt", float64),
|
|
})
|
|
clz = libdevice_extern_elementwise({
|
|
(int32,): ("__nv_clz", int32),
|
|
(int64,): ("__nv_clzll", int64),
|
|
})
|
|
exp = libdevice_extern_elementwise({
|
|
(float32,): ("__nv_expf", float32),
|
|
(float64,): ("__nv_exp", float64),
|
|
})
|
|
exp2 = libdevice_extern_elementwise({
|
|
(float32,): ("__nv_exp2f", float32),
|
|
(float64,): ("__nv_exp2", float64),
|
|
})
|
|
expm1 = libdevice_extern_elementwise({
|
|
(float32,): ("__nv_expm1f", float32),
|
|
(float64,): ("__nv_expm1", float64),
|
|
})
|
|
log = libdevice_extern_elementwise({
|
|
(float32,): ("__nv_logf", float32),
|
|
(float64,): ("__nv_log", float64),
|
|
})
|
|
log1p = libdevice_extern_elementwise({
|
|
(float32,): ("__nv_log1pf", float32),
|
|
(float64,): ("__nv_log1p", float64),
|
|
})
|
|
floor = libdevice_extern_elementwise({
|
|
(float32,): ("__nv_floorf", float32),
|
|
(float64,): ("__nv_floor", float64),
|
|
})
|
|
ceil = libdevice_extern_elementwise({
|
|
(float32,): ("__nv_ceilf", float32),
|
|
(float64,): ("__nv_ceil", float64),
|
|
})
|
|
abs = libdevice_extern_elementwise({
|
|
(int32,): ("__nv_abs", int32),
|
|
(int64,): ("__nv_llabs", int64),
|
|
(float32,): ("__nv_fabsf", float32),
|
|
(float64,): ("__nv_fabs", float64),
|
|
})
|
|
max = partial(
|
|
wrap_with_builder(tl.math.max),
|
|
propagate_nan=tl.PropagateNan.NONE,
|
|
)
|
|
min = partial(
|
|
wrap_with_builder(tl.math.min),
|
|
propagate_nan=tl.PropagateNan.NONE,
|
|
)
|
|
nextafter = wrap_with_builder(tl.math.nextafter)
|
|
popc = libdevice_extern_elementwise({
|
|
(int32,): ("__nv_popc", int32),
|
|
(int64,): ("__nv_popcll", int64),
|
|
})
|
|
pow = wrap_with_builder(tl.math.pow)
|
|
sqrt = libdevice_extern_elementwise({
|
|
(float32,): ("__nv_sqrtf", float32),
|
|
(float64,): ("__nv_sqrt", float64),
|
|
})
|
|
rsqrt = libdevice_extern_elementwise({
|
|
(float32,): ("__nv_rsqrtf", float32),
|
|
(float64,): ("__nv_rsqrt", float64),
|
|
})
|
|
|
|
|
|
class semantic:
|
|
add = wrap_with_builder(tl.semantic.add)
|
|
and_ = wrap_with_builder(tl.semantic.and_)
|
|
ashr = wrap_with_builder(tl.semantic.ashr)
|
|
cast = wrap_with_builder(tl.semantic.cast)
|
|
equal = wrap_with_builder(tl.semantic.equal)
|
|
floordiv = wrap_with_builder(tl.semantic.floordiv)
|
|
greater_equal = wrap_with_builder(tl.semantic.greater_equal)
|
|
greater_than = wrap_with_builder(tl.semantic.greater_than)
|
|
invert = wrap_with_builder(tl.semantic.invert)
|
|
less_equal = wrap_with_builder(tl.semantic.less_equal)
|
|
less_than = wrap_with_builder(tl.semantic.less_than)
|
|
lshr = wrap_with_builder(tl.semantic.lshr)
|
|
minus = wrap_with_builder(tl.semantic.minus)
|
|
mod = wrap_with_builder(tl.semantic.mod)
|
|
mul = wrap_with_builder(tl.semantic.mul)
|
|
not_equal = wrap_with_builder(tl.semantic.not_equal)
|
|
or_ = wrap_with_builder(tl.semantic.or_)
|
|
shl = wrap_with_builder(tl.semantic.shl)
|
|
sub = wrap_with_builder(tl.semantic.sub)
|
|
trans = wrap_with_builder(tl.semantic.trans)
|
|
truediv = wrap_with_builder(tl.semantic.truediv)
|
|
where = wrap_with_builder(tl.semantic.where)
|
|
xor_ = wrap_with_builder(tl.semantic.xor_)
|