mirror of
https://github.com/ROCm/jax.git
synced 2025-04-18 12:56:07 +00:00
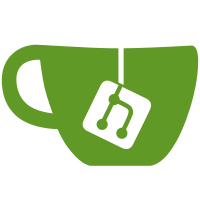
It turns out that the backend is rarely needed when lowering, e.g., for lowering callbacks. Whenever we need the backend for lowering, we must be in single-platform lowering mode (`len(platforms) == 1`) and we can look up the backend from `platforms[0]`. However, in some rare cases we can have a custom `XlaBackend` whose platform matches `platforms[0]`. We rename `backend_or_name` to just `backend` and we restrict its type to be an optional `XlaBackend` (not a platform string). PiperOrigin-RevId: 712926140
564 lines
22 KiB
Python
564 lines
22 KiB
Python
# Copyright 2018 The JAX Authors.
|
|
#
|
|
# Licensed under the Apache License, Version 2.0 (the "License");
|
|
# you may not use this file except in compliance with the License.
|
|
# You may obtain a copy of the License at
|
|
#
|
|
# https://www.apache.org/licenses/LICENSE-2.0
|
|
#
|
|
# Unless required by applicable law or agreed to in writing, software
|
|
# distributed under the License is distributed on an "AS IS" BASIS,
|
|
# WITHOUT WARRANTIES OR CONDITIONS OF ANY KIND, either express or implied.
|
|
# See the License for the specific language governing permissions and
|
|
# limitations under the License.
|
|
|
|
"""The implementation of custom partitioning APIs.
|
|
|
|
It was moved out of ``jax.experimental`` to avoid import cycles.
|
|
"""
|
|
|
|
from __future__ import annotations
|
|
|
|
from functools import partial
|
|
import inspect
|
|
from typing import Any
|
|
import weakref
|
|
|
|
import numpy as np
|
|
import jax
|
|
from jax import tree_util
|
|
from jax._src import api_util
|
|
from jax._src import core
|
|
from jax._src import custom_api_util
|
|
from jax._src import dispatch
|
|
from jax._src import linear_util as lu
|
|
from jax._src import mesh as mesh_lib
|
|
from jax._src import sharding_impls
|
|
from jax._src import xla_bridge as xb
|
|
from jax._src.interpreters import mlir
|
|
from jax._src.interpreters import partial_eval as pe
|
|
from jax._src.lib import xla_client as xc
|
|
from jax._src.lib.mlir import ir
|
|
from jax._src.lib.mlir.dialects import hlo
|
|
from jax.errors import UnexpectedTracerError
|
|
|
|
|
|
def _resolve_kwargs(fun, args, kwargs):
|
|
ba = inspect.signature(fun).bind(*args, **kwargs)
|
|
ba.apply_defaults()
|
|
if ba.kwargs:
|
|
raise TypeError("keyword arguments could not be resolved to positions")
|
|
else:
|
|
return ba.args
|
|
|
|
|
|
class _ShardingCallbackInfo:
|
|
|
|
def __init__(self, propagate_user_sharding, partition, to_mesh_pspec_sharding,
|
|
in_tree, out_tree, infer_sharding_from_operands, module_context, mesh,
|
|
static_args):
|
|
self.propagate_user_sharding = propagate_user_sharding
|
|
self.partition = partition
|
|
self.to_mesh_pspec_sharding = to_mesh_pspec_sharding
|
|
self.in_tree = in_tree
|
|
self.out_tree = out_tree
|
|
self.infer_sharding_from_operands = infer_sharding_from_operands
|
|
self.module_context = module_context
|
|
self.mesh = mesh
|
|
self.static_args = static_args
|
|
|
|
def unflatten_arg_shape(self, s, sharding):
|
|
return _to_jax_sharded_shape(
|
|
s, self.to_mesh_pspec_sharding(sharding, len(s.dimensions()))
|
|
)
|
|
|
|
def unflatten_arg_shapes(self, arg_shapes, arg_shardings):
|
|
return self.in_tree.unflatten(
|
|
[
|
|
self.unflatten_arg_shape(s, sharding)
|
|
for s, sharding in zip(arg_shapes, arg_shardings)
|
|
]
|
|
)
|
|
|
|
|
|
_sharding_callbacks = weakref.WeakValueDictionary() # type: ignore
|
|
|
|
_CUSTOM_PARTITIONING_CALL_NAME = "CustomSPMDPartitioning"
|
|
|
|
|
|
def _to_jax_shape(s):
|
|
return core.ShapedArray(s.dimensions(), s.numpy_dtype())
|
|
|
|
|
|
def _to_jax_sharded_shape(s, sharding):
|
|
return jax.ShapeDtypeStruct(
|
|
s.dimensions(), s.numpy_dtype(), sharding=sharding
|
|
)
|
|
|
|
|
|
def _pack_result_sharding(shape, result_shardings):
|
|
if shape.is_tuple():
|
|
return xc.HloSharding.tuple_sharding(shape, result_shardings)
|
|
else:
|
|
return result_shardings[0]
|
|
|
|
|
|
def _flatten_sharding(tree, shardings, shapes):
|
|
return [
|
|
_to_hlo_sharding(sharding, len(shape.dimensions()))
|
|
for sharding, shape in zip(
|
|
tree.flatten_up_to(shardings), shapes
|
|
)
|
|
]
|
|
|
|
|
|
def _custom_partitioning_propagate_user_sharding(user_sharding, shape,
|
|
backend_string):
|
|
info = _sharding_callbacks[backend_string]
|
|
if info.propagate_user_sharding is None:
|
|
return user_sharding
|
|
if shape.is_tuple():
|
|
user_shapes = shape.tuple_shapes()
|
|
user_shardings = user_sharding.tuple_elements()
|
|
else:
|
|
user_shapes = (shape,)
|
|
user_shardings = (user_sharding,)
|
|
user_shape = info.out_tree.unflatten(
|
|
[
|
|
info.unflatten_arg_shape(s, sharding)
|
|
for s, sharding in zip(user_shapes, user_shardings)
|
|
]
|
|
)
|
|
result_sharding = info.propagate_user_sharding(
|
|
*info.static_args, info.mesh, user_shape
|
|
)
|
|
result_shardings = _flatten_sharding(
|
|
info.out_tree, result_sharding, user_shapes)
|
|
return _pack_result_sharding(shape, result_shardings)
|
|
|
|
|
|
def _to_hlo_sharding(sharding, num_dimensions):
|
|
if not isinstance(sharding, jax.sharding.Sharding):
|
|
raise ValueError("Custom Partitioning rules must return Sharding.")
|
|
return sharding._to_xla_hlo_sharding(num_dimensions)
|
|
|
|
|
|
def _custom_partitioning_partition(arg_shapes, arg_shardings, result_shape,
|
|
result_sharding, backend_string):
|
|
info = _sharding_callbacks[backend_string]
|
|
if result_shape.is_tuple():
|
|
result_shapes = result_shape.tuple_shapes()
|
|
result_shardings = result_sharding.tuple_elements()
|
|
else:
|
|
result_shapes = (result_shape,)
|
|
result_shardings = (result_sharding,)
|
|
mesh, lower_fn, result_sharding, arg_shardings = info.partition(
|
|
*info.static_args,
|
|
info.mesh,
|
|
info.unflatten_arg_shapes(arg_shapes, arg_shardings),
|
|
info.out_tree.unflatten(
|
|
[
|
|
info.unflatten_arg_shape(s, sharding)
|
|
for s, sharding in zip(result_shapes, result_shardings)
|
|
]
|
|
),
|
|
)
|
|
module_context = info.module_context
|
|
|
|
result_shardings = _flatten_sharding(
|
|
info.out_tree, result_sharding, result_shapes)
|
|
arg_shardings = _flatten_sharding(info.in_tree, arg_shardings, arg_shapes)
|
|
tiled_args = [
|
|
_to_jax_shape(sharding.tile(s))
|
|
for sharding, s in zip(arg_shardings, arg_shapes)
|
|
]
|
|
tiled_results = [
|
|
_to_jax_shape(sharding.tile(s))
|
|
for sharding, s in zip(result_shardings, result_shapes)
|
|
]
|
|
closed_jaxpr = jax.make_jaxpr(lower_fn, axis_env=list(mesh.shape.items()))(
|
|
*tiled_args
|
|
)
|
|
if closed_jaxpr.out_avals != tiled_results:
|
|
raise ValueError(
|
|
"Mismatch in result shapes. %s vs %s"
|
|
% (repr(closed_jaxpr.out_avals), repr(tiled_results))
|
|
)
|
|
axis_context = sharding_impls.SPMDAxisContext(mesh)
|
|
with core.extend_axis_env_nd(mesh.shape.items()):
|
|
module = mlir.build_mlir_module_helper(
|
|
closed_jaxpr,
|
|
name="tmp_xla_computation",
|
|
platforms=module_context.platforms,
|
|
backend=module_context.backend,
|
|
axis_context=axis_context.extend_manual(frozenset(mesh.axis_names)),
|
|
)
|
|
result_sharding = _pack_result_sharding(result_shape, result_shardings)
|
|
return mlir.module_to_bytecode(module), arg_shardings, result_sharding
|
|
|
|
|
|
def _custom_partitioning_infer_sharding_from_operands(arg_shapes, arg_shardings,
|
|
result_shape,
|
|
backend_string):
|
|
info = _sharding_callbacks[backend_string]
|
|
if result_shape.is_tuple():
|
|
result_shapes = result_shape.tuple_shapes()
|
|
else:
|
|
result_shapes = (result_shape,)
|
|
result_sharding = info.infer_sharding_from_operands(
|
|
*info.static_args,
|
|
info.mesh,
|
|
info.unflatten_arg_shapes(arg_shapes, arg_shardings),
|
|
info.out_tree.unflatten([_to_jax_shape(s) for s in result_shapes]),
|
|
)
|
|
result_shardings = _flatten_sharding(
|
|
info.out_tree, result_sharding, result_shapes)
|
|
return _pack_result_sharding(result_shape, result_shardings)
|
|
|
|
|
|
custom_partitioning_p = core.Primitive("custom_partitioning")
|
|
custom_partitioning_p.multiple_results = True
|
|
dispatch.prim_requires_devices_during_lowering.add(custom_partitioning_p)
|
|
|
|
|
|
def _custom_partitioning_abstract_eval(*avals, call, in_tree, out_tree,
|
|
propagate_user_sharding, partition,
|
|
infer_sharding_from_operands,
|
|
decode_shardings,
|
|
static_args):
|
|
del in_tree, out_tree, propagate_user_sharding, partition
|
|
del infer_sharding_from_operands, decode_shardings, static_args
|
|
return call.out_avals
|
|
|
|
|
|
def _custom_partitioning_impl(*args, call, in_tree, out_tree,
|
|
propagate_user_sharding,
|
|
partition, infer_sharding_from_operands,
|
|
decode_shardings, static_args):
|
|
del in_tree, out_tree, propagate_user_sharding, partition
|
|
del infer_sharding_from_operands, decode_shardings, static_args
|
|
return core.jaxpr_as_fun(call)(*args)
|
|
|
|
|
|
custom_partitioning_p.def_abstract_eval(_custom_partitioning_abstract_eval)
|
|
custom_partitioning_p.def_impl(_custom_partitioning_impl)
|
|
|
|
|
|
def _check_for_tracers(x):
|
|
if any(isinstance(leaf, core.Tracer) for leaf in tree_util.tree_leaves(x)):
|
|
raise UnexpectedTracerError(
|
|
"Found a JAX Tracer object passed as an argument to a"
|
|
"custom_partitioning function in a position indicated as static by"
|
|
"static_argnums. "
|
|
)
|
|
|
|
|
|
@custom_api_util.register_custom_decorator_type
|
|
class custom_partitioning:
|
|
"""Inserts a CustomCallOp into the XLA graph with custom SPMD lowering rules.
|
|
|
|
.. code-block:: python
|
|
|
|
@custom_partitioning
|
|
def f(*args):
|
|
return ...
|
|
|
|
def propagate_user_sharding(mesh, user_shape):
|
|
'''Update the sharding of the op from a user's shape.sharding.'''
|
|
user_sharding = jax.tree.map(lambda x: x.sharding, user_shape)
|
|
|
|
def partition(mesh, arg_shapes, result_shape):
|
|
def lower_fn(*args):
|
|
... builds computation on per-device shapes ...
|
|
result_shardings = jax.tree.map(lambda x: x.sharding, result_shape)
|
|
arg_shardings = jax.tree.map(lambda x: x.sharding, arg_shapes)
|
|
# result_sharding and arg_shardings may optionally be modified and the
|
|
# partitioner will insert collectives to reshape.
|
|
return mesh, lower_fn, result_sharding, arg_shardings
|
|
|
|
def infer_sharding_from_operands(mesh, arg_shapes, shape):
|
|
'''Compute the result sharding from the sharding of the operands.'''
|
|
arg_shardings = jax.tree.map(lambda x: x.sharding, arg_shapes)
|
|
|
|
|
|
f.def_partition(partition, propagate_user_sharding, infer_sharding_from_operands)
|
|
|
|
The args to ``def_partition`` are as follows:
|
|
|
|
* ``propagate_user_sharding``: Callable which takes the sharding of a user (in the dag)
|
|
and returns a suggestion for a new `NamedSharding`. The default
|
|
implementation is just to return the suggested sharding.
|
|
* ``partition``: Callable which takes the SPMD suggested partition shapes and
|
|
partition specs and returns the mesh, a per-shard lowering function, and the final
|
|
input and output sharding specs (the SPMD partitioner will repartition the
|
|
inputs to match). The mesh is returned to allow configuring axis_names for
|
|
collectives when no mesh is provided.
|
|
* ``infer_sharding_from_operands``: Callable which computes an output ``NamedSharding``
|
|
from the ``NamedSharding`` chosen for each argument.
|
|
* ``decode_shardings``: When set to True, convert input ``GSPMDSharding``s to
|
|
``NamedSharding`` if possible. This may not be possible if the user does not
|
|
provide a contextual mesh.
|
|
|
|
Positional arguments can be specified as static using static_argnums. JAX uses
|
|
:code:`inspect.signature(fun)` to resolve these positional arguments.
|
|
|
|
Examples:
|
|
|
|
As an example, assume we want to enhance the existing ``jax.numpy.fft.fft``. This function computes
|
|
the discrete Fourier transform of an N-dimensional input along the last dimension, and is batched
|
|
along the first N-1 dimensions.
|
|
By default, however, it will ignore the sharding of the input and gather the input on all devices.
|
|
However, since ``jax.numpy.fft.fft`` is batched along the first N-1 dimensions,
|
|
this is unnecessary. We will create a new ``my_fft`` op that, instead, does not alter the sharding
|
|
along the first `N-1` dimensions, and only gathers the input along the last dimension if needed.
|
|
|
|
.. code-block:: python
|
|
|
|
import jax
|
|
from jax.sharding import NamedSharding
|
|
from jax.experimental.custom_partitioning import custom_partitioning
|
|
from jax.experimental.pjit import pjit
|
|
from jax.sharding import PartitionSpec as P
|
|
from jax.sharding import Mesh
|
|
from jax.numpy.fft import fft
|
|
import regex as re
|
|
import numpy as np
|
|
|
|
# Pattern to detect all-gather or dynamic-slice in the generated HLO
|
|
_PATTERN = '(dynamic-slice|all-gather)'
|
|
|
|
# For an N-D input, keeps sharding along the first N-1 dimensions
|
|
# but replicate along the last dimension
|
|
def supported_sharding(sharding, shape):
|
|
rank = len(shape.shape)
|
|
max_shared_dims = min(len(sharding.spec), rank-1)
|
|
names = tuple(sharding.spec[:max_shared_dims]) + tuple(None for _ in range(rank - max_shared_dims))
|
|
return NamedSharding(sharding.mesh, P(*names))
|
|
|
|
def partition(mesh, arg_shapes, result_shape):
|
|
result_shardings = jax.tree.map(lambda x: x.sharding, result_shape)
|
|
arg_shardings = jax.tree.map(lambda x: x.sharding, arg_shapes)
|
|
return mesh, fft, \
|
|
supported_sharding(arg_shardings[0], arg_shapes[0]), \
|
|
(supported_sharding(arg_shardings[0], arg_shapes[0]),)
|
|
|
|
def infer_sharding_from_operands(mesh, arg_shapes, result_shape):
|
|
arg_shardings = jax.tree.map(lambda x: x.sharding, arg_shapes)
|
|
return supported_sharding(arg_shardings[0], arg_shapes[0])
|
|
|
|
@custom_partitioning
|
|
def my_fft(x):
|
|
return fft(x)
|
|
|
|
my_fft.def_partition(
|
|
infer_sharding_from_operands=infer_sharding_from_operands,
|
|
partition=partition)
|
|
|
|
Now create a 2D array sharded along the first axis, pass it through ``my_fft``
|
|
and notice how it is still sharded as expected, and identical to the output
|
|
of ``fft``. However, inspecting the HLO
|
|
(using ``lower(x).compile().runtime_executable().hlo_modules()``) reveals that
|
|
``my_fft`` does not create any all-gather or dynamic-slice, while ``fft`` does.
|
|
|
|
.. code-block::
|
|
|
|
with Mesh(np.array(jax.devices()), ('x',)):
|
|
x = np.asarray(np.random.randn(32*1024, 1024), dtype=np.complex64)
|
|
y = pjit(lambda x: x, in_shardings=None, out_shardings=P('x'))(x)
|
|
pjit_my_fft = pjit(my_fft, in_shardings=P('x'), out_shardings=P('x'))
|
|
pjit_fft = pjit(fft, in_shardings=P('x'), out_shardings=P('x'))
|
|
print(pjit_my_fft(y))
|
|
print(pjit_fft(y))
|
|
# dynamic-slice or all-gather are not present in the HLO for my_fft, because x is a 2D array
|
|
assert(re.search(_PATTERN, pjit_my_fft.lower(x).compile().runtime_executable().hlo_modules()[0].to_string()) is None)
|
|
# dynamic-slice or all-gather are present in the HLO for fft
|
|
assert(re.search(_PATTERN, pjit_fft.lower(x).compile().runtime_executable().hlo_modules()[0].to_string()) is not None)
|
|
|
|
.. code-block::
|
|
|
|
# my_fft
|
|
[[-38.840824 +0.j -40.649452 +11.845365j
|
|
...
|
|
-1.6937828 +0.8402481j 15.999859 -4.0156755j]]
|
|
|
|
# jax.numpy.fft.fft
|
|
[[-38.840824 +0.j -40.649452 +11.845365j
|
|
...
|
|
-1.6937828 +0.8402481j 15.999859 -4.0156755j]]
|
|
|
|
Because of the logic in ``supported_sharding``, ``my_fft`` also works on 1-dimensional arrays.
|
|
However, in this case, the HLO of ``my_fft`` does show a dynamic-slice, since the last dimension
|
|
is the dimension along which FFTs are calculated and needs to be replicated on all devices before
|
|
the computation can be done.
|
|
|
|
.. code-block::
|
|
|
|
with Mesh(np.array(jax.devices()), ('x',)):
|
|
x = np.asarray(np.random.randn(32*1024*1024), dtype=np.complex64)
|
|
y = pjit(lambda x: x, in_shardings=None, out_shardings=P('x'))(x)
|
|
pjit_my_fft = pjit(my_fft, in_shardings=P('x'), out_shardings=P('x'))
|
|
pjit_fft = pjit(fft, in_shardings=P('x'), out_shardings=P('x'))
|
|
print(pjit_my_fft(y))
|
|
print(pjit_fft(y))
|
|
# dynamic-slice or all-gather are present in the HLO for my_fft, because x is a 1D array
|
|
assert(re.search(_PATTERN, pjit_my_fft.lower(x).compile().runtime_executable().hlo_modules()[0].to_string()) is None)
|
|
# dynamic-slice or all-gather are present in the HLO for fft
|
|
assert(re.search(_PATTERN, pjit_fft.lower(x).compile().runtime_executable().hlo_modules()[0].to_string()) is not None)
|
|
|
|
.. code-block::
|
|
|
|
# my_fft
|
|
[ 7.217285 +0.j -3012.4937 +4287.635j -405.83594 +3042.984j
|
|
... 1422.4502 +7271.4297j -405.84033 -3042.983j
|
|
-3012.4963 -4287.6343j]
|
|
|
|
# jax.numpy.fft.fft
|
|
[ 7.217285 +0.j -3012.4937 +4287.635j -405.83594 +3042.984j
|
|
... 1422.4502 +7271.4297j -405.84033 -3042.983j
|
|
-3012.4963 -4287.6343j]
|
|
|
|
"""
|
|
|
|
def __init__(self, fun, static_argnums=()):
|
|
self.fun = fun
|
|
self.partition = None
|
|
self.static_argnums = static_argnums
|
|
self.propagate_user_sharding = None
|
|
self.infer_sharding_from_operands = None
|
|
|
|
__getattr__: Any = custom_api_util.forward_attr
|
|
|
|
def def_partition(self, partition, infer_sharding_from_operands,
|
|
propagate_user_sharding=None, decode_shardings=True):
|
|
self.partition = partition
|
|
self.propagate_user_sharding = propagate_user_sharding
|
|
self.infer_sharding_from_operands = infer_sharding_from_operands
|
|
self.decode_shardings = decode_shardings
|
|
return partition
|
|
|
|
def __call__(self, *args, **kwargs):
|
|
args = _resolve_kwargs(self.fun, args, kwargs)
|
|
if self.static_argnums:
|
|
static_argnums = set(self.static_argnums)
|
|
args = tuple(x if i in static_argnums else x for i, x in enumerate(args))
|
|
dyn_argnums = [i for i in range(len(args)) if i not in static_argnums]
|
|
f_, dyn_args = api_util.argnums_partial(
|
|
lu.wrap_init(self.fun),
|
|
dyn_argnums,
|
|
args,
|
|
require_static_args_hashable=False,
|
|
)
|
|
static_args = [args[i] for i in self.static_argnums]
|
|
_check_for_tracers(static_args)
|
|
else:
|
|
static_args = []
|
|
f_, dyn_args = lu.wrap_init(self.fun), args
|
|
args_flat, in_tree = tree_util.tree_flatten(dyn_args)
|
|
flat_fun, out_tree = api_util.flatten_fun_nokwargs(f_, in_tree)
|
|
in_avals = [core.get_aval(x) for x in args_flat]
|
|
debug = pe.debug_info(self.fun, in_tree, out_tree, False,
|
|
"custom_partitioning")
|
|
mesh = mesh_lib.thread_resources.env.physical_mesh
|
|
with core.extend_axis_env_nd(mesh.shape.items()):
|
|
jaxpr, _, consts, () = pe.trace_to_jaxpr_dynamic(flat_fun, in_avals, debug)
|
|
assert not len(consts)
|
|
closed_call = core.ClosedJaxpr(pe.convert_constvars_jaxpr(jaxpr), ())
|
|
out_flat = custom_partitioning_p.bind(
|
|
*consts,
|
|
*args_flat,
|
|
call=closed_call,
|
|
partition=self.partition,
|
|
propagate_user_sharding=self.propagate_user_sharding,
|
|
infer_sharding_from_operands=self.infer_sharding_from_operands,
|
|
decode_shardings=self.decode_shardings,
|
|
in_tree=in_tree,
|
|
out_tree=out_tree(),
|
|
static_args=static_args
|
|
)
|
|
return tree_util.tree_unflatten(out_tree(), out_flat)
|
|
|
|
|
|
def _custom_partitioning_lowering_rule(ctx: mlir.LoweringRuleContext, *values,
|
|
call, in_tree, out_tree,
|
|
propagate_user_sharding, partition,
|
|
infer_sharding_from_operands,
|
|
decode_shardings,
|
|
static_args):
|
|
axis_context = ctx.module_context.axis_context
|
|
if (isinstance(axis_context, sharding_impls.SPMDAxisContext) and
|
|
set(axis_context.manual_axes) == set(axis_context.mesh.axis_names)):
|
|
return mlir.lower_fun(core.jaxpr_as_fun(call), multiple_results=True)(ctx, *values)
|
|
|
|
mesh = mesh_lib.thread_resources.env.physical_mesh
|
|
if isinstance(axis_context, sharding_impls.ShardingContext):
|
|
devices = axis_context.device_assignment
|
|
if devices is None:
|
|
raise AssertionError(
|
|
'Please file a bug at https://github.com/jax-ml/jax/issues')
|
|
am = axis_context.abstract_mesh
|
|
if am is not None:
|
|
mesh = mesh_lib.Mesh(np.array(devices).reshape(am.axis_sizes),
|
|
am.axis_names)
|
|
elif isinstance(axis_context, sharding_impls.SPMDAxisContext):
|
|
devices = axis_context.mesh._flat_devices_tuple
|
|
else:
|
|
devices = None
|
|
|
|
if not devices or len(devices) == 1:
|
|
return mlir.lower_fun(
|
|
core.jaxpr_as_fun(call), multiple_results=True)(ctx, *values)
|
|
|
|
def to_mesh_pspec_sharding(hlo_sharding: xc.HloSharding | None, ndim):
|
|
if hlo_sharding is None:
|
|
return hlo_sharding
|
|
if mesh.empty or not decode_shardings:
|
|
assert devices is not None
|
|
return sharding_impls._op_sharding_to_pos_sharding(hlo_sharding, devices)
|
|
pspec = sharding_impls.parse_flatten_op_sharding(
|
|
hlo_sharding, mesh)[0].get_partition_spec()
|
|
pspec = jax.sharding.PartitionSpec(*pspec, *((None,) * (ndim - len(pspec))))
|
|
return jax.sharding.NamedSharding(mesh, pspec)
|
|
|
|
sharding_callback_info = _ShardingCallbackInfo(propagate_user_sharding,
|
|
partition, to_mesh_pspec_sharding, in_tree, out_tree,
|
|
infer_sharding_from_operands, ctx.module_context, mesh, static_args)
|
|
key = str(id(sharding_callback_info))
|
|
_sharding_callbacks[bytes(key, 'utf8')] = sharding_callback_info
|
|
# We need to make sure `sharding_callback_info` is still alive when the SPMD
|
|
# partitioner runs so we keep it alive by attaching it to the executable.
|
|
ctx.module_context.add_keepalive(sharding_callback_info)
|
|
|
|
result_types = [mlir.aval_to_ir_type(s) for s in call.out_avals]
|
|
out = hlo.CustomCallOp(
|
|
result_types,
|
|
list(values),
|
|
call_target_name=ir.StringAttr.get(_CUSTOM_PARTITIONING_CALL_NAME),
|
|
has_side_effect=ir.BoolAttr.get(False),
|
|
api_version=mlir.i32_attr(2),
|
|
called_computations=ir.ArrayAttr.get([]),
|
|
backend_config=ir.StringAttr.get(key),
|
|
operand_layouts=None,
|
|
result_layouts=None)
|
|
return out.results
|
|
|
|
mlir.register_lowering(custom_partitioning_p,
|
|
_custom_partitioning_lowering_rule)
|
|
|
|
xc.register_custom_call_partitioner(
|
|
_CUSTOM_PARTITIONING_CALL_NAME,
|
|
_custom_partitioning_propagate_user_sharding,
|
|
_custom_partitioning_partition,
|
|
_custom_partitioning_infer_sharding_from_operands,
|
|
can_side_effecting_have_replicated_sharding=True,
|
|
)
|
|
xb.register_plugin_callbacks(
|
|
partial(
|
|
xc.register_custom_call_partitioner,
|
|
name=_CUSTOM_PARTITIONING_CALL_NAME,
|
|
prop_user_sharding=_custom_partitioning_propagate_user_sharding,
|
|
partition=_custom_partitioning_partition,
|
|
infer_sharding_from_operands=_custom_partitioning_infer_sharding_from_operands,
|
|
can_side_effecting_have_replicated_sharding=True,
|
|
)
|
|
)
|